- Centre for Cognitive Neuroimaging, Institute of Neuroscience and Psychology, University of Glasgow, Glasgow, UK
The notion of driving brain oscillations by directly stimulating neuronal elements with rhythmic stimulation protocols has become increasingly popular in research on brain rhythms. Induction of brain oscillations in a controlled and functionally meaningful way would likely prove highly beneficial for the study of brain oscillations, and their therapeutic control. We here review conventional and new non-invasive brain stimulation protocols as to their suitability for controlled intervention into human brain oscillations. We focus on one such type of intervention, the direct entrainment of brain oscillations by a periodic external drive. We review highlights of the literature on entraining brain rhythms linked to perception and attention, and point out controversies. Behaviourally, such entrainment seems to alter specific aspects of perception depending on the frequency of stimulation, informing models on the functional role of oscillatory activity. This indicates that human brain oscillations and function may be promoted in a controlled way by focal entrainment, with great potential for probing into brain oscillations and their causal role.
Introduction
Physiological rhythms are ubiquitous in living organisms (e.g., Glass, 2001). In the brain, oscillations are supported by distinct neuronal elements across different spatial scales (e.g., Buzsáki and Draguhn, 2004). These elements range from the single neuron (Hutcheon and Yarom, 2000) over neuronal circuits (e.g., Whittington et al., 1997) to thalamo-cortical and cortico-cortical networks at the macroscopic level (e.g., Lorincz et al., 2009). Furthermore, brain oscillations occur in distinct frequency bands as a function of micro- and macro-network architecture (Buzsáki and Draguhn, 2004). Some aspects of brain activity can therefore be described in a simplified model as a system of hierarchically stacked neuronal oscillators, that cycle and interact at different frequencies (Buzsáki and Draguhn, 2004). But are these brain rhythms causally implicated in brain function, or do they merely reflect by-products of other, underlying mechanisms?
One of the computational processes that oscillations likely enable is the dynamic routing/gating of information through the synchronization of neuronal elements (Salinas and Sejnowski, 2001; Fries, 2005; Jensen and Mazaheri, 2010). From such a network perspective, lower frequency oscillations in the theta- to alpha-bands (4–12 Hz) have been related to long-distance, area-to-area interactions, and higher frequency gamma-oscillations (20–100 Hz) to local neuronal communication (von Stein et al., 2000), coordinated in a multi-band neuronal workspace through cross-frequency interactions (e.g., Palva and Palva, 2007). Perception also relies on processes that enable effective selection and integration of relevant information from the vast amount of sensory inputs that is constantly bombarding our brain. In support of the hypothesis that differences in frequencies across structures may provide important clues to these functions, posterior gamma-oscillations (30–100 Hz, of likely intracortical origin) have been assigned roles in feature binding (Singer and Gray, 1995), and posterior alpha-band oscillations (8–14 Hz, of thalamo-cortical origin) in input regulation (Lorincz et al., 2009), and attentional selection (Worden et al., 2000; Sauseng et al., 2005; Kelly et al., 2006; Thut et al., 2006).
System characteristics therefore endow neuronal elements or networks to oscillate at specific frequencies, and these oscillations may implement specific processes. This raises an interesting set of questions. Can we promote oscillations in distinct, neuronal elements in vivo by directly stimulating them (e.g., through transcranial stimulation protocols)? If so, to what extent would this impact brain function? One prerequisite would be the availability of techniques that allow for non-invasive human brain stimulation in a sufficiently controlled and focal manner to drive distinct oscillators. But do these techniques exist, and at what spatial scales do they operate? We here cover these questions, by reviewing recent findings on the interaction of non-invasive human brain stimulation with human brain rhythms.
General Concepts and Formal Models of Entrainment by a Periodic External Drive
One property of oscillating elements commonly found in nature is that they are self-sustained and dynamic (e.g., Glass, 2001; Pikovsky et al., 2003). Such oscillators can be perturbed by an external force. If this force is periodic, the natural oscillation may then become synchronized to the periodic event. Synchronization here means that the oscillating element starts to cycle with the same period as the external force. Or in other terms, the oscillator becomes entrained or locked to the external event. In this context, synchronization, entrainment, and locking are synonymous1 (Pikovsky et al., 2003). We will use the term entrainment to denote synchronization to a rhythmic stream (or train) of external events.
For a better illustration of these concepts, we present one possible, simple model of entrainment in Box 1. This simulates a population of single, self-sustained oscillating elements (here n = 3) that become synchronized to an external, rhythmic pulse train (Box 1; Figures 1A,B). A single oscillator in the simulation could correspond to a small oscillating neuronal assembly. Electro- or magnetoencephalography (EEG/MEG) capture the sum of many single oscillating assemblies. This sum (see Box 1; Figure 1C) illustrates the two characteristics of entrainment at the population level: (i) adjustment of phase of the underlying oscillation to the periodic force, and (ii) increase in amplitude. In other terms, as more and more single oscillating elements (e.g., neurons) start to cycle with the periodic force, this translates into phase alignment and amplitude-increase at the population level. Entrainment therefore is about driving brain oscillations by external forces – rather than disruption of oscillations by these forces.
Box 1. Entrainment in a population model of self-sustained neuronal oscillators.
The inherent anatomical and functional complexity of even small neuronal networks calls for simplified models that preserve important aspects of their functional characteristics. Computational neuroscientists in this area have carried out a significant amount of research and we refer the interested reader to the specialized literature (Glass, 2001; Tass, 2002; Pikovsky et al., 2003; Izhikevich, 2010; Winfree, 2001).
For the purpose of this review, we present a very simple simulation of entrainment. To this end, we use a simple phase oscillator model (Winfree, 2001; Tass, 2002). The dynamics of this model can be described conveniently as a vector rotating counter-clockwise along the unit circle in the complex plane (Figure 1A, left panel). In the absence of stimulation, the vector cycles with a constant rate around the circle depending on the frequency of the oscillation. The sinusoid (Figure 1A, right panel) describes the position of the oscillation with respect to the 360° rotation of one full cycle, i.e., with respect to the phase (=φ, 0°–360°) over time.
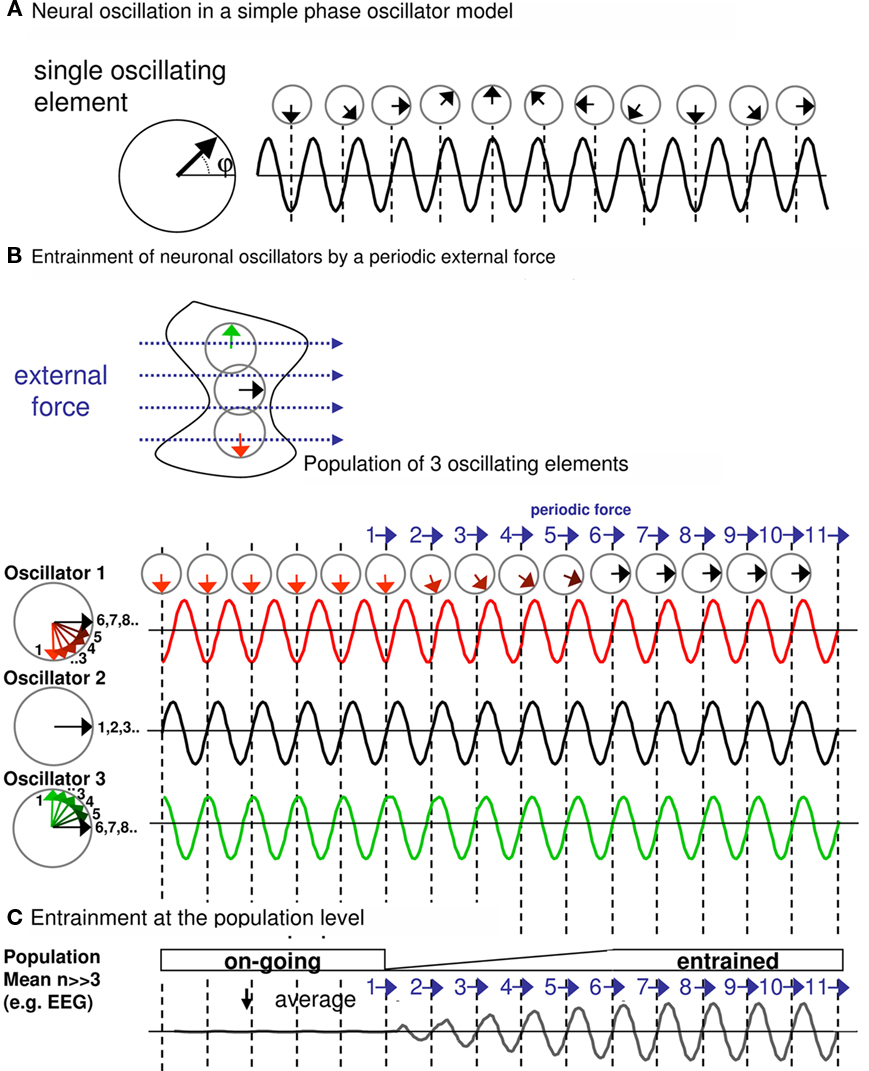
Figure 1. (A) Neural oscillation in a simple phase oscillator model. (B) Entrainment of neuronal oscillators by a periodic external force. (C) Entrainment at the population level.
