- 1 Psychological and Brain Science, Indiana University, Bloomington, IN, USA
- 2 Cognitive Science, Indiana University, Bloomington, IN, USA
A growing set of data show that adults are quite good at accumulating statistical evidence across individually ambiguous learning contexts with multiple novel words and multiple novel objects (Yu and Smith, 2007; Fitneva and Christiansen, 2011; Kachergis et al., 2012; Yurovsky et al., under resubmission); experimental studies also indicate that infants and young children do this kind of learning as well (Smith and Yu, 2008; Vouloumanos and Werker, 2009). The present study provides evidence for the operation of selective attention in the course of cross-situational learning with two main goals. The first was to show that selective attention is critical for the underlying mechanisms that support successful cross-situational learning. The second one was to test whether an associative mechanism with selective attention can explain momentary gaze data in cross-situational learning. Toward these goals, we collected eye movement data from participants when they engaged in a cross-situational statistical learning task. Various gaze patterns were extracted, analyzed and compared between strong learners who acquired more word-referent pairs through training, and average and weak learners who learned fewer pairs. Fine-grained behavioral patterns from gaze data reveal how learners control their attention after hearing a word, how they selectively attend to individual objects which compete for attention within a learning trial, and how statistical evidence is accumulated trial by trial, and integrated across words, across objects, and across word–object mappings. Taken together, those findings from eye movements provide new evidence on the real-time statistical learning mechanisms operating in the human cognitive system.
Introduction
Everyday word learning occurs in noisy contexts with many words and many potential referents for those words, and much ambiguity about which word goes with which referent. One way to resolve this ambiguity is for learners to accumulate evidence across individually ambiguous contexts (Pinker, 1984; Gleitman, 1990). Recent experimental studies showed that both adults and young children possess powerful statistical computation capabilities – they can infer the referent of a word from highly ambiguous contexts involving many words and many referents by aggregating cross-situational statistical information across contexts (Fisher et al., 1994; Akhtar and Montague, 1999; Smith and Yu, 2008; Vouloumanos et al., 2010; Scott and Fisher, 2011). The open question is what the responsible learning mechanism is.
One way to attempt to understand this learning process is to start with the simplest mechanisms that are known to exist in the human learning repertoire and see how well these simple and known mechanisms can do. One such possible learning process is Hebbian-like associative learning, a form of learning known to be fundamental to many perceptual and cognitive capabilities (Smith, 2000). In statistical cross-situational learning, a learner could simply store all associations between words and referents. For example, given four words {a, b, c, d} and four visual objects {A, B, C, D} in a training trial, if the learning system stored only associations between words and whole objects, there would be 16 associations formed on trial one (a–A, a–B,…,b–A, b–B, ……, d–A, d–D). On the second trial containing {e, f, d, g, E, F, D, G}, one of the associations (d–D, etc.) would be strengthened more than the others. Across trials, the relative strengths of associations between words and their potential referents would come to reflect the correct word–referent mappings.
Simple associative models such as this have been criticized on the grounds (Keil, 1992) that there are just too many possible associations across situations to store and to keep track of. This raises the key question for the present study: whether learners do not actually store all co-occurrences, but only some of them. If so, on each trial of statistical learning, how much and what kind of information is selected, processed and stored by learning processes? Even if one assumes that the units for learning are whole words (not their parts or phrases) and whole objects (not their parts, properties or sets) and even if one limits the learning environment to that of laboratory cross-situational studies, there are still several words and several referents at each moment and thus potentially many different solutions to information selection. As illustrated earlier, an ideal learner could register all the word–referent pairs on every trial; that is, all the possible hypotheses or associations consistent with the input on each trial might be stored. Alternatively, and consistent with what is known about human attention (Kruschke, 2003; Rehder and Hoffman, 2005), one might attend to only a subset of words and referents, registering just partial information –some words, some referents – from all that is available on a single trial. Selection, could be very narrow (e.g., looking at only one object after hearing a word) or it could be broader. Further, if learners do select just some of the information, what guides information selection? It could be random and unrelated to past experience. Or the learner could attend to words, referents and word–referent pairs based on prior knowledge. Recent simulation studies in Yu and Smith (2012) show that the same statistical computational mechanism can generate dramatically different results depending on the amount and the kind of information selected and used, suggesting the importance of understanding information selection as a critical part of statistical learning mechanisms.
Selective attention is fundamental to almost all learning tasks, which allows learners to focus cognitive resources on vital information (while ignoring unnecessary input), and by so doing facilitate internal cognitive processes. As pointed out in Shiffrin and Schneider (1977), the extent to which attentional resources are necessary during a task greatly depends on the ease of cognitive processing. With multiple words and multiple referents co-occurring within and across multiple trials, successful learning requires considerable attentional effort to perceive, select and then map the phonological sequences of a word with its referent object, focusing on correct word–object mappings while disregarding irrelevant spurious co-occurrences between words and referents. Selective Attention in cross-situational statistical learning can be driven by multiple forces (Kachergis et al., 2012; Smith and Yu, accepted), such as low-level perceptual characteristics of stimuli which do not necessarily have any bearing on building word–referent mappings, familiarity effects of heard words or seen objects, prior knowledge of word–object mappings, internal learning states of word–object pairs, and competition of attention between multiple objects within a learning trial. Influenced by these forces, where learners look reflects, in real-time learning, what information is required from the internal learning processes. Therefore, if we were able to decode their looking behavior, we would advance our understanding of the mechanisms of statistical word learning. Thus, selective attention is so closely tied to real-time learning processes that not only is it driven by statistical learning processes but it also provides input to learning processes to update internal learning states which in turn drive selective attention and information selection in subsequent learning.
Our empirical approach in the present study, then, is to continuously track eye-gaze direction throughout learning as a direct measure of selective attention. The assumption here is that when a learner associates a word with a referent among other simultaneously presented referents, the learner is likely to be preferentially looking toward that referent and this looking behavior indicates that the learner selects this word–object pair to register the association between the two. In this way, different learners may attend to different referents in a visual scene when hearing the same word. Further, by the assumption that learners link the word to the object they are attending to at that moment; these differences in attention will lead directly to different learning results.
Recent psycholinguistic studies already suggest that speech and eye movements are closely linked in both language comprehension and production (Tanenhaus et al., 1995; Griffin and Bock, 1998; Meyer et al., 1998; Griffin, 2004; Trueswell and Gleitman, 2004; Knoeferle and Crocker, 2006). For example, Griffin and Bock (1998) demonstrated that speakers have a strong tendency to look toward objects referred to by speech and that words begin roughly a second after speakers gaze at their referents. Meyer et al. (1998) found that when speakers were asked to describe a set of objects from a picture, they usually looked at each new object before mentioning it, and their gaze remained on the object until they were about to say the last word about it. Several recent developmental studies, though not addressed to the specific questions in this paper, have shown the utility of using these finer-grained real-time measures in studies of early development and learning (von Hofsten et al., 1998; Johnson et al., 2003; Aslin and McMurray, 2004; Trueswell and Gleitman, 2004; Halberda, 2006; Plunkett et al., 2008).
Moreover, Ballard et al. (1997) proposed that momentary eye movements entered directly into cognitive computations, e.g., eye-direction does not just reflect internal cognitive states but directly influences them. Studies in adult category learning showed that learners tend to fixate all stimulus dimensions early in learning but selectively attend to relevant dimensions useful for classification only after errors were largely eliminated (Rehder and Hoffman, 2005). The study was the first to use eye tracking to examine attention in category learning, showing the usefulness of eye tracking for testing existing categorization theories and forming new hypotheses. More recently, Fitneva and Christiansen (2011) used eye movement data to measure word–referent pairs that participants attend at the beginning of training in the cross-situational learning paradigm and showed that inaccurate initial word–referent mappings may actually lead to better learning.
Motivated by those studies, an eye-tracking paradigm is used in the present study of cross-situational word–referent learning which relies on eye movements, and the synchrony of those movements with respect to the heard object names, as a measure of moment-by-moment learning and as a clue to the momentary internal states of the learner. Thus, we will take the presence of eye fixations to spatially separated objects after hearing a spoken word as a proxy measure of attention to those objects, which are tightly tied to and revealing of internal cognitive learning processes. We ask whether learners’ attention to and thus selective storage of word–referent pairs affects learning and if this is so, could eye movement patterns in training be directly related to successful learning at test? If looking does predict learning, then we need to know more about the looking patterns themselves. Accordingly, a major component of the present study is a deeper understanding of the dynamics of those looking patterns, how they change over the course of the learning trials, and how they relate to more or less successful learning outcomes. If learners are not simply passive accumulators of data but instead actively learn by selecting among the available data, information selection becomes a critical component in statistical learning. Even with the same association mechanism to register selected word–referent associations, learners choose some pairings over others to notice and store – and if these pairings guide later selections – then individual learners may distort the regularities in the input both in ways that enhance the learning of the right word–referent pairs and in ways that hinder them. Hence, understanding moment-by-moment selective attention and information selection in statistical learning can shed light on fundamental aspects of cross-situational learning.
Materials and Methods
The stimuli used are exactly the same as those in Yu and Smith (2007) and the current study followed the design from Klein et al. (2008) in which participants were trained to learn a small set of words before cross-situational training. Later, these pre-trained words were mixed with to-be-learned words. There were three purposes of using this design. First, previous results (Klein et al., 2008) show that a small number of learned words can significantly improve overall learning outcomes as participants effectively use these words to reduce the degree of uncertainty in cross-situational learning trials and thus bootstrap statistical learning. The present study intended to replicate such finding. Second, since we know the learning states of these pre-trained words at the beginning of training, we can then measure their looking behaviors toward these pre-trained words and further use these behavioral patterns from pre-trained words to estimate and infer the learning states of other to-be-learned words which we cannot otherwise directly access in the middle of training. For instance, if participants generate similar looking patterns toward a to-be-learned object as what they did toward a pre-trained object, this observation can be used as evidence to infer that they also learned that to-be-learned object in the course of statistical learning. Third, we can investigate the underlying mechanisms of using prior knowledge in cross-situational statistical learning to facilitate the learning of new words.
