- 1Leibniz Institute for Psychology (ZPID), Trier, Germany
- 2Leibniz Institute for Resilience Research (LIR), Mainz, Germany
Plain language summaries (PLS) aim to communicate research findings to laypersons in an easily understandable manner. Despite the societal relevance of making psychological research findings available to the public, our empirical knowledge on how to write PLS of psychology studies is still scarce. In this article, we present two experimental studies investigating six characteristics of PLS for psychological meta-analyses. We specifically focused on approaches for (1) handling technical terms, (2) communicating the quality of evidence by explaining the methodological approach of meta-analyses, (3) explaining how synthesized studies operationalized their research questions, (4) handling statistical terms, (5) structuring PLS, and (6) explaining complex meta-analytic designs. To develop empirically validated guidelines on writing PLS, two randomized controlled studies including large samples stratified for education status, age, and gender (NStudy1=2,288 and NStudy2=2,211) were conducted. Eight PLS of meta-analyses from different areas of psychology were investigated as study materials. Main outcome variables were user experience (i.e., perceived accessibility, perceived understanding, and perceived empowerment) and knowledge acquisition, as well as understanding and knowledge of the quality of evidence. Overall, our hypotheses were partially confirmed, with our results underlining, among other things, the importance of explaining or replacing content-related technical terms (i.e., theoretical concepts) and indicating the detrimental effects of providing too many details on statistical concepts on user experience. Drawing on these and further findings, we derive five empirically well-founded rules on the lay-friendly communication of meta-analytic research findings in psychology. Implications for PLS authors and future research on PLS are discussed.
Introduction
Insightful and well-written scientific papers are sometimes difficult to understand. Even researchers like ourselves at times encounter papers, perhaps focusing on new techniques or concepts that we are unfamiliar with, that we find hard to understand. This is more likely to occur when reading papers from disciplines outside our field of expertise – at least if these papers were not explicitly written for an interdisciplinary audience. Simply grasping the main ideas and results of this kind of paper might constitute an arduous task and could potentially hinder the interdisciplinary exchange of knowledge. If this is the case for scientists, imagine the difficulties that non-scientists (i.e., laypersons) face when they are interested in (the findings of) scientific publications with high societal or personal relevance.
Understanding science in general and especially scientific evidence published in journal articles is not easy for laypersons for multiple reasons (Bromme and Goldman, 2014). For a start, scientific articles often employ technical terms and refer to statistical concepts without explaining them to their readers (e.g., Rakedzon et al., 2017; Hauck, 2019). This procedure is undoubtedly often well-justified, as the main audience of scientific journals consists of fellow researchers who are frequently working in the same field as the authors and who have considerable theoretical and statistical expertise (this is closely linked to the notion of the “arena of internal scientific communication” by Peters, 2013). To specifically address the needs and interests of their scientific audience (and relevant gatekeepers such as editors), researchers may pay more attention to the scientific relevance of their findings and less attention to their practical relevance to the broader public when discussing their results in their publications (see also Radford, 2011; Salita, 2015). This strong focus on a scientific audience may, however, make it burdensome for non-scientists to access the current scientific literature to fulfill their need for valid and understandable information (see also Nunn and Pinfield, 2014). There are manifold relevant groups of laypersons in this context: Stakeholders (e.g., for decisions on policies), patients (for health-related decisions in medicine), practitioners, but also the interested public in general may benefit from access to research findings (Kaslow, 2015; Rodgers, 2017). For example, the current COVID-19 crisis highlighted that the public yearns – at least to some degree – for reliable scientific information and guidance (e.g., Post et al., 2021).
To close this gap (i.e., to make knowledge contained in scientific articles available to the public), plain language summaries (PLS) – easily comprehensible research summaries that complement scientific abstracts – were introduced for biomedical research (FitzGibbon et al., 2020). The pioneering role this particular area of research has taken is probably due to the high relevance that its findings have for laypeople’s everyday life (see Santesso et al., 2008). We argue that psychological findings are also of considerable interest to laypeople (see also Kaslow, 2015). Yet most guidelines on how to write PLS focus solely on biomedical research, for example, the Cochrane guidelines (Cochrane Methods, 2013; Cochrane Norway, 2016, 2019), and the scarce empirical research to validate PLS guidelines has almost exclusively taken place in the biomedical field (e.g., Santesso et al., 2015; Raynor et al., 2018; Anzinger et al., 2020; Buljan et al., 2020). Writing PLS for psychological research is thus not a straightforward task. Validated discipline-specific guidance – based on robust evidence from experimental research and taking characteristics idiosyncratic to psychology as a discipline situated at the crossroads between the humanities and the sciences into account – is practically non-existent (see also Teo, 2017). For example, how does a researcher communicate psychological concepts like “attention” or “attachment,” terms commonly used in everyday language that actually describe very sophisticated issues, views, or theories as scientific terms? Alternatively, how should meta-analytic findings that deviate considerably from the usual methodological approach in medicine be explained? This may, for instance, be the case when personality variables are correlated with indicators of well-being. The typical strategy of comparing intervention groups with each other and a control group, as used in drug effectiveness studies, does not apply here. Therefore, we argue that there is a considerable need to develop empirically validated guidelines for writing PLS in psychology. To tackle this issue, we present two randomized controlled studies investigating psychology-specific aspects of how to optimally communicate meta-analytical evidence to laypersons. Based on the findings from these studies, we subsequently provide rules on writing PLS.
In a recent systematic review of theory, guidelines and empirical research on PLS, we included a total of 90 records. These include 36 theoretical articles primarily discussing the rationale behind and the aims of PLS, 21 guidance-related articles and 33 articles reporting empirical studies (Stoll et al., 2021). Based on extracted information from these records, we developed a conceptual framework of the aims, characteristics, criteria, and outcomes investigated in PLS research. The aims of PLS (e.g., to impart knowledge about scientific findings to laypersons) determine the characteristics taken into account when composing PLS (e.g., the kind of words that are used). PLS criteria outlined in guidelines are specifications of such characteristics (e.g., the PLS should not be written in jargon). The effectiveness of such criteria, in turn, is evaluated by their impact on predefined outcomes constituting measureable operationalizations of the aims (e.g., knowledge tests). We found that the aims of PLS mentioned, discussed or investigated in the literature could be classified into six categories: accessibility, understanding, knowledge, empowerment, communication of research, and improvement of research. In the current studies, we focus on the first four aims because they target the individual PLS user level, whereas improvement and communication of research aims relate to (the relationship between) society and academia at a higher level. Accessibility, understanding and empowerment directly relate to a subjective user’s perspective and can, therefore, also be described as aims that refer to user experience, as user experience research focuses primarily on the attractiveness, understandability, and usefulness of a product or medium (e.g., Buchanan, 1992; Morville, 2004; Rosenbaum, 2010). Outcomes measuring user experience aims of PLS in experimental studies comprise subjective statements and self-reports on perceived readability of a PLS (accessibility), perceived ease of comprehending the meaning of a PLS on a content level (understanding) and/or usefulness of a PLS for making decisions (empowerment). Knowledge can be measured using (objective) knowledge tests (e.g., Alderdice et al., 2016; Buljan et al., 2018; Kerwer et al., 2021).
In our review, we identified several shortcomings of the empirical research on PLS carried out so far. Of the 33 empirical articles, we found only eight (24%) contrasted different versions of PLS, whereby only six of them (18%) used an experimental research design to do so (Santesso et al., 2015; Alderdice et al., 2016; Kirkpatrick et al., 2017; Buljan et al., 2020; Silvagnoli et al., 2020). Many empirical studies on the effectiveness of PLS, however, either compared PLS to different summary formats (e.g., Buljan et al., 2018) or only evaluated one single PLS (e.g., Santesso et al., 2015), making it hard to infer the suitability of certain characteristics of a PLS from the observed outcomes or to generalize study results. Furthermore, most empirical studies on PLS employed rather small samples that were far from representative for the general public. Participants were often highly educated (e.g., students, Alderdice et al., 2016, Kerwer et al., 2021; or healthcare professionals, Opiyo et al., 2013; Maguire and Clarke, 2014). The empirical evidence that PLS are effective for the public in general – outside narrow settings of professionals or student samples – is therefore still scarce. Research on PLS needs to address this issue by collecting data of larger and more diverse groups. This is especially important since PLS, for example, when accompanying scientific abstracts in journals, sometimes do not even aim to address a specific target group but are rather directed toward anyone who might be interested in psychological research or wants to know something about the topic at hand. To allow PLS to fulfill their purpose of educating and empowering the public, we thus need to investigate their performance in a heterogeneous readership, as well as how reader characteristics affect this performance (specifically, individual differences in interest in psychological research).
Against the background of an ongoing replication crisis in psychology (Open Science Collaboration, 2015; Świątkowski and Dompnier, 2017; Klein et al., 2018), we have argued that PLS may be a powerful tool for rebuilding public trust in psychological science, and first experimental findings (Kerwer et al., 2021) seem to support this notion (see also Carvalho et al., 2019 for non-experimental findings that point in the same direction). Because meta-analyses possess a higher quality of evidence and are instrumental for summarizing findings and guiding practitioners (Borenstein et al., 2009; Bastian et al., 2010), we further argue that the lay-friendly communication of psychological meta-analytical findings is of particular importance – even though legitimate criticisms on the validity of their findings may exist (e.g., Sharpe and Poets, 2020; Burgard et al., 2021; Patall, 2021).
When it comes to communicating meta-analytic psychological evidence to a broad audience via PLS, several aspects quite specific to psychological research or especially relevant in the context of psychological research exist (e.g., the vocabulary or the operationalization of psychological constructs in primary studies). Our reasoning for choosing such aspects here does not arise from the notion that they are especially difficult to explain in the context of psychology or even that they serve a unique function in this field. Rather, according to Shneider (2009), use of specific language (technical terms describing subject matter) as well as methodology (e.g., operationalization) constitute the core characteristics of a scientific discipline, which distinguish it from others. In psychology, for example, the necessity of operationalizing latent psychological constructs is distinct from other disciplines. Other aspects exist that are highly relevant in the context of psychological research – but not discipline-specific by nature – and for which empirical evidence beyond biomedical research is lacking (e.g., research designs and effect size measures in the social sciences). More specifically, in the life sciences, laypeople might be unfamiliar with the jargon typically used in scientific publications due to the fact that researchers use scientific names for species or illnesses uncommon in everyday language. In this case, technical terms need to be “translated” for some readers. For example, a plain language translation of the title “Salvage systemic therapy for advanced gastric and oesophago-gastric junction adenocarcinoma” is “Which treatments work best for advanced stomach cancer that has not responded to standard chemotherapy?” (Tomita et al., 2020). In psychological research, however, this problem is complex in another way, since the vocabulary of psychological science often does not differ from everyday language (e.g., the word “attachment,” see above). The underlying meaning might differ, however, or these words might represent very specific ideas. Without prior knowledge, laypersons, for example, might not understand what developmental psychologists mean when they are referring to “secure attachment.” In guidelines on writing PLS in medicine (Cochrane Methods, 2013; Cochrane Norway, 2016, 2019) and other disciplines (AGU, n.d.; People and Nature, n.d.), typical approaches for handling technical terms are: (1) Replacing the technical terms by non-technical terms within the text of the PLS. Transferred to the context of psychological PLS, this means that instead of using technical terms, these terms are circumscribed by using everyday language (e.g., “feeling safe in relationships” instead of “secure attachment”) and this simplified description is used consistently throughout the PLS text (replacement); (2) using technical terms within the text but providing an additional glossary on technical terms in which these terms are explained (glossary). In our research, we wanted to compare these two approaches to simply ignoring the problem, that is, to using technical terms within the text of the PLS without any explanation (no explanation). In hypotheses 1 and 2, we assumed that both replacing technical terms with non-technical terms and providing a glossary on technical terms would improve the user experience (perceived accessibility, perceived understanding, and perceived empowerment) and knowledge acquisition of laypeople:
Hypothesis 1: Technical terms: glossary vs. no explanation: Accessibility (H1a), understanding (H1b), content-related knowledge (H1c), and empowerment (H1d) are higher when a glossary explaining technical terms is provided (glossary) compared to leaving technical terms unexplained (no explanation).
Hypothesis 2: Technical terms: replacement vs. no explanation: Accessibility (H2a), understanding (H2b), content-related knowledge (H2c), and empowerment (H2d) are higher when technical terms are replaced by non-technical terms within the text of the PLS (replacement) compared to using technical terms without explanation (no explanation).
Second, we have argued above that meta-analytic research findings constitute an important source of information for laypeople and that this is especially true for psychology in times of the replication crisis. However, communicating meta-analytic evidence in a lay-friendly manner can be challenging. Most laypersons will likely not know that meta-analytical findings draw on multiple studies and therefore have a higher level of evidence quality than individual research findings based on one single study. Thus, to provide laypersons the opportunity to grasp the quality of evidence more accurately when evaluating a meta-analysis, they need to understand that the presented evidence does not stem from a single individual study (see Cochrane Norway, 2019). We argue that laypersons’ further understanding is improved by providing them with a lay-friendly explanation of the methodological approach underlying meta-analytic research. In fact, very current and therefore non-peer reviewed research suggests that this approach (i.e., an explanatory statement) allowed non-scientists to take the quality of evidence of preprints compared to peer-reviewed articles into account when it comes to perceived credibility (Wingen et al., 2021). In hypothesis 3, we assumed that the communication of quality of evidence by an explanation of the methodological approach affects laypersons’ knowledge on the quality of evidence (i.e., factual knowledge on the concept “meta-analysis”) and the understanding of the quality of evidence (taking this knowledge adequately into account in a transfer task).
Hypothesis 3: Quality of evidence communication: statement vs. no statement: Understanding of the quality of evidence (H3a) and knowledge on the quality of evidence (H3b) are higher when an explanation of the methodological approach “meta-analysis” (quality of evidence statement) is given compared to a condition in which no such explanation is given (no statement).