Rhythmic stimulation of the oscillating neural population can be modeled as a periodic force acting in a certain direction on the phase vector (Pikovsky et al., 2003). The effect of such rhythmic stimulation on the ongoing oscillation depends on several parameters: the amplitude of the stimulation (relative to the amplitude of the ongoing oscillation), the phase of the stimulation (relative to the phase of the ongoing oscillation), the duration of stimulation (or number of pulses in the case of pulsed rhythmic stimulation), and the frequency of the stimulation (relative to the frequency of the ongoing neural oscillation). The number of relevant parameters already suggests that a number of possible scenarios have to be considered as outcomes of rhythmic stimulation. In our simulation, we focus on the special case where the stimulation frequency is identical to the frequency of the neural oscillation, i.e., where the rhythmic stimulus is frequency-tuned to the to-be-entrained oscillation. This is the most relevant scenario for controlled interaction with the underlying oscillator, as representing ideal condition for entrainment (for the outcome of more complex scenarios, see, e.g., Glass, 2001; Pikovsky et al., 2003).
Figure 1B illustrates the case of a periodic stimulation with a directional external force (blue vector) when this is repeated (here 11 times). The effects of the force are examined for three oscillators (oscillator 1–3) forming a neuronal population. All considered oscillators cycle at the same frequency (giving rise to the fundamental frequency of the population) but not necessarily at the same phase. Accordingly, the effects of the force differ for each oscillator, depending on the phase at which the force catches the ongoing oscillation. Consider the black oscillator (oscillator 2), the periodic force and the neural oscillation are already in phase. The force (pulling to the right side) catches the oscillation when its phase is already pointing to the right, and therefore, the phase vector at time of the force is unaffected (see left unit circle) and the sinusoid is unperturbed. Consequently, there is no effect (i.e., change in phase) on the neural oscillation. A different scenario characterizes the response of the red oscillator (oscillator 1). Initially, the ongoing oscillation is not phase-aligned with the periodic force. The phase-vector representation shows that each periodic force causally advances the phase vector slightly, until (here, after the sixth stimulation) the neural oscillation phase-aligns with the periodic stimulation. Similarly, the green oscillator (oscillator 3) moves back in phase with each successive stimulation until both are aligned.
On the level of the population of oscillators, as recordable with EEG/MEG (Figure 1C, Population mean, number of modeled oscillators >> 3), this would translate not only in phase-adjustment but also in amplitude-increase. Prior to stimulation with the periodic force, a certain number of oscillators of this population are likely to show random phase relations, which tends to nullify the population sum. After the starting point of periodic stimulation and as more and more underlying oscillators become synchronized, progressive amplitude-enhancement through phase alignment is observed (entrainment).
Note that we present here one possible model of entrainment mostly for illustrative purposes. There are other scenarios, such as the external forces directly triggering an oscillation, i.e., enhancing the amplitude per se. This would lead to the same outcome, namely phase-aligned and amplitude-increased oscillations, in case the periodic force is frequency-locked to the to-be-induced oscillation.
If we could drive oscillations of distinct neuronal elements in a controlled manner as modeled above, how could this then impact perception and performance? Provided that these oscillations play a causal role for a specific cognitive function, it is conceivable that their entrainment has a functional impact. Phase-adjustment of distinct oscillating neuronal elements, on the one hand, could change selective communication within constituents of the entrained population, or with distant neuronal elements cycling at the same phase, since an oscillatory input has a stronger effect on those populations that are tuned to the input frequency (Fries, 2005; Schnitzler and Gross, 2005). Enhancing amplitude of distinct neuronal oscillators, on the other hand, may be used to reproduce functionally relevant oscillatory signatures, mimicking task-related modulation of oscillations, and thereby benefit functions (e.g., Thut and Miniussi, 2009).
Controlled entrainment of brain rhythms may therefore prove highly beneficial for the study of human brain oscillation. First, by generating oscillatory signatures through entrainment, we would be able to study in detail the behavioral consequences of these modulations, thereby increasing our understanding of the role of oscillations for behavior. Second, EEG-recording during controlled experimental induction of oscillations would enable the detailed study of the system’s behavior under various scenarios. Third, further research in this area may well lead to applications in rehabilitation or treatment of disease.
We here review emerging evidence for entrainment of human brain oscillations in a controlled and functionally meaningful way. We also highlight challenges in the quest for entrainment. In light of these challenges, we argue that providing converging evidence for entrainment on both the level of EEG-signals and behavioral measures will likely prove essential to disambiguate entrainment from other forms of interactions between rhythmic brain stimulation and brain rhythms.
Entrainment of Human Brain Oscillations: Main Confound
The models of entrainment by external driving introduced above translate into a set of requirements for entrainment.
(1) Entrainment requires the involvement of a neural oscillator (i.e., a neural population that exhibits oscillations at the entrainment frequency or is capable of doing so under natural conditions).
(2) Entrainment requires periodicity in the input stream of external events.
(3) Entrainment requires synchronization (phase alignment) between the input stream and the neural oscillator.
(4) Crucially, the models also assume that the external force influences the oscillating elements by direct interaction (see Box 1; Figure 1B).
The ideal scenario to achieve this form of entrainment is to tune the frequency of the periodic force to the natural frequency of the to-be-entrained neuronal elements (which is the scenario illustrated in Box 1). This is equivalent to a 1:1 frequency locking between the natural oscillation and the periodic event. Under these conditions, entrainment of neuronal oscillations is feasible in vitro (Gouwens et al., 2010) and in vivo in rats (Ozen et al., 2010). But is it also feasible in the human brain with the currently available stimulation techniques, and what are its confounding factors?
In contrast to the study of entrainment in slice preparation of neurons (e.g., Gouwens et al., 2010), there is an important confound of entrainment to external rhythmic events in humans, even if the external force directly interacts with the neuronal elements (as modeled). Unless the external force is imperceptible, the periodicity of the external event will likely engage attentional mechanisms, such as reflexive orienting or anticipation of the next, predicable event in the event sequence (Nobre, 2001). Because these processes are also likely to be associated with changes to oscillatory activity, they may themselves cause synchronization of brain waves to the input stream, as well as changes to perception and performance (for the case of EEG-changes in anticipation of upcoming events see, e.g., Worden et al., 2000; Sauseng et al., 2005; Thut et al., 2006). In the wider sense, this would also reflect entrainment of functionally relevant oscillations to periodic events, but through indirect (internal) control, and not through direct impact of the external force as modeled above. We think of this distinction as direct (or purely externally driven) entrainment versus indirect (or internally controlled) entrainment (the latter being a main confound of external driving).
