- 1 Brain Mapping Center, University of Pittsburgh Medical Center, Pittsburgh, PA, USA
- 2 Department of Psychology, Center for the Neural Basis of Cognition, Carnegie Mellon University, Pittsburgh, PA, USA
- 3 Speech, Language and Hearing Sciences, University of Arizona, Tucson, AZ, USA
Voices have unique acoustic signatures, contributing to the acoustic variability listeners must contend with in perceiving speech, and it has long been proposed that listeners normalize speech perception to information extracted from a talker’s speech. Initial attempts to explain talker normalization relied on extraction of articulatory referents, but recent studies of context-dependent auditory perception suggest that general auditory referents such as the long-term average spectrum (LTAS) of a talker’s speech similarly affect speech perception. The present study aimed to differentiate the contributions of articulatory/linguistic versus auditory referents for context-driven talker normalization effects and, more specifically, to identify the specific constraints under which such contexts impact speech perception. Synthesized sentences manipulated to sound like different talkers influenced categorization of a subsequent speech target only when differences in the sentences’ LTAS were in the frequency range of the acoustic cues relevant for the target phonemic contrast. This effect was true both for speech targets preceded by spoken sentence contexts and for targets preceded by non-speech tone sequences that were LTAS-matched to the spoken sentence contexts. Specific LTAS characteristics, rather than perceived talker, predicted the results suggesting that general auditory mechanisms play an important role in effects considered to be instances of perceptual talker normalization.
Introduction
A long-standing, core theoretical problem in understanding speech perception is the lack of a one-to-one mapping between acoustic input and intended linguistic categories (Liberman et al., 1967). One major source of this lack of invariance is acoustic variation across talkers who differ in vocal tract size and anatomy, age, gender, dialect, and idiosyncratic speech mannerisms (Johnson et al., 1993). This results in substantially different acoustic realizations of the same linguistic unit (e.g., Peterson and Barney, 1952). Yet, human listeners maintain remarkably accurate speech perception across an unlimited number of communication partners, even without having extensive experience with the talker. The mechanisms by which speech perception accommodates talker variability have been a central issue since the inception of the field (Potter and Steinberg, 1950), but they are poorly understood. This is evident in the fact that even the most advanced computerized speech recognition systems require substantial talker-specific training to achieve high accuracy.
The problem, however, is not unconstrained – a change in vocal tract anatomy or vocal fold physiology changes the acoustic signature systematically. For example, adult women tend to have shorter and differently proportioned vocal tracts than adult males. As a result, female-produced vowels have formant frequencies (peaks in energy of a voice spectrum; Fant, 1960) shifted to higher frequencies relative to males’. It would seem likely that effective listeners make use of these regularities in acoustic variation to achieve more accurate and efficient speech categorization.
One early demonstration of talker-dependent speech categorization was made by Ladefoged and Broadbent (1957), who presented listeners with a constant target word (a relatively ambiguous vowel in a /b_t/ frame) at the end of a context phrase (Please say what this word is…). The acoustic characteristics of the context phrase were manipulated by raising or lowering the first (F1) and/or second (F2) formant frequencies of the vowels. Shifting formant frequencies up and down can be roughly conceptualized as a decrease or increase in vocal tract length and, correspondingly, as a change in talker. When these phrases preceded a constant speech target, categorization of the vowel in the target word shifted as a function of the context phrase, suggesting that listeners compensate for vocal tract differences across talkers. The target was more often heard as “bit” following a higher formant frequency phrase (as might be produced by a shorter vocal tract), but more often as “bet” following the phrase with the lower formant frequencies. These classic results suggest that listeners extract some type of talker information from the context phrase and use it in perceiving the target word. The critical question, then, is: What type of information is extracted from the context phrase?
One possibility is that listeners construct an explicit representation of the talker-specific phonemic sound patterns produced by the talker, which could serve as a reference for subsequent speech perception. When an ambiguous vowel is encountered in the target word, the relative position of the formant frequencies in the remapped vowel space could reveal the intended vowel. Thus, talker-specific, speech-specific information gathered during the carrier phrase might tune speech perception to talker-specific patterns (Joos, 1948; Ladefoged and Broadbent, 1957).
Alternatively, listeners may estimate talker-specific vocal tract dimensions. Recent work by Story (2005) examining vocal tract shapes across talkers using magnetic resonance images reveals that most inter-talker variability is captured by the shape of the neutral, non-articulating vocal tract shape that, when excited by vocal fold vibration, results in a neutral schwa sound as in the second vowel of sofa. If one subtracts a talker’s neutral vocal tract shape from other vowel vocal tract shapes, the resulting vocal air space shape for various vowels is quite consistent across talkers. Thus, estimating the neutral vocal tract shape of the talker from the carrier phrase and using this estimate to normalize the vocal tract shape determined for the target vowel might tune speech perception to talker-specific patterns. This might be accomplished by one of a number of mechanisms, such as reconstructing the intended articulatory movements of the vocal tract to identify speech sounds as described by the motor theory of speech perception (Liberman et al., 1967; Liberman, 1996), explicitly extracting vocal tract dimensions from the carrier phrase to rescale perception of subsequent speech sounds (McGowan, 1997; McGowan and Cushing, 1999), or creating an internal vocal tract model against which to compare ambiguous sounds to a set of possible targets (Halle and Stevens, 1962; Poeppel et al., 2008). Each of these strategies relies on explicit representation of some type of vocal tract-specific information. A challenge for these accounts is that it is notoriously difficult to solve the inverse problem of determining a unique vocal tract shape from speech acoustics (Atal et al., 1978) and there is currently no good model of how listeners would retrieve the neutral vocal tract from speech that does not explicitly include an instance of a neutral production.
