- 1 Laboratory of Experimental Psychology, Department of Psychology, Katholieke Universiteit Leuven, Leuven, Belgium
- 2 Department of Psychology, Center for Cognitive Science, University of Turin, Turin, Italy
Adults show remarkable individual variation in the ability to detect felt enjoyment in smiles based on the Duchenne marker (Action Unit 6). It has been hypothesized that perceptual and attentional factors (possibly correlated to autistic-like personality traits in the normative range) play a major role in determining individual differences in recognition performance. Here, this hypothesis was tested in a sample of 100 young adults. Eye-tracking methodology was employed to assess patterns of visual attention during a smile recognition task. Results indicate that neither perceptual–attentional factors nor autistic-like personality traits contribute appreciably to individual differences in smile recognition.
Introduction
A smiling face does not always signal the experience of enjoyable emotions; people smile for many different reasons, for example to regulate conversation (Ekman, 2001), to mask other emotional states (e.g., anger or sadness), or to manipulate and deceive others (Ekman and Friesen, 1982; Ekman et al., 1988). The ability to discriminate between felt emotions and simulated emotional displays is essential to cope with the complexity of human social world; indeed, this ability starts developing early in childhood (Gross and Harris, 1988; Bugental et al., 1991; Gosselin et al., 2002a; Pons et al., 2004; Del Giudice and Colle, 2007) and is usually considered a key facet of emotional intelligence (Mayer and Salovey, 1993; Goleman, 1995).
Whereas some people are very good at recognizing felt and simulated expressions, others appear to lack this ability more or less completely. Normal adults usually perform at ceiling level when asked to distinguish between “basic” emotional expressions (see Ekman, 2001, 2006; Suzuki et al., 2006). However, when they are asked to judge whether a person is actually feeling a specific emotion, accuracy varies widely – ranging from 100% correct responses to chance-level performance (Ekman and O’Sullivan, 1991; Frank et al., 1993; Ekman et al., 1997; Frank and Ekman, 1997; Warren et al., 2009; see Ekman, 2003a for a review). What are the factors responsible for these striking individual differences? Several studies have been carried out to identify the cues used in detecting expression authenticity and to track the development of emotion recognition skills (e.g., Gosselin et al., 2002b; Ekman, 2003a,b; Chartrand and Gosselin, 2005; Del Giudice and Colle, 2007; Miles and Johnston, 2007). However, we still know very little about the factors accounting for individual variation. This is unfortunate, as a better understanding of individual differences would help clarify the cognitive processes underlying emotion recognition and shed light on their real impact in ecological contexts (for example, do people keep track of who is better at recognizing emotional expression? What are the social benefits or costs of being a skilled expression “reader”? Does emotion recognition ability covary with other cognitive and personality traits? And so on).
In the present study, we focus on individual differences in the ability to recognize felt and simulated enjoyment smiles (Ekman and Friesen, 1982). There are three main reasons for this choice. First, smile recognition is a crucial aspect of emotion recognition and has obvious implications for everyday social behavior. Second, smiling is among the most thoroughly investigated facial expressions, and there is ample evidence of consistent individual differences in recognition performance (Ekman, 2003a). Finally, a number of testable hypotheses on the origins of individual differences in smile recognition have been advanced in the literature and are thus amenable to empirical evaluation.
The Duchenne Marker
Felt and simulated smiles typically differ in several ways; for example, there are measurable differences in the symmetry, speed, timing, and synchrony of muscle activation (e.g., Ekman et al., 1981; Ekman and Friesen, 1982; Frank et al., 1993; Chartrand and Gosselin, 2005; Krumhuber and Kappas, 2005; Schmidt et al., 2006, 2009; Krumhuber et al., 2007). However, research in the past decades has focused overwhelmingly on a single morphological cue – the Duchenne marker – often considered as the most reliable, robust, and diagnostic cue of felt enjoyment (see Ekman, 2003b).
The Duchenne marker corresponds to Action Unit 6 (AU6) in the facial action coding system (FACS; Ekman et al., 2002), and is produced by the contraction of the external strand of the Orbicularis Oculi muscle; its main visible cues are the raising of the cheek, the lowering of the eye cover fold, the narrowing of the eye aperture, and the appearance of wrinkles (“crow’s feet”) on the external side of the eye. Ekman (2003b), Ekman and Friesen (1982) estimated that less of 10% of people have voluntary control of AU6, thus being able to simulate a credible enjoyment smile. Behavioral and psychophysiological studies converged in suggesting that AU6 is reliably associated with the experience of positive emotions in adults, children, and even young infants (Ekman et al., 1988, 1990; Fox and Davidson, 1988; Davidson et al., 1990; Surakka and Hietanen, 1998; Fogel et al., 2000; Messinger et al., 2001).
As concerns the production of facial expressions, recent evidence suggests that the hypothesis of a univocal association between Duchenne marker and felt enjoyment may be way too simplistic. Indeed, in contrast to Ekman’s (2003b) estimate, it has been shown that Duchenne smiles can often be elicited by asking people to pretend being happy (Krumhumer and Manstead, 2009). Furthermore, there is some evidence that Duchenne smiles can be displayed in situations eliciting negative emotions as well (e.g., Keltner and Bonanno, 1997; Papa and Bonanno, 2008).
However, there is also convincing evidence that the Duchenne marker is consistently used by untrained people to recognize felt and simulated enjoyment smiles (Frank et al., 1993; Williams et al., 2001; Miles and Johnston, 2006, 2007; Thibault et al., 2009). Naïve observers rate Duchenne smiles as more genuine compared to non-Duchenne smiles, even when Duchenne smiles are produced voluntarily (Krumhumer and Manstead, 2009). Furthermore, the Duchenne marker is the best predictor of performance when participants are asked to rate smile sincerity starting from static displays (e.g., Frank et al., 1993; Krumhumer and Manstead, 2009). Gaze patterns confirm the centrality of AU6: when people are asked to distinguish happiness from other facial expressions, they look at the mouth region most of the time (Williams et al., 2001; Leppänen and Hietanen, 2007), but they preferentially look at the eyes when the task concerns smile authenticity (Boraston et al., 2008). In particular, the focus of visual attention centers on the external side of the eye, where the most distinctive cues of AU6 activation are displayed (Williams et al., 2001).
