- 1School of Educational Technology, Beijing Normal University, Beijing, China
- 2Department of Industrial Education, Institute for Research Excellence in Learning Science, National Taiwan Normal University, Taipei, Taiwan
The outbreak of the COVID-19 epidemic has promoted the popularity of online learning, but has also exposed some problems, such as a lack of interaction, resulting in loneliness. Against this background, students' attitudes toward peer interaction may have become even more important. In order to explore the impact of attitude toward peer interaction on students' mindset including online learning motivation and critical thinking practice that could affect their problem-solving self-efficacy during the COVID-19 pandemic, we developed and administered a questionnaire, receiving 1,596 valid responses. The reliability and validity of the questionnaire were re-tested, and structural equation modeling was applied. It was found that attitude toward peer interaction could positively predict middle school students' online learning motivation and critical thinking. Learning motivation and critical thinking also positively supported problem-solving self-efficacy. It is expected that the results of this study can be a reference for teachers to adopt student-centered online learning in problem solving courses.
Introduction
The outbreak of COVID-19 has led to the widespread practice of online learning in schools (Zhao et al., 2021). Teachers and students in middle schools continue to integrate online learning into classrooms, which is further promoting the process of online and offline blended learning development (Lee et al., 2021). A conceptual ecology of learning is necessary to embrace a series of learning environment “across boundaries traditionally separating institutions of education, popular culture, home, and community” (Kumpulainen and Mikkola, 2014, p. 51). However, the coevolution of people and their environments is an ongoing process (Hilty and Aebischer, 2015). That is, online learning is intertwined with sociocultural environments (Allen et al., 2015), and when people are not able to meet physically during the COVID-19 pandemic, they need more social interactions (Kalmar et al., 2022). Peer interaction has been proved to benefit learning progression and contribute to deep learning (Chadha, 2019). Online peer interaction embedded in learning design is useful for promoting students' learning outcomes, but previous research has mainly focused on the higher education group (Lin et al., 2017; Martin et al., 2020). While online learning during the COVID-19 pandemic could have been a new experience for middle school students to interact with peers and their teachers (Clark et al., 2021), attention to K-12 education in the literature is rare, and online learning had hardly been adopted by Chinese middle schools before the COVID-19 pandemic. As the pandemic came suddenly, most teachers in Chinese K-12 schools only conducted online one-way live-streamed lectures, and did not pay attention to interactive activities, leading to surface learning and polarization of students (Yu and Wang, 2020). Thus, the present study aimed to explore the role of peer interaction in online learning based on Chinese K-12 students.
In fact, the pandemic promoted the universalization and ubiquity of online learning; thus, there is a need for more and deeper attention to online learning outcomes (Ngo and Ngadiman, 2021). Learning outcomes consist of affective outcomes, cognitive outcomes, and skill-based outcomes (Kraiger et al., 1993), of which motivation (as an affective outcome), critical thinking (as a cognitive outcome) and problem solving (as a skill-based outcome) have been the factors of most concern in the online learning research (Zhou et al., 2021). These learning outcomes are important for not only higher education students, but also K-12 students. To explore the role of peer interaction in online learning for K-12 students during the pandemic, it is necessary to study the relationship among peer interaction, motivation, critical thinking, and problem solving.
Attitude is defined as a favorable or unfavorable evaluative reaction toward something or someone, exhibited in one's beliefs, motivation, or intended behavior (Ajzen, 2005). Attitudes provide meaningful approaches to seek some degree of order, clarity, and stability in our personal motivation of reference (Harmon-Jones and Harmon-Jones, 2021). As the measurement of the real interactive behavior is difficult, students' attitude toward peer interaction is used to explore the role of peer interaction in online learning. Attitudes include affective and cognitive components to predict motivation and behavior (Ajzen, 2005). In contrast, mindsets consist of a collection of attitude judgments and cognitive processes and procedures to facilitate problem solving and completion of a particular task (Gollwitzer et al., 1990). Moreover, mindsets drive cognitive processing, and capture the critical thinking that is an important behavioral outcome judgement (Nolder and Kadous, 2018). Students' attitude toward peer interaction could have an impact on their mindsets (Zulkifli et al., 2020; Thanasi-Boce, 2021), leading to different learning performance (Kwon et al., 2019). To date, few researchers have investigated online learner profiles based on the mindset shared among students (Zamecnik et al., 2022). Online learning profiles, problem solving, critical thinking, teamwork, and motivation are different in different areas and backgrounds (Lawter and Garnjost, 2021). In particular, the profiles of middle school students who were born and grew up in the digital age, and so are known as “digital natives” (Becker and Birdi, 2018) have not been studied. In line with this, the correlates between middle school students' attitude toward peer interaction, motivation, critical thinking, and problem solving were explored in this study.
Theoretical background
Attitude toward peer interaction in online learning
Peer interaction is usually considered to be at the heart of the development of constructivist learning theory research (Tenenbaum et al., 2020). In peer interaction, students can question others as free and active participants in social discourse, argument, and learning (Castellaro and Roselli, 2015). The comparison of perspective will produce social cognitive conflict, and then generate consensus through interaction (Tenenbaum et al., 2020). It has been shown that peer interaction can benefit learning progression and make students learn more deeply (Chadha, 2019). Learner-learner interaction refers to the two-way communication among learners, such as exchanging ideas with classmates, discussing with each other, and getting feedback from other learners (Wang et al., 2022). Moreover, middle school students had hardly ever experienced complete online learning before the COVID-19 pandemic. When the pandemic suddenly broke out, some students could have had difficulty adapting to online learning, leading to learning anxiety, loneliness, and even depression (An et al., 2020; Perkins et al., 2021; Ying et al., 2021). Peer interaction might be the key to solving these affective and psychological problems (Yao and Zheng, 2017; An et al., 2020). Especially, social and peer influence is of great importance for adolescents (Tsai et al., 2015), so peer interaction could be an extremely important factor for middle school students' online learning. In brief, peer interaction is beneficial for students' development of knowledge and ability (Chadha, 2019), and could also help improve students' attitudes toward online learning during the COVID-19 pandemic (Ala et al., 2021; Chu et al., 2021; Ngo and Ngadiman, 2021). However, it is difficult to measure the real peer interactive behaviors of students, as the peer interactions occur naturally in social media, but are not limited to a specific learning platform. Students' attitude could show their preference for a certain behavior (Ajzen, 2005). Thus, students' attitude toward peer interaction in middle high school was explored in this study.
Motivation as emotional mindset
The concept of mindsets is based on Dweck (1999) framework in which it was proposed that mindsets determine one's goals, motivation, and beliefs about effort. As a result, mindsets can organize associated constructs into a coherent motivational framework or “meaning system” (Yu and McLellan, 2020). An important motivational factor that might influence individuals' willingness to engage with a task is their emotional mindset (Wols et al., 2020). Students' motivation plays a fundamental role in academic achievement. In fact, Yu and McLellan (2020) applied person-centered motivation to study some key elements of the mindset-based meaning system. Although there are different ways to induce students' growth mindset, most of the studies endorsed that the growth mindset can indeed modify the learning processes (Kania et al., 2017). Learning motivation is an important factor leading to success in online learning, especially for K-12 students (Zuo et al., 2021). The COVID-19 pandemic has brought about great changes to students' learning, such as the disruption of social networks and teachers being less focused on the individual. Students in middle school have lost their traditional learning motivation sources, but have attained new motivation sources (Uka and Uka, 2020). Learning motivation can improve academic outcomes by catering to learners' needs in online learning platforms (Baker et al., 2016). Although it has been studied in much of the online learning research (Zhou et al., 2021), little research has covered learning motivation in emotional mindset under the threat of COVID-19; thus, in this research, learning motivation was studied as an emotional mindset of online learning.