Third, exactly how psychological studies investigate their research questions is often not self-evident – at least to laypersons – thereby establishing a certain need to “translate psychological science” (see Kaslow, 2015). For example, how is arithmetic competence in very young infants that cannot even speak studied? How are anxiety or resilience measured? One might argue that providing laypersons with (exemplary) information on how the individual studies included in a meta-analysis (i.e., the synthesized studies) operationalized their research questions will substantially improve their understanding of what was actually analyzed in the meta-analysis and their ability to draw conclusions based on its findings. In line with this reasoning, Hoogeveen et al. (2020, p. 269) showed that laypeople were able to rate the likelihood of successful replications when “a short description of the research question, its operationalization, and the key finding” were provided in plain language. However, one might also argue that presenting this additional information may increase task-related cognitive load (e.g., Sweller et al., 2011) and therefore impede laypersons’ user experience and knowledge acquisition regarding the findings of the meta-analysis. To shed some light onto how providing information on the operationalization of the research question in the synthesized studies affects the perception of PLS (i.e., user experience) as well as the knowledge acquired through them, we examined effects of providing laypersons with this information. More specifically, we varied whether or not a subsection on the operationalization of the research question in the individual studies was included in the PLS (information on the operationalization provided vs. not provided).
Hypothesis 4: Operationalization: subsection vs. no subsection. Providing information on how the research question was operationalized in the synthesized studies affects the following outcome variables: Accessibility (H4a), understanding (H4b), content-related knowledge (H4c), and empowerment (H4d).
Hypotheses 1–4 were tested in a first empirical study (Study 1). Hypotheses 5–8 were tested in a second empirical study (Study 2) and partly built upon results of Study 1. For example, participants repeatedly asked for more information about what “effect size” means, how effect sizes are computed and how to interpret their values after being provided with a qualitative statement and an effect size estimate in Study 1. Against this background, the fourth aspect addressed by our research, albeit not completely psychology-specific, becomes especially relevant for psychological meta-analyses. Laypeople are most likely not familiar with the statistical concepts and especially effect size measures employed in scientific publications. In spite of the fact that first studies on communicating statistical evidence to non-scientists via PLS exist – especially for Cochrane PLS (e.g., Glenton et al., 2010; Santesso et al., 2015; Buljan et al., 2020) – broad consensus on this issue is still lacking in relevant guidelines. For example, Cochrane (Cochrane Methods, 2013; Cochrane Norway, 2016, 2019) states that statistical terms need to be explained if included. For this purpose, Cochrane offers specific guidelines on how to report descriptive statistics as well as standardized statements for reporting effect sizes. Other guidelines recommend that researchers remove all statistics (e.g., American Psychological Association, 2018) or do not provide any guidance on this issue (see Stoll et al., 2021 for a comprehensive review). This illustrates the need for further research in this area. We compared four approaches for explaining statistical terms and effect sizes in psychological meta-analyses: (1) the effect size is not reported – instead, a qualitative statement on the interpretation of its size is provided, e.g., “The relationship was medium-sized.” (qualitative statement); (2) the effect size is reported and a qualitative statement on the interpretation of its size is provided, e.g., “The correlation was r=0.40. This is a medium-sized relationship.” (effect size+qualitative statement); (3) no qualitative statement is provided, only the effect size is reported in the text and an explanation of the effect size, its boundaries and cut-off values at assist interpretation are provided below the PLS in a glossary, e.g., “What is r and what value of r implies a large effect?” (effect size+glossary); (4) the effect size, a qualitative statement and a glossary are provided (effect size+glossary+qualitative statement). In hypotheses 5 and 6, based on user feedback of Study 1, we propose that providing qualitative statements in the text of a PLS and a glossary on statistical terms promotes user experience and knowledge acquisition:
Hypothesis 51: Statistical terms: incremental effect glossary: Accessibility (H5a), understanding (H5b), content-related knowledge (H5c), and empowerment (H5d) are higher when an effect size is reported and a glossary that explains statistical terms is provided after the PLS and a qualitative statement on the interpretation of the effect size is provided within the text of the PLS (effect size+glossary+qualitative statement) compared to reporting the effect size and providing a qualitative statement without a glossary (effect size+qualitative statement).
Hypothesis 61: Statistical terms: incremental effect qualitative statement: Accessibility (H6a), understanding (H6b), content-related knowledge (H6c), and empowerment (H6d) are higher when an effect size is reported and a glossary explaining statistical terms is provided after the PLS and a qualitative statement on the interpretation of the effect size is provided within the text of the PLS (effect size+glossary+qualitative statement) compared to reporting the effect size and providing a glossary without a qualitative statement (effect size+glossary).
The fifth aspect, we investigated is not psychology-specific but applies to PLS in general. Cochrane guidelines (Cochrane Methods, 2013; Cochrane Norway, 2016, 2019) and existing evidence on research summary characteristics clearly point toward subheadings as an important formal element that should be included in research summaries and PLS (e.g., Kerwer et al., 2021 for PLS; but see also Hartley, 2004, 2014; Hartley and Betts, 2007 for structured abstracts in general). Additionally, first evidence suggests that further structuring the text of PLS (e.g., by means of bullet points) improves the user experience for information recipients (e.g., Ellen et al., 2014; Raynor et al., 2018; Anzinger et al., 2020). To test if these findings can be transferred to PLS of psychological meta-analyses (and can also be replicated on a conceptual level in a considerably larger and more representative sample in a randomized controlled experimental design), we compared structured text with formatted text blocks (e.g., by bullet points) to unstructured text without further formatting of text blocks within subsections. More specifically, we made the following assumptions in hypothesis 7:
Hypothesis 71: Structuring: structured vs. unstructured: Accessibility (H7a), understanding (H7b), content-related knowledge (H7c), and empowerment (H7d) are higher when the text is structured compared to a condition, where no structuring is provided (unstructured).
The rationale for hypothesis 8 was derived from our experience testing hypotheses 1 and 2 in Study 1. We found that our participants faced problems with regard to knowledge acquisition and user experience when confronted with PLS that reported complex meta-analytical designs (i.e., results of a meta-analytic structural equation model). One might argue that meta-analytic structural equation models belong to the most complex meta-analytic techniques in the current literature. Still, we had to concede that the statement explaining the methodological approach of meta-analyses (i.e., the quality of evidence statement; see hypothesis 3 and Supplementary Material 1) solely focused on an overly simple type of “univariate” meta-analytical design (i.e., a design testing whether one effect is different from zero in the literature). This type of statement might not promote laypersons’ understanding of more complex meta-analytic designs. This is especially important because an integral feature of (even simple) meta-analytic designs is that they can model heterogeneity in effect sizes, and, as a consequence, estimate moderator effects (e.g., Sánchez-Meca and Marín-Martínez, 2010). Simple meta-analytical designs including moderator effects are arguably still not very complex compared to structural equation modeling approaches (Steinmetz et al., 2020). They are, however, significantly more complex than the design presented in our statement to explain the meta-analytic approach in hypothesis 3. Drawing on this notion, we chose this “slightly more complex” meta-analytical design (i.e., moderator analyses) as a starting point for investigating how to support PLS readers in their understanding of more complex meta-analytic design types. For this purpose, we extended the quality of evidence statement, we used for examining hypothesis 3 to outline moderator analyses. The following hypothesis was specified for comparing this extended quality of evidence statement compared to the regular, “unextended” quality of evidence statement that was used to test hypothesis 3:2
Hypothesis 81: Quality of evidence communication: regular statement vs. extended statement: Explaining complex meta-analytic designs affects the following outcome variables: Accessibility (H8a), understanding (H8b), content-related knowledge (H8c), and empowerment (H8d).
Hypotheses 1–4 and hypotheses 5–8 were preregistered at PsychArchives. Hypotheses 5–8 were preregistered after results regarding hypotheses 1–4 were available but before data collection for Study 2 began.
Study 1
In our first study, we investigated the following PLS characteristics: approaches for explaining technical terms, approaches for communicating the quality of evidence, and approaches for communicating how the research question was operationalized in the synthesized studies. Study procedures, as well as the original German items and full texts of the PLS, were preregistered at PsychArchives.3 English translations of items are reported in the manuscript and exemplary English translations of PLS, the quality of evidence statement as well as knowledge items are provided in Supplementary Material 1.
Materials and Methods
Sample
We recruited a large general population sample (NTarget=2,004, see Sample Size Calculation and Power Analysis) via the panel provider Respondi. More specifically, Respondi was tasked with the recruitment and monetary compensation of study participants. The following specific quota conditions were specified: Age (18–44, 45, or older), gender (men, women), and secondary school education level (lower track: “Hauptschulabschluss,” middle track: “Mittlere Reife,” and higher track: “Hochschulreife”). As there were 2×2×3=12 overall combinations of those quota conditions (e.g., male participants with “Mittlere Reife” and younger than 45years), the target sample size for each of these combinations was 2,004/12=167. Additionally, the following inclusion criteria applied: Participants (1) had to possess German language skills at native speaker level, (2) had to have successfully graduated from secondary school, and (3) were not currently studying psychology or holding a degree in psychology.
Because all participants were allowed to complete started sessions, the final sample size was slightly larger than planned: N=2,038 participants completed the questionnaire and N=2,288 responded to at least one outcome variable (two PLS were presented to each participant, and some participants dropped out after answering outcome variables on the first PLS). The number of complete observations for each combination of quota conditions ranged from 165 to 174. On average, participants were 46.31years old (Mdn=45, minimum=18, maximum=90, SD=14.94), and our sample contained slightly more women (50.48%) than men. Moreover, the proportion of participants with lower educational status was slightly higher compared to the other educational status groups (31.73% Hochschulreife, 32.74% Mittlere Reife, and 35.53% Hauptschulabschluss). Our specific aim guiding sample selection was to investigate PLS performance of a heterogeneous readership. The multivariate distribution of demographic variables, however, is not intended to be representative of the German population.
Design
There were two study arms. Study arm A was designed for testing the effects of different approaches for explaining technical terms of psychological core concepts (hypotheses 1 and 2). In study arm B, effects of providing information on the operationalization of the research question in the synthesized studies were tested (hypothesis 4). Hypothesis 3, on the effect of (not) providing a statement on the quality of evidence (i.e., on the methodological approach of meta-analyses) was tested in both study arms. Participants either received or did not receive a statement on the methodological approach underlying meta-analyses (i.e., quality of evidence statement).
In study arm A, participants were randomly assigned to one of the three approaches for explaining technical terms (replacement, glossary, and no explanation). Within each condition, all participants read two PLS, each summarizing a different meta-analysis from resilience research (based on meta-analyses by Rasmussen et al., 2019, PLS_RR1, and Groth et al., 2019, PLS_RR2). The same approach for explaining technical terms was applied across both PLS read by each participant. No information on operationalization was provided for these PLS.
In study arm B, participants were randomly assigned to one of the two conditions for providing information on the operationalization of the research question in the synthesized studies (information provided, information not provided). Within each condition, all participants read two PLS, each summarizing a different meta-analyses from research on infant development (based on meta-analyses by Christodoulou et al., 2017, PLS_ID1, and Dunst et al., 2012, PLS_ID2). In both PLS presented to test hypothesis 4, technical terms were consistently replaced by non-technical terms.
Table 1 illustrates the design and conditions of Study 1. As can be seen in Table 1, Study 1 had a between-subjects design with three factors and – because two of these factors were varied separately in different study arms (see above) – there were 10 (3×2+2×2) conditions overall.
Procedure
The study was conducted online using the survey software Unipark. The online questionnaire was created and hosted by the authors. After being invited by Respondi, participants completed an informed consent form. Thereafter, inclusion criteria were checked and participants were assigned to one of the 10 experimental conditions of our study. Two PLS were presented to each participant. The design and content of these PLS were dependent on the assigned experimental condition. Participants read each of these two PLS for at least 3min and answered the outcome measures on (perceived) accessibility, (perceived) understanding, understanding of the quality of evidence, and (perceived) empowerment on the same webpage. After reading the PLS, knowledge items were presented on the following page. Finally, participants completed a short user survey and were redirected to Respondi for monetary compensation. All study procedures were approved by the ethics committee of Trier University. Participants spent a mean duration of approximately 18min on completing the questionnaire.
Variables
Information on the following outcomes was assessed on the same page as the corresponding PLS for confirmatory analyses: (perceived) accessibility (“The language in this text is easy to read.”), (perceived) understanding (“The information in this text is understandable.”), and (perceived) empowerment (“After reading this text, I can participate in a discussion about the topic.”). We measured responses to these items on Likert scales ranging from 1 (fully disagree) to 8 (fully agree).
Understanding of the quality of evidence was measured via a simple decision task as the preference of meta-analytic evidence over the evidence of an individual study. More specifically, after participants read the PLS, we stated that results of a fictitious new study contradicted the result of the meta-analysis the participants had just read (“Imagine you are talking to a friend about the topic of the review. Your friend knows of a slightly more recent study that contradicts the result of the review.”). Thereafter, we asked them to decide which study result they perceived as more trustworthy (“Which result would you trust?” 1=“The result of the slightly more recent study,” 8=“The result of the review”). Therefore, higher values on this semantic differential indicated that participants correctly understood the higher level of quality of evidence represented in a meta-analysis.
PLS-specific knowledge items were administered on a separate page after each PLS: Content-related knowledge on the key message of the PLS, and PLS-specific knowledge of the quality of evidence referring to what the researchers did (i.e., that the results of X studies were summarized in the review) were assessed via one forced-choice format item with four options. After PLS-specific knowledge items on the second PLS were presented, knowledge of the quality of evidence in general (i.e., what a meta-analysis is) was assessed via one forced-choice format item with six options. Responses on all knowledge items were recoded to correct (“1”) or incorrect (“0”) for statistical analyses.
A full list of exploratory outcomes and further potential covariates that were collected is included in the preregistration of Study 1 materials. In this article, we present exploratory analyses regarding the covariate interest in psychological research (“I am interested in psychological research.”). Agreement to this statement was assessed on an eight-point Likert scale.
