- 1School of Public Health, Graduate School of Medicine, The University of Tokyo, Tokyo, Japan
- 2National Research Institute of Police Science, Kashiwa, Japan
There is no reason to suppose that neighborhood effects based on residents’ trust vary according to administrative boundaries. We examined the relationship between neighborhood trust and cooperative behaviors using the spatial Durbin model which assumed that people are influenced by closer neighbors regardless of administrative boundaries, comparing the results with those of the multilevel model. We used data from 476 residents in Arakawa Ward, Tokyo, Japan. For each respondent, we assigned a unique ‘neighborhood trust’ value weighted by the inverse distance between the respondent and all other respondents as an independent variable. The dependent variables were perceived neighbors’ cooperative behaviors and respondents’ own cooperative behaviors. The spatial Durbin model showed that spatially weighted neighborhood trust was positively associated with cooperative behaviors. Meanwhile, the multilevel models did not show the statistically significant effect of neighborhood trust. We concluded that the spatial model might model the neighborhood effects in society more precisely.
Introduction
In the fields of neighborhood research such as social psychology, public health, and criminology, researchers have studied the effects of ‘place’ as the source of social/environmental influences on people’s behaviors, well-being, and quality of life. For example, the previous studies have demonstrated that the amount of social capital/cohesion (Sampson et al., 2002; Kawachi et al., 2013), economic situation (Robert, 1999; Ross, 2000), welfare policy (Gnanasekaran et al., 2008), and quality of social milieu (Jia et al., 2009) vary among states or municipalities and that they explain a certain level of variance in the health and safety of residents in different areas. In these studies, geographical boundaries such as states, counties, municipalities, school districts, and police districts are used to define the sources of neighborhood effects on residents (Sampson et al., 2002; Takagi, 2013). Given that social policies differ among administrative districts, it is natural that the effects of welfare policy and social milieu on people’s health and safety vary depending on such ministerially defined boundaries.
However, determining neighborhood psychosocial effects such as social capital and cohesion according to administrative boundaries is arbitrary. While neighborhood social capital and cohesion affect residents’ health and safety through social interaction, social support, collective efficacy, informal social control, and cooperative behavior among neighbors (Sampson et al., 1997; Berkman et al., 2014), there is no reason to suppose that these differ according to administrative boundaries (Morenoff et al., 2001). The purpose of this paper is to propose an analytic framework using a spatial definition of ‘neighborhood’ defined by physical distances among residents instead of by administrative boundaries when analyzing social influences. Through this, we sought to bring the perspective of spatial analysis to neighborhood research in the psychology field.
Previous studies have shown that ‘neighborhood-level trust’ is associated with residents’ health and safety. For example, Kawachi et al. (1999) combined multiple datasets and examined the correlation between the percentages of people who did not trust others and those with low self-rated health using data aggregated by states. In their analysis, they found that the proportion of people who were mistrustful was positively associated with those who had poor self-rated health (r = 0.71). Snelgrove et al. (2009) used 250 postcode areas in the United Kingdom as neighborhood units and examined the relationship between trust aggregated at neighborhood-level and individual-level self-rated health using a multilevel model. They found a contextual effect wherein those who live in a neighborhood with a high level of trust were likely to report good self-rated health. Mazerolle et al. (2010) divided the entire city of Brisbane, Australia, into 82 statistical local areas (SLA) and examined the association of SLA-level collective efficacy consisting of social cohesion and trust with self-reported violent victimization. Their results showed that individuals living in areas with high collective efficacy were statistically significantly less likely to be victims of violent crime. Roh and Lee (2013) used a multilevel model to investigate the association between the aggregate-level generalized trust and individual-level robbery victimization using data from 56,071 individuals residing in 56 countries. They demonstrated that country-level generalized trust was negatively related to individual-level robbery victimization, after adjusting for individual-level covariates such as income and lifestyle.
In communities with a high level of neighborhood trust, mutual support and cooperative behaviors among neighbors are likely to increase, resulting in positive effects such as health promotion and crime control. Trust is a facet of one’s expectations of others’ intentions (Yamagishi, 2011). The belief that other people will behave as expected is based on their competence or intentions (Barber, 1983). Yamagishi (2011) and the present study take the view that trust is one’s expectations about whether others have intentions to exploit him/her or act selfishly, not competence to do so. According to Gambetta (1988, p. 217), when we trust someone in this sense, “we implicitly mean that the probability that he will perform an action that is beneficial or at least not detrimental to us is high enough for us to consider engaging in some form of cooperation with him.” A person who trusts that others will cooperate may believe that he/she will not be exploited by them when cooperating; therefore, he/she can cooperate with others (Ahn and Ostrom, 2008). Thus, in neighborhoods where most people trust each other, it is expected that residents will tend to cooperate with each other, and that a person living in such a neighborhood perceives a high rate of cooperative behaviors among neighbors.
In addition, people who are trusted by others are more likely to cooperate on reciprocity with the trustors. Many trust game studies have confirmed that when the trustor shows a trusting behavior, the trustee generally tends to reciprocate to it (Berg et al., 1995; Pillutla et al., 2003; Eilam and Suleiman, 2004). Therefore, it is expected that people surrounded by neighborhood residents demonstrating high trust are more likely to cooperate with their neighbors. Thus, as a social consequence of residents living in a neighborhood where neighboring residents’ trust is high, both perceived surrounding neighbors’ cooperative behaviors and their own cooperative behaviors are expected to increase.
As mentioned previously, while a multilevel model using aggregated data at municipal level would be appropriate when examining the effect of a place characteristic such as a social policy, a model incorporating spatial proximity among residents may be useful for capturing the effects of ‘grass-roots’ social interactions. The relationship between neighborhood trust and cooperative behaviors is an appropriate candidate for the spatial proximity theory; it is reasonable to suggest that trust of those living spatially close by–not just of those living in the same neighborhood–is more likely to be related to one’s own cooperative behaviors and perception of neighbors’ cooperation.