Importantly, direct entrainment by a periodic external drive should demonstrate different temporal constraints than indirect (internally controlled) entrainment. The two forms should therefore be distinguishable on empirical grounds. Specifically, the fastest frequency for direct entrainment should correspond to the upper range of observable, biological brain rhythms (which is the ripple range, at 200–600 Hz; see Buzsáki and Draguhn, 2004). Direct entrainment should also be confined to a narrow bandwidth, where the stimulation frequency matches the targeted brain oscillation, and this frequency–specificity should make flanker stimulation frequencies comparatively ineffective. In contrast, the fastest frequency of indirect (internally controlled) entrainment should be much slower, e.g., limited by the dynamics of the build-up of attentional processes. In regards to anticipatory attention, for instance, this build-up has been estimated to 100–300 ms, the time it takes that neuronal excitability measures reach an asymptotic level during attentional orienting (e.g., Müller et al., 1998). Indirect (internally controlled) entrainment should therefore be effective over a large range of input frequencies, as long as attention engagement is possible, i.e., the stimulus periodicity does no reach the upper dynamic limits of the attentional process. For anticipatory attention, the upper frequency that can be tracked by attention should be in the alpha- to theta-range (due to the estimated limit of 100–300 ms periodicity).
We here focus on externally driven entrainment. Our review is therefore about generating brain oscillations without the need for active engagement of the participants in a task. When of concern, we weigh apparent evidence for direct entrainment against possible indirect (endogenous) confounds.
Methods for Non-Invasive Entrainment of Brain Rhythms
What are the non-invasive stimulation methods that could in principle be used for entrainment of human brain oscillations, and is such entrainment physiologically plausible?
Several methods are available for non-invasive brain stimulation in rhythmic patterns. We here classify these methods along two dimensions. Rhythmic stimulation can come in (a) pulsed forms which repeat short, transient stimulus events at regular intervals, or (b) continuous forms which generate oscillating (e.g., sinusoidal) stimulus patterns. In addition, rhythmic stimulation can be applied through (c) sensory input pathways, e.g., via presentation of visual stimuli, or (d) using transcranial stimulation techniques that stimulate the brain directly bypassing sensory input. Pulsed and continuous forms are available for both sensory and transcranial stimulation techniques, detailed below.
The classical, pulsed form of rhythmic stimulation is steady-state sensory presentation. In visual flicker, for instance, transient visual events are repeated at fixed frequency (pulsed sensory stimulation; e.g., Herrmann, 2001). Repetitive (also rhythmic) transcranial magnetic stimulation (TMS) – a further pulsed form of stimulation – uses the principle of electromagnetic current induction through a coil placed over the scalp to stimulate brain areas directly (pulsed transcranial stimulation; Hallett, 2007). With both of these techniques, stimuli can be presented in a wide range of repetition frequencies, covering the physiological range of brain oscillations from slow-waves to gamma-frequencies (at around 100 Hz; e.g., Herrmann, 2001; Thut and Miniussi, 2009). More recently developed transcranial stimulation techniques apply weak electrical currents in continuous oscillatory patterns through large sponge scalp electrodes (continuous transcranial stimulation). The latter techniques consist of oscillatory transcranial direct current stimulation (o-tDCS; Marshall et al., 2006) or transcranial alternating current stimulation (tACS; Antal et al., 2008). These techniques go beyond the pulsed stimulation forms as they allow to stimulate up to the ripple range (>200 Hz; Moliadze et al., 2010; Siebner and Ziemann, 2010).
In terms of spatial scale, pulsed sensory stimulation simultaneously drives many primary sensory structures and areas of the input pathways (sub-cortically and cortically), while transcranial stimulation directly drives underlying neuronal elements bypassing sensory input, albeit with different spatial resolutions (TMS has better resolution than o-tDCS and tACS) and with subsequent propagation to connected nodes. All techniques however have in common that they interact with the brain on the scale of large neuronal populations, such as those that are recordable with EEG and MEG. Note also that in contrast to sensory stimulation and TMS, the periodic stimulus is not noticeable during tACS and o-tDCS. Indirect (internally controlled) entrainment can therefore be ruled out a priori with the latter protocols.
All these methods therefore allow non-invasive, human brain stimulation at appropriate frequencies and spatial scales. But can they possibly induce entrainment? We will first examine whether their mechanisms of interaction with neuronal activity would permit entrainment, before reviewing the evidence for it.
Neurophysiological Basis of Entrainment
Neurophysiological mechanisms of entrainment are likely to differ between the pulsed and the continuous oscillatory forms of rhythmic stimulation. Pulsed forms of stimulation are ubiquitous in psychophysics and cognitive neuroscience, and numerous studies have focused on the neuronal consequences of one pulsed event in terms of the generation of evoked EEG activity (e.g., Regan, 1988; Niedermeyer and Silva, 2004). One influential theory of the evoked potential generation is also of great interest for the study of entrainment. This theory posits that EEG responses to sensory stimuli can partially be explained through transient, stimulus-induced adjustment of the phase of ongoing oscillations (phase-resetting; Sayers et al., 1974; Makeig et al., 2002; Klimesch et al., 2007b). An analogous explanation has been put forth to explain the EEG responses to a single TMS pulse, involving phase-resetting of ongoing rhythms of the stimulated cortex (Paus et al., 2001; Fuggetta et al., 2005; Van Der Werf and Paus, 2006; Rosanova et al., 2009). This implies that components of the evoked responses echo spectral features of ongoing oscillations, and by extension, that some of these components reflect ongoing oscillatory brain activity that has been reorganized (Sayers et al., 1974; Makeig et al., 2002; Klimesch et al., 2007b), albeit with a fast desynchronization rate since evoked potentials tend to fade rapidly after stimulus presentation. Yet, this also implies that entrainment of ongoing brain oscillations through the pulsed form of rhythmic stimulation is physiologically plausible, when the initial stimulus (sensory event or TMS pulse) is repeated in a way that catches the realigned oscillations at the correct phase, leading to further synchronization (see also Zaehle et al., 2010a).
Likewise, entrainment through continuous, time-varied (sinusoidal) forms of stimulation (o-tDCS or tACS) is plausible, albeit via another mechanism than phase-reset. In contrast to sensory stimuli or TMS pulses that excite neurons directly, transcranial stimulation with an electric current modulates the spontaneous firing rate of neurons through a shift of the cell’s resting membrane potential (Purpura and McMurtry, 1965; Nitsche et al., 2003). Although such changes in membrane potential have so far only been established using constant current strength (Purpura and McMurtry, 1965; Nitsche et al., 2003), it is conceivable that time-varying current strength, such as in tACS or o-tDCS, will also impose an oscillatory shift on the membrane potential, thereby affecting endogenous brain rhythms (Bergmann et al., 2009; Zaehle et al., 2010b).
Entrainment of human brain oscillations by these methods is therefore conceivable on physiological grounds. What is the evidence for such entrainment in the literature?