Statistical Analysis
Sample Size Calculation and Power Analysis
We conducted a power analysis using the software GPower (Faul et al., 2009). As a statistical test, we selected F-tests on between-level factors in repeated measures ANOVAs. The following parameters were specified: Small (f=0.10) effect size, alpha=0.05, power=0.90 with three groups, two measurements and an expected correlation between measures of 0.50. This power analysis indicated that a sample size of 954 participants was required. Thus, we decided to recruit at least 1,000 participants for each study arm. Because additional quota restrictions applied, our total target sample size was 2,004 participants.
Statistical Model
We analyzed our data in R (R Core Team, 2021) by means of mixed models (to draw on their flexibility compared to simple repeated measures ANOVAs) using the lme4 package (Bates et al., 2015). Using mixed models allowed us, for example, to include incomplete data of participants that rated only one PLS (and not both). For inferential statistics and group comparisons, the packages lmertest (Kuznetsova et al., 2017) and multcomp (Hothorn et al., 2008) were employed.4 As indicated above, hypotheses 1 and 2 were tested in the subsample of participants in study arm A and hypothesis 4 in the subsample of participants in study arm B. Hypothesis 3 was tested based on the whole sample. Our hypotheses were tested based on the significance of regression coefficients of dummy-coded variables. In study arm A, the reference category for dummy variables was PLS_RR1 with no explanation on technical terms and no statement on the quality of evidence (i.e., condition 5 in Table 1). In study arm B, the reference category was PLS_ID2 with no information on operationalization in synthesized studies (not provided) and no statement on the quality of evidence (i.e., condition 9 in Table 1). One-sided hypothesis tests were conducted when appropriate, and the significance of effects was tested at p<0.05. To obtain effect size estimates for individual fixed effects, we computed partial R-squared statistics as a measure of incrementally explained variance using the r2glmm package (Jaeger et al., 2017). Additionally, marginal and conditional R-squared statistics for mixed models were calculated (Nakagawa et al., 2013; as implemented in the R package MuMIN; Barton, 2020). Finally, data were discarded if participants failed to respond to any confirmatory outcome (i.e., dropped out prior to providing a response, 599 participants) or if participants completed the survey more than once (in this case, only data from the first completion were analyzed, 13 participants). We provide information on the final analysis-specific number of participants and observations (i.e., rated PLS) in the relevant tables on confirmatory analyses.
Results
Descriptive statistics on confirmatory outcomes from Study 1 are provided in Table 2. Accessibility, understanding, and empowerment were highly correlated (all rs>0.56), which is why we will report results of the corresponding confirmatory analyses in an aggregated manner within the text. Mixed model results on Study 1, separated by outcome variable, are provided in Tables 3 and 4.
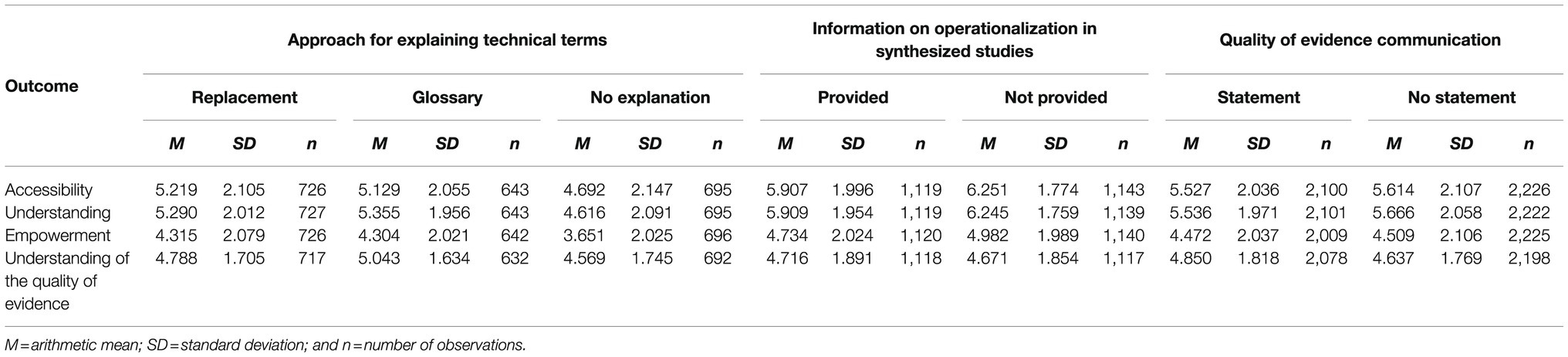
Table 2. Study 1 descriptive statistics (means and SDs) of confirmatory outcomes separated by design condition.
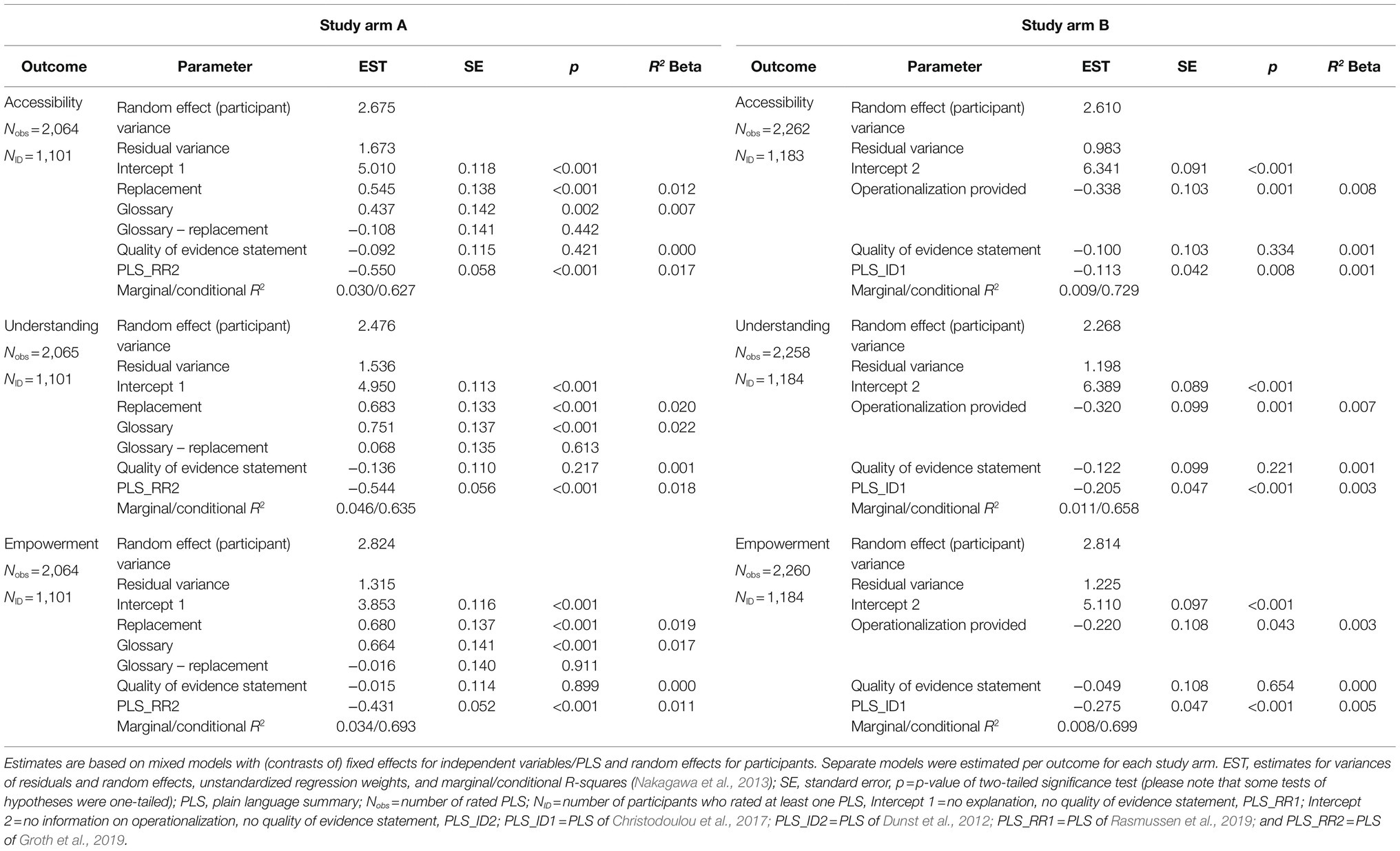
Table 3. Study 1 results of confirmatory analyses for user experience outcomes: accessibility, understanding, and empowerment.
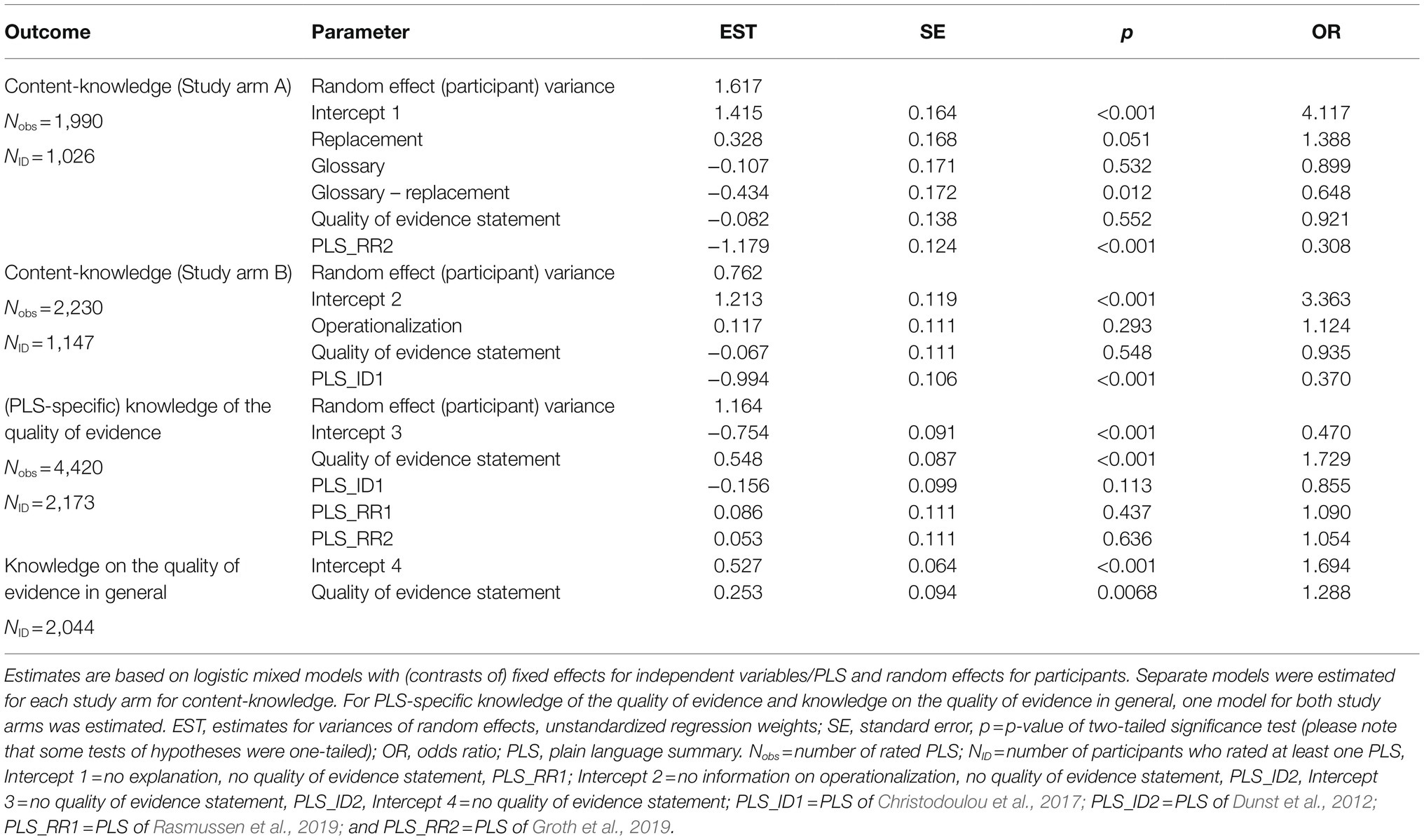
Table 4. Study 1 results of confirmatory analyses for knowledge acquisition, PLS-specific knowledge of the quality of evidence and knowledge on the quality of evidence in general.
H1 and H2 Approach for Explaining Technical Terms
Overall, accessibility, understanding, and empowerment ratings as well as knowledge acquisition were lower for PLS_RR2 compared to PLS_RR1 (see inferential statistics in Table 3).
Accessibility, Understanding, and Empowerment
Mean values for accessibility and understanding indicated that participants tended to agree to the corresponding statements (mean values higher than 4.50). More specifically, mean values on accessibility and understanding ranged from 4.62 to 5.36 (on a 1–8 scale) across experimental conditions. Descriptively, mean values for empowerment were, however, lower and ranged from 3.65 to 4.32 (see Table 2). Perceived accessibility, understanding, and empowerment of the PLS were significantly higher in the replacement or glossary condition compared to the no explanation condition (see Table 3; Figures 1A–C). Thus, H1a, H1b, H1d, and H2a, H2b and H2d were fully confirmed. All differences between the replacement and glossary conditions were non-significant on user experience outcome measures (see Table 3).