In this context, who are the neighborhood ‘others’ in neighborhood studies? In other words, what is the geographical range that defines a neighborhood? Previous studies examining the relationships between neighborhood social capital/cohesion and health/crime victimization outcomes using multilevel models have incorporated a variety of spatial scales: countries (Roh and Lee, 2013), states (Desai et al., 2005), census areas (Blakely et al., 2006), municipalities (Islam et al., 2008), school districts (Takagi et al., 2013), and zip code areas (Wen et al., 2005). However, there are two problems in defining a neighborhood by geographical boundaries. First, if the size (geographical definition) of the neighborhoods differs, the data may lead to different analysis results depending on that size. This problem is known in geography as the modifiable areal unit problem (MAUP) (Fotheringham and Wong, 1991; Cockings and Martin, 2005). An example is when Mobley et al. (2008) investigated the neighborhood-level factors associated with mammographies among women in California using multilevel models. They conducted their modeling with four different geographical units: postal zip code areas (area-level n = 1450), primary care service area (n = 333), the medical service study area (n = 519), and county (n = 57). The results showed that the associations of neighborhood-level racial segregation index and the poverty rate with the individual-level outcome (i.e., mammography examination) varied greatly depending on the areal units used for the analyses. Tarkiainen et al. (2010) conducted two multilevel model analyses for the relationship between neighborhood-level independent variables, such as the proportion of manual workers, and individual-level mortality, using two different areal units in Helsinki (70 Districts and 258 smaller sub-districts). They reported that the effects of neighborhood-level variables on mortality was slightly greater in the model with a smaller areal unit.
Although, as mentioned above, some studies using the multilevel model framework empirically examined the changeability of results due to the definition of neighborhood, as long as certain geographical boundaries are used, the problem of ignoring the spatial proximity between residents occurs regardless of the geographical unit. As Takagi et al. (2012) stated, people living near the border of an administrative boundary are more affected by their neighbors living in close by but in the next district than they are by residents distant to them but in the same district. Thus, dividing a neighborhood with an administrative boundary ignores the social influences of proximity, and it arbitrarily posits the premise that people are influenced by others who live in the same district. This problem can be briefly expressed in the following statement by Morenoff et al. (2001, p. 522): “Two families living across the street from one another may be arbitrarily assigned to live in different “neighborhoods” even though they share social ties.” Defining a neighborhood based on administrative boundaries ignores grass-root level social interactions and the spatial spillover effect among residents across neighborhood boundaries.
Takagi (2013) defined neighborhood not by administrative boundaries but by the distance between each participant in their survey. They defined ‘neighborhood participants’ by some geographical ranges (e.g., 50 meters, 100 meters, 500 meters, etc.) from each participant and examined the relationship between neighbors’ trust and likelihood of victimization in a crime. Chaix et al. (2005) defined each participant’s nearest 100, 200, 500, 1,000, and 1,500 other participants as his/her ‘neighbors’ and investigated the association between neighbors’ income and his/her mental health. Both studies showed that neighborhood effects were maximized when using the smallest definition of neighborhood. However, in the field of psychology, there are no neighborhood studies that define neighborhood not by administrative boundaries but by distance. In addition, although the previous studies defined multiple neighborhoods regardless of administrative boundaries by using various geographical ranges (Takagi, 2013) and number of surrounding participants (Chaix et al., 2005), and compared neighborhood effects among them, these methods assumed zero influence from people outside of the defined neighborhood, as in the multilevel model framework using administrative boundaries.
To address the above-mentioned limitations in neighborhood effect studies, we defined the neighborhood for each resident using inverse distances to other residents rather than defining it by geographical boundaries. It used the simple idea that regardless of boundaries people are strongly influenced by those nearer to them and less influenced by those more distant. This definition of neighborhood by the inverse distances among respondents is in line with Tobler’s first law of geography: “everything is related to everything else, but near things are more related than distant things” (Tobler, 1970, p. 236). To examine this, we plotted the respondents to the postal survey on a geographic information system (GIS) and created a spatially weighted matrix to represent the inverse distances among all the respondents. Following this, we used the spatial Durbin model (Anselin, 1988) to examine the relationships between neighborhood trust and perceived neighbors’ cooperative behaviors/respondent’s own cooperative behaviors. Thereafter, we compared the results of the spatial analyses with those of multilevel models.
Materials and Methods
Data
This study used data from the second wave of a panel survey (Survey on Neighborhood Crime Prevention and Environmental Problems) conducted in Arakawa Ward, Tokyo. Arakawa Ward is a metropolitan area with a population of 203,296, area of 10.20 km2, and population density of 19,931.0/km2 (2010 Census). In 2009, the researchers conducted a postal survey of 1,000 men and women aged 20 to 69 years (the first-wave survey). In this first-wave survey, the researchers used two-stage random sampling of eligible voters. In the first stage, 10 voter registration ledgers from 32 ledgers were randomly sampled. In the second stage, 100 individuals from each voter registration ledger (100 × 10 = 1,000) were randomly sampled. The response rate was 48.0% (n = 480). In 2011, a postal survey was sent to 469 traceable respondents who responded to the first-wave survey and 500 new men and women aged 20 to 69 years (the second-wave survey). For the 500 new targets in the second-wave survey, the researchers randomly sampled 50 individuals from each voter registration ledger chosen in the first wave survey (50 × 10 = 500). As a result, a questionnaire was sent to 969 (469 + 500) individuals in the second wave survey with the response rate of 57.6% (n = 558).
In the analyses, we omitted 33 respondents with missing values on variables used. Also, for respondents who shared the same address because they lived in the same apartment building, the distance between them equaled zero and the spatial-weighting matrix using the inverse-distance between respondents could not be created. Therefore, we randomly chose one respondent from among those at the same address. As a result, we excluded 49 respondents from the analyses. Finally, we used data from 476 respondents for the analyses. Figure 1 represents the spatial distribution of respondents used in the analyses.
In the multilevel model analyses conducted for later comparison, 476 respondents resided in 26 neighborhoods (18.3 respondents on average per neighborhood, standard deviation was 7.5). The neighborhood unit of the multilevel models in this study was a choumoku, which is the smallest ministerial defined areal unit in Japan. The average size of a choumoku in Arakawa Ward was 0.1962 km2.