Critical thinking as cognitive mindset
Critical thinking is a kind of ability acquisition of online learning (Zhou et al., 2021), which usually refers to skills of reasoning, evaluation, analysis, judgment, conceptualization, understanding, and reflection (Guiller et al., 2008; van Laar et al., 2017). Individuals with a deliberative mindset are also more likely than those with an implemental cognitive mindset to take longer to reach a judgment (Henderson et al., 2008), indicating their openness to information and suspension of judgment. The deliberative mindset captures the mechanics behind a “questioning mind,” a “critical evaluation of evidence,” and the responsibility to “be alert” to evidence (Nolder and Kadous, 2018). In this research, critical thinking was studied as a mindset to question and to be alert for knowledge acquisition during online learning.
The Internet provides a good way for students to develop their critical thinking, as students could gain much information to help them think critically. Critical thinking is thought to be one of the most important outcomes of online learning, and previous researchers have tried to design online courses and tools to promote students' development of their critical thinking (Goodsett, 2020; Varenina et al., 2021). This study focused on self-reporting by middle school students to evaluate their cognitive mindset related to online learning.
Problem-solving self-efficacy
Problem solving is an important ability acquisition of online learning (Zhou et al., 2021), and refers to the skills of using information and communication technology to cognitively process and understand a problem situation in combination with the active use of knowledge to find a solution to a problem (van Laar et al., 2017). Problem solving involves different skills, such as finding the nature of the problem, choosing problem-solving steps and strategies, selecting appropriate information, allocating appropriate sources, and monitoring the problem-solving process (Sternberg, 1988). Problem solving is a key competency in online learning (Aslan, 2021), and the Internet provides convenient support for learners to solve problems (Jordan and McDaniel, 2014). For learners, problem solving is the key to learning success in a future-oriented society (OECD, 2017). As little research has considered how students' peer interaction supports their problem solving, the present study would explore the relationship between peer interaction and problem solving.
In studies using self-reported scales, self-efficacy of performance or ability has been used widely in problem solving (Calaguas and Consunji, 2022). Self-Efficacy refers to individuals' beliefs about their abilities to perform expected behaviors (Bandura, 1994). According to Bandura (1997), the problem-solving self-efficacy could be defined as the students' perceptions of their problem-solving success. In previous researches, problem-solving self-efficacy (PSSE) have been used widely to represented learners' perceptions of their problem-solving abilities (Bandura, 2006; Kyung-hee, 2016; Salazar and Hayward, 2018). In this research, student' PSSE would be measured to reflect the perception of problem-solving process.
Hypotheses
Previous studies found that online learning is useful for students, particularly in terms of learning outcomes during the COVID-19 pandemic (Jung and An, 2021; Choi-Sung, 2022). On the contrary, Hong et al. (2021a, 2022) found learning ineffectiveness through online learning, particularly in practical skill development (Hong et al., 2021b). Koehler et al. (2022) found that while students collaboratively interacted in the problem-solving process, individuals with a strong problem-solving presence valued peer interaction as an important part of the learning process, were willing to invest time engaging in the discussion, and maintained a consistent presence. Peer interaction could help to improve the motivation of students in online learning. For example, Yang and Chang (2012) found that peer interaction via blogs could improve students' learning motivation. Researchers found that for students in higher education, online peer interaction could improve their level of motivation during the COVID-19 pandemic (Thanasi-Boce, 2021; Kang and Zhang, 2022), but there has been a lack of focus on K-12. From this, we could speculate that for middle school students, the attitude toward online peer interaction during the epidemic could positively affect their online learning motivation. Hence, the following hypothesis was formulated in this study:
H1: The attitude toward online peer interaction could positively predict the online learning motivation of middle school students.
The mindset reflects the idea that individuals' cognitive processing determines both the content and strength of their resulting attitudes (Yu and McLellan, 2020). Critical thinking was thought to need a cognitive process, referring to the exchange and discussion of ideas with peers involved in the collaborative process of knowledge construction (Kuhn, 1991). Therefore, we could speculate that peer interaction could positively help to develop students' critical thinking. In fact, this has been proved for primary school students (Chou et al., 2015) and for undergraduate students (Oh et al., 2018; Zulkifli et al., 2020). What is more, previous researchers found that online peer discussion helped to develop university students' critical thinking better than face-to face discussion, as they would provide more well thought-out and reasonable evidence (Guiller et al., 2008). We could speculate that this might also be applicable to junior middle school students. Hence, the hypothesis formulated in this study was as follows:
H2: Attitude toward online peer interaction could positively predict the critical thinking of middle school students.
Students collaborate in groups to solve a problem, and analyze the formation of the problem to identify facts about the problem situation so that they can establish their representation of the problem and have a deeper understanding of the causes of problems. Then, they propose possible solutions, where they evaluate the gap between the current state and the desired state and address the solutions to the problem (Wu and Nian, 2021). These processes sometimes provide both an autonomy-controlling and an autonomy-supportive need to learn knowledge that may be maintained and enhanced by students' motivation (Wu et al., 2020). Moreover, individuals with a fixed emotion mindset believe that emotions are not changeable and cannot be controlled. Individuals with a growth emotion mindset believe that emotions are malleable and can be changed with effort and experience (Wols et al., 2020). For example, learning motivation is one of the major predictors of problem solving that is key in the nursing training field (Yardimci et al., 2017). To understand the correlates between middle school students' learning motivation and PSSE in online learning, the following hypothesis was proposed:
H3: Learning motivation could positively predict the PSSE of middle school students.
A problem is generally viewed as a discrepancy between desired goals and an existing state (Chi and Glaser, 1985), and problem solving is the process of taking actions to resolve this discrepancy (Shermerhorn, 2013). Researchers have suggested specific frameworks to capture effective problem solving; together, all frameworks provide insight into the critical reasoning processes learners engage in as they resolve ill-structured problems (Tawfik et al., 2020). While variation exists across focus and articulated problem-solving in online learning phases, these frameworks involve two main areas: problem finding with critical thinking (e.g., articulating a problem, constraints, clarifying diverse perspectives) and generating solutions with critical thinking (e.g., suggesting and evaluating solutions addressing identified problems) (Koehler et al., 2022). Researchers found that critical thinking had positive effects on problem solving (Kanbay and Okanli, 2017). Thus, the following hypothesis was proposed:
H4: Critical thinking could positively predict the PSSE of middle school students.
Peer interaction has an influence on problem solving because students rely on supportive social responses to enact most of their strategies to solve problems (Jordan and McDaniel, 2014). Students need to clarify or reorganize their views and plans when solving problems in peer groups, so it could be considered that the social interaction in groups is an important aspect of production power (Jordan and McDaniel, 2014). In online learning, peer interaction is also important for problem solving, but the outcome depends on the peers' competency levels and their motivation (Kwon et al., 2019). Cheng and Chau (2016) revealed that peer interaction in online learning was not at the desired level, and there was a need for studies to promote attitude toward peer interaction in online learning to enhance problem solving. However, peer interaction is one of the limitations of distance education which is widely used throughout the pandemic (Aslan, 2021). To understand how attitude toward peer interaction is related to online problem solving, in this research, the following hypothesis was proposed:
H5: For middle school students, attitude toward online peer interaction could positively predict PSSE mediated by learning motivation and critical thinking.