Individual Differences in Smile Recognition
Studies of adults’ ability to discriminate between felt and simulated smiles routinely detect sizable individual differences. Differences are minimized only when adults engage in very simple tasks and performance is at ceiling level (e.g., Boraston et al., 2008). As tasks become more difficult (e.g., when the intensity of expressions varies, or when dynamic information is manipulated), substantial individual differences emerge. The weighted average accuracy in five studies employing complex smile recognition tasks (Frank et al., 1993; Gosselin et al., 2002a; Del Giudice and Colle, 2007; Boraston et al., 2008; McLennan et al., 2009) was 0.67, with a weighted SD = 0.17. Assuming normally distributed scores, one can make a rough estimate that accuracy ranges from 0.33 to 1.00 in 95% of participants, indicating large individual variation in performance.
Little is presently known about the causes of individual variation in smile recognition. Two main explanatory hypotheses involving perceptual and attentional factors have been advanced in the literature. First, it has been hypothesized that autistic-like personality traits should predict lower recognition ability (Dalton et al., 2007; Boraston et al., 2008); this effect would be mediated by decreased attention to the eye region of faces. Second, it has been proposed that other perceptual factors – such as the ability to discriminate between different AUs and the ability to detect discrepancy between the expression of eyes and that of the mouth – may be partly responsible for the observed individual differences in performance (Del Giudice and Colle, 2007).
Autism, Autistic-like Traits, and the Attentional Hypothesis
It is well known that people with autism show deficits in the recognition of emotional facial expressions (e.g., Hobson, 1986; Ozonoff et al., 1990; Capps et al., 1992; Celani et al., 1999; Adolphs et al., 2001). It has been proposed that recognition deficits depend on abnormal face processing, and more specifically on reduced exploration of the eye region of the face (Langdell, 1978; Klin et al., 1999; van der Geest et al., 2002). Indeed, it has been repeatedly shown that individuals with autism do not look at the eyes as often as controls when viewing face pictures (Pelphrey et al., 2002; Dalton et al., 2005; Neumann et al., 2006; Spezio et al., 2007) or movie clips of social interactions (Klin et al., 2002). Moreover, they often direct attention toward uninformative regions, such as the nose and chin (van der Geest et al., 2002; see Jemel et al., 2006 for a review). This atypical pattern of face exploration might explain why autistic patients show more severe impairments in the detection of fear – which relies heavily on information conveyed by the eyes – compared to other basic emotions (Howard et al., 2000; Pelphrey et al., 2002; Adolphs et al., 2005).
The ability to recognize the authenticity of smiles also depends strongly upon the information conveyed by the eye region. Recently, Boraston et al. (2008) confirmed that autistic patients are impaired in smile recognition, as predicted by the attentional hypothesis. They asked participants to discriminate felt from simulated smiles on the basis of AU6 involvement and recorded gaze patterns with eye-tracking methodology; autistic patients were less accurate in detecting smile authenticity and looked at the eye region significantly less than controls.
Autism and Asperger syndrome are diagnosable developmental disorders, but autistic-like personality traits are continuously distributed in the non-clinical population (Wing, 1988; Frith, 1991; Baron-Cohen, 1995; Le Couteur et al., 1996). They are higher in males, in scientists (especially in the hard sciences and engineering) and in relatives of autistic-spectrum patients (Baron-Cohen et al., 2001; Woodbury-Smith et al., 2005; Wheelwright et al., 2006; Dalton et al., 2007). Thus, individual differences in autistic-like traits may contribute to explain individual differences in smile recognition skills; people high in autistic-like traits may show reduced visual attention to the eyes, which in turn would limit their ability to detect felt enjoyment smiles (e.g., Knapp and Comadena, 1979; Ekman and O’Sullivan, 1991; Chartrand and Gosselin, 2005; Del Giudice and Colle, 2007). Consistent with this hypothesis, a study of face processing in subjects high in autistic-like traits (Dalton et al., 2007) showed that they made fewer fixations in the eye region compared to controls while looking at face pictures.
Other Perceptual Factors
Based on previous findings and new data from adults and children, Del Giudice and Colle (2007) hypothesized that individual differences in smile recognition may depend on three perceptual–attentional factors: attention to facial features, AU discrimination, and discrepancy detection. Attention to features refers to individual differences in the amount of attention devoted to the eye region vs. the mouth region of the face. AU discrimination refers to the ability to operate fine discriminations between similar action units in the eye region. In order to evaluate smile authenticity, people need to detect the cues of AU6 activation and to distinguish AU6 from similar action units under voluntary control. For example, AU7 is produced by the inner strand of the Orbicularis Oculi muscle, and it narrows the eye aperture similarly to AU6. Adults as a group (in contrast to children) are usually quite accurate in distinguishing between AU6 and AU7 (Del Giudice and Colle, 2007), but some adults may still be less accurate than others, and this may contribute to lower their performance on recognition tasks. The third perceptual factor is the ability to detect discrepancies between eye and mouth activation. Adults seem to compare eye and mouth activity and to perform a sort of “consistency check”: smiles displaying pronounced discrepancy (e.g., strong mouth activation but no eyes involvement) are more easily detected as simulated (Del Giudice and Colle, 2007). Differences in sensitivity to eye–mouth discrepancy may thus contribute to individual variation in recognition performance.
Aim of the Study
The hypothesis that perceptual–attentional factors play a major role in explaining individual differences in smile recognition has been advanced a number of times, and often implicitly assumed, but – to our knowledge – it has never been tested empirically. The present study employed eye-tracking methodology and tests of AU discrimination skills to investigate (1) to what extent perceptual and attentional factors explain individual differences in smile recognition; and (2) whether autistic-like personality traits in the non-clinical range predict individual differences in visual attention to different regions of the face.
Materials and Methods
Participants
One hundred twenty college undergraduates from the University of Turin took part in the study. In order to obtain a heterogeneous sample with respect to autistic-like personality traits (Baron-Cohen et al., 2001), we recruited students with diverse academic backgrounds, including physical and biological sciences, social sciences, and the humanities. Twenty participants were excluded from the study sample after administration, due to the loss of more that 20% of the their gaze fixations by the eye-tracker software (data loss may result from a variety of causes, including calibration problems due to the shape or color of participants’ eyes, excessive head movements, and so on). The final sample size was thus N = 100 (53 females, 47 males). Participant’s age ranged from 18 to 35 years (M = 23.1; SD = 2.9). All participants had normal or corrected-to-normal vision.
Materials and Procedure
Participants were tested individually by a trained experimenter. They were administered a smile recognition task, a perceptual discrimination task, and a paper-and-pencil questionnaire measuring autistic-like personality traits. During the smile recognition task, participants’ eye movements (scanpaths) were recorded by means of a remote eye-tracker. The order of task presentation was randomized, and the whole session lasted approximately 40–60 min.
