- 1School of Applied Psychology and (International) Human Resource Management, Program Employability Transition, Section Inclusive Society, Saxion University of Applied Sciences, Deventer, Netherlands
- 2Faculty of Veterinary Medicine, Utrecht University, Utrecht, Netherlands
- 3Section Health Psychology, Department of Health Sciences, University Medical Center Groningen, University of Groningen, Groningen, Netherlands
Introduction: Self-regulated learning (SRL) has traditionally been associated with study success in higher education. In contrast, study success is still rarely associated with executive functions (EF), while it is known from neuropsychological practice that EF can influence overall functioning and performance. However some studies have shown relationships between EF and study success, but this has mainly been investigated in school children and adolescents. EF refer to higher-order cognitive processes to regulate cognition, behavior, and emotion in service of adaptive and goal-directed behaviors. SRL is a dynamic process in which learners activate and maintain cognitions, affects, and behaviors to achieve personal learning goals. This study explores the added value of including EF and SRL to predict study success (i.e., the obtained credits).
Methods: In this study, we collected data from 315 first-year psychology students of a University of Applied Sciences in the Netherlands who completed questionnaires related to both EF (BRIEF) and SRL (MSLQ) two months after the start of the academic year. Credit points were obtained at the end of that first academic year. We used Structural Equation Modeling to test whether EF and SRL together explain more variance in study success than either concept alone.
Results: EF explains 19.8% of the variance, SRL 22.9%, and in line with our hypothesis, EF and SRL combined explain 39.8% of the variance in obtained credits.
Discussion: These results indicate that focusing on EF and SRL could lead to a better understanding of how higher education students learn successfully. This might be the objective of further investigation.
1 Introduction
Executive functions (EFs) strategies, i.e., strategies that help students learn new content or solve problems are vital in developing lifelong learning skills. However, up to now, most educational research has focused on self-regulated learning (SRL) to explain successful learning and study success (e.g., Hayat et al., 2020; Moghadari-Koosha et al., 2020; Sun et al., 2023). In contrast, EF is mainly approached from a neuropsychological and clinical perspective, focusing on EF dysfunction and related educational problems (e.g., Meltzer et al., 2018, pp. 109–141; Dijkhuis et al., 2020), and it is hardly studied in ecological settings (i.e., standard learning settings). The studies conducted on EF in the educational context have primarily focused on children and adolescents (e.g., Diamond and Ling, 2016; Pascual et al., 2019; Zelazo and Carlson, 2020), not on young adults in higher education. To our best knowledge, EF and SRL have yet to be examined in combination within the context of higher education. Therefore, this study explores the relationship between EF and SRL and the extent to which they impact students’ study success in higher education.
Executive function and SRL originate from two paradigms, respectively founded in neuropsychology and based on educational research. Both have their methods, tests, and language. Researchers generally base their research on one of two perspectives. Nonetheless, both concepts have been associated with successful studying and study success in young adults (e.g., Garner, 2009; Musso et al., 2019; Pinochet-Quiroz et al., 2022) and are essential to a broader understanding of student’s ability to learn. The following section describes the definitions, similarities and differences between EF and SRL.
Self-regulated learning is about students becoming masters of their learning process which implies being able to adopt the most appropriate strategy for a learning task to be developed (Zimmerman, 2015; Dent and Koenka, 2016). SRL is generally considered a dynamic, cyclical process consisting of different phases and sub-processes of learning (Panadero, 2017). One of the most used and well-operationalized SRL models states that the cyclical process contains the following phases: (1) forethought, planning, and activation; (2) monitoring; (3) control; and (4) reaction and reflection (Pintrich, 2004). Each phase has four different areas for regulation: cognition, motivation/affect, behavior, and context.
Executive functions can be defined as a set of cognitive processes, partially independent and involved in top-down control of behavior, emotion, and cognition (Baggetta and Alexander, 2016; Nigg, 2017). EF refer to the most basic level of behavioral analyses or the neuropsychological level (De la Fuente et al., 2022). EF are effortful and invoked when automatic responses and routines do not work. This mainly happens in novel, complex, or otherwise challenging situations (Miller and Cohen, 2001; Barkley, 2012; Diamond, 2013). Therefore, EF are critical in learning, study success, and flexible behavior (Denckla and Mahone, 2018, p. 6).
Executive functions are a multidimensional concept; the literature describes several classifications of EF. Baggetta and Alexander (2016) found 39 different components or processes of EF in their review, with three core EF being the most commonly mentioned in the 106 studies they examined, i.e., inhibition (68%), working memory (35%), and cognitive flexibility (31%):
1. Inhibition (inhibitory control, including self-control or behavioral inhibition; or interference control, including selective attention and cognitive inhibition). This is the ability to control one’s attention or inhibit dominant or automatic behavior, responses, thoughts, and emotions (e.g., Baggetta and Alexander, 2016). For example, being able to study for a more extended time without being distracted.
2. Working memory. This ability is described as keeping the information in mind and working with it (e.g., Baddeley, 2010; Diamond, 2013). For example, reading a textbook, remembering what you read, and coming up with examples from your own experience that relate to what is described in the learning materials.
3. Cognitive (or mental) flexibility (or set-shifting). This refers to the ability to literally and figuratively change perspective, remove irrelevant information and retrieve new information, think differently, or change your behavior (e.g., Diamond, 2013; Nigg, 2017). Essentially, it is about adapting to a changed situation. For instance, while working on a group assignment in an (interdisciplinary) team, being able to put yourself in someone else’s perspective.
Combining these core “lower-order” processes creates “higher-order” or complex processes such as planning, reasoning, and problem-solving (Diamond, 2013).
One of the classifications that describes both the core and complex EF and is often used in research (in both academic and clinical contexts) is that of Gioia et al. (2000). We chose this classification because of its well-operationalized EF components and its emphasis on assessing behavioral manifestations of EF in an individual’s daily life (Baggetta and Alexander, 2016). Additionally, based on this classification Gioia et al. (2000) developed the Behavioral Rating Inventory Executive Functions (BRIEF), a self-reported questionnaire that has been translated into Dutch and standardized for children (Huizinga and Schmidts, 2012) and adults (Scholte and Noens, 2011).
This classification – based on factor analyses of EF behavioral descriptions – comprises nine EF, including the three core EF described before, next to the more complex or higher-order EF “self-monitor,” “emotional control,” “initiate,” “task monitor,” “plan/organize,” and “organization of materials.”
Executive function can be conceptualized on two levels: the core EF on a cognitive level (i.e., how the brain thinks) and the core EF and complex EF on a behavioral level (i.e., how the brain thinks expressed in behavior). Both levels refer to EF; however, in studies, they are operationalized and measured differently and refer to different underlying mechanisms of EF (e.g., Barkley and Fischer, 2011; Toplak et al., 2013). Researchers hypothesize that this is why directly or task-based measured core EF hardly overlap with the indirectly or self-reported measured EF (e.g., Barkley and Fischer, 2011; Toplak et al., 2013).
An advantage of directly assessing EF is that these task-based tests better test the actual performance of a specific EF. However, these results provide information about how well the student functions in an optimal and highly structured environment and, therefore, are not easily generalized across settings (Naglieri and Otero, 2014, pp. 191–208). The advantage of a self-reported EF is its higher ecological validity because it provides information about how well the student functions in a less structured environment, such as a school or home setting (Barkley and Fischer, 2011). An assumption with self-reports is that they measure behaviors related to the cognitive processes measured by task-based measures of EF. Because we are interested in how students experience their EF in their daily settings, we use self-report questionnaires in this study to assess EF.