Empirical Evidence for Direct Entrainment of Perceptually Relevant Human Brain Rhythms by External Drive
We dissociate between purely electrophysiological evidence, purely behavioral evidence, or combined evidence for entrainment of brain oscillations. To date, most of the electrophysiological evidence stems from rhythmic sensory stimulation protocols (e.g., visual flicker) and research on steady-state responses (SSRs) in EEG and MEG, which we review first. EEG evidence for entrainment during TMS, tACS, or o-tDCS is only starting to emerge (see Electrophysiological evidence below). This bias in favor of sensory stimulation is due to the added complexity of dealing with electrically or magnetically induced artifacts during TMS, tACS, or o-tDCS, which is leading to rhythmic contaminations of the neural signals in EEG (e.g., for available TMS–EEG methods see Ilmoniemi and Kicić, 2010). Importantly, electrophysiological evidence for entrainment is corroborated by recent reports of frequency-specific behavioral consequences of rhythmic stimulation, reviewed later (see Behavioral evidence). Finally, reports of combined evidence are reviewed in the last section.
Electrophysiological Evidence for Entrainment
Such evidence is provided, if the origin of synchronization can be allocated to a direct interaction of the stimulus train with the ongoing brain oscillation (see Box 1). This should show in 1:1 phase alignment, and can only be demonstrated unequivocally through the study of brain responses during (online to) stimulation, although this may not constitute conclusive evidence (i.e., may be insufficient for a proof of entrainment in some instances, see controversies below).
Rapid rhythmic sensory stimulation gives rise to SSRs in MEG, EEG, or local field potential (LFP) recordings (Picton et al., 2003). SSRs show discrete frequency components that remain constant in amplitude and phase during (online to) stimulation (Regan, 1988). As would be expected from entrainment, the SSR-signal shows 1:1 phase alignment with the rhythmic stimulus, after a short transient period at the beginning of stimulation. Studies that tested SSRs for the visual cortex across a wide range of frequencies (1–100 Hz) have revealed enhanced phase-locked rhythmic activity at the fundamental frequency of stimulation up to frequencies of at least 50 Hz (Rager and Singer, 1998; Herrmann, 2001). In line with the entrainment of natural ongoing brain oscillations, these SSRs show further response–enhancement to stimulation at discrete (preferred) frequencies (Rager and Singer, 1998; Herrmann, 2001; Picton et al., 2003; Zaehle et al., 2010a). For the human visual system, this occurs at around 10, 20, and 40 Hz, which seems largely consistent with a scenario where rhythmic sensory stimulation entrains natural brain oscillations. Because frequency dependence occurs in discrete, narrow bandwidths, this is suggestive of direct entrainment by external drive. Interestingly, these SSRs do generally not persist after the offset of the stimulus train (e.g., Capilla et al., 2011).
Electrophysiological evidence for entrainment from transcranial stimulation protocol is still sparse. A recent EEG study has examined changes to oscillatory activity in response to tACS in human participants (Zaehle et al., 2010b). In comparison to sham stimulation, occipital tACS at individual alpha-frequency (alpha-tACS) selectively enhanced oscillatory EEG activity in the alpha-band, measured offline to stimulation over a 3-min post-tACS period (Zaehle et al., 2010b). EEG-data online to stimulation was not available as a consequence of tACS-induced artifacts. The offline results are therefore suggestive of entrainment that outlasted stimulation offset. But other accounts are conceivable, because in the absence of EEG recordings during tACS, entrainment cannot be unequivocally established, and because the specificity of alpha-enhancement to stimulation at alpha-frequency had not been assessed. For instance, long-term alpha-enhancement after occipital alpha-tACS could reflect a general tendency of the occipital cortex to respond in its preferred frequency (the alpha rhythms) to any active stimulation protocol (see Veniero et al., 2011, for analogy over motor cortex). Note that the external application of a sinusoidally oscillating electric field in animals (akin to o-tDCS in humans) entrains neuronal spiking in widespread areas, but that this effect does not persist beyond stimulation offset (Ozen et al., 2010), suggesting entrainment at a short time-scale.
More recently, we have demonstrated entrainment during rhythmic TMS (Thut et al., 2011) capitalizing on methods that allow for online TMS–EEG recordings. Rhythmic TMS over parietal cortex tuned to the preferred frequency of the underlying generator (i.e., alpha) entrained its oscillatory (alpha) activity (Thut et al., 2011). Entrainment could be inferred because alpha-enhancement was specific to rhythmic alpha-TMS, i.e., (1) was not observed in an arrhythmic TMS control in which the same number of pulses was randomly jittered over the same time interval (ruling out a general tendency of the parietal cortex to respond at its preferred frequency to any active protocol), and (2) was not observed with sham (inactive) TMS emulating the rhythmic auditory clicks of TMS (ruling out indirect confounds). In further accord with entrainment, the local alpha-response during alpha-TMS was explained by phase-locking of ongoing alpha activity to the TMS train. Hence, it was concluded that rhythmic TMS entrained a natural oscillation as opposed to the imposition of an artificial rhythm. Note that entrainment was of brief duration, i.e., vanished shortly after the end of TMS administration, at least with the used protocol (5-pulse TMS bursts; Thut et al., 2011).
Controversies
The view that SSRs reflect entrainment of brain oscillations has been challenged for several reasons. First, although spectral analysis of brain responses demonstrates dominant activity at stimulation frequency across a wide frequency range (at least 1–50 Hz), there is no evidence of (ongoing) oscillations at most of these frequencies. This questions whether the spectral peak at the fundamental frequency (stimulation frequency) is indeed caused by an underlying entrained oscillation. Second and alternatively, the spectral peak at fundamental frequency may rather be caused by the periodicity of stimulation (and subsequently evoked components). In keeping with this notion, SSRs can be explained by linear superposition of adequately constructed transient responses (Bohorquez and Ozdamar, 2008; Capilla et al., 2011). Specifically, transient responses derived from jittered stimulus trains (with a mean stimulation frequency corresponding to the steady-state frequency) allow precise reconstruction of SSRs. Third, entrainment (in the sense of phase alignment of self-sustained oscillations) is expected to last beyond the end of the stimulation train. This is because once the self-sustained oscillator has been phase-aligned, it is not expected to revert to its initial phase, since phase is a free parameter of self-sustained systems (e.g., Pikovsky et al., 2003). However, SSR studies generally fail to observe components at the end of the stimulation train beyond what can be explained by a transient, evoked component (Capilla et al., 2011, but see Ross et al., 2005).
However, these controversial results can be reconciled by a model in which transient EEG/MEG-responses to external stimuli and self-sustained (ongoing) EEG/MEG oscillations are tightly linked (Basar, 1980; David et al., 2006; Zaehle et al., 2010a). According to this view, the neural elements under study have specific oscillatory properties which determine both the (early parts of the) EEG/MEG-response to, e.g., a single pulse stimulus (e.g., Van Der Werf and Paus, 2006; Rosanova et al., 2009), and the frequencies of oscillations that can be entrained per area (e.g., Herrmann, 2001). Short, transiently induced oscillations may then speak for entrainment in a dampened system. In this view, the finding that linear superposition of short, transient responses can explain SSRs would neither prove nor disprove entrainment.
In the light of these controversies, we argue that complementary probes into entrainment, e.g., via behavioral measures, can provide important information (see below and Box 2: criteria for direct entrainment of brain oscillations through a periodic external drive). In addition, if entrainment does not persist after train offset, it would be of importance to show that entrainment depends on the ongoing oscillations prior to the train. Entrainment of self-sustained, natural oscillations should depend on the phase at which the stimulus train catches the ongoing oscillation, leading to a very specific (sinusoidal) relationship of entrainment strength with pre-stimulus phase. Accordingly, such a phase-dependence would provide strong support for entrainment of natural oscillations.
Box 2. Criteria for direct entrainment of brain oscillations through a periodic external drive.
EEG/MEG-evidence
(1) Synchronization of brain oscillations to the rhythmic stream of external events, as measured with EEG/MEG.
(2) Frequency–specificity of entrainment effects in EEG/MEG-signals, meaning that stimulation is maximally effective when corresponding to the natural frequency of the stimulated generator.
(3) Spatial specificity of entrainment effects in EEG/MEG-signals, meaning that a oscillatory signature with distinct topography involving the target area is emerging from entrainment.