Recent studies in context-dependent auditory perception suggest that carrier phrases may provide an alternative type of information that is neither explicitly phonemic nor linked to speech production, but that may contribute to talker normalization effects in speech perception (Holt, 2005, 2006; Huang and Holt, 2009, 2012). These experiments mirrored the Ladefoged and Broadbent paradigm in that context sounds preceded speech targets. However, the contexts were not speech phrases, but rather a sequence of 21 non-speech sine-wave tones whose frequencies were sampled from one of two distributions. The resulting sounds were something like a simple tune. The mean of the distribution of tones was either a relatively high-frequency (mean 2800 Hz, 2300–3300 Hz range) or low-frequency (mean 1800 Hz, 1300–2300 Hz range). When these tone sequences preceded target speech sounds drawn from a series varying perceptually from /ga/ to /da/, speech categorization was influenced by the distribution from which the context tones had been drawn. Tones with a higher mean frequency led to more /ga/ responses, whereas the same targets were more often categorized as /da/ when lower-frequency tones preceded them.
Of note in interpreting the results, the tones comprising the context were randomly ordered on a trial-by-trial basis. Thus, each context stimulus was unique, and only the long-term average spectrum (LTAS, the distribution of acoustic energy across frequency for the entire duration of the tone sequence) defined conditions. The distributional nature of the contexts in these studies indicates that auditory processing is sensitive to the LTAS of context stimuli and that perception of target speech sounds is relative to, and spectrally contrastive with, the LTAS. These results are consistent with demonstrations that speech categorization shifts when the LTAS of the carrier phrase is changed by applying a filter (Watkins, 1991; Watkins and Makin, 1994, 1996; Kiefte and Kluender, 2008), spectral tilt (Kiefte and Kluender, 2001), or reverberation (Watkins and Makin, 2007). They are also consonant with findings of classic adaptation effects on phoneme categorization (e.g., Eimas and Corbit, 1973; Diehl et al., 1978; Sawusch and Nusbaum, 1979; Lotto and Kluender, 1998) in that both effects are spectrally contrastive, but the tone sequence effects differ in their time course, persisting across silences as long as 1.3 s, and even across intervening spectrally neutral sound (Holt and Lotto, 2002; Holt, 2005).
Holt (2005) and Lotto and Sullivan (2007) have speculated that the general auditory processes underlying these effects may prove useful for talker normalization. To put this into the context of the classic talker normalization effects reported by Ladefoged and Broadbent (1957), consider the acoustic consequences of long versus short vocal tracts. A talker with a long vocal tract produces speech with relatively greater low-frequency energy than a talker with a shorter vocal tract. In line with the pattern of spectral contrast described above, listeners’ sensitivity to the lower-frequency energy in the LTAS of the longer-vocal tract talker’s speech should result in target speech being perceived as relatively higher-frequency. Applying this prediction to the stimulus scheme of Ladefoged and Broadbent, constant vowel targets should be more often perceived as “bet” following a phrase synthesized to mimic a long vocal tract (“bet” is characterized by higher formant frequencies than “bit”) whereas a phrase mimicking a talker with a shorter vocal tract should lead listeners to label the same speech targets more often as “bit.” These are, in fact, the results of Ladefoged and Broadbent (1957). Thus, the analogy between the non-speech context results of Holt (2005, 2006) and talker normalization carrier phrase effects appears compelling, but explicit comparison of these two types of effects has not been made. In particular, talker normalization effects have been typically demonstrated with shifts in vowel categorization, whereas the non-speech categorization tasks have typically utilized consonant contrasts as targets. In addition, there has not been an effort to match speech and non-speech contexts on duration, frequency ranges, and other acoustic dimensions. As a result, to this point, the proposal of an LTAS account of talker normalization has primarily been supported through analogy.
The purpose of the present study is to test three predictions of an LTAS-based model of talker normalization (Lotto and Sullivan, 2007). The first prediction is that the direction of the shift in target phoneme categorization is predictable from a comparison of the LTAS of the carrier phrase and the spectrum of the targets. In particular, carrier phrases with higher-frequency concentrations of energy should result in target representations that are shifted to lower-frequency concentrations of energy; a spectral contrast effect (Lotto and Holt, 2006).
The second prediction is that not all talkers will elicit normalization for all speech targets. The LTAS model makes specific predictions about which talkers will produce perceptual normalization effects, and which will not. Although the Ladefoged and Broadbent findings are foundational in the talker normalization literature, they have a reputation for being difficult to replicate (Hawkins, 2004). We suspect that this may arise because it may not be sufficient to simply change the talker of the carrier phrase if the relationship between LTAS of target and carrier phrase is not matched. We predict that pairs of talkers who vary in LTAS in the range of frequencies important for target speech categorization (e.g., in the vicinity of F3 for /ga/-/da/ consonant targets) will produce target speech categorization shifts typical of the Ladefoged and Broadbent results but that LTAS differences outside this range will produce highly discriminable talkers that do not elicit “talker normalization” effects.
The final prediction of the LTAS model to be tested is that similar shifts in target categorization will be elicited from non-speech contexts to the extent that the LTAS is matched to the speech contexts (in the relevant frequency region). Such a result would strongly suggest general auditory, as opposed to vocal tract-representation or acoustic-phonemic based, mechanisms of talker normalization, because the non-speech contexts carry no information about vocal tract anatomy, talker identity, neutral vowel patterns, or phoneme identity. Should non-speech contexts influence speech target categorization when listeners have no access to articulatory referents, it would provide evidence for contributions of general auditory processes to talker normalization.
Materials and Methods
Participants
Twenty volunteers from Carnegie Mellon University and the University of Pittsburgh participated for a small payment. All listeners reported normal hearing, were native monolingual English speakers, and provided written informed consent to participate.
The experiment was approved by the Carnegie Mellon University Institutional Review Board.