Measurements
Dependent Variables
Since the original purpose of the survey was to examine the associations between neighborhood social relationships and crime victimization, measurements of cooperative behaviors included items related to crime prevention in the neighborhood. For perceived neighbors’ cooperative behaviors, the survey asked respondents about their perception of neighborhood watch, which is one of the most common informal social controls conducted in the neighborhood in Japan (Richey, 2005): “Patrol by residents on foot or bicycle” and “Residents watching children making their way to and from school.” Participants responded on a four-point Likert scale (1 = not seen at all, 2 = not seen very much, 3 = sometimes seen, 4 = frequently seen). In the analyses, we used the average of these two items (Cronbach’s alpha = 0.613). Since neighborhood watches can be conducted without governmental approval or financial support (Garofalo and McLeod, 1989) and are based on collective action among residents (Richey, 2005), it is appropriate to set neighbors’ participation in such activities as a dependent variable for the purpose of examining the relationship between trust and cooperative behaviors in the neighborhood.
A previous study measured the neighborhood watch variable as a dichotomous variable, representing whether neighbors joined with other neighbors to prevent crime (Richey, 2005). Other studies asked respondents whether “neighborhood or community watches” and “volunteer surveillance of residential neighborhoods by residents” would deter crime. This was done to measure the perceived impact of informal control on deterrence of committing crime and demonstrate their reasonable internal consistency (α = 0.73) and high factor loadings on a factor in factor analysis (0.81 for both items) (Jiang et al., 2010, 2012). However, the respondents’ perceptions of effectiveness of neighborhood watches are inappropriate for measuring perceptions of how much such cooperative behaviors are conducted in the neighborhood. While some previous studies dealt with neighborhood watches, there are no existing measurements that fit the purpose of the present study; therefore, this study created the above two specific items. These items reflect the fact that neighborhood watches in Japan are carried out by volunteers traveling by foot or bicycle or when children are walking to and from school (National Police Agency, 2014), enabling respondents to recall more specific situations than the above previous studies.
In addition, respondents’ cooperative behaviors was measured by asking the following items: “Participation in a self-governing association and discussion of various facets of the neighborhood,” “When seeing a suspicious person in neighborhood, questioning him/her,” “Usually, I care when unusual things (strange sounds or suspicious figures) can be seen around neighbors’ houses,” “Cooperating with neighbors to clean up parks and roads in the neighborhood,” and “When seeing a car or bike that is illegally parked in the neighborhood, notify the police.” Participants again responded on a four-point Likert scale (1 = never, 2 = not so much, 3 = sometimes, 4 = often). In the analyses, we used the average of these five items (Cronbach’s alpha = 0.786).
In questions about values and attitudes, Japanese respondents are more likely than respondents from Taiwan, Canada, and the United States to choose the midpoint on scales (Chen et al., 1995). Therefore, for the Likert scale items, this study adopted an even number of options without a midpoint. Although six possible responses is the usual practice for an even-numbered Likert scale (DeVellis, 2012), this study used 4-point scales in order to reduce respondents’ response time and cognitive load.
Independent Variable
The present study measured respondents’ trust in others using the following single item extracted from Yamagishi and Yamagishi’s (1994) General Trust Scale: “Most people are trustworthy.” This item indicated the highest or second highest factor loading in the factor analyses of the General Trust Scale items (Yamagishi and Yamagishi, 1994; Lazzarini et al., 2008; Yamagishi et al., 2015). Participants responded on a four-point Likert scale (1 = strongly disagree, 2 = disagree, 3 = agree, 4 = strongly agree). This was used as a continuous variable in the analyses.
Previous studies have demonstrated that this single item of trust was statistically significantly associated with lifestyle (Xue and Cheng, 2017), depressive symptoms (Qin and Hsieh, 2018), and happiness (Yamada et al., 2013). In addition, a Turkish study using this item showed that people’s trust pronouncedly decreased after the failed coup d’état attempt, as theoretically expected (Akkemik et al., 2019). These studies suggest the criterion-related validity of this item.
Covariates
We adjusted the analyses for sociodemographic covariates including the sex of the respondents, educational level, perceived social class, residence year, and the presence of primary school children in the household (as this has been reported associated with crime prevention participation in local communities) (Hope and Lab, 2001; Lee and Cho, 2018). In particular, socioeconomic positions indicators such as educational level and perceived social class can also be confounding factors related to both dependent and independent variables (e.g., selection of residential area). While age has also been reported to be associated with crime prevention-related cooperative behaviors (Hope and Lab, 2001; Lee and Cho, 2018), it was not included in the analyses in order to avoid multicollinearity with the variable of residence year. For educational level, we coded respondents with college/vocational school or less education as 0 and those with a university degree or higher as 1. Since asking about actual income tends to lower response rate, we asked respondents about their perceived social class as an alternative indicator of their economic situation: “If Japanese society is divided into five groups, to which group do you think you belong?” We obtained responses from four predetermined categories (1 = lowest, 2 = lower middle, 3 = upper middle, 4 = highest). We considered residence year as a continuous variable. For the presence of primary school children in the household, we coded respondents who lived with primary school children as 1, without as 0.
As mentioned previously, the dependent variables of the survey included cooperative behaviors relevant to crime prevention. Therefore, we adjusted for fear of crime as a potential confounder of the independent variable (i.e., trust) and the dependent variables (i.e., cooperative behaviors) (Skogan and Lurigio, 1992). The fear of crime was assessed by asking about each respondent’s fears of “burglary,” “car or motorbike theft/car break-ins,” “bicycle theft,” “vandalism to cars or houses,” “street crime (purse-snatching, indecency, or extortion),” and “life-threatening crimes.” Participants responded on a four-point Likert scale (1 = do not feel anxious at all, 2 = do not feel anxious, 3 = feel anxious, 4 = feel highly anxious). In the analyses, the average of these six items was used (Cronbach’s alpha = 0.845).
Statistical Analysis
We used the spatial Durbin model (Anselin, 1988) to examine the relationships between neighborhood trust and cooperative behaviors:
where W is the inverse-distance spatial-weighting matrix. ρ is the spatial autoregression parameter, representing the spatial dependency of neighbors’ dependent variable. β1 is the regression coefficient of the independent variable X1 (e.g., trust), and β2 is the regression coefficient of other respondents’ independent variable X1 weighted by the inverse-distance weighting matrix. β3 is the regression coefficient of a covariate X2 (e.g., sex). ε is the error term having a mean of 0 and a variance of σ2.
The present study created the spatial-weighting matrix using inverse distances among respondents. That is, since there were 476 respondents in this study, we created a matrix of 476 × 476. Table 1 shows the dimension of the inverse-distance spatial-weighting matrix and the minimum and maximum of the inverse distance among respondents. For respondents living in the same apartment building, we randomly used data from one of them for the analyses and did not include respondents with 0 distance in the matrix.
