- Center for Mind/Brain Sciences (CIMeC) – University of Trento, Rovereto, Italy
A reduction in cognitive resources has been originally proposed to account for age-related decrements in several cognitive domains. According to this view, aging limits the pool of available cognitive supplies: Compared to younger adults, elderly exhaust the resources more rapidly as task difficulty increases, hence a dramatic performance drop. Neurophysiological indexes (e.g., BOLD response and EEG activity) may be instrumental to quantify the amount of such cognitive resources in the brain and to pinpoint the stage of stimulus processing where the decrement in age-related resources is evident. However, as we discuss in this mini-review, the most recent studies on the neurophysiological markers of age-related changes lack a consistent coupling between neural and behavioral effects, which casts doubt on the advantage of measuring neural indexes to study resource deployment in aging. For instance, in the working memory (WM) domain, recent cross-sectional studies found varying patterns of concurrent age-related brain activity, ranging from equivalent to reduced and increased activations of old with respect to younger adults. In an attempt to reconcile these seemingly inconsistent findings of brain-behavior coupling, we focus on the contribution of confounding sources of variability and propose ways to control for them. Finally, we suggest an alternative perspective to explain age-related effects that implies a qualitative (instead of or along with a quantitative) difference in the deployment of cognitive resources in aging.
Age-Related Reduction in Processing Resources
A marked decline across several cognitive domains is a common feature of aging (Hedden and Gabrieli, 2004). To account for this performance reduction, the processing resources framework (Craik and Byrd, 1982; Salthouse, 1988, 1990) posits that aging implies a decline in the amount of available cognitive resources, in that older individuals exhaust them more rapidly than younger adults. According to this account, the performance drop, measured as task requests increase, occurs because old individuals consume processing resources already at low levels of cognitive demand. The age-related changes in working memory (WM) capacity, a hallmark of cognitive aging (Myerson et al., 2003), nicely fit with this perspective. Indeed, in simple short-term memory tasks (mainly tapping on storage capacity, e.g., forward digit span), old adults are negligibly compromised. However, compared to young, they become impaired in WM tasks requiring additional cognitive processing (namely, concurrent storage and manipulation of items, e.g., backward or complex digit span; see Bopp and Verhaeghen, 2005, for a review). These effects may be interpreted within the processing resources framework: The reduced pool of cognitive resources in aging is sufficient for the elderly to efficiently perform in simple tasks (e.g., short-term memory tasks), but not when they have to face higher cognitive demands (e.g., in WM tasks), and thus, a greater performance decrement is visible. An interesting approach has been proposed to substantiate the hypothesis of age-related reduction in processing resources (thought to be responsible for the observed behavioral deficits): measuring the neural activity (e.g., BOLD response and M/EEG activity) during the execution of various tasks, and isolating specific indexes that mirror the hypothesized resource decrement (e.g., McEvoy et al., 2001; Mattay et al., 2006). This approach is beneficial for at least two reasons. First, it provides an additional (cerebral) measure to quantify the amount of available resources in the elderly; second, it individuates the specific neural and functional mechanism where age-related differences in processing deployment originate. As Salthouse (1988) originally suggested, the candidate neural index of cognitive resources should positively correlate with cognitive performance (i.e., the greater the cerebral recruitment, the better the performance) and negatively correlate with age (i.e., the older the participant, the more reduced the cerebral activity). However, finding a neural measure that satisfies these requirements has proven to be difficult in the field of aging research. Indeed, results obtained from cross-sectional studies highlight that a consistent coupling between neural and cognitive modulations is currently lacking, which complicates predictions on how the neural markers of cognitive resource deployment should be modulated by age. A review of the most recent (in the last 5years) imaging studies (fMRI and M/EEG) on WM provides substantial examples of these non-unidirectional patterns of age-related brain activity. Indeed, WM has been proposed as an ideal domain to test for the presence of any age-related variation in cognitive resources, since it is defined by a limited capacity and is relevant to other cognitive domains (Salthouse, 1990). In the next section, we will briefly illustrate some of the most recent results (note, however, that similar conclusions can be drawn when also considering articles published earlier than 2016). As we will describe, linking brain and cognition in an attempt to quantify the amount of available processing resources in aging is far from being a straightforward research approach.
Neural Indexes Underlying Cognitive Resource Deployment in Aging
fMRI and M/EEG studies investigating WM in young and older adults have used various tasks (e.g., verbal and visuo-spatial n-back, delayed match-to-sample, Corsi-Block Tapping, and Sternberg paradigm; see Table 1 for further details on recent studies). Across these paradigms, elderly usually exhibit a reduction of WM capacity compared to young adults. However, such decrements in performance are mirrored by different patterns of brain activity.