The items of the smile recognition and perceptual discrimination tasks were presented on a 21″ monitor at a viewing distance of 70 cm. Pictures had a resolution of 1024 by 768 pixels, and subtended a vertical visual angle of 15° and a horizontal angle of 22°. They were displayed at the center of the screen against a white background with Presentation™ 9.3 software (Neurobehavioral Systems). Eye movements were recorded with an infrared remote camera (RED-III pan tilt) connected to an iViewX™ eye-tracking system (SensoMotoric Instruments). The system analyzed on-line monocular pupil and corneal reflection (50 Hz sampling rate). Before starting the task, the system was calibrated with nine predefined fixation points. Participants sat in front of a desk with a chin support minimizing head movements; room lights were dimmed for the duration of the task.
Tasks and Measures
Smile recognition
Smile recognition was assessed with the smiles picture set (SPS; Del Giudice and Colle, 2007). SPS items consist of 25 color pictures of an actor’s face displaying symmetrical smiles of different intensity, either with closed lips (AU12) or bared teeth (AU12 + AU25). The set contains 11 Duchenne smiles with AU6 activation and 14 non-Duchenne smiles (seven with AU7 activation and seven with a neutral eye region; see Figure 1A). Complete FACS codings of the SPS pictures can be found in Del Giudice and Colle (2007).
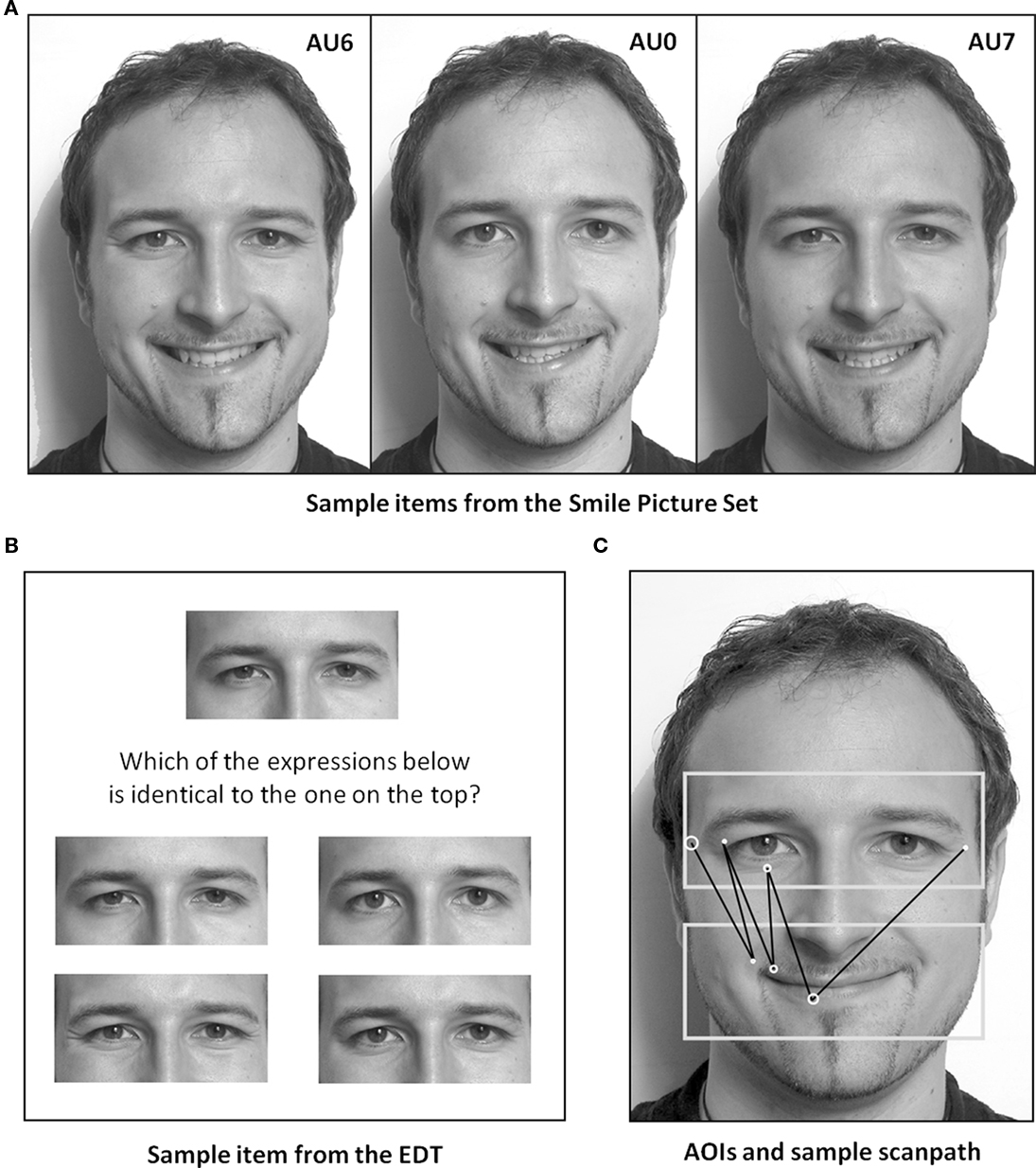
Figure 1. (A) Examples of the three smile types in the Smile Picture Set. From left to right: a Duchenne smile with AU6, a smile without eye region (AU0), and a smile with AU7. To facilitate comparison, the three items shown all involve bared-teeth smiles (AU12 + AU25) of similar intensity. See Del Giudice and Colle (2007) for complete Facial Action Coding System coding. (B) Example of scanpath in response to a Duchenne smile picture (AU6 + AU12). Light gray boxes indicate Areas of Interests (AOIs); circle size indicates fixation duration. (C) Sample item from the Eyes Discrimination Task. To prevent discrimination based on chromatic cues, the figure of the top was shown in color, while the four response alternatives were displayed in black and white.
The task started with a preliminary phase, in which four pictures of the same actor performing different expressions (anger, sadness, surprise, and disgust) were presented, each preceded by the picture of the actor’s neutral face. This phase gave participants the possibility to get accustomed to the actor’s face. Instructions were: “Now you are going to see this person smile. Each time, you will see a smile and then will be asked to tell if this person is really happy (left key) or just pretending to be happy (right key). If you are unable to decide, do not press any key.” The original instructions were in Italian; contento was the Italian word for “happy.” The 25 items were shown in one of two randomized orders; each item had a duration of 3 s and started with a neutral face (1 s) followed by the smiling face (2 s). After the smiling face, a 1-s fixation cross was displayed, followed by the question: “Do you think this person was really happy, or was he pretending to be happy?” Participants answered by pressing the corresponding key. The question was displayed for 5 s, and was followed by a 2-s fixation cross. During the task, participants did not receive feedback about their performance.