Stimuli
Speech targets
Nine speech target stimuli were derived from natural /ga/ and /da/ recordings from a monolingual male native English speaker (Computer Speech Laboratory, Kay Elemetrics, Lincoln Park, NJ, USA; 20-kHz sampling rate, 16-bit resolution) and were identical to those utilized in several earlier studies (Holt, 2005, 2006; Wade and Holt, 2005). To create the nine-step series, multiple natural productions of the syllables were recorded and, from this set, one /ga/ and one /da/ token were selected that were nearly identical in spectral and temporal properties except for the onset frequencies of F2 and F3. Linear predictive coding (LPC) analysis was performed on each of the tokens to determine a series of filters that spanned these endpoints (Analysis-Synthesis Laboratory, Kay Elemetrics) such that the onset frequencies of F2 and, primarily, F3 varied approximately linearly between /ga/ and /da/ endpoints. These filters were excited by the LPC residual of the original /ga/ production to create an acoustic series spanning the natural /ga/ and /da/ endpoints in approximately equal steps. Creating stimuli in this way provides the advantage of very natural-sounding speech tokens. These 411-ms speech series members served as categorization targets. Figure 1B shows spectrograms for the endpoints of the series, appended to two different types of context. The top spectrogram depicts the /ga/ endpoint whereas the bottom spectrogram shows the /da/ endpoint. Notice that the main difference between the targets is the onset F3 frequency.
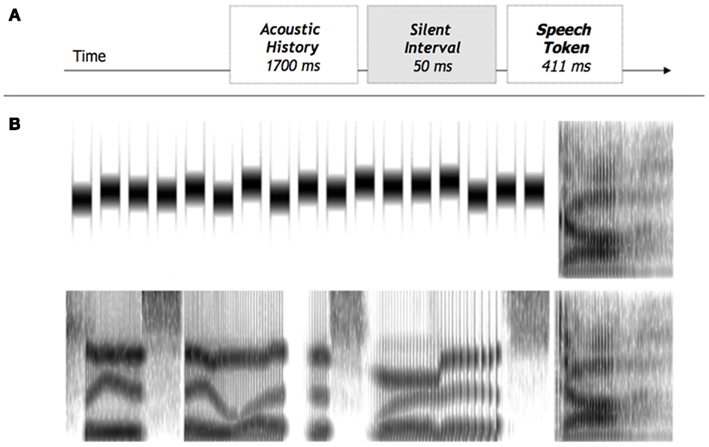
Figure 1. (A) Schematic illustration of stimulus construction; (B) Spectrograms of non-speech context followed by /ga/ (top) and speech context followed by /da/ (bottom).
Context stimuli: speech
The speech targets were preceded by one of eight context stimuli. Four of these contexts were the phrase “Please say what this word is…,” mimicking the contexts studied by Ladefoged and Broadbent (1957). Variants were synthesized to sound as though the phrase was spoken by four different talkers. This was accomplished by raising or lowering the formant frequencies in either the region of the F1 or F3. To create the voices, a 1700-ms phrase was generated by extracting formant frequencies and bandwidths from recording a male voice reciting “Please say what this word is…,” and using these values to synthesize the phrase in the parallel branch of the Klatt and Klatt (1990) synthesizer. The phrase created with these natural parameters was spectrally manipulated by adjusting formant center frequencies and bandwidths to create the different “talkers.” These manipulations resulted in two independent variables: context frequency peak (High, Low) and context frequency range (F1, F3).
The context frequency peak manipulation arises from previous research, indicating that context effects in speech categorization are spectrally contrastive (e.g., Lotto and Kluender, 1998; Holt, 2005; Lotto and Holt, 2006). Lower-frequency contexts shift categorization responses toward higher-frequency alternatives (e.g., /da/) whereas higher-frequency contexts shift responses toward lower-frequency alternatives (e.g., /ga/). Figure 2B plots the LTAS for /ga/ and /da/ endpoint speech targets, demonstrating that the tokens are maximally distinctive at two areas within the F3 frequency range (approximately 1800 and 2800 Hz). Thus, following the hypotheses of the LTAS model, one “talker” was synthesized to possess relatively higher-frequency energy in the F3 region with a peak in energy at about 2866 Hz. Another “talker” was created with relatively lower-frequency F3 energy peaking at about 1886 Hz. This manipulation is very similar to the type used by Ladefoged and Broadbent (1957) to synthesize talker differences in their classic study, although they manipulated only F1 and F2 frequencies.
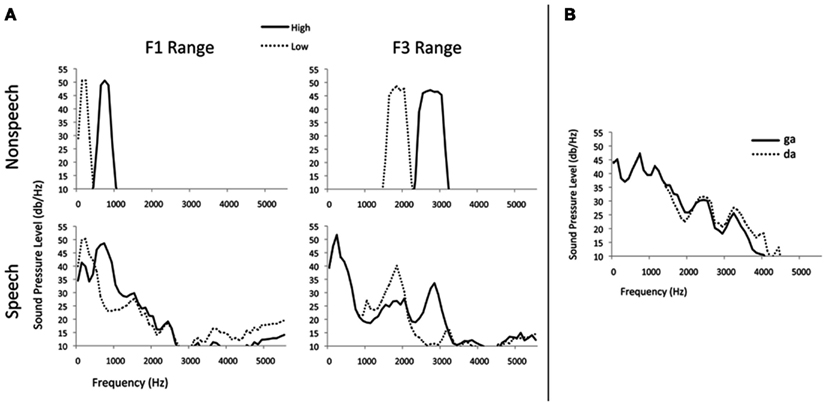
Figure 2. (A) Long-term average spectra (LTAS) of representative context stimuli from each condition; (B) LTAS of speech target endpoints /ga/ and /da/.
A similar manipulation was made in the F1 frequency region to create two additional synthesized voices. The peak frequencies in this region were chosen to match the perceptual distance of the High and Low peaks and bandwidths in the F3 frequency region (equating the peak frequency difference on the Mel scale, a psychoacoustic scale that may better model the non-linear characteristics of human auditory processing along the frequency dimension than the linear Hz scale; Stevens et al., 1937). This resulted in phrases with peaks in the LTAS at 318 Hz for the lower-frequency context and 808 Hz for the higher-frequency context.