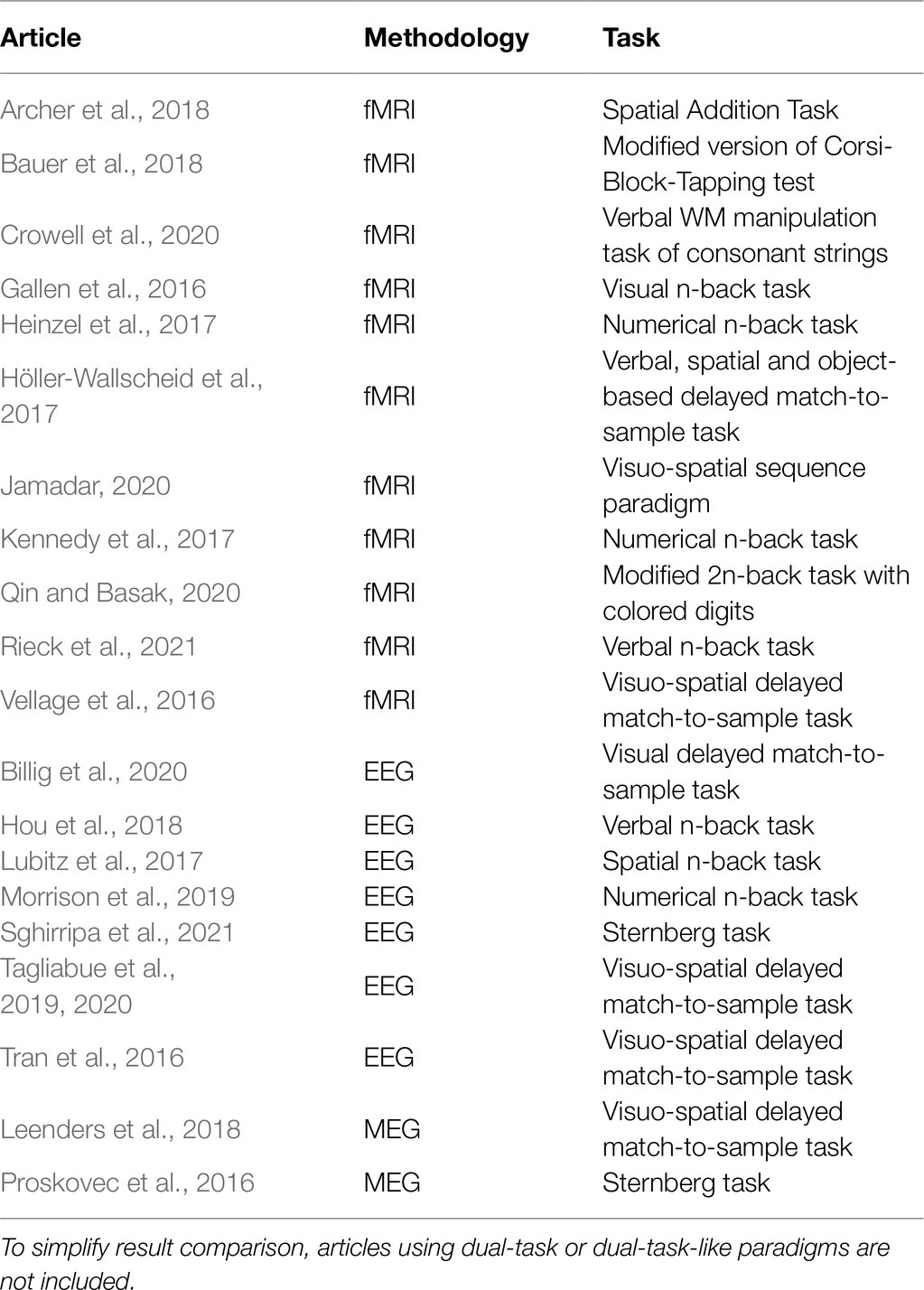
Table 1. Neuroimaging studies comparing young and older adults in WM tasks and published from 2016 onwards.
Recent fMRI studies (Gallen et al., 2016; Heinzel et al., 2017; Kennedy et al., 2017; Archer et al., 2018; Bauer et al., 2018; Jamadar, 2020; Qin and Basak, 2020) show that the behavioral decrease in WM capacity of old adults is coupled with equal, increased, or reduced brain activation relative to younger adults. Moreover, different brain regions (or even different portions within the same region) show opposite patterns of age-related activity: While some of them are underrecruited, others appear overactive with respect to young. For instance, age-related decrement in WM performance can be accompanied by a reduced activation of task-related areas – middle frontal gyrus and bilateral precunei – together with increased activation of task-unrelated regions – cuneus, temporal gyrus, and cerebellum (Archer et al., 2018). In addition, at lower levels of task demand elderly can exhibit larger activations in frontal and parietal areas (Heinzel et al., 2017), but also reduced BOLD activity in frontal and temporal regions, with concurrent larger activation in the bilateral cuneus (Jamadar, 2020). Likewise, connectivity measures for easy task conditions indicate increased connectivity between lateral frontal areas and other networks with increasing age (Gallen et al., 2016), but no difference in connectivity strength between frontal and parietal regions (Heinzel et al., 2017).
Similar findings are observed when M/EEG studies are considered (Schwarzkopp et al., 2016; Tran et al., 2016; Lubitz et al., 2017; Morrison et al., 2019; Tagliabue et al., 2019, 2020): Taken together, the findings indicate that components reflecting attentional engagement and maintenance in WM may be enhanced, reduced or similar between age groups, even in the presence of marked behavioral differences. For instance, some EEG studies found decrements in older adults’ WM with a concurrent less pronounced (Lubitz et al., 2017) or enhanced fronto-central P200 (Morrison et al., 2019), an ERP component reflecting deployment of attentional resources. Additionally, when individuals are presented with different memory loads, older adults might show either similar (Schwarzkopp et al., 2016; Tran et al., 2016) or reduced (Tagliabue et al., 2019, 2020) load-related modulations of the CDA, an ERP response indexing the amount of items maintained in the WM short-term storage.
To summarize, recent findings on aging highlight an apparent lack of a unidirectional coupling between brain and behavioral outcomes. The absence of a consistent brain-behavior pattern ultimately questions the possibility of formulating testable hypotheses on age-related effects at the neural level and, more generally, whether we can reliably interpret neural activity (being it BOLD signals, ERPs, neural oscillations, or functional connectivity) to infer the amount of deployed cognitive resources in aging. In the next section, we will consider potential sources of variability accounting for these different effects.