The current study adopted a person-centered approach to examine the ways in which mindsets and associated attitude toward peer interaction constructs cohered with emotional motivation and functioned together with critical thinking as a meaningful system related to PSSE. Specifically, drawing on attitude theory and mindset theory, this study addressed the research model as explained in Figure 1.
Method
Participants
From July 15 to July 21, 2020, questionnaires were distributed to students in eight middle schools for the prior study, and a total of 352 sample data were collected for the EFA of the scale. The prior study samples were mainly from Beijing, Liaoning, Shandong, and Henan provinces. Invalid samples were deleted according to polygraph items, and a total of 301 valid samples were retained of which 155 (51.5%) were from boys and 146 (48.5%) from girls. There were 90 (29.9%) seventh graders, 164 (54.5%) eighth graders, and 47 (15.6%) ninth graders.
From October 20 to November 1, 2020, questionnaires were randomly distributed to junior high school students in 34 provinces or districts of China. A total of 61,419 responses were received, of which 25,805 were retained after polygraph screening. We randomly selected a similar number of different types of people in the large sample to approximate stratified sampling. A total of 1,596 valid samples were randomly selected. The demographic information of the samples is shown in Table 1. There were 803 (50.3%) boys and 793 (49.7%) girls. The proportion of students from different districts was similar, as well as that of different grades.
Instrument
In this study, the questionnaire items were adapted from the relevant literature. The original measurement of peer interaction was adapted from six items of Active and Collaborative Learning in the National Survey of Student Engagement (Kuh, 2001), the measurement of learning motivation was adapted from five items of Deep Motivation in R-SPQ-2F of Biggs et al. (2001), the measurement of critical thinking was adapted from five items of the California Critical Thinking Disposition Inventory (Facione, 1990), and the measurement of PSSE was adapted from five items of Han (2020) combined with Bandura (2006) Self-Efficacy scale.
Content validity was then examined by two educational technology experts and three middle school students. Some items with unclear meaning were revised. The questionnaire used a 5-point Likert scale, with options “strongly disagree,” “disagree,” “neutral,” “agree,” and “strongly agree.” The higher the score, the higher the degree of agreement. After data collection, we tested the reliability and validity of the questionnaire items and constructs for subsequent structural equation modeling. The remaining items are listed in the Appendix.
Data analysis
To further test the content validity of the scale, nine experts were invited to judge and score the relevance between each item of the scale and the construct it belongs to. A 5-point scale was used in this study, with options “uncorrelated,” “weakly correlated,” “moderately correlated,” “strongly correlated,” and “very correlated.” For each item, the proportion of experts who agreed that this item was strongly related to the construct (score 4 or 5) to the total number is called the Item Content Validity Index (I-CVI); it needs to reach 0.78 (Lynn, 1986).
Before data analyses were performed, normality was tested. All the measured items had appropriate skewness (ranging from −0.893 to −0.295) and kurtosis (ranging from −0.216 to 0.954), smaller than the requisite maximum values of |1| and |2|, respectively, indicating that the data of all items were close to the normal distribution (Noar, 2003).
Data analysis consisted of four stages: Exploratory Factor Analysis (EFA), Confirmatory Factor Analysis (CFA), reliability analysis, and Structural Equation Modeling (SEM). EFA and reliability analysis of the scale were conducted with SPSS20.0, and CFA and SEM were conducted with Mplus 8.3. In the prior study, 301 samples were used for EFA and reliability analysis to test the scale. In the formal study, a randomly selected subsample 1 (n = 799) was used for EFA, and subsample 2 (n = 797) was used for CFA and SEM. In the reliability analysis, all 1,596 samples were used. Bootstrapping was used 1,000 times in the indirect effect test in SEM.
In EFA, principal component analysis and the maximum variance rotation method were used to extract the factors, and components were extracted with eigenvalues > 1. If the explained variance of the first factor before rotation is <50%, it can be considered that there is no serious significant common method bias (Hair et al., 2014). Items with cross factor loadings or low loadings (<0.5) were deleted (Deng et al., 2017).
In CFA and SEM, the standards recommended by Hair et al. (2014) were adopted. Accordingly, indices of χ2/df (<5), Root Mean Square Error of Approximation (RMSEA) (<0.10), Standardized Root Mean Square Residual (SRMR) (< 0.05), Comparative Fit Index (CFI) (>0.90), and the Tucker-Lewis Index (TLI) (>0.90) were used to check the model fit degree. Then Average Variance Extracted (AVE) (>0.5) and Construct Reliability (CR) (>0.7) were calculated using factor loadings (λ) to check the convergent validity of the scale. The square root values of AVEs of components were compared with the correlations between components to check the discriminant validity of the scale. The correlations between all factors were tested for significance before SEM.
In the reliability analysis of the scale, the internal consistency coefficients' Cronbach's α values were calculated, where the whole scale and all constructs needed to be higher than 0.7 (Fornell and Larcker, 1981).
Results
Measurement model
In the content validity test stage, according to the nine experts' evaluation, four items were deleted as their I-CVI did not reach 0.78. A total of 21 items with good content validity were saved in the scale.
In the prior study, we conducted EFA using the 301 valid samples to explore the structural validity of the scale. Three items were deleted in three rounds of principal component analysis, as they have cross factor loadings (FL > 0.5 on two factors). The deleted items and retained items are shown in the Appendix. After that, the EFA result showed good validity. The internal consistency coefficient test showed good reliability of the scale. The Cronbach's α of the whole scale was 0.954, and the values of the subscales were between 0.850 and 0.945. All the construct reliabilities were higher than 0.7, indicating good reliability of the scale in the prior study.
In the formal study stage, the EFA results showed good validity of the scale. The Kaiser-Meyer-Olkin measure value was 0.917 (p < 0.001), indicating that it was suitable for factor analysis. The explained variance of the first factor before rotation was 43.520%, indicating no serious significant common method bias. A total of four main factors were obtained, and the total explained variance was 67.013%. The loadings of each item on the factor were between 0.580 and 0.823 (see Table 2).
In the formal study stage, CFA was carried out to verify the structural validity of the scale. The model fit index of χ2 was 608.769, df was 129, χ2/df was 4.719 (<5), RMSEA was 0.068 (<0.08), CFI was 0.942 (>0.90), TLI was 0.931 (>0.90), and SRMR was 0.041(< 0.05), indicating that the fit for the items of the scale was acceptable. All standardized factor loadings were in a good range of 0.631–0.876. The values of AVE were all higher than 0.5, and CR was higher than 0.7, indicating good convergent validity. All the square root values of AVE of each component were higher than the correlations between it and other components (see Table 3), indicating good discriminant validity. All the correlations between every two factors were significant.
In the formal study stage, the internal consistency coefficient test showed good reliability of the scale. The Cronbach's α of the whole scale was 0.920, and the values of the subscales are shown in Table 2. All the construct reliabilities were higher than 0.7, indicating good reliability. Means of components were all above the midpoint 3, as shown in Table 2.
Structural model
The model fit indices of the structural equation model (SEM) were good using the 797 samples for verification. The value of χ2 was 632.437, df was 130, χ2/df was 4.865 (<5), RMSEA was 0.070 (<0.08), CFI was 0.939 (>0.90), TLI was 0.929 (>0.90), and SRMR was 0.048 (< 0.05), indicating a good fit of the structural equation model. The verification of the research model is shown in Figure 2.