The context frequency range manipulation (F1 versus F3) provided a test of the hypothesis that context-dependent speech categorization characterized as “talker normalization” is sensitive to spectral differences in the region of the spectra relevant to target speech categorization. Although both F1 and F3 manipulations are expected to produce discriminable differences in perceived talker, the LTAS model predicts that spectral peak differences in the region of F3 should influence categorization of /ga/-/da/ exemplars because F3 is critical to the /ga/-/da/ distinction. However, spectral peak differences in the region of F1 are predicted to have no influence on /ga/-/da/ categorization. Figure 1B presents representative spectrograms and Figure 2A shows the LTAS of each voice. Pairing these four contexts with the nine speech targets resulted in 36 unique stimuli; each was presented 10 times in the experiment, for a total of 360 speech context trials. Speech stimuli were sampled at 11,025 Hz and converted to *.wav files using MATLAB (Mathworks, Inc.).
Context stimuli: non-speech
Following the methods of Holt (2005), four non-speech contexts comprised of sequences of sine-wave tones with frequencies chosen to mirror the Low and High-frequency peaks in the F1 and F3 regions of the speech contexts’ LTAS were also synthesized. Figure 2A shows the LTAS of these tone sequences. Note that, whereas the LTAS of sentence contexts are somewhat difficult to manipulate because speech inherently possesses energy across the frequency spectrum, non-speech contexts are more easily controlled with explicit placement of sine-wave tones. Thus, for the non-speech contexts acoustic energy may be focused on precisely the spectral regions predicted to have (or not to have) an effect on target /ga/-/da/ categorization. This may have important implications for the magnitude of the influence of speech versus non-speech contexts on speech categorization, as discussed below.
These sequences of tones, similar to those described by Holt (2005), did not sound like speech and did not possess articulatory or talker-specific information. Seventeen 70-ms tones (5 ms linear onset/offset amplitude ramps) with 30 ms silent intervals modeled the 1700-ms duration of the speech contexts. As in previous experiments (Holt, 2005, 2006; Huang and Holt, 2009), the order of the tones making up the non-speech contexts was randomized on a trial-by-trial basis to minimize effects elicited by any particular tone ordering. Thus, any influence of the non-speech contexts on the speech categorization is indicative of listeners’ sensitivity to the LTAS of the context and not merely to the simple acoustic characteristics of any particular segment of the tone sequence.
The bandwidth of frequency variation was approximately matched to the bandwidth of the peak in the corresponding speech context’s LTAS, as measured 10 dB below the peak. The low-frequency F1 range distribution sampled 150 Hz in 10 Hz steps (mean 200 Hz, 125–275 Hz range), and the high-frequency F1 range distribution sampled 240 Hz in 16 Hz steps (mean 750 Hz, range 630–870 Hz). The low-frequency F3 range distribution sampled 435 Hz in 29 Hz steps (mean 1873.5 Hz, range 1656–2091 Hz), and the high-frequency F3 range distribution sampled 570 Hz in 38 Hz steps (mean 2785 Hz, range 2500–3070 Hz).
Tones comprising the non-speech contexts were synthesized with 16-bit resolution, sampled at 11,025 Hz, and concatenated to form random orderings. Ninety unique contexts were created so that each non-speech context could be paired with each of the nine speech targets 10 times. Across the four non-speech LTAS conditions, this resulted in 360 unique stimuli.
All speech and non-speech contexts and speech targets were digitally matched to the RMS energy of the /da/ endpoint of the target speech series, and a 50-ms silent interval separated the context and the speech target. Figure 1A provides a schematic illustration of stimulus construction and Figures 1B and 2A show spectrograms and LTAS of representative stimuli from each condition. The LTAS of the speech target series endpoints, /ga/ and /da/, are shown in Figure 2B.
Procedure
Listeners categorized the nine speech targets in each of the eight contexts. Trials were divided into four blocks so that listeners heard higher- and lower-frequency versions of each context condition [2 (speech/non-speech) × 2 (LTAS peak in F1 region/F3 region)] within the same block. The order of the blocks was fully counterbalanced across participants and, within a block, trial order was random. On each trial, listeners heard a context plus speech target stimulus and categorized the speech target as /ga/ or /da/ using buttons on a computer keyboard.
The categorization blocks were followed by a brief discrimination test to measure the extent to which manipulations of the LTAS were successful in producing perceived talker differences among the speech contexts. On each trial, participants heard a pair of context sentences and judged whether the voice speaking the sentences was the same or different by pressing buttons on a computer keyboard. The task was divided into two blocks according to the LTAS peak region (F1 versus F3). Within a block, listeners heard both higher-frequency and lower-frequency versions of the sentences across 20 randomly ordered trials. One-half of the trials were different talker pairs (High-Low or Low-High, five repetitions each) and the remaining trials were identical voices (High-High, Low-Low, five repetitions each).
For both speech categorization and talker discrimination tests, acoustic presentation was under the control of E-Prime (Schneider et al., 2002) and stimuli were presented diotically over linear headphones (Beyer DT-150) at approximately 70 dB SPL (A). The experiment lasted approximately 1 h.
Results
The results of the talker discrimination task indicate that the synthesized voices were highly discriminable as different talkers (F1 manipulation d′ = 3.46; F3 manipulation d′ = 3.09). Moreover, participants’ ability to discriminate talkers did not differ for talkers created with manipulations to LTAS in the F1 versus F3 frequency regions, t(19) = 1.603, p = 0.126. Thus, there is sufficient information available in the synthesized speech contexts to support talker identity judgments and this information does not differ depending on whether voices were synthesized via spectral manipulations of F1 versus F3 spectral regions. Each might be reasonably expected to elicit talker normalization.