Each response was coded as correct (1 point) or incorrect (0 points). A correct response was scored for a “really happy” answer to items with AU6 activation, and for a “pretending to be happy” answer to items with AU7 activation or a neutral eye region. “Don’t know” answers were awarded 0 points; this option was include so as to minimize guessing. The number of correct answers was used as a measure of smile recognition ability, and ranged from 0 to 25. As in yes–no tasks the proportion of correct responses represents a biased measure (i.e., it does not consider systematic errors in performance), we also evaluated performance at the SPS by computing Signal Detection Theory parameters (Macmillan and Creelman, 2005). The proportion of hits and false alarms were used to calculate the location of criterion c (i.e., the general tendency to respond “really happy” or “pretending to be happy”; e.g., a value of zero indicates no bias) and sensitivity d″, an unbiased sensitivity index independent of the criterion the participant is adopting (e.g., a value of zero indicates complete inability to discriminate Duchenne smile trials from non-Duchenne smile trials, whereas larger values indicate correspondingly better discrimination).
Perceptual discrimination between eye-region AUs
In order to assess the ability to perceive subtle differences between different AUs in the eye region, we developed a novel task – the eyes discrimination task (EDT). The 12 items of the EDT were assembled from pictures of the eye region taken from the SPS pictures. In the EDT, participants were shown four alternative pictures of a person’s eyes (differing only in the type and intensity of activated AUs) and asked to select the one matching a target picture (see Figure 1B). Participants were asked to match the pictures as quickly as possible, and had to give their response within 6 s. Each response was coded as correct (1) or incorrect (0), yielding a total discrimination score ranging from 0 to 12. The EDT was designed to measure task-specific discrimination skills – that is, participants had to make the same type of perceptual distinctions on the eye region required by the smile recognition task. For this reason, the eye-region expressions shown in the EDT were taken directly from the items employed in the recognition task.
Visual attention
Eye-tracker data recorded during the smile recognition task were analyzed to assess participants’ patterns of visual attention to different regions of the face. Two identical rectangular areas of interest (AOIs) were drawn, one covering the eye region (the eyes and the lateral area where “crow’s feet” wrinkles appear) and another covering the mouth region (Figure 1C). Each AOI sustained an horizontal visual angle of 13° and a vertical visual angle of 4.5°. First, the overall duration of gaze fixations in each AOI was calculated. A fixation was recorded whenever the scanpath remained within 1° of visual angle for at least 100 ms. Following Merin et al. (2006), we calculated an eye–mouth index (EMI) for each participant by dividing the total amount of time spent looking at the eye region by the time spent looking at either the eyes or the mouth [EMI = Eye-region fixation time/(Eye-region fixation time + Mouth-region fixation time)]. A low EMI (less than 0.50) indicates that a participant gazes preferentially at the mouth region; a high EMI (greater than 0.50) indicates that a participant gazes preferentially at the eye region.
Discrepancy detection
The ability to detect discrepancies between the eye region and the mouth region is an important component of smile recognition. Our procedure did not allow us to obtain a direct measure of individual differences in discrepancy detection; however, we hypothesized that participants would respond to an incongruous facial expression by gazing back and forth between the eye region and the mouth region. Thus, the increase in the number of gaze shifts when looking at discrepant smiles would provide an indirect measure of discrepancy detection. We tested our hypothesis by comparing the average number of gaze shifts made in the three types of SPS items, from the least (AU6) to the most discrepant (AU7). A shift was scored when two consecutive fixations fell inside the two different AOIs. Repeated measures ANOVA showed a main effect of stimulus type [F(2,198) = 9.25, p < 0.001], with shift frequency increasing as predicted (AU6 < AU0 < AU7).
For each participant, we estimated the regression of the average number of shifts per item on item type (AU6 = −1, AU0 = 0, AU7 = +1). The standardized regression slope (beta) quantifies the increase in gaze shifts with increasing stimulus discrepancy, and serves as an indirect index of discrepancy detection. For example, beta = 0 means that there is no change in gaze shifts between the different types of smiles; positive betas indicate that the number of shifts increases from AU6 items to AU7 items, with larger values indicating a larger relative increase.
Autistic-like traits
Autistic-like traits were measured with the autism-spectrum quotient (AQ; Baron-Cohen et al., 2001; Italian version from the University of Cambridge Autism Research Centre website: http://www.autismresearchcentre.com). The AQ is a 50-item self-report measure of autistic-like traits in normal IQ individuals, and comprises five subscales: social skills, attention switch, attention to details, communication, and imagination. Whereas in the original AQ participants’ responses are dichotomized, we followed the recent literature (e.g., Hoekstra et al., 2008) and employed four-point scores in order to maximize scale reliability. Psychometric studies (Hoekstra et al., 2008; Hurst et al., 2009) suggest that the AQ can be adequately represented by two correlated factors: an attention to details factor and a so-called interpersonal factor (composed by the other four scales). We thus computed two summary scores corresponding to the two factors of the AQ: an attention to detail score (Cronbach’s α = 0.66) and an interpersonal score (α = 0.75).
Results
Descriptive Statistics
Consistent with previous literature findings, substantial individual variation in the ability to recognize smile authenticity was observed (see Table 1). The proportion of correct responses ranged from 0.44 to 0.92, with a mean of 0.70. To ascertain whether these data represented true individual differences in recognition ability rather than mere random noise, we compared the distribution of scores in our sample with the expected binomial distribution assuming a fixed success probability P = 0.70. The score distribution was significantly different from the one expected by chance [, p = 0.001]; also, the variance of the score distribution was larger than that of the corresponding binomial (variance ratio: 1.48), indicating that individual variability in recognition scores was higher than expected by chance alone.
Sensitivity (d′) ranged from −0.44 to 2.67, with a mean of 1.28 (see Table 1). Criterion c ranged from −0.64 to 1.66, with a mean of 0.06. The average criterion did not differ significantly from zero [t(99) = 1.65, p = 0.102], suggesting that participants did not show any systematic bias toward reporting a signal (happiness) or a no-signal (pretend happiness). Accordingly, no differences between false positive and false negative error rates were found [paired-sample t-test, t(99) = −1.78, p = 0.08].
