- 1 Institute of Cognitive Neuroscience, Division of Psychology and Language Sciences, University College London, London, UK
- 2 Department of Psychological Sciences, Birkbeck, University of London, London, UK
- 3 Wellcome Department of Imaging Neuroscience, University College London, London, UK
- 4 Niels Bohr project “interacting minds,” Center of Functionally Integrative Neuroscience (CFIN), University of Aarhus, Aarhus, Denmark
The “default mode network” is commonly described as a set of brain regions in which activity is suppressed during relatively demanding, or difficult, tasks. But what sort of tasks are these? We review some of the contrasting ways in which a task might be assessed as being difficult, such as error rate, response time, propensity to interfere with performance of other tasks, and requirement for transformation of internal representations versus accumulation of perceptual information. We then describe a fMRI study in which 18 participants performed two “stimulus-oriented” tasks, where responses were directly cued by visual stimuli, alongside a “stimulus-independent” task, with a greater reliance on internally generated information. When indexed by response time and error rate, the stimulus-independent task was intermediate in difficulty between the two stimulus-oriented tasks. Nevertheless, BOLD signal in medial rostral prefrontal cortex (MPFC) – a prominent part of the default mode network – was reduced in the stimulus-independent condition in comparison with both the more difficult and the less difficult stimulus-oriented conditions. By contrast, other regions of the default mode network showed greatest deactivation in the difficult stimulus-oriented condition. There was therefore significant functional heterogeneity between different default mode regions. We conclude that task difficulty – as measured by response time and error rate – does not provide an adequate account of signal change in MPFC. At least in some circumstances, a better predictor of MPFC activity is the requirement of a task for transformation and manipulation of internally represented information, with greatest MPFC activity in situations predominantly requiring attention to perceptual information.
Introduction
“Task-induced deactivation” was originally identified as a phenomenon whereby a set of regions exhibited greater blood flow or BOLD signal during baseline rest or fixation conditions than during task performance (e.g., Shulman et al., 1997). This phenomenon has been reported to occur in widespread brain regions including medial prefrontal and parietal cortices, inferior parietal lobule, and medial temporal lobe (Buckner et al., 2008). While there is general consensus that this effect represents the occurrence of mental processes that are more common during unconstrained baseline conditions than performance of cognitive tasks, the exact nature of these processes is not fully understood. The most common theoretical interpretation has been that “task-induced deactivations” reflect the occurrence of spontaneous internally directed thought processes such as autobiographical memory retrieval and internally oriented mindwandering during unconstrained baseline conditions (Buckner et al., 2008). However, others have argued that activity in the default mode network – particularly medial rostral prefrontal cortex (MPFC) – may at least in some situations reflect enhanced stimulus-oriented attention, e.g., watchfulness toward the external environment rather than internally focused attention (Gilbert et al., 2006a, 2007a; Hahn et al., 2007).
In the years following the discovery of task-induced deactivations, subsequent studies demonstrated similar patterns of results not only when unconstrained baseline conditions were compared against cognitive tasks, but also when relatively easy tasks were contrasted against relatively difficult tasks. For example, MPFC activity is greater during performance of a relatively easy 0-back task than a 2-back working memory task (Leech et al., 2011). Furthermore, parametric designs reveal increasing activity in MPFC as task demands decrease (McKiernan et al., 2003). This is perhaps not surprising. After all, rather than being wholly unconstrained, “rest” is still an externally imposed task in the sense that participants are expected to lie still in the scanner, to keep their eyes open or closed as instructed, to refrain from speaking, to await upcoming instructions and so on. There is not therefore any necessary qualitative difference between “rest” or “fixation” and other low-demand tasks (e.g. 0-back).
The foregoing discussion raises an obvious question. What exactly is meant by a low-demand (or easy) task versus a high-demand (or difficult) one? If we can identify what factors are associated with task difficulty, as it relates to task-induced deactivation, this may help understand the processes contributing to this effect. There are many different ways of defining or measuring “task difficulty.” Below, we consider some of the ways in which difficulty might be conceptualized. Some of these approaches reflect operationalizations that can directly be measured, while others reflect putative cognitive processes that cannot directly be observed. Although clearly interrelating, these different approaches need not overlap perfectly with each other.
The most obvious test of task difficulty is whether or not a particular task can be performed at all. Related to this is a developmental perspective, according to which an easier task is defined as one that can first be performed at an earlier developmental stage. More helpfully in research with healthy adult participants, rather than defining a difficult task as one that cannot be performed at all, the difficulty of a task might be indexed by some quantitative measure of task compliance, such as percent correct in a task split into a series of trials.
A second measure of task difficulty is response time, typically the time taken between the appearance of an imperative stimulus and the production of an appropriate motor response; by this measure a longer average response time indicates a more difficult task. These first two indices of task difficulty are complicated by their relationship with each other. It has long been recognized that participants are able voluntarily to trade accuracy and response time against each other, i.e., respond relatively quickly and inaccurately or slowly and accurately (Fitts, 1966; Wickelgren, 1977). Thus, both response time and accuracy must be considered to derive conclusions about task difficulty, as opposed to shifts in a speed/accuracy tradeoff. Considered together, response time and accuracy are the most common indices of task difficulty, and it is not uncommon for authors to state that tasks or conditions have been “matched in difficulty,” meaning that these measures do not differ significantly between conditions. We will refer to accuracy and response time below as the standard indices of task difficulty. However, we consider below some other potential measures.
A third index of task difficulty might be in terms of dual-task interference. According to this idea, a task can be defined as more difficult insofar as it causes greater interference to concurrent performance of an additional task. On a practical level, this definition may be particularly helpful when it is difficult to assess the task itself (e.g., if it has no response requirements), seeing as the effects on a secondary task may be measured. However, it should be noted that propensity to disturb performance of a second task is affected by both tasks under consideration. It is quite possible that task X interferes with performance of task A but not task B, while task Y shows the reverse pattern. This is likely to depend upon competition for specific resources such as those related to particular input (e.g., Allport et al., 1972) or output (e.g., McLeod, 1977) modalities.
Under a fourth definition, difficult tasks might be defined as those that are most vulnerable to factors adversely affecting performance, including age, fatigue, neurological insult, or neurodisruption (e.g., transcranial magnetic stimulation). This definition is highly relevant to the interpretation of a differential pattern of impairment across two tasks (i.e., a “single dissociation”). Suppose that a particular brain lesion impairs performance of task A but not task B. This might be evidence that task A requires a particular cognitive resource, supported by the relevant brain region, whereas task B does not. However, an alternative interpretation might be that task A is simply more difficult than task B, in the sense that the same resources are required by both tasks but task A places greater demands on those resources. This motivates the “double dissociation” logic in neuropsychology (Shallice, 1988).
A fifth definition of task difficulty might encompass the concept of “executive function” or “cognitive control.” Executive function is an umbrella term encompassing a variety of cognitive processes that optimize flexible, goal-directed behavior, particularly in the face of novelty (Norman and Shallice, 1986; Miller and Cohen, 2001; Gilbert and Burgess, 2008). Difficult tasks are associated with high demands on executive function, rather than being performed in a routine manner.
Seeing as executive function is an umbrella term, more specific definitions of task difficulty are possible, relating to particular facets of this concept. For example, a sixth measure of task difficulty might be in terms of subjective effort and motivation, i.e., a difficult task would be one that participants perceive as more demanding and/or one that they have less intrinsic motivation to perform. In this sense, most people find that eating enough fruit and vegetables is a more difficult task than eating enough saturated fat. This definition relates to the concept of executive function seeing as processes required for overcoming temptation might be considered to involve cognitive control (Muraven and Baumeister, 2000; Vohs and Heatherton, 2000).