Stimuli
Word stimuli were 18 computer-generated disyllabic pseudowords pronounced by a computerized voice. Referents were 18 100 × 100 pixel color images of uncommon objects. Each word was randomly selected and paired with an object to form a word–object mapping. In total, there were 18 word–object pairs. These stimuli were taken from a subset of audio–visual stimuli used in one of the five conditions in Yu and Smith (2007).
There were 27 training trials with a total duration of 303.25 s. Each trial simultaneously presented four objects on the screen for 11.25 s; the onset of a learning trial was followed first by a 2250-ms silence and then by the four spoken words. This salience at the beginning of a trial provided enough time for participants to quickly examine the four objects presented in a new trial if they decided to do so. Following that, four words were played sequentially and each said once with a 2250-ms window between the onset of the current word and the onset of the next word. Figure 1 illustrates the timing of words in a trial with gaze data examples from participants. Across trials, the words and the objects were arranged such that there was no relation between the temporal order of the words and the spatial position of the referents. Each correct word–object pair occurred six times in total across the whole training session. The four words and four objects appearing together on a trial were randomly determined. Among 16 possible word–referent associations within a trial, only 4 were correct and the others were spurious correlations of irrelevant words and referents that created within-trial ambiguities.
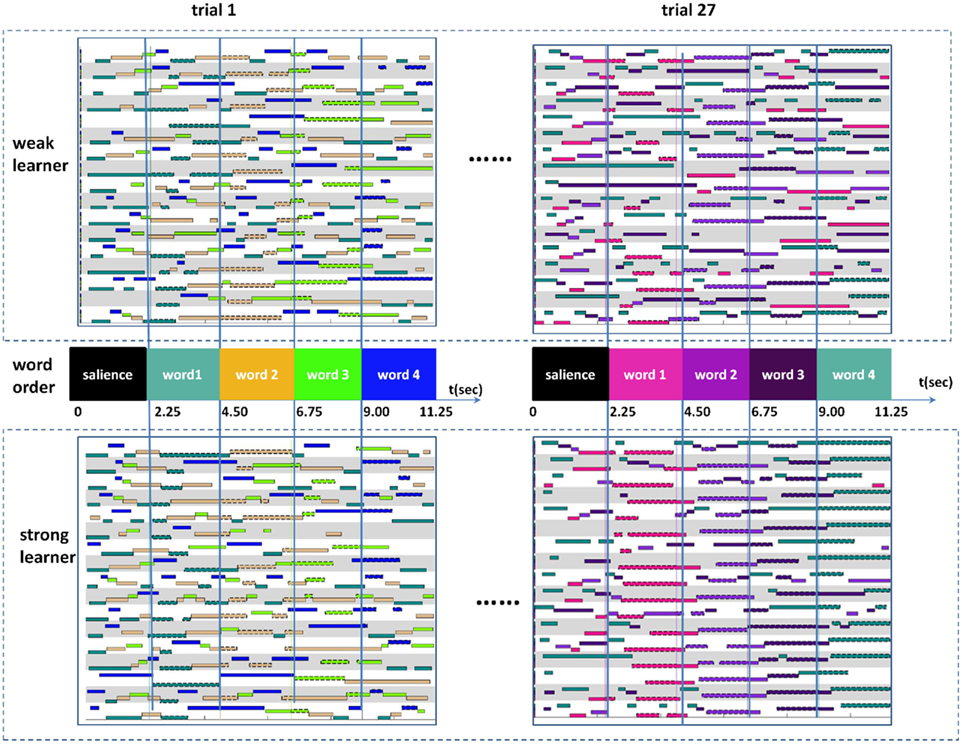
Figure 1. The temporal order of four spoken words is shown in the middle panel. Each trial has a length of 11.25 s which can be decomposed as a salience period at the beginning followed by four word segments each corresponding to one heard word. Examples of gaze data from two learner groups at the first and last (27th) learning trials are shown at the top and bottom panels. Since four objects appeared in each trial, there were four distinct colors in each panel. Each row in a panel shows a temporal sequence of eye fixations from a participant when hearing four words sequentially. Both learner groups started with rather random looks. However, at the last learning trial, strong learners show more consistent gaze patterns toward correct objects after hearing corresponding words.
Apparatus
The learners’ eye gaze was measured by a Tobii 1750 eye tracker (www.tobii.se). The principle of this corneal reflection tracking technique is that an infrared light source is directed at the eye and the reflection of the light on the corneal relative to the center of the pupil is measured and used to estimate where the gaze is fixated. The eye-tracking system recorded gaze data at 50 Hz (accuracy = 0.5°, and spatial resolution = 0.25°) as a learner watched an integrated 17 inch monitor with a resolution of 1280 × 1024 pixels.
Participants
Sixty-four undergraduate students at Indiana University participated in this study for course credit or $8 for their participation. Fine-grained data analyses require reliable eye tracking. In an ideal scenario, a complete eye-tracking session should collect 15,162 (303.25 s × 50 Hz) gaze data points from a participant. In practice, perfect tracking in a continuous mode is not possible due to both participants’ involuntary head movements and technical limitations of the eye tracker. However, the overall tracking results in the present study are quite good – 51 (out of 64) participants reached 85% (roughly 13,000 data points per participant) and therefore were included in the following data analysis.
Procedure
The whole experiment consisted of three consecutive phases. The first phase provided pre-training of three objects. During this phase, three objects were displayed on the computer screen, along with a button labeled “Ready for Test.” Participants were told that they were to learn the correspondence between the referents displayed and the words that correspond with them, and that clicking on a referent would cause its corresponding word to be played over the computer speakers. They were instructed to study these items freely until they were ready to be tested on them. During this test, names for the pre-trained words were played and participants had to select the correct referent for each name from a set composed of three pre-trained objects and five novel referents. These novel referents were not used in any other part of the experiment. If performance at test was not errorless, the pre-training screen was redisplayed and participants were instructed to study more before being retested.
The second phase was identical to the training phase employed by Yu and Smith (2007). A series of training trials were displayed to participants, during each of which four objects appeared on four corners of the screen, and the corresponding names were presented auditorily in a temporal order having no relation to the locations of the referents on the screen. Participants made no responses during this phase; they were simply instructed that they would be trying to learn a set of name–referent correspondences that would include the pre-trained vocabulary. The whole training took about 303.25 s.
The third phase was an 18-alternative forced-choice test. During each test trial, all of the 18 objects in training were displayed on the screen while the name corresponding to one of those referents was played auditorily. Participants were instructed to select the referent of the heard name using a computer mouse. A response was required to advance to the next trial. Every word was tested once; thus, there were 18 test trials in total.
Word Learning Results at Test
Both three pre-trained words and 15 to-be-learned words were tested at the end of training. For pre-trained words, as expected, participants performed very well (Mpre_trained = 91.87%). We have taken this to mean that very little forgetting of these items occurred over the course of training, and that prior knowledge was available throughout the training session. On average, participants learned 58.12% of the to-be-learned words, which was far above chance [t(50) = 12.35; p < 0.001]. However, learning results at test have a rather wide range from 0% (one participant) to 100% (six participants), clearly showing that some participants learned many word–referent mappings close to perfect, some learned quite a few and others learned very few. To systematically analyze their looking behavior in the course of learning, we divided participants into three groups – strong, average, or weak learners – based on their performance at test. We then extracted the eye movement patterns characteristic of these groups during the learning phase, with the goal to compare and discover both shared patterns across groups and different patterns that may contribute to more successful or less successful statistical learning between the groups. The shared patterns reveal general underlying factors and constraints that control learners’ looking behavior in the task, while understanding behavioral patterns of strong learners that support successful learning in comparison to the less success from weak and average learners provides an empirical route to understanding the mechanisms that underlie cross-situational word learning.
The grouping rule was straightforward and meant as an approximate division by learning. More specifically, participants who correctly selected more than 13 out of 18 answers were grouped as strong learners (Mstrong = 91%); those who selected 8 to 13 correct word–referent mappings were labeled as average learners (Maverage = 62%); and the rest who selected correct answers for fewer than 8 words were treated as weak learners (Mweak = 26%). As a result, among 51 adult participants, 17 were in the strong learner group, 17 in the average learner group and 17 in the weak learner group. Note that even weak learners acquired a certain number of words from training which was far above chance [t(16) = 6.95; p < 0.001]. Dividing three learner groups allows us to have a finer-grained distinction to separate learners, which also decreases within-group variations, and meanwhile ensures a sufficient number of participants in each group. This grouping-based approach on analyzing gaze data has been successfully used in previous research (Johnson et al., 2004, 2008; Amso and Johnson, 2006; Yu and Smith, 2011).