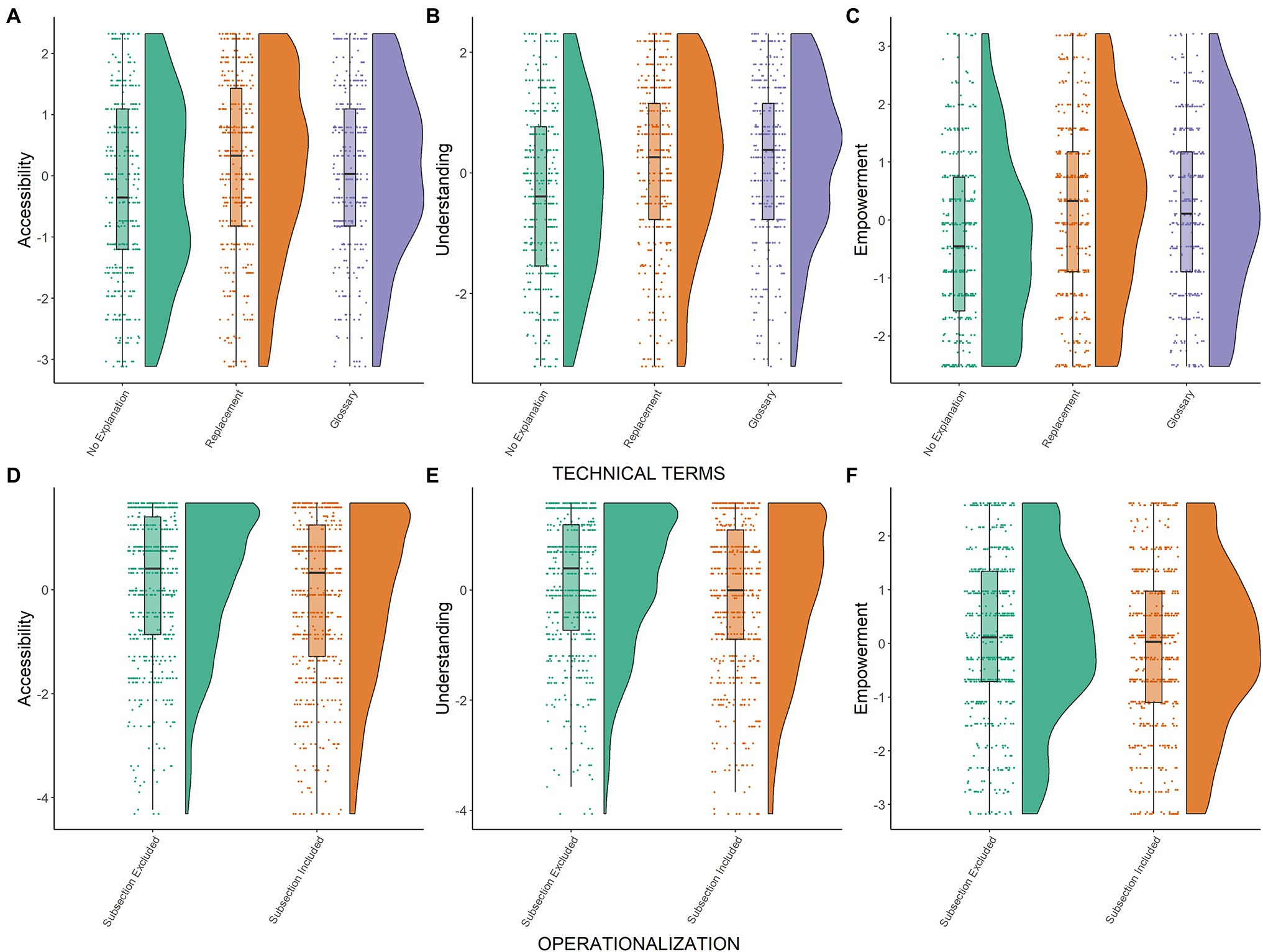
Figure 1. Results study 1. Raincloud plots for user experience outcomes: accessibility (A,D), understanding (B,E), and empowerment (C,F). Residual scores are depicted separated by experimental conditions: Technical terms (A–C), operationalization (D,E). Residual scores were obtained from a mixed model that controlled for participant as random factor and for presented plain language summaries (PLS)/quality of evidence statement as fixed factors.
Content-Related Knowledge
Descriptively, the proportion of correct answers was higher in the replacement condition (68.84%), compared to the no explanation condition (63.60%) or the glossary condition (62.03%). Inferential analyses revealed that the likelihood of answering content-related knowledge items correctly was significantly higher in the replacement condition compared to the other conditions (i.e., no explanation and glossary), whereas the difference between providing no explanation and providing a glossary was non-significant (see Table 4). Consequently, H2c was confirmed and H1c was rejected.
H3 Quality of Evidence Statement
Understanding of the Quality of Evidence, User Experience, and Content-Related Knowledge
Overall, mean values for preferring meta-analytic evidence over evidence from single studies in our decision task (indicating a better understanding of the quality of evidence) were lower in the no statement on the quality of evidence condition (M=4.64) compared to the statement condition (M=4.85, on a 1–8 semantic differential, with 1 indicating preference of the single study and 8 indicating preference of meta-analytic evidence). This difference was statistically significant. The preference of meta-analytical evidence significantly increased when a statement on the quality of evidence explaining the methodological approach underlying meta-analyses had been presented (b=0.22, SE=0.07, p<0.001, see also Figure 2). Consequently, H3a is confirmed. There were no significant effects of the quality of evidence statement on user experience outcome measures or content-related knowledge (all ps>0.068, see Supplementary Material 2).
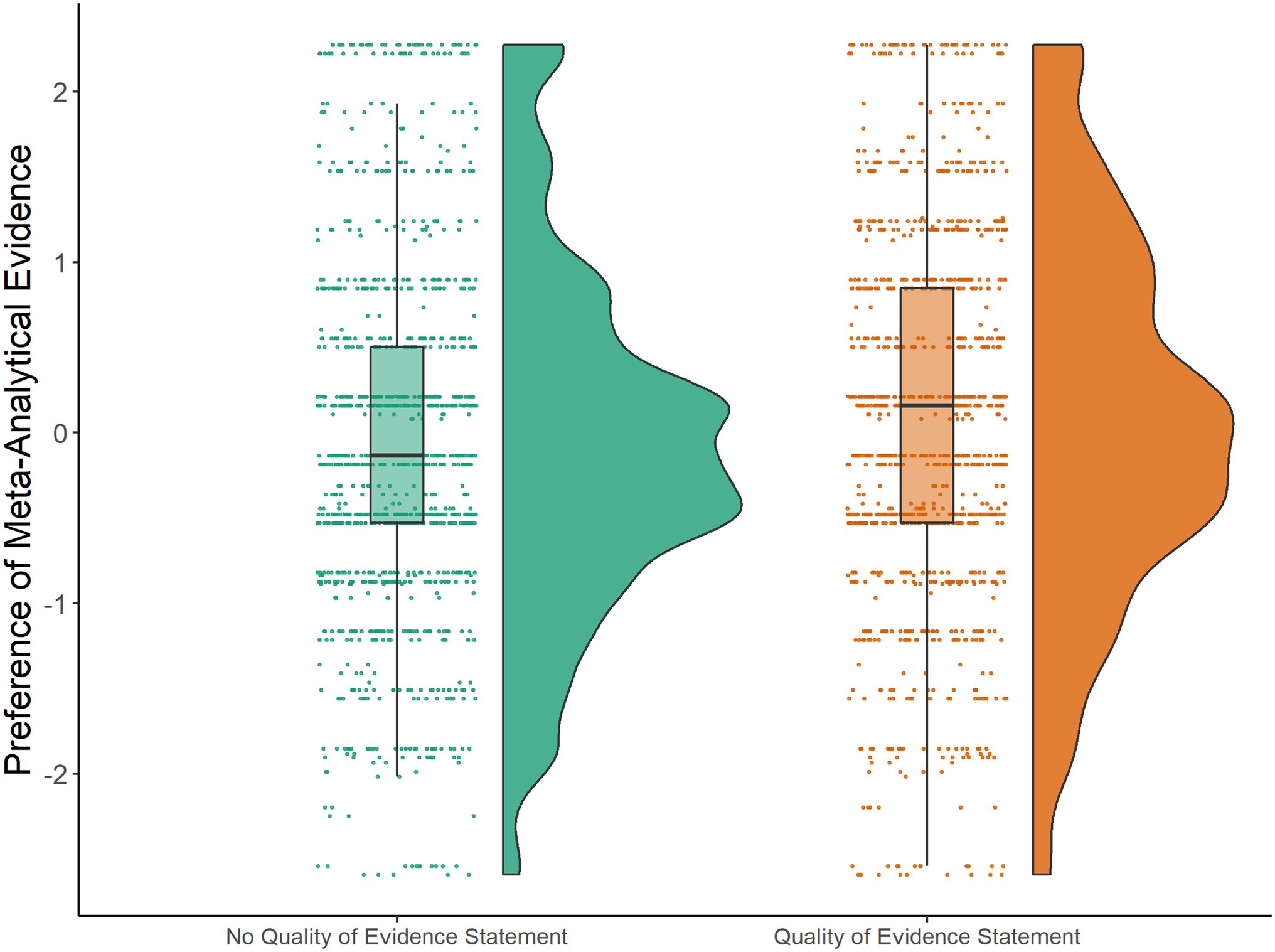
Figure 2. Results study 1. Raincloud plots for understanding of the quality of evidence as a preference of meta-analytic evidence in a decision task. Residual scores are depicted separated by experimental conditions: statement on the quality of evidence presented vs. no statement on the quality of evidence presented. Residual scores were obtained from a mixed model that controlled for participant/presented PLS as random factors.
Knowledge on the Quality of Evidence in General and PLS-Specific
For PLS-specific knowledge of the quality of evidence (i.e., what the researchers did) and knowledge on the quality of evidence in general (i.e., what a meta-analysis is), we found significant effects for our statement on the methodological approach underlying meta-analyses (see Table 4). Participants were more likely to correctly respond to the PLS-specific quality of evidence knowledge items (45.86 vs. 35.50%) and were more likely to provide the correct answer to a general knowledge item on the methodological approach of meta-analyses (68.57 vs. 62.88%, see Table 4). Thus, H3b was fully confirmed.
H4 Information on Operationalization in Synthesized Studies
Overall, perceived accessibility, understanding, and empowerment ratings as well as knowledge acquisition were lower for PLS_ID1 compared to PLS_ID2 (see Table 3).
Accessibility, Understanding, and Empowerment
Mean values for accessibility, understanding, and empowerment were lower when information on the operationalization of the research question was provided in the PLS compared to when it was not provided (see Table 2). Inferential analyses indicate that the corresponding differences were significant (see Table 3; and Figures 1D–F), thus confirming H4a, H4b, and H4d.
Content-Related Knowledge
Descriptively, the proportion of correct answers was slightly higher when information on the operationalization was provided (65.79%), compared to the condition providing no such information (63.64%). The difference between both conditions was, however, far from statistical significance (see Table 4). H4c is therefore rejected.
Exploratory Analysis: Interest in Psychological Research
In exploratory analyses, we found that interest in psychological research generally seemed to compensate – at least to some extent – for the negative effects of providing additional information on the operationalization of the research question reported above. More specifically, a positive linear effect of interest in psychological research existed for all outcomes that were related to user experience (Accessibility: b=0.51, SE=0.05, p<0.001, R2=0.073, Understanding: b=0.55, SE=0.05, p<0.001, R2=0.086, and Empowerment: b=0.71, SE=0.05, p<0.001, R2=0.125). In a separate model, we also tested whether effects of providing additional information on the operationalization differed depending on interest in psychological research. Our data appear to tentatively suggest that interest in psychological research might moderate the effects of providing information on the operationalization. However, the corresponding interaction was not consistently significant (Accessibility: b=0.27, SE=0.10, p=0.006, R2=0.005, Understanding: b=0.18, SE=0.09, p=0.054, R2=0.003, and Empowerment: b=0.18, SE=0.10, p=0.074, R2=0.002). Linear effects of interest in psychological research were also found for PLS of resilience research. Detailed results of all analyses on interest in psychological research are provided in Supplementary Material 2.
Interim Discussion
Our results on approaches for explaining technical terms (hypotheses 1 and 2) suggest that both approaches tested for handling technical terms – replacing technical terms in the text and explaining technical terms in a glossary – seem to work satisfactorily with respect to user experience (perceived accessibility, understanding, and empowerment). However, replacing technical terms instead of retaining them in the text or explaining them via a glossary appears to have some beneficial effects on knowledge acquisition. Some technical issues notwithstanding (see General Discussion on technical limitations of implementing glossaries), we decided to pursue the replacement approach in Study 2.
Our analyses on hypothesis 3 show that our quality of evidence statement (i.e., the explanation of the methodological approach underlying meta-analyses) worked as intended: Providing this explanation improved our subjects’ understanding and knowledge on the quality of evidence. Further analyses suggest that the statement had no “side effects” (i.e., no statistically significant negative effects on any content-related knowledge or user experience measures); thus, we propose providing this kind of statement alongside PLS of psychological meta-analyses to improve laypersons’ understanding of the quality of evidence and assist them in their decision making.
In our tests of hypothesis 4, we found no evidence in favor of including information on the operationalization of the synthesized studies. Including this subsection seemed to have negative effects on user experience and did not affect knowledge acquisition. On the one hand, the latter finding is encouraging because it illustrates that knowledge on the key message of the PLS did not suffer when providing additional textual information on the operationalization of the research question. On the other hand, indisputable user experience losses were found, which is why we opted to not include this information in Study 2. However, it should be noted that negative effects of adding this information might be less pronounced for participants that are highly interested in psychological research (see also General Discussion on cognitive load theory and writing PLS).
We also found that, in each study arm, the two PLS presented to participants differed considerably in user experience and knowledge acquisition. We argue that this finding points toward the complexity of PLS as an important PLS feature. We suggest that PLS might differ in their complexity for various reasons. They might display complexity because the design of the summarized meta-analyses is complex, and they strive to communicate this in detail. This might have been the case, for example, with PLS_RR2 of study of Groth et al. (2019), which reported mediator effects in meta-analytic structural equation models. PLS may also be more complex because they strive to explain the complex theoretical background of a meta-analysis. An example for this might be PLS_ID1 of study of Christodoulou et al. (2017). This study searched the literature for evidence on an effect initially found by another author. By describing this background, our PLS might have introduced a “replicability” meta-level. In contrast, comparably simple meta-analytic designs might focus on one specific effect size (e.g., one treatment effect or correlation). We further suggest that the complexity of PLS also increases if meta-analyses report a large number of effect sizes (e.g., various simple correlation coefficients) and the PLS aims to convey this information to the reader. Against this background, negative effects of providing additional information on the operationalization may also be interpretable as a result of the increased PLS complexity due to the inclusion of additional content. We further pursued this issue of PLS complexity in Study 2.