Finally, a seventh definition of task difficulty – again relating to the concept of executive function – might be that difficult tasks require participants to transform internal representations relevant to task performance, in a manner decoupled from current sensory input. This contrasts with tasks that may be performed on the basis of relatively direct stimulus-response links (see Gilbert et al., 2006a,b for discussion of this definition of task difficulty as it relates to signal change within MPFC). By this criterion, a task requiring participants simply to accumulate perceptual evidence for a decision and then respond on the basis of a learned stimulus-response link is less difficult than one that requires participants to effect one or more transformations of an internal representation of the stimulus before responding. Note that the requirement to manipulate stimulus-independent information itself describes a wide variety of situations (e.g., autobiographical memory retrieval, forms of mathematical cognition, etc.), which may potentially involve quite different cognitive processes.
It is clear that in everyday language the word “difficult” is used in many related but non-identical senses. Of course, there does not have to be a single definition of difficulty that precisely captures all of these senses. However, returning to the literature on task-induced deactivations, this raises the question of the most appropriate definition of task difficulty for predicting the effect of different tasks on activity within regions of the so-called “default mode” network. If task-induced deactivation is defined as the finding of greater brain activity during an easy task such as “rest” or 0-back than a difficult task (e.g., 2-back), we cannot understand this effect without understanding what is meant by “difficulty.” Can we achieve a more scientifically precise definition of task difficulty, as it relates to task-induced deactivations? One possibility is that the concept of difficulty is used in so many disparate senses that it cannot play a useful neuroscientific role, because it has “wildly disjunctive” meanings (Fodor, 1974). This would be akin to other concepts of folk psychology like belief or desire that are unlikely to map neatly onto neuroscientific theories (Churchland, 1981). Alternatively, it might be argued that the family resemblance between different uses of “task difficulty” is sufficient for it to play a helpful conceptual role, but that its meaning needs to be defined more precisely in order to better understand the phenomenon of task-induced deactivation.
In the present study we contrast the standard operationalization of task difficulty – that difficult tasks are those with high response time and/or error rate – with the final conceptualization: that difficult tasks are those with greater requirement for transformation internal representations. In many situations, these different criteria will converge. For example, a well-learned response to the magnitude of visually presented digits will have faster response time than a task requiring more extensive arithmetical operations (Dumontheil et al., 2010). However, response time and requirement for transformation of internal representations can be dissociated. A task with minimal requirement for transformation of internal representations can nevertheless be made very difficult, in terms of response time and error rate, if it requires a fine perceptual discrimination. Such a task may require a long period of evidence accumulation on each trial before a response can be made with any confidence (i.e., according to the diffusion model of Ratcliff, 1978 this would be a task with a low drift rate). We therefore contrasted two stimulus-oriented tasks, one easy and one difficult but both with minimal requirement for transformation of internal representations, with a stimulus-independent task that had an intermediate response time and error rate, but a greater demand than either of the other two tasks for transformation of internal representations. This allowed us to investigate which of these two definitions of task difficulty best predicts signal change within “default mode” brain regions.
Materials and Methods
The present article reports data from the control group of Gilbert et al. (2008), including an experimental condition that was not described in the original study.
Participants
Eighteen volunteers participated in the study (13 male; mean age 32, SD 8). All participants were right-handed, had normal or corrected-to-normal vision and were naïve with respect to the purpose of the experiment. None had performed the present experimental tasks, or related tasks, previously. The experiment was performed with local ethical committee approval and in accordance with the ethical standards laid down in the 1964 Declaration of Helsinki. Written informed consent was obtained from all participants before their inclusion in the study.
Tasks
The experimental task was modified from Gilbert et al., 2005, Task 3). In the main experimental condition, participants alternated between the easy stimulus-oriented (SOeasy) and stimulus-independent (SI) conditions. During SOeasy phases, participants classified capital letters by pressing one of two buttons, according to whether the letter was composed entirely of straight lines (e.g., “A”), or whether it had any curves (e.g., “B”). Immediately following each button press, the subsequent letter in the alphabet was presented. Stimuli were presented in Arial typeface, approximately 1° tall and wide. During SI phases, randomly chosen letters were presented and participants were required to mentally continue the sequence from their current position in the alphabet, performing the same classification task for each self-generated letter. In this condition, the correct continuation of the alphabet sequence was never presented on screen. Stimuli in the two phases were presented in different colors (red or blue), with the assignment of each color to a particular phase counterbalanced across participants. The first letter to be presented in each SO phase was the appropriate continuation of the sequence, assuming that the sequence had been correctly maintained during the preceding SI phases. Transitions between the SO and SI phases occurred with a mean interval of 7.5 s (range 3–21 s). In additional blocks of trials, participants performed the SOdifficult condition. In this task, non-alphanumeric, non-meaningful stimuli were presented on each trial and the task, like the SOeasy condition, was to decide whether the stimulus was composed entirely of straight lines or contained a curve. The stimuli were generated by an algorithm that randomly chose between three and five lines for each item, which were approximately matched in size with the letter stimuli in the other two conditions. On a randomly selected 50% of trials one of these lines was slightly curved (randomly in a concave or convex direction). See Figure 1 for example stimuli. As in the SOeasy and SI conditions, the next stimulus was presented immediately after each response, and the stimulus color switched between red and blue with the same timing parameters as switches between the SOeasy and SI conditions. In all conditions, participants were asked to respond as quickly and as accurately as possible. Seeing as the tasks were self-paced, each block contained a variable number of trials.
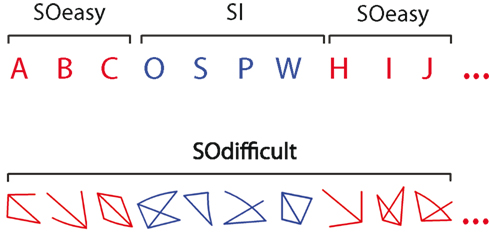
Figure 1. Example stimuli from the three conditions. In the SOeasy and SOdifficult tasks, participants judged whether the stimulus contained a curve or was composed entirely of straight lines. This perceptual discrimination differed in difficulty between the two conditions. In the SI condition, participants continued the alphabet stimulus from the last SOeasy trial and performed the same straight/curved discrimination task on internally generated letters, while ignoring distractor stimuli.
Scanning Procedure
Participants were familiarized with the tasks during a practice session lasting approximately 15 min, immediately before the scanning session. A 3T Siemens Allegra head-only system was used to acquire both T1-weighted structural images and T2*-weighted echoplanar (EPI) images [64 × 64; 3 mm × 3 mm pixels; echo time (TE), 30 ms] with BOLD contrast. Each volume comprised 48 axial slices (2 mm thick, separated by 1 mm), oriented approximately parallel to the AC–PC plane, covering the whole brain. Volumes were acquired continuously with an effective repetition time (TR) of 3.12 s per volume. Functional scans were acquired during two sessions, each comprising 121 volumes (lasting approximately 6 min). The first five volumes in each session were discarded to allow for T1 equilibration effects. Half of the participants performed two sessions of an unrelated random generation task before the two sessions of the tasks described in the present study (see Gilbert et al., 2008 for details). Within each session, participants alternated between 40 s blocks where they performed the SOeasy and SI conditions and 20 s blocks where they performed the SOdifficult condition. Each block was preceded by a 6 s instruction period. Note that there was no “rest” condition in this experiment, so there is no meaningful baseline with which to compare the three experimental conditions. Although this makes it difficult to directly compare the present results with studies of “rest,” it maximizes our power to detect differences between our theoretically motivated experimental conditions, which aim to disambiguate alternative definitions of task difficulty.