Eye Movement Data Processing and Analysis Methods
Learning the mappings between words and referents requires attending to the whole objects as a candidate referent. Therefore, we treated a visual object as a whole and measured sustained attention on individual objects and attention switches between objects (but not at which spatial location or object part a participant was looking). However, participants most often did not fixate on only one specific location of a visual object. Instead, they switched their gaze from one part of the object to another part, yet still be attending to the same object and potentially linking that object to the heard word. To deal with such situation, a region-based fixation finding method was implemented in which we defined four rectangular region-of-interests (ROIs) that cover the areas of four visual objects displayed on screen. Each ROI covers the area occupied by one of four visual objects with a 10-pixel margin along four directions. We then grouped raw eye position data (x and y coordinates) that fell within the same ROI as a single fixation. This process converts continuous gaze data into five categories, namely, four visual objects, or somewhere else. One potential problem with this thresholding-based approach is that it cannot handle data points close to the boundaries of ROIs. For example, in a segment in which all data points belong to a pre-defined ROI except one single data point within the segment that is just out of the pre-defined ROI. This outlier would split the whole segment into two fixations instead of maintaining one big fixation. In order to generate more reasonable results and remove artificial effects from the thresholding method, two additional data processing steps were applied to smooth fixation data. First, we merged two consecutive fixations sharing the same target object into one big fixation if the gap between these two was small enough (<200 ms or 10 data points). This smoothing step was based on the assumption that a short period of time out of a ROI was likely to be caused by artificial effects of the thresholding-based method because a participant was less likely to switch their visual attention to the background (e.g., the middle of the screen with nothing displayed, etc.) and immediately switch back to the target object in such a short period of time. The second step was to remove those short fixations that lasted less than 200 ms (10 data points). Again, we suspected that those transitional fixations were likely caused either by accidental eye-tracking errors or by the thresholding-based fixation finding method and therefore are not relevant to register word–referent associations. The final result of this thresholding and smoothing is an event stream with each fixation entry consisting of three elements (t1, t2, target) representing the onset of a fixation, the offset of the fixation, and the target object fixated upon respectively. Figure 1 shows an example of eye fixation data in which each color represents 1 of 18 visual objects that participants attended trial by trial (4 distinct colors in each panel corresponds to four objects in a trial). Raw fixation data shown in Figure 1 reveal that participants’ looking behaviors were quite dynamic – they actively switched their attention among four objects while perceiving heard words.
From such dense data, the research goal in the analyses was to discover the nature of looking patterns during training, and particularly those that may lead to more successful learning. The statistical analyses and results reported next are based on linear mixed-effects models by using the lmer function of the R package lme4 (Bates and Sarkar, 2007). Unless specified otherwise, each of gaze patterns extracted from raw data is treated as a dependent variable (e.g., fixation duration, longest long time, number of looks). The model included group (strong, average, or weak learners) as a fixed factor. Random effects for subjects, trials and objects were also included to account for any non-independence among different learners’ looking behaviors, among their looks toward different objects, and in different trials (Baayen et al., 2008). All p-values and confidence intervals reported in mixed-model analyses were derived from posterior simulation using the language package (Baayen, 2008), which can be used to assess statistical significance like a standard p-value in t-test and ANOVA.
Results from Data Mining Eye Movement Data
The first empirical question is this: what looking patterns did participants generate in the course of cross-situational learning. As noted earlier, our data analyses are based on the following principle: their looking behavior in this paradigm was driven by spoken words. After hearing a word, participants dynamically allocated their attention between four objects on the screen and thus where they looked indicated what word–object mapping they selected and processed. We also note here it is plausible that participants may occasionally attend to an object while trying to link that object with another word that was not presented at the moment, and more generally, attention can dissociate from eye gaze under certain circumstances (Posner, 1980). But we argue that given the accumulation of empirical evidence using eye tracking in many domains, the interpretation of eye movements as a surrogate measure of attention is a reasonable and feasible idea. Eye movements are most often tightly coupled with attention and immediately driven by on-going audio–visual stimuli and the learning task (Kowler et al., 1995). This assumption is further confirmed by both recent psycholinguistic studies on language comprehension, showing that listeners are likely to look at the target object after hearing its name (Allopenna et al., 1998), and the preferential looking paradigm in developmental studies (Hollich et al., 2000), demonstrating that the object infants choose to attend after hearing a word indicates the knowledge of the association between the two.
Accordingly, we divided a learning trial into four segments, each of which was based on the onset of a spoken word. As shown in Figure 1, eye movements that were generated from the 350 ms following the word’s onset to the onset of the other word for the first three words or the end of the current trial for the last word were grouped together and treated as eye movements driven by the concurrent spoken word. The definition of the relevant window as beginning 350 ms after a word onset is based on the assumptions that it takes at least 150 ms to process and recognize a word and that it takes at least 200 ms for participants to plan and execute an eye movement1. So defined, a whole learning trial was decomposed into four 1900-ms word segments (2250 − 350 = 1900), each of which corresponded to one spoken word. Also note here that there was a 2250-ms salience at the beginning of each trial (before the onset of the first spoken word) which was designed for participants to quickly examine what objects were in a trial before hearing the first word. We found that among 48.51% of the total number of trials (27 trials × 51 participants), learners have briefly attended to all four visual objects before the first word, and among 24.62% of the total number of trials, they attended to three objects in the silence period at the beginning of a trial. These empirical results from participants’ looking behavior further support our assumption that learners’ eye movements thereafter were primarily driven by and dedicated to the learning of spoken words as they already knew what visual objects were presented in a trial.
With the present cross-situational learning paradigm, information selection and information processing may happen at different temporal scales, in different ways and be driven by different nested factors moment by moment (Smith and Yu, accepted; Yu and Smith, 2012). As shown in Figure 2, we proposed a principled way to systematically analyze gaze data at three temporal scales/levels. First, at each word segment level with a temporal window of 1900 ms, we measured participants’ visual attention toward objects as a direct and immediate response to spoken words. Next, at the trial level which was composed of four word segments, we analyzed how information selection was accomplished within a single learning trial with multiple visual objects competing for attention in the context of multiple heard words. Third, we investigated selective attention across multiple learning trials which revealed how participants aggregated statistical information across trials.
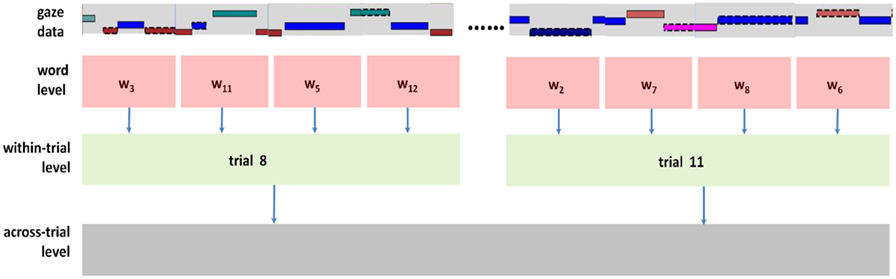
Figure 2. Three levels of eye movement analysis: (1) word level: where participants attended when hearing a word; (2) within-trial level: how they coordinated their attention on objects when hearing several words within a trial; (3) across-trial level: how they adjusted their attention on objects in a new trial based on where they looked in previous trials.
Analysis of Eye Movements at the Word Level
After hearing a word, participants had a temporal segment of 1900 ms to look at visual objects that may link to the heard word before hearing the next word. The first question here is how they distributed their attention in each word segment. Human adults produced on average three fixations per second (Ballard et al., 1997). Given this, each word segment in the present study had enough time for participants to generate multiple fixations and attend to all of the four objects in a trial if they wanted to do so. But effective statistical learning may require their attention to be more selective and more stable. Indeed, we found that the average number of fixations per word segment is approximately two with no significant difference between strong, average and weak learners (Mstrong = 2.05, Maverage = 2.19, and Mweak = 2.15, β = 2.03, p = 0.20). A closer examination revealed that 21.26% of word segments had only one look on a particular object, 47.59% had two looks on two objects, 26.58% had three looks on three objects, and only 4.30% had four looks on all four objects.
The number of looks is just one aspect of the dynamics of eye movements that might be relevant to learning. With the same number of attention switches, learners can generate different looking durations. For example, one group might have more or less uniform looking durations for each attended object. The other group might have a more uneven distribution of looking durations containing one longer fixation with several shorter looks. To capture the dynamics of looking durations, we measured the average length of the longest accumulated look on a particular object for each word segment. The results show that participants spent on average more than 60% of time (1320 ms out of 1900 ms) focusing on one object per word segment, and there is no significant difference between three learner groups (Mstrong = 1360 ms; Maverage = 1260 ms; Mweak = 1300 ms; β = 1.32, p = 0.13).
Previous research studies show that the location and duration of the longest looks reveal learners’ referent decision (Schafer and Plunkett, 1998; Fitneva and Christiansen, 2011). The longer duration that they looked at a particular object, the more likely that they attempted to learn to map that object as the target referent of the concurrent word compared with objects that were fixated less frequently and with shorter durations. In light of this, we next measured the proportion of word segments that contained more than 1200 ms of the accumulated look on a particular object; that is, an object was attended for more than 60% (1200 ms/1900 ms) of the time after hearing a word. On average, there were 61.76% of word segments for strong learners, 52.12% for average learners and 44.12% for weak learners, in which participants selected and fixed on one particular object for longer than 1200 ms after hearing a word. Figure 3 shows the proportion of long look word segments based on word occurrence as each word appeared 6 times throughout the whole training. Note that the order of word appearance is closely related to the trial order – the first appearances of 18 words are always in the first several training trials and the last appearances of words are always at the last few training trials. For average and weak learners, there are no differences between when a word was heard the first time and when a word was heard the last (sixth) time (βaverage = 0.01, p = 0.32; βweak = 0.01, p = 0.42). Strong learners, on the other hand, generated more long accumulated looks at the end of training (from 53.59 to 75.16%, βstrong = 0.05, p < 0.001). A longer fixation indicates more stable attention on a particular object when hearing a word. More stable attention from strong learners can be viewed as either the cause or the outcome of successful learning – a question we investigate with further data analysis in the next section.