Finally, results of our exploratory analyses illustrated that interest has to be taken into consideration as an important covariate when analyzing laypeople’s perception of PLS. It is necessary to keep in mind that the target audience ultimately reading PLS will likely be more interested in psychological research than the general public. For this reason, we opted to only include participants in Study 2 who reported a certain degree of interest in psychological research. This was done to test our PLS in a sample more similar to the target audience of PLS.
Study 2
In our second study, we investigated the following PLS characteristics: approaches for explaining statistical terms, approaches for communicating complex meta-analytic designs via an extended quality of evidence statement, and approaches for structuring PLS. Study procedures, as well as the original German items and full texts of the PLS, were preregistered at PsychArchives.5 English translations of items are reported in the manuscript and exemplary English translations of PLS, the extended quality of evidence statement as well as knowledge items are provided in Supplementary Material 1.
Materials and Methods
Sample
As in Study 1, we recruited a general population sample (NTarget=2,004) via Respondi. Quota conditions were the same as in Study 1, and all inclusion criteria of Study 1 also applied to Study 2. Additionally, to obtain a sample that was more similar to the natural target audience of PLS, participants had to indicate that they were interested in psychological research (Item: “I am interested in psychological research.”). The inclusion criterion was an agreement of “4” or higher on an eight-point Likert scale. The choice of this specific cut-off value was based upon the lowest medium value observed for this item across all quota condition combinations of Study 1.
Once again, the final sample size was slightly larger than planned: N=2,078 participants completed the questionnaire and N=2,211 participants responded to at least one confirmatory outcome variable. Despite best efforts, the target sample size was not completely achieved for one quota condition combination, namely men who were younger than 45years of age with a Hauptschulabschluss. However, this group still reached about 90% (150 out of 167) of its intended size. The number of complete observations for each quota condition combination ranged from 150 to 194. Our participants were, on average, 46.10years old (Mdn=45, minimum=18, maximum=83, SD=15.14) and our sample contained slightly more women (50.47%) than men. Education status was evenly distributed (33.92% Hochschulreife, 32.56% Mittlere Reife, and 33.51% Hauptschulabschluss).
Design
The results of Study 1 pointed toward the complexity of PLS as an important design factor. In our second study, we therefore strived to examine to what extent can effects be generalized to more or less complex PLS. For this purpose, Study 2 also had two study arms. In study arm A, two PLS with comparably low complexity were presented: One PLS reported only one correlation coefficient as an effect size (based on a meta-analysis by Schwalm et al., 2021, PLS_LC1), and one PLS reported only one mean difference as an effect size (based on a meta-analysis by Bucher et al., 2020; in the ZPID’s PsychOpen CAMA system, see Burgard et al., 2021, PLS_LC2). Study arm B included two entirely different PLS with comparably high complexity: One PLS reported meta-moderator analyses (based on a meta-analysis by Bergmann and Cristia, 2016, PLS_HC1), and one PLS reported nine correlation coefficients (based on a meta-analysis by Yule et al., 2019, PLS_HC2).
As can be seen in Table 5, Study 2 had a between-subjects design with three fully crossed factors: Communication of complex meta-analytic designs by an extended quality of evidence statement, approach for explaining statistical terms and formal text structuring. Each participant was randomly assigned to one study arm and read its two corresponding PLS. Both PLS were randomly assigned to one condition (i.e., combination of the different levels of the three independent variables depicted in Table 5): one of the four approaches for explaining statistical terms, one of the two approaches for communicating complex meta-analytic designs and one of the two approaches for formal text structuring. The assigned condition was the same for both PLS. As a result, there were 16 (4×2×2) conditions in total, identical within each study arm (see Table 5).
It is important to note that the complexity of PLS was not varied within each PLS (i.e., the same PLS was not presented with different “degrees of complexity”). Rather, two entirely different PLS were presented. This approach differs from the procedure for the independent variables statistical terms and structuring, which were varied for each PLS. Consequently, investigating more and less complex PLS constitutes a test of the generalizability of the effects of the other independent variables, which is why all hypotheses were tested in both study arms separately.
Procedure
Study procedures were the same as in Study 1. The study was approved by the ethics committee of Trier University. Participants spent a mean duration of approximately 24min on completing the questionnaire.
Variables
The same confirmatory outcome variables as in Study 1 were assessed. Because intercorrelations of our outcome measures were very high in Study 1, we made some minor changes to the wording of our confirmatory outcome variables (i.e., we no longer referred to the PLS as “text” but as “summary” and slightly revised our perceived understanding item to stress that this statement aimed at a subjective perception instead of a text characteristic; “I understood the information” instead of “The information is understandable”). Otherwise, the wording and Likert scales of confirmatory outcome variables remained unchanged. Exploratory outcomes and potential covariates assessed for this study are listed in the preregistration of Study 2 materials.
Statistical Analysis
Sample Size Calculation and Power Analysis
The following parameters were specified in our power analysis for Study 2: Small (f=0.10) effect size, alpha=0.05, power=0.875, with four groups (i.e., the maximum number of independent variable conditions realized with regard to the approach for explaining statistical terms), two measures and an expected correlation between measures of 0.50. This power analysis indicated that a sample size of 992 participants was required. Thus, we decided to recruit at least 1,000 participants to test hypotheses 5 and 6. As all hypotheses are tested twice in our study – once for more complex and once for less complex PLS – and additional quota restrictions applied, our target sample size was 2,004 participants.
Statistical Model
Study 2 employed the same statistical model and statistical procedures as Study 1. Hypotheses 5, 6, 7, and 8 were tested on the subsample of participants in study arms A and B separately. In study arm A, the reference category for dummy variables was PLS_LC1 with the regular statement for quality of evidence communication, unstructured as an approach for structuring and effect size+statement+glossary as an approach for explaining statistical terms (i.e., condition 14 in Table 5). In study arm B, the reference category for dummy variables was PLS_HC1 with the regular statement for quality of evidence communication, unstructured as an approach for structuring and effect size+statement+glossary as an approach for explaining statistical terms (i.e., condition 30 in Table 5). Again, data were discarded if participants failed to provide information on any confirmatory outcomes (i.e., dropped out prior to providing a response, 399 participants) or if participants completed the survey more than once (five participants). Analysis-specific sample sizes are reported in the relevant tables on confirmatory analyses.
Results
Descriptive statistics on confirmatory outcomes from Study 2 are provided in Table 6. Accessibility, understanding, and empowerment were again highly correlated (all rs>0.52). Consequently, we will again report the results of the corresponding confirmatory analyses in an aggregated manner. Mixed model results on Study 2 separated by outcome variable are provided in Tables 7 and 8.
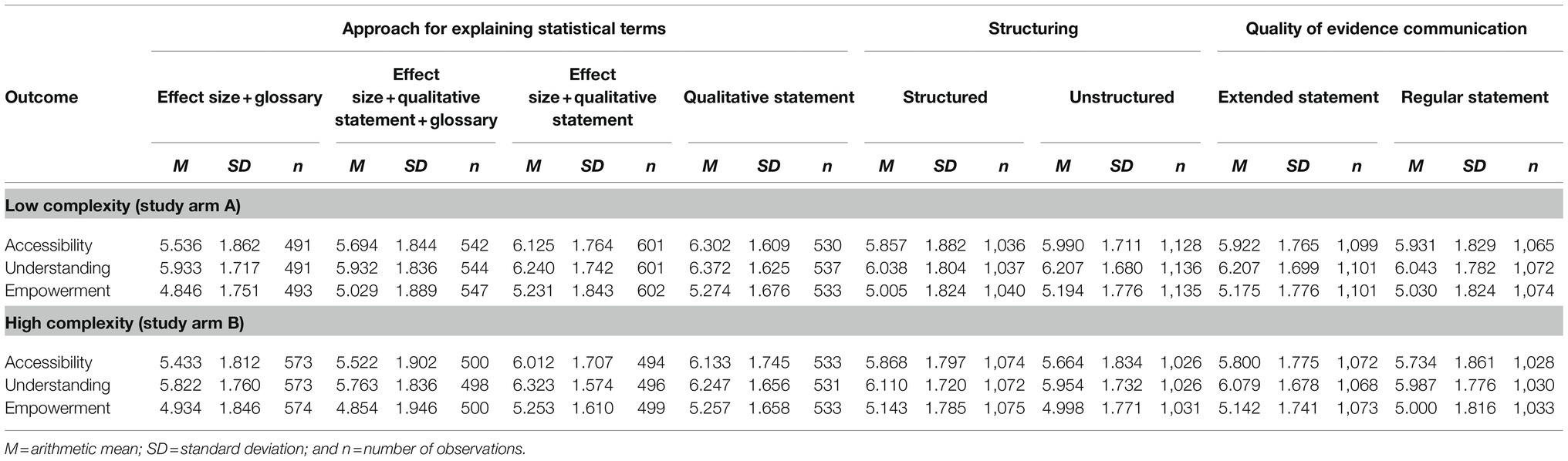
Table 6. Study 2 descriptive statistics (means and SDs) of confirmatory outcomes separated by design condition.
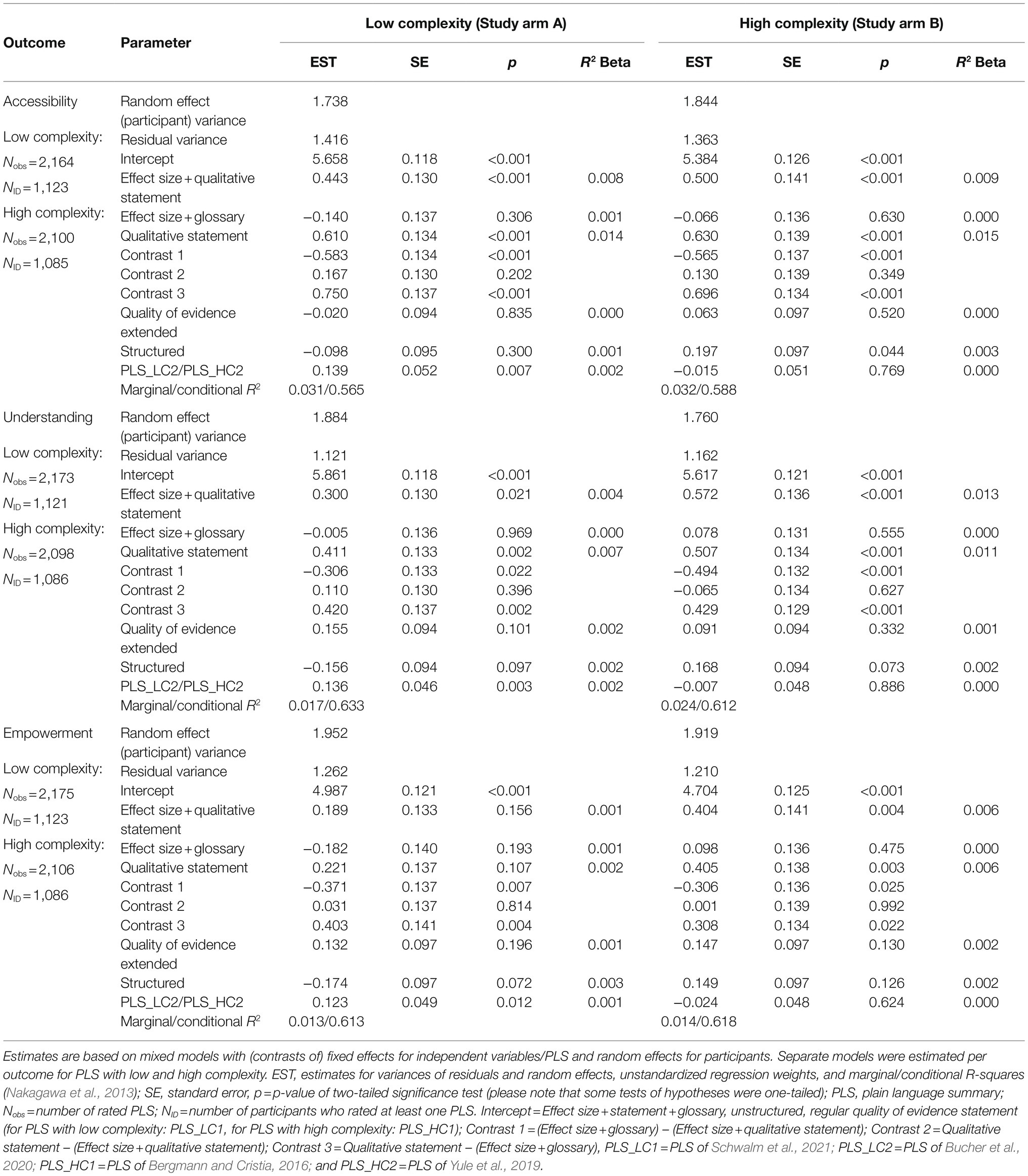
Table 7. Study 2 results of confirmatory analyses for user experience outcomes: accessibility, understanding, and empowerment.
H5 and H6 Approach for Explaining Statistical Terms
Accessibility, Understanding, and Empowerment
Descriptively, mean values for user experience outcomes (accessibility, understanding, and empowerment) indicated that participants tended to agree to the corresponding statements (mean values higher than 4.50, see Table 6). In both conditions including a glossary of statistical terms (i.e., effect size+glossary+qualitative statement, effect size+glossary), user experience was lower compared to conditions providing no glossary (effect size+qualitative statement, qualitative statement; see Table 6; Figures 3A–C). Inferential analyses revealed that almost all differences were statistically significant (see Table 7). The only exception was that differences for empowerment in PLS with low complexity were non-significant for comparisons involving the effect size+glossary+qualitative statement condition (both p>0.107, see Table 7). Based on these results, H5a, H5b, H5d, and H6a, H6b and H6d are rejected. Combining a qualitative statement and a glossary in the effect size+glossary+qualitative statement condition clearly had no beneficial effects. Finally, throughout all analyses, we observed no significant differences between both conditions that provided no glossary (effect size+qualitative statement vs. qualitative statement, see Table 7).