(4) The targeted area can also cycle at the entrainment frequency in the absence of rhythmic stimulation (natural oscillator).
This natural oscillator can be:
(4.1) A self-sustained oscillator observable with EEG/MEG at baseline, in which case entrainment should show a relation with the ongoing oscillation (phase-dependence). We would grade this as strong evidence for entrainment.
(4.2) An oscillator that is activated by a task (task-related oscillatory EEG/MEG activity), in which case a relationship with ongoing oscillations might be absent. We grade this as moderate evidence for entrainment.
(4.3) No evidence for the targeted area to cycle at the most effective stimulation frequency (during baseline or a task) does not automatically disqualify as entrainment of natural oscillations, but would constitute only weak evidence.
Converging behavioral evidence
(5) Frequency-specific behavioral consequences during rhythmic stimulation (under the provision that there is a causal link between the power of the induced oscillations and behavior; for a review of correlational evidence see, e.g., Foxe and Snyder, 2011). Here, we would consider a double dissociation as the strongest evidence, followed by single dissociations.
(6) A cycling pattern of behavior during rhythmic stimulation (under the provision that phase of the induced oscillation causally relates to behavior; for a review of correlational evidence see, e.g., VanRullen et al., 2011; Mathewson et al., 2011).
Behavioral Evidence for Entrainment
Such evidence can add important information, given the challenges in interpreting electrophysiological evidence (see above).
Frequency-dependent behavioral effects of rhythmic stimulation would support entrainment, particularly when the effective stimulation frequency matches a background or task-related EEG/MEG-frequency of the stimulated area (1:1 frequency locking). Consider a frequency F(x) of known origin which has been related to a particular function X, via EEG- or MEG-experimentation. If stimulation of the appropriate brain areas at frequency F(x) – but not at a control frequency F(y) – were to promote function X, as assessed via behavioral measures, this would strongly indicate that entrainment of underlying brain oscillations cycling at F(x) may have caused the functional change (and by extension that there is a causal link between this rhythm and a distinct function). For a prominent recent example see Marshall et al. (2006) who showed that o-tDCS during sleep, when tuned to slow-wave EEG oscillations (<4 Hz) that correlate with overnight memory consolidation, promotes memory in a frequency-specific fashion. This evidence is of the order of a single dissociation (in neuropsychological terms; e.g., Shallice, 1988). If stimulation at the (control) frequency F(y) [but not at F(x)] promoted another function Y, then this would constitute a double dissociation2.
The example above is on memory and sleep-related EEG-frequencies. Recent behavioral evidence also points toward entrainment of rhythms of visual brain areas. Frequency-tuned stimulation of occipito-parietal areas alters specific aspects of perception both under rhythmic TMS (Klimesch et al., 2003; Sauseng et al., 2009; Romei et al., 2010, 2011) and tACS (Kanai et al., 2008; Feurra et al., 2011).
Rhythmic TMS at parietal brain rhythms changed performance in parietal lobe tasks, namely parietal stimulation at alpha-frequency altered location-based attention and working memory (Sauseng et al., 2009; Romei et al., 2010). These TMS-studies were based on a priori EEG-information that parietal alpha-oscillations (8–14 Hz) may play a regulatory role in visual attention/perception (Worden et al., 2000; Kelly et al., 2006; Thut et al., 2006; Mathewson et al., 2009; Gould et al., 2011) and visual spatial working memory (e.g., Freunberger et al., 2011) through inhibitory gating (Klimesch et al., 2007a; Jensen and Mazaheri, 2010; Foxe and Snyder, 2011). In line with this EEG evidence, tuning parietal TMS to alpha-frequency suppressed processing of information in the visual field contralateral to TMS both in working memory (Sauseng et al., 2009), and detection tasks (Romei et al., 2010).
Frequency-specific effects of rhythmic TMS were also obtained for another parietal lobe function and other oscillations, namely feature-based attention and the theta- and beta-rhythms (Romei et al., 2011). Building on a priori EEG evidence for a role of parietal theta-(5 Hz) and beta-(20 Hz) oscillations in the processing of global versus local features of a reversible visual scene (Smith et al., 2005, 2007), Romei et al. (2011) recently studied whether parietal TMS at theta or beta frequency can causally benefit visual processing at global or local levels, respectively. In contrast to the studies on parietal alpha (which are showing a single dissociation), these findings on parietal theta and beta revealed a double dissociation. Stimulation at theta frequency improved perception of global features whereas stimulation at beta frequency improved perception of local features.
Notably, all these findings show that the behavioral effects are confined to frequencies that had been identified as perceptually relevant in earlier EEG research (Worden et al., 2000; Sauseng et al., 2005; Smith et al., 2005, 2007; Kelly et al., 2006; Thut et al., 2006). Because the same protocols were ineffective at flanker frequencies (Sauseng et al., 2009; Romei et al., 2010) or showed a double dissociation (Romei et al., 2011), this behavioral evidence best accords with entrainment of natural oscillations of the stimulated occipital or parietal areas through the periodic electromagnetic force generated by TMS. Note that where tested, the timing of behavioral effects to rhythmic TMS was short-term, vanishing shortly after train offset (Romei et al., 2010), in line with the majority of electrophysiological evidence.
Similarly, tACS-research has revealed frequency-depended perceptual consequences at specific bandwidths of stimulation (Kanai et al., 2008; Feurra et al., 2011). Stimulation of primary sensory cortices evoked strongest illusory percepts when tACS was tuned to the preferred frequencies of these areas – i.e., the alpha-band. This was true for both visual sensations (phosphenes) evoked during occipital tACS (eyes closed condition, Kanai et al., 2008; but see Schwiedrzik, 2009) and tactile sensations evoked during tACS over somatosensory areas (Feurra et al., 2011). In addition to showing frequency–specificity, the most effective frequency also depended on the current state of the cortex (specificity shifted from alpha with eyes closed to beta with eyes open; Kanai et al., 2008), a further argument that there is interaction with ongoing cortical rhythms (but see Schwiedrzik, 2009). It is of interest to note that in these tACS-examples, it is alpha-tACS that led to an enhancement not to reduction of tACS-induced sensations, which is in apparent contradiction with the inhibition theory of the alpha oscillation (e.g., Klimesch et al., 2007a). Yet, this can be explained by entrainment and a variant of the inhibition model (“pulsed inhibition model”; Mathewson et al., 2009), if tACS-effectivity and sensory cortex receptivity co-cycle in the appropriate phase. Overall, these behavioral results suggest that it may also be possible through tACS to directly interact with natural brain oscillations in a frequency-specific fashion.
Finally, pulsed sensory stimulation at alpha-frequency prior to a presentation of a target leads to enhanced target detection, but only when the target coincides in time with the next expected (but not shown) event in the rhythmic event sequence (Mathewson et al., 2010). This is evidence for entrainment of neuronal processes to the rhythmic stream of events. However, the design of this study did not allow to resolve to what extent expectations and internal control drove these effects, or alternatively whether direct sensory entrainment of visual brain rhythms may have played a pivotal role.
Limitations
In the absence of EEG recordings, it is unclear whether oscillations have been induced by rhythmic interventions, in parallel to the behavioral effects. However, entrainment is a likely scenario, given that the most effective frequencies match with oscillations that have been linked to perception by EEG. Together with the above electrophysiological evidence, the behavioral findings therefore provide strong support for entrainment. Overall, the finding of a frequency-specific impact on perception and performance by rhythmic interventions suggests that these brain rhythms play a causal role in driving perception.