The same reason applies to SRL; we are interested in the students’ perceptions of their SRL in general. SRL self-reports fall under the category of “offline measures,” referring to the timing of the measurements, in this case, that the self-reports are taken before or after the task and not during the task (i.e., “online measures,” such as think-aloud protocols or systematic observations) (Veenman, 2005; Schellings, 2011). When taking an SRL self-report, the student reflects on how they usually approach the learning task, so it provides more general information than specific information about a task at that moment. Thus, it depends on the research question of which measurement instrument is most appropriate (Rovers et al., 2019).
Self-reports also have drawbacks. Paradoxically, being able to complete the self-reports requires EF of the student to reflect on past and future behaviors (Garner, 2009), suggesting that a student with weak EF will be less able to self-report.
Another issue might be that students over- or underestimate themselves and whether there is a discrepancy between their intentional behavior and what they actually demonstrate [as demonstrated for SRL by Broadbent and Poon (2015)]. Students who overstated their performance on EF performance measures also achieved significantly higher scores on self-reports (Follmer, 2021).
Rovers et al. (2019) showed that students can report – via questionnaires – relatively accurately what their general self-regulatory functioning is, while at a detailed level, they have difficulty pinpointing exact SRL strategies. They argue that the level of granularity is of influence and that different types of measurement are valuable, depending on the research question. The same kind of reasoning could apply to the practical use of these measurements. For example, the benefit of self-reporting is that students become aware of their SRL strategies, which is an intervention in itself (Panadero et al., 2016). So, if self-monitoring is the objective, self-reports are an excellent option.
Conceptually, both EF and SRL refer to higher-order (top-down) cognitive processes. However, they differ in the context in which they are applied. EF are essential for navigating everyday life and engaging in social interactions (Barkley, 2012). EF become active when a student faces new, complex, or challenging daily life problems, including but not limited to problems encountered in the learning environment. In those moments, the student must make decisions, resolve issues, learn from mistakes, mentally play with ideas (be creative), think before acting, resist temptations, and stay focused (Diamond, 2013). In contrast, SRL occurs specifically and exclusively in the learning context and focuses on acquiring knowledge, automating skills, and achieving learning results. SRL involves both conscious and unconscious deep processing of information, or the repetition of facts, to eventually consolidate this information in long-term memory (Wirth et al., 2020).
Another difference is that SRL strongly emphasizes motivation or the “why” someone does something and the willingness to put effort into it (Schunk and Greene, 2018), in contrast to EF, which focus more on the “how,” i.e., “how do I solve this problem or adapt to the situation?”
Studies on the relationship between EF and SRL suggest a partial overlap between the constructs (e.g., Garner, 2009; Effeney et al., 2013; Follmer and Sperling, 2016). In these studies, EF and SRL are – indirectly – measured via self-reports demonstrating that EF expressed on a behavioral level overlap partially with SRL, also expressed on a behavioral level. Moreover, it seems that, in particular, the metacognitive dimensions of SRL are associated with or coincide with EF, for instance, planning (Effeney et al., 2013; Pinochet-Quiroz et al., 2022). The ability to plan allows a student to set and achieve goals in everyday life (context of EF) and focus explicitly on prioritizing learning tasks (context of SRL). However, self-reported EF and SRL are not the same in learning environments, and when overlapping, EF appear to contribute to variability in SRL processes, and the other way around, SRL processes implicate EFs (Garner, 2009).
However, in contrast, EF appear to be more unidirectionally related to SRL when measured directly through neuropsychological tasks, i.e., meaning task-based EF mediate through SRL on academic achievement and not the other way around (e.g., Rutherford et al., 2018; Musso et al., 2019). Only the core EF (i.e., working memory, inhibition, or cognitive flexibility) are task-based measured in these cases. In other words, SRL strategies seem to employ core EF – on a cognitive level – to achieve learning results, which makes sense because to sustain a learning strategy, the student must focus, keep information online, avoid distractions, and be cognitively flexible in disregarding old information in favor of new information.
In summary, EF can be conceptualized and measured at a cognitive and behavioral level (typically task-based and self-reported). Self-reported EF are most likely to have a partially overlapping relationship with self-reported SRL. In contrast, task-based EF are more likely to support self-reported SRL in achieving study success, thus showing a mediating role.
Studies about EF, SRL, and study success are scarce, particularly in young adult students. In this population, we identified only the study by Musso et al. (2019), who investigated the coherence between EF, SRL, and study success in a group of first-year university students. They found mediating effects of EF via SRL on math performance. Musso et al. (2019) measured EF directly (i.e., measured with neuropsychological tasks) and focused solely on working memory and executive attention.
To our knowledge, no study has focused on self-reported EF and SRL together as predictors of study success in higher education. There are a few studies that have investigated the relationship between self-reported EF and study success in the context of higher education. These studies indicate that self-reported EF problems negatively affect study success (e.g., Knouse et al., 2014; Baars et al., 2015; Ramos-Galarza et al., 2020). On the other hand, numerous studies have shown a positive relationship between SRL and study success in higher education (e.g., Honicke and Broadbent, 2016; Virtanen et al., 2017; Sun et al., 2018). The added value of EF in conjunction with SRL and their explanatory value for study success still needs to be determined to investigate if the concepts combined have the potential power to improve study success. Therefore, this study investigates the following research question:
Do self-reported EF and SRL combined explain variations in study success among higher education students better than either separately? Notably, since there appears to be a reciprocal non-mediating relationship between self-reported EF and self-reported SRL, we assume two independent variables that can directly or combined affect study success.
Following the research model depicted in Figure 1, we will investigate (1) the relationships between EF and SRL (measured 2 months after the start of the academic year), and study success (measured at the end of the academic year, and (2) the combined effect of EF and SRL on study success.
We hypothesize that EF and SRL combined explain statistically significantly more variance in the number of credits obtained at the end of the academic year than each construct separately.
In addition, the aim is not to examine the different dimensions of EF and SRL and their relationships. Given the inter- and intra-individual differences due to the developmental trajectory of both EF and SRL, we expect these specific dimensions to have little expressive power when looking at individual students. We expect the results to provide insight into the group. However, this picture may differ if students have been developing for 6 months or if a different group of students is involved. Therefore, we explore the concepts of EF and SRL without identifying the specific dimensions.
The COVID-19 pandemic made studying and life more challenging for students due to lockdowns and regulations (e.g., Copeland et al., 2021; Ihm et al., 2021). During this time, students may have faced a constant stream of new and complex issues, which could have impacted their EF. While not the main focus of our research, we also wanted to understand how these circumstances affected students’ self-reported EF and SRL. As such, we asked students if the lockdowns and regulations influenced how they completed our questionnaire.
2 Materials and methods
2.1 Procedures
This study was conducted following a retrospective cohort design (Ato et al., 2013). EF and SRL data for this longitudinal survey study were collected from the last week of November 2020 through the first week of December 2020 during the first-year module “Diagnostic Research Part 1 (DR1).” At the end of the academic year in July 2021, we collected the obtained credits. One of the main objectives of module DR1, is learning to conduct research. In that context, the students fill out various questionnaires to experience what participating in research entails.
The study measurements were integrated into the educational program so students could complete the online questionnaires during a lesson. All the students received their results and feedback regarding their test performances. As a follow-up, students were offered to discuss their results with the researcher, lecturer, or mentor.