However, the results indicate that this was not the case. The patterns of speech target categorization in the context of these four talkers was assessed with a 2 (context frequency range, F1/F3 region) × 2 (context frequency, High/Low) × 9 (speech target, /ga/-/da/) repeated-measures ANOVA of percent /ga/ responses. The analysis revealed a significant main effect of speech target, F(8, 152) = 130.196, p < 0.0001, = 0.873, indicating that /ga/ responses varied as intended across the speech targets. Higher-order interactions involving speech target were also significant (p < 0.05); however, since our predictions center on context-dependent speech target categorization, the focus of interpretation is placed on interactions that do not involve target. Figure 3 plots listeners’ average percent /ga/ categorization responses across speech targets as a function of context.
Overall, there was a robust main effect of speech context frequency (High, Low) on speech target categorization, F(1, 19) = 34.66, p < 0.0001, = 0.646, such that stimuli were more often labeled as /ga/ following high-frequency contexts (M = 68.3%, S.E. = 2.1%) than low-frequency contexts (M = 63.4%, S.E. = 2.1%). This is consistent with the spectrally contrastive pattern of results found for sentence-length contexts in previous research (Holt, 2005, 2006; Huang and Holt, 2009) and extends the original Ladefoged and Broadbent effects from target vowels to target consonants. There was no main effect of context frequency range, F < 1, indicating that the overall percent /ga/ responses did not vary between F1 and F3 conditions. Most importantly, however, context frequency (High, Low) significantly interacted with context range (F1, F3), F(1, 19) = 7.467, p = 0.013, = 0282. Whereas the voice differences created by high- versus low-frequency peaks in the F1 frequency range did not elicit a significant shift in speech target categorization [1.9%, F(1, 19) = 1.717, p = 0.206, = 0.083], the F3 range conditions did elicit a significant categorization shift [7.89%, F(1, 19) = 37.110, p < 0.001, = 0661]. This is curious given that the voice discrimination task revealed that voices created via F1 range spectral manipulations were just as discriminable as different talkers as those differing in the F3 frequency range. As described above, however, the LTAS model predicts this pattern of results.
A stronger test of an LTAS-based account rests with the non-speech contexts, which do not carry any speech or vocal tract information from which to accomplish talker normalization and, in fact, are perceived as sequences of non-linguistic tones. Qualitatively, the pattern of results for non-speech was very similar to that obtained for speech contexts (Figure 3). The effect of non-speech context frequency (High versus Low) was significant for the F3 range, F(1, 19) = 202.836, p < 0.0001, = 0.914, but not for the F1 range, F(1, 19) = 2.862, p = 0.107, = 0.131. Non-speech contexts elicited a shift in target categorization, but only when the spectral manipulations were in the F3 region.
There is a significant difference between the influence speech and non-speech contexts had on speech categorization that bears note. The effect of speech versus non-speech contexts varied as a function of context frequency (High, Low), F(1, 19) = 83.54, p < 0.0001, = 0.815, revealing that the size of the spectrally contrastive shift in speech target categorization differed across context type. The directionality of this difference is interesting; non-speech contexts had a bigger effect on speech categorization. Whereas the speech contexts elicited about a 7% shift in target categorization, the non-speech elicited a 38% shift. The dramatic difference in effect size may be understood with respect to the LTAS differences between the speech and non-speech conditions. Whereas, by necessity, speech contexts possess more diffuse energy across the frequency spectrum, the non-speech contexts had extremely concentrated energy in the spectral region significant to target speech categorization. This concentration of LTAS energy appears to be particularly effective in altering the subsequent perception of speech.
Discussion
The classic effect of context on speech categorization observed by Ladefoged and Broadbent (1957) demonstrated that listeners extract information from precursor phrases that affects categorization of subsequent vowels. The nature of this information has remained in question. Do listeners extract talker-specific representations of vocal tract dimensions or of acoustic-phonemic mappings for different talkers? The current study tested the predictions of an alternative model – that listeners compute a general auditory representation of the average energy across frequency, the LTAS. The LTAS then serves as a referent for representation for subsequent perception. Three predictions of the LTAS model were tested: (1) the direction of the shift in target categorizations would be predictable from the distribution of energy in the carrier phrase relative to that in the targets; (2) only manipulations to the carrier phrase that affect relevant frequency ranges would result in categorization shifts; and (3) non-speech contexts matched in LTAS (in the correct frequency range) to the speech contexts would result in similar categorization shifts for speech targets.
The first prediction of the LTAS model was supported. Following the carrier phrase (Please say what this word is…) synthesized with higher F3 frequencies, listeners categorized the target stimuli more often as /ga/, which has a lower F3 onset frequency than the alternative /da/. This is a spectrally contrastive pattern of results for which the greater relative energy in the higher-frequency region of F3 in the LTAS of the carrier phrase results in an effective lowering of the perceived F3 of the target stimulus (see Lotto and Holt, 2006). Note that this result extends the original findings of Ladefoged and Broadbent from vowel to consonant targets. This extension allows a clearer link to be made between talker normalization effects and recent work on non-speech context effects on speech perception (e.g., Lotto and Kluender, 1998; Holt, 2005; Lotto and Holt, 2006). Note, that whereas this pattern of results supports the predictions of the LTAS model, it does not rule out a model based on vocal tract or phoneme-acoustic specific representations. For example, if listeners were to track F3 values for each consonant during the high-F3 carrier phrase to map the phonemic space of a talker, the target would have a relatively low F3 onset frequency when compared to these referents (and be more likely perceived as a /ga/).