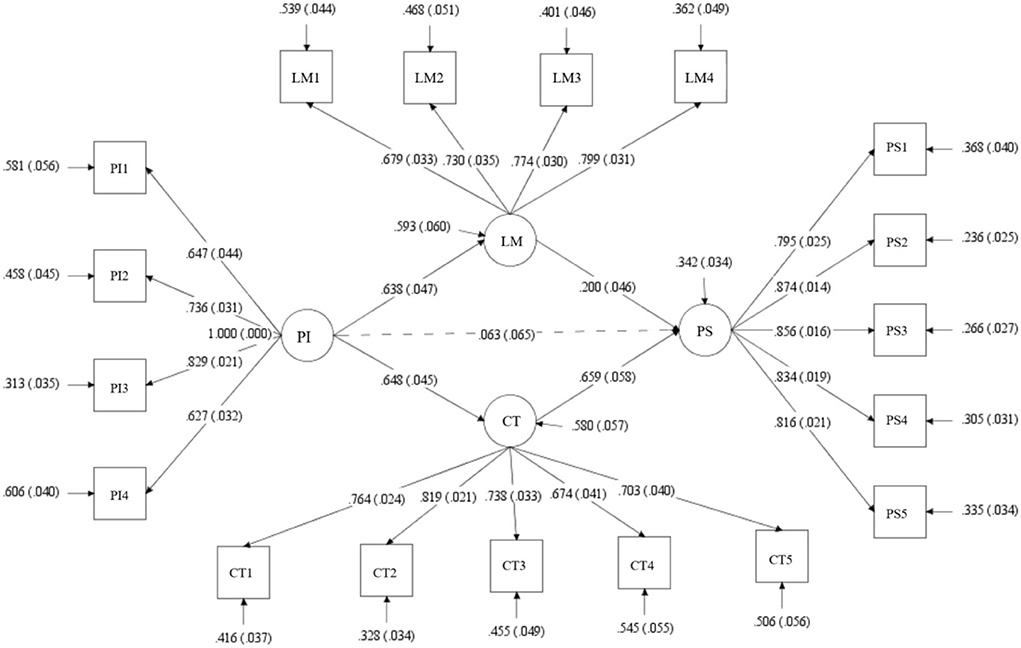
Figure 2. The verification of the structural model. The figure shows the SEM results of bootstrap 1,000 times. PI, Attitude toward Peer Interaction; LM, Learning Motivation; CT, Critical Thinking; PS, PSSE.
For middle school students, the attitude toward peer interaction could significantly positively predict their online learning motivation, of which the effect was 0.638 (p < 0.001), indicating that H1 was supported. Attitude toward peer interaction could significantly positively predict critical thinking, of which the effect was 0.648 (p < 0.001), indicating that H2 was supported. Learning motivation could positively predict PSSE, of which the effect was 0.200 (p < 0.001), indicating that H3 was supported. Critical thinking could positively predict PSSE, of which the effect was 0.659 (p < 0.001), indicating that H4 was supported.
In the path analysis, the attitude toward peer interaction did not have a significant direct effect on PSSE, of which the path effect was 0.063 (p = 0.331). To test the indirect effect, the bootstrap method was used. The 95% Confidence Interval (CI) was used to test whether there was an indirect effect. If the 95% CI does not include 0, an indirect effect exists (Guo et al., 2018). As shown in Table 4, the indirect effect from the attitude toward peer interaction to PSSE through learning motivation was 0.128 (p < 0.001), and the 95% CI did not include 0. The indirect effect from attitude toward peer interaction to PSSE through critical thinking was 0.427 (p < 0.001), and the 95% CI did not include 0. This indicated that attitude toward peer interaction could indirectly predict PSSE through learning motivation and critical thinking, respectively. This suggested that learning motivation and critical thinking played a full mediating role in how attitude toward peer interaction predicted PSSE. H5 was supported.
The values of explanatory power (R2) of learning motivation, critical thinking, and PSSE were, respectively, 0.407, 0.420, and 0.658. This showed that the variables of each facet had effective explanatory power of the model, as they were above the threshold of 0.3 (Cohen, 1977).
Discussion
A previous study indicated that participation in the peer online learning program had a positive impact on the development of communication and collaboration skills related competencies—interaction and sharing with technologies (Carvalho and Santos, 2022). Peer interaction could enhance students' engagement in online learning and increase their academic emotions (Wang et al., 2022). During the COVID-19 pandemic, it seems that online learning has greatly promoted teaching and learning reforms in the Internet environment, but problems have been exposed such as the lack of a collective atmosphere of study and social communication (Xu, 2021). Against this background, this study measured the online peer interaction of middle school students, and measured their online learning outcomes from the perspectives of psychology, knowledge, and ability. The scale used in this study had good reliability and validity. On this basis, this study explored the predictive effect of the attitude toward online peer interaction on the online learning motivation, knowledge construction, critical thinking, and PSSE of middle school students.
From the results, the attitude toward peer interaction could significantly predict the online learning motivation of junior middle school students, and the path coefficient was high at 0.645. This showed that when students in middle school had positive attitudes toward online peer interaction, they would more likely be interested in online learning and be eager to learn online. This provides support to the view of the growth emotion mindset, which posits that emotions are malleable and can be changed with effort and experience (Wols et al., 2020). The result was similar to previous studies in higher education (Yang and Chang, 2012; Thanasi-Boce, 2021), but the prediction effect of junior middle school students during the COVID-19 pandemic was much stronger. For teenagers, peer socializing is an important source of motivation for them to participate in many activities (Tsai et al., 2015). Especially during the COVID-19 pandemic, most students felt lonely and anxious during online learning (Alshammari et al., 2021). Improving students' attitude toward peer interaction could help them improve their online learning motivation.
The attitude toward online peer interaction also had a strong predictive effect on the critical thinking of middle school students, with a path coefficient of 0.670. This is consistent with previous research about university students (Guiller et al., 2008). With active attitudes toward online peer interaction, middle school students could exchange different views, question others, debate, and negotiate (Goodsett, 2020; Varenina et al., 2021). In this way, they could learn to evaluate the views of others, instead of blindly listening to authority. They would also think more from different perspectives and multiple angles, so as to develop their critical thinking. Previous research found that middle school students usually gave surface and rapid responses instead of engaging in critical thinking during online learning, even though the instructor guided them to think critically (Zhang, 2013). Improving peer interactions among students might be a useful solution to the problem.
Online learning motivation could positively predict PSSE. This is consistent with the nursing training field (Yardimci et al., 2017). The autonomy-controlling and autonomy-supportive needs to learn knowledge might arise from the problem-solving process, and be maintained and enhanced by students' motivation (Wu et al., 2020). During the COVID-19 pandemic, the level of learning motivation of middle school students might be lower than that of traditional learning, as there was a lack of peer pressure, classroom experience, and teachers' attention (Uka and Uka, 2020). To improve students' performance of problem-solving, some measures should be taken to help students maintain and enhance their learning motivation.
