- 1Chengdu Second People’s Hospital, Chengdu, Sichuan, China
- 2Chengdu Medical College, Chengdu, Sichuan, China
Background: Online lending on campus is given more attention by researchers as its prominent adverse effects on students. The deficiencies of the previous studies on its psychological factors and intervention strategies were only based on qualitative research. Moreover, there is no study on gender differences. Therefore, our study aims to analyze the gender differences in psychological risk factors and give some practical suggestions for the intervention by quantitative methods.
Method: This is a cross-sectional survey among medical college students in Chengdu. A total of 984 effective questionnaires were collected. The questionnaire includes demographic data, monthly expenses, self-evaluation for three central psychology causing online lending based on empiricism (conformity, comparison, and hedonism), and three psychological assessment instruments (the Chinese version of the Satisfaction with Life Scale, Egna Minnen av Barndoms Uppfostran, and 144-item version of Temperament and Character Inventory). T-test/χ2-test and Binary logistic regression were used to analyze the gender differences in variables and the risk factors of online lending for males and females, respectively.
Results: The utilization rate of online lending exhibited a significant gender difference (p < 0.001). In addition, there were gender differences in the scores on SWLS and some subscales of C-EMBU and TCI-144. The risk factors for males’ were family members using online lending (OR = 5.527, 95% CI = 1.784–17.125) and lower scores on HA (OR = 0.938, 95% CI = 0.888–0.990). The risk factors for females’ online lending were family members using online lending (OR = 2.288, 95% CI = 1.201–4.362), hedonism (OR = 5.913, 95% CI = 1.327–26.341), and higher scores on mother’s punishment (OR = 1.099, 95% CI = 1.007–1.199).
Conclusion: The utilization rate of online lending in males was significantly higher than in females. More attention should be paid to gender differences and the impact of family members’ using online lending on students when intervening in online lending.
Introduction
Various kinds of online lending platforms are expanding as China’s Internet economy grows. Online lending in China currently has no official definition and is split into two categories: platforms for e-commerce services like Ant Check Later, and lending platforms for reducing financial stress, such as P2P, Ant Jie-bei (Chang, 2018). As its application procedure does not require the permission of students’ parents or legal guardians and its approval is fast (even in a few minutes), online lending overspreads quickly among college students. Despite the government’s efforts to crack down on P2P with high-interest rates, many students continue to use online lending, such as Ant Jie-bei. Thus, the shortcomings, influencing factors, and intervention strategies of online lending became the research foci for Chinese scholars.
Studies abroad on college students’ debt revealed that the debt in medical students increased yearly and had a negative effect on their choice of internship, marriage age, graduate destination, and quality of life (Jette, 2016; Ling et al., 2018; Gray et al., 2019; Amin et al., 2021). On the other hand, although the annual interest rate of online lending is above 13%, many college students (approximately 42%) still hold a positive attitude toward it. Additionally, in repayment, more than half of college students transferred the loans to their parents; worse still, some students repaid the debt by applying for another online lending (Xiao et al., 2018; Tang and Tang, 2020).
According to some scholars’ conclusions based on qualitative research, there were three critical psychological factors (conformity, comparison, and hedonism) and several intervening strategies for online lending, including conducting ideological and political education, strengthening legal supervision, and enhancing risk awareness (Dong, 2017; Xiao et al., 2018; Yang et al., 2019; Tang and Tang, 2020). The deficiencies of previous studies were the lack of quantitative research and gender differences in online lending. Thus, this study aims to analyze the gender differences in psychological risk factors and offer some practical suggestions for the intervention by quantitative methods.
Materials and methods
Study population and setting
This was a cross-sectional survey. A total of 984 questionnaires (from 349 males and 635 females) were obtained after excluding 316 invalid questionnaires with the artificial method by three prominent researchers, including the same choice for each question, two-thirds of options blank, or regular answers (effective rate = 75.69%). The questionnaire consisted of demographic information (Table 1), monthly expenses, self-evaluation for conformity, comparison, and hedonism (Table 2), as well as the following three psychological assessment instruments. Informed consent was secured from all participants.