Data Analysis
fMRI data were analysed using SPM2 software (http://www.fil.ion.ucl.ac.uk/spm/spm2.html). Volumes were realigned, corrected for different slice acquisition times, normalized into 2 mm cubic voxels using a standard EPI template based on the Montreal Neurological Institute (MNI) reference brain using fourth-degree B-spline interpolation, and smoothed with an isotropic 8 mm full-width half-maximum Gaussian kernel. The volumes acquired during the two sessions were treated as separate time series. For each series, the variance in the BOLD signal was decomposed with a set of regressors in a general linear model (Friston et al., 1995). Variance was decomposed into components associated with responses made during the SOeasy, SI, red SOdifficult, and blue SOdifficult phases. An additional pair of regressors indexed (1) switches between SOeasy and SI, and (2) switches between red SOdifficult and blue SOdifficult. Thus the red and blue trials in the SOeasy and SI conditions were modeled identically to the red and blue trials in the SOdifficult condition. These regressors were derived from delta functions aligned to each stimulus onset convolved with a canonical hemodynamic response function. An additional regressor indexed sustained activity during the instruction periods, using a boxcar function convolved with a canonical hemodynamic response function. These regressors, together with regressors representing residual movement-related artifacts and the mean over scans, comprised the full model for each session. The data and model were high-pass filtered to a cut-off of 1/128 Hz.
Parameter estimates for each regressor were calculated from the least mean squares fit of the model to the data. Effects of interest were assessed in random effects analyses using t-tests on contrast images generated from subject-specific analyses. Seeing as the difference between red and blue SOdifficult trials was not of theoretical interest, averaged parameter estimates for regressors representing these two conditions were compared against either SOeasy or SI. Comparisons between the three experimental conditions were performed on regressors indexing non-switch trials only, in order to discard activity related to switching between the SOeasy and SI conditions, or between the two colors in the SOdifficult condition. Contrasts were thresholded at p < 0.001 uncorrected for multiple comparisons, with a minimum extent of five contiguous voxels. These exploratory analyses were supplemented with results at a corrected threshold, using small-volume corrections based on independently defined regions of interest as defined below. For each of these region-of-interest analyses, and the analyses of behavioral data, an initial omnibus test was conducted across regions and/or conditions. Where this test was significant (p < 0.05), exploratory (i.e., non-Bonferroni-corrected) follow-up tests were performed in order to characterize the pattern of results that led to the significant omnibus test.
Results
Behavioral Results
Figure 2 illustrates mean response time and error rate in the three conditions. For both measures, there was a main effect of condition [F(2,16) > 8.8, p < 0.003]. The SI condition was intermediate between the SOeasy and SOdifficult conditions in both cases. Furthermore, all pairwise comparisons between conditions were significant [t(17) > 2.8, p < 0.012] with the exception of the comparison between the error rates for the SOeasy and SI conditions [t(17) = 1.7, p = 0.10].
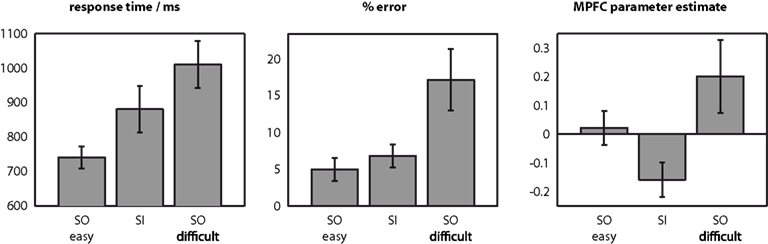
Figure 2. Behavioural data, and signal change in medial prefrontal cortex (MPFC). Response time and error rate were both intermediate in the SI condition between SOeasy and SOdifficult conditions. All pairwise comparisons were significant, except for the comparison in error rates between SOeasy and SI conditions. Contrastingly, signal change in MPFC was relatively high in SOeasy and SOdifficult, relative to SI. Error bars indicate within-subject 95% confidence intervals (Loftus and Masson, 1994) for the comparison of each bar against the bar with the closest value.
fMRI Results
Full results of the contrasts between the three conditions are shown in Figure 3 and Table 1.
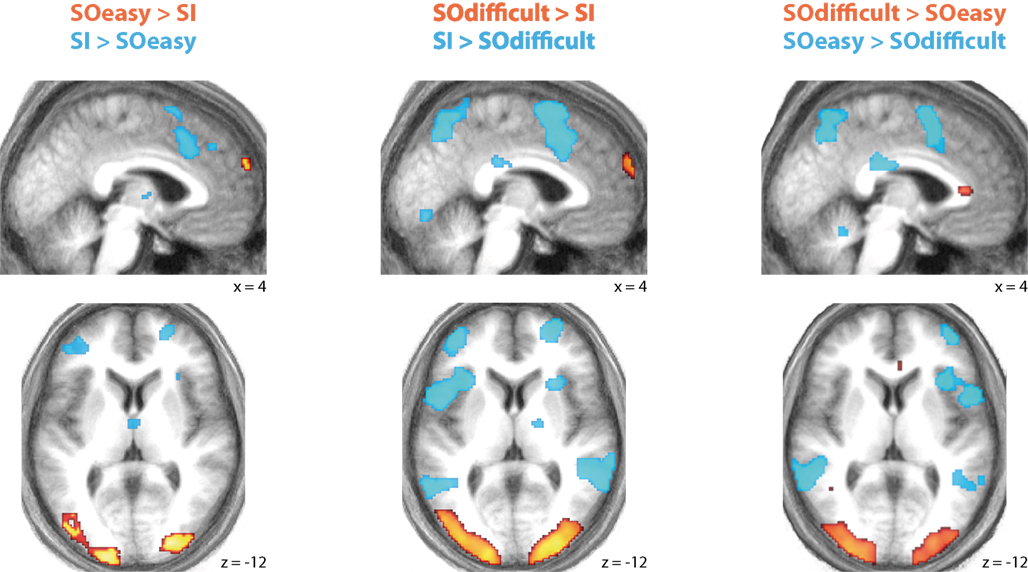
Figure 3. Significant differences in activation between SOeasy, SOdifficult, and SI conditions. Both SO conditions showed relatively high activity in medial rostral prefrontal cortex and occipital cortex, relative to SI. Conversely, the SI condition showed relatively high activity in lateral prefrontal cortex and posterior medial prefrontal cortex relative to both SO conditions. Results are plotted on the mean normalized structural scan.