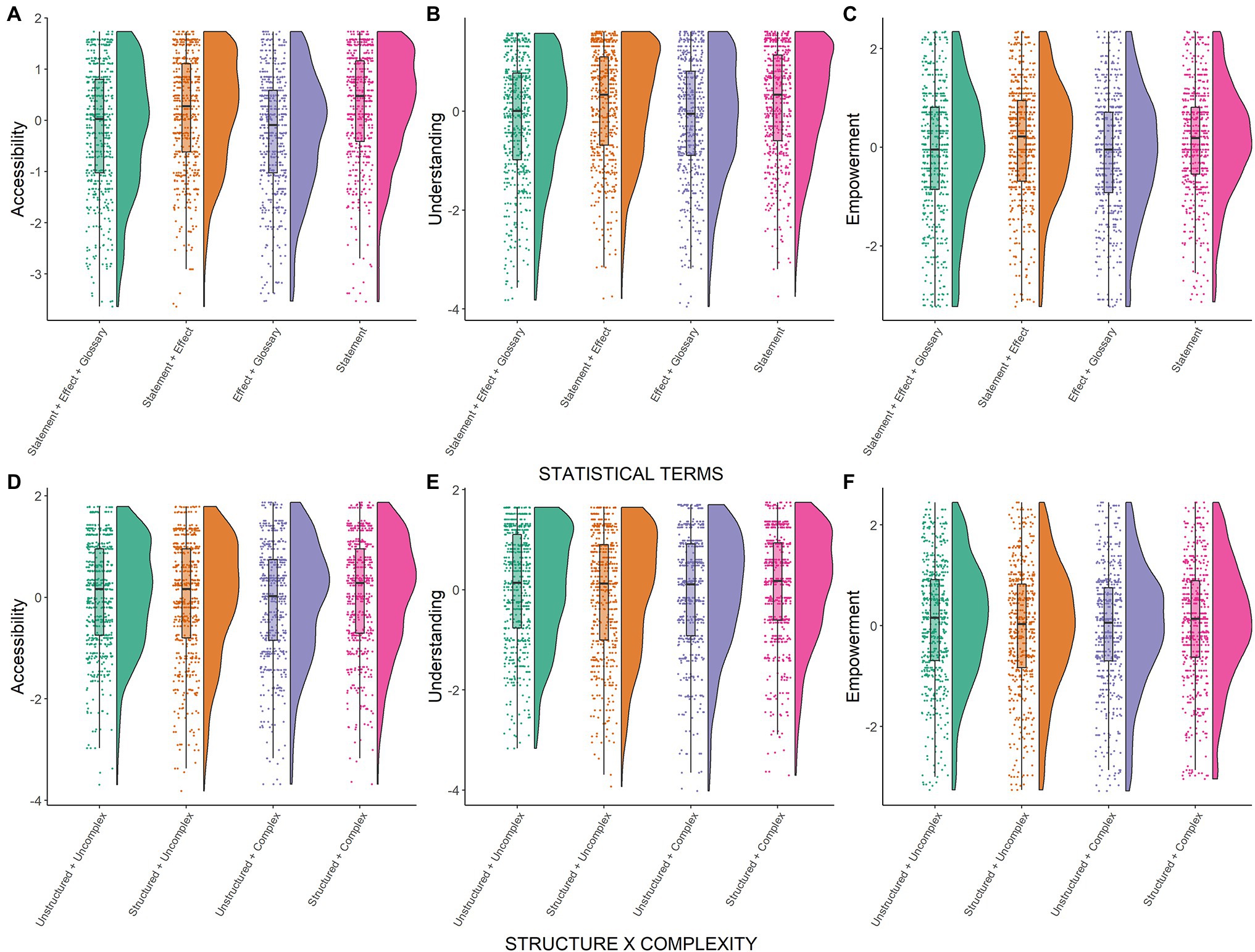
Figure 3. Results study 2. Raincloud plots for user experience outcomes: accessibility (A,D), understanding (B,E), and empowerment (C,F). Residual scores are depicted separated by experimental conditions: Statistical terms (A–C), interaction between structuring and complexity (D–F). Residual scores were obtained from a mixed model that controlled for participant/presented PLS as random factors and for the other independent variables as well as their interactions with complexity as fixed factors.
Content-Related Knowledge
For PLS with high complexity, the proportion of correct answers was descriptively lower in the effect size+glossary+qualitative statement condition (61.82%) compared to the effect size+glossary condition (69.53%), the effect size+qualitative statement condition (68.55%), or the qualitative statement condition (69.26%). For PLS with low complexity, the proportion of correct answers was generally higher than for PLS with high complexity, and differences in the proportion of correct answers were less pronounced on a descriptive level (effect size+glossary+qualitative statement condition 72.38%, effect size+glossary condition 73.17%, effect size+qualitative statement condition 74.17%, and qualitative statement condition 76.43%). Inferential analyses of our data on PLS with high complexity revealed that the likelihood to answer knowledge items correctly was significantly lower when a qualitative statement combined with a glossary was provided compared to all other conditions (i.e., in the effect size+glossary+qualitative statement condition, see Table 8)6. There were no significant differences between the other conditions for PLS with high complexity (all ps>0.800, see Table 8). For PLS with low complexity, there were no significant differences between all conditions (all ps>0.238, see Table 8). Thus, H5c and H6c are rejected as well.
H7 Structuring
Accessibility, Understanding, and Empowerment
For PLS with high complexity, user experience mean values were descriptively higher for structured PLS compared to unstructured PLS (see Table 6). The corresponding effect of structuring our PLS was significant for accessibility and understanding, but not for empowerment (see Table 7; Figures 3D–F). For PLS with low complexity, the opposite was true on a descriptive level. Contrary to our hypotheses, user experience mean values were lower for structured PLS compared to unstructured PLS (see Table 6). Corresponding two-sided inferential tests revealed that these differences were not significant (p=0.072–0.300, see Table 7). In sum, H7a and H7b are confirmed for PLS with high complexity only, while H7a and H7b are rejected for PLS with low complexity and H7d is fully rejected.
Content-Related Knowledge
For PLS with high and low complexity, the proportion of correct answers was virtually the same for structured (more complex=67.07%, less complex=73.20%) and unstructured PLS (more complex=67.75%, less complex=74.80%). Inferential analyses revealed that these differences, which were far from significant, did not point in the direction that was proposed in H7c (see Table 8). Thus, H7c is rejected.
H8 Extended Quality of Evidence Statement
Accessibility, Understanding, and Empowerment
Descriptively, user experience mean values were higher for the extended quality of evidence statement that explained complex meta-analytic designs – with accessibility for less complex PLS as an exception (see Table 6). All effects on user experience of the extension of our quality of evidence statement were, however, non-significant (all ps>0.109, see Table 7). Thus, H8a, H8b, and H8d are rejected.
Content-Related Knowledge
There was a negative effect of our extended quality of evidence statement on content-related knowledge for PLS with high complexity. The proportion of correct answers was significantly lower (see Table 8) when the extended statement was provided (64.75%) compared to the regular statement (70.14%). The corresponding effect for PLS with low complexity was not significant (see Table 8) but pointed in the opposite direction (extended=76.05%, regular=71.97%). As a consequence, H8c is partially confirmed.
Interim Discussion
Our results on approaches for explaining statistical terms in PLS (hypotheses 5 and 6) imply that glossaries of statistical terms negatively affect PLS readers’ user experience, regardless of PLS complexity. This might be due to the fact that additional cognitive effort is required to understand this (technical) information. Furthermore, exploratory evidence suggests that content-related knowledge acquisition might have suffered when a glossary and qualitative statements were presented for more complex PLS. Thus, our findings clearly implicate that PLS authors should refrain from using glossaries of statistical terms (see also General Discussion on technical limitations of implementing glossaries). Instead, using qualitative statements with or without reporting the corresponding effect size appears to be more beneficial for laypersons.
Our findings on hypothesis 7 suggest that structuring PLS has comparably small beneficial effects on more complex PLS – yet these findings cannot be generalized to less complex PLS. Contrary to our expectations, structuring descriptively impaired user experience for less complex PLS. However, we suggest that our “high complexity” PLS still set a rather low bar for “complexity” and, by far, do not represent the upper end of the obtainable “complexity continuum.” Possible side effects that we have (descriptively) observed for less complex PLS therefore appear unlikely to occur in practice. Thus, we argue that our results tentatively point toward structuring PLS as a way of improving user experience, which is associated with more benefits than drawbacks – especially in the case of highly complex PLS.
The pattern of effects, we observed with respect to the extension of our quality of evidence statement to explain complex meta-analytical designs was rather unexpected. One may argue, in hindsight, that the potential side effects on content-related knowledge for PLS with high complexity are attributable to the reported moderation being non-significant in one of our PLS with high complexity. Because the extended quality of evidence statement explicitly stated that meta-analyses are able to reveal moderator effects, this might have lured readers of our PLS into expecting that these effects indeed exist in the subsequently presented PLS. If future studies confirm this finding, this implies possible drawbacks of educating the public on the potential of scientific methods. This is because statements using illustrative examples as in our study might lead individuals to expect certain findings from applying certain scientific methods. However, our data clearly do not point toward any beneficial effects of an extended statement on the quality of evidence for communicating complex meta-analytic designs in terms of knowledge acquisition or user experience. Consequently, we cannot recommend the wider use of the extended version of this statement.
General Discussion
Main Findings
What lessons have we learned so far? As the overarching aim of our research was to develop guidelines for writing PLS, we will summarize our main findings in the form of brief writing instructions before discussing implications, strengths, and limitations of our two studies. The underlying rationale of these instructions – regarding specific statistical results and our hypotheses – can be found in the interim discussion sections of Study 1 and Study 2.
1. Do not provide too extensive information on the operationalization of synthesized studies, as this might negatively affect user experience.
However, corresponding effect sizes were very small and providing this additional information did not significantly diminish acquired knowledge. This might indicate that side effects on user experience can be tolerated if you are convinced that providing information on the operationalization is essential for your study.
2. Replace technical terms by non-technical terms if you want to support your audience in grasping the key message of your research.
Providing information on technical terms in a glossary had beneficial effects on user experience in Study 1, where we observed small effect sizes in the glossary and the replacement condition. Side effects of explaining technical terms in a glossary seem to exist, however, in terms of diminished knowledge acquisition. As these side effects showed very small to small effect sizes only, exceptions to this rule might still be appropriate if authors aim to specifically educate their readers about technical terms and concepts. In this specific case, providing a glossary with non-technical expressions might prove worthwhile. However, additional studies explicitly testing PLS effects on the acquisition of the corresponding conceptual knowledge (i.e., do readers really learn the meaning of technical terms) are required to substantiate this claim. We further argue that replacing technical terms might be easier and feel more natural for researchers when writing PLS in their native language (e.g., Cochrane provides PLS in languages other than English, see Kadic et al., 2016; Jakus et al., 2021). Because many technical terms stem from English as the lingua franca of science, no direct translations exist for some technical terms in other languages, which is why researchers from non-English speaking countries might automatically replace technical terms by non-technical ones when writing in their native language.
3. Provide information on the quality of evidence of meta-analyses (i.e., an explanation of meta-analyses as a methodological approach) when writing PLS of meta-analyses.
Providing this additional background information will allow audiences unfamiliar with the distinction between primary research and research syntheses to adequately grasp the strengths of claims brought forward in the meta-analysis you summarize. Corresponding effect sizes were very small to small. Moreover, utilizing such an explanatory statement appeared to have no significant negative effects on user experience and knowledge acquisition in our first study. However, findings of our second study suggest that this information should not be too extensive.
4. Carefully consider the amount and type of information on statistical terms you want to provide. If you do not specifically aim at statistically educating your audience, merely provide information on interpreting the effect size without additional details.
Our data indicate that glossaries on statistical terms come at the expense of user experience (with small effect sizes for different approaches on dealing with statistical terms). However, the way in which our glossaries (on technical terms in Study 1 and on statistical terms in Study 2) were presented might have impaired their effectiveness. Providing these texts embedded in websites or as infographics might be more appealing to laypersons (see Strengths, Implications, and Limitations).
5. Formally structure your PLS – especially when they are complex – by means of bullet points and/or use boldface text to highlight key words, if possible.
Our results show that, for PLS with high complexity, the application of formal text structuring improved user experience. Corresponding effects were, however, very small in our study and an exception to this rule exists: Structuring seems to be unnecessary for simple PLS – for example, when PLS focus on only one research finding and a very limited number of theoretical constructs.
Strengths, Implications, and Limitations
A major strength of our research – especially compared to previous studies on PLS – is that we investigated a total of eight PLS stemming from various psychological (sub-)disciplines. Thus, we are confident that our effects can be generalized to PLS of psychological meta-analyses in general and do not only apply to the specific PLS we studied. Our studies also make a valuable contribution to the existing body of research on PLS by experimentally investigating PLS characteristics in samples that were heterogeneous with regard to age, gender, and educational background (i.e., were not professional or student samples). Overall, we analyzed data of almost 4,500 participants. Thus, we are also quite confident that PLS complying with our rules will appeal to a large and diverse population. Another advantage of the large samples, we recruited is that the power of our analyses for detecting even small effects was high (Study 1: 0.900, Study 2: 0.875). As a consequence, non-significant study findings can be interpreted as evidence of an absence of effects with some degree of certainty.