Table 1 shows that the distance between the nearest respondents was 0.013154 km (1/76.0237) and that of the most distant respondents was 3.180662 km (1/0.3144). That is, in this matrix, large values are stored in dyads with a short distance, and small ones are stored in dyads with a long distance. In our analysis, we used the minmax-normalized matrix in which each element was divided by the minimum of the largest row sum and column sum of the matrix (Drukker et al., 2013).
For each respondent, we weighted other respondents’ values of trust according to the inverse distances among them. Following this, we assigned the average of the weighted values of all other respondents’ trust to each respondent as an independent variable. Thus, each respondent was assigned a unique exposure to neighborhood trust in which the inverse distances regardless of geographical boundaries defined neighborhood influence. In addition to trust, for each respondent, we also weighted other respondents’ dependent variable (i.e., perceived neighborhood residents’ cooperative behavior/respondent’s behaviors) by the inverse-distance spatial-weighting matrix and included them in our statistical models as the spatial autoregression term.
Additionally, multilevel models were conducted for comparison. In these models, individuals were treated as level-1 and neighborhoods were level-2. The unit of the neighborhood was the choumoku. The level-1 model was represented by the following equation that predicts the dependent variable of individual i within neighborhood j (Raudenbush and Bryk, 2002):
where β0j is the intercept, β1j is the regression coefficient of the individual-level explanatory variable X1ij (e.g., trust), β2j is the regression coefficient of an individual-level covariate X2ij (e.g., sex), and rij is the level-1 residuals. The intercept β0j is differed for each neighborhood, that is, representing the variation in the average value of the dependent variable among neighborhoods. The level-2 model that predicts β0j using the neighborhood-level explanatory variable is expressed as follows:
where γ00 is the level-2 intercept, γ01 is the regression coefficient of the neighborhood-level explanatory variable Wj (i.e., the average value of trust for each neighborhood), and u0j is the level-2 residuals (i.e., level-2 random effect). That is, these models predict the individual-level dependent variable using individual-level independent variables (i.e., individual-level trust and covariates) and the neighborhood-level independent variable (i.e., neighborhood-level trust). A statistically significant γ01 means that neighborhood-level trust explains the variation in the dependent variable among neighborhoods, in other words, that neighborhood-level trust is associated with the dependent variable. Since this study used two dependent variables (perceived neighbors’ cooperative behaviors and respondents’ own cooperative behaviors), we conducted two multilevel model analyses applied to each.
Guided by Kreft and de Leeuw (1998), in order to avoid multicollinearity between individual- and neighborhood-level trust, they were centered on group mean and grand mean, respectively. That is, for neighborhood-level trust, we subtracted the grand mean of trust (2.464) from each neighborhood’s average value of trust. For individual-level trust, the average value of neighborhood (i.e., group mean) in which each individual was embedded was subtracted from each individual’s value of trust. The number of neighborhood-level observations was 26, and that of individual-level was 476, with an average of 18.3 respondents per neighborhood (standard deviation was 7.5).
We used spmat, spreg, and mixed commands of Stata 15.1 (Stata Corp, Texas, United States) for creating the inverse-distance spatial-weighting matrix, running the spatial Durbin model analyses, and running the multilevel model analyses, respectively.
Results
Table 2 shows the descriptive statistics of the participants. The number of men and women were almost the same, and those with educational background beyond university graduate were about 30%. Based on the responses on the four-point Likert scales, means of respondents’ cooperative behaviors and their perceived neighborhood residents’ cooperative behaviors were 1.71 and 2.98, respectively.
Table 3 shows results of the spatial Durbin models. Perceived neighbors’ cooperative behaviors was positively associated with subjective social class, residence year, the presence of elementary school children in the household, and fear of crime. Respondents’ trust, as well as neighborhood trust weighted by the inverse distance (spatial lag term), also had statistically significant positive associations with perceived neighbors’ cooperative behaviors. In addition, the dependent variable showed statistically significant spatial autocorrelation (rho). This meant that perceived neighborhood residents’ cooperation was spatially dependent on each other.
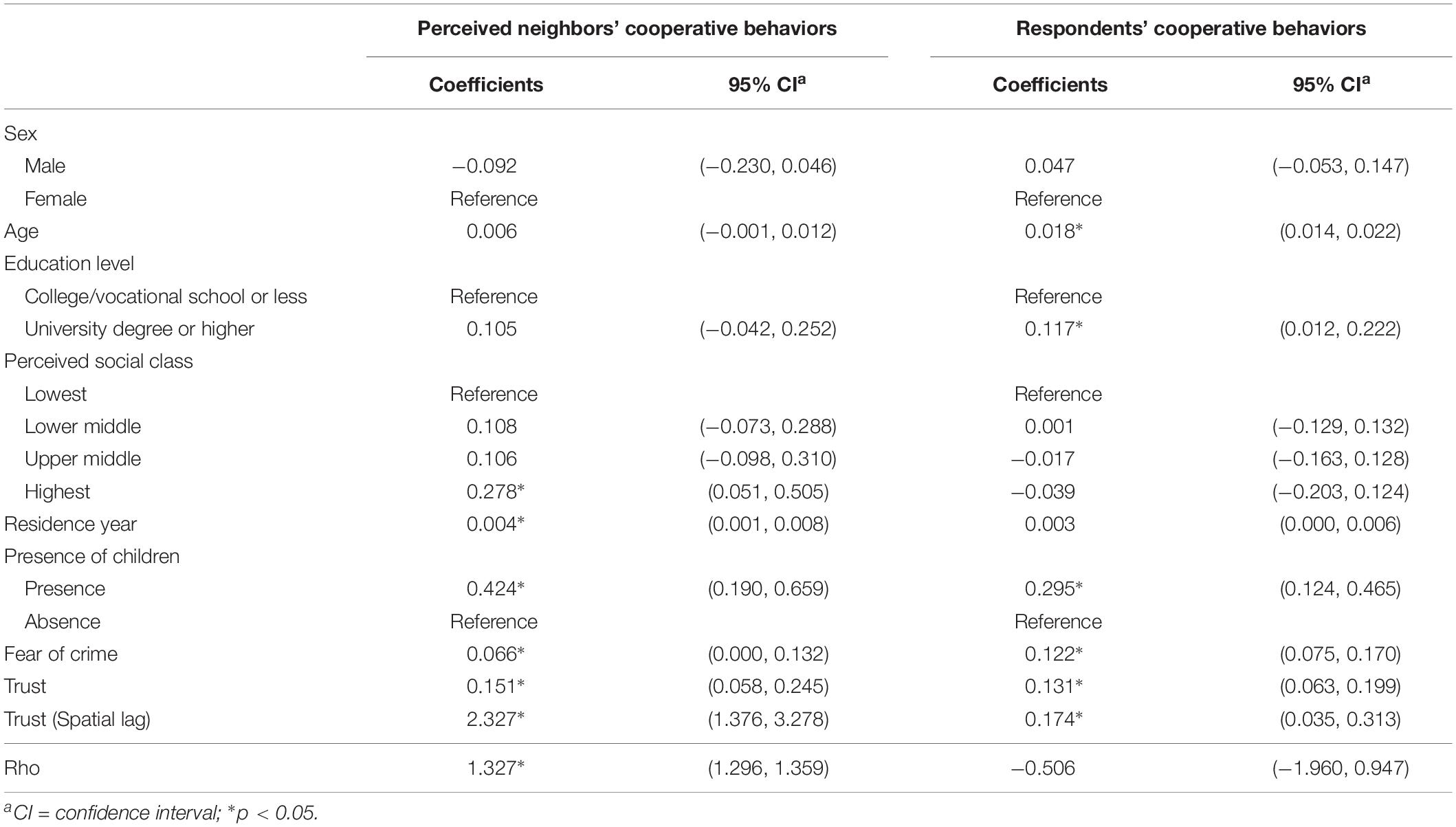
Table 3. Spatial Durbin model estimates for perceived neighbors’/respondents’ cooperative behaviors (n = 476).