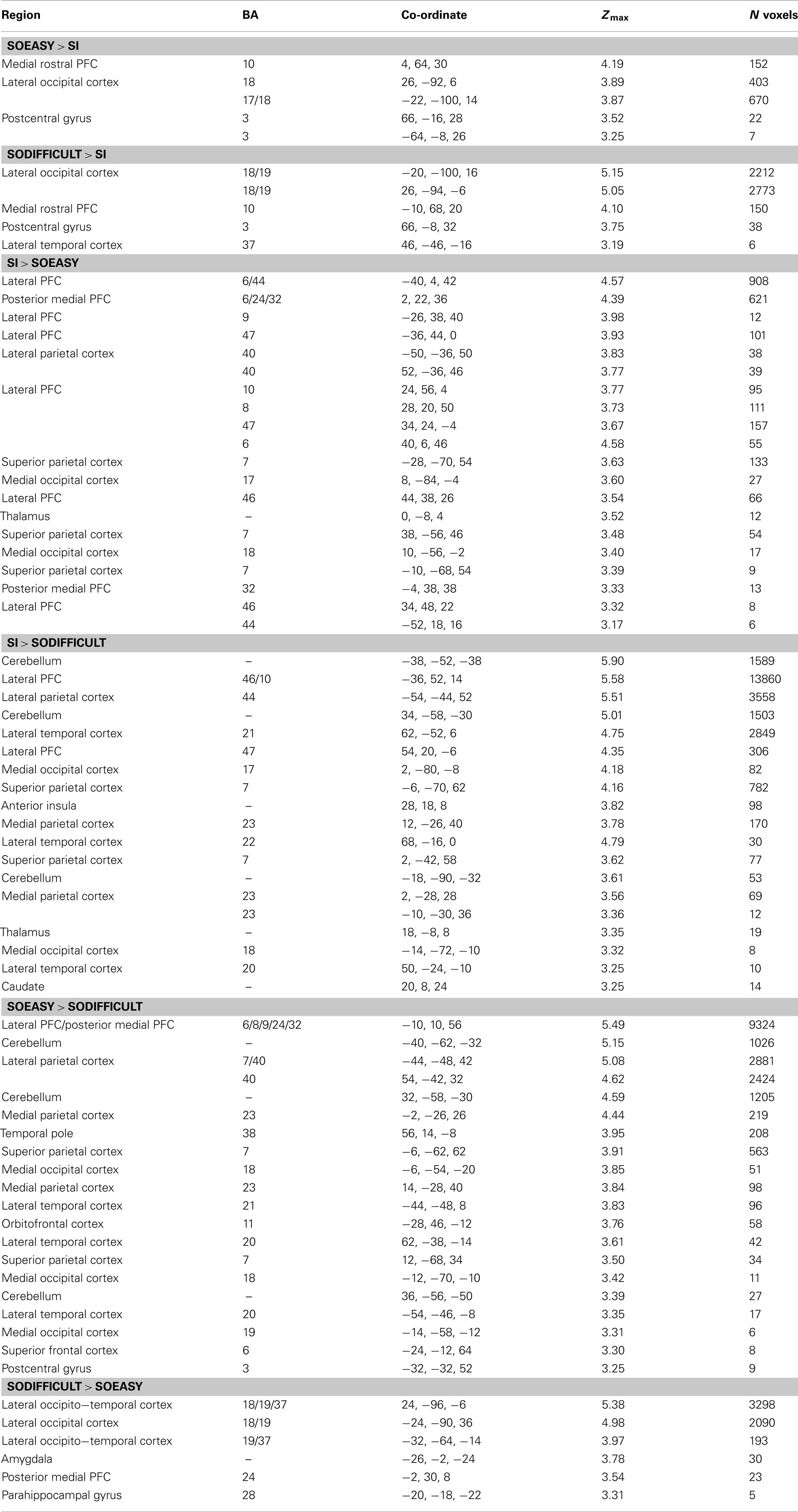
Table 1. Regions of significant difference in activation between conditions (p < 0.001 uncorrected; minimum extent five voxels).
Two patterns of results can be seen. One network of brain regions, predominantly comprising lateral prefrontal cortex, anterior cingulate, lateral parietal, and premotor cortex is activated by contrasts between SI and SO conditions, regardless of difficulty as indexed by response time and error rate. A similar network is seen in the contrast of SOeasy versus SOdifficult. This network overlaps with three partially overlapping networks identified by previous studies: the “task-positive” (Fox et al., 2005), “multiple-demand” (Duncan, 2010), and “frontoparietal control” (Vincent et al., 2008) networks. By contrast, another set of brain regions, including occipital cortex and MPFC, is activated by the contrasts between SO and SI conditions, regardless of task difficulty. The MPFC activation overlaps with the default mode network (Buckner et al., 2008), although other nodes of this network did not show significant signal change at the selected threshold.
We followed up these exploratory analyses by investigating an independently defined region-of-interest based on the peak MPFC co-ordinate from our previous study investigating a version of the present task (0, 64, 26, derived from Gilbert et al., 2005). Mean signal for an 8mm-radius sphere centered on this co-ordinate is illustrated in Figure 2. As in the behavioral data, there was a significant effect of condition [F(2,16) = 14.6, p < 0.001]. However, the pattern of results was clearly different between the behavioral and BOLD data. Whereas the SI condition was intermediate between the other two conditions in both behavioral measures, signal in MPFC was significantly lower in the SI condition than either the SOeasy [t(17) = 4.5, p < 0.001] or the SOdifficult [t(17) = 4.2, p < 0.001] conditions. In addition, signal was slightly higher in the SOdifficult than the SOeasy condition [t(17) = 2.1, p = 0.049]. Thus, insofar as difficulty is indexed by response time and error rate, MPFC signal was higher in the easier condition when comparing SOeasy with SI, but higher in the more difficult condition when comparing SOdifficult with SI or SOeasy.
We next investigated how far these results extended to other regions of the default mode network, defined by nine peak co-ordinates described in the meta-analysis of Laird et al. (2009). Again, results were based on 8 mm-radius spheres centered on the relevant co-ordinates, after conversion from the Talairach co-ordinates reported by Laird et al. (2009) into MNI co-ordinates using the Lancaster transform (Lancaster et al., 2007). The following analyses focus on the contrast between SOdifficult and SI conditions, seeing as it is this contrast clearly distinguishes the two definitions of task difficulty: if activity is suppressed by task difficulty (as standardly measured), this predicts reduced signal for the SOdifficult condition, but if activity is suppressed by transformation of internal representations, this predicts reduced signal for the SI condition. Across all nine regions, there was a significant Region × Condition interaction [F(8,10) = 3.5, p = 0.035], indicating significant regional heterogeneity. This interaction remained marginally significant even after the data for each region were rescaled into z scores based on parameter estimates across participants and conditions [F(8,10) = 2.7, p = 0.07], suggesting that that this result did not arise simply from scaling differences between regions.
In order to explore this regional heterogeneity, Figure 4 illustrates mean signal change in each of the nine “default mode” regions. In each graph, parameter estimates for the SOdifficult and SI conditions are plotted relative to a zero point representing the SOeasy condition. Thus error bars that do not cross zero represent a significant difference from the SOeasy condition. Furthermore, the direct comparison between SOdifficult and SI conditions is represented by the color of the bars (note that the error bars are only informative with respect to the comparison against the SOeasy condition, not the direct comparison between the other two conditions). Of the two medial prefrontal regions defined by Laird et al. one showed significantly greater activity in the SOdifficult than the SI condition [ventral anterior cingulate; t(17) = 2.6, p = 0.017] and the other showed a non-significant trend in the same direction [medial prefrontal cortex; t(17) = 1.7, p = 0.10], consistent with the MPFC results reported above. Furthermore, the posterior cingulate region identified by Laird et al. (2009) showed a borderline significant increase in signal for the SOdifficult than the SI condition [t(17) = 2.1, p = 0.052]. However, four other regions showed significant effects in the opposite direction: precuneus [t(17) = 2.4, p = 0.030], right inferior parietal lobule [t(17) = 2.3, p = 0.032], left middle frontal gyrus [t(17) = 4.2, p < 0.001], and left inferior parietal lobule [t(17) = 5.4, p < 0.001]. Thus it is possible to find regions within the default mode network where activity is preferentially suppressed either by the requirement to transform internal representations, or by conditions with slow response times and high error rates.