Combined Electrophysiological and Behavioral Evidence for Entrainment
Such evidence would firmly establish a case for entrainment, and for the relevance of oscillatory activity for behavior. Indeed, recent intracranial recordings in monkeys suggest that successful entrainment of neural oscillations leads to shorter reaction times (Lakatos et al., 2008). In this study a rhythmic stream of alternating visual and auditory stimuli was presented at a mean frequency of 1.5 Hz with the monkey attending to either visual or auditory stimuli in different experimental runs. Interestingly, LFP recordings in monkey V1 revealed stronger phase alignment of delta activity (1.5 Hz) to attended visual and auditory stimuli compared to unattended stimuli. Stimuli presented at the preferred phase of delta oscillations lead to shorter reaction times. These results can be interpreted as a top-down initiated alignment of V1 oscillations to the rhythmic structure of the sensory input, which is facilitated by the low presentation frequency. This phase alignment ensures that expected stimuli arrive at the preferred phase corresponding to a high excitability state of the neural population (Lakatos et al., 2009). Such control of oscillatory phase can implement attentional selection and gating of selected stimuli (Mazzoni et al., 2010; Panzeri et al., 2010). It should be noted that the entrainment effect discussed here would correspond to internally controlled, indirect entrainment. To the best of our knowledge, there is no combined evidence yet that unequivocally shows direct entrainment of brain activity by an external periodic drive, and simultaneously links this with behavior.
Lack of Evidence for Entrainment
While there is emerging evidence for entrainment in the human brain, there are also unsuccessful attempts to entrain.
Negative reports as to synchronization of oscillatory activity during o-tDCS and rhythmic TMS suggest that entrainment by direct interaction with oscillations is not straightforward (Bergmann et al., 2009; Johnson et al., 2010). In Bergmann et al. (2009), o-tDCS was applied at slow-wave frequencies over the motor cortex of awake participants and phase-aligned cycling of cortical excitability was probed through measuring TMS-evoked muscle twitches online to this rhythmic stimulation. No cycling pattern was found, suggesting that brain rhythms may not be entrained if applied at an inappropriate frequency; that is at a slow sleep-like oscillation when participants are awake (Bergmann et al., 2009). Similarly, in Johnson et al. (2010), rhythmic parietal alpha-TMS did not lead to entrainment, as shown by a lack of alpha-power increases over the target area during stimulation. This may suggest that fine tuning of stimulation parameters (e.g., site and frequency) is important to achieve entrainment. In addition, entrainment effects are expected to depend on the phase of the oscillation at the start of stimulation. Simple averaging across all trials (with different pre-stimulation phase) might obliterate existing entrainment effects.
Finally, negative reports of sustained entrainment after the end of the stimulus train seem to indicate that entrainment is short-term. In a meta analysis of TMS–EEG studies exploring long-term changes in oscillatory activity (EEG-after effects > 10 min) following rhythmic (repetitive) TMS, we could not find any evidence for a consistent match between affected EEG- and applied TMS-frequency, i.e., forfrequency-locking between TMS-input and long-term EEG-outcome (Thut and Miniussi, 2009; Thut and Pascual-Leone, 2010).
Criteria for Direct Entrainment through a Periodic External Drive
Many findings reviewed here constitute emerging, supportive evidence that rhythmic stimulation protocols can directly entrain human brain oscillations, with potential consequences for ongoing and upcoming perception and performance. This evidence is however not always conclusive. In some instances, there is only indirect evidence, for example when frequency-dependent effects on behavior are reported that match with prior EEG research. In other instances, entrainment seems likely, but alternative explanations may apply. There are also difficulties inherent to rhythmic stimuli, as these stimuli may entrain brain waves directly, but also engage attention of the participants and thereby provoke indirect (internally controlled) entrainment. To further complicate matters, both types of entrainment may co-exists, and even interact, as used for instance in research on attentional modulation of steady-state visual responses (Morgan et al., 1996; Müller et al., 1998; Ding et al., 2006; Kim et al., 2007; Parkkonen et al., 2008). Therefore, these forms of entrainment have to be disambiguated, e.g., through experimental designs that test for the bandwidth of the frequency response function. To inform further research, we conclude with a list of criteria for direct entrainment of natural rhythms through an external, periodic drive. We classify each criterion as providing weak, moderate, or strong support for entrainment (see Box 2).
Conclusion
The converging evidence for entrainment from several protocols (sensory stimulation, TMS, tACS, and o-tDCS) and measures (behavioral to electrophysiological) speaks in favor of controlled entrainment of human brain oscillations by an external periodic drive. The reviewed studies collectively suggest that such rhythmic stimulation may change attention and perception by modifying communication in oscillatory networks through their entrainment. How stimulation parameters and ongoing oscillations interact to give rise to entrainment will need to be studied in detail in future research. In addition, the duration of entrainment effects after stimulation will determine future uses beyond basic research on brain oscillations. By entraining biological brain oscillations in a controlled manner, and revealing the behavioral consequences on perception or cognition, we would come much closer to understand how brain oscillations map onto functions, and ultimately what is driving perception and cognition.
Conflict of Interest Statement
The authors declare that the research was conducted in the absence of any commercial or financial relationships that could be construed as a potential conflict of interest.
Acknowledgments
This work was supported by the Biotechnology and Biological Sciences Research Council [grant number BB/I006494/1] and the Wellcome Trust [091928].
Footnotes
- ^Note on the related term resonance: resonance denotes in physics the response of a passive system to external driving, whereas entrainment, synchronization, or locking is about driving an endogenous (self-sustained) oscillator.
- ^Note that a frequency (e.g., 4 Hz) may play multiple roles depending on the network of brain areas under investigation, and context. Hence, the terms single or double dissociation might only make sense if these variables (engaged network and context) are also considered.
References
Antal, A., Boros, K., Poreisz, C., Chaieb, L., Terney, D., and Paulus, W. (2008). Comparatively weak after-effects of transcranial alternating current stimulation (tACS) on cortical excitability in humans. Brain Stimul. 1, 97–105.
Basar, E. (1980). EEG-Brain Dynamics: Relation Between EEG and Brain Evoked Potentials. Amsterdam: Elsevier.
Bergmann, T. O., Groppa, S., Seeger, M., Molle, M., Marshall, L., and Siebner, H. R. (2009). Acute changes in motor cortical excitability during slow oscillatory and constant anodal transcranial direct current stimulation. J. Neurophysiol. 102, 2303–2311.
Bohorquez, J., and Ozdamar, O. (2008). Generation of the 40-Hz auditory steady-state response (ASSR) explained using convolution. Clin. Neurophysiol. 119, 2598–2607.
Buzsáki, G., and Draguhn, A. (2004). Neuronal oscillations in cortical networks. Science 304, 1926–1929.
Capilla, A., Pazo-Alvarez, P., Darriba, A., Campo, P., and Gross, J. (2011). Steady-state visual evoked potentials can be explained by temporal superposition of transient event-related responses. PLoS ONE 6, e14543. doi: 10.1371/journal.pone.0014543
David, O., Kilner, J. M., and Friston, K. J. (2006). Mechanisms of evoked and induced responses in MEG/EEG. Neuroimage 31, 1580–1591.
Ding, J., Sperling, G., and Srinivasan, R. (2006). Attentional modulation of SSVEP power depends on the network tagged by the flicker frequency. Cereb. Cortex 16, 1016–1029.
Feurra, M., Paulus, W., Walsh, V., and Kanai, R. (2011). Frequency specific modulation of human somatosensory cortex. Front. Psychol. 2:13. doi: 10.3389/fpsyg.2011.00013
Foxe, J. J., and Snyder, A. C. (2011). The role of alpha-band brain oscillations as a sensory suppression mechanism during selective attention. Front. Psychol. 2:154. doi: 10.3389/fpsyg.2011.00154
Freunberger, R., Werkle-Bergner, M., Griesmayr, B., Lindenberger, U., and Klimesch, W. (2011). Brain oscillatory correlates of working memory constraints. Brain Res. 1375, 93–102.