Next, respondents’ cooperative behaviors was associated with age, educational level, the presence of elementary school children in the household, and fear of crime. Individual trust, as well as spatially weighted neighborhood trust, were positively related to the respondents’ cooperative behaviors. On the other hand, respondents’ cooperative behaviors did not have a statistically significant spatial autocorrelation.
Table 4 shows the results of the multilevel models. Perceived neighborhood cooperative behaviors was associated with residence year, the presence of elementary school children in the household, and fear of crime. Individual- and neighborhood-level trust were not statistically significant. In addition, the variance component was small, indicating that there is a slight unexplained variance in the perceived neighbors’ cooperation among neighborhoods.
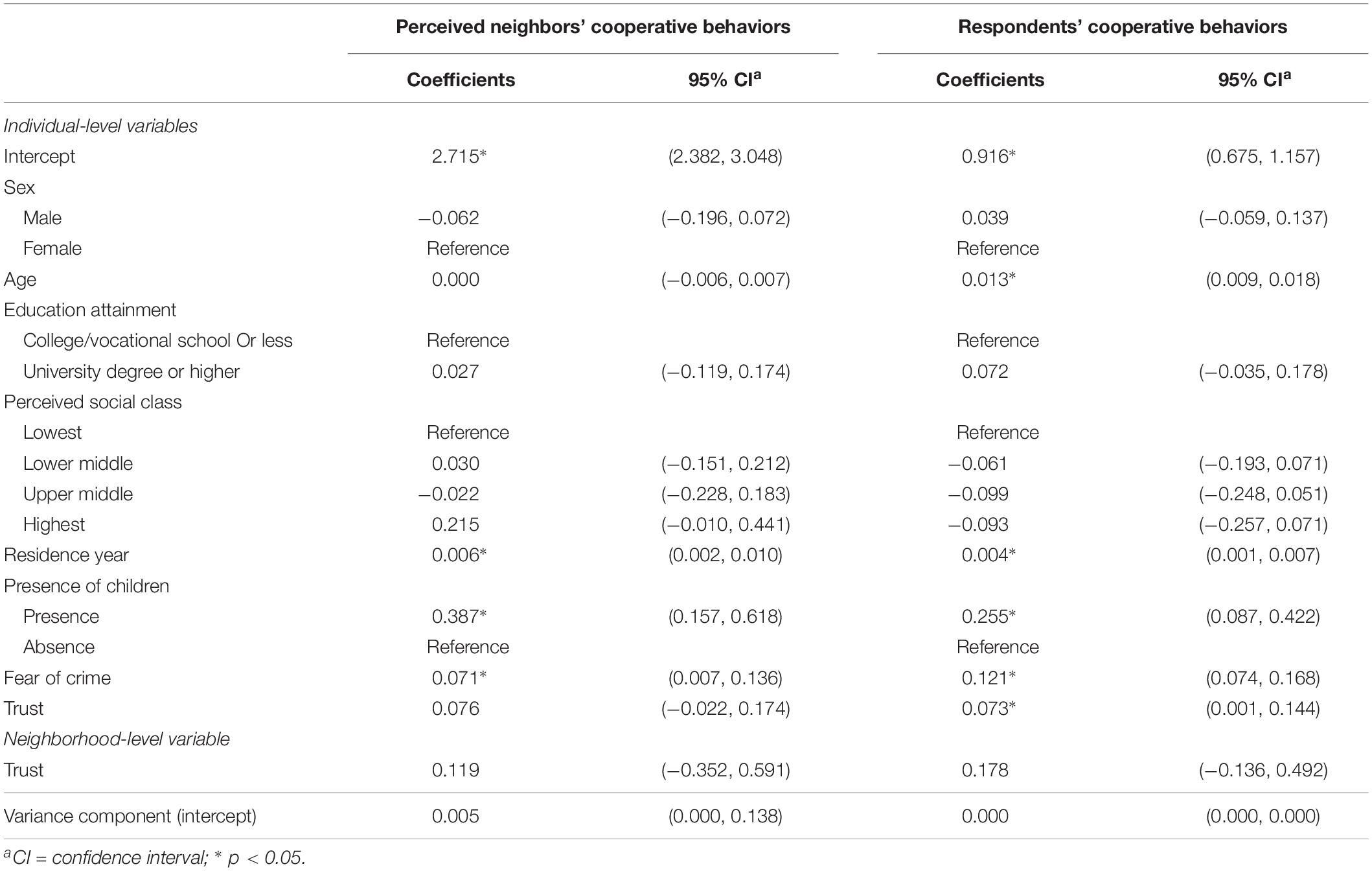
Table 4. Multilevel estimates for perceived neighbors’/respondents’ cooperative behaviors (individual-level n = 476, neighborhood-level n = 26).