Critical thinking could positively predict PSSE during the COVID-19 pandemic, of which the explanation rate was the highest for predicting PSSE. When students have better critical thinking, they are more likely to find the problem and evaluate the solution to the problem reasonably (Koehler et al., 2022), which can lead to better PSSE. This is consistent with the finding of Kanbay and Okanli (2017). For middle school students, it is sometimes difficult to guide them to develop critical thinking online (Zhang, 2013), which may negatively lead to PSSE. More useful measures should be taken to improve students' critical thinking, which is the key to promoting good performance in problem solving.
Developing problem-solving ability through peer collaboration has become a focus of learning in recent years (OECD, 2017), in which motivation as emotional mindset and critical thinking as cognitive mindset should be considered. As the results of this study showed, the direct prediction of attitude toward peer interaction on the PSSE of middle school students was not significant, but the indirect effects analysis showed that learning motivation and critical thinking were two total mediating factors of the effects from attitude toward peer interaction to PSSE. This is further exploration and a complement to previous research findings (Jordan and McDaniel, 2014; Setyowidodo et al., 2020). Previous research found that peer interaction could have an impact on PSSE (Jordan and McDaniel, 2014), but did not consider the role of mindset in this process. This research explores the role of two important mindsets in this process, and showed that attitude toward peer interaction could have an effect on PSSE through learning motivation and critical thinking. This showed that the peer interaction of middle school students during the epidemic mainly played a direct role in promoting their mindsets of motivation and critical thinking, but it was difficult to directly help students solve problems. This might be due to the fact that online peer interactions among middle school students during the pandemic were mostly spontaneous interactions, with less involving online collaborative problem-solving learning activities designed and organized by teachers in China (Yu and Wang, 2020); thus, the direct prediction effect was not obvious. To explore this possibility in depth, more elaborate experimental designs are needed in the future.
Drawing on learning ecology, this study provides evidence for the importance of attitude toward peer interaction for the online learning of middle school students during the COVID-19 pandemic. The findings of this study reflect the importance of online peer interaction for middle school students during the pandemic. The results demonstrated the positive predictive effect of peer interaction on motivation and critical thinking, and reflected that the two mindsets can positively predict students' PSSE. Lacking an online collaborative problem-based activity design provided by teachers during the pandemic, online peer interaction did not directly support PSSE, but indirectly predicted it through promoting mindsets. Briefly, this study explored the relationship among attitude toward peer interaction, motivation, critical thinking, and PSSE from a new perspective in a special period. The structural model is innovative and reflects the particularity of middle school students. In the post-epidemic era, online learning has become an important learning method for junior high school students, for which peer interaction is of great significance.
Conclusion
Implications
This study used structural equation modeling to demonstrate the positive effect of attitude toward peer interaction on middle school students' learning outcomes during the pandemic. On the one hand, this fills the gap of previous research, that is, the lack of attention to the development of higher-order thinking in online learning in K-12 education. On the other hand, the study proposes a new model, which has a theoretically innovative role for studying peer interaction, mindsets, and PSSE in online learning.
This study provides a reference for organizing middle school students to carry out online learning. The study found that attitude toward peer interaction had a very positive predictive effect on learning mindsets and PSSE. Based on this finding, educators can guide learners to interact more with peers based on social networking sites when organizing middle school students' online learning. In practice, the scale developed in this study can be used to measure students' online learning outcomes and to adjust the design and organization of online learning in a timely manner.
Future research suggestions
The peer interaction studied in this research was general interaction without a specific classification. In previous studies, some researchers studied peer interaction more specifically and deeply, such as whether there were task instructions for peer interaction and how many people interacted in groups (Tenenbaum et al., 2020). What is more, different kinds of interaction platforms, such as formal learning forums (MOOC forum) and social media (Facebook, Wechat) may also lead to different results. In previous research, digital natives preferred to interact with each other through the social networks that they were familiar with rather than the forum embedded in a learning platform (Butrime et al., 2015). This preference could be further studied in the future.
In addition, some researchers have studied students' competencies in more specific online learning courses, such as online nursing courses (Song et al., 2022) or person-centered online courses (Li and Tsai, 2017; Koenka, 2020). However, this study did not specify what kind of online course affects participants' cognitive and emotional factors. Future studies may explore what kinds of online courses trigger learners' emotional and cognitive mindsets in online peer interaction.
Due to the limitations of the self-report scale, the data obtained in this study may lack a certain degree of objectivity. In future research, the results of this study can be validated through learning analysis based on data collected from the learning platform.
Data availability statement
The raw data supporting the conclusions of this article will be made available by the authors, without undue reservation.
Ethics statement
Ethical review and approval was not required for the study on human participants in accordance with the local legislation and institutional requirements. The patients/participants provided their written informed consent to participate in this study.
Author contributions
XA contributed to the conception of the study, performed instrument development, data analysis, and wrote the manuscript. J-CH contributed to the theoretical framework of the study and wrote the manuscript. YL contributed the instrument development, data collection, and manuscript preparation. YZ contributed to the instrument development and manuscript revision. All authors contributed to the article and approved the submitted version.
Funding
This work described in this paper was supported by a grant from the National Social Science Fund of China (Grant Number: 21&ZD238). This work was financially supported by the Institute for Research Excellence in Learning Sciences of National Taiwan Normal University (NTNU) from the Featured Areas Research Center Program within the framework of the Higher Education Sprout Project by the Ministry of Education (MOE) in Taiwan and BNU Interdisciplinary Research Foundation for First-year Doctoral Candidates BNUXKJC2102.
Conflict of interest
The authors declare that the research was conducted in the absence of any commercial or financial relationships that could be construed as a potential conflict of interest.
Publisher's note
All claims expressed in this article are solely those of the authors and do not necessarily represent those of their affiliated organizations, or those of the publisher, the editors and the reviewers. Any product that may be evaluated in this article, or claim that may be made by its manufacturer, is not guaranteed or endorsed by the publisher.
References
Ajzen, I. (2005). Attitudes, Personality and Behaviour, 2nd Edn. Berkshire, GBR: McGrawHill Professional Publishing.
Ala, O. G., Yang, H., and Ala, A. A. (2021). Leveraging integrated peer-assisted learning clusters as a support for online learning. Interact. Learn. Environ. doi: 10.1080/10494820.2021.1943454 [Epub ahead of print].
Allen, T. D., Golden, T. D., and Shockley, K. M. (2015). How effective is telecommuting? Assessing the status of our scientific findings. Psychol. Sci. Public Interest 16, 40–68. doi: 10.1177/1529100615593273
Alshammari, A. A., Alshammari, A. M., Alotaibi, N. M., Mujtaba, M. A., and Alam, M. T. (2021). The challenges faced by pharm. D students in online learning due to Covid-19 Pandemic and its impact on their mental health in the Northern Border Region of Saudi Arabia. Pakistan J. Med. Health Sci. 15, 2819–2822. doi: 10.53350/pjmhs211592819
An, X., Gong, X., Zhao, R., and Ma, N. (2020). A comparative study of the interaction features of barrage and forum in social learning course. Int. J. Inform. Educ. Technol. 10, 383–388. doi: 10.18178/ijiet.2020.10.5.1394
Aslan, A. (2021). Problem- based learning in live online classes: learning achievement, problem-solving skill, communication skill, and interaction. Comput. Educ. 171, 104237. doi: 10.1016/j.compedu.2021.104237
Baker, R., Evans, B., and Dee, T. (2016). A randomized experiment testing the efficacy of a scheduling nudge in a massive open online course (MOOC). AERA Open. 2, 1–18. doi: 10.1177/2332858416674007
Bandura, A. (1994). “Self-efficacy,” in Encyclopedia of Human Behavior, ed V. S. Ramachaudran (Academic Press), 71–81. Available online at: https://www.sciencedirect.com/referencework/9780080961804/encyclopedia-of-human-behavior#book-info (accessed July 25, 2015).