Potential Sources of Variability Accounting For Different Age-Related Patterns of Resource Deployment in Working Memory
At least two sources of variability can account for the different brain-behavior associations in the WM domain. First, as previously mentioned, various cognitive tasks have been used to test WM functioning. Even if meant to assess the same cognitive function, different experimental paradigms can yield different results for at least two (partially related) main reasons. Different tasks might selectively engage different cognitive subcomponents (and their respective neural substrates), depending on their experimental structure (e.g., delayed match-to-sample paradigms tax more information maintenance and retrieval abilities, whereas n-back tasks rely more on information updating; see Daniel et al., 2016; Yaple et al., 2019) and type of material (verbal and visuo-spatial). Consequently, some tasks can intrinsically be more difficult than others. For instance, regarding the experimental structure, the overall accuracy in an n-back task is lower than in a Sternberg test (see Heinzel et al., 2016). With reference to stimulus material, elderly are usually more impaired with visuo-spatial than verbal items (Jenkins et al., 2000).
The second source of variability that may account for non-unidirectional age-related patterns is interindividual variability. Interindividual variability is prominent in aging (Lindenberger and von Oertzen, 2006) and may lead to optimal or less successful aging trajectories (Reuter-Lorenz and Park, 2010; Reuter-Lorenz and Park, 2014; Cabeza et al., 2018). Indeed, in some studies, the sample of older adults might include high-performing participants that can bias the group average performance toward one direction (and vice versa in the case of low-performing elderly). This heterogeneity in aging trajectories is largely due to age-related changes at multiple levels of neurobiological function and structure (Raz and Daugherty, 2018), in interaction with environmental factors (Daffner et al., 2011). Thus, interindividual variability in aging may underlie differences in the expression of brain activations (Cabeza et al., 2018). Specifically, preserving a good cognitive level at old age could be reflected by either a youth-like functioning brain (i.e., no age-related differences in brain activity; e.g. Pudas et al., 2013), an overactivation of some areas and/or supplementary engagement of an alternative set of brain networks (see Spreng et al., 2010 for a review) that might act as compensatory mechanisms to support the behavioral performance.
In the next section, we will consider possible solutions to minimize task-related variability and to better operationalize individual differences. Indeed, when sources of variability are (at least partially) accounted for, a more consistent pattern of age-related neural effects emerges, that can be more easily interpreted in the framework of cognitive resource deployment with a life span perspective.
How Can Cognitive Resources in Aging Be More Reliably Measured Through Neural Indexes?
The use of various experimental paradigms to address the same cognitive function and individual differences are two major sources of variability that could explain the heterogeneity of findings in aging research. In particular, since individual differences are typically more prominent in older than young adults, they have been suggested to bias (e.g., by under- or over-estimating) the age-related differences observed in cross-sectional studies, where aging is implicitly treated as a uniform process (Schneider-Garces et al., 2010).
In an attempt to reduce the joint influence of task-related and interindividual variability, some studies (e.g., Höller-Wallscheid et al., 2017) have exploited procedures to equate the subjectively perceived difficulty of a specific task between age cohorts (and, in turn, across participants). These studies often apply titration procedures to match the difficulty level between young and older adults, namely, a stimulation “threshold” yielding the same accuracy value is chosen for each individual. WM studies using these procedures often find that elderly exhibit equal or increased neural activity (with reference to the compensation mechanism previously discussed) or similar load-related modulations (but see Billig et al., 2020). Indeed, recent fMRI studies with no age-related difference in accuracy found a similar modulation as a function of task demands in frontal and parietal areas between young and old adulthood (Höller-Wallscheid et al., 2017; Crowell et al., 2020), recruitment of a more extended network of areas (Vellage et al., 2016), and increased between-networks integration with increasing difficulty in the elderly (Crowell et al., 2020). In addition, M/EEG aging studies with individually titrated difficulty levels (Leenders et al., 2018) or no absolute difference in performance between age groups (Proskovec et al., 2016; Sghirripa et al., 2021) revealed that elderly showed greater increase in cortical excitability (as indexed by greater alpha power decrease; see Rihs et al., 2009) in both hemispheres (Leenders et al., 2018; Sghirripa et al., 2021) with respect to young participants (in which larger cortical excitability was instead specific to the hemisphere primarily processing targets, i.e., the contralateral hemisphere), or greater oscillatory activity in the alpha and beta bands in additional homologous frontal and parieto-occipital regions (Proskovec et al., 2016).
However, matching task difficulty between groups (likely selecting easier task conditions for the elderly) cancels out baseline differences in performance between age cohorts and (only) reveals (potential) age-related modulations of neural activity to attain the same accuracy level. In other words, this approach proves to be useful when the research focus is on within-subject effects (e.g., in the case of individual gains in training procedures), rather than on between-groups differences. Indeed, when difficulty-matching procedures are adopted, what remains to be explained is why older adults are deficient in their WM capacity (see Ramscar et al., 2014) from the very beginning (i.e., why they perceive the same subjective difficulty of younger adults at easier task levels).
When the research focus is on the comparison between different ages, two approaches can be adopted to overcome some of the limitations imposed by cross-sectional studies previously described. On one hand, dividing individuals (both young and old) in high and low performers may offer a less spurious estimate of age-related neural changes in the utilization of cognitive resources. For instance, in an EEG study by Daffner et al. (2011), low and high performers similarly allocated processing resources with increasing difficulty, regardless of age (see also Lubitz et al., 2017 and Morrison et al., 2019 for more recent EEG studies). Similarly, an fMRI study of Nagel et al. (2009) showed that, when considered altogether, elderly exhibited compromised brain responsivity compared to younger adults. Interestingly, when participants were instead split in high and low performers, the neural pattern of high-performing older adults resembled those of low-performing, equally accurate younger adults (see also Heinzel et al., 2017, Bauer et al., 2018 and Vaqué-Alcázar et al., 2020 for more recent fMRI studies).