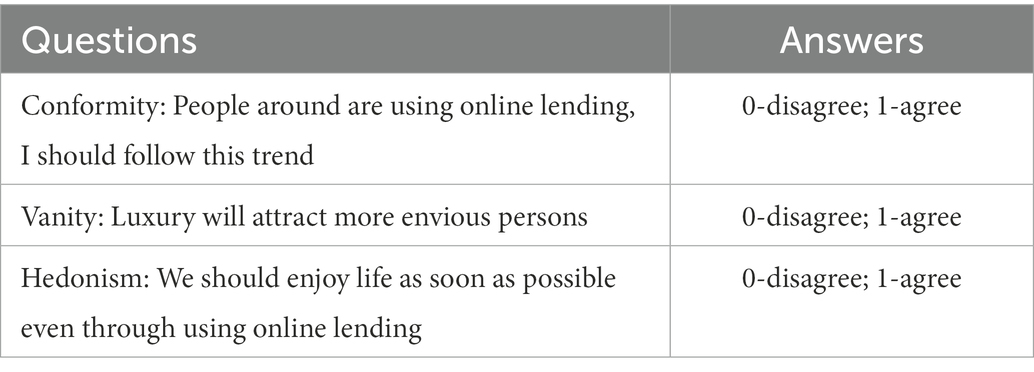
Table 2. The survey questions of three central psychology causing online lending based on empiricism.
Assessment instruments
The Chinese version of SWLS for life satisfaction (C-SWLS)
The SWLS is widely used to measure life satisfaction and contains five items scored on a 7-point Likert scale (1, strongly disagree, to 7, strongly agree) (Diener et al., 1985). It has measurement invariance across gender and age and high internal reliability with Cronbach’s α > 0.70 (Bai et al., 2011; Silva et al., 2014).
The Chinese version of EMBU for parenting styles (C-EMBU)
The C-EMBU involves six subscales for the father’s parenting styles, including emotional warmth, punishment, over-interference, favoring subjects, rejection, and over-protection; five subscales for the mother’s parenting styles with emotional warmth, punishment, favoring subjects, rejection, and over-protection. The subjects evaluated 116 items by using a 4-point Likert scale (1-never, to 4- always). The Cronbach’s α for each subscale ranged from 0.46–0.88 (Yue et al., 1993).
The 144-item Chinese version of TCI for personality (TCI-144)
The TCI-144 consisted of a four-factor structure for temperament domains and a three-factor structure for character domains as follows: novelty seeking (NS), harm avoidance (HA), reward dependence (RD), persistence (P), self-directedness (SD), cooperativeness (C), and self-transcendence (ST). The subjects evaluated the items using a 5-point Likert scale (1, “very unlikely” to 5, “very likely”). The Cronbach’s α for each subscale ranged from 0.488 to 0.823 (Chen et al., 2013).
Data analysis
Data analysis was conducted using IBM SPSS Statistics 19.0. Data interpolation was used to supplement the missing data. χ2-test/t-test was used to examine the gender differences among variables, and binary logistic regression was used to explore the risk factors of online lending for males and females, respectively. The level of statistical significance was set at p < 0.05.
Results
The gender differences among variables
A total of 681 students had used online lending (utilization rate = 68.2%). The comparisons for using online lending, monthly living expenses, the scores of SWLS, family parenting styles, and TCI-144 between genders are listed in Table 3. In terms of the utilization rate of online lending, negative parenting styles (punishment, over-interference, rejection, and over-protection in father’s parenting styles; punishment, rejection, over-protection in mother’s parenting styles), NS, C, and St, males were significantly higher than females. In contrast, positive parenting styles (emotional warmth of father’s parenting style and mother’s parenting style), HA, RD, and SD in males were significantly lower than that of females.