In the first week of the module, the students were informed about the study aim and procedure during an online lecture. They were told that completing the questionnaires would take approximately 45–60 min, that participation was voluntary and confidential, and that no credits were involved. According to institutional ethical advice committee (SEAC) guidelines, informed consent was drawn and provided for signature at the start of the procedure. All students were invited to participate, but we only used the results of students who signed the informed consent for analyses.
During class in the second week of the module, students completed the questionnaires on first EF (Behavior Rating Inventory of Executive Function – Adult version) then SRL (Motivated Strategies for Learning Questionnaire), then descriptive questions, such as the COVID-19 control question. The completion of the questionnaires would take students approximately 30–60 min.
The credits earned at the end of the school year were retrieved from the school’s database and could be up to 60 credits.
2.2 Participants
This study included all first-year students of the program Applied Psychology of the University of Applied Sciences in the Netherlands. The inclusion criteria were first-year higher education students between 18 and 25 years, assuming that around 25, the prefrontal cortex is mature, and the EF are optimally developed (Giedd and Rapoport, 2010). We are particularly interested in first-year students because the transition from high school to higher education impacts this group because they must learn new ways of learning and personal changes, such as living independently (Lowe and Cook, 2003; Carragher and McCaughey, 2022). We excluded student younger or older than 25 years.
A total of 484 first-year students participated in module DR1 and completed the questionnaires. Of them, 444 signed the informed consent. We excluded 129 students for various reasons (Table 1). The final sample contained 315 first-year higher education students. Table 2 includes the descriptive data.
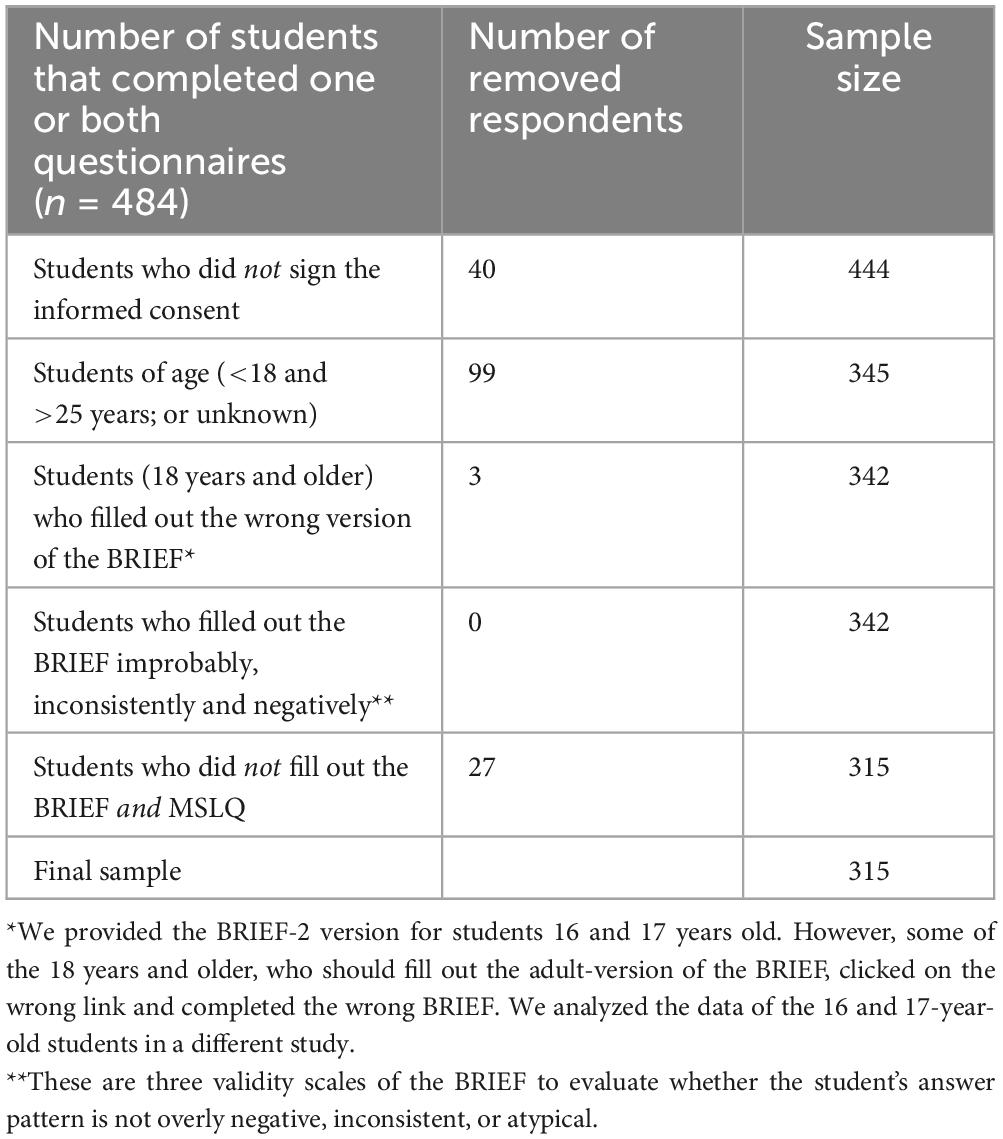
Table 1. Overview of the selection process of the final sample size for the structural equation modeling analyses.
2.3 Measures
2.3.1 Study success
Study success was measured by retrieving the number of credits earned after the first school year (including two semesters) from the university’s database.
In addition, we used two self-report measures, namely for EF and SRL, which we discuss further below.
2.3.2 Behavior Rating Inventory of Executive Function – Adult version
The BRIEF-A is a self-report questionnaire to describe EF based on behaviors of adults (18–90 years) (Roth et al., 2005, 2013). This instrument has been standardized for the executive functioning of adults in everyday environments, and specifically in the Netherlands for adults aged 18–65 years (Scholte and Noens, 2011).
The BRIEF-A includes nine non-overlapping and empirical-based scales (Table 3). The nine scales are measured by 75 items about perceived EF deficits over the past month on a three-point scale (1 = never; 2 = sometimes; 3 = often). The higher the score on specific behaviors, the higher the level of perceived EF deficits.
The total raw scores of the subscales can be transformed into T-scores, making it possible to compare them with a representative norm group. A T-score of 65 or greater indicates “clinical” problems with a specific EF or a cluster of EF (the total or index scores) (Roth et al., 2005; Scholte and Noens, 2011). However, Schwartz et al. (2020) and Abeare et al. (2021) demonstrated that in some cases, particularly in clinical samples, a cut-off T-score of ≥80 or ≥90 demonstrates higher specificity and is a more realistic representation.
There is always a percentage of the participants unwilling or unable to complete the questionnaires credibly. Therefore, we should be aware of the symptom validity, i.e., the extent to which scores on self-reports reflect true levels of emotional distress (Larrabee, 2012). In particular, young adults (i.e., students) cannot always realistically assess their EF deficits (Toplak et al., 2013), which is demonstrated by the lack of relationship between both subjectively and objectively measured cognitive abilities, with other psychological factors believed to play a role, such as depressive symptoms (Toplak et al., 2013). Therefore, it is recommended to control for this through symptom validity testing. The BRIEF-A contains three validity scales to measure three aspects of non-credible responding (negativity, inconsistency, and infrequency) (Roth et al., 2005; Scholte and Noens, 2011).