Respondents’ cooperative behaviors was positively associated with age, residence year, the presence of elementary school children, and fear of crime. While individual-level trust had a statistically significant positive association with respondents’ cooperative behaviors, neighborhood-level trust was not statistically significantly related to it. Similar to the model of perceived neighbors’ cooperation, there is a slight unexplained variance among neighborhoods.
Discussion
The spatial Durbin model analyses showed that neighborhood trust had positive associations with perceived neighbors’/respondents’ cooperative behaviors. The important point of this study is that when we examined the relationships between the neighborhood-level (choumoku) trust and individual-level cooperative behaviors using the conventional multilevel models, the relationships between them were not statistically significant. One reason for these contrasting results between spatial models and multilevel models may be that the variances in the dependent variables among neighborhoods were very small in multilevel models. The neighborhood effects of trust addressed in this study may have occurred with neighborhood residents living very nearby, and the analyses using administrative districts might not be appropriate for capturing it. If we had analyzed our data using only conventional multilevel models with a single unit of the neighborhood, our study conclusion would have been that “neighborhood trust was not associated with cooperative behaviors.”
The implication of this study is that the influence from neighbors who live closer may be more important than that of a neighborhood unit such as a choumoku. That is, while the effect of trust seems to have a spatial spillover effect, our analyses suggest that this may not be captured by using neighborhood geographical districts such as a multilevel model that ignores the proximity between the respondents. As mentioned in the introduction, while it is fairly obvious that cooperative behaviors occur when surrounded by neighbors who trust each other (e.g., Ahn and Ostrom, 2008; Yamagishi, 2011), the neighbors mentioned here are not those who merely live within the same geographical district but those who live in the closer neighborhood (specifically, in terms of geographical range where influence actually reaches). The reason for this is obvious: while trust among neighborhood residents–who are likely to have social interactions–is important for people’s cognition (e.g., intention to behave), the importance of ‘distant neighbors’ who are unlikely to have actual social interactions is low. The multilevel model analyses included information on residents who had a weak neighborhood effect as “noise” in neighborhood-level variables.
However, in a society wherein people’s social influence processes are clearly defined by a geographical boundary, results different to this study may be found. For example, in areas where administrative neighborhood units such as a school district undertake social activities (e.g., neighborhood watch), analyses using the units (e.g., a multilevel model) may well explain the variance of the dependent variable.
Some limitations of this study should be noted. First, for variables used in the present study, reliability and validity were not established. For example, perceived neighbors’ cooperative behaviors contained only two items, indicating low Cronbach’s alpha value (0.613), and was mainly relevant to neighborhood watch activities, suggesting low validity as items to measure the concept of cooperative behaviors. In addition, trust, the independent variable of this study, was measured by only one item. Although this item was extracted from the General Trust Scale (Yamagishi and Yamagishi, 1994), whose reliability and validity have been repeatedly confirmed (Yamagishi, 1988; Yamagishi and Cook, 1993; Yamagishi et al., 2005, 2015), and was one of the most representative items of the scale, using only a single item did not guarantee reliability and validity. Future studies need to examine whether the spatial analysis proposed in this study is effective in psychological research, using a set of psychological scales whose reliability and validity are established.
Second, this study used 4-point Likert-type scales for measuring the dependent and independent variables because Japanese respondents are more likely to choose the midpoint when odd-numbered options are used (Chen et al., 1995). However, the small number of options may contribute to the low reliability coefficients for the variables of this study (Churchill and Peter, 1984; Weng, 2004). Third, although residents of urban areas of Japan living in a large apartment building are likely to have social interactions mainly within the building, this study used data from only one respondent from each apartment building. This may have led to an underestimation of the neighborhood effect. Fourth, the null findings of the effects of neighborhood-level trust in multilevel models may be attributable to the small number of neighborhood-level observations (n = 26). In addition, the geographical size of neighborhood units used in the multilevel models (choumoku) might be too small. Fifth, since we conducted this study in one of Japan’s metropolitan areas, the generalizability of our findings is limited.
Nevertheless, this study suggested that the spatial model may be appropriate for modeling a certain type of neighborhood effect in society more precisely. On the other hand, multilevel models or ecological analyses that use a more understandable unit of analysis may be more convenient when communicating with policy makers/practitioners and developing neighborhood intervention methods. It is essential to choose an appropriate approach with theoretical and practical considerations to understand the relationships between independent and dependent variables examined.
Data Availability Statement
The datasets generated for this study are available on request to the corresponding author.
Ethics Statement
The studies involving human participants were reviewed and approved by the Ethics Committee at Graduate School of Humanities and Sociology, The University of Tokyo. Written informed consent for participation was not required for this study in accordance with the national legislation and the institutional requirements.
Author Contributions
DT contributed to developing the theoretical framework, data collection, data analysis, and overall writing. TS contributed to data analysis and revising the manuscript critically.
Funding
This study was supported by the Japan Society for the Promotion of Science (Grant Numbers: 22-8772 and 17H02046).
Conflict of Interest
The authors declare that the research was conducted in the absence of any commercial or financial relationships that could be construed as a potential conflict of interest.
References
Ahn, T., and Ostrom, E. (2008). “Social capital and collective action,” in The Handbook of Social Capital, eds D. Castiglione, J. van Deth, and G. Wolleb, (Oxford: Oxford University Press), 70–100.
Akkemik, K. A., Çiçek, G., Horioka, C. Y., and Niimi, Y. (2019). The impact of a failed coup d’état on happiness, life satisfaction, and trust: the case of the plot in Turkey on July 15, 2016. Appl. Econ. Lett. doi: 10.1080/13504851.2019.1683137
Anselin, L. (1988). Spatial Econometrics: Methods and Models. Boston, MA: Kluwer Academic Publisher.
Berg, J., Dickhaut, J., and McCabe, K. (1995). Trust, reciprocity, and social history. Game Econ. Behav. 10, 122–142. doi: 10.1006/game.1995.1027
Berkman, L. F., Kawachi, I., and Glymour, M. M. (2014). Social Epidemiology, 2nd Edn, New York, NY: Oxford University Press.