A second approach to account for interindividual variability and overcome the drawbacks of cross-sectional studies consists of longitudinal investigations. Indeed, results obtained from cross-sectional studies might be biased by cohort effects related to preexisting generational differences (e.g., educational attainment; see Archer et al., 2018) that can “anticipate” age-related decrements (Rönnlund et al., 2005). Longitudinal studies allow researchers to (partially) isolate the effects due to aging from those linked to other experience-related variables (e.g., historical/social background). Notably, some discrepancies between cross-sectional and longitudinal studies have been found also in neural results. For instance, several cross-sectional studies documented over-recruitment of prefrontal areas in old compared to younger adults (e.g., Rajah and D’Esposito, 2005; Davis et al., 2008). However, some longitudinal studies (Nyberg et al., 2010; Rieckmann et al., 2017) reported an age-related reduction in frontal activity. More specifically, older adults defined as decliners (i.e., individuals with WM performance decline across time, as opposed to so-called maintainers) showed decreased recruitment of the prefrontal cortex (Rieckmann et al., 2017; Vaqué-Alcázar et al., 2020, 2021). To reduce the confound of cohort effects, it might be worth contemplating the administration of routine assessment of cognitive functions throughout an individual’s life span.
Concluding Remarks
In a framework arguing for a reduction of processing resources in aging (Craik and Byrd, 1982; Salthouse, 1988, 1990), recent neuroimaging evidence in the domain of WM has not conveyed a unidirectional coupling between behavioral and neural data. However, apparent discrepancies can be reconciled if (at least) two sources of variability are controlled for, namely, task-related and interindividual differences. Indeed, when these factors are considered, two consistent findings emerge as: (1) elderly exhibit similar or augmented neural activity with respect to younger adults and (2) older low performers or longitudinal decliners engage task-related areas to a lesser extent than their more cognitively fit peers.
Taken together, results on age-related differences in brain activity prompt for a deeper understanding of these effects, especially in the case of neural over-recruitment in the elderly, which would ideally challenge the view of reduced processing resources in aging (Salthouse, 1988). In this respect, we suggest to enlarge the hypothesis space: Rather than having a limited pool of resources as originally postulated (Craik and Byrd, 1982; Salthouse, 1988, 1990), older individuals may use them in a different (sometimes less efficient) way compared to young adults. This interpretation would imply a shift from the original view that sees aging as a (quantitative) reduction of processing resources to a novel viewpoint considering a qualitative change, not necessarily a reduction, in resource allocation (Figure 1). Several pieces of evidence support this latter perspective. First, it is well documented that aging is characterized by increased susceptibility to distraction (e.g., Hasher and Zacks, 1988; Gazzaley et al., 2008) and broader attentional focusing (Greenwood and Parasuraman, 1999, 2004). These deficits are responsible for the inadvertent processing of irrelevant material, and this may result in the typical age-related WM capacity reduction (e.g., Jost et al., 2011; Tagliabue et al., 2019). Since WM storage has a limited capacity (Cowan, 2010), WM may become deficient because old adults tend to maintain both target and distracting items. Similarly, evidence of age-related reduced suppression of the default mode network (Raichle et al., 2001) during task execution has been linked to a deficit in cognitive control, which hampers efficient resource allocation to task-related areas with a consequent negative impact on WM performance in the elderly (Sambataro et al., 2010). Finally, the idea of an alternative use of processing resources would also be in line with the Compensation-Related Utilization of Neural Circuits Hypothesis (CRUNCH; Reuter-Lorenz and Cappell, 2008). CRUNCH states that, compared to younger adults, elderly recruit more neural resources (and exhaust them) at lower loads and are left without additional cognitive supplies when task demands further increase. A practical example of age-related qualitative changes in resource allocation might come from studies on the Posterior-Anterior Shift in Aging (PASA; Davis et al., 2008) research line: Elderly show increased engagement of frontal areas that correlates with reduced activity of posterior regions. Such activation pattern was suggested to reflect the involvement of high-order cognitive processes in response to deficits of posterior brain areas.