The risk factors of online lending for males and females
Binary logistic regression was used to explore the risk factors of online lending for males and females. Both of the models were well fitted (χ2male = 7.362, p = 0.498, Cox & Snell R2 = 0.179; χ2female = 9.155, p = 0.329, Cox & Snell R2 = 0.107). Family members using online lending and low harm avoidance are the risk factors for males’ online lending (Table 4). The number of male users whose family members also used online lending was 5.527 times that of those whose family members did not. Each unit increase in the scores of injury avoidance was associated with a 0.062 times reduction in the risk of online lending. Family members using online lending, hedonism, and mother punishment are the risk factors for females’ online lending (Table 5). The number of female users whose family members also used online lending was 2.288 times that of those whose family members did not. The number of female users who were hedonistic was 5.931 times that of those who were not. Every one-unit increment of the scores of mother punishment was a 0.099 times increase in the risk of online lending. The most important explanatory variable was the family members using online lending in the males’ model (Wald = 8.780) and in the females’ model (Wald = 6.327).
Discussion
Utilization rate of online lending
The utilization rate for online lending among medical students in Chengdu was 68.2%, much higher than the other studies based in different cities (Table 6; Zhang et al., 2018; Fu et al., 2019; Zhang, 2019; Pan, 2020; Li, 2021; Shi et al., 2021). Comparing the surveys conducted in Chengdu in 2018, it could be deduced that the utilization rate increases over time, which could be attributed to the widespread advertising of online lending platforms. Therefore, it is crucial to strengthen online lending platforms’ supervision actively. Additionally, a correlation study was conducted between the utilization rate of online lending and the annual GDP for each city by assuming that the utilization rate might have a relationship with the city’s GDP (Table 6). Sadly, the correlation coefficient was only 0.5 and was not statistically significant (p = 0.253). The reason might be that few quantitative studies can be studied for correlation analysis. As a result, our assumption should be explored by more quantitative research in the future.
Gender differences in C-EMBU, TCI-144 and risk factors of online lending
Despite the fact that the Japanese version of EMBU differed from C-EMBU in some subscales, we found the following similarities: In contrast to females, males scored lower on the emotional warmth subscale and higher on the emotional rejection subscale (Someya et al., 2000). Besides, the parenting styles for males were more negative (rejection, punishment, and less communication) than that of females in our study. The reason might be that there are some parallels in child-rearing practices due to East Asian culture. However, it is crucial to note that the C-EMBU is different from the Japanese’s, and that there is little literature on gender differences in parenting styles among college students’ in China. Thus, more research needs to be done on our findings.
In line with other research in China and abroad, females in our study scored significantly higher on the HA than males. However, the findings for other subscales varied from different samples (Zohar et al., 2001; Chen et al., 2002; Shimizu and Ishikawa, 2011) and even were inconsistent with the research conducted in Beijing and the southwestern and northeastern regions of China, which also used TCI-144 as the assessment tool (Chen et al., 2013). Therefore, we speculate that the HA is mainly affected by biological factors, and the other subscales might be more related to sociocultural factors.
The results of the risk factors of online lending exhibited gender differences, which was in line with our hypothesis. The low score of HA was the risk factor for males only, and hedonism was solely for females (except for conformity and comparison). Thus, among many intervention measures based on qualitative research, our study showed that the effective ones were increasing education about the harm of online lending for males and ideological education about hedonism for females. Most notably, the variable of family members using online lending was the most important explanatory variable in the two models, consistent with data analyzed by neural networks. Furthermore, Bussmann and Giudici suggested that the Shapley-Lorenz-based approach was more accurate and explanatory than the above methods; therefore, it can be used in future risk management (Bussmann et al., 2020; Giudici and Raffinetti, 2021). To sum up, while intervening in students’ online lending, the community should actively intervene in their family members’ online lending.
Conclusion
We should intervene in online lending rapidly because it is expanding quickly over time. Moreover, the impact of family members using online lending on students and gender differences should also be taken into consideration.