Bandura, A. (2006). “Guide for constructing self-efficacy scales,” in Self-Efficacy Beliefs of Adolescents, eds F. Pajares and T. Urdan, Vol. 5 (Greenwich, CT: Information Age), 307–337.
Becker, R., and Birdi, A. (2018). Flipping the classroom: old ideas, new technologies. Int. Rev. Econ. Educat. 29, 1–5. doi: 10.1016/j.iree.2018.06.001
Biggs, J., Kember, D., and Leung, D. Y. (2001). The revised two-factor study process questionnaire: R-SPQ-2F. Br. J. Educ. Technol. 71, 133–149. doi: 10.1348/000709901158433
Butrime, E., Vitkute-Adzgauskiene, D., Zuzeviciute, V., and Statkeviciene, V. (2015). “Digital native student attitude on learning with modern ICT tools,” in EDULEARN15: 7TH International Conference on Education and New Learning Technologies, 5651–5661. Available online at: https://library.iated.org/view/BUTRIME2015DIG (accessed July 25, 2015).
Calaguas, N. P., and Consunji, P. M. P. (2022). A structural equation model predicting adults' online learning self-efficacy. Educ. Inform. Technol. 27, 6233–6249. doi: 10.1007/s10639-021-10871-y
Carvalho, A. R., and Santos, C. (2022). Developing peer mentors' collaborative and metacognitive skills with a technology-enhanced peer learning program. Comput. Educ. Open 3, 100070. doi: 10.1016/j.caeo.2021.100070
Castellaro, M. A., and Roselli, N. D. (2015). Peer collaboration in childhood according to age, socioeconomic context and task. Eur. J. Psychol. Educ. 30, 63–80. doi: 10.1007/s10212-014-0228-3
Chadha, A. (2019). Deepening engagement: the intimate flow of online interactions. Int. J. Online Pedag. Course Design 9, 32–47. doi: 10.4018/IJOPCD.2019070103
Cheng, G., and Chau, J. (2016). Exploring the relationships between learning styles, online participation, learning achievement and course satisfaction: an empirical study of a blended learning course. Br. J. Educ. Technol. 47, 257–278. doi: 10.1111/bjet.12243
Chi, M. T. H., and Glaser, R. (1985). “Problem-solving ability,” in Human Abilities: An Information Processing Approach, ed R. J. Sternberg (New York, NY: W. H. Freeman and Company).
Choi-Sung, K. (2022). Focus group interview on school teachers' experience in responding to learning gap due to COVID-19: focusing on e-learning. J. Learner Centered Curriculum Instruction 22, 245–261. doi: 10.22251/jlcci.2022.22.5.245
Chou, C., Huang, M., Huang, C., Lu, F. J. H., and Tu, H. (2015). The mediating role of critical thinking on motivation and peer interaction for motor skill performance. Int. J. Sport Psychol. 46, 391–408. doi: 10.7352/IJSP.2015.46.391
Chu, A. M. Y., Liu, C. K. W., So, M. K. P., and Lam, B. S. Y. (2021). Factors for sustainable online learning in higher education during the COVID-19 pandemic. Sustainability 13, 50389. doi: 10.3390/su13095038
Clark, A. E., Nong, H., Zhu, H., and Zhu, R. (2021). Compensating for academic loss: online learning and student performance during the COVID-19 pandemic. China Econ. Rev. 68, 101629. doi: 10.1016/j.chieco.2021.101629
Cohen, J. (1977). Statistical Power Analysis for the Behavioral Sciences, Vol. 31. Academic Press, NIC. doi: 10.1016/C2013-0-10517-X
Deng, F., Chai, C. S., So, H., Qian, Y., and Chen, L. (2017). Examining the validity of the technological pedagogical content knowledge (TPACK) framework for preservice chemistry teachers. Aust. J. Educ. Technol. 33, 1–14. doi: 10.14742/ajet.3508
Dweck, C. S. (1999). “Essays in social psychology: self-theories,” in Their Role in Motivation, Personality, and Development, ed C. S. Dweck (New York, NY: Psychology Press).
Facione, P. A. (1990). Critical Thinking: A State of Expert Consensus for Perposes of Educational Assessment and Instruction. The Delphi Report. Millbrae: California Academic Press.
Fornell, C., and Larcker, D. F. (1981). Evaluating structural equation models with unobservable variables and measurement error. J. Market. Res. 24, 337–346. doi: 10.1177/002224378702400401
Gollwitzer, P. M., Heckhausen, H., and Steller, B. (1990). Deliberative and implemental mind-sets: Cognitive tuning toward congruous thoughts and information. J. Pers. Soc. Psychol. 59, 1119–1127. doi: 10.1037/0022-3514.59.6.1119
Goodsett, M. (2020). Best practices for teaching and assessing critical thinking in information literacy online learning objects. J. Acad. Librarianship 46, 1021635. doi: 10.1016/j.acalib.2020.102163
Guiller, J., Dumdell, A., and Ross, A. (2008). Peer interaction and critical thinking: face-to-face or online discussion? Learn. Instruction 18, 187–200. doi: 10.1016/j.learninstruc.2007.03.001
Guo, X., Lv, B., Zhou, H., Liu, C., Liu, J., Jiang, K., et al. (2018). Gender differences in how family income and parental education relate to reading achievement in China: the mediating role of parental expectation and parental involvement. Front. Psychol. 9, 783. doi: 10.3389/fpsyg.2018.00783
Hair, J. F., Black, B., Babin, B. J., and Anderson, R. (2014). Multivariate Data Analysis. Prentice Hall.
Han, J. W. (2020). Structural relationship among learning motivation, learning confidence, critical thinking skill and problem-solving ability, using digital textbooks. Int. J. Adv. Smart Converg. 9, 140–146. doi: 10.7236/IJASC.2020.9.2.140
Harmon-Jones, E., and Harmon-Jones, C. (2021). On defining positive affect (PA): considering attitudes toward emotions, measures of PA, and approach motivation. Curr. Opin. Behav. Sci. 39, 46–51. doi: 10.1016/j.cobeha.2021.01.008
Henderson, M. D., De Liver, Y., and Gollwitzer, P. M. (2008). The effects of an implemental mind-set on attitude strength. J. Pers. Soc. Psychol. 94, 396. doi: 10.1037/0022-3514.94.3.396
Hilty, L. M., and Aebischer, B. (2015). “ICT for sustainability: an emerging research field,” in ICT Innovations for Sustainability: Advances in Intelligent Systems and Computing, eds L. M. Hilty and B. Aebischer (New York, London: Springer International Publishing), 3–36.
Hong, J. C., Lee, Y. F., and Ye, J. H. (2021a). Procrastination predicts online self-regulated learning and online learning ineffectiveness on the Covid-19 lockdown. Pers. Individ. Dif. 174, 110673. doi: 10.1016/j.paid.2021.110673
Hong, J. C., Liu, X., Cao, W., Tai, K. H., and Zhao, L. (2022). Effects of self-efficacy and online learning mind states on learning ineffectiveness during the COVID-19 lockdown. Educ. Technol. Soc. 25, 142–154.