Findings of our studies also fit in well with broader psychological theories on instructional design – especially cognitive load theory (Sweller, 1994, 2011; Orru and Longo, 2019) – and therefore imply that concepts from cognitive load theory should be considered when drafting PLS for psychological meta-analyses. At its core, cognitive load theory describes relationships between task aspects and the cognitive resources (i.e., working memory capacity) required by those tasks. Intrinsic cognitive load relates to the inherent difficulty of a task, whereas extraneous cognitive load is caused by other non-inherent factors, such as the presentation mode of a task. It is important that all types of cognitive load draw on the same limited working memory capacity (Engle, 2002). Thus, extraneous load should be minimized to unlock cognitive resources for learning processes, which generate germane cognitive load. In Study 1, we observed a positive interaction between interest in psychological topics and providing additional information on operationalization on some outcome variables, as well as a lower knowledge acquisition when a glossary on technical terms was provided. In Study 2, providing additional information via a glossary of statistical terms had a negative impact on user experience for PLS with high complexity. Additionally, for complex PLS, participants showed poorer content-related knowledge when receiving an extended quality of evidence statement. Based on cognitive load theory, one might argue that providing additional information on statistics, technical terms or the quality of evidence increases task-relevant intrinsic load (i.e., they make the task of reading and understanding the PLS more difficult) and that this is especially true for readers with low levels of interest or prior psychological concept knowledge. Furthermore, glossaries were presented as separate materials at the end of the respective PLS. This, contrary to a more integrated approach within the PLS itself, may have forced readers to switch between different text passages to mentally integrate information, thereby substantially increasing extrinsic cognitive load and hindering learning processes (see also split-attention effect, e.g., Schroeder and Cenkci, 2018, and contiguity principle, e.g., Mayer and Fiorella, 2014).
Nonetheless, our study is not without some limitations. With regard to our recommendation to leave out glossaries on statistical and technical terms, one might object that educating the public about research findings via PLS explicitly includes fostering public understanding of scientific jargon or statistical terms. Starting from this point, replacing technical jargon with non-technical terms or leaving out statistical information do not seem to be feasible approaches. One could argue instead that if one PLS aim entails enhancing knowledge of technical and statistical terms, glossaries (or different types of explanations) should be provided. The same argument could be made for providing information on operationalization, and our knowledge items were possibly limited in this regard as they solely focused on knowledge about the key message of the PLS. This underlines the need for a more nuanced assessment of knowledge gains in future studies on PLS as well as the necessity for individual PLS authors to reflect on specific aims they want to achieve by writing PLS.
Moreover, the way in which, we presented our glossaries on technical and statistical terms might have affected their impact. Participants might prefer explanations to be directly linked to the explicated terms (e.g., by means of pop-up windows) instead of an attached glossary at the end of the PLS. However, this kind of sophisticated approach requires appropriate software solutions which, in turn, might hinder the integration of the corresponding PLS into established reference databases (such as PubMed, PsycInfo, Psyndex, Google Scholar, and Web of Science). The same might hold true for our approaches on structuring PLS. Most journal abstracts consist of non-formatted text, and reference databases might not be able to add bullet points or bold text to text-based objects. Therefore, it might be an encouraging finding that the impact of structuring PLS was comparably small. Nonetheless, addressing these issues in future empirical research, and also from a technical infrastructure perspective, would be highly desirable.
To assess our outcomes, we employed single item measures to put as little strain as possible on our educationally diverse sample in a repeated-measures design. From a psychometric perspective, this is certainly a limitation. There is indeed an urgent need to develop empirically validated measurement tools for scientific research in educationally diverse layperson samples and to translate such tools to languages other than English. In the same vein, we tried to improve the wording of our user experience items in Study 2, but the correlations between accessibility, understanding, and empowerment were still high.
Using eight PLS from various topics might strengthen the generalizability of our findings. There are, however, certain costs of this procedure that become evident when interpreting our results because topic and study arm are confounded. For example, participants did not directly compare two versions of the same PLS with varying complexity in Study 2; rather PLS on different topics were presented here. Especially in Study 2, this makes it hard to interpret differences in the perception of PLS with high complexity and low complexity. Future studies should experimentally vary how many and what kind of effects are reported in a PLS to address this issue. One might also test whether our findings of the first study can be replicated for PLS on other topics or if PLS of the study arms are “switched” (i.e., when information on operationalization is provided for resilience research).
Finally, we acknowledge that many more PLS characteristics exist that are not yet empirically-validated and should be investigated in future research studies. We will refer to our review on PLS (Stoll et al., 2021; see also above) to illustrate this point. In this review, we identified six broad categories of PLS characteristics: linguistic attributes, formal attributes, general content, presentation of results, presentation of quality of evidence, and contextual attributes. In the studies reported in this article, we addressed characteristics of most of these categories: We outlined linguistic attributes as dealing with technical terms, formal attributes as structuring PLS, general content as inclusion of information on the operationalization in synthesized studies or glossaries, presentation of results as approaches for dealing with statistical terms and the presentation of the quality of evidence via introductory statements on the meta-analytical approach. Although, we covered a broad range of categories, it is easy to see that we only investigated some isolated (but important) aspects within these broad categories. Because no comprehensive framework on discipline-specific aspects of the lay-friendly communication of psychological science exists, the selection of these investigated characteristics in our studies certainly involved some degree of subjectivity. However, our selection is based on thorough theoretical reasoning, as we followed a comprehensive approach to (1) investigate such aspects that were reflected as core characteristics in our systematic review and (2) investigate those aspects that constitute the core elements (Shneider, 2009) of psychology as a scientific discipline. Not least, in a rather pragmatic approach, we selected such characteristics of which criteria could be readily derived and summarized in a writing guideline. Nonetheless, more work is needed to achieve the aim of empirically validated guidelines on writing psychological PLS.
Conclusion
Drawing on strong samples and a diverse set of PLS of psychological meta-analyses, our research demonstrates that the way we implement PLS characteristics (i.e., how we write PLS) affects PLS readers’ knowledge acquisition and user experience. Based on the results of two randomized controlled studies, we derived five simple rules that will hopefully support PLS authors in significantly increasing user experience and information uptake in educationally diverse samples. Returning to the basic notion with which we started this article, we hope that the research presented in this article will be valuable to a broad audience. First and foremost, we hope that this article is useful as a means of raising psychological researchers’ awareness of PLS as a tool for transferring psychological evidence to the public and that its findings will maximize the beneficial impact of PLS in psychology. Sticking to the rationale underlying PLS, we, however, also hope that this research will prove to be valuable – beyond this initial target audience – for PLS authors from all disciplines of science.7
Data Availability Statement
Data and code for this publication are available at PsychArchives: data (http://dx.doi.org/10.23668/psycharchives.5211), code (http://dx.doi.org/10.23668/psycharchives.5210).
Ethics Statement
The studies involving human participants were reviewed and approved by the ethics committee of Trier University. The participants provided their written informed consent to participate in this study.
Author Contributions
AC, MK, and MS contributed to conception and design of Study 1. All authors contributed to the conception and design of Study 2. MK managed the data, performed the statistical analysis, and wrote the first draft of the manuscript. AC, GB, MJ, and MS wrote sections of the manuscript. All authors contributed to the article and approved the submitted version.
Funding
This work was funded by internal funds of the ZPID. The authors received no third-party funding for this work.
Conflict of Interest
The authors declare that the research was conducted in the absence of any commercial or financial relationships that could be construed as a conflict of interest.
Publisher’s Note
All claims expressed in this article are solely those of the authors and do not necessarily represent those of their affiliated organizations, or those of the publisher, the editors and the reviewers. Any product that may be evaluated in this article, or claim that may be made by its manufacturer, is not guaranteed or endorsed by the publisher.
Acknowledgments
We thank Eva Becker for her support in preparing study materials, and Michelle Bähr for her support in preparing study materials and tables for this manuscript. We would also like to thank Lisa Trierweiler for the English proof of the manuscript.
Supplementary Material
The Supplementary Material 1 and 4 for this article can be found online at: https://www.frontiersin.org/articles/10.3389/fpsyg.2021.771399/full#supplementary-material
Supplementary Material 1 | Translations of study materials.
Supplementary Material 2 | R-Markdown Files: http://dx.doi.org/10.23668/psycharchives.5210.
Supplementary Material 3 | Datasets and Codebooks: http://dx.doi.org/10.23668/psycharchives.5210.
Supplementary Material 4 | Plain language summary of this article.
Footnotes
1. ^The numbering of hypotheses 5–8 deviates from the numbering in the corresponding preregistration (hypotheses 1, 2, 3, and 4) because we opted to consecutively number hypotheses throughout this article to avoid any potential confusion.
2. ^Since we observed detrimental effects of our operationalization statement in Study 1 (see below), one could, however, argue that presenting this extended quality of evidence statement also increases the complexity of the relevant task, which is why we pre-specified no strict assumption on the direction of effects for hypothesis 8.
3. ^http://dx.doi.org/10.23668/psycharchives.4471
4. ^To stay closely in line with our power analysis, we also provide additional ANOVA statistics on these mixed models in Supplementary Material 2.
5. ^http://dx.doi.org/10.23668/psycharchives.4791
6. ^This exploratory finding was, however, non-significant when applying the Benjamini-Hochberg correction for multiple testing, with p values ranging from 0.055 to 0.074.
7. ^For this reason – and to set a good example – we wrote our own PLS for this article which is available as Supplementary Material 4.
References
AGU (n.d.). “Creating a Plain Language Summary.” AGU Advancing Earth and Space Science. Available at: https://www.agu.org/-/media/Files/Share-and-Advocate-for-Science/Toolkit---PLS.pdf (Accessed December 21, 2020).
Alderdice, F., McNeill, J., Lasserson, T., Beller, E., Carroll, M., Hundley, V., et al. (2016). Do cochrane summaries help student midwives understand the findings of cochrane systematic reviews: the BRIEF randomised trial. Syst. Rev. 5:40. doi: 10.1186/s13643-016-0214-8
American Psychological Association (2018). Guidance for Translational Abstracts and Public Significance Statements: Demonstrating the Public Significance of Research. Available at: https://www.apa.org/pubs/journals/resources/translational-messages (Accessed November 22, 2021).
Anzinger, H., Elliott, S. A., and Hartling, L. (2020). Comparative usability analysis and parental preferences of three web-based knowledge translation tools: multimethod study. J. Med. Internet Res. 22:e14562. doi: 10.2196/14562
Barton, K. (2020). “MuMIn: Multi-Model Inference.” Available at: https://CRAN.R-project.org/package=MuMIn (Accessed November 22, 2021).
Bastian, H., Glasziou, P., and Chalmers, I. (2010). Seventy-five trials and eleven systematic reviews a day: how will we ever keep up? PLoS Med. 7:e1000326. doi: 10.1371/journal.pmed.1000326
Bates, D., Mächler, M., Bolker, B., and Walker, S. (2015). Fitting linear mixed-effects models using Lme4. J. Stat. Softw. 67, 1–48. doi: 10.18637/jss.v067.i01
Bergmann, C., and Cristia, A. (2016). Development of infants’ segmentation of words from native speech: a meta-analytic approach. Dev. Sci. 19, 901–917. doi: 10.1111/desc.12341
Borenstein, M., Hedges, L. V., Higgins, J. P. T., and Rothstein, H. R. (2009). Introduction to Meta-Analysis. Chichester, UK: John Wiley & Sons, Ltd.
Bromme, R., and Goldman, S. R. (2014). The public’s bounded understanding of science. Educ. Psychol. 49, 59–69. doi: 10.1080/00461520.2014.921572
Bucher, L., Tran, U. S., Prinz, G. M., Burgard, T., Bosnjak, M., and Voracek, M. (2020). Keeping meta-analyses alive and well: Using PsychOpenCAMA to implement a community-augmented meta-analysis on the Dark Triad of personality. Leibniz Institut für Psychologische Information und Dokumentation (ZPID). doi: 10.23668/PSYCHARCHIVES.2752
Buljan, I., Malički, M., Wager, E., Puljak, L., Hren, D., Kellie, F., et al. (2018). No difference in knowledge obtained from infographic or plain language summary of a cochrane systematic review: three randomized controlled trials. J. Clin. Epidemiol. 97, 86–94. doi: 10.1016/j.jclinepi.2017.12.003
Buljan, I., Tokalić, R., Roguljić, M., Zakarija-Grković, I., Vrdoljak, D., Milić, P., et al. (2020). Framing the numerical findings of cochrane plain language summaries: two randomized controlled trials. BMC Med. Res. Methodol. 20:101. doi: 10.1186/s12874-020-00990-4
Burgard, T., Bošnjak, M., and Studtrucker, R. (2021). Community-augmented meta-analyses (CAMAs) in psychology: potentials and current systems. Z. Psychol. 229, 15–23. doi: 10.1027/2151-2604/a000431
Carvalho, F. A., Elkins, M. R., Franco, M. R., and Pinto, R. Z. (2019). Are plain-language summaries included in published reports of evidence about physiotherapy interventions? Analysis of 4421 randomised trials, systematic reviews and guidelines on the physiotherapy evidence database (PEDro). Physiotherapy 105, 354–361. doi: 10.1016/j.physio.2018.11.003
Christodoulou, J., Lac, A., and Moore, D. S. (2017). Babies and math: a meta-analysis of infants’ simple arithmetic competence. Dev. Psychol. 53, 1405–1417. doi: 10.1037/dev0000330
Cochrane Methods (2013). Methodological Expectations of Cochrane Intervention Reviews (MECIR): Standards for the Reporting of Plain Language Summaries in New Cochrane Intervention Reviews 2013. 1st Edn. Available at: https://methods.cochrane.org/sites/default/files/public/uploads/pleacs_2019.pdf (Accessed November 22, 2021).
Cochrane Norway (2016). How to Write a Plain Language Summary of a Cochrane Intervention Review: Checklist. Available at: https://www.cochrane.no/sites/cochrane.no/files/public/uploads/checking_a_cochrane_pls_15th_june_2018.pdf (Accessed November 22, 2021).
Cochrane Norway (2019). How to Write a Plain Language Summary of a Cochrane Intervention Review. Available at: https://www.cochrane.no/sites/cochrane.no/files/public/uploads/how_to_write_a_cochrane_pls_12th_february_2019.pdf (Accessed November 22, 2021).
Dunst, C., Gorman, E., and Hamby, D. (2012). “Preference for infant-directed speech in preverbal young children.” Center for Early Literacy Learning 5, 1–13.