The analysis of visual attention (EMI) showed that, on average, participants looked significantly longer at the eye region compared to the mouth region: the mean EMI was 0.65, and was significantly different from 0.50 [t(99) = 7.05, p < 0.001; see Table 1], i.e., the value indicating that gaze is equally distributed between the eye region and the mouth region. These results are consistent with the findings by Boraston et al. (2008) and confirm that, when evaluating smile authenticity, people rely preferentially on the information conveyed by the eyes. EMI scores at the different SPS item types were compared by performing repeated measures ANOVA. Results showed a significant effect of item type [F(2,98) = 8.23, p < 0.001]. Post hoc comparisons revealed that EMI was significantly lower in AU0 smiles (neutral eye region) compared to smiles with AU6 (p < 0.001) and AU7 (p = 0.007). No significant difference in EMI between AU6 and AU7 was found (p = 1.000). In other words, participants gazed more to the eye region in AU6 and AU7 conditions compared to the AU0 condition. This result suggest that participants were not sensitive to the eye-region per se, but to eye-region activation, and looked at the eyes only when information was present.
Autism-spectrum quotient scores were similar to those in normative samples (e.g., Hoekstra et al., 2008; Hurst et al., 2009). The attention to details score ranged from 12 to 36 (M = 25.3; SD = 4.7) and the interpersonal score ranged from 58 to 109 (M = 80.8; SD = 10.1). In contrast with previous findings, AQ scores did not significantly correlate with sex [Attention to details: r(98) = 0.062, p = 0.543; Interpersonal: r(98) = 0.007, p = 0.945]. However, it is important to note that our sample was not demographically representative, having been selected so as to maximize the variance in autistic-like traits in both sexes.
Correlation Analysis
A strong positive correlation was found between the two measures of performance at the SPS [raw accuracy and d′; r(98) = 0.94, p < 0.001]. This result confirms that accuracy can be considered a good measure of performance in our smile recognition task, given that no systematic response bias was found. In the SPS, criterion was negatively correlated with accuracy [r(98) = −0.20, p = 0.04] and d′ [r(98) = 0.30, p = 0.003].
Correlations between performance at the SPS and the other variables were small and non-significant (see Table 2), thus suggesting that none of the perceptual factors, nor autistic-like traits were able to predict individual differences in the ability to recognize smile authenticity. Correlations among the three perceptual factors (visual attention, AU discrimination, and discrepancy detection) were also small and non-significant. We found a significant negative correlation between discrepancy detection and the “attention to details” subscale of the AQ [r(98) = −0.21; p = 0.038]. This may reflect the local perceptual processing style by people scoring high on the AQ-detail scale; local processing and a focus on details would reduce the ability to detect global discrepancies in facial expressions.
Path Analysis
Correlation analysis suggested that none of the perceptual and personality variables we measured was able to reliably predict performance in smile recognition. We then proceeded to test the study hypotheses more formally, by fitting a series of path models to the data. The full model (with the hypothesized effects directions) is shown in Figure 2; it includes direct effects of the three perceptual variables (EDT, EMI, and beta), direct effects of autistic-like traits, indirect effects of autistic-like traits through visual attention, and the effect of sex on autistic-like traits. In addition to the full model, we tested a null model with no direct or indirect effects of any measured variable, and 11 alternative models representing different effect combinations (e.g., a non-zero effect of visual attention but no effects of autistic-like traits). Models were fitted to the covariance matrix and compared with the small-sample AIC statistic (AICc). Model fitting was carried out in R 2.8.0 (R Development Core Team, 2008) with the package sem 0.9-13 (Fox, 2008). Prior to model fitting, some variables (EDT, EMI, and beta) were log-transformed with the addition of appropriate constants in order to reduce skew.
Consistent with the results of correlation analysis, the best-fitting model in the set turned out to be the null one, i.e., the model in which none of the measured variables predicts performance in smile recognition (accuracy), either directly or indirectly [, RMSEA = 0, GOFI = 0.957].
Discussion
Felt and simulated enjoyment smiles differ with respect to several morphological cues, including the activation of a prominent action unit in the eye region (the Duchenne marker). This has led to the prediction that perceptual factors, and especially the allocation of attention to the eyes, would play a major role in explaining individual differences in the ability to recognize smile authenticity (Del Giudice and Colle, 2007). The results of the present study, however, provide evidence against this hypothesis. Participants were asked to discriminate Duchenne from non-Duchenne enjoyment smiles when looking at an actor’s smiling face. As in previous studies, large individual variation in discrimination accuracy was found, with performance ranging from 40% to over 90% correct responses. The average performance level (about 70% correct) suggests that, as a group, participants made use of perceptual information to detect smile authenticity; as predicted, they attended to the eye region more than to the mouth region (see Boraston et al., 2008), and were sensitive to discrepancies between the expression of the eyes and that of the mouth.
In contrast, perceptual factors did not appear to play any significant role in explaining individual differences in smile recognition. Taken together, the three perceptual mechanisms we considered – attention allocation, AU discrimination, and discrepancy detection – accounted for about 4% of variance in accuracy. A null result was also found concerning the role of autistic-like traits in the normative range in explaining individual differences in performance. Contrary to expectations, participants’ autistic-like traits did not predict their pattern of attention allocation, nor their performance in the smile recognition task.
This negative result is consistent with data recently collected by other research groups, both with normal participants and with autistic patients (Boraston et al., 2008; E. Heerey, personal communication, August 8, 2009). Indeed, Boraston et al. (2008) found that, as a group, autistic participants performed significantly worse on smile authenticity detection, and looked significantly less at the eye region compared to controls. However, performance in smile recognition did not correlate with the proportion of time spent looking at the eye region, neither in the autistic nor in the control group. While caution should be paid to extend results concerning autistic-like traits to autistic patients, the lack of any reliable association between autistic-like traits, attention allocation, and smile recognition may contribute to cast doubt on current explanations of emotion recognition deficits in autistic patients (see also Jones et al., 2011). In other words, it is possible that the association between impairments in emotion recognition and atypical patterns of attention to facial features in autistic patients does not reflect a true causal pathway (Boraston et al., 2008). Although absence of evidence is not evidence of absence, and null findings from a single study cannot be taken as proof that an effect does not exist, our study does suggest that the explanatory power of perceptual–attentional factors and autistic-like traits may have been overestimated in the emotion recognition literature.
Limitations of the Present Study
Our study has some limitations, which may have contributed to underestimate the role of perceptual–attentional factors and autistic-like traits in smile recognition. First of all, our smile recognition task was based on the manipulation of a single perceptual cue – the Duchenne marker. Recent evidence indicates that symmetry and dynamic features can be even better predictors of participants’ ability to assess smile sincerity, especially when dynamic facial displays are evaluated (e.g., Krumhumer and Manstead, 2009). Thus it is possible that, using a different smile recognition task, perceptual factors and autistic-like traits would be able to explain a greater amount of inter-individual variation. Second, discrepancy detection was measured only indirectly. Given that discrepancy detection seems to be especially critical in adults (Del Giudice and Colle, 2007), it would be important to assess this process in a more direct way before dismissing it as unimportant. Third, before concluding that autistic-like traits play no role in smile recognition and in the modulation of attention, it would be interesting to repeat the study in a population with higher autistic-like traits, such as the relatives of autistic patients (Dalton et al., 2007).