Blakely, T., Atkinson, J., Ivory, V., Collings, S., Wilton, J., and Howden-Chapman, P. (2006). No association of neighbourhood volunteerism with mortality in New Zealand: a national multilevel cohort study. Int. J. Epidemiol. 35, 981–989. doi: 10.1093/ije/dyl088
Chaix, B., Merlo, J., Subramanian, S. V., Lynch, J., and Chauvin, P. (2005). Comparison of a spatial perspective with the multilevel analytical approach in neighborhood studies: the case of mental and behavioral disorders due to psychoactive substance use in Malmö, Sweden, 2001. Am. J. Epidemiol. 162, 171–182. doi: 10.1093/aje/kwi175
Chen, C., Lee, S., and Stevenson, H. W. (1995). Response style and cross-cultural comparisons of rating scales among East Asian and North American students. Psychol. Sci. 6, 170–175. doi: 10.1111/j.1467-9280.1995.tb00327.x
Churchill, G. A., and Peter, J. P. (1984). Research design effects on the reliability of rating scales: a meta-analysis. J. Mark. Res. 21, 360–375. doi: 10.1016/j.jsurg.2019.07.007
Cockings, S., and Martin, D. (2005). Zone design for environment and health studies using pre-aggregated data. Soc. Sci. Med. 60, 2729–2742. doi: 10.1016/j.socscimed.2004.11.005
Desai, R. A., Dausey, D. J., and Rosenheck, R. A. (2005). Mental health service delivery and suicide risk: the role of individual patient and facility factors. Am. J. Psychiat. 162, 311–318. doi: 10.1176/appi.ajp.162.2.311
DeVellis, R. F. (2012). Scale Development: Theory and Applications, 3rd Edn, Thousand Oaks, CA: Sage Publications.
Drukker, D. M., Peng, H., Prucha, I. R., and Raciborski, R. (2013). Creating and managing spatial-weighting matrices with the spmat command. Stata J. 13, 242–286. doi: 10.1177/1536867x1301300202
Eilam, O., and Suleiman, R. (2004). Cooperative, pure, and selfish trusting: their distinctive effects on the reaction of trust recipients. Eur. J. Soc. Psychol. 34, 729–738. doi: 10.1002/ejsp.227
Fotheringham, S. A., and Wong, D. W. S. (1991). The modifiable areal unit problem in multivariate statistical analysis. Environ. Plan. A 23, 1025–1044. doi: 10.1186/1476-072X-10-58
Gambetta, D. (1988). “Can we trust trust?,” in Trust, ed. D. Gambetta, (New York, NY: Basil Blackwell), 213–234.
Garofalo, J., and McLeod, M. (1989). The structure and operations of neighborhood watch programs in the United States. Crime Delinq. 35, 326–345.
Gnanasekaran, S. K., Boudreau, A. A., Soobader, M., Yucel, R., Hill, K., and Kuhlthau, K. (2008). State policy environment and delayed or forgone care among children with special health care needs. Matern. Child Health J. 12, 739–746. doi: 10.1007/s10995-007-0296-y
Hope, T., and Lab, S. P. (2001). Variation in crime prevention participation: evidence from the British crime survey. Crime Prev. Commun. Saf. 3, 7–22. doi: 10.1057/palgrave.cpcs.8140078
Islam, K. M., Gerdtham, U., Gullberg, B., Lindström, M., and Merlo, J. (2008). Social capital externalities and mortality in Sweden. Econ. Hum. Biol. 6, 19–42. doi: 10.1016/j.ehb.2007.09.004
Jia, H., Moriarty, D. G., and Kanarek, N. (2009). County-level social environment determinants of health-related quality of life among US adults: a multilevel analysis. J. Commun. Health 34, 430–439. doi: 10.1007/s10900-009-9173-5
Jiang, S., Lambert, E., and Jenkins, M. (2010). East meets west: Chinese and U.S. college students’ views on formal and informal crime control. Int. J. Offender. Ther. 54, 264–284. doi: 10.1177/0306624X08330191
Jiang, S., Lambert, E. G., Saito, T., and Hara, J. (2012). University students’ views of formal and informal control in Japan: an exploratory study. Asian J. Criminol. 7, 137–152. doi: 10.1007/s11417-012-9126-2
Kawachi, I., Kennedy, B. P., and Glass, R. (1999). Social capital and self-rated health: a contextual analysis. Am. J. Public Health 89, 1187–1193. doi: 10.2105/ajph.89.8.1187
Kawachi, I., Takao, S., and Subramanian, S. V. (2013). Global Perspectives on Social Capital and Health. New York, NY: Springer.
Lazzarini, S. G., Miller, G. J., and Zenger, T. R. (2008). Dealing with the paradox of embeddedness: the role of contracts and trust in facilitating movement out of committed relationships. Organ. Sci. 19, 709–728. doi: 10.1287/orsc.1070.0336
Lee, J., and Cho, S. (2018). The impact of crime rate, experience of crime, and fear of crime on residents’ participation in association: studying 25 districts in the City of Seoul, South Korea. Crime Prev. Commun. Saf. 20, 189–207. doi: 10.1057/s41300-018-0047-6
Mazerolle, L., Wickes, R., and McBroom, J. (2010). Community variations in violence: the role of social ties and collective efficacy in comparative context. J. Res. Crime Delinq. 47, 3–30. doi: 10.1177/0022427809348898
Mobley, L. R., Kuo, T., and Andrews, L. (2008). How sensitive are multilevel regression findings to defined area of context? A case study of mammography use in California. Med. Care Res. Rev. 65, 315–337. doi: 10.1177/1077558707312501
Morenoff, J. D., Sampson, R. J., and Raudenbush, S. W. (2001). Neighborhood inequality, collective efficacy, and the spatial dynamics of urban violence. Criminology 39, 517–558. doi: 10.1111/j.1745-9125.2001.tb00932.x
National Police Agency (2014). Activities of Local Residents and Volunteer Groups Conducting Voluntary Crime Prevention. Available at: http://www.npa.go.jp/safetylife/seianki55/news/doc/seianki20140403.pdf doi: 10.1111/j.1745-9125.2001.tb00932.x (accessed November 20, 2019).