Fries, P. (2005). A mechanism for cognitive dynamics: neuronal communication through neuronal coherence. Trends Cogn. Sci. 9, 474–480.
Fuggetta, G., Fiaschi, A., and Manganotti, P. (2005). Modulation of cortical oscillatory activities induced by varying single-pulse transcranial magnetic stimulation intensity over the left primary motor area: a combined EEG and TMS study. Neuroimage 27, 896–908.
Gould, I. C., Rushworth, M. F., and Nobre, A. C. (2011). Indexing the graded allocation of visuospatial attention using anticipatory alpha oscillations. J. Neurophysiol. 105, 1318–1326.
Gouwens, N. W., Zeberg, H., Tsumoto, K., Tateno, T., Aihara, K., and Robinson, H. P. (2010). Synchronization of firing in cortical fast-spiking interneurons at gamma frequencies: a phase-resetting analysis. PLoS Comput. Biol. 6, e1000951. doi: 10.1371/journal.pcbi. 1000951
Herrmann, C. S. (2001). Human EEG responses to 1-100 Hz flicker: resonance phenomena in visual cortex and their potential correlation to cognitive phenomena. Exp. Brain Res. 137, 346–353.
Hutcheon, B., and Yarom, Y. (2000). Resonance, oscillation and the intrinsic frequency preferences of neurons. Trends Neurosci. 23, 216–222.
Ilmoniemi, R. J., and Kicić, D. (2010). Methodology for combined TMS and EEG. Brain Topogr. 22, 233–248.
Izhikevich, E. M. (2010). Dynamical Systems in Neuroscience: The Geometry of Excitability and Bursting. Cambridge: MIT Press.
Jensen, O., and Mazaheri, A. (2010). Shaping functional architecture by oscillatory alpha activity: gating by inhibition. Front. Hum. Neurosci. 4:186. doi: 10.3389/fnhum.2010.00186
Johnson, J. S., Hamidi, M., and Postle, B. R. (2010). Using EEG to explore how rTMS produces its effects on behavior. Brain Topogr. 22, 281–293.
Kanai, R., Chaieb, L., Antal, A., Walsh, V., and Paulus, W. (2008). Frequency-dependent electrical stimulation of the visual cortex. Curr. Biol. 8, 1839–1843.
Kelly, S. P., Lalor, E. C., Reilly, R. B., and Foxe, J. J. (2006). Increases in alpha oscillatory power reflect an active retinotopic mechanism for distracter suppression during sustained visuospatial attention. J. Neurophysiol. 95, 3844–3851.
Kim, Y. J., Grabowecky, M., Paller, K. A., Muthu, K., and Suzuki, S. (2007). Attention induces synchronization-based response gain in steady-state visual evoked potentials. Nat. Neurosci. 10, 117–125.
Klimesch, W., Sauseng, P., and Gerloff, C. (2003). Enhancing cognitive performance with repetitive transcranial magnetic stimulation at human individual alpha frequency. Eur. J. Neurosci. 17, 1129–1133.
Klimesch, W., Sauseng, P., and Hanslmayr, S. (2007a). EEG alpha oscillations: the inhibition-timing hypothesis. Brain Res. Rev. 53, 63–88.
Klimesch, W., Sauseng, P., Hanslmayr, S., Gruber, W., and Freunberger, R. (2007b). Event-related phase reorganization may explain evoked neural dynamics. Neurosci. Biobehav. Rev. 31, 1003–1016.
Lakatos, P., Karmos, G., Mehta, A. D., Ulbert, I., and Schroeder, C. E. (2008). Entrainment of neuronal oscillations as a mechanism of attentional selection. Science 320, 110–113.
Lakatos, P., O’Connell, M. N., Barczak, A., Mills, A., Javitt, D. C., and Schroeder, C. E. (2009). The leading sense: supramodal control of neurophysiological context by attention. Neuron 64, 419–430.
Lorincz, M. L., Kékesi, K. A., Juhász, G., Crunelli, V., and Hughes, S. W. (2009). Temporal framing of thalamic relay-mode firing by phasic inhibition during the alpha rhythm. Neuron 63, 683–696.
Makeig, S., Westerfield, M., Jung, T. P., Enghoff, S., Townsend, J., Courchesne, E., and Sejnowski, T. J. (2002). Dynamic brain sources of visual evoked responses. Science 295, 690–694.
Marshall, L., Helgadottir, H., Molle, M., and Born, J. (2006). Boosting slow oscillations during sleep potentiates memory. Nature 444, 610–613.
Mathewson, K. E., Fabiani, M., Gratton, G., Beck, D. M., and Lleras, A. (2010). Rescuing stimuli from invisibility: inducing a momentary release from visual masking with pre-target entrainment. Cognition 115, 186–191.
Mathewson, K. E., Gratton, G., Fabiani, M., Beck, D. M., and Ro, T. (2009). To see or not to see: prestimulus alpha phase predicts visual awareness. J. Neurosci. 29, 2725–2732.
Mathewson, K. E., Lleras, A., Beck, D. M., Fabiani, M., Ro, T., and Gratton, G. (2011). Pulsed out of awareness: EEG alpha oscillations represent a pulsed inhibition of ongoing cortical processing. Front. Psychol. 2:99. doi: 10.3389/fpsyg.2011.00099
Mazzoni, A., Whittingstall, K., Brunel, N., Logothetis, N. K., and Panzeri, S. (2010). Understanding the relationships between spike rate and delta/gamma frequency bands of LFPs and EEGs using a local cortical network model. Neuroimage 52, 956–972.
Moliadze, V., Antal, A., and Paulus, W. (2010). Boosting brain excitability by transcranial high frequency stimulation in the ripple range. J. Physiol. 588, 4891–4904.
Morgan, S. T., Hansen, J. C., and Hillyard, S. A. (1996). Selective attention to stimulus location modulates the steady-state visual evoked potential. Proc. Natl. Acad. Sci. U.S.A. 93, 4770–4774.
Müller, M. M., Teder-Sälejärvi, W., and Hillyard, S. A. (1998). The time course of cortical facilitation during cued shifts of spatial attention. Nat. Neurosci. 1, 631–634.
Niedermeyer, E., and Silva, F. D. (2004). Electroencephalography: Basic Principles, Clinical Applications and Related Fields, 5th Edn. Philadelphia, PA: Lippincott Williams and Wilkins.
Nitsche, M. A., Fricke, K., Henschke, U., Schlitterlau, A., Liebetanz, D., Lang, N., Henning, S., Tergau, F., and Paulus, W. (2003). Pharmacological modulation of cortical excitability shifts induced by transcranial direct current stimulation in humans. J. Physiol. 553, 293–301.
Ozen, S., Sirota, A., Belluscio, M. A., Anastassiou, C. A., Stark, E., Koch, C., and Buzsaki, G. (2010). Transcranial electric stimulation entrains cortical neuronal populations in rats. J. Neurosci. 30, 11476–11485.
Palva, S., and Palva, J. M. (2007). New vistas for alpha-frequency band oscillations. Trends Neurosci. 30, 150–158.
Panzeri, S., Brunel, N., Logothetis, N. K., and Kayser, C. (2010). Sensory neural codes using multiplexed temporal scales. Trends Neurosci. 33, 111–120.
Parkkonen, L., Andersson, J., Hämäläinen, M., and Hari, R. (2008). Early visual brain areas reflect the percept of an ambiguous scene. Proc. Natl. Acad. Sci. U.S.A. 105, 20500–20504.
Paus, T., Sipila, P. K., and Strafella, A. P. (2001). Synchronization of neuronal activity in the human primary motor cortex by transcranial magnetic stimulation: an EEG study. J. Neurophysiol. 86, 1983–1990.
Picton, T. W., John, M. S., Dimitrijevic, A., and Purcell, D. (2003). Human auditory steady-state responses. Int. J. Audiol. 42, 177–219.