Future Research Directions
The present study provides evidence that perceptual–attentional factors and autistic-like traits play a minor role (if any) in explaining individual differences in smile recognition. What other factors could account for the remarkable amount of individual variation in this ability? Two promising candidates are emotion knowledge and simulation processes.
Chartrand and Gosselin (2005) suggested that perceptual cues are not sufficient to discriminate felt and simulated emotions; they argued that a major role is played by the meaning attributed to those cues, that is, by the semantic knowledge on emotions. It is well known that recognition of emotions from facial expressions is modulated by contextual information and emotional knowledge (e.g., Balconi and Pozzoli, 2005; Lindquist et al., 2006; Balconi and Lucchiari, 2007). For example, non-prototypical facial displays tend to be categorized as expressions of “basic emotions” when congruent contextual/semantic information is provided (Férnandez-Dols et al., 2008). Smile recognition could then depend heavily on contextual information, as well as on the observer’s previous knowledge concerning simulated emotions and their facial expression.
Niedenthal et al. (2010) recently proposed that smile recognition is the outcome of a processes of embodied simulation. Embodied simulation models posit that the conscious and non-conscious perception of emotional expressions automatically triggers the activation of the facial configuration associated with the observed emotion (mimicry), and induces the experience of the very same emotion in the observer (emotional contagion; see Goldman and Sripada, 2005; Tamietto et al., 2009; Tamietto and de Gelder, 2010). There is evidence that Duchenne and non-Duchenne smiles produce different facial reactions in the observer (Surakka and Hietanen, 1998); thus, it is plausible that automatic simulation plays a critical role in the detection of smile authenticity. Preliminary evidence collected in a recent mimicry study suggests that this, indeed, could be the case (Maringer et al., 2011).
Conclusion
We believe that our negative results, though not conclusive, represent an opportunity to rethink the role of perceptual factors in complex emotion recognition. Whereas the analysis of facial features is probably sufficient to discriminate between prototypical expressions in simple tasks, representing the subtle meaning of more complex displays may require the involvement of additional cognitive processes. Further research will be needed in order to investigate these processes, to elucidate when they are accurate and when they fail, and to discover how they relate to other aspects of cognition and personality.
Conflict of Interest Statement
The authors declare that the research was conducted in the absence of any commercial or financial relationships that could be construed as a potential conflict of interest.
Acknowledgments
This research was supported by a grant from San Paolo Foundation (San Paolo Foundation: ‘Action representations and their impairment’, 2010–2013).
References
Adolphs, R., Gosselin, F., Buchanan, T. W., Tranel, D., Schyns, P., and Damasio, A. R. (2005). A mechanism for impaired fear recognition after amygdala damage. Nature 433, 68–72.
Adolphs, R., Sears, L., and Piven, J. (2001). Abnormal processing of social information from faces in autism. J. Cogn. Neurosci. 13, 232–240.
Balconi, M., and Lucchiari, C. (2007). Consciousness and emotional facial expression recognition. J. Psychophysiol. 21, 100–108.
Balconi, M., and Pozzoli, U. (2005). Morphed facial expressions elicited a N400 ERP effect: a domain-specific semantic module? Scand. J. Psychol. 46, 467–474.
Baron-Cohen, S. (1995). Mindblindness: An Essay on Autism and Theory of Mind. Cambridge, MA: MIT Press.
Baron-Cohen, S., Wheelwright, S., Skinner, R., Martin, J., and Clubley, E. (2001). The autism-spectrum quotient (AQ): evidence from Asperger syndrome/high-functioning autism, males and females, scientists and mathematicians. J. Autism Dev. Disord. 31, 5–17.
Boraston, Z. L., Corden, B., Miles, L. K., Skuse, D. H., and Blakemore, S. J. (2008). Brief report: perception of genuine and posed smiles by individuals with autism. J. Autism Dev. Disord. 38, 574–580.
Bugental, D. B., Kopelkin, H., and Lazowski, L. (1991). “Children’s response to authentic versus polite smiles,” in Children’s Interpersonal Trust: Sensitivity to Lying, Deception, and Promise Violation, ed. K. J. Rotenberg (New York: Springer-Verlag), 59–79.
Capps, L., Yirmiya, N., and Sigman, M. (1992). Understanding of simple and complex emotions in non-retarded children with autism. J. Child Psychol. Psychiatry 33, 1169–1182.
Celani, G., Battacchi, M. W., and Arcidiacono, L. (1999). The understanding of the emotional meaning of facial expressions in people with autism. J. Autism Dev. Disord. 29, 57–66.
Chartrand, J., and Gosselin, P. (2005). Judgement of authenticity of smiles and detection of facial indexes. Can. J. Exp. Psychol. 59, 179–189.
Dalton, K. M., Nacewicz, B. M., Alexander, A. L., and Davidson, R. J. (2007). Gaze-fixation, brain activation, and amygdala volume in unaffected siblings of individuals with autism. Biol. Psychiatry 61, 512–520.
Dalton, K. M., Nacewicz, B. M., Johnstone, T., Schaefer, H. S., Gernsbacher, M. A., Goldsmith, H. H., Alexander, A. L., and Davidson, R. J. (2005). Gaze fixation and the neural circuitry of face processing in autism. Nat. Neurosci. 8, 519–526.
Davidson, R. J., Ekman, P., Saron, C., Senulius, J., and Friesen, W. J. (1990). Emotional expression and brain physiology. I: approach/withdrawal and cerebral asymmetry. J. Pers. Soc. Psychol. 58, 330–341.
Del Giudice, M., and Colle, L. (2007). Differences between children and adults in the recognition of enjoyment smiles. Dev. Psychol. 43, 796–803.
Ekman, P. (2001). Telling Lies: Clues to Deceit in the Marketplace, Politics, and Marriage. New York: W. W. Norton.
Ekman, P. (2006). Darwin and Facial Expression: A Century of Research in Review. Cambridge, MA: Malor Books.
Ekman, P., Davidson, R. J., and Friesen, W. V. (1990). The Duchenne smile: emotional expression and brain physiology II. J. Pers. Soc. Psychol. 58, 342–353.