We calculated the internal consistency of the BRIEF-A and its component subscales. The analysis yielded acceptable internal consistency reliability (Table 3).
2.3.3 Motivated Strategies for Learning Questionnaire
The MSLQ assesses SRL (Duncan and McKeachie, 2015). It is a self-report questionnaire designed to assess students’ motivational orientations and the use of different learning strategies. Self-reports have proven reliable and valid instruments for gaining general insight into students’ SRL (Rovers et al., 2019).
The questionnaire consists of a motivational and a learning strategies scale, consisting of respectively six and nine subscales (Table 4).
The subscales of the MSLQ demonstrate moderate to good internal consistency reliability, except for Peer learning, which was poor (Table 4). This may be because this scale contains the least number of items, namely three items, or that it is a different population than the one with which the MSLQ was validated.
2.3.4 Impact of COVID-19
To gain insights into the impact of the COVID-19 pandemic, we asked the students if the lockdowns and regulations influenced how they filled out the questionnaire. Response options were as follows: 1 = I am more negative/I experience more problems; 2 = I do not act differently than before COVID-19; 3 = I am more optimistic/I notice challenges.
2.4 Statistical analyses
Pearson’s correlation coefficients are calculated to explore the relationships between the BRIEF-A and MSLQ subscale scores. According to Cohen (1992), <0.3 means a weak correlation, 0.3–0.5 is a moderate correlation, and 0.5 or higher is a strong correlation effect.
To test our hypothesis, structural equation modeling (SEM) was conducted. SEM is a statistical method that uses various models to test hypothesized relationships among observed variables (Schumacker and Lomax, 2015). The following standard model fit indices were used: Chi-square-test (χ2), standardized root mean residual (SRMR) confirmatory fit index (CFI), and root mean square error of approximation (RMSEA).
A non-significant χ2 test is considered as good. In contrast, a large and significant χ2 test indicates a big discrepancy and, thus, a poor fit between the model and original data (Hu and Bentler, 1999). Because the χ2 becomes increasingly unreliable when the sample size is more significant than 250 (Byrne, 2006), the statistic χ2 divided by its degrees of freedom (df) is used (Bollen, 1989), where a ratio >2.00 represents an inadequate fit (Byrne, 2006).
A value less than 0.08 is considered a good fit for the SRMR, an absolute measure indicating zero as a perfect fit. The SRMR has no penalty for model complexity (Hu and Bentler, 1999). CFI values >0.90 and 0.95 indicate acceptable and excellent fit, respectively, and RMSEA values <0.06 and <0.08 indicate a good to acceptable fit (Hu and Bentler, 1999).
A rule of thumb of confirmatory factor analyses (CFA)/SEM is often a ratio of cases to free parameters, or N:p, namely at least 10:1 to 20:1 (e.g., Schumacker and Lomax, 2015; Kline, 2016). In our study, we sufficiently achieve the minimum ratio of 10:1 with our sample of 315 cases and 27 variables.
The first step of SEM involves specifying a set of latent variables and their relations. We tested the constructs (EF and SRL) with CFA, using maximum likelihood (ML) estimation, with AMOS 26.0, to identify the measurement model for study success. This resulted in a model with low CFI (0.64) and high multicollinearity.
Therefore, we conducted an exploratory factor analysis (EFA) per theoretical construct. As a result, the latent variables were established, and the construct –“Peer learning”- was removed due to low reliability (α = 0.50), inadequate convergent validity (factor loadings were 0.27, 0.44, and 0.87) and the fact that the construct loads with Help-Seeking (for an overview of the included items, see Supplementary Tables 1, 3, 4). Also before and after the EFA some of the other constructs had a suboptimal reliability (e.g., Self-Monitor and Anger Outbursts). Although a Cronbach’s alpha of minimally 0.7 is preferable, a value of 0.6 is acceptable (Hajjar, 2018). The observable measures for the latent variables were partially adjusted (for an overview of the removed items, see Supplementary Tables 2–4).
Again, a CFA was conducted, showing a reasonable amount of multicollinearity, but the model fit measures are between acceptable margins (χ2/df = 1.55; SRMR = 0.06; RMSEA = 0.04; CFI = 0.85).
The second step comprises creating a SEM, including all the defined latent variables to test our hypothesis. This Model 1 combined all the variables to test how EF and SRL would explain the total variance in study success. Again, we expected that not all variables would be (equally) significantly correlated and contribute to the variance of study success.
Additionally, two models were created and tested to establish the contribution of SRL and EF separately to gain insights into the differences between the concepts separately and combined. Model 2 comprised all the SRL latent variables, and Model 3 the EF latent variables. A Chi-square difference test statistic was used to measure the differences between the models (where p < 0.05 means a significant difference).
3 Results
The study was conducted as planned with no significant details in implementation. The results will answer the research question of the combined value of EF added to SRL to better understand the differences in study success. We hypothesize that EF and SRL combined explain statistically significantly more variance in the number of credits obtained at the end of the academic year than each construct separately.
First, we provide descriptive data, then discuss the correlations between the different constructs (EF and SS, SRL and SS, and EF and SRL) and finally, test the hypothesis.
3.1 Descriptive statistics
The students’ total BRIEF-A raw scores ranged between 75 and 178. The average was 118.01 (SD = 19.10). Their average normative scores fell in the range of “normal or average” level of perceived EF deficits (T-score between 30 and 59) to “clinical (T-scores 65 and higher).” Approximately 17% of the student population was in the subclinical range (defined as a T-score of 60–64), whereas 20% of the students perceived EF deficits in the clinical range (T-score ≥65). It is expected that for some EF scales a cut-off score of T ≥ 80 may be more valid, for instance, “working memory” (Abeare et al., 2021). So, the percentage of students that realistically report “clinical” EF deficits, might be lower than 20%.
The MSLQ has no specific cut-off scores. The average total score of motivated strategies of students was 5.06 (SD = 0.57) on a scale of 1–7. The average total score of learning strategies was 4.49 (SD = 0.65) on a scale of 1–7.
Finally, the obtained credits after one school year ranged from 0 to 60, with 60 points being the maximum possible. The average was 49.03 (SD = 15.54).
3.2 The correlations between executive functions, self-regulated learning, and study success
We conducted correlation analyses to examine the relationships between EF-SRL, EF-study success, and SRL-study success. Table 5 shows the relationships between EF and SRL. We found weak correlations (range r = −0.23 to r = −0.26) among the composite scores of the self-reported measures: between EF behavioral and metacognitive indices and SRL motivational beliefs index, and the EF indices and SRL learning strategies index.