Ellen, M. E., Lavis, J. N., Wilson, M. G., Grimshaw, J. R., Haynes, B., Ouimet, M., et al. (2014). Health system decision makers’ feedback on summaries and tools supporting the use of systematic reviews: a qualitative study. Evid. Policy J. Res. Deb. Pract. 10, 337–359. doi: 10.1332/174426514X672362
Engle, R. W. (2002). Working memory capacity as executive attention. Curr. Dir. Psychol. Sci. 11, 19–23. doi: 10.1111/1467-8721.00160
Faul, F., Erdfelder, E., Buchner, A., and Lang, A.-G. (2009). Statistical power analyses using G*power 3.1. Behav. Res. Methods 41, 1149–1160. doi: 10.3758/BRM.41.4.1149
FitzGibbon, H., King, K., Piano, C., Wilk, C., and Gaskarth, M. (2020). Where are biomedical research plain-language summaries? Health Sci. Rep. 3:e175. doi: 10.1002/hsr2.175
Glenton, C., Santesso, N., Rosenbaum, S., Nilsen, E. S., Rader, T., Ciapponi, A., et al. (2010). Presenting the results of cochrane systematic reviews to a consumer audience: a qualitative study. Med. Decis. Mak. 30, 566–577. doi: 10.1177/0272989X10375853
Groth, N., Schnyder, N., Kaess, M., Markovic, A., Rietschel, L., Moser, S., et al. (2019). Coping as a mediator between locus of control, competence beliefs, and mental health: a systematic review and structural equation modelling meta-analysis. Behav. Res. Ther. 121:103442. doi: 10.1016/j.brat.2019.103442
Hartley, J. (2004). Current findings from research on structured abstracts. J. Med. Libr. Assoc. 92, 368–371.
Hartley, J. (2014). Current findings from research on structured abstracts: an update. J. Med. Libr. Assoc. 102, 146–148. doi: 10.3163/1536-5050.102.3.002
Hartley, J., and Betts, L. (2007). The effects of spacing and titles on judgments of the effectiveness of structured abstracts. J. Am. Soc. Inf. Sci. Technol. 58, 2335–2340. doi: 10.1002/asi.20718
Hauck, S. A. (2019). Sharing planetary science in plain language. J. Geophys. Res. Planets 124, 2462–2464. doi: 10.1029/2019JE006152
Hoogeveen, S., Sarafoglou, A., and Wagenmakers, E.-J. (2020). Laypeople can predict which social-science studies will be replicated successfully. Adv. Methods Pract. Psychol. Sci. 3, 267–285. doi: 10.1177/2515245920919667
Hothorn, T., Bretz, F., and Westfall, P. (2008). Simultaneous inference in general parametric models. Biom. J. 50, 346–363. doi: 10.1002/bimj.200810425
Jaeger, B. C., Edwards, L. J., Das, K., and Sen, P. K. (2017). An R2 statistic for fixed effects in the generalized linear mixed model. J. Appl. Stat. 44, 1086–1105. doi: 10.1080/02664763.2016.1193725
Jakus, D., Behmen, D., Buljan, I., Marušić, A., and Puljak, L. (2021). Efficacy of reminders for increasing volunteer engagement in translating cochrane plain language summaries: a pilot randomised controlled trial. BMJ Evid. Based Med. 26, 49–50. doi: 10.1136/bmjebm-2020-111378
Kadic, J., Antonia, M. F., Vujcic, M., Saric, F., Propadalo, I., Marelja, I., et al. (2016). Cochrane plain language summaries are highly heterogeneous with low adherence to the standards. BMC Med. Res. Methodol. 16:61. doi: 10.1186/s12874-016-0162-y
Kaslow, N. J. (2015). Translating psychological science to the public. Am. Psychol. 70, 361–371. doi: 10.1037/a0039448
Kerwer, M., Chasiotis, A., Stricker, J., Günther, A., and Rosman, T. (2021). Straight from the scientist’s mouth—plain language summaries promote laypeople’s comprehension and knowledge acquisition when reading about individual research findings in psychology. Collabra Psychol. 7:18898. doi: 10.1525/collabra.18898
Kirkpatrick, E., Gaisford, W., Williams, E., Brindley, E., Tembo, D., and Wright, D. (2017). Understanding plain english summaries. A comparison of two approaches to improve the quality of plain english summaries in research reports. Res. Involv. Engagem. 3, 1–14. doi: 10.1186/s40900-017-0064-0
Klein, O., Hardwicke, T. E., Aust, F., Breuer, J., Danielsson, H., Mohr, A. H., et al. (2018). A practical guide for transparency in psychological science. Collabra Psychol. 4:20. doi: 10.1525/collabra.158
Kuznetsova, A., Brockhoff, P. B., and Christensen, R. H. B. (2017). LmerTest package: tests in linear mixed effects models. J. Stat. Softw. 82, 1–26. doi: 10.18637/jss.v082.i13
Maguire, L. K., and Clarke, M. (2014). How much do you need: a randomised experiment of whether readers can understand the key messages from summaries of cochrane reviews without reading the full review. J. R. Soc. Med. 107, 444–449. doi: 10.1177/0141076814546710
Martínez Silvagnoli, L., Shepherd, C., Pritchett, J., and Gardner, J. (2020). How Can We Optimize the Readability and Format of Plain Language Summaries for Medical Journal Articles? A Cross-sectional Survey. Study. J. Med. Int. Res. 22122 (in press).
Mayer, R. E., and Fiorella, L. (2014). “Principles for reducing extraneous processing in multimedia learning: coherence, signaling, redundancy, spatial contiguity, and temporal contiguity principles,” in The Cambridge Handbook of Multimedia Learning. ed. R. Mayer. 2nd ed (Cambridge: Cambridge University Press), 279–315.
Morville, P. (2004). “User Experience Design.” Semantic Studios (blog). Available at: http://semanticstudios.com/user_experience_design/ (Accessed November 22, 2021).
Nakagawa, S., Schielzeth, H., and O’Hara, R. B. (2013). A general and simple method for obtaining R2 from generalized linear mixed-effects models. Methods Ecol. Evol. 4, 133–142. doi: 10.1111/j.2041-210x.2012.00261.x
Nunn, E., and Pinfield, S. (2014). Lay summaries of open access journal articles: engaging with the general public on medical research. Learn. Publish. 27, 173–184. doi: 10.1087/20140303
Open Science Collaboration (2015). Estimating the reproducibility of psychological science. Science 349:aac4716. doi: 10.1126/science.aac4716
Opiyo, N., Shepperd, S., Musila, N., Allen, E., Nyamai, R., Fretheim, A., et al. (2013). Comparison of alternative evidence summary and presentation formats in clinical guideline development: a mixed-method study. PLoS One 8:e55067. doi: 10.1371/journal.pone.0055067
Orru, G., and Longo, L. (2019). “The evolution of cognitive load theory and the measurement of its intrinsic, extraneous and germane loads: a review,” in Human Mental Workload: Models and Applications. eds. L. Longo and M. C. Leva, vol. 1012 (Cham: Springer International Publishing), 23–48.
Patall, E. A. (2021). Implications of the open science era for educational psychology research syntheses. Educ. Psychol. 56, 142–160. doi: 10.1080/00461520.2021.1897009
People and Nature (n.d.). “Plain Language Summary Guidelines.” British Ecological Society Journals. Available at: https://besjournals.onlinelibrary.wiley.com/pb-assets/hub-assets/besjournals/2575-8314_PaN/People%20and%20Nature%20Plain%20Language%20Summary%20Guidelines-1540981909900.pdf (Accessed December 21, 2020).
Peters, H. P. (2013). Gap between science and media revisited: scientists as public communicators. Proc. Natl. Acad. Sci. U. S. A. 110, 14102–14109. doi: 10.1073/pnas.1212745110
Post, S., Bienzeisler, N., and Lohöfener, M. (2021). A desire for authoritative science? How citizens’ informational needs and epistemic beliefs shaped their views of science, news, and policymaking in the COVID-19 pandemic. Public Underst. Sci. 30, 496–514. doi: 10.1177/09636625211005334
R Core Team (2021). R: A Language and Environment for Statistical Computing. Vienna, Austria: R Foundation for Statistical Computing. Available at: https://www.R-project.org/ (Accessed November 22, 2021).
Rakedzon, T., Segev, E., Chapnik, N., Yosef, R., and Baram-Tsabari, A. (2017). Automatic jargon identifier for scientists engaging with the public and science communication educators. PLoS One 12:e0181742. doi: 10.1371/journal.pone.0181742
Rasmussen, P. D., Storebø, O. J., Løkkeholt, T., Voss, L. G., Shmueli-Goetz, Y., Bojesen, A. B., et al. (2019). Attachment as a core feature of resilience: a systematic review and meta-analysis. Psychol. Rep. 122, 1259–1296. doi: 10.1177/0033294118785577
Raynor, D. K., Myers, L., Blackwell, K., Kress, B., Dubost, A., and Joos, A. (2018). Clinical trial results summary for laypersons: a user testing study. Ther. Innov. Regul. Sci. 52, 606–628. doi: 10.1177/2168479017753129
Rodgers, P. (2017). Plain-language summaries of research: writing for different readers. ELife 6:e25408. doi: 10.7554/eLife.25408
Rosenbaum, S. (2010). “Improving the User Experience of Evidence. A Design Approach to Evidence-Informed Health Care.” PhD Thesis. The Oslo School of Architecture and Design.
Salita, J. T. (2015). Writing for lay audiences: a challenge for scientists. Med. Writ. 24, 183–189. doi: 10.1179/2047480615Z.000000000320
Sánchez-Meca, J., and Marín-Martínez, F. (2010). Meta-analysis in psychological research. Int. J. Psychol. Res. 3, 150–162. doi: 10.21500/20112084.860
Santesso, N., Glenton, C., and Lang, B. (2008). Evidence that patients can understand and use? Z. Evid. Fortbild. Qual. Gesundheitswe. 102, 493–496. doi: 10.1016/j.zefq.2008.08.027
Santesso, N., Rader, T., Nilsen, E. S., Glenton, C., Rosenbaum, S., Ciapponi, A., et al. (2015). A summary to communicate evidence from systematic reviews to the public improved understanding and accessibility of information: a randomized controlled trial. J. Clin. Epidemiol. 68, 182–190. doi: 10.1016/j.jclinepi.2014.04.009
Schroeder, N. L., and Cenkci, A. T. (2018). Spatial contiguity and spatial split-attention effects in multimedia learning environments: a meta-analysis. Educ. Psychol. Rev. 30, 679–701. doi: 10.1007/s10648-018-9435-9
Schwalm, F. D., Zandavalli, R. B., de Castro Filho, E. D., and Lucchetti, G. (2021). “Is there a relationship between spirituality/religiosity and resilience? A systematic review and meta-analysis of observational studies.” J. Health Psychol. doi: 10.1177/1359105320984537 [Epub ahead of print].
Sharpe, D., and Poets, S. (2020). Meta-analysis as a response to the replication crisis. Can. Psychol. 61, 377–387. doi: 10.1037/cap0000215
Shneider, A. M. (2009). Four stages of a scientific discipline; four types of scientist. Trends Biochem. Sci. 34, 217–223. doi: 10.1016/j.tibs.2009.02.002
Steinmetz, H., Bosnjak, M., and Isidor, R. (2020). Meta-analytische strukturgleichungsmodelle: potenziale und grenzen illustriert an einem beispiel aus der organisationspsychologie. Psychol. Rundsch. 71, 111–118. doi: 10.1026/0033-3042/a000483
Stoll, M., Kerwer, M., Lieb, K., and Chasiotis, A. (2021). “Plain language summaries: a systematic review of theory, guidelines, and empirical research.” PsychArchives [Preprint]. doi: 10.23668/PSYCHARCHIVES.5044
Sweller, J. (1994). Cognitive load theory, learning difficulty, and instructional design. Learn. Instr. 4, 295–312. doi: 10.1016/0959-4752(94)90003-5
Sweller, J. (2011). “Cognitive load theory,” in Psychology of Learning and Motivation, vol. 55. eds. J. P. Mestre and B. H. Ross (San Diego, United States: Elsevier), 37–76.
Sweller, J., Ayres, P., and Kalyuga, S. (2011). Cognitive Load Theory. New York, NY: Springer New York.
Świątkowski, W., and Dompnier, B. (2017). Replicability crisis in social psychology: looking at the past to find new pathways for the future. Int. Rev. Soc. Psychol. 30:111. doi: 10.5334/irsp.66
Teo, T. (2017). From psychological science to the psychological humanities: building a general theory of subjectivity. Rev. Gen. Psychol. 21, 281–291. doi: 10.1037/gpr0000132
Tomita, Y., Moldovan, M., Lee, R. C., Hsieh, A. H. C., Townsend, A., and Price, T. (2020). Salvage systemic therapy for advanced gastric and oesophago-gastric junction adenocarcinoma. Cochrane Database Syst. Rev. 11:CD012078. doi: 10.1002/14651858.CD012078.pub2
Wingen, T., Berkessel, J., and Dohle, S. (2021). “Caution, preprint! Brief explanations allow non-scientists to differentiate between preprints and peer-reviewed journal articles.” [Preprint]. doi: 10.31219/osf.io/7n3mj
Keywords: plain language summaries, science communication, lay summaries, guideline, knowledge acquisition, accessibility, understanding, empowerment
Citation: Kerwer M, Stoll M, Jonas M, Benz G and Chasiotis A (2021) How to Put It Plainly? Findings From Two Randomized Controlled Studies on Writing Plain Language Summaries for Psychological Meta-Analyses. Front. Psychol. 12:771399. doi: 10.3389/fpsyg.2021.771399
Edited by:
Bernhard Ertl, Munich University of the Federal Armed Forces, GermanyReviewed by:
Lena Wimmer, University of Freiburg, GermanyCornelia Schoor, Leibniz Institute for Educational Trajectories (LG), Germany
Copyright © 2021 Kerwer, Stoll, Jonas, Benz and Chasiotis. This is an open-access article distributed under the terms of the Creative Commons Attribution License (CC BY). The use, distribution or reproduction in other forums is permitted, provided the original author(s) and the copyright owner(s) are credited and that the original publication in this journal is cited, in accordance with accepted academic practice. No use, distribution or reproduction is permitted which does not comply with these terms.
*Correspondence: Martin Kerwer, bWtAbGVpYm5pei1wc3ljaG9sb2d5Lm9yZw==