Ekman, P., and Friesen, W. V. (1982). Felt, false, and miserable smiles. J. Nonverbal Behav. 6, 238–252.
Ekman, P., Friesen, W. V., and Hager, J. C. (2002). Facial Action Coding System. Salt Lake City, UT: A Human Face.
Ekman, P., Friesen, W. V., and O’Sullivan, M. (1988). Smiles when lying. J. Pers. Soc. Psychol. 54, 414–420.
Ekman, P., Friesen, W. V., and O’Sullivan, M. (1997). “Smiles when lying,” in What the Face Reveals. Basic and Applied Studies of Spontaneous Expression Using the Facial Action Coding System (FACS), eds P. Ekman and E. L. Rosenberg (Oxford: Oxford University Press), 201–214.
Ekman, P., Hager, J. C., and Friesen, W. V. (1981). The symmetry of emotional and deliberate facial actions. Psychophysiology 18, 101–106.
Férnandez-Dols, J. M., Carrera, P., Barchard, K. A., and Gacitua, M. (2008). False recognition of facial expressions of emotion: causes and implications. Emotion 8, 530–539.
Fogel, A., Nelson-Goens, G. C., and Hsu, H. C. (2000). Do different infant smiles reflect different positive emotions? Soc. Dev. 9, 497–520.
Fox, J. (2008). The SEM Package Version 0.9-13. Available at: http://cran.r-project.org/
Fox, N., and Davidson, R. J. (1988). Patterns of brain electrical activity during facial signs of emotion in 10-month-old infants. Dev. Psychol. 24, 230–236.
Frank, M. G., and Ekman, P. (1997). The ability to detect deceit generalizes across different types of high-stake lies. J. Pers. Soc. Psychol. 72, 1429–1439.
Frank, M. G., Ekman, P., and Friesen, W. V. (1993). Behavioral markers and recognizability of the smile of enjoyment. J. Pers. Soc. Psychol. 64, 83–93.
Goldman, A. I., and Sripada, C. S. (2005). Simulationist models of faced-based emotion recognition. Cognition 94, 193–213.
Gosselin, P., Perron, M., Legault, M., and Campanella, P. (2002a). Children’s and adults’ knowledge of the distinction between enjoyment and non-enjoyment smiles. J. Nonverbal Behav. 26, 83–108.
Gosselin, P., Warren, M., and Diotte, M. (2002b). Motivation to hide emotion and children’s understanding of the distinction between real and apparent emotions. J. Genet. Psychol. 163, 479–495.
Gross, D., and Harris, P. L. (1988). False beliefs about emotion: children’s understanding of misleading emotional displays. Int. J. Behav. Dev. 11, 475–488.
Hobson, R. P. (1986). The autistic child’s appraisal of expressions of emotion. J. Child Psychol. Psychiatry 27, 321–342.
Hoekstra, R. A., Bartels, M., Cath, D. C., and Boomsma, D. I. (2008). Factor structure, reliability and criterion validity of the autism-spectrum quotient (AQ): a study in Dutch population and patient groups. J. Autism Dev. Disord. 38, 1555–1566.
Howard, M. A., Cowell, P. E., Boucher, J., Broks, P., Mayes, A., Farrant, A., and Roberts, N. (2000). Convergent neuroanatomical and behavioral evidence of an amygdala hypothesis of autism. Neuroreport 11, 2931–2935.
Hurst, R. M., Nelson-Gray, R. O., Mitchell, J. T., and Kwapil, T. R. (2009). The relationship of Asperger’s characteristics and schizotypal personality traits in a non-clinical adult sample. J. Autism Dev. Disord. 37, 1711–1720.
Jemel, B., Mottron, L., and Dawson, M. (2006). Impaired face processing in autism: fact or artifact? J. Autism Dev. Disord. 6, 1–16.
Jones, C. R. G., Pickles, A., Falcaro, M., Marsden, A. J. S., Happé, F., Scott, S. K., Sauter, D., Tregay, J., Phillips, R. J., Baird, G., Simonoff, E., and Charman, T. (2011). A multimodal approach to emotion recognition ability in autism spectrum disorders. J. Child Psychol. Psychiatry 52, 275–285.
Keltner, D., and Bonanno, G. A. (1997). A study of laughter and dissociation: distinct correlates of laughter and smiling during bereavement. J. Pers. Soc. Psychol. 73, 687–702.
Klin, A., Jones, W., Schultz, R., Volkmar, F., and Cohen, D. (2002). Visual fixation patterns during viewing of naturalistic social situations as predictors of social competence in individuals with autism. Arch. Gen. Psychiatry 59, 809–816.
Klin, A., Sparrow, S. S., de Bildt, A., Cicchetti, D. V., Cohen, D. J., and Volkmar, F. R. (1999). A normed study of face recognition in autism and related disorders. J. Autism Dev. Disord. 29, 499–508.
Knapp, M. L., and Comadena, M. E. (1979). Telling it like it isn’t: a review of theory and research on deceptive communications. Hum. Commun. Res. 5, 270–285.
Krumhuber, E., and Kappas, A. (2005). Moving smiles: the role of dynamic components for the perception of the genuineness of smiles. J. Nonverbal Behav. 29, 3–24.
Krumhuber, E., Manstead, A. S. R., and Kappas, A. (2007). Temporal aspects of facial displays in person and expression perception: the effects of Smile dynamics, head-tilt, and gender. J. Nonverbal Behav. 31, 39–56.
Krumhumer, E., and Manstead, A. (2009). Can Duchenne smiles be feigned? New evidence on felt and false smiles. Emotion 6, 807–820.
Langdell, T. (1978). Recognition of faces: an approach to the study of autism. J. Child Psychol. Psychiatry 19, 255–268.
Le Couteur, A., Bailey, A., Goode, S., Pickles, A., Robertson, S., Gottesman, I., and Rutter, M. (1996). A broader phenotype of autism: the clinical spectrum in twins. J. Child Psychol. Psychiatry 37, 785–801.
Leppänen, J. M., and Hietanen, J. K. (2007). Is there more in a happy face than just a big smile? Vis. Cogn. 15, 468–490.
Lindquist, K. A., Feldman-Barrett, L., Bliss-Moreau, E., and Russell, J. A. (2006). Language and the perception of emotion. Emotion 6, 125–138.
Macmillan, N. A., and Creelman, C. D. (2005). Detection Theory: A User’s Guide, 2nd Edn, Mahwah, NJ: Lawrence Erlbaum Associates.
Maringer, M., Krumhuber, E. G., Fischer, A. H., and Niedenthal, P. M. (2011). Beyond smile dynamics: mimicry and beliefs in judgments of smiles. Emotion 11, 181–187.