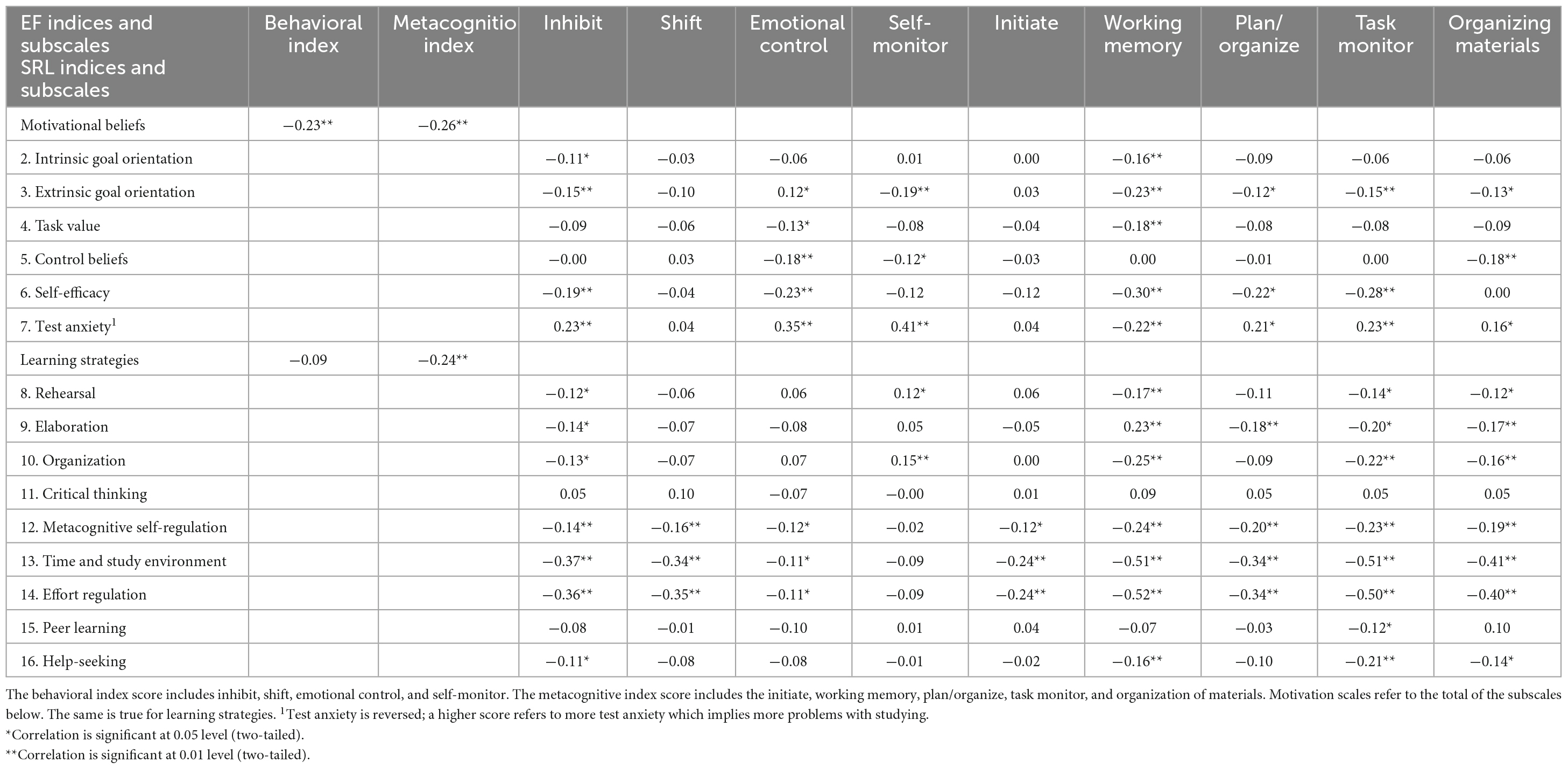
Table 5. Pearson product-moment-correlations between self-regulated learning and executive functions.
At the level of subscales, there are many weak to strong negative correlations between SRL subscales and EF subscales. The directions of the significant correlations indicate that students who report more EF problems also report using fewer SRL strategies.
Table 6 describes the correlations between EF and study success. We found weak but significant correlations between study success and six EF subscales (range r = −0.12 to r = −0.24). These (weak) correlations suggest that an increase in EF problems is associated with less study success.
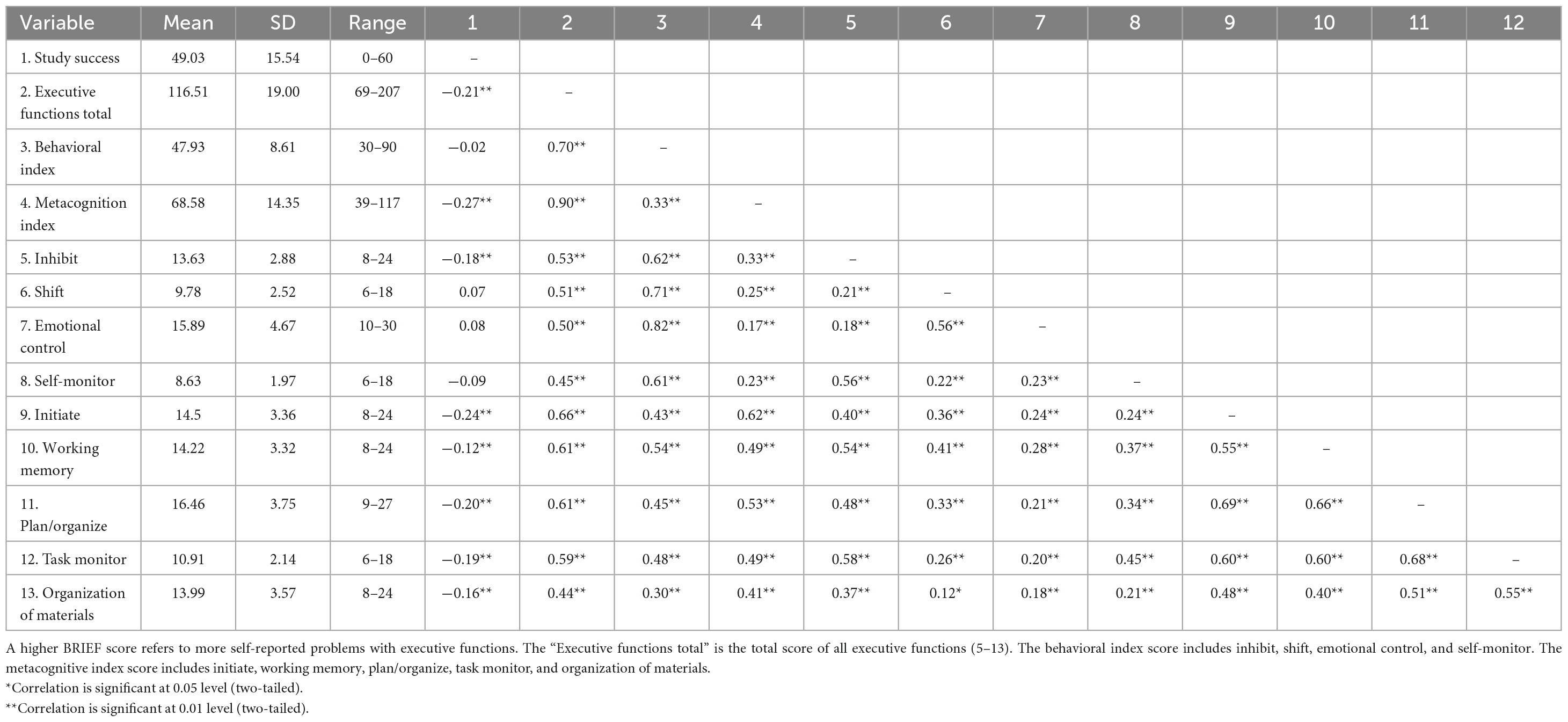
Table 6. Pearson product-moment-correlations between executive functions and executive functions and study success (obtained credits).
Table 7 describes the correlations between SRL and study success. The correlation analysis between SRL and study success resulted in weak significant correlations between study success and six SRL subscales (range r = −0.11 to r = 0.21). Overall, these findings suggest that an increase in applying SRL is associated with more study success.