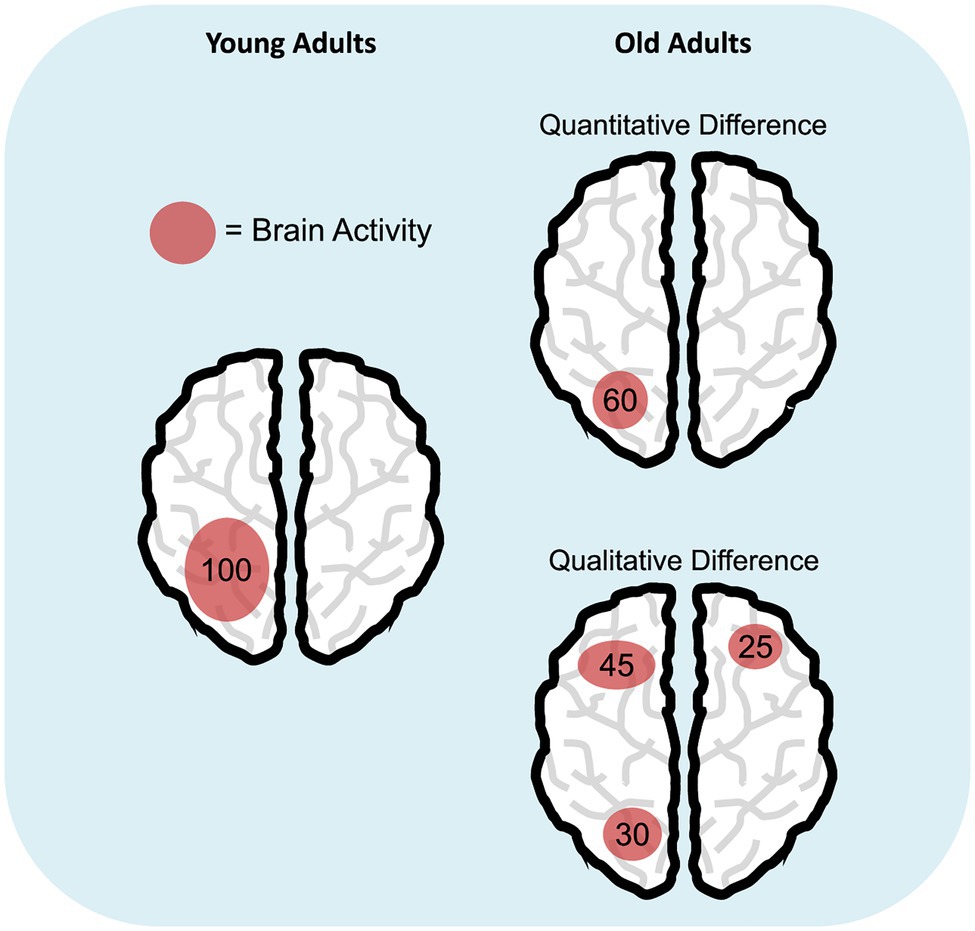
Figure 1. Age-related differences in cognitive resource allocation. The schematic representations illustrate the hypothesis of quantitative (i.e., reduction; top right panel) versus qualitative difference (bottom right panel) in the deployment of cognitive resources in aging, compared to young adulthood (left panel). Numbers represent a hypothetical amount of engaged cognitive resources and how they are distributed during a specific task.
Some final considerations need to be addressed. Given that we focused our mini-review on the WM domain, our conclusions might not be generalized to other cognitive domains, even though age-related limitations in WM seem to account for age-related differences across different tasks (including memory – Baudouin et al., 2009 – and non-memory related domains – Van der Linden et al., 1999; Chen and Li, 2007; Borella et al., 2011). Moreover, while it would be desirable to obtain measures of general cognitive functioning to correlate with neural activations (e.g., Wiegand et al., 2018), such a unique and exhaustive performance index is not easy to choose or compute.
To conclude, on the basis of the recent findings discussed in this mini-review, we suggest that neural measures represent a powerful tool when investigating age-related differences in cognitive resource deployment, provided that some confounding factors are taken into account. Moreover, according to our view, a qualitative change in the deployment of cognitive resources instead or along with a quantitative reduction in the pool of available resources is an alternative hypothesis that deserves further consideration.
Author Contributions
CT and VM equally contributed to conceptualization, literature overview, and writing of the manuscript. All authors contributed to the article and approved the submitted version.
Funding
The publication of this article was funded by the Reversing Age and Resilience in the Elderly (RARE-net) project, University of Trento. CT is supported by a research fellowship from the Fondazione Cassa Di Risparmio Di Trento e Rovereto (CARITRO).
Conflict of Interest
The authors declare that the research was conducted in the absence of any commercial or financial relationships that could be construed as a potential conflict of interest.
Publisher’s Note
All claims expressed in this article are solely those of the authors and do not necessarily represent those of their affiliated organizations, or those of the publisher, the editors and the reviewers. Any product that may be evaluated in this article, or claim that may be made by its manufacturer, is not guaranteed or endorsed by the publisher.
Acknowledgments
The authors thank Alexandria Nicole Holcomb for proofreading the manuscript.
References
Archer, J. A., Lee, A., Qiu, A., and Chen, S.-H. A. (2018). Working memory, age and education: a lifespan fMRI study. PLoS One 13:e0194878. doi: 10.1371/journal.pone.0194878
Baudouin, A., Clarys, D., Vanneste, S., and Isingrini, M. (2009). Executive functioning and processing speed in age-related differences in memory: contribution of a coding task. Brain Cogn. 71, 240–245. doi: 10.1016/j.bandc.2009.08.007
Bauer, E., Sammer, G., and Toepper, M. (2018). Performance level and cortical atrophy modulate the neural response to increasing working memory load in younger and older adults. Front. Aging Neurosci. 10:265. doi: 10.3389/fnagi.2018.00265
Billig, A. R., Feng, N. C., Behforuzi, H., McFeeley, B. M., Nicastri, C. M., and Daffner, K. R. (2020). Capacity-limited resources are used for managing sensory degradation and cognitive demands: implications for age-related cognitive decline and dementia. Cortex 133, 277–294. doi: 10.1016/j.cortex.2020.09.005
Bopp, K. L., and Verhaeghen, P. (2005). Aging and verbal memory span: a meta-analysis. J. Gerontol: Ser B 60, P223–P233. doi: 10.1093/geronb/60.5.P223
Borella, E., Ghisletta, P., and de Ribaupierre, A. (2011). Age differences in text processing: the role of working memory, inhibition, and processing speed. J. Gerontol. Ser. B Psychol. Sci. Soc. Sci. 66B, 311–320. doi: 10.1093/geronb/gbr002
Cabeza, R., Albert, M., Belleville, S., Craik, F. I. M., Duarte, A., Grady, C. L., et al. (2018). Maintenance, reserve and compensation: the cognitive neuroscience of healthy ageing. Nat. Rev. Neurosci. 19, 701–710. doi: 10.1038/s41583-018-0068-2
Chen, T., and Li, D. (2007). The roles of working memory updating and processing speed in mediating age-related differences in fluid intelligence. Aging Neuropsychol. Cognit. 14, 631–646. doi: 10.1080/13825580600987660
Cowan, N. (2010). The magical mystery four: how is working memory capacity limited, and why? Curr. Dir. Psychol. Sci. 19, 51–57. doi: 10.1177/0963721409359277
Craik, F. I. M., and Byrd, M. (1982). “Aging and Cognitive Deficits,” in Aging and Cognitive Processes. eds. F. I. M. Craik and S. Trehub (Boston, MA: Springer US), 191–211.