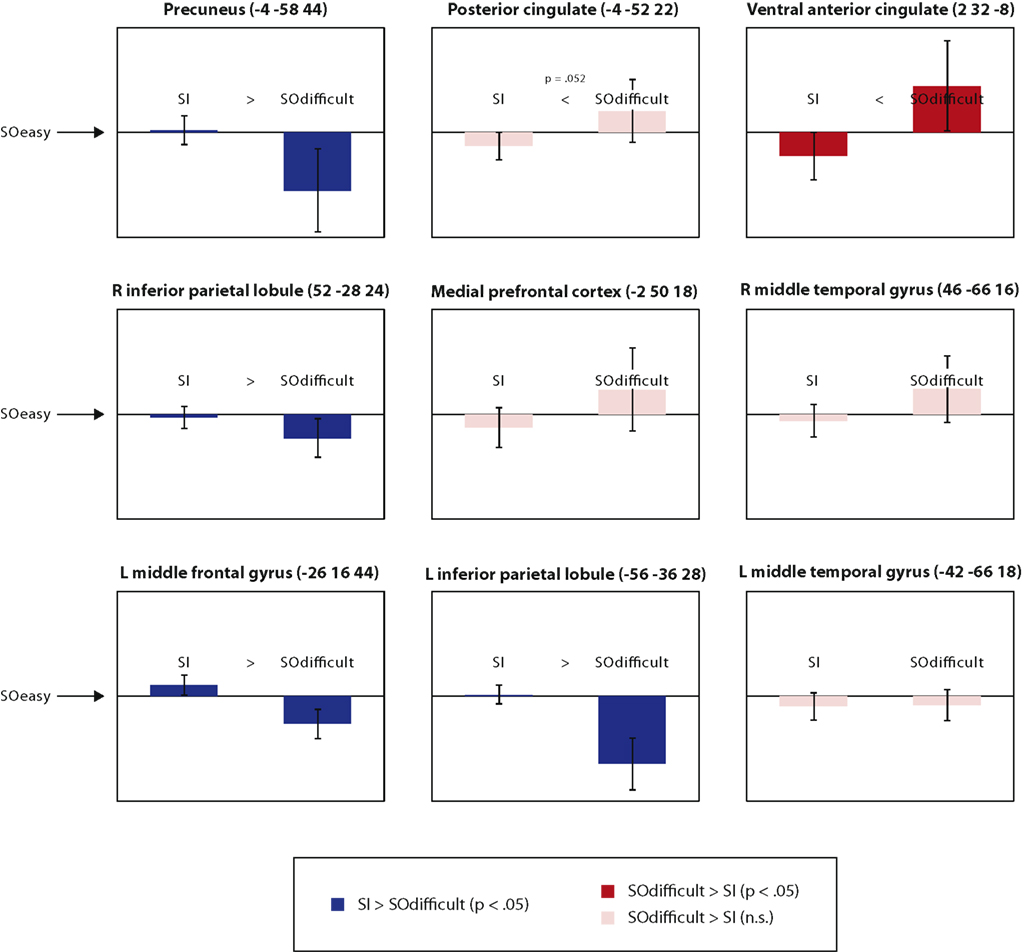
Figure 4. Significant differences in activation between SOeasy, SOdifficult, and SI conditions, in the nine regions of the default mode network identified by the meta-analysis of Laird et al. (2009). All graphs are presented on the same scale, in arbitrary units, with the zero point representing the SOeasy conditions and the two bars representing the other two conditions. Error bars represent 95% confidence intervals for the comparison between each bar and the SOeasy condition. Regions showing numerically greater signal for SI than SOdifficult are presented in blue; regions showing the reverse pattern are presented in red. Note that the locations provided for each region correspond to the Talairach co-ordinates provided by Laird et al. (2009); these co-ordinates were transformed into MNI space in order to perform region-of-interest analyses.
Discussion
In this study we investigated the relationship between activity in regions of the “default mode network” and a standard index of task difficulty: mean response time and error rate. In one prominent region, MPFC, this relationship was non-monotonic. Compared with a task requiring stimulus-independent attention, MFPC activity was greater in both an easier and a more difficult task requiring stimulus-oriented attention. This shows that task difficulty, as standardly defined, is an inadequate predictor of MPFC activity. Instead, at least in some circumstances, the requirement for attention toward and/or manipulation of internally represented information is a better predictor of BOLD signal within this region, with greater signal associated with situations that require attention to be directed toward incoming perceptual information rather than representations decoupled from current sensory input. These findings corroborate the results of Gilbert et al. (2006a) indicating a double dissociation between standard measures of task difficulty and signal change within MPFC. Whereas signal in MPFC was lowest in the condition requiring attention to stimulus-independent information, signal in other regions of the default mode network was lowest in the (stimulus-oriented) condition with the slowest response times and greater error rates. Thus, there was significant heterogeneity between different regions of the default mode network.
Implications for Theories of MPFC Function
What can we learn from these results about the nature of task-induced deactivations in MPFC? First, it is clear that the concept of task difficulty, as standardly defined, provides at best an incomplete means of understanding this phenomenon. One might go beyond this and additionally suggest that, at least in some circumstances, the role of MPFC is to promote attention toward incoming perceptual information rather than internally represented information, seeing as greater signal was associated with conditions requiring greater attention to perceptual information. This contrasts with the standard account that activity in “default-mode” brain regions, particularly MPFC, reflects the occurrence of unconstrained internally directed cognitive processes (Buckner et al., 2008). However, before accepting this conclusion it is important to consider an alternative interpretation. This interpretation relates to the third definition of task difficulty considered in the Introduction, i.e., that difficult tasks are those that most interfere with performance of concurrent tasks. According to this interpretation, it is possible that the SO conditions of the present study were easier than the SI condition specifically in the sense that they could be more readily combined with off-task spontaneous thinking (or “mindwandering”) than the SI condition. In this case, the greater MPFC activation observed in the SO conditions might reflect task-unrelated thought processes rather than anything functionally related to the tasks.
In the behavioral literature on stimulus-independent or task-unrelated thoughts, there is already evidence that gives this possibility some superficial plausibility. For example, the occurrence of such off-task thinking is reduced when participants engage in various working memory tasks that might be considered more similar to the SI condition of the present study than the SO conditions (Teasdale et al., 1993, 1995). However, there are several empirical and theoretical reasons to doubt that the enhanced MPFC activation seen during SO than SI conditions is entirely reducible to the increased occurrence of internally directed off-task thinking in SO conditions. We elaborate on these below:
1. Gilbert et al. (2006a) investigated activity in MPFC during performance of a simple reaction time task requiring participants to respond to an unpredictable visual cue with a button press response. They argued that insofar as MPFC activation reflects the occurrence of off-task thinking, trials with relatively high MPFC activity should be associated with relatively slow response time, seeing as those trials should be those on which participants are most distracted from the task. In fact, the opposite correlation was observed, consistent with a role in directing attention toward perceptual information rather than task-unrelated processes. This is consistent with other studies that have found that MPFC activity is associated with faster RTs in perceptually driven tasks (Small et al., 2003; Hahn et al., 2007). Note that the correlation with trial-by-trial RT fluctuation is not so interpretable in the present study, seeing as variation in RT might reflect differences in the nature of individual trials rather than differences in attentional state. Thus, slow RTs in the present study might reflect particularly difficult trials, or alternatively trials where participants did not attend adequately to the stimuli. In the Gilbert et al. (2006a) study the stimulus, response, and stimulus-response mapping was identical on every trial, removing this ambiguity.
2. Gilbert et al. (2006b) conducted a meta-analysis of functional neuroimaging studies reporting signal change within rostral prefrontal cortex (approximating Brodmann Area 10). Tasks with relatively fast RT (compared with their control conditions) were particularly associated with signal change in MPFC. This could be explained by at least two factors. First, tasks with relatively fast RT might on average involve particularly strong engagement with the external environment, permitting relatively fast responses triggered by salient stimulus features instead of requiring a time-consuming process of transforming internal representations. Second, tasks with relatively fast RT could permit additional time for task-unrelated thinking between responding on one trial and seeing the stimulus on the next. Seeing as some studies involved fixed inter-stimulus intervals, whereas others involved fixed response-stimulus intervals, we were able to deconfound these two factors. When both factors were included in a regression model, fast RTs had a significant association with signal change in MPFC but there was no effect of long response-stimulus intervals. Thus, the opportunity for off-task thinking was not associated with MPFC activity whereas there was an association with tasks that could be responded to rapidly.