Mayer, J. D., and Salovey, P. (1993). The intelligence of emotional intelligence. Intelligence 17, 433–442.
McLennan, T., Johnston, L., Dalrymple-Alford, J., and Porter, R. (2009). Sensitivity to genuine versus posed emotion specified in facial displays. Cogn. Emot. 24, 1277–1292.
Merin, N., Young, G. S., Ozonoff, S., and Rogers, S. J. (2006). Visual fixation patterns during reciprocal social interaction distinguish a subgroup of 6-month-old infants at risk for autism from comparison infants. J. Autism Dev. Disord. 37, 108–121.
Messinger, D. S., Fogel, A., and Dickson, K. L. (2001). All smiles are positive, but some smiles are more positive than others. Dev. Psychol. 37, 642–653.
Miles, L., and Johnston, L. (2006). “Not all smiles are created equal: perceiver sensitivity to the differences between posed and genuine smiles,” in Cognition and Language: Perspectives from New Zealand, eds G. Haberman, and C. Flethcher-Flinn (Bowen Hills: Australian Academic Press), 51–64.
Miles, L., and Johnston, L. (2007). Detecting happiness: perceiver sensitivity to enjoyment and non-enjoyment smiles. J. Nonverbal Behav. 31, 259–275.
Neumann, D., Spezio, M. L., Piven, J., and Adolphs, R. (2006). Looking you in the mouth: abnormal gaze in autism resulting from impaired top-down modulation of visual attention. Soc. Cogn. Affect. Neurosci. 1, 194–202.
Niedenthal, P. M., Mermillod, M., Maringer, M., and Hess, U. (2010). The simulation of smiles (SIMS) model: embodied simulation and the meaning of facial expression. Behav. Brain Sci. 33, 417–433.
Ozonoff, S., Pennington, B. F., and Rogers, S. J. (1990). Are there emotional perception deficits in young autistic children? J. Child Psychol. Psychiatry 31, 343–361.
Papa, A., and Bonanno, G. A. (2008). Smiling in the face of adversity: the interpersonal and intrapersonal functions of smiling. Emotion 8, 1–12.
Pelphrey, K. A., Sasson, N. J., Reznick, J. S., Paul, G., Goldman, B. D., and Piven, J. (2002). Visual scanning of faces in autism. J. Autism Dev. Disord. 32, 249–261.
Pons, F., Harris, P. L., and de Rosnay, M. (2004). Emotion comprehension between 3 and 11 years: developmental periods and hierarchical organization. Eur. J. Dev. Psychol. 1, 127–152.
R Development Core Team. (2008). R: A Language and Environment for Statistical Computing. Vienna: R Foundation for Statistical Computing.
Schmidt, K. L., Ambadar, Z., Cohn, J. F., and Reed, I. (2006). Movement differences between deliberate and spontaneous facial expressions: zygomaticus major action in smiling. J. Nonverbal Behav. 30, 37–52.
Schmidt, K. L., Bhattacharya, S., and Denlinger, R. (2009). Comparison of deliberate and spontaneous facial movement in smiles and eyebrow raises. J. Nonverbal Behav. 33, 35–45.
Spezio, M. L., Adolphs, R., Hurley, R. S., and Piven, J. (2007). Abnormal use of facial information in high-functioning autism. J. Autism Dev. Disord. 37, 929–939.
Surakka, V., and Hietanen, J. K. (1998). Facial and emotional reactions to Duchenne and non-Duchenne smiles. Int. J. Psychophysiol. 29, 23–33.
Suzuki, A., Hoshino, T., and Shigemasu, K. (2006). Measuring individual differences in sensitivities to basic emotions in faces. Cognition 99, 327–353.
Tamietto, M., Castelli, L., Vighetti, S., Perozzo, P., Geminiani, G., Weiskrantz, L., and de Gelder, B. (2009). Unseen facial and bodily expressions trigger fast emotional reactions. Proc. Natl. Acad. Sci. U.S.A. 106, 17661–17666.
Tamietto, M., and de Gelder, B. (2010). Neural bases of the non-conscious perception of emotional signals. Nat. Rev. Neurosci. 11, 697–709.
Thibault, P., Gosselin, P., Brunel, M., and Hess, U. (2009). Children’s and adolescents’ perception of the authenticity of smiles. J. Exp. Child. Psychol. 102, 360–367.
van der Geest, J. N., Kemner, C., Verbaten, M. N., and van Engeland, H. (2002). Gaze behavior of children with pervasive developmental disorder toward human faces: a fixation time study. J. Child Psychol. Psychiatry 43, 1–11.
Warren, G., Schertler, E., and Bull, P. (2009). Detecting deception from emotional and unemotional cues. J. Nonverbal Behav. 33, 59–69.
Wheelwright, S., Baron-Cohen, S., Goldenfeld, N., Delaney, J., Fine, D., Smith, R., Weil, L., and Wakabayashi, A. (2006). Predicting autism spectrum quotient (AQ) from the systemizing quotient-revised (SQ-R) and empathy quotient (EQ). Brain Res. 1079, 47–56.
Williams, L. M., Senior, C., David, A., Loughland, C. M., and Gordon, E. (2001). In search of the “Duchenne Smile”? Evidence from eye movements. J. Psychophysiol. 15, 122–127.
Wing, L. (ed.), (1988). Aspects of Autism: Biological Research. London: Gaskell/Royal College of Psychiatrists.
Keywords: attention, autistic-like traits, Duchenne marker, eye-tracker, facial expressions, FACS, individual differences, smiling
Citation: Manera V, Del Giudice M, Grandi E and Colle L (2011) Individual differences in the recognition of enjoyment smiles: no role for perceptual–attentional factors and autistic-like traits. Front. Psychology 2:143. doi: 10.3389/fpsyg.2011.00143
Received: 11 February 2011;
Paper pending published: 18 May 2011;
Accepted: 14 June 2011;
Published online: 07 July 2011.
Edited by:
Marco Tamietto, Tilburg University, NetherlandsReviewed by:
Marco Tamietto, Tilburg University, NetherlandsLynden Miles, University of Aberdeen, UK
Copyright: © 2011 Manera, Del Giudice, Grandi and Colle. This is an open-access article subject to a non-exclusive license between the authors and Frontiers Media SA, which permits use, distribution and reproduction in other forums, provided the original authors and source are credited and other Frontiers conditions are complied with.
*Correspondence: Valeria Manera, Laboratory of Experimental Psychology, Katholieke Universiteit Leuven, Leuven, Belgium. e-mail: valeria.manera@unito.it