Hong, J. C., Liu, Y., Liu, Y., and Zhao, L. (2021b). High school students' online learning ineffectiveness in experimental courses during the COVID-19 pandemic. Front. Psychol. 12, 738695. doi: 10.3389/fpsyg.2021.738695
Jordan, M. E., and McDaniel, R. R. (2014). Managing uncertainty during collaborative problem solving in elementary school teams: the role of peer influence in robotics engineering activity. J. Learn. Sci. 23, 490–536. doi: 10.1080/10508406.2014.896254
Jung, S., and An, Y. (2021). A study on the current situation of the achievement gap in schools before and after COVID-19: focusing on the distribution of academic achievement grades of middle schools in Seoul. Korean J. Sociol. Educ. 31, 53–74. Available online at: https://www.kci.go.kr/kciportal/ci/sereArticleSearch/ciSereArtiView.kci?sereArticleSearchBean.artiId=ART002727545
Kalmar, E., Aarts, T., Bosman, E., Ford, C., de Kluijver, L., Beets, J., et al. (2022). The COVID-19 paradox of online collaborative education: when you cannot physically meet, you need more social interactions. Heliyon 8, e088231. doi: 10.1016/j.heliyon.2022.e08823
Kanbay, Y., and Okanli, A. (2017). The effect of critical thinking education on nursing students' problem-solving skills. Contemp. Nurse 53, 313–321. doi: 10.1080/10376178.2017.1339567
Kang, X., and Zhang, W. (2022). An experimental case study on forum-based online teaching to improve student's engagement and motivation in higher education. Interact. Learn. Environ. 1–12.
Kania, B. F., Wronska, D., and Zieba, D. (2017). Introduction to neural plasticity mechanism. Behav. Brain Sci. 7, 41–49. doi: 10.4236/jbbs.2017.72005
Koehler, A. A., Cheng, Z., Fiock, H., Wang, H., Janakiraman, S., and Chartier, K. (2022). Examining students' use of online case-based discussions to support problem solving: considering individual and collaborative experiences. Comput. Educ. 179, 104407. doi: 10.1016/j.compedu.2021.104407
Koenka, A. (2020). Academic motivation theories revisited: an interactive dialog between motivation scholars on recent contributions, underexplored issues, and future directions. Contemp. Educ. Psychol. 61, 101831. doi: 10.1016/j.cedpsych.2019.101831
Kraiger, K., Ford, J. K., and Salas, E. (1993). Application of cognitive, skill-based, and affective theories of learning outcomes to new methods of training evaluation. J. Appl. Psychol. 78, 311-328. doi: 10.1037/0021-9010.78.2.311
Kuh, G. D. (2001). The National Survey of Student Engagement: Conceptual Framework and Overview of Psychometric Properties. Bloomington, IN: Indiana University Center for Postsecondary Research.
Kumpulainen, K., and Mikkola, A. (2014). Boundary crossing of discourses in pupils' chat interaction during computer-mediated collaboration. Learn. Cult. Soc. Interact. 3, 43–53. doi: 10.1016/j.lcsi.2013.12.002
Kwon, K., Song, D., Sari, A. R., and Khikmatillaeva, U. (2019). Different types of collaborative problem-solving processes in an online environment: solution oriented versus problem oriented. J. Educ. Comput. Res. 56, 1277–1295. doi: 10.1177/0735633117740395
Kyung-hee, K. (2016). The effects of young children teachers' ego-strength and self-efficacy on problem solving. J. Korea Contents Assoc. 16, 638–648. doi: 10.5392/JKCA.2016.16.03.638
Lawter, L., and Garnjost, P. (2021). Cross-cultural comparison of digital natives in flipped classrooms. Int. J. Manage. Educ. 19, 100559. doi: 10.1016/j.ijme.2021.100559
Lee, J., Lim, H., Allen, J., and Choi, G. (2021). Effects of learning attitudes and COVID-19 risk perception on poor academic performance among middle school students. Sustainability 13, 554110. doi: 10.3390/su13105541
Li, L. Y., and Tsai, C.-C. (2017). Accessing online learning material: quantitative behavior patterns and their effects on motivation and learning performance. Comput. Educ. 114, 286–297. doi: 10.1016/j.compedu.2017.07.007
Lin, C. H., Zheng, B., and Zhang, Y. (2017). Interactions and learning outcomes in online language courses. Br. J. Educ. Technol. 48, 730–748. doi: 10.1111/bjet.12457
Lynn, M. R. (1986). Determination and quantification of content validity. Nurs. Res. 35, 382–386. doi: 10.1097/00006199-198611000-00017
Martin, F., Sun, T., and Westine, C. D. (2020). A systematic review of research on online teaching and learning from 2009 to 2018. Comput. Educ. 159, 104009. doi: 10.1016/j.compedu.2020.104009
Ngo, J., and Ngadiman, B. A. (2021). Investigating student satisfaction in remote online learning settings during COVID-19 in Indonesia. J. Int. Compar. Educ. 10, 73–95. doi: 10.14425/jice.2021.10.2.0704
Noar, S. M. (2003). The role of structural equation modeling in scale development. Struct. Eq. Model. Multidiscip. J. 10, 622–647. doi: 10.1207/S15328007SEM1004_8
Nolder, C. J., and Kadous, K. (2018). Grounding the professional skepticism construct in mindset and attitude theory: a way forward. Account. Org. Soc. 67, 1–14. doi: 10.1016/j.aos.2018.03.010
OECD (2017). PISA 2015 Results (Volume V): Collaborative Problem Solving. Paris: OECD Publishing. Available online at: https://www.oecd.org/education/pisa-2015-results-volume-v-9789264285521-en.htm (accessed July 25, 2015).
Oh, E. G., Huang, W. D., Mehdiabadi, A. H., and Ju, B. (2018). Facilitating critical thinking in asynchronous online discussion: comparison between peer- and instructor-redirection. J. Comput. Higher Educ. 30, 489–509. doi: 10.1007/s12528-018-9180-6
Perkins, K. N., Carey, K., Lincoln, E., Shih, A., Donalds, R., Schneider, S. K., et al. (2021). School connectedness still matters: the association of school connectedness and mental health during remote learning due to COVID-19. J. Primary Prevent. 42, 641–648. doi: 10.1007/s10935-021-00649-w
Salazar, L. R., and Hayward, S. L. (2018). An examination of college students' problem-solving self-efficacy, academic self-efficacy, motivation, test performance, and expected grade in introductory-level economics courses. Decis. Sci. J. Innovat. Educ. 16, 217–240. doi: 10.1111/dsji.12161
Setyowidodo, I., Jatmiko, B., Susantini, E., Handayani, A. D., and Pramesti, Y. S. (2020). “The role of science project based peer interaction on improving collaborative skills and physical problem solving: a mini review,” in International Conference on Mathematics and Science Education 2019 (ICMSCE 2019), 1521(022032). doi: 10.1088/1742-6596/1521/2/022032
Song, Y., Lee, Y., and Lee, J. (2022). Mediating effects of self-directed learning on the relationship between critical thinking and problem-solving in student nurses attending online classes: a cross-sectional descriptive study. Nurse Educ. Today 109, 105227. doi: 10.1016/j.nedt.2021.105227
Sternberg, R. J. (1988). The Nature of Creativity: Contemporary Psychological Perspectives. New York, NY: Cambridge University Press.