Pillutla, M. M., Malhotra, D., and Murnighan, K. J. (2003). Attributions of trust and the calculus of reciprocity. J. Exp. Soc. Psyhcol. 39, 448–455. doi: 10.1016/s0022-1031(03)00015-5
Qin, X., and Hsieh, C. (2018). Understanding and addressing the treatment gap in mental healthcare: economic perspectives and evidence from China. Paper Presented at the UNNC School of Economics Working Paper Series: Series H Health Economics, Nottingham.
Raudenbush, S. W., and Bryk, A. S. (2002). Hierarchical Linear Models: Applications and Data Analysis Methods, 2nd Edn, Thousand Oakes, CA: Sage Publications.
Richey, S. (2005). Informal social networking and community involvement: determining participation in neighborhood crime watches in Japan. J. Polit. Sci. 33, 143–161.
Robert, S. A. (1999). Socioeconomic position and health: the independent contribution of community socioeconomic context. Annu. Rev. Sociol. 25, 489–516. doi: 10.1146/annurev.soc.25.1.489
Roh, S., and Lee, J. (2013). Social capital and crime: a cross-national multilevel study. Int. J. Law Crime Justice 41, 58–80. doi: 10.1016/j.ijlcj.2012.11.004
Ross, C. E. (2000). Neighborhood disadvantage and adult depression. J. Health Soc. Behav. 41, 177–187.
Sampson, R. J., Morenoff, J. D., and Gannon-Rowley, T. (2002). Assessing “neighborhood effects”: social processes and new directions in research. Annu. Rev. Sociol. 28, 443–478. doi: 10.1146/annurev.soc.28.110601.141114
Sampson, R. J., Raudenbush, S. W., and Earls, F. (1997). Neighborhoods and violent crime: a multilevel study of collective efficacy. Science 277, 918–924. doi: 10.1126/science.277.5328.918
Skogan, W. G., and Lurigio, A. J. (1992). The correlates of community antidrug activism. Crime Delinq. 38, 510–521. doi: 10.1177/0011128792038004007
Snelgrove, J. W., Pikhart, H., and Stafford, M. (2009). A multilevel analysis of social capital and self-rated health: evidence from the British household panel survey. Soc. Sci. Med. 68, 1993–2001. doi: 10.1016/j.socscimed.2009.03.011
Takagi, D. (2013). “Neighborhood social capital and crime,” in Global Perspectives on Social Capital and Health, eds I. Kawachi, S. Takao, and S. V. Subramanian, (New York, NY: Springer), 143–166. doi: 10.1007/978-1-4614-7464-7_6
Takagi, D., Ikeda, K., and Kawachi, I. (2012). Neighborhood social capital and crime victimization: comparison of spatial regression analysis and hierarchical regression analysis. Soc. Sci. Med. 75, 1895–1902. doi: 10.1016/j.socscimed.2012.07.039
Takagi, D., Kondo, K., Kondo, N., Cable, N., Ikeda, K., and Kawachi, I. (2013). Social disorganization/social fragmentation and risk of depression among older people in Japan: multilevel investigation of indices of social distance. Soc. Sci. Med. 83, 81–89. doi: 10.1016/j.socscimed.2013.01.001
Tarkiainen, L., Martikainen, P., Laaksonen, M., and Leyland, A. H. (2010). Comparing the effects of neighbourhood characteristics on all-cause mortality using two hierarchical areal units in the capital region of Helsinki. Health Place 16, 409–412. doi: 10.1016/j.healthplace.2009.10.008
Tobler, W. R. (1970). A computer movie simulating urban growth in the detroit region. Econ. Geogr. 46, 234–240.
Wen, M., Cagney, K. A., and Christakis, N. A. (2005). Effect of specific aspects of community social environment on the mortality of individuals diagnosed with serious illness. Soc. Sci. Med. 61, 1119–1134. doi: 10.1016/j.socscimed.2005.01.026
Weng, L. (2004). Impact of the number of response categories and anchor labels on coefficient alpha and test-retest reliability. Educ. Psychol. Measur. 64, 956–972. doi: 10.1177/0013164404268674
Xue, X., and Cheng, M. (2017). Social capital and health in China: exploring the mediating role of lifestyle. BMC Public Health 17:863. doi: 10.1186/s12889-017-4883-6
Yamada, T., Chen, C., Hanaoka, C., and Ogura, S. (2013). Empirical investigation of declining childbirth: psychosocial and economic conditions in Japan. Paper Presented at the Asia Health Policy Program Working Paper Series, Stanford, CA.
Yamagishi, T. (1988). The provision of a sanctioning system in the United States and Japan. Soc. Psychol. Q. 51, 265–271.
Yamagishi, T., Akutsu, S., Cho, K., Inoue, Y., Li, Y., and Matsumoto, Y. (2015). Two component model of general trust: predicting behavioral trust from attitudinal trust. Soc. Cogn. 33, 436–458. doi: 10.1521/soco.2015.33.5.436
Yamagishi, T., and Cook, K. S. (1993). Generalized exchange and social dilemma. Soc. Psychol. Q. 56, 235–248.
Yamagishi, T., Kanazawa, S., Mashima, R., and Terai, S. (2005). Separating trust from cooperation in a dynamic relationship: prisoner’s dilemma with variable dependence. Ration. Soc. 17, 275–308. doi: 10.1177/1043463105055463
Keywords: trust, cooperative behavior, spatial Durbin model, spatial regression analysis, multilevel model
Citation: Takagi D and Shimada T (2019) A Spatial Regression Analysis on the Effect of Neighborhood-Level Trust on Cooperative Behaviors: Comparison With a Multilevel Regression Analysis. Front. Psychol. 10:2799. doi: 10.3389/fpsyg.2019.02799
Received: 30 August 2019; Accepted: 27 November 2019;
Published: 19 December 2019.
Edited by:
Edson Filho, University of Central Lancashire, United KingdomReviewed by:
Umit Tokac, University of Missouri–St. Louis, United StatesFrancisco Miguel Leo, University of Extremadura, Spain
Copyright © 2019 Takagi and Shimada. This is an open-access article distributed under the terms of the Creative Commons Attribution License (CC BY). The use, distribution or reproduction in other forums is permitted, provided the original author(s) and the copyright owner(s) are credited and that the original publication in this journal is cited, in accordance with accepted academic practice. No use, distribution or reproduction is permitted which does not comply with these terms.
*Correspondence: Daisuke Takagi, ZHRha2FnaS11dG9reW9AdW1pbi5hYy5qcA==