Crowell, C. A., Davis, S. W., Beynel, L., Deng, L., Lakhlani, D., Hilbig, S. A., et al. (2020). Older adults benefit from more widespread brain network integration during working memory. NeuroImage 218:116959. doi: 10.1016/j.neuroimage.2020.116959
Daffner, K. R., Chong, H., Sun, X., Tarbi, E. C., Riis, J. L., McGinnis, S. M., et al. (2011). Mechanisms underlying age- and performance-related differences in working memory. J. Cogn. Neurosci. 23, 1298–1314. doi: 10.1162/jocn.2010.21540
Daniel, T. A., Katz, J. S., and Robinson, J. L. (2016). Delayed match-to-sample in working memory: a BrainMap meta-analysis. Biol. Psychol. 120, 10–20. doi: 10.1016/j.biopsycho.2016.07.015
Davis, S. W., Dennis, N. A., Daselaar, S. M., Fleck, M. S., and Cabeza, R. (2008). Que PASA? The posterior-anterior shift in aging. Cereb. Cortex. 18, 1201–1209. doi: 10.1093/cercor/bhm155
Gallen, C. L., Turner, G. R., Adnan, A., and D’Esposito, M. (2016). Reconfiguration of brain network architecture to support executive control in aging. Neurobiol. Aging 44, 42–52. doi: 10.1016/j.neurobiolaging.2016.04.003
Gazzaley, A., Clapp, W., Kelley, J., McEvoy, K., Knight, R. T., and D’Esposito, M. (2008). Age-related top-down suppression deficit in the early stages of cortical visual memory processing. Proc. Natl. Acad. Sci. 105, 13122–13126. doi: 10.1073/pnas.0806074105
Greenwood, P. M., and Parasuraman, R. (1999). Scale of attentional focus in visual search. Percept. Psychophys. 61, 837–859. doi: 10.3758/BF03206901
Greenwood, P. M., and Parasuraman, R. (2004). The scaling of spatial attention in visual search and its modification in healthy aging. Percept. Psychophys. 66, 3–22. doi: 10.3758/BF03194857
Hasher, L., and Zacks, R. T. (1988). “Working memory, comprehension, and aging: a review and a new view,” in Psychology of Learning and Motivation. ed. G. H. Bower (San Diego, CA: Academic Press), 193–225.
Hedden, T., and Gabrieli, J. D. E. (2004). Insights into the ageing mind: a view from cognitive neuroscience. Nature reviews. Neuroscience 5, 87–96. doi: 10.1038/nrn1323
Heinzel, S., Lorenz, R. C., Duong, Q.-L., Rapp, M. A., and Deserno, L. (2017). Prefrontal-parietal effective connectivity during working memory in older adults. Neurobiol. Aging 57, 18–27. doi: 10.1016/j.neurobiolaging.2017.05.005
Heinzel, S., Lorenz, R. C., Pelz, P., Heinz, A., Walter, H., Kathmann, N., et al. (2016). Neural correlates of training and transfer effects in working memory in older adults. NeuroImage 134, 236–249. doi: 10.1016/j.neuroimage.2016.03.068
Höller-Wallscheid, M. S., Thier, P., Pomper, J. K., and Lindner, A. (2017). Bilateral recruitment of prefrontal cortex in working memory is associated with task demand but not with age. Proc. Natl. Acad. Sci. 114, E830–E839. doi: 10.1073/pnas.1601983114
Hou, F., Liu, C., Yu, Z., Xu, X., Zhang, J., Peng, C.-K., et al. (2018). Age-related alterations in electroencephalography connectivity and network topology During n-Back working memory task. Front. Hum. Neurosci. 12:484. doi: 10.3389/fnhum.2018.00484
Jamadar, S. D. (2020). The CRUNCH model does not account for load-dependent changes in visuospatial working memory in older adults. Neuropsychologia 142:107446. doi: 10.1016/j.neuropsychologia.2020.107446
Jenkins, L., Myerson, J., Joerding, J. A., and Hale, S. (2000). Converging evidence that visuospatial cognition is more age-sensitive than verbal cognition. Psychol. Aging 15, 157–175. doi: 10.1037/0882-7974.15.1.157
Jost, K., Bryck, R. L., Vogel, E. K., and Mayr, U. (2011). Are old adults just Like low working memory young adults? Filtering efficiency and age differences in visual working memory. Cereb. Cortex 21, 1147–1154. doi: 10.1093/cercor/bhq185
Kennedy, K. M., Boylan, M. A., Rieck, J. R., Foster, C. M., and Rodrigue, K. M. (2017). Dynamic range in BOLD modulation: lifespan aging trajectories and association with performance. Neurobiol. Aging 60, 153–163. doi: 10.1016/j.neurobiolaging.2017.08.027
Leenders, M. P., Lozano-Soldevilla, D., Roberts, M. J., Jensen, O., and De Weerd, P. (2018). Diminished alpha lateralization during working memory but not During attentional cueing in older adults. Cereb. Cortex 28, 21–32. doi: 10.1093/cercor/bhw345
Lindenberger, U., and von Oertzen, T. (2006). “Variability in cognitive aging: From taxonomy to theory,” in Lifespan CognitionMechanisms of Change (Oxford, UK: Oxford University Press), 297–314.