Tawfik, A. A., Graesser, A., Gatewood, J., and Gishbaugher, J. (2020). Role of questions in inquiry-based instruction: towards a design taxonomy for question-asking and implications for design. Educ. Tech Res. Dev. 68, 653–678. doi: 10.1007/s11423-020-09738-9
Tenenbaum, H. R., Winstone, N. E., Leman, P. J., and Avery, R. E. (2020). How effective is peer interaction in facilitating learning? A meta-analysis. J. Educ. Psychol. 112, 1303–1319. doi: 10.1037/edu0000436
Thanasi-Boce, M. (2021). The role of the instructor, motivation, and interaction in building online learning satisfaction during the COVID-19 pandemic. Electron. J. E Learning 19, 401–415. doi: 10.34190/ejel.19.5.2475
Tsai, A. C., Pierce, C. M., and Papachristos, A. V. (2015). From social networks to health: Durkheim after the turn of the millennium Introduction. Soc. Sci. Med. 125, 1–7. doi: 10.1016/j.socscimed.2014.10.045
Uka, A., and Uka, A. (2020). The effect of students experience with the transition from primary to secondary school on self-regulated learning and motivation. Sustainability 12, 8519. doi: 10.3390/su12208519
van Laar, E., van Deursen, A. J. A. M., van Dijk, J. A. G. M., and de Haan, J. (2017). The relation between 21st-century skills and digital skills: a systematic literature review. Comput. Human Behav. 72, 577–588. doi: 10.1016/j.chb.2017.03.010
Varenina, L., Vecherinina, E., Shchedrina, E., Valiev, I., and Islamov, A. (2021). Developing critical thinking skills in a digital educational environment. Thinking Skills Creativity 41, 100906. doi: 10.1016/j.tsc.2021.100906
Wang, Y., Cao, Y., Gong, S., Wang, Z., Li, N., and Ai, L. (2022). Interaction and learning engagement in online learning: the mediating roles of online learning self-efficacy and academic emotions. Learn. Individ. Differ. 94, 102128. doi: 10.1016/j.lindif.2022.102128
Wols, A., Poppelaars, M., Lichtwarck-Aschoff, A., and Granic, I. (2020). The role of motivation to change and mindsets in a game promoted for mental health. Entertain. Comput. 35, 100371. doi: 10.1016/j.entcom.2020.100371
Wu, J.-Y., Hsiao, Y.-C., and Nian, M.-W. (2020). Using supervised machine learning on large-scale online forums to classify course-related Facebook messages in predicting learning achievement within the personal learning environment. Interact. Learn. Environ. 28, 65–80. doi: 10.1080/10494820.2018.1515085
Wu, J. Y., and Nian, M. W. (2021). The dynamics of an online learning community in a hybrid statistics classroom over time: implications for the question-oriented problem-solving course design with the social network analysis approach. Comput. Educ. 166, 104120. doi: 10.1016/j.compedu.2020.104120
Xu, T. (2021). How do students view online learning: an empirical study of online learning during the Covid-19 Pandemic. Revista Rasileira De Educacao Do Campo Brazilian J. Rural Educ. 6, e11853. doi: 10.20873/uft.rbec.e11853
Yang, C., and Chang, Y. S. (2012). Assessing the effects of interactive blogging on student attitudes towards peer interaction, learning motivation, and academic achievements. J. Computer Assist. Learn. 28, 126–135. doi: 10.1111/j.1365-2729.2011.00423.x
Yao, X., and Zheng, Y. (2017). “The probe to the educational model of “Peer-to-Peer Interaction” for college students' negative employment psychology,” in International Conference on Information, Computer and Education Engineering (ICICEE 2017), 257–261. doi: 10.12783/dtcse/icicee2017/17156
Yardimci, F., Bektas, M., Ozkütük, N., Muslu, G. K., Gerçeker, G. O., and Bas,bakkal, Z. (2017). A study of the relationship between the study process, motivation resources, and motivation problems of nursing students in different educational systems. Nurse Educ. Today 48, 13–18. doi: 10.1016/j.nedt.2016.09.017
Ying, B., Geng, Y., Lin, J., and Li, Q. (2021). A large-sample survey of English learning anxiety in middle school students during class resumption in COVID-19 Pandemic. J. Southwest University Natural Sci. Edition 43, 22–30. doi: 10.13718/j.cnki.xdzk.2021.01.003
Yu, J., and McLellan, R. (2020). Same mindset, different goals and motivational frameworks: profiles of mindset-based meaning systems. Contemp. Educ. Psychol. 62, 101901. doi: 10.1016/j.cedpsych.2020.101901
Yu, S., and Wang, H. (2020). How to better organize online learning in extreme environments such as the epidemic. China Educ. Technol. 2020, 6–14+33.
Zamecnik, A., Kovanovi'c, V., Joksimovi'c, S., and Liu, L. (2022). Exploring non-traditional learner motivations and characteristics in online learning: a learner profile study. Comput. Educ. Artif. Intell. 3, 100051. doi: 10.1016/j.caeai.2022.100051
Zhang, M. (2013). Prompts-based scaffolding for online inquiry: design intentions and classroom realities. Educ. Technol. Soc. 16, 140–151. Available online at: https://www.jstor.org/stable/jeductechsoci.16.3.140
Zhao, L., Thomas, P., and Zhang, L. (2021). Do our children learn enough in Sky Class? A case study: online learning in Chinese primary schools in the COVID era March to May 2020. Smart Learn. Environ. 8, 351. doi: 10.1186/s40561-021-00180-9
Zhou, Y., An, X., Li, X., Li, L., Gong, X., Li, Y., et al. (2021). A literature review of questionnaires for the assessment of online learning with a specific focus on the factors and items employed. Aust. J. Educ. Technol. 38, 182–204. doi: 10.14742/ajet.6719
Zulkifli, N. N., Abd Halim, N. D., Yahaya, N., and van der Meijden, H. (2020). Patterns of critical thinking processing in online reciprocal peer tutoring through facebook discussion. IEEE Access 8, 24269–24283. doi: 10.1109/ACCESS.2020.2968960
Zuo, M., Hu, Y., Luo, H., Ouyang, H., and Zhang, Y. (2021). K-12 students' online learning motivation in China: an integrated model based on community of inquiry and technology acceptance theory. Educ. Inform. Technol. 27, 4599–4620. doi: 10.1007/s10639-021-10791-x
Appendix
Keywords: attitude, peer interaction, motivation, critical thinking, problem-solving, middle school, online learning, COVID-19
Citation: An X, Hong J-C, Li Y and Zhou Y (2022) The impact of attitude toward peer interaction on middle school students' problem-solving self-efficacy during the COVID-19 pandemic. Front. Psychol. 13:978144. doi: 10.3389/fpsyg.2022.978144
Received: 25 June 2022; Accepted: 08 August 2022;
Published: 25 August 2022.
Edited by:
Juzhe Xi, East China Normal University, ChinaReviewed by:
Chunhui Qi, Henan Normal University, ChinaChang Lu, Shanghai Jiao Tong University, China
Copyright © 2022 An, Hong, Li and Zhou. This is an open-access article distributed under the terms of the Creative Commons Attribution License (CC BY). The use, distribution or reproduction in other forums is permitted, provided the original author(s) and the copyright owner(s) are credited and that the original publication in this journal is cited, in accordance with accepted academic practice. No use, distribution or reproduction is permitted which does not comply with these terms.
*Correspondence: Yushun Li, bHlzaHVuQGJudS5lZHUuY24=