Limitations
Here are some suggestions for future studies: first, our study’s generalizability was limited by the fact that it only included medical college students in Chengdu as a sample. We hope that the sample in future studies can be more diversified. In addition, the explanatory power of prediction variables to the utilization rate of online lending for males and females was 17.9 and 10.1%, respectively; thus, other risk factors (such as peer influence) need to be further studied.
Data availability statement
The raw data supporting the conclusions of this article will be made available by the authors, without undue reservation.
Ethics statement
The studies involving human participants were reviewed and approved by Chengdu Medical College. The patients/participants provided their written informed consent to participate in this study.
Author contributions
All authors listed have made a substantial, direct, and intellectual contribution to the work and approved it for publication.
Conflict of interest
The authors declare that the research was conducted in the absence of any commercial or financial relationships that could be construed as a potential conflict of interest.
Publisher’s note
All claims expressed in this article are solely those of the authors and do not necessarily represent those of their affiliated organizations, or those of the publisher, the editors and the reviewers. Any product that may be evaluated in this article, or claim that may be made by its manufacturer, is not guaranteed or endorsed by the publisher.
References
Amin, K. A., Ulbrich, T. R., Kirk, L. M., and Gothard, M. D. (2021). Investigating the impact of student loan debt on new practitioners. J. Am. Pharm. Assoc. 61, 191–197. doi: 10.1016/j.japh.2020.11.007
Bai, X., Wu, C., Rui, Z., and Ren, X. (2011). The psychometric evaluation of the satisfaction with life scale using a nationally representative sample of China. J. Happiness Stud. 12, 183–197. doi: 10.1007/s10902-010-9186-x
Bussmann, N., Giudici, P., Marinelli, D., and Papenbrock, J. (2020). Explainable AI in Fintech risk management. Front. Artific. Intellig. 3:26. doi: 10.3389/frai.2020.00026
Chang, A. (2018). A Questionnaire-Based Study on Campus Net Loan. M.A thesis. Nanning: Guangxi University.
Chen, W. J., Chen, H. M., Chen, C. C., Yu, W. Y., and Cheng, A. T. (2002). Cloninger’s tridimensional personality questionnaire: psychometric properties and construct validity in Taiwanese adults. Compr. Psychiatry 43, 158–166. doi: 10.1053/comp.2002.30797
Chen, Z., Lu, X., and Kitamura, T. (2013). The factor structure of the Chinese version of the temperament and character inventory: factorial robustness and association with age and gender. Compr. Psychiatry 54, 292–300. doi: 10.1016/j.comppsych.2012.08.003
Diener, E., Emmons, R. A., Larsen, R. J., and Griffin, S. (1985). The satisfaction with life scale. J. Pers. Assess. 49, 71–75. doi: 10.1207/s15327752jpa4901_13
Dong, X. (2017). Research on the Risk Prevention of College Students’ Bad Internet Lending from the Perspective of Collaborative Governance. M.A. thesis. Yunnan: Yunnan University of Finance and Economics.
Fu, X. L., Zhu, M., and Gao, F. (2019). Research on college students’ network loan and risk prevention-taking Changde vocational and technical college as an example. Theory Pract. Innovat. Enntrepreneursh. 12, 176–177.
Giudici, P., and Raffinetti, E. (2021). Explainable AI methods in cyber risk management. Qual. Reliab. Eng. 38, 1318–1326. doi: 10.1002/qre.2939
Gray, K., Kaji, A. H., Wolfe, M., Calhoun, K., and Virgilio, C. D. (2019). Influence of student loan debt on general surgery resident career and lifestyle decision-making. J. Am. Coll. Surg. 230, 173–181. doi: 10.1016/j.jamcollsurg.2019.10.016
Jette, D. U. (2016). Physical therapist student loan debt. Phys. Ther. 96, 1685–1688. doi: 10.2522/ptj.20160307
Li, J. Y. (2021). A study of current situation and influence of college students’ internet loan in Dongguan-A case study from City College of Dongguan University of Technology. Rural Econ. Sci. 34:3.