Pikovsky, A., Rosenblum, M., and Kurths, J. (2003). Synchronization: A Universal Concept in Nonlinear Sciences, 1st Edn. New York, NY: Cambridge University Press.
Purpura, D. P., and McMurtry, J. G. (1965). Intracellular activities and evoked potential changes during polarization of motor cortex. J. Neurophysiol. 28, 166–185.
Rager, G., and Singer, W. (1998). The response of cat visual cortex to flicker stimuli of variable frequency. Eur. J. Neurosci. 10, 1856–1877.
Regan, D. (1988). Human Brain Electrophysiology: Evoked Potentials and Evoked Magnetic Fields in Science and Medicine. New York, NY: Appleton & Lange.
Romei, V., Driver, J., Schyns, P., and Thut, G. (2011). Rhythmic TMS over right parietal cortex causally links distinct brain frequencies to global versus local visual processing. Curr. Biol. 21, 334–733.
Romei, V., Gross, J., and Thut, G. (2010). On the role of prestimulus alpha rhythms over occipito-parietal areas in visual input regulation: correlation or causation? J. Neurosci. 30, 8692–8697.
Rosanova, M., Casali, A., Bellina, V., Resta, F., Mariotti, M., and Massimini, M. (2009). Natural frequencies of human corticothalamic circuits. J. Neurosci. 29, 7679–7685.
Ross, B., Herdman, A. T., and Pantev, C. (2005). Stimulus induced desynchronization of human auditory 40-Hz steady-state responses. J. Neurophysiol. 94, 4082–4093.
Salinas, E., and Sejnowski, T. J. (2001). Correlated neuronal activity and the flow of neural information. Nat. Rev. Neurosci. 2, 539–550.
Sauseng, P., Klimesch, W., Heise, K. F., Gruber, W. R., Holz, E., Karim, A. A., Glennon, M., Gerloff, C., Birbaumer, N., and Hummel, F. C. (2009). Brain oscillatory substrates of visual short-term memory capacity. Curr. Biol. 19, 1846–1852.
Sauseng, P., Klimesch, W., Stadler, W., Schabus, M., Doppelmayr, M., Hanslmayr, S., Gruber, W. R., and Birbaumer, N. (2005). A shift of visual spatial attention is selectively associated with human EEG alpha activity. Eur. J. Neurosci. 22, 2917–2926.
Sayers, B. M., Beagley, H. A., and Henshall, W. R. (1974). The mechanism of auditory evoked EEG responses. Nature 247, 481–483.
Schnitzler, A., and Gross, J. (2005). Normal and pathological oscillatory communication in the brain. Nat. Rev. Neurosci. 6, 285–296.
Schwiedrzik, C. M. (2009). Retina or visual cortex? The site of phosphene induction by transcranial alternating current stimulation. Front. Integr. Neurosci. 3:6. doi: 10.3389/neuro.07.006.2009
Shallice, T. (1988). From Neuropsychology to Mental Structure. Cambridge: Cambridge University Press.
Siebner, H. R., and Ziemann, U. (2010). Rippling the cortex with high-frequency (>100 Hz) alternating current stimulation. J. Physiol. 588, 4851–4852.
Singer, W., and Gray, C. M. (1995). Visual feature integration and the temporal correlation hypothesis. Annu. Rev. Neurosci. 18, 555–586.
Smith, M. L., Gosselin, F., and Schyns, P. G. (2005). Perceptual moments of conscious visual experience inferred from oscillatory brain activity. Proc. Natl. Acad. Sci. U.S.A. 103, 5626–5631.
Smith, M. L., Gosselin, F., and Schyns, P. G. (2007). From a brain to its category via a few information processing states in the brain. Neuroimage 37, 974–984.
Tass, P. A. (2002). Desynchronization of brain rhythms with soft phase-resetting techniques. Biol. Cybern. 87, 102–115.
Thut, G., and Miniussi, C. (2009). New insights into rhythmic brain activity from TMS-EEG studies. Trends Cogn. Sci. 13, 182–189.
Thut, G., Nietzel, A., Brandt, S. A., and Pascual-Leone, A. (2006). Alpha-band electroencephalographic activity over occipital cortex indexes visuospatial attention bias and predicts visual target detection. J. Neurosci. 26, 9494–9502.
Thut, G., and Pascual-Leone, A. (2010). A review of combined TMS-EEG studies to characterize lasting effects of repetitive TMS and assess their usefulness in cognitive and clinical neuroscience. Brain Topogr. 22, 219–232.
Thut, G., Veniero, D., Romei, V., Miniussi, C., Schyns, P., and Gross, J. (2011). Rhythmic TMS causes local entrainment of natural oscillatory signatures. Curr. Biol. doi: 10.1016/j.cub.2011.05.049. [Epub ahead of print].
Van Der Werf, Y. D., and Paus, T. (2006). The neural response to transcranial magnetic stimulation of the human motor cortex. I. Intracortical and cortico-cortical contributions. Exp. Brain Res. 175, 231–245.
VanRullen, R., Busch, N., Drewes, J., and Dubois, J. (2011). Ongoing EEG phase as a trial-by-trial predictor of perceptual and attentional variability. Front. Psychol. 2:60. doi: 10.3389/fpsyg.2011.00060
Veniero, D., Brignani, D., Thut, G., and Miniussi, C. (2011). Alpha-generation as basic response-signature to transcranial magnetic stimulation (TMS) targeting the human resting motor cortex: a TMS/EEG co-registration study. Psychophysiology. doi: 10.1111/j.1469-8986.2011.01218.x. [Epub ahead of print].
von Stein, A., Chiang, C., and König, P. (2000). Top-down processing mediated by interareal synchronization. Proc. Natl. Acad. Sci. U.S.A. 97, 14748–14753.
Whittington, M. A., Stanford, I. M., Colling, S. B., Jefferys, J. G., and Traub, R. D. (1997). Spatiotemporal patterns of gamma frequency oscillations tetanically induced in the rat hippocampal slice. J. Physiol. 502, 591–607.
Worden, M. S., Foxe, J. J., Wang, N., and Simpson, G. V. (2000). Anticipatory biasing of visuospatial attention indexed by retinotopically specific alpha-band electroencephalography increases over occipital cortex. J. Neurosci. 20, RC63.
Zaehle, T., Lenz, D., Ohl, F. W., and Herrmann, C. S. (2010a). Resonance phenomena in the human auditory cortex: individual resonance frequencies of the cerebral cortex determine electrophysiological responses. Exp. Brain Res. 203, 629–635.
Keywords: entrainment, brain oscillations, rhythmic brain stimulation, perception
Citation: Thut G, Schyns PG and Gross J (2011) Entrainment of perceptually relevant brain oscillations by non-invasive rhythmic stimulation of the human brain. Front. Psychology 2:170. doi: 10.3389/fpsyg.2011.00170
Received: 18 February 2011;
Accepted: 06 July 2011;
Published online: 20 July 2011.
Edited by:
Ole Jensen, Radboud University, NetherlandsReviewed by:
Christoph S. Herrmann, Carl von Ossietzky University, GermanyRyota Kanai, University College London, UK
Michael Rosenblum, Potsdam University, Germany
Copyright: © 2011 Thut, Schyns and Gross. This is an open-access article subject to a non-exclusive license between the authors and Frontiers Media SA, which permits use, distribution and reproduction in other forums, provided the original authors and source are credited and other Frontiers conditions are complied with.
*Correspondence: Gregor Thut, Centre for Cognitive Neuroimaging, Institute of Neuroscience and Psychology, University of Glasgow, 58 Hillhead Street, Glasgow G12 8QB, UK. e-mail:Z3JlZ29yLnRodXRAZ2xhc2dvdy5hYy51aw==