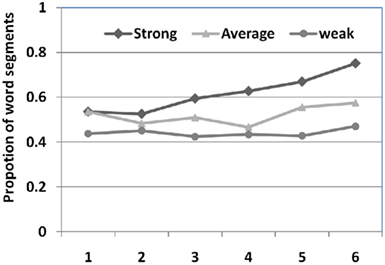
Figure 3. The proportion of word segments containing a long accumulated look on an object (>1200 ms) after hearing a word. Each word appeared six times in training. For all three groups, more than 40% of time they generated a long look throughout the training. In addition, strong learners gradually produced more and more long looks (75% at the end of training) while average and weak learners did not show the same trend.
The results so far show the overall patterns of their looking behavior – how long they looked at objects and how frequently they switched their attention, but do not have information on where they looked. In particular, the relevant question to statistical word learning is whether they looked at the correct object after hearing a word. Figure 4 shows the proportion of time looking at the correct object after hearing a word. There is no difference between three learner groups in the first and second occurrences of a word (β1-appearance = 0.01, p = 0.31; β2-appearance = −0.03, p = 0.12). After the second appearance, their looking patterns began to diverge. Average and weak learners generated fewer looks toward the correct objects compared with strong learners who increasingly looked at the correct object for the heard word while they were exposed to more statistical evidence of those word–referent correspondences. At the end of training, strong learners spent almost 75% of time looking at the target object after hearing a word while weak learners spent only less than 40% of time on the target object (β6-appearance = −0.145, p < 0.001). This dramatic difference between strong and weak learners directly reflects their learning performance at test. Also note that average learners showed a similar trend toward looking at correct objects more (βaverage = −0.03, p < 0.005), but not as significantly as strong learners did (βstrong = 0.12, p < 0.001). Overall, the results indicate that all learners started by randomly selecting candidate objects (which may or may not be correct) after hearing a word. This rules out one plausible explanation of successful learning – initial information selection determines more or less successful learning – thus, strong learners happened to select correct ones to start with and therefore can easily confirm these correct word–referent mappings in later learning, while average and weak learners happened to randomly select wrong ones and had to recover and correct these wrong selections in subsequent learning.
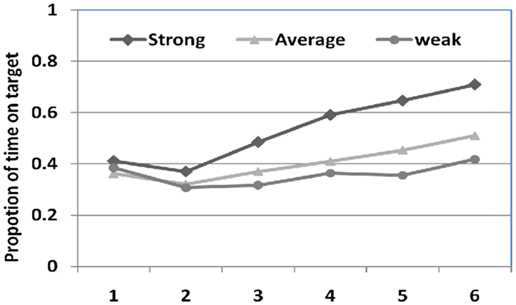
Figure 4. The proportion of time on target after hearing a word. Strong learners looked more and more toward correct objects in the course of statistical learning while average learners showed the similar trend. However, weak learners did not show any linear increases.
To summarize the results of gaze patterns at the word-segment level, participants did not look randomly when hearing a word. Instead, their attention is selective and most often they spend a larger proportion of time on a single object after hearing a word. All learners, no matter if they were more or less successful in statistical learning, revealed several similar characteristics in their visual attention at this level, such as the number of fixations per word segment, and the duration of the longest accumulated look on a particular object. However, strong learners tended to look more toward the target object after hearing a corresponding word. What might these patterns mean? One possibility is that all learners are alike at the beginning because they enter the task with the same knowledge, knowing three pre-trained words but not knowing any of the to-be-learned word–referent pairs. All learners on the initial trials must randomly sample some word–referent pairs by preferentially looking at one of the four objects when hearing one of the words. They may all start this sampling process in a similar way and thus there are no differences in their eye movement patterns. The diverging patterns of learning that then follow are built upon this initial information selection which sets up different learning trajectories to ultimately lead to either more successful or less successful resolution of the statistical ambiguities inherent in the learning trials. However, our results rule out this possibility as both learners that acquire more words and learners that acquire fewer words have similar looking behaviors at the beginning of training. Given that they seem to have a similar start, a new conjecture is that with similar initial random guesses/looks, how learners select and integrate statistical information in subsequent learning, both across multiple words in a trial and across trials, may be the key for successful statistical learning. With this conjecture in mind, we next examine looking behavior within a learning trial – how multiple objects within a learning trial may compete for attention with co-occurring words.
Analysis of Eye Movements within a Trial
As reported earlier, participants were likely to generate a long look at a particular object after hearing a word, which may be directly related to register a word–object association while other short looks within a word segment may be transitional and sporadic. Each learning trial is composed of four word segments. Within each trial, participants produced on average 2.33 long looks (> 1200 ms). How did they distribute more than one long look in each trial? Did they pay more attention to the same object across multiple word segments in a trial?
As shown in Figure 5, in less than 10% of trials across all three learner groups, participants did not generate any long fixation on any particular object in a trial (Mstrong = 5.22%; Maverage = 7.84%; Mweak = 7.18%; β = −0.04, p = 0.53). Meanwhile, in about 20% of trials, they generated only one long fixation within a trial (others are short fixations on the rest of three word segments, Mstrong = 18.74%; Maverage = 17.42%; Mweak = 23.97%; β = −0.08, p = 0.23). For the rest of the cases with more than one long look per trial, both strong, average and weak learners rarely looked at the same object more than once in different word segments (Mstrong = 2.17%; Maverage = 5.66%; Mweak = 3.92%). Further, strong learners are less likely to do so than average and weak learner (β = 0.09, p = 0.16). Instead, participants most often attended to different objects with long looks when hearing different words (Mstrong = 73.86%; Maverage = 69.06%; Mweak = 64.92%). This suggests a mutually exclusive looking behavior within a trial – looking at different objects in different word segments, and by doing so each object receives at most one long look in one of the four word segments. In addition, even though all learners followed a similar trend, strong learners showed a stronger mutual-exclusivity effect within a trial than average and weak learners (β = −0.03, p < 0.01), indicating that selective attention based on within-trial mutually exclusive looks is an important component in statistical computation and may directly contribute to successful learning.
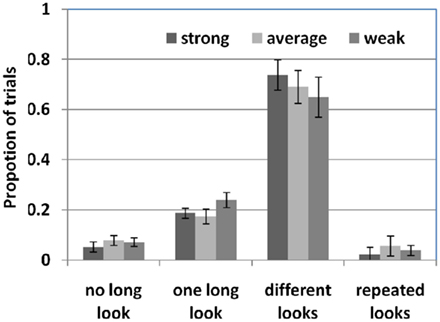
Figure 5. The proportion of trials with long looks across multiple word segments within a trial. There are four trial categories: (1) no long look in a trial; (2) only one long look in one of the four word segments; (3) more than one long look on different objects when hearing different words; and (4) long looks on the same object across different word segments.
Another way to measure the distribution of their attention within a trial is to ask whether they attended to all of the four objects presented. Figure 6 shows the results using two thresholds – 500 and 1000 ms, measuring the proportion of trials that participants at least spent a certain amount of time on each of four objects in a trial. For example, in more than 90% of trials (Mstrong = 94.71%; Maverage = 94.11%; Mweak = 94.55%), learners from all three groups spent at least 500 ms on each of the four objects in a trial and on average they spent more than 1000 ms on each object in about 70% of trials (Mstrong = 79.73%; Maverage = 72.11%; Mweak = 74.72%). There are no differences between different learners groups (β500ms = −0.001, p = 0.94; β1000ms = −0.02, p = 0.32), suggesting that these patterns capture fundamental properties of their selective attention and information selection, no matter they learned more or fewer words at the end.
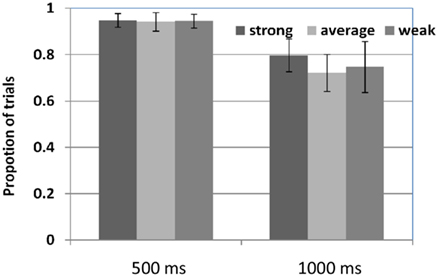
Figure 6. The proportion of trials that participants paid attention to each of four objects for at least 500 or 1000 ms.
In summary, selective attention and information selection within a trial follows the form of mutual exclusivity at two levels. Fist, at the trial level, participants’ attention was more or less evenly distributed over all of the four objects instead of focusing on one to two particular objects. Second, at the word level, they tended to attend to different objects when hearing different words. If an object has already been attended in a previous word segment, the same object would not be attended again in any next word segment of the same trial.