Lubitz, A. F., Niedeggen, M., and Feser, M. (2017). Aging and working memory performance: electrophysiological correlates of high and low performing elderly. Neuropsychologia 106, 42–51. doi: 10.1016/j.neuropsychologia.2017.09.002
Mattay, V. S., Fera, F., Tessitore, A., Hariri, A. R., Berman, K. F., Das, S., et al. (2006). Neurophysiological correlates of age-related changes in working memory capacity. Neurosci. Lett. 392, 32–37. doi: 10.1016/j.neulet.2005.09.025
McEvoy, L. K., Pellouchoud, E., Smith, M. E., and Gevins, A. (2001). Neurophysiological signals of working memory in normal aging. Cogn. Brain Res. 11, 363–376. doi: 10.1016/S0926-6410(01)00009-X
Morrison, C., Kamal, F., and Taler, V. (2019). The influence of working memory performance on event-related potentials in young and older adults. Cogn. Neurosci. 10, 117–128. doi: 10.1080/17588928.2019.1570104
Myerson, J., Emery, L., White, D. A., and Hale, S. (2003). Effects of age, domain, and processing demands on memory span: evidence for differential decline. Aging Neuropsychol. Cognit. 10, 20–27. doi: 10.1076/anec.10.1.20.13454
Nagel, I. E., Preuschhof, C., Li, S.-C., Nyberg, L., Bäckman, L., Lindenberger, U., et al. (2009). Performance level modulates adult age differences in brain activation during spatial working memory. Proc. Natl. Acad. Sci. 106, 22552–22557. doi: 10.1073/pnas.0908238106
Nyberg, L., Salami, A., Andersson, M., Eriksson, J., Kalpouzos, G., Kauppi, K., et al. (2010). Longitudinal evidence for diminished frontal cortex function in aging. Proc. Natl. Acad. Sci. 107, 22682–22686. doi: 10.1073/pnas.1012651108
Proskovec, A. L., Heinrichs-Graham, E., and Wilson, T. W. (2016). Aging modulates the oscillatory dynamics underlying successful working memory encoding and maintenance. Hum. Brain Mapp. 37, 2348–2361. doi: 10.1002/hbm.23178
Pudas, S., Persson, J., Josefsson, M., de Luna, X., Nilsson, L.-G., and Nyberg, L. (2013). Brain characteristics of individuals resisting age-related cognitive decline over two decades. J. Neurosci. 33, 8668–8677. doi: 10.1523/JNEUROSCI.2900-12.2013
Qin, S., and Basak, C. (2020). Age-related differences in brain activation during working memory updating: an fMRI study. Neuropsychologia 138:107335. doi: 10.1016/j.neuropsychologia.2020.107335
Raichle, M. E., MacLeod, A. M., Snyder, A. Z., Powers, W. J., Gusnard, D. A., and Shulman, G. L. (2001). A default mode of brain function. Proc. Natl. Acad. Sci. 98, 676–682. doi: 10.1073/pnas.98.2.676
Rajah, M. N., and D’Esposito, M. (2005). Region-specific changes in prefrontal function with age: a review of PET and fMRI studies on working and episodic memory. Brain 128, 1964–1983. doi: 10.1093/brain/awh608
Ramscar, M., Hendrix, P., Shaoul, C., Milin, P., and Baayen, H. (2014). The myth of cognitive decline: non-linear dynamics of lifelong learning. Top. Cogn. Sci. 6, 5–42. doi: 10.1111/tops.12078
Raz, N., and Daugherty, A. M. (2018). Pathways to brain aging and their modifiers: free-radical-induced energetic and neural decline in senescence (FRIENDS) model - a mini-review. Gerontology 64, 49–57. doi: 10.1159/000479508
Reuter-Lorenz, P. A., and Cappell, K. A. (2008). Neurocognitive aging and the compensation hypothesis. Curr. Dir. Psychol. Sci. 17, 177–182. doi: 10.1111/j.1467-8721.2008.00570.x
Reuter-Lorenz, P. A., and Park, D. C. (2010). Human neuroscience and the aging mind: a new look at old problems. J. Gerontol. Ser. B Psychol. Sci. Soc. Sci. 65B, 405–415. doi: 10.1093/geronb/gbq035
Reuter-Lorenz, P. A., and Park, D. C. (2014). How does it STAC up? Revisiting the scaffolding theory of aging and cognition. Neuropsychol. Rev. 24, 355–370. doi: 10.1007/s11065-014-9270-9
Rieck, J. R., Baracchini, G., and Grady, C. L. (2021). Contributions of brain function and structure to three different domains of cognitive control in Normal aging. J. Cogn. Neurosci. 33, 1–22. doi: 10.1162/jocn_a_01685
Rieckmann, A., Pudas, S., and Nyberg, L. (2017). Longitudinal changes in component processes of working memory. Eneuro 4:0052-17.2017. doi: 10.1523/ENEURO.0052-17.2017
Rihs, T. A., Michel, C. M., and Thut, G. (2009). A bias for posterior α-band power suppression versus enhancement during shifting versus maintenance of spatial attention. NeuroImage 44, 190–199. doi: 10.1016/j.neuroimage.2008.08.022
Rönnlund, M., Nyberg, L., Bäckman, L., and Nilsson, L.-G. (2005). Stability, growth, and decline in adult life span development of declarative memory: cross-sectional and longitudinal data From a population-based study. Psychol. Aging 20, 3–18. doi: 10.1037/0882-7974.20.1.3
Salthouse, T. A. (1988). “The role of processing resources in cognitive aging,” in Cognitive Development in Adulthood: Progress in Cognitive Development Research. eds. M. L. Howe and C. J. Brainerd (New York, NY: Springer), 185–239.