3. The idea that SO tasks may activate MPFC due to the task-unrelated processes that tend to accompany them, rather than any intrinsic property of the tasks themselves, may be questioned on a conceptual level. Why might SI tasks suppress the occurrence of concurrent task-unrelated thoughts? Presumably because SI tasks and task-unrelated thoughts compete for an overlapping resource, making it difficult for them to be combined (Teasdale et al., 1995). According to this hypothesis, there is at least one resource that is required by both task-unrelated thoughts and SI tasks, but not SO tasks. But if this resource is supported by MPFC, then the most obvious prediction would be that MPFC should be engaged equally by SI tasks and by off-task thinking during SO tasks, seeing as it is putatively involved in both. Instead, one must explain why MPFC is engaged more by off-task thinking in SO conditions than by SI thinking when it is required by the instructed task. In order to explain this, a more complex position is required. One possibility would be that (1) MPFC supports a process that is engaged more during task-unrelated thoughts than either SI or SO tasks, and (2) a second process is involved in both SI tasks and task-unrelated thoughts, thus suppressing the emergence of task-unrelated thoughts during SI tasks. For example, a model of this type might suggest that both SI tasks and task-unrelated thoughts require the use of an articulatory loop (Baddeley, 1986), explaining why they cannot be combined. Furthermore, it would need to be assumed that an additional process supported by MPFC is engaged primarily by task-unrelated thoughts rather than SI tasks, such as retrieval of autobiographical memories. This is a very complex hypothesis, for which we are not aware of any direct evidence. By contrast, an alternative hypothesis is simply that some SO tasks require a process supported by MPFC to a greater degree than some SI tasks. This hypothesis does not invoke the concept of off-task thinking, and is supported by the empirical findings reviewed above. We suggest that this simpler hypothesis should be preferred in the absence of direct evidence supporting the complex model described above.
Comparison with McKiernan et al. (2003)
The present study follows a similar approach to McKiernan et al. (2003), who conducted a parametric examination of task-induced deactivation related to three indices of task difficulty in an auditory target detection task: stimulus presentation rate, target discriminability, and short-term memory load. In the behavioral data, all three manipulations of task difficulty led to increases in response time and error rate. Unlike the present study, McKiernan et al. (2003) also found that their three operationalizations of task difficulty had broadly consistent effects on task-induced deactivation, i.e., manipulations leading to greater response time and error rate tended to suppress activity in a variety of “default mode” brain regions, including a region labeled left anterior cingulate/superior frontal gyrus which was close to (but not identical with) the region of MPFC identified in the present study. This is particularly surprising seeing as McKiernen et al.’s “target distinctiveness” criterion seems similar to the present manipulation of perceptual discriminability between the SOeasy and SOdifficult conditions. However, the contrast between SOeasy and SOdifficult did not produce task-induced deactivation within MPFC in the present study. How can these results be reconciled?
There are at least two possibilities. First, it should be noted that the left anterior cingulate region reported by McKiernan et al. (2003) was centered posteriorly to the region identified in the present study. Seeing as MPFC exhibits functional specialization on a fine-grained scale (Gilbert et al., 2006c, 2007b, 2010), there is no necessary reason to expect consistent results between nearby but non-identical MPFC regions, although results were consistent between the MPFC regions based on regions of interest defined by our earlier study (Gilbert et al., 2005) and the Laird et al. (2009) meta-analysis. Secondly, the task used by McKiernen et al. involved detection of infrequent targets, rather than a two alternative perceptual discrimination. In such a target detection task, a more difficult perceptual discrimination may lead to increased attention during task performance toward a memorized target representation, in order to detect its occasional occurrence. Thus, it is not clear how well the target distinctiveness criterion of task difficulty used by McKiernan et al. (2003) fits with our distinction between SO and SI tasks. More generally, we do not disagree with McKiernan et al. (2003) that there is often a monotonic relationship between standard measures of task difficulty and task-induced deactivation in functional neuroimaging. Our purpose in the present study is simply to point out that this is not always the case, indicating that standard indices of task difficulty are not sufficient to explain task-induced deactivation in all circumstances.
While the study of McKiernan et al. (2003) suggests that diverse cognitive demands cause relatively consistent patterns of deactivation, other recent studies have suggested that a single measure of cognitive demand is inadequate to explain task-induced deactivation across all regions (Mayer et al., 2010; Harrison et al., 2011). Consistent with this, the qualitative pattern of results in eight of the nine regions shown in Figure 4 was a non-monotonic relationship between BOLD signal and RT/accuracy across the three conditions. Recently, Mayer et al. (2010) investigated a task that manipulated both the difficulty of a visual search to identify targets, and the number of targets that then needed to be remembered over a retention interval. Some regions were deactivated by the two task demands in an additive manner. However, this did not characterize the default mode network as a whole, many regions of which were selectively deactivated by one or the other task demand. In particular, a region of MPFC near to the peak investigated in the present study (−7, 54, 13) was deactivated by the requirement to remember more targets over the retention interval (i.e., greater requirement for attention toward internally represented information) but not by the difficulty of the visual discrimination required to identify targets. The results of Mayer et al. (2010) are thus highly consistent with the present study.
Task-Induced Activations
As well as an MPFC region showing greater activation in conditions requiring strong engagement with perceptual information, a network of predominantly lateral and posterior medial prefrontal and parietal regions was more active in conditions requiring attention toward representations decoupled from current sensory input, showing a mirror image of the pattern of results seen in MPFC. These regions correspond well with those identified as being part of the “task-positive” (Fox et al., 2005), “multiple demand” (Duncan, 2010), or “frontoparietal control” (Vincent et al., 2008) networks. Thus, the present results fit well with the hypothesis of specialization within rostral PFC for attention toward SO and SI information in medial and lateral subregions respectively (Burgess et al., 2003, 2007).
Similar brain regions were activated not only when comparing the SI condition with either of the SO conditions, but also when comparing SOeasy with SOdifficult. Two factors can explain this pattern of results. First, increased activation in lateral PFC when comparing easy with relatively difficult conditions has been reported previously (Christoff et al., 2004; Dumontheil et al., 2010), and attributed to the emergence of off-task stimulus-independent thought in easy conditions. Secondly, in the SOeasy condition of the present study, participants needed to maintain an internal representation of their position in the alphabet, so that they could continue from this point if a question mark was presented in the next trial. By contrast, the SOdifficult condition could be performed on the basis of attention to current perceptual information alone.
Of particular theoretical interest is the pattern of results in posterior dorsal medial PFC, corresponding to anterior cingulate cortex and extending into supplementary motor area. This region was activated both by the contrast of SOeasy > SOdifficult and also by the contrast of SI > SOdifficult. In both of these contrasts, activity was greater in an easier than a more difficult condition, using the standard definition of difficulty. The cognitive functions of this region have been a matter of intense theoretical debate, with theoretical accounts linking anterior cingulate with monitoring for response conflict (Botvinick et al., 2001; Kerns et al., 2004), detecting error likelihood (Brown and Braver, 2005), evaluating action-response contingencies (Alexander and Brown, 2011), and goal setting (Anderson et al., 2009). The present results do not clearly adjudicate between these accounts, although they are perhaps difficult to reconcile with theories in terms of action-outcome monitoring, seeing as the only condition with non-random action-outcome contingencies was the SOeasy condition, yet this condition was associated with anterior cingulate activity that was intermediate between the other two conditions. Recently, Grinband et al. (2011) have suggested that signal change in this region can be explained wholly in terms of response time (i.e., “time on task”). The present study constitutes a clear exception to this suggestion, seeing as greater activity in anterior cingulate could be observed both when contrasting a slower task against a faster one (SI > SOeasy) and also when contrasting a faster task against a slower one (SI > SOdifficult, and SOeasy > SOdifficult).