The second prediction of the LTAS model was also supported by the data. Although each of the context phrase manipulations resulted in a discriminably different voice, not all of the phrases produced a shift in target categorization. As predicted, the F3 manipulation resulted in a categorization shift, but the F1 manipulation, which is not in the range of the acoustic energy relevant for the /ga/-/da/ distinction, did not. This implies that the observed change in speech categorization was not based simply on a perceived change in talker (or vocal tract shape) per se. Rather, a particular task-relevant acoustic characteristic (F3 range) of talkers seems to be the critical factor that drives the normalization effect across conditions. It is reasonable to suspect that the sensitivity of the effects to the F3 (and not F1) spectral range is primarily due to its match to the range of frequencies discriminating the target speech contrast. Supportive of this, experiments have demonstrated that carrier phrases differentiated by energy in other spectral ranges (e.g., F2; Holt and Lotto, 2002, fundamental frequency, f0; Huang and Holt, 2009, 2012) produce similar effects on speech categorization if they match the spectral range relevant to the target contrast. Other research has also emphasized the importance of acoustic details of the context in predicting effects of context on categorizing auditory targets (Sjerps et al., 2011). A strength of the LTAS model is that it predicts which changes in talker will result in shifts in categorization and which will not. From the perspective of the spectral-based LTAS model, the target contrast should only be affected by shifts in carrier phrase LTAS if the spectral ranges of carrier and target are well-matched (Holt, 1999). Simply changing the talker of the context phrase does not suffice – the listener clearly is not just using a shift in formant values to recalibrate judgments about vocal tract size.
The third prediction of the LTAS was tested by substituting a series of tones for the context phrases. The LTAS of these tone sequences had high or low-frequency energy in the F1 or F3 regions that were similar to the LTAS for the respective speech conditions, but they sounded nothing like speech, and carried no information about talker, voice, vocal tract anatomy, or phonemes. As predicted, there was a significant contrastive shift in target categorization for the non-speech sequences that mimicked the F3 manipulations of the speech contexts (but no shift for the F1 region tone sequences). In fact, the perceptual shift observed was greater for the non-speech than the speech contexts. This difference was likely due to the fact that the peaks in the LTAS for the non-speech were greater in amplitude and more discrete than those of the more acoustically complex sentences (see Figure 2A). The fact that prominence and focus of the spectral peaks, rather than any speech- or talker-specific characteristic, had the greatest effect on speech categorization provides further evidence that the processes and representations underlying these context-dependent effects may be of a general perceptual nature. This generality allows the LTAS model to extend naturally to other types of talker normalization that do not originate at the segmental (phoneme, syllable) level, such as normalization for lexical tone in tone languages (e.g., Leather, 1983; Fox and Qi, 1990; Moore and Jongman, 1997; Huang and Holt, 2009, 2012). Furthermore, the spectral-context-based effects reported here are not constrained to the specific consonant contrast (/g/ versus /d/) reported in the present study. Other research has shown that categorization of vowel contrasts is shifted by the LTAS of preceding context (Vitela et al., 2010; Huang and Holt, 2012) and that categorization of Mandarin tones is shifted by the average voice pitch (fundamental frequency, f0) in preceding context (Huang and Holt, 2009, 2011). In these studies, the effects were elicited by both speech and non-speech precursors and were in the directions predicted by the LTAS model (spectrally contrastive to the LTAS of preceding contexts).
The correspondence of the effects of speech and non-speech contexts on speech categorization strongly implicates the involvement general auditory mechanisms. One ubiquitous neural mechanism consistent with the contrastive shifts in perception observed in these effects is neural adaptation (Harris and Dallos, 1979; Smith, 1979; Carandini, 2000). In a pool of auditory neurons encoding frequency, a precursor with a higher-frequency LTAS would be better encoded by a particular subset of this pool. Having fired robustly to the precursor, neural adaptation would predict that this subset of neurons would exhibit decreased responsiveness to any subsequent stimuli. Thus, at the population level, the encoding of the subsequent speech target would be shifted relative to encoding in isolation or following a precursor with a lower-frequency LTAS.
However, the present results are unlikely to arise from sensory adaptation (e.g., at the level of the cochlea or auditory nerve) because they persist even when non-speech contexts and speech targets are presented to opposite ears (Holt and Lotto, 2002; Lotto et al., 2003), when silent intervals between context and target preclude peripheral interactions (Holt, 2005), and when spectrally neutral sounds intervene between context and target (Holt, 2005).
Stimulus specific adaptation, a mechanism demonstrated in inferior colliculus, thalamus, and cortex in the auditory system (Ulanovsky et al., 2004; Perez-Gonzalez et al., 2005; Malmierca et al., 2009; Antunes et al., 2010), may be a better candidate than neural fatigue for supporting the LTAS-driven context effects (Holt, 2006). Research on SSA suggests that auditory neurons track statistical distributions of sounds across rather extended temporal windows and modulate their responsiveness in reaction to this regularity such that responses to infrequent sounds are exaggerated. Thus, SSA serves to enhance acoustic contrast, as observed in the present behavioral results (see Holt, 2006 for further discussion).
An important aspect of the present results is the finding that there is an interaction between the acoustic energy that elicits spectral contrast effects and the range of spectral information relevant for categorizing the speech targets; energy in the region of F3 exerted an influence whereas lower-frequency F1 energy did not. One possibility is that there may be limitations on the spectral range across which a mechanism such as SSA is effective. Another possibility is that there is a top-down, task-, or attention-driven modulation of the frequency range distinguishing speech targets (e.g., the F3 range, in the current experiment) such that the effects of adaptive mechanisms in this range are enhanced (or, conversely, the effects of adaptive mechanisms outside this range are attenuated). The current data do not differentiate between these possibilities and the accounts are not mutually exclusive. Our understanding of neural mechanisms supporting the range-specificity of context effects observed in the current data will benefit from continued development of models of the interaction between effects influencing perceptual encoding, such as adaptation, and top-down modulatory mechanisms (see Jääskeläinen et al., 2011 for further discussion of such interactions).
The present findings do not suggest that LTAS is the only information involved in talker normalization or phonetic context effects. Listeners exhibit long-term effects of talker familiarity (Nygaard and Pisoni, 1998), and speech processing can be influenced even as a function of whether a listener imagines that speech is produced by a male versus female talker (Johnson, 1990; Johnson et al., 1999) or that there are one versus two talkers present (Magnuson and Nusbaum, 2007). Whereas the present data demonstrate talker-specific information is not necessary to observed shifts in speech categorization, they do not preclude the possibility that voice- or speech-specific processes (e.g., Belin et al., 2000) may also contribute. These expectation-based and long-term memory effects are not inconsistent with mechanisms that support LTAS effects. Rather, they are likely to complement talker normalization through other information sources.