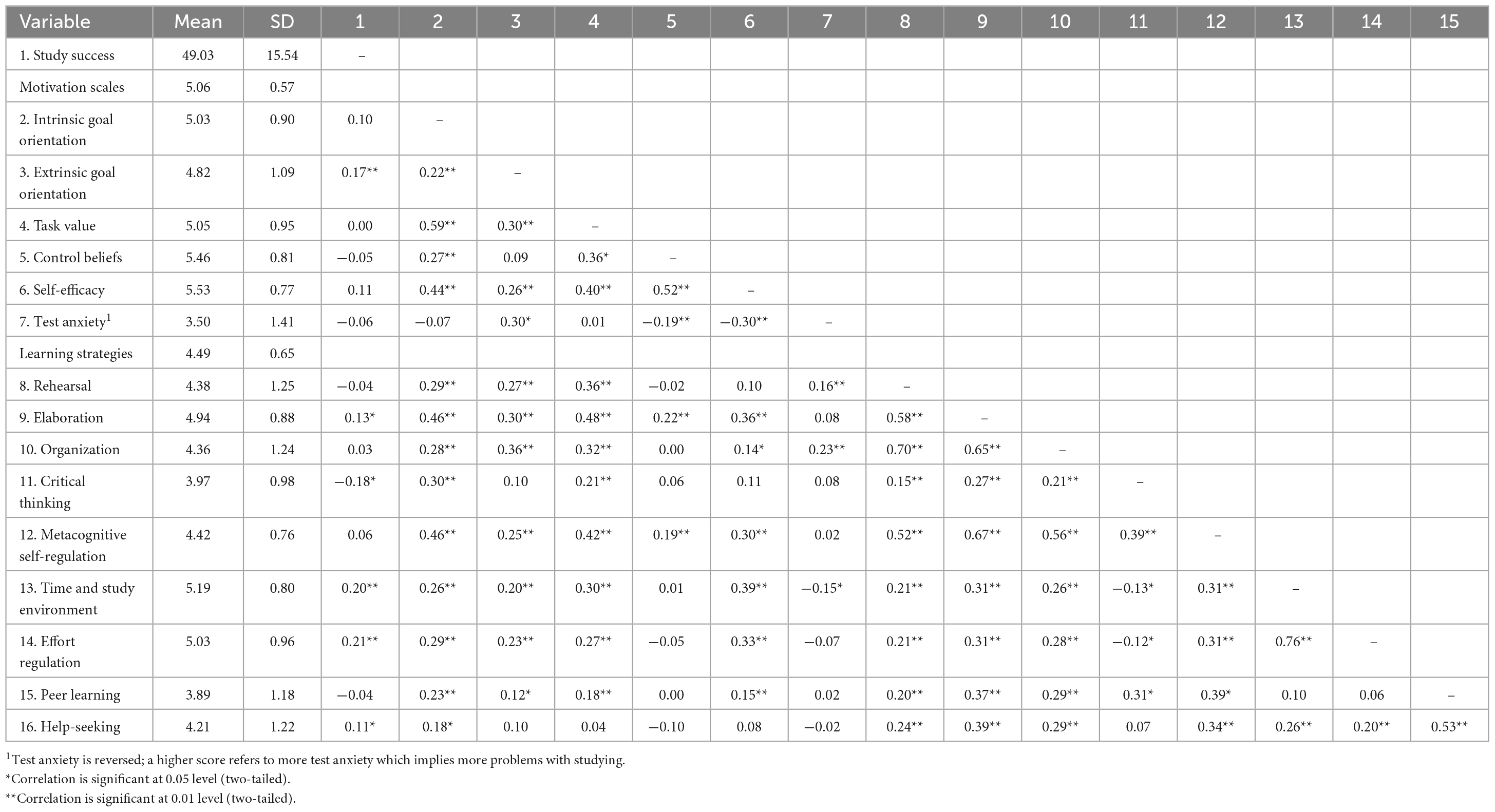
Table 7. Pearson product-moment-correlations between self-regulated learning, and self-regulated learning and study success (obtained credits).
To sum up, we found significant correlations between all the constructs.
3.3 Hypothesis test of the explanatory model of variances in study success
To test our hypothesis, we evaluated the model fit through SEM after performing confirmatory and exploratory analyses. The model shown in Figure 2 was tested.
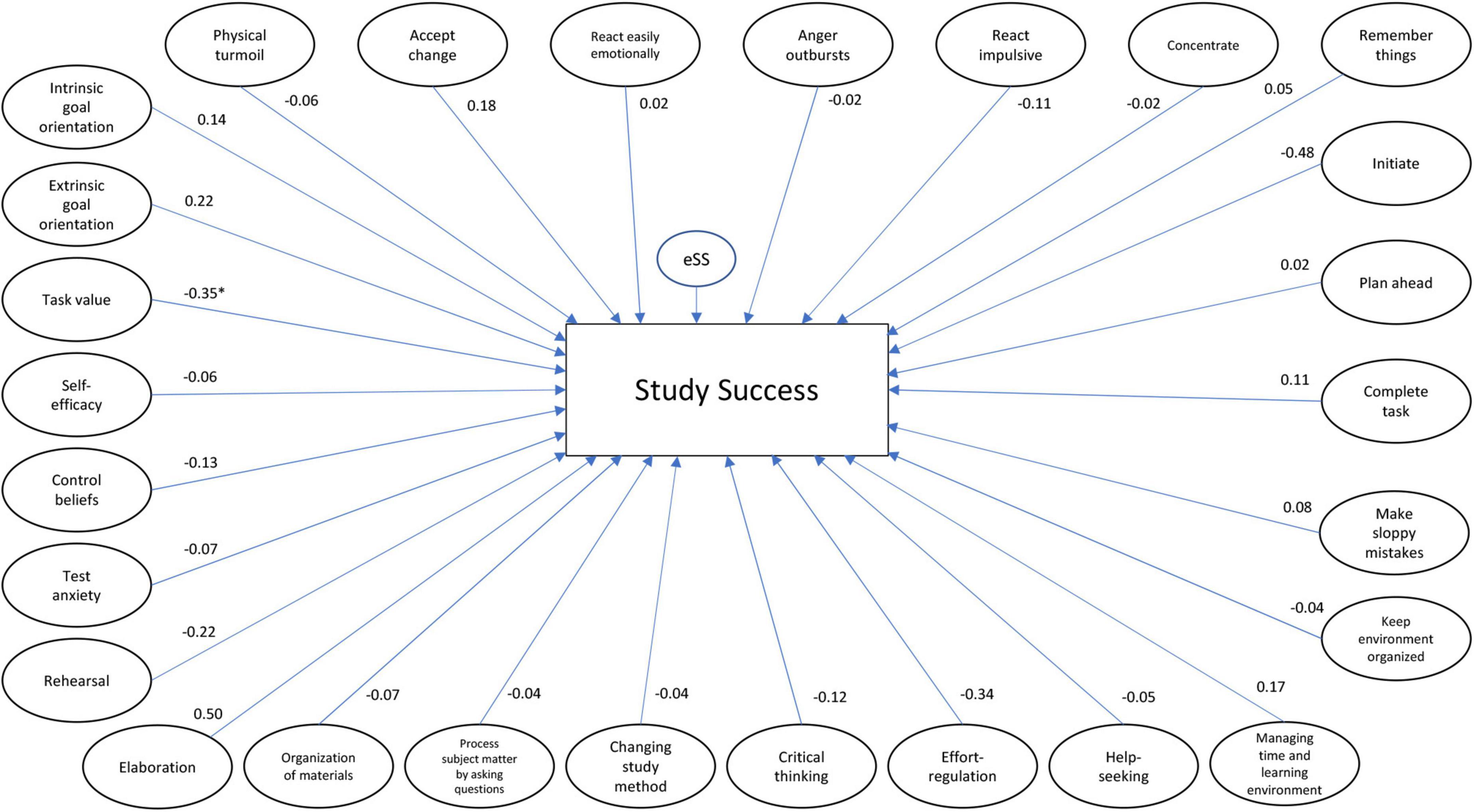
Figure 2. Total model, including all the latent variables of EF (starting above left “physical turmoil to keep environment organized”) and SRL variables, standardized betas, and p-values. *p < 0.05.