Salthouse, T. A. (1990). Working memory as a processing resource in cognitive aging. Dev. Rev. 10, 101–124. doi: 10.1016/0273-2297(90)90006-P
Sambataro, F., Murty, V. P., Callicott, J. H., Tan, H.-Y., Das, S., Weinberger, D. R., et al. (2010). Age-related alterations in default mode network: impact on working memory performance. Neurobiol. Aging 31, 839–852. doi: 10.1016/j.neurobiolaging.2008.05.022
Schneider-Garces, N. J., Gordon, B. A., Brumback-Peltz, C. R., Shin, E., Lee, Y., Sutton, B. P., et al. (2010). Span, CRUNCH, and Beyond: working memory capacity and the aging brain. J. Cogn. Neurosci. 22, 655–669. doi: 10.1162/jocn.2009.21230
Schwarzkopp, T., Mayr, U., and Jost, K. (2016). Early selection versus late correction: age-related differences in controlling working memory contents. Psychol. Aging 31, 430–441. doi: 10.1037/pag0000103
Sghirripa, S., Graetz, L., Merkin, A., Rogasch, N. C., Semmler, J. G., and Goldsworthy, M. R. (2021). Load-dependent modulation of alpha oscillations during working memory encoding and retention in young and older adults. Psychophysiology 58:e13719. doi: 10.1111/psyp.13719
Spreng, R. N., Wojtowicz, M., and Grady, C. L. (2010). Reliable differences in brain activity between young and old adults: a quantitative meta-analysis across multiple cognitive domains. Neurosci. Biobehav. Rev. 34, 1178–1194. doi: 10.1016/j.neubiorev.2010.01.009
Tagliabue, C. F., Assecondi, S., Cristoforetti, G., and Mazza, V. (2020). Learning by task repetition enhances object individuation and memorization in the elderly. Sci. Rep. 10:19957. doi: 10.1038/s41598-020-75297-x
Tagliabue, C. F., Brignani, D., and Mazza, V. (2019). Does numerical similarity alter age-related distractibility in working memory? PLoS One 14:e0222027. doi: 10.1371/journal.pone.0222027
Tran, T. T., Hoffner, N. C., LaHue, S. C., Tseng, L., and Voytek, B. (2016). Alpha phase dynamics predict age-related visual working memory decline. NeuroImage 143, 196–203. doi: 10.1016/j.neuroimage.2016.08.052
Van der Linden, M., Hupet, M., Feyereisen, P., Schelstraete, M.-A., Bestgen, Y., Bruyer, R., et al. (1999). Cognitive mediators of age-related differences in language comprehension and verbal memory performance. Aging Neuropsychol. Cognit. 6, 32–55. doi: 10.1076/anec.6.1.32.791
Vaqué-Alcázar, L., Abellaneda-Pérez, K., Solé-Padullés, C., Bargalló, N., Valls-Pedret, C., Ros, E., et al. (2021). Functional brain changes associated with cognitive trajectories determine specific tDCS-induced effects among older adults. J. Neurosci. Res. 99:24849. doi: 10.1002/jnr.24849
Vaqué-Alcázar, L., Sala-Llonch, R., Abellaneda-Pérez, K., Coll-Padrós, N., Valls-Pedret, C., Bargalló, N., et al. (2020). Functional and structural correlates of working memory performance and stability in healthy older adults. Brain Struct. Funct. 225, 375–386. doi: 10.1007/s00429-019-02009-1
Vellage, A., Becke, A., Strumpf, H., Baier, B., Schönfeld, M. A., Hopf, J., et al. (2016). Filtering and storage working memory networks in younger and older age. Brain. Behav. 6:e00544. doi: 10.1002/brb3.544
Wiegand, I., Lauritzen, M. J., Osler, M., Mortensen, E. L., Rostrup, E., Rask, L., et al. (2018). EEG correlates of visual short-term memory in older age vary with adult lifespan cognitive development. Neurobiol. Aging 62, 210–220. doi: 10.1016/j.neurobiolaging.2017.10.018
Keywords: cognitive aging, cognitive resources, working memory, interindividual variability, aging trajectories, neural correlates, age-related brain activity change
Citation: Tagliabue CF and Mazza V (2021) What Can Neural Activity Tell Us About Cognitive Resources in Aging? Front. Psychol. 12:753423. doi: 10.3389/fpsyg.2021.753423
Edited by:
Gesine Dreisbach, University of Regensburg, GermanyReviewed by:
Iris Wiegand, Radboud University Nijmegen, NetherlandsAlessia Rosi, University of Pavia, Italy
Copyright © 2021 Tagliabue and Mazza. This is an open-access article distributed under the terms of the Creative Commons Attribution License (CC BY). The use, distribution or reproduction in other forums is permitted, provided the original author(s) and the copyright owner(s) are credited and that the original publication in this journal is cited, in accordance with accepted academic practice. No use, distribution or reproduction is permitted which does not comply with these terms.
*Correspondence: Chiara F. Tagliabue, Y2hpYXJhLnRhZ2xpYWJ1ZUB1bml0bi5pdA==