The design of the experiment included 3 (out of 18) pre-trained words and these three words were mixed with other 15 to-be-learned words in the learning session. Given that participants already knew three word–referent mappings at the beginning, this design allows us to systematically study how learners distributed their attention within a trial with a mixture of pre-trained and to-be-learned words. Figure 7A shows the proportion of time that participants looked at the target object when hearing a pre-trained word with two noticeable patterns: (1) participants did attend to the target object which is consistent with the finding in language comprehension – listeners tend to look at the referred object when they hear the object name (Allopenna et al., 1998); they spent, on average, a significant proportion of time on the pre-trained objects (Mstrong = 46.46%; Maverage = 36.29%; Mweak = 41.05%, β = −0.02, p = 0.24) when hearing pre-trained words; (2) participants also distributed their attention on other to-be-learned objects. One plausible explanation is after participants heard a pre-trained word, they first looked for the target object to confirm the correct word–referent mapping that they have already learned. Thereafter, they used the rest of the time to study new objects even though they knew those objects should not go with the pre-trained word. This attention strategy seems to be more effective than spending all of the time on looking at and confirming pre-trained word–object pairs (that they already knew). Instead, attending more to to-be-learned objects (even without correct words heard at the moment) may help learners to recognize and memorize those objects better for later learning. This observation can also be explained as visual novelty effects toward new objects in this context. That is, speech-driven visual attention and visual novelty effects jointly control learners’ attention. When they heard a to-be-learned word, both novel words and novel objects pulled their attention toward novel objects. When they heard a pre-trained word, they first attended to the corresponding pre-trained object as a response to the heard word, but thereafter their attention was attracted by the novel objects presented in the same trial. According to this explanation, strong learners, who presumably already learned most (if not all) word–object pairs at the end of training, would look more toward the correct object (but not other objects) since the novelty effects of to-be-learned objects diminished as learning proceeded. That is, at the end of training, their attention was primarily driven by spoken words for strong learners, while these two forces still competed for attention in the cases of weak and average learners who have not yet acquired all the word–object mappings. Indeed, our prediction was confirmed by empirical data. Participants in the strong learner group tended to look more toward correct objects at the end of training (from 42.12 to 75.25%, β = 0.06, p < 0.001) while weak learners seemed to maintain unchanged patterns from the beginning to the end (from 48.32 to 53.18%; β = 0.01, p = 0.33). Note that average learners had a moderate increase (from 35.23 to 55.24%, β = 0.02, p = 0.02).
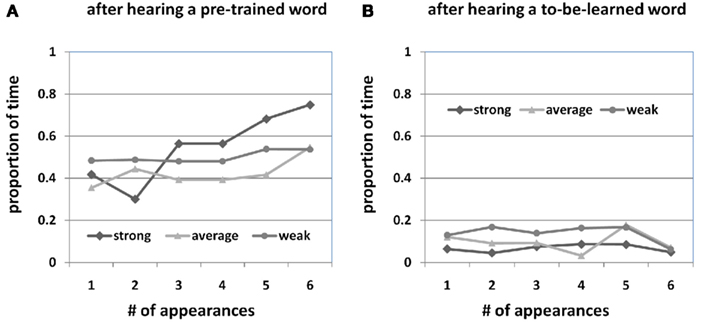
Figure 7. The proportion of time looking at pre-trained objects when hearing pre-trained words (A) and to-be-learned words (B).
However, there is an alternative explanation of gaze patterns from participants when hearing pre-trained words. They may not remember pre-trained word–object associations and failed to successfully identify the correct object. Therefore, they looked at both the correct object and other objects in a trial, considering both as candidate referents of a pre-trained word. One way to distinguish these two explanations is to examine whether they looked at pre-trained objects when hearing to-be-learned words. If they knew the pre-trained object should go with a pre-trained word, when hearing a new word, they should be less likely to consider pre-trained objects as a candidate of the new word and therefore they should look more toward novel objects instead of pre-trained objects (Markman, 1992). Figure 7B shows the proportion of time looking at pre-trained objects after hearing to-be-learned words. Strong learners looked at pre-trained objects only 6.74% of time compared with a 25% chance (four objects in a trial, β = −0.19, p < 0.001). Even average and weak learners looked less toward a pre-trained object (Maverage = 9.53%, β = −0.17, p < 0.001; Mweak = 12.12%, β = −0.15, p < 0.001), showing a preference for to-be-learned objects when hearing a to-be-learned word. A similar finding was reported in many developmental studies of young children who map a novel word onto a previously unnamed object (Golinkoff et al., 1992; Halberda, 2006). In summary, in the learning trials with a pre-trained word, participants in all three groups tended to briefly check the target object when hearing a pre-trained word and thereafter attended to other to-be-learned objects. When to-be-learned words were heard, they tended to not look at pre-trained objects but only focus on to-be-learned objects as candidate referents. This result suggests that learners use prior knowledge as the mutual exclusivity constraint within a trial, assuming that one word goes with one object and therefore to-be-learned words should go with to-be-learned objects but not pre-trained objects. By so doing, prior knowledge of correct word–object mappings limits the number of candidate objects for each to-be-learned word and reduces the degree of uncertainty within a trial to facilitate cross-situational learning.
Putting together the results from within-trial data analysis, we conclude that participants from three learner groups shared similar looking patterns and their attention within a trial demonstrates mutual exclusivity in their information selection, suggesting that the ME constraint is clearly a part of statistical computation. Further, the ME constraint is implemented through external information selection. ME is most often considered as a constraint or an inference in internal computations (Halberda, 2006). In this top-down view, external information selection can be viewed as reflecting the outcome of internal ME-based computations and inferences as the internal learning mechanism controls where and how participants should allocate their attention when hearing a word. Alternatively, the exact same outcome can be achieved through information selection itself. Thus, selective attention can be viewed as a part of computation – implementing the ME constraint in a bottom up way, by selectively attending to certain word–referent pairs before such information is fed into an internal learning mechanism. For example, mutually exclusive long looks across multiple word segments within a trial can emerge from participants’ preference to attend to novel objects that have not attended before when hearing a new word – a novelty effect at the perceptual level. In this bottom-up view, since the outcome from information selection already provides ME-compliant input to further internal computations, there is no need to add the ME constraint as a part of internal computations. Further, compared with an internal mechanism to enforce ME, an external solution through selective attention can be more efficient by reducing the computational load in internal learning processes.
As further studies are needed to explicitly test these two plausible explanations, at the very least, the results here further highlight the importance of selective attention and information selection in understanding learning mechanisms – they not only provide input to internal learning processes but they are a part of learning processes. Toward this goal, however, the consistent results of looking behavior from three learner groups cannot explain away the differences between strong and weak learners – an open question that leads to the next analysis on selective attention and information selection across trials.
Selective Attention across Trials
In the cross-situational learning paradigm, learners cannot figure out correct word–referent mappings from a single trial. Instead, cross-trial statistics need to be selected, processed, and aggregated to lead to successful learning. Hence, it is critical to understand how selective attention and information selection in the present trial depends on where they looked before. At the beginning of training, after hearing a new word, statistical learners had to select one or more objects to attend from all the novel objects in a trial without any prior knowledge which to-be-learned word goes with which novel object. Their initial guess/selection may be right or wrong. However, as learning proceeds, given that the learner already paid attention to a certain object when hearing a word, would they attend to the same object again if that object co-occurs with the word in a new trial. We hypothesized and tested two potential effects through which prior knowledge and looking behavior from previous trials may influence selective attention and information selection in subsequent learning.
Familiarity effects to confirm previously attended word–referent pairs
If the learner paid attention to an object after hearing a word in previous trials, the learner is likely to continue to attend to the same object after hearing the same word again in subsequent learning but not switch his attention to other co-occurring objects. This is evident from the results shown in Figure 8 which divided gaze patterns into four cases based on two factors – whether statistical learners looked at the same object that they attended to before when hearing the same word and whether the repeatedly attended object was correct: (1) repeated/correct: when hearing the same word again, participants generated a long look (using a 1200-ms threshold as before) at the object that was attended previously, and that repeatedly seen object was the correct referent of the heard word; (2) repeated/incorrect: when hearing a word again, participants looked at the same object as before but that repeatedly seen object was not the correct referent; (3) new/correct: even though one of previously seen objects co-occurred with the same word in the present trial, participants selected a new object to attend instead and the newly selected object was the correct referent of the heard word; (4) new/incorrect: Participants selected a new object in the present trial which was not the correct referent. Figure 8 shows the results from these four cases by computing the proportions of word segments that participants either looked at repeated objects, or ignored repeated ones and instead switched to attend new objects.
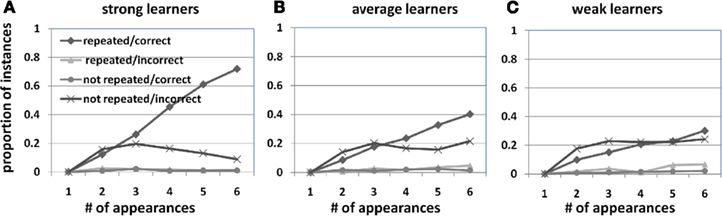
Figure 8. Looking behavior across trials organized by object/word appearance for strong (A), average (B), and weak learners (C). When hearing a word, participants may repeatedly look at the same object that they attended before, or they may decide to look at a new object instead. Meanwhile, whether repeated or not, the attended object in the current trial may or may not be correct. Taken together, there were four possible gaze patterns across trials.
Since this measure is based upon where they looked previously when hearing a word, as shown in Figure 8, all learners started from 0 in the first appearance of a word with no previous experience. Starting from the third appearance and after, two dramatic differences between strong and weak learners were shown. First, strong learners were more likely to look at the object they were attending to before when hearing the same word. That is, if the same object appeared again with the word, strong learners preferred to look at that object (from 12% in the second appearance to 72% in the last appearance). There were significant effects of learner groups (β = 0.08, p < 0.001) and appearance (β = 0.11, p < 0.005). In particular, with each additional appearance, there is a significant increase of repeatedly looking toward correct objects generated by strong learners (β = 0.23, p < 0.001). At the perceptual level, this can be explained as familiarity effects across trials – looking at the familiar object when hearing a familiar word. At the cognitive level, this can be explained as when learners paid attention to the word–referent pair in previous trials, they built working memory representations of the stimulus which can be viewed as an initial association or hypothesis; in other words, the repeated word–referent pair allowed learners to confirm the initial hypothesis or strengthen the initial association (Schöner and Thelen, 2006; Turk-Browne et al., 2008). Meanwhile, the likelihood of looking at non-repeated objects decreased over the course of learning for strong learners (β = 0.02, p < 0.001). Note that in the context of statistical learning, correct word–referent pairs repeatedly co-occurred together more often than incorrect mappings, therefore the chance that repeated objects were correct was much higher than non-repeated ones. For this reason, both repeated/incorrect and non-repeated/correct cases rarely happened. In contrast to strong learners, weak learners tended to look more to new objects but not repeated ones, and therefore they were less successful in looking at correct ones (repeated ones were more likely to be correct while non-repeated ones were more likely to be incorrect). The results in Figure 8 also show that average learners seemed to be in between strong and weak learners – their attention to repeated ones increased but not as dramatically as the one from strong learners did (β = 0.14, p < 0.001). Putting together this result with those from the word-segment and within-trial levels, we suggest that successful learning seems to critically rely on integrating information across trials. Strong learners were able to keep track of information attended to in previous trials and used that information to guide attention and learning in subsequent trials. By so doing, they successfully integrated statistical evidence across multiple trials to gradually converge to correct word–referent mappings.