Ling, S., Robert, J., Rhys, P., Julia, S., Antonia, V., Webster, C. S., et al. (2018). Influence of student debt on health career location and specialty. J. Prim. Health Care 10, 54–61. doi: 10.1071/HC17052
Pan, H. A. (2020). Study of the Consumption of College Students’ Campus Online Loans-A Case Study on C University. M.A. thesis. Jilin: Changchun University of Technology.
Shi, H. Y., Deng, X. C., Wei, C., and Zhao, S. M. (2021). Survey and analysis of campus online loans. J. Shanxi Datong Univ. 37, 58–61.
Shimizu, T., and Ishikawa, M. (2011). Examining familiarity through the temperament and character inventory: a structural equation modeling analysis. Behaviormetrika 38, 139–151. doi: 10.2333/bhmk.38.139
Silva, A. D., Taveira, M., Marques, C., and Gouveia, V. V. (2014). Satisfaction with life scale among adolescents and young adults in Portugal: extending evidence of construct validity. Soc. Indic. Res. 120, 309–318. doi: 10.1007/s11205-014-0587-9
Someya, T., Uehara, T., Kadowaki, M., Tang, S. W., and Takahashi, S. (2000). Effects of gender difference and birth order on perceived parenting styles, measured by the EMBU scale, in Japanese two-sibling subjects. Psychiatry Clin. Neurosci. 54, 77–81. doi: 10.1046/j.1440-1819.2000.00640.x
Tang, X. J., and Tang, S. Q. (2020). Research on the causes and prevention of college students’ online loan in the perspective of ideological and political education. J. Hubei Normal Univ. 40, 93–97. doi: 10.3969/j.issn.2096-3130.2020.05.020
Xiao, X., Fu, C. J., Du, H. T., Li, A., and Su, J. (2018). Analysis on the current situation of college campus network loan and the exploration of the joint risk evadable mechanism of the colleges—taking Shandong Agricultural University as an example. J. Hubei Correspond. Univ. 31, 38–40.
Yang, J. J., Cui, X., Wu, P., Bao, T. H., and Yang, Y. (2019). Investigation and analysis on consumption status of medical students-taking medical students in Kunming as an example. Soft Sci. Health 33, 84–88. doi: 10.3969/j.issn.1003-2800.2019.11.018
Yue, D. M., Li, M. G., Jin, K. H., and Ding, B. K. (1993). Preliminary revision of EMBU and its application in neurotic patients J. Chin. Ment. Health J., 7:97-101, 143.
Zhang, Y. F. (2019). A study on the current situation and causes of online loans to college students born after 1995. J. Zaozhuang Univ. 36, 131–135.
Zhang, B. Y., Yang, Y. N., Yang, R., Yang, J. Y., Li, M. E., and Zhu, C. R. (2018). Research of college students’ usage of network loan and its influencing factors – A case study of Sichuan University. J. Educat. Modernizat. 5, 314–318.
Keywords: online lending, risk factors, gender difference, medical college students, intervention strategies
Citation: Zhang Y, Luo L, Li P, Xu Y and Chen Z (2023) Risk factors for college students’ online lending between different genders-A cross-sectional study in China. Front. Psychol. 14:965049. doi: 10.3389/fpsyg.2023.965049
Edited by:
Mohsen Saffari, Baqiyatallah University of Medical Sciences, IranReviewed by:
Vsevolod Konstantinov, Penza State University, RussiaPaolo Giudici, University of Pavia, Italy
Copyright © 2023 Zhang, Luo, Li, Xu and Chen. This is an open-access article distributed under the terms of the Creative Commons Attribution License (CC BY). The use, distribution or reproduction in other forums is permitted, provided the original author(s) and the copyright owner(s) are credited and that the original publication in this journal is cited, in accordance with accepted academic practice. No use, distribution or reproduction is permitted which does not comply with these terms.
*Correspondence: Zi Chen, ✉ enk4NjEyMjNAMTI2LmNvbQ==