Are Between-Condition Differences in Task Difficulty a Confound?
Our results are relevant to the debate over whether differences in response time between tasks or conditions should be considered a confound in functional neuroimaging studies, and therefore controlled for. In some circumstances a pair of tasks might require the same underlying cognitive processes or resources, with one task placing greater demands on those resources than the other. In this case, it would be wrong to infer from differences in activation between the tasks that they require qualitatively distinct cognitive processes. Instead, greater activity in the task with slower response time might just reflect “more of the same,” i.e., increased utilization of whatever processes are required by the other task. In such a scenario, it might be appropriate to control for differences in response time between the two tasks (e.g., using the method suggested by Grinband et al., 2008). However, we suspect that more commonly a difference in response time between tasks reflects the engagement of partially distinct cognitive processes. A strong form of this view is the hypothesis of “pure insertion” which underlies interpretation of the subtraction methodology (Friston et al., 1996). Controlling for response time in this situation might end up controlling for precisely the factor of interest, i.e., the cognitive processes that differ between the tasks. It is therefore not always appropriate to control for response time differences between conditions, and this should not be seen as necessarily a confound. In order to make an argument on the appropriateness or otherwise of controlling for response time differences, it is important to carry out task analyses to identify putative cognitive processes that may differ between tasks or conditions. If such differences can be identified, this provides an argument against controlling for response time differences between conditions.
Relationship between MPFC and Other Regions of the Default Mode Network
The present study demonstrated functional heterogeneity between distinct nodes of the default mode network. Unlike MPFC, signal in precuneus, left middle frontal gyrus, and bilateral inferior parietal lobule showed the strongest suppression in the condition with slowest RTs and greatest error rates. Thus, the present results need not rule out the idea that task-induced deactivation in these regions is best predicted by standard behavioral indices of task difficulty. However, the pattern in MPFC was clearly different, consistent with other recent demonstrations of functional heterogeneity within the default mode network (Leech et al., 2011). We therefore conclude that:
1. Standard measures of task difficulty are inadequate as a tool to understand task-induced deactivation in MPFC.
2. In order to better understand the default mode network, rather than investigating the so-called “resting state,” which is likely to reflect a disparate, uncontrolled, and highly variable set of cognitive processes from one study to the next, a better approach may be to investigate well-defined cognitive tasks, in conjunction with a clear task analysis (see Christoff et al., 2009; Stawarczyk et al., 2011 for a related approach).
3. This approach may be expected to help define the contrasting roles of disparate brain regions that typically exhibit relatively high activation during low-demand tasks.
Conflict of Interest Statement
The authors declare that the research was conducted in the absence of any commercial or financial relationships that could be construed as a potential conflict of interest.
Acknowledgments
Supported by the Wellcome Trust (061171 to Paul W. Burgess) and the Royal Society (University Research Fellowship to Sam J. Gilbert).
References
Alexander, W. H., and Brown, J. W. (2011). Medial prefrontal cortex as an action-outcome predictor. Nat. Neurosci. 14, 1338–1344.
Allport, D. A., Antonis, B., and Reynolds, P. (1972). On the division of attention: a disproof of the single channel hypothesis. Q. J. Exp.Psychol. 24, 225–235.
Anderson, J. R., Anderson, J. F., Ferris, J. L., Fincham, J. M., and Jung, K. J. (2009). Lateral inferior prefrontal cortex and anterior cingulate cortex are engaged at different stages in the solution of insight problems. Proc. Natl. Acad. Sci. U.S.A. 106, 10799–10804.
Botvinick, M. M., Braver, T. S., Barch, D. M., Carter, C. S., and Cohen, J. D. (2001). Conflict monitoring and cognitive control. Psychol. Rev. 108, 624–652.
Brown, J. W., and Braver, T. S. (2005). Learned predictions of error likelihood in the anterior cingulate cortex. Science 307, 1118–1121.
Buckner, R. L., Andrews-Hanna, J. R., and Schacter, D. L. (2008). The brain’s default network: anatomy, function, and relevance to disease. Ann. N. Y. Acad. Sci. 1124, 1–38.
Burgess, P. W., Dumontheil, I., and Gilbert, S. J. (2007). The gateway hypothesis of rostral prefrontal cortex (area 10) function. Trends Cogn. Sci. (Regul. Ed.) 11, 290–298.
Burgess, P. W., Scott, S. K., and Frith, C. D. (2003). The role of the rostral frontal cortex (area 10) in prospective memory: a lateral versus medial dissociation. Neuropsychologia 41, 906–918.
Christoff, K., Gordon, A. M., Smallwood, J., Smith, R., and Schooler, J. W. (2009). Experience sampling during fMRI reveals default network and executive system contributions to mind wandering. Proc. Natl. Acad. Sci. U.S.A. 106, 8719–8724.
Christoff, K., Ream, J. M., and Gabrieli, J. D. (2004). Neural basis of spontaneous thought processes. Cortex 40, 623–630.
Churchland, P. M. (1981). Eliminative materialism and the propositional attitudes. J. Philos. 78, 67–90.
Dumontheil, I., Gilbert, S. J., Frith, C. D., and Burgess, P. W. (2010). Recruitment of lateral rostral prefrontal cortex in spontaneous and task-related thoughts. Q. J. Exp. Psychol. (Hove) 63, 1740–1756.
Duncan, J. (2010). The multiple-demand (MD) system of the primate brain: mental programs for intelligent behaviour. Trends Cogn. Sci. (Regul. Ed.) 14, 172–179.
Fitts, P. M. (1966). Cognitive aspects of information processing. III. Set for speed versus accuracy. J. Exp. Psychol. 71, 849–857.
Fodor, J. (1974). Special sciences and the disunity of science as a working hypothesis. Synthese 28, 71–115.
Fox, M. D., Snyder, A. Z., Vincent, J. L., Corbetta, M., Van Essen, D. C., and Raichle, M. E. (2005). The human brain is intrinsically organized into dynamic, anticorrelated functional networks. Proc. Natl. Acad. Sci. U.S.A. 102, 9673–9678.
Friston, K. J., Holmes, A. P., Worsley, K. J., Poline, J. B., Frith, C. D., and Frackowiak, R. S. J. (1995). Statistical parametric maps in functional imaging: a general linear approach. Hum. Brain Mapp. 2, 189–210.
Friston, K. J., Price, C. J., Fletcher, P., Moore, C., Frackowiak, R. S., and Dolan, R. J. (1996). The trouble with cognitive subtraction. Neuroimage 4, 97–104.
Gilbert, S. J., Bird, G., Brindley, R., Frith, C. D., and Burgess, P. W. (2008). Atypical recruitment of medial prefrontal cortex in autism spectrum disorders: an fMRI study of two executive function tasks. Neuropsychologia 46, 2281–2291.
Gilbert, S. J., Dumontheil, I., Simons, J. S., Frith, C. D., and Burgess, P. W. (2007a). Comment on wandering minds: the default network and stimulus-independent thought. Science 317, 43.
Gilbert, S. J., Williamson, I. D., Dumontheil, I., Simons, J. S., Frith, C. D., and Burgess, P. W. (2007b). Distinct regions of medial rostral prefrontal cortex supporting social and nonsocial functions. Soc. Cogn. Affect. Neurosci. 2, 217–226.