Novelty effects in new information selection
If the learner attended to an object after hearing a word in a previous trial, but the previously seen object did not appear in the current trial with the target word, then the learner had to select other objects to attend. Which object(s) should be chosen in this context? One principled way to do that is to select objects that have not been attended before when hearing other previous words. For example, assume that a learner hears a word “a” with four visual objects {A B C D}. If the learner has already attended to {C D} in previous trials when hearing other words, participants should select a novel object {A or B} as a candidate referent for the novel word “a.” This can be viewed of applying the mutual exclusivity principle across trials – resulting in novel words mapped to novel (previously not attended) objects across trials. Four selection strategies are defined based on ME and correctness: (1) ME/correct: participants decided to attend to a new object which has not been previously attended to and this selected object was the correct referent of the heard word; (2) ME/incorrect: participants attended to a new object that has not been attended previously, and that object was not the correct referent of the heard word; (3) Not ME/correct: participants selected an object which has been attended to in previous learning trials when hearing other words, and that object was correct; (4) Not ME/incorrect: participants selected a previously attended object which was not correct. As shown in Figure 9, there are no significant differences in three out of four strategies (except for not-ME/incorrect) between strong, average and weak learners. More specifically, at the beginning, they all randomly selected an object when hearing a word which may or may not follow ME. There are no effects of learning group in the first appearance (β = 0.05, p = 0.34). Thereafter, however, average and weak learners (compared with strong learners) were more likely to select not-ME objects and those objects were likely to be incorrect (β = 0.03, p < 0.001). Taken together with the results shown in Figure 8, strong learners attended more to objects that they previously attended and they seemed to rarely attend to the same object that was previously selected when hearing other words. In contrast, when hearing a word, average and weak learners were more likely to select an object that they have attended before (not-ME) when hearing other words in previous trials. Consequently, these not-ME selections were more likely to be incorrect which caused less successful learning.
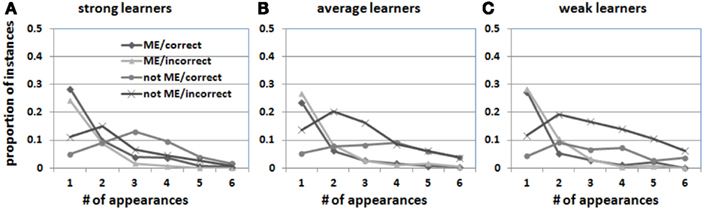
Figure 9. Looking behavior across trials organized by object/word appearance for strong (A), average (B), and weak learners (C). When previous attended objects were not in the current trial co-occurring with the target word, participants had to select a new object. This selection can be based on not choosing the objects that have already associated with other previous words (ME constraint) or choosing ones that have already attended before when hearing other words. Four gaze patterns were shown in three learner groups based on ME and correctness.
In summary, even though all learners started with randomly selecting objects when hearing a word, strong learners were capable of remembering what they have attended before and they tended to attend to the same objects repeatedly if these objects co-occurred with the same words. By doing so, they created a “rich get richer” effect through selective attention and information selection which ultimately led to successful learning. In contrast, weak learners did not seem to be able to recall (or reluctant to attend to) the objects that they attended before, and selective attention and information selection in weak learners seemed to be more or less isolated trial by trial without showing a sign of using prior knowledge accumulated from previous trials to guide information selection in subsequent trials. This capability of integrating and using information across trials seems to be the key to statistical word learning.
General Discussion
Table 1 summarizes the major findings from analyzing gaze data at three temporal levels in the course of cross-situational learning. In the following, we consider the implications of these findings for understanding how information selection operates in real-time learning, and, in particular, for understanding different principles of attention at different temporal contexts, the role of initial states of learning, and how information is aggregated as learning proceeds.
Attention at Multiple Temporal Levels
One critical question in cross-situational learning is how participants may aggregate information within and across individual trials. A better understanding of this topic may shed light on on-going debates about the nature of fundamental learning mechanisms, e.g., hypothesis-testing vs associative learning debate (Colunga and Smith, 2005; Smith et al., 2006; Medina et al., 2011; Yu and Smith, 2012; Yurovsky et al., under resubmission). The results of gaze data analyses at three temporal scales, from a word level, to a trial level, and finally to an across-trial level, show that attention is controlled by several nested factors. However, at each level, there seems to be one dominating factor/constraint that controls learners’ attention. More specifically, at the word level, learners most often selectively pay attention to one object after hearing a word. At the trial level, learners distribute their attention on all of the four objects. For example, given four words {a b c d} and four objects {A B C D}, if a learner happens to take a long look at object A after hearing a, then the learner is less likely to look at A again when hearing any other words. This attention mechanism can facilitate learning if the previously attended pair is correct (e.g., A–a). Therefore, the learner can use prior knowledge of some learned pairs in a trial to limit the candidate referents for a new word. However, the same mechanism may also hinder learning if the previously attended pairs are not correct. Finally, across trials, strong learners use knowledge gained from previous trials to guide selective attention in the current trial which gradually leads to more looks toward correct objects.
Putting everything together, we can see how the above factors/constraints at different levels may work together as an integrated learning system. First, across-trial aggregation allows strong learners to pay more attention to pairs that they paid attention to before – those pairs are more likely to be correct as correct word–referent pairs co-occur more frequently than spurious pairs do. In addition, within-trial ME at the trial level can work together with across-trial integration as a complimentary way to further propagate previously accumulated knowledge to not only just confirm already learned pairs but also generate knowledgeable guesses by linking new words with to-be-learned objects in the trial, from familiar-word-to-familiar-referent to novel-word-to-novel referent. By so doing, strong learners achieve better learning performance since the sensitivity to across-trial statistics should lead to correct word–referent pairs which co-occur more frequently than incorrect ones, and furthermore correct pairs inferred from across-trial statistics can facilitate the learning of new pairs in the trial through within-trial ME. This explanation supports an associative account with cross-trial integration and within-trial competition. Indeed, recent associative models of cross-situational learning have implemented these principles through various routes (Siskind, 1996; Yu, 2008; Frank et al., 2009; Fazly et al., 2010a,b; Nematzadeh et al., 2011; Kachergis et al., 2012; Yurovsky et al., under resubmission). However, it is not clear that a single hypothesis model without integrating information within and across trials can explain empirical findings of gaze data reported in the present study (Smith et al., 2006; Medina et al., 2011). Even though the results here cannot rule out other possible learning mechanisms, any valid mechanism or theory should be able to account for behavioral data by offering a mechanistic explanation. Hence, fine-grained gaze patterns extracted from the present study pose a challenge for both associative and hypothesis-testing models to account for micro-level gaze behaviors in the course of statistical learning (e.g., shown in Figure 1) – modeling not only just test results but also moment-by-moment attention (Siskind, 1996; Smith et al., 2006; Yu, 2008; Frank et al., 2009; Fazly et al., 2010b). A computational model that can explicitly model both real-time attentional processes and latent learning states will give us a leap to understand the mechanisms of moment-by-moment and trial-by-trial cross-situational learning (Kachergis et al., 2012).
Accuracy of Initial Information Selection
In the present study (and as well as in previous ones), participants as a group learned a number of word–referent mappings through a brief training. Meanwhile learning performance reveals individual differences – some learners were able to acquire a larger number of words while others could learn only a few. There are two plausible explanations of individual learning results. One is that all learners follow the same learning strategy to start with. However, strong learners happen to select correct word–referent pairs by chance and this good first guess bootstraps learning as they can quickly confirm and strengthen correct mappings through repeatedly seeing the same object while hearing the same word. In contrast, weak learners with the same learning strategy may start with linking wrong pairs. Therefore, in the next encounter of a word, the best they could do is to identify the wrong pair (the previously attended object does not co-occur with the word) and replace it with a new hypothesized pair which requires further evidence to confirm. In this way, the same learning mechanism may naturally create individual differences in learning performance, building on the accuracy of word–referent selection at the beginning of learning. Alternatively, the other possibility is that bad initial guesses may lead to better learning. This idea was evidenced by a recent study (Fitneva and Christiansen, 2011) showing the initial accuracy of word–referent mappings was negatively correlated with test performance. The result is explained as participants with incorrect initial word–referent mappings tend to engage in more systematic and elaborate processing (Oppenheimer, 2008) and adopt a more analytical approach which results in better learning. In addition, if participants are more sensitive to disconfirming than confirming evidence when they accumulate cross-situational statistics, then inaccurate initial word–referent mappings may actually benefit learning.