The total model without restrictions fit the data well according the Hu and Bentler (1999) thresholds (χ2/df = 1.53; SRMR = 0.06; RMSEA = 0.04; CFI = 0.84), except for the CFI.
The CFI does not reach the preferred threshold of a minimal 0.90, meaning that the hypothesized model may not fit the observed data as well as is preferred (Van Laar and Braeken, 2021). Nevertheless, a model with a CFI value below 0.90 can be interpreted if the other measures meet the stated requirements – which is the case (Marsh et al., 2004). Another consideration is that the CFI might have dropped because our model is large and complex (Pat-El et al., 2013), and Hu and Bentler’s cut-off values may be too stringent in these cases (Marsh et al., 2004, 2005). Specifically, Marsh (2007, p. 785) states that “It is almost impossible to get an acceptable fit (e.g., CFI, TLI > 0.9; RMSEA < 0.05) for even ‘good’ multifactor rating instruments when analyses are done at the item level and there are multiple factors (e.g., 5–10), each measured with a reasonable number of items (e.g., at least 5–10/per scale) so that there are at least 50 items overall” which is the case in our study. Therefore, we continued testing the model, demonstrating that the total model explains 40.1% of the variance in obtained credits (Table 8).
To explore if the combination of EF and SRL explains the variance in the number of credits better than EF and SRL separately, Models 2 and 3 were tested (respectively, Figures 3, 4). The model with the EF variables provided a good fit to the data (χ2/df = 1.47; SRMR = 0.06; RMSEA = 0.04; CFI = 0.93), explaining 19.8% of the variance in obtained credits. The model with the SRL variables explained 22.9% of the variance in obtained credits and had a worse fit than the model of the EF, but can be considered a sufficient fit to the data (χ2/df = 1.88; SRMR = 0.07; RMSEA = 0.05; CFI = 0.85), again except the CFI threshold. Chi-square difference tests showed that both the EF and SRL models differ significantly from the total model (respectively EF model: χ2diff = 5,439.29; df = 3,535; p < 0.001 and SRL model: χ2diff = 3,965.71; df = 2,643; p < 0.001), indicating that the combination of EF and SRL better explains the variance in obtained credits than EF and SRL separately.
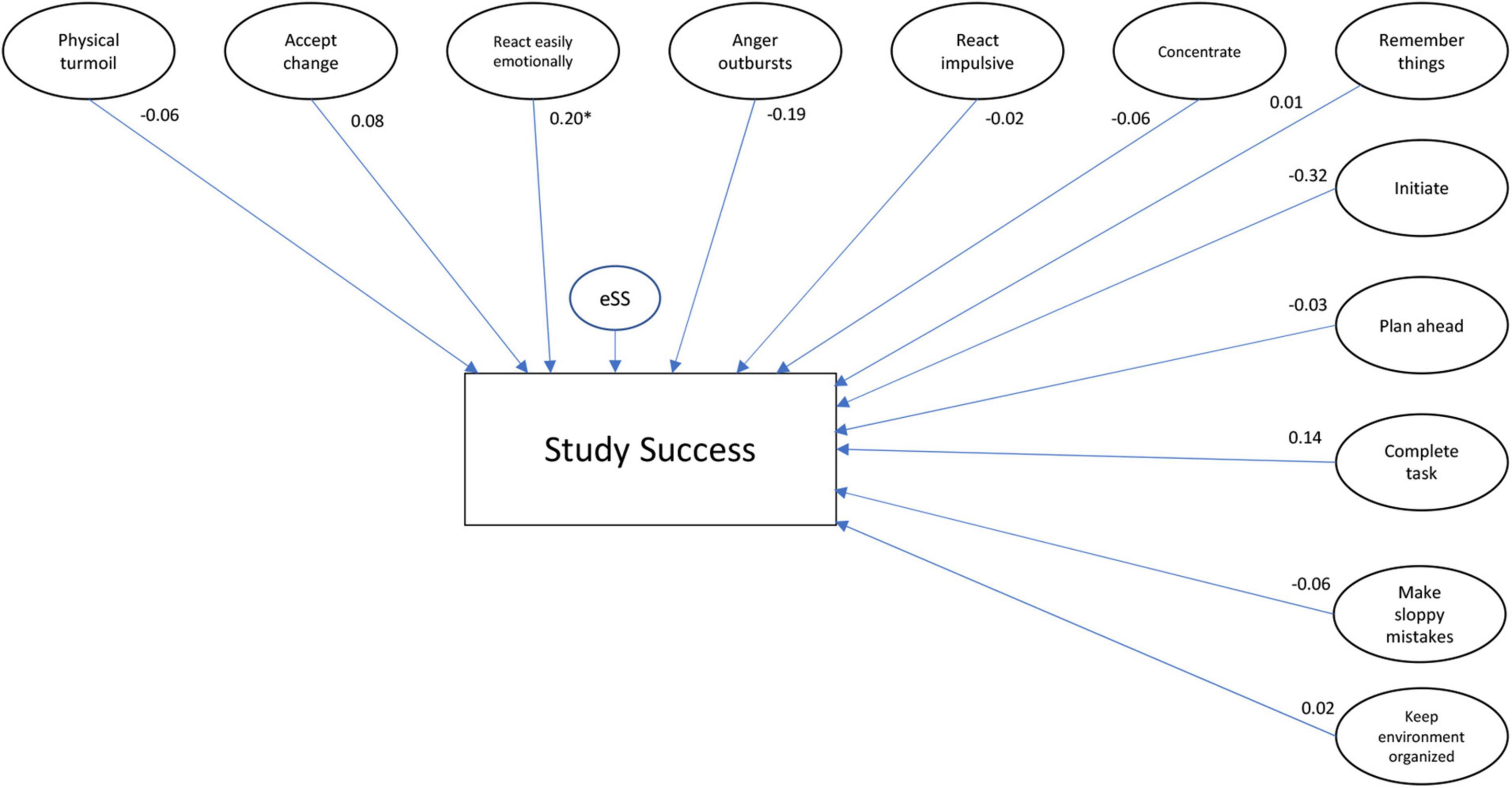
Figure 3. (Self-reported) EF model, including all the latent variables of EF, standardized betas, and p-values. *p < 0.05.