The flip-side of the question of how a general auditory process affects speech processing is asking what purpose LTAS computations may serve in non-speech auditory perception, in general. Lotto and Sullivan (2007) have proposed that sensitivity to LTAS may be useful for noise reduction in natural environments. If noise sources such as babbling brooks and ceiling fans have relatively constant spectra, then perception of sound events (including speech) would be more efficient by determining the LTAS of the noise and subtracting it off or, equivalently, using it as a reference so that all sounds are perceived relative to their spectral change from the ambient sound environment. Such a system would be very effective at dealing with other structured variance in auditory signals such as the filtering characteristics of communication channels (Watkins, 1991) or the systematic acoustic differences among talkers. How significant a role this general process plays in speech communication and other complex sound processing remains to be described, but the data from the current experiment strongly support the significance of the phenomenon in talker normalization, one of the most enduring theoretical issues in speech perception research.
Conflict of Interest Statement
The authors declare that the research was conducted in the absence of any commercial or financial relationships that could be construed as a potential conflict of interest.
Acknowledgments
The authors thank Christi Gomez and Anthony Kelly for their assistance. The research was supported by a grant from the National Institutes of Health (R01DC004674), training grants from the National Institutes of Health (T90DA022761, T32MH019983), and a National Science Foundation Graduate Research Fellowship to Ran Liu. Correspondence may be addressed to Lori L. Holt, loriholt@cmu.edu.
References
Antunes, F. M., Nelken, I., Covey, E., and Malmierca, M. S. (2010). Stimulus-specific adaptation in the auditory thalamus of the anesthetized rat. PLoS ONE 5, e14071. doi:10.1371/journal.pone.0014071
Atal, B. S., Chang, J. J., Mathews, M. V., and Tukey, J. W. (1978). Inversion of articulatory-to-acoustic transformation in the vocal tract by a computer-sorting technique. J. Acoust. Soc. Am. 63, 1535–1555.
Belin, P., Zatorre, R. J., Lafaille, P., Ahad, P., and Pike, B. (2000). Voice-selective areas in human auditory cortex. Nature 403, 309–312.
Diehl, R. L., Elman, J. L., and McCusker, S. B. (1978). Contrast effects on stop consonant identification. J. Exp. Psychol. Hum. Percept. Perform. 4, 599–609.
Eimas, P. D., and Corbit, J. D. (1973). Selective adaptation of linguistic feature detectors. Cogn. Psychol. 4, 99–109.
Fox, R., and Qi, Y. (1990). Contextual effects in the perception of lexical tone. J. Chin. Linguist. 18, 261–283.
Halle, M., and Stevens, K. N. (1962). Speech recognition: a model and a program for research. IEEE Trans. Inf. Theory 8, 155–159.
Harris, D. M., and Dallos, P. (1979). Forward masking of auditory-nerve fiber responses. J. Neurophysiol. 42, 1083–1107.
Hawkins, S. (2004). “Puzzles and patterns in 50 years of research on speech perception,” in From Sound to Sense: 50+ Years of Discoveries in Speech Communication, eds J. Slifka, S. Manuel, and M. Matthies (Cambridge, MA: MIT Press), 223–246.
Holt, L. L. (1999). Auditory Constraints on Speech Perception: An Examination of Spectral Contrast. Doctoral dissertation. Madison: University of Wisconsin-Madison.
Holt, L. L. (2005). Temporally non-adjacent non-linguistic sounds affect speech categorization. Psychol. Sci. 16, 305–312.
Holt, L. L. (2006). The mean matters: effects of statistically-defined nonspeech spectral distributions on speech categorization. J. Acoust. Soc. Am. 120, 2801–2817.
Holt, L. L., and Lotto, A. J. (2002). Behavioral examinations of the neural mechanisms of speech context effects. Hear. Res. 167, 156–169.
Huang, J., and Holt, L. L. (2009). General perceptual contributions to lexical tone normalization. J. Acoust. Soc. Am. 125, 3983–3994.
Huang, J., and Holt, L. L. (2011). Evidence for the central origin of lexical tone normalization. J. Acoust. Soc. Am. 129, 1145–1148.
Huang, J., and Holt, L. L. (2012). Listening for the norm: adaptive coding in speech categorization. Front. Psychol. 3:10 doi:10.3389/fpsyg.2012.00010.
Jääskeläinen, I. P., Ahveninen, J., Andermann, M. L., Belliveau, J. W., Raij, T., and Sams, M. (2011). Short-term plasticity as a neural mechanism supporting memory and attentional functions. Brain Res. 1422, 66–81.
Johnson, K., Ladefoged, P., and Lindau, M. (1993). Individual differences in vowel production. J. Acoust. Soc. Am. 94, 701–714.
Johnson, K., Strand, E. A., and D’Imperio, M. (1999). Auditory-visual integration of talker gender in vowel perception. J. Phon. 27, 359–384.
Kiefte, M., and Kluender, K. R. (2008). Absorption of reliable spectral characteristics in auditory perception. J. Acoust. Soc. Am. 123, 366–376.
Kiefte, M. J., and Kluender, K. R. (2001). Spectral tilt versus formant frequency in static and dynamic vowels. J. Acoust. Soc. Am. 109, 2294–2295.
Klatt, D. H., and Klatt, L. C. (1990). Analysis, synthesis, and perception of voice quality variations among female and male talkers. J. Acoust. Soc. Am. 87, 820–857.
Ladefoged, P., and Broadbent, D. E. (1957). Information conveyed by vowels. J. Acoust. Soc. Am. 29, 98–104.