However, the results from the present study do not support the above two accounts. Instead, as we reported earlier, various measures of gaze data (e.g., shown in Figures 3–6), such as long looks toward correct objects, proportion of long looks, and probability of looking at the objects previously attended, show no difference between strong and weak learners at the beginning of learning (e.g., the first and second appearances of word–referent pairs). Their looking behavior begins to diverge later as learning proceeds. Therefore, how they start may not matter much for successful learning. Instead, what may really matter is how they aggregate information over cross-situational trials which leads to the different learning outcomes – the topic discussed next.
We suggest that the differences between our findings and the results reported in Fitneva and Christiansen (2011) can be caused by the different degrees of within-trial uncertainty in the designs of the two studies. In their experiment, there were two words and two objects presented in each trial (two correct mappings among four possible associations), while our learning trials are composed of four words and four objects, which is much more complex. As a result, participants in Fitneva and Christiansen (2011) can recover from initial wrong hypotheses from cleaner learning environments while participants in our case which demands greater cognitive, attentional, and computational resources did not show any difference due to a tradeoff between having more or less accurate guesses at the beginning. Namely, even though having incorrect guesses may ultimately improve learning for the reasons suggested by Fitneva and Christiansen (2011), those learners with inaccurate initial guesses also have a disadvantage. With the fixed number of training trials, learners with correct initial guesses can rely on their correct initial states and further confirm those correct guesses in subsequent learning while learners with incorrect initial guesses do not accumulate and gain any useful information from wrong initial guesses and have to change and correct them later in training. Therefore, when facing with more complex learning environments, learners with correct initial guesses can accumulate and take advantage of more statistical regularities than learners with initial incorrect guesses. Therefore, both the learners with initial correct guesses and the learners with initial inaccurate guess may produce similar learning results but in different ways – the first group can easily take advantage of their good starting point while the second group is more engaged in later learning due to the detection of their wrong initial guesses. This observation also points out the flexibility and complexity of statistical learning mechanisms: on the one hand, as explained here, the same learning results can be achieved through different routes; on the other hand, as we illustrated earlier, the same learning mechanism can produce different learning outcomes depending on selective attention and information selection. At the very least, both the study in Fitneva and Christiansen (2011) and our study here argue for the importance of understanding the role of information selection at the beginning of statistical learning as a necessary step to gain a better understanding of learning mechanisms.
Aggregation of Cross-Situational Statistics
There are two noticeable patterns that are characteristic of the temporal dynamics of various measures reported in the present study (e.g., Figures 3, 4, and 8). First, for strong learners, learning is incremental as more statistical evidence is accumulated. At this point, it becomes clear that at the very least, learners in the cross-situational paradigm need to accumulate statistical evidence across trials and by so doing they gradually look more toward correct objects. A recent simulation study offered for an alternative account of cross-situational results, showing that it is mathematically possible that a simulated learner may not integrate information across trials but still demonstrate above-chance learning performance (Smith et al., 2006). This argument cannot explain gradually increasing and consistent gaze patterns toward correct objects produced by strong learners. Meanwhile, weak learners seem to more or less randomly select objects to attend trial by trial without evidence of retaining previous experiences. Indeed, there are three plausible reasons on how weak learners were much less successful. First, at the perceptual level, they may not be sensitive to multimodal occurrences between correct word–referent pairs. They may not be able to remember what they were exposed to before and therefore they did not demonstrate familiarity effects – looking at familiar objects after hearing familiar words, nor novelty effects – looking at novel objects when hearing new words. These basic perceptual capabilities may be sufficient to build correct word–referent associations from ambiguous data. At the cognitive level, weak learners may not be able to form longer-term representations but only have transient working memory representations in linking heard words and seen objects. Therefore, they could not use information in previous exposures to guide attention and learning in subsequent trials. At the computational level, weak learners may generate individual hypotheses in a trial but they never cross-tabulate these hypotheses via statistical procedures (Medina et al., 2011). Although the current eye-tracking study cannot yet further distinguish these possibilities, it is clear that the key to successful learning is to carry out knowledge and experiences gained from past learning situations into subsequent learning. The above three explanations at different levels can be based on the same underlying resource but just be conceptualized in different ways and from different perspectives. However, it is also possible that the three explanations may have profound theoretical differences.
Second, strong and weak learners do not differ in the first few training trials but looking behaviors begin to diverge only after/around the middle of training. In particular, there are no significant differences in various temporal profiles shown in Figures 3 and 4, until the third appearance of co-occurring pairs. At a first thought, this observation seems to be counter intuitive with the fundamental idea of associative learning which would predict incremental learning from the beginning as more and more statistical evidence is accumulated. However, simulation studies of statistical associative learning have shown that the same statistical learning mechanism, operating incrementally and without any significant internal changes, is able to give rise to a dramatic change in learning rate (Plunkett et al., 1992; McMurray, 2007; Yu, 2008; Yurovsky et al., under resubmission). The performance of the same learning mechanism can be significantly improved by storing lexical knowledge previously accumulated and then recruiting it in subsequent learning. With more knowledge accumulated and then recruited, participants become more efficient word learners. However, without enough statistical evidence in the early part of training, the very same mechanisms cannot operate efficiently and demonstrate their effects with sparse data. Only after a certain amount of cross-situational statistics has been accumulated, dramatic behavioral changes are observed, suggesting the effects of accumulating statistical evidence. In such a learning system, even though there are no observed changes in the early training, the initial accumulation of statistics is critical as they incrementally build an underlying foundation for the later bootstrapping. Without latent (and probably partial) knowledge accumulated, the learning system would not be able to produce more learning outcomes at a faster pace which makes the same associative mechanism much more effective. Compared with hypothesis-testing based mechanisms, the power of statistical learning is to continuously and accumulatively gather various kinds of statistical evidence which can later be utilized to lead to efficient learning. This accumulated effect can be a key characteristic of statistical associative learning, which is certainly consistent with many formal theories of early word learning. For example, it has been shown that vocabulary growth begins slowly with a gradual increase in the number of new words but then quickens to a noticeably fast rate of word acquisitions (Benedict, 1979; Dromi, 1987; Gopnik and Meltzoff, 1987; Lifter and Bloom, 1989; Goldfield and Reznick, 1990; Gershkoff-Stowe and Smith, 1997). However, empirical evidence to support this idea focuses on such changes in a rather large temporal span/scale (e.g., vocabulary growth over several months or computational simulations over large corpora). Here we demonstrate that the accumulated effects that bootstrap learning also happen at a much short temporal span of statistical learning (less than a 6-min training, etc.). Taken together, accumulative effects through associative learning may operate at multiple temporal scales, from second to second information aggregation, to day by day and month by month learning, suggesting that the same mechanism of associative learning may serve as a fundamental mechanism to learning and cognition.
Conclusion
Participants in cross-situational paradigms (and as well as young language learners in the real world) cannot pay attention to all the regularities in a complex environment with many objects and many words co-occurring, and many events happening concurrently. Therefore, selective attention and information selection provide the foundation for what is perceived and learned, as selecting right information is critical for successful statistical learning. It is one thing to point out that information selection in cross-situational learning is likely to be driven by spoken words, and is likely to be influenced by mutual exclusivity, or to make a grand argument on underlying mechanisms, whether it is associative learning or hypothesis testing, but it is another to quantify and describe what exactly happens moment by moment in real-time learning, how several nested factors may work individually and together, and what drives attention to more successful or less successful learning. The application of eye tracking to statistical cross-situational learning is new (Fitneva and Christiansen, 2011; Yu and Smith, 2011), and from this perspective, our results provide a useful initial framework for the evaluation of eye movements as a way to understand real-time learning mechanisms. Toward this goal, various results derived from learners’ gaze data not only provide useful insights to understand statistical learning, but also generate testable predictions and hypotheses for future empirical and modeling work.
Conflict of Interest Statement
The authors declare that the research was conducted in the absence of any commercial or financial relationships that could be construed as a potential conflict of interest.
Footnote
- ^Two other timing offsets (400 and 500 ms, etc.) were selected but this parameter did not make any difference in our results.
References
Akhtar, N., and Montague, L. (1999). Early lexical acquisition: the role of cross-situational learning. First Lang. 19, 347.
Allopenna, P., Magnuson, J., and Tanenhaus, M. (1998). Tracking the time course of spoken word recognition using eye movements: evidence for continuous mapping models. J. Mem. Lang. 38, 419–439.
Amso, D., and Johnson, S. P. (2006). Learning by selection: visual search and object perception in young infants. Dev. Psychol. 42(6), 1236.
Aslin, R., and McMurray, B. (2004). Automated corneal-reflection eye tracking in infancy: methodological developments and applications to cognition. Infancy 6, 155–163.
Baayen, R. H. (2008) Analyzing Linguistic Data: A Practical Introduction to Statistics Using R. Cambridge: Cambridge University Press.
Baayen, R. H., Davidson, D. J., and Bates, D. M. (2008). Mixed-effects modeling with crossed random effects for subjects and items. J. Mem. Lang. 59, 390–412.
Ballard, D. H., Hayhoe, M. M., Pook, P. K., and Rao, R. P. N. (1997). Deictic codes for the embodiment of cognition. Behav. Brain Sci. 20, 723–742.
Bates, D., and Sarkar, D. (2007). Lme4: Linear Mixed-Effects Models Using S4 Classes. Madison: University of Wisconsin.
Benedict, H. (1979). Early lexical development: comprehension and production. J. Child Lang. 6, 183–200.
Colunga, E., and Smith, L. B. (2005). From the lexicon to expectations about kinds: a role for associative learning. Psychol. Rev. 112(2), 347.
Fazly, A., Ahmadi-Fakhr, F., Alishahi, A., and Stevenson, S. (2010a). “Cross-situational learning of low frequency words: the role of context familiarity and age of exposure,” in Proceedings of the 32nd Annual Conference of the Cognitive Science Society (Portland: Cognitive Science Society), 2615–2620.