Gilbert, S. J., Frith, C. D., and Burgess, P. W. (2005). Involvement of rostral prefrontal cortex in selection between stimulus-oriented and stimulus-independent thought. Eur. J. Neurosci. 21, 1423–1431.
Gilbert, S. J., Henson, R. N., and Simons, J. S. (2010). The scale of functional specialization within human prefrontal cortex. J. Neurosci. 30, 1233–1237.
Gilbert, S. J., Simons, J. S., Frith, C. D., and Burgess, P. W. (2006a). Performance-related activity in medial rostral prefrontal cortex (area 10) during low-demand tasks. J. Exp. Psychol. Hum. Percept. Perform. 32, 45–58.
Gilbert, S. J., Spengler, S., Simons, J. S., Frith, C. D., and Burgess, P. W. (2006b). Differential functions of lateral and medial rostral prefrontal cortex (area 10) revealed by brain-behavior associations. Cereb. Cortex 16, 1783–1789.
Gilbert, S. J., Spengler, S., Simons, J. S., Steele, J. D., Lawrie, S. M., Frith, C. D., and Burgess, P. W. (2006c). Functional specialization within rostral prefrontal cortex (area 10): a meta-analysis. J. Cogn. Neurosci. 18, 932–948.
Grinband, J., Savitskaya, J., Wager, T. D., Teichert, T., Ferrera, V. P., and Hirsch, J. (2011). The dorsal medial frontal cortex is sensitive to time on task, not response conflict or error likelihood. Neuroimage 57, 303–311.
Grinband, J., Wager, T. D., Lindquist, M., Ferrera, V. P., and Hirsch, J. (2008). Detection of time-varying signals in event-related fMRI designs. Neuroimage 43, 509–520.
Hahn, B., Ross, T. J., and Stein, E. A. (2007). Cingulate activation increases dynamically with response speed under stimulus unpredictability. Cereb. Cortex 17, 1664–1671.
Harrison, B. J., Pujol, J., Contreras-Rodriguez, O., Soriano-Mas, C., Lopez-Sola, M., Deus, J., and Menchon, J. M. (2011). Task-induced deactivation from rest extends beyond the default mode brain network. PLoS ONE 6, e22964. doi:10.1371/journal.pone.0022964
Kerns, J. G., Cohen, J. D., MacDonald, A. W. III, Cho, R. Y., Stenger, V. A., and Carter, C. S. (2004). Anterior cingulate conflict monitoring and adjustments in control. Science 303, 1023–1026.
Laird, A. R., Eickhoff, S. B., Li, K., Robin, D. A., Glahn, D. C., and Fox, P. T. (2009). Investigating the functional heterogeneity of the default mode network using coordinate-based meta-analytic modeling. J. Neurosci. 29, 14496–14505.
Lancaster, J. L., Tordesillas-Gutierrez, D., Martinez, M., Salinas, F., Evans, A., Zilles, K., and Fox, P. T. (2007). Bias between MNI and talairach coordinates analyzed using the ICBM-152 brain template. Hum. Brain Mapp. 28, 1194–1205.
Leech, R., Kamourieh, S., Beckmann, C. F., and Sharp, D. J. (2011). Fractionating the default mode network: distinct contributions of the ventral and dorsal posterior cingulate cortex to cognitive control. J. Neurosci. 31, 3217–3224.
Loftus, G. R., and Masson, M. E. J. (1994). Using confidence-intervals in within-subject designs. Psychon. Bull. Rev. 1, 476–490.
Mayer, J. S., Roebroeck, A., Maurer, K., and Linden, D. E. J. (2010). Specialization in the Default Mode: task-induced brain deactivations dissociate between visual working memory and attention. Hum. Brain Mapp. 31, 126–139.
McKiernan, K. A., Kaufman, J. N., Kucera-Thompson, J., and Binder, J. R. (2003). A parametric manipulation of factors affecting task-induced deactivation in functional neuroimaging. J. Cogn. Neurosci. 15, 394–408.
McLeod, P. (1977). A dual task response modality effect: multiprocessor models of attention. Q. J. Exp. Psychol. 29, 651–667.
Miller, E. K., and Cohen, J. D. (2001). An integrative theory of prefrontal cortex function. Annu. Rev. Neurosci. 24, 167–202.
Muraven, M., and Baumeister, R. F. (2000). Self-regulation and depletion of limited resources: does self-control resemble a muscle? Psychol. Bull. 126, 247–259.
Norman, D. A., and Shallice, T. (1986). “Attention to action: willed an automatic control of behaviour,” in Consciousness and Self-Regulation, Vol. 4, eds R. J. Davidson, G. E. Schwartz, and D. Shapiro (New York: Plenum), 1–18.
Shallice, T. (1988). From Neuropsychology to Mental Structure. Cambridge: Cambridge University Press.
Shulman, G. L., Fiez, J. A., Corbetta, M., Buckner, R. L., Miezin, F. M., Raichle, M. E., and Petersen, S. E. (1997). Common blood flow changes across visual tasks. 2: decreases in cerebral cortex. J. Cogn. Neurosci. 9, 648–663.
Small, D. M., Gitelman, D. R., Gregory, M. D., Nobre, A. C., Parrish, T. B., and Mesulam, M. M. (2003). The posterior cingulate and medial prefrontal cortex mediate the anticipatory allocation of spatial attention. Neuroimage 18, 633–641.
Stawarczyk, D., Majerus, S., Maquet, P., and D’Argembeau, A. (2011). Neural correlates of ongoing conscious experience: both task-unrelatedness and stimulus-independence are related to default network activity. PLoS ONE 6, e16997. doi:10.1371/journal.pone.0016997
Teasdale, J. D., Dritschel, B. H., Taylor, M. J., Proctor, L., Lloyd, C. A., Nimmo-Smith, I., and Baddeley, A. D. (1995). Stimulus-independent thought depends on central executive resources. Mem. Cognit. 23, 551–559.
Teasdale, J. D., Proctor, L., Lloyd, C. A., and Baddeley, A. D. (1993). Working-memory and stimulus-independent thought – effects of memory load and presentation rate. Eur. J. Cogn. Psychol. 5, 417–433.
Vincent, J. L., Kahn, I., Snyder, A. Z., Raichle, M. E., and Buckner, R. L. (2008). Evidence for a frontoparietal control system revealed by intrinsic functional connectivity. J. Neurophysiol. 100, 3328–3342.
Vohs, K. D., and Heatherton, T. F. (2000). Self-regulatory failure: a resource-depletion approach. Psychol. Sci. 11, 249–254.
Keywords: default mode, task difficulty, stimulus-independent thought, fMRI, medial prefrontal cortex
Citation: Gilbert SJ, Bird G, Frith CD and Burgess PW (2012) Does “task difficulty” explain “task-induced deactivation?” Front. Psychology 3:125. doi: 10.3389/fpsyg.2012.00125
Received: 07 December 2011; Accepted: 09 April 2012;
Published online: 25 April 2012.
Edited by:
Robert Leech, Imperial College London, UKReviewed by:
Ben Harrison, Melbourne Neuropsychiatry Centre, AustraliaR. Nathan Spreng, Harvard University, USA
Copyright: © 2012 Gilbert, Bird, Frith and Burgess. This is an open-access article distributed under the terms of the Creative Commons Attribution Non Commercial License, which permits non-commercial use, distribution, and reproduction in other forums, provided the original authors and source are credited.
*Correspondence: Sam J. Gilbert, Institute of Cognitive Neuroscience, Division of Psychology and Language Sciences, University College London, 17 Queen Square, London WC1N 3AR, UK. e-mail:c2FtLmdpbGJlcnRAdWNsLmFjLnVr