Liberman, A. M., Cooper, F. S., Shankweiler, D. P., and Studdert-Kennedy, M. (1967). Perception of the speech code. Psychol. Rev. 74, 431–461.
Lotto, A. J., and Holt, L. L. (2006). Putting phonetic context effects into context: a commentary on Fowler (2006). Percept. Psychophys. 68, 178–183.
Lotto, A. J., and Kluender, K. R. (1998). General contrast effects of speech perception: effect of preceding liquid on stop consonant identification. Percept. Psychophys. 60, 602–619.
Lotto, A. J., and Sullivan, S. C. (2007). “Speech as a sound source,” in Auditory Perception of Sound Sources, eds W. A. Yost, R. R. Fay, and A. N. Popper (New York: Springer Science and Business Media, LLC), 281–305.
Lotto, A. J., Sullivan, S. C., and Holt, L. L. (2003). Central locus of non-speech context effects on phonetic identification. J. Acoust. Soc. Am. 113, 53–56.
Magnuson, J. S., and Nusbaum, H. C. (2007). Acoustic differences, listener expectations, and the perceptual accommodation of talker variability. J. Exp. Psychol. Hum. Percept. Perform. 33, 391–409.
Malmierca, M. S., Cristaudo, S., Perez-Gonzalez, D., and Covey, E. (2009). Stimulus-specific adaptation in the inferior colliculus of the anesthetized rat. J. Neurosci. 29, 5483–5493.
McGowan, R. S., and Cushing, S. (1999). Vocal tract normalization for midsagittal articulatory recovery with analysis-by-synthesis. J. Acoust. Soc. Am. 106, 1090–1105.
Moore, C. B., and Jongman, A. (1997). Speaker normalization in the perception of Mandarin Chinese tones. J. Acoust. Soc. Am. 102, 1864–1877.
Nygaard, L. C., and Pisoni, D. B. (1998). Talker-specific learning in speech perception. Percept. Psychophys. 60, 355–376.
Perez-Gonzalez, D., Malmierca, M. S., and Covey, E. (2005). Novelty detector neurons in the mammalian auditory midbrain. Eur. J. Neurosci. 22, 2879–2885.
Peterson, G. E., and Barney, H. L. (1952). Control methods used in a study of the vowels. J. Acoust. Soc. Am. 24, 175–184.
Poeppel, D., Idsardi, W. J., and van Wassenhove, V. (2008). Speech perception at the interface of neurobiology and linguistics. Philos. Trans. R. Soc. B Biol. Sci. 363, 1071–1086.
Potter, R., and Steinberg, J. (1950). Toward the specification of speech. J. Acoust. Soc. Am. 22, 807–820.
Sawusch, J. R., and Nusbaum, H. C. (1979). Contextual effects in vowel perception I: anchor-induced contrast effects. Percept. Psychophys. 25, 292–302.
Schneider, W., Eschman, A., and Zuccolotto, A. (2002). E-Prime User’s Guide. Pittsburgh: Psychology Software Tools, Inc.
Sjerps, M. J., Mitterer, H., and McQueen, J. M. (2011). Constraints on the processes responsible for the extrinsic normalization of vowels. Atten. Percept. Psychophys. 73, 1195–1215.
Smith, R. L. (1979). Adaptation, saturation, and physiological masking in single auditory-nerve fibers. J. Acoust. Soc. Am. 65, 166–178.
Stevens, S. S., Volkman, J., and Newman, E. (1937). A scale for the measurement of the psychological magnitude of pitch. J. Acoust. Soc. Am. 8, 185–190.
Story, B. H. (2005). A parametric model of the vocal tract area function for vowel and consonant simulation. J. Acoust. Soc. Am. 117, 3231–3254.
Ulanovsky, N., Las, L., Farkas, D., and Nelken, I. (2004). Multiple time scales of adaptation in auditory cortex neurons. J. Neurosci. 24, 10440–10453.
Vitela, A. D., Story, B. H., and Lotto, A. J. (2010). Predicting the effect of talker differences on perceived vowel categories. J. Acoust. Soc. Am. 128, 2349.
Wade, T., and Holt, L. L. (2005). Effects of later-occurring non-linguistic sounds on speech categorization. J. Acoust. Soc. Am. 118, 1701–1710.
Watkins, A. J. (1991). Central, auditory mechanisms of perceptual compensation for spectral-envelope distortion. J. Acoust. Soc. Am. 90, 2942–2955.
Watkins, A. J., and Makin, S. J. (1994). Perceptual compensation for speaker differences and for spectral-envelope distortion. J. Acoust. Soc. Am. 96, 1263–1282.
Watkins, A. J., and Makin, S. J. (1996). Effects of spectral contrast on perceptual compensation for spectral-envelope distortions. J. Acoust. Soc. Am. 99, 3749–3757.
Keywords: speech perception, talker normalization, auditory perception
Citation: Laing EJC, Liu R, Lotto AJ and Holt LL (2012) Tuned with a tune: talker normalization via general auditory processes. Front. Psychology 3:203. doi: 10.3389/fpsyg.2012.00203
Received: 21 February 2012; Paper pending published: 01 April 2012;
Accepted: 31 May 2012; Published online: 22 June 2012.
Edited by:
Josef P. Rauschecker, Georgetown University School of Medicine, USAReviewed by:
Iiro P. Jääskeläinen, University of Helsinki, FinlandElia Formisano, Maastricht University, Netherlands
Maria Chait, University College London, UK
Copyright: © 2012 Laing, Liu, Lotto and Holt. This is an open-access article distributed under the terms of the Creative Commons Attribution Non Commercial License, which permits non-commercial use, distribution, and reproduction in other forums, provided the original authors and source are credited.
*Correspondence: Lori L. Holt, Department of Psychology, Carnegie Mellon University, Baker Hall 254K, 5000 Forbes Avenue, Pittsburgh, PA 15213, USA. e-mail: lholt@andrew.cmu.edu