Fazly, A., Alishahi, A., and Stevenson, S. (2010b). A probabilistic computational model of cross situational word learning. Cogn. Sci. 34, 1017–1063.
Fisher, C., Hall, D. G., Rakowitz, S., and Gleitman, L. (1994). When it is better to receive than to give: syntactic and conceptual constraints on vocabulary growth* 1. Lingua. 92, 333–375.
Fitneva, S. A., and Christiansen, M. H. (2011). Looking in the wrong direction correlates with more accurate word learning. Cogn. Sci. 35, 367–380.
Frank, M. C., Goodman, N. D., and Tenenbaum, J. B. (2009). Using speakers’ referential intentions to model early cross-situational word learning. Psychol. Sci. 20, 578.
Gershkoff-Stowe, L., and Smith, L. B. (1997). A curvilinear trend in naming errors as a function of early vocabulary growth* 1. Cogn. Psychol. 34, 37–71.
Goldfield, B. A., and Reznick, J. S. (1990). Early lexical acquisition: rate, content, and the vocabulary spurt. J. Child Lang. 17, 171–183.
Golinkoff, R. M., Hirsh-Pasek, K., Bailey, L. M., and Wenger, N. R. (1992). Young children and adults use lexical principles to learn new nouns. Dev. Psychol. 28(1), 99.
Gopnik, A., and Meltzoff, A. (1987). The development of categorization in the second year and its relation to other cognitive and linguistic developments. Child Dev. 1523–1531.
Griffin, Z. (2004). “Why look? Reasons for eye movements related to language production,” in The Interface of Language, Vision, and Action: Eye Movements and the Visual World, ed. J. Henderson and F. Ferreira (New York: Taylor and Francis), 213–247.
Griffin, Z., and Bock, K. (1998). Constraint, word frequency, and the relationship between lexical processing levels in spoken word production. J. Mem. Lang. 38, 313–338.
Halberda, J. (2006). Is this a dax which I see before me? Use of the logical argument disjunctive syllogism supports word-learning in children and adults. Cogn. Psychol. 53, 310–344.
Hollich, G. J., Hirsh-Pasek, K., Golinkoff, R. M., Brand, R. J, Brown, E., Chung, H. L., Hennon, E., and Rocroi, C. (2000). Breaking the language barrier: an emergentist coalition model for the origins of word learning. Monogr. Soc. Res. Child Dev. 65, 1–123.
Johnson, S., Amso, D., and Slemmer, J. (2003). Development of object concepts in infancy: evidence for early learning in an eye-tracking paradigm. Proc. Natl. Acad. Sci. 100, 10568–10573.
Johnson, S. P., Davidow, J., Hall-Haro, C., and Frank, M. C. (2008). Development of perceptual completion originates in information acquisition. Dev. Psychol. 44, 1214.
Johnson, S. P., Slemmer, J. A., and Amso, D. (2004). Where infants look determines how they see: eye movements and object perception performance in 3-month-olds. Infancy 6, 185–201.
Kachergis, G., Yu, C., and Shiffrin, R. M. (2012). An associative model of adaptive inference for learning word–referent mappings. Psychon. Bull. Rev. 1–8.
Klein, K. A., Yu, C., and Shiffrin, R. M. (2008). “Prior knowledge bootstraps cross-situational learning,” in Proceedings of Annual Meeting of Cognitive Science Society (Washington: Cognitive Science Society), 1930–1935.
Knoeferle, P., and Crocker, M. (2006). The coordinated interplay of scene, utterance, and world knowledge: evidence from eye tracking. Cogn. Sci. 30, 481–529.
Kowler, E., Anderson, E., Dosher, B., and Blaser, E. (1995). The role of attention in the programming of saccades. Vision Res. 35, 1897–1916.
Lifter, K., and Bloom, L. (1989). Object play and the emergence of language. Infant Behav. Dev. 12, 395–423.
Markman, E. (1992). Constraints on word learning: speculations about their nature, origins, and domain specificity. Modularity and Constraints in Language and Cognition, 25, 59–101.
Medina, T. N., Snedeker, J., Trueswell, J. C., and Gleitman, L. R. (2011). How words can and cannot be learned by observation. Proc. Natl. Acad. Sci. 108, 9014.
Meyer, A., Sleiderink, A., and Levelt, W. (1998). Viewing and naming objects: eye movements during noun phrase production. Cognition 66, 25–33.
Nematzadeh, A., Fazly, A., and Stevenson, S. (2011). “A computational study of late talking in word-meaning acquisition,” in Proceedings of the 33rd Annual Conference of the Cognitive Science Society. (Boston: Cognitive Science Society), 705–710.
Pinker, S. (1984). Language Learnability and Language Development. Cambridge, MA: Harvard University Press.
Plunkett, K., Hu, J., and Cohen, L. (2008). Labels can override perceptual categories in early infancy. Cognition 106, 665–681.
Plunkett, K., Sinha, C., Martin, F. M., and Strandsby, O. (1992). Symbol grounding or the emergence of symbols? Vocabulary growth in children and a connectionist net. Connect. Sci. 4, 293–312.
Rehder, B., and Hoffman, A. B. (2005). Eyetracking and selective attention in category learning. Cogn. Psychol. 51, 1–41.
Schafer, G., and Plunkett, K. (1998). Rapid word learning by fifteen month olds under tightly controlled conditions. Child Dev. 69, 309–320.
Schöner, G., and Thelen, E. (2006). Using dynamic field theory to rethink infant habituation. Psychol. Rev. 113(2), 273.
Scott, R. M., and Fisher, C. (2011). 2.5-Year-olds use cross-situational consistency to learn verbs under referential uncertainty. Cognition 122, 163–180.
Shiffrin, R. M., and Schneider, W. (1977). Controlled and automatic human information processing: II. Perceptual learning, automatic attending and a general theory. Psychol. Rev. 84, 127–190.
Siskind, J. M. (1996). A computational study of cross-situational techniques for learning word-to-meaning mappings. Cognition 61, 39–91.
Smith, K., Smith, A. D. M., Blythe, R. A., and Vogt, P. (2006). “Cross-situational learning: a mathematical approach,” in Symbol Grounding and Beyond: Proceedings of the Third International Workshop on the Emergence and Evolution of Linguistic Communication, eds P. Vogt, Y. Sugita, E. Tuci and C. Nehaniv (Berlin: Springer), 31–44.
Smith, L. B. (2000). “Learning how to learn words: an associative crane,” in Becoming a Word Learner: A Debate on Lexical Acquisition, eds R. M. Golinkoff, K. Hirsh-Pasek, L. Bloom, L. Smith, A. Woodward, N. Akhtar, M. Tomasello, and G. Hollich (London: Oxford University Press), 51–80.
Smith, L., and Yu, C. (2008). Infants rapidly learn word-referent mappings via cross-situational statistics. Cognition 106, 1558–1568.
Tanenhaus, M. K., Spivey-Knowlton, M. J., Eberhard, K. M., and Sedivy, J. C. (1995). Integration of visual and linguistic information in spoken language comprehension. Science 268, 1632.
Trueswell, J. C., and Gleitman, L. (2004). “Children’s eye movements during listening: developmental evidence for a constraint-based theory of sentence processing,” in The Interface of Language, Vision, and Action: Eye Movements and the Visual World, eds J. M. Henderson and F. Ferreira (New York: Psychology Press), 319–346.
Turk-Browne, N. B., Scholl, B. J., and Chun, M. M. (2008). Babies and brains: habituation in infant cognition and functional neuroimaging. Front. Hum. Neurosci. 2:16. doi:10.3389/neuro.09.016.2008
von Hofsten, C., Vishton, P., Spelke, E., Feng, Q., and Rosander, K. (1998). Predictive action in infancy: tracking and reaching for moving objects. Cognition 67, 255–285.
Vouloumanos, A., Hauser, M. D., Werker, J. F., and Martin, A. (2010). The tuning of human neonates’ preference for speech. Child Dev. 81, 517–527.
Vouloumanos, A., and Werker, J. F. (2009). Infants’ learning of novel words in a stochastic environment. Dev. Psychol. 45, 1611.
Yu, C. (2008). A statistical associative account of vocabulary growth in early word learning. Lang. Learn. Dev. 4, 32–62.
Yu, C., and Smith, L. B. (2007). Rapid word learning under uncertainty via cross-situational statistics. Psychol. Sci. 18, 414.
Yu, C., and Smith, L. B. (2011). What you learn is what you see: using eye movements to study infant cross-situational word learning. Dev. Sci. 16, 165–180.
Keywords: word learning, eye tracking, statistical learning
Citation: Yu C, Zhong Y and Fricker D (2012) Selective attention in cross-situational statistical learning: evidence from eye tracking. Front. Psychology 3:148. doi: 10.3389/fpsyg.2012.00148
Received: 04 December 2011; Accepted: 24 April 2012;
Published online: 14 June 2012.
Edited by:
Catherine M. Sandhofer, University of California, Los Angeles, USAReviewed by:
Yuyan Luo, University of Missouri, USA; Afsaneh Fazly, University of Toronto, CanadaCopyright: © 2012 Yu, Zhong and Fricker. This is an open-access article distributed under the terms of the Creative Commons Attribution Non Commercial License, which permits non-commercial use, distribution, and reproduction in other forums, provided the original authors and source are credited.
*Correspondence: Chen Yu, Psychological and Brain Science, and Cognitive Science, Indiana University, Bloomington, IN 47401, USA. e-mail:Y2hlbnl1QGluZGlhbmEuZWR1