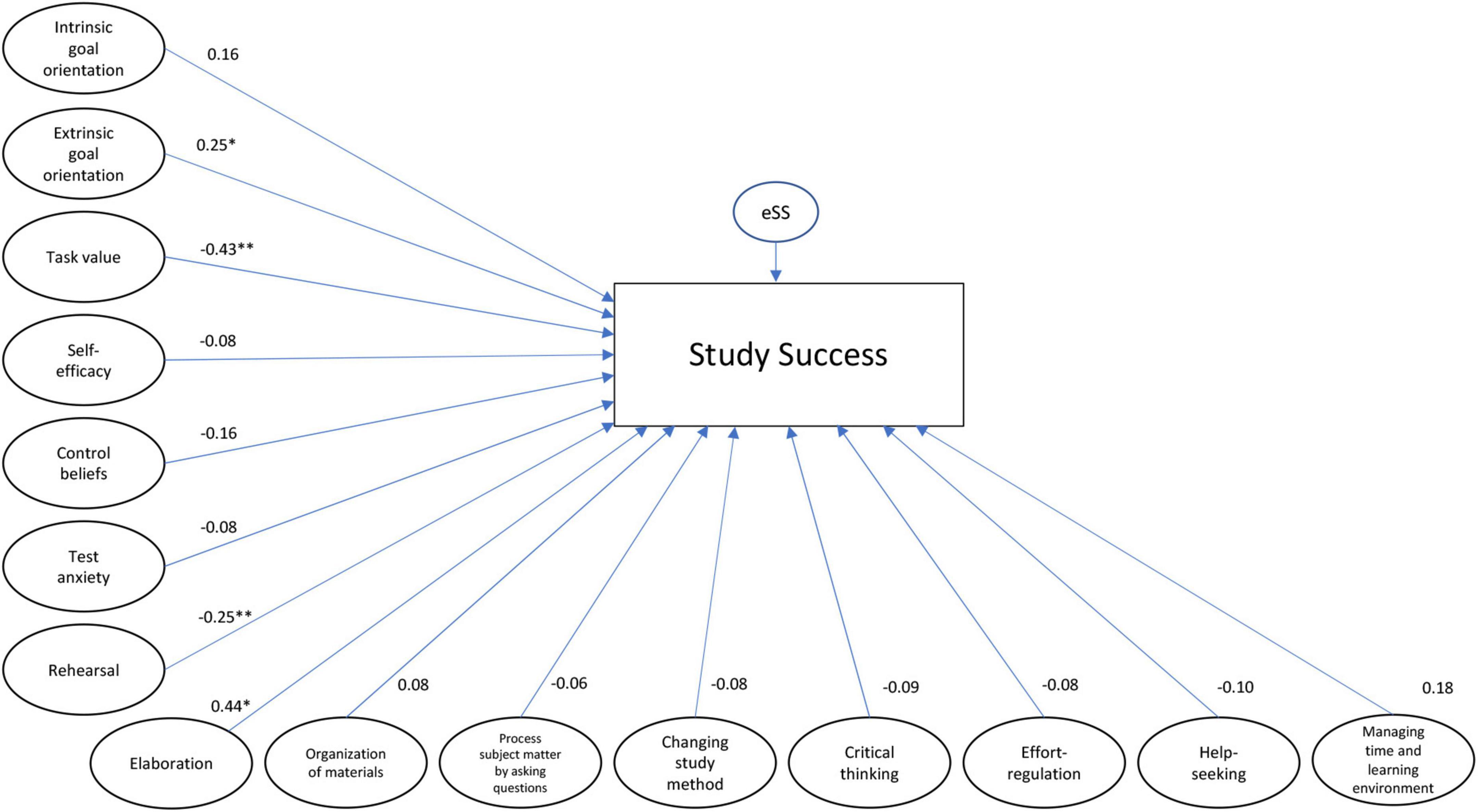
Figure 4. (Self-reported) SRL model, including all the latent variables of SRL, standardized betas, and p-values. *p < 0.05; **p < 0.01.
In conclusion, the total model explains the most variance (39.8%) in the obtained credits.
3.4 Impact of the COVID-19 pandemic on executive functions and self-regulated learning
We used Pearson’s correlation method to calculate the mean score on the question assessing the impact of the COVID-19 pandemic (M = 1.74; SD = 0.78) with the index scores of the BRIEF-A (EF) and MSLQ (SRL). The data showed weak, significant correlations between the answer to this question and EF metacognition (r = −0.21; p < 0.001), SRL motivational strategies (r = 0.18; p = 0.001), and SRL learning strategies (r = 0.15; p = 0.007). These results imply that the more the COVID period has led students to have a more pessimistic attitude toward their study process, the more EF metacognition problems were reported, and the fewer SRL strategies were used.
4 Discussion
This study sought to investigate the added value of including EFs and SRL in predicting study success after one academic year among higher education students. We explored (1) the relationship between EF and SRL the relationship between EF and study success and SRL and study success, and we hypothesized that (2) the combination of self-reported EF and SRL would explain differences in study success better than separately. First, Regarding the relationships between the constructs our findings show that EF and SRL are correlated. This is consistent with previous studies demonstrating associations between EF and SRL among high school students (Effeney et al., 2013; Rutherford et al., 2018) and university students (Garner, 2009; Follmer and Sperling, 2016). The weak to moderate correlations between EF and SRL makes it clear that these concepts partially overlap but are not the same (Garner, 2009). In addition, EF and SRL both correlate – partially and weakly – with study success, indicating a trend of more EF problems or less SRL comes with fewer credits earned after one school year, in line with studies such as those by Baars et al. (2015) and Ramos-Galarza et al. (2020) for EF, and Li et al. (2018) and Moghadari-Koosha et al. (2020) for SRL.
Second, to better understand the influence of EF and SRL combined on the differences in study success, we compared the imposed models separately and combined. In line with our hypothesis, EF and SRL combined explained the variance in study success after one academic year better than EF and SRL separately. This indicates that a student who performs poorly on EF will likely demonstrate less effective SRL and likely have less study success. Similar results were found by Musso et al. (2019), although they used task-based EF measurements, whereas we used self-reported EF. Even though more research is needed, Musso’s and our findings indicate that combining EF and SRL is vital for the learning processes. A student with more developed EF strategies can reflect on, choose from, or integrate rules where appropriate (Moran and Gardner, 2018, pp. 29–56) and thus be better able to self-regulate their learning and achieve more success.
The current study has some important strengths, such as the empirical confirmation of the need for integration of two theoretical models relevant to education and study success, with the use of proven valid and reliable instruments and the use of SEM to test the models while better accounting for measurement error (Tomarken and Waller, 2005).
On the other hand, this study has a few limitations. First, a non-probabilistic sample was used, namely students of Applied Psychology, which limits the generalization of the results to other groups of students or young adults. Future research could include students from different studies and levels as a more representative sample of young adult learners.
Second, self-reporting measurements were used, which have apparent advantages, such as surveying a large population without much effort and high ecological validity (Barkley and Fischer, 2011). However, a known pitfall with self-reporting is that students may (un)consciously fill out the questionnaires differently than they would show in observed behavior (e.g., McDonald, 2008; Demetriou et al., 2015).
Particularly, self-reports of cognitive abilities are sensitive to response bias and psychological factors, such as depression, anxiety, or chronic pain. For instance, Schwartz et al. (2020) found that self-reporting EF with the BRIEF-A probably measured emotional distress over executive dysfunction. However, they argued that this could be the case in specific samples such as theirs, namely middle-aged veterans who all showed intact EF and experienced heightened psychiatric distress. Abeare et al. (2021) demonstrated inconsistent results to the conclusion of Schwartz et al. (2020), suggesting a more plausible explanation that “non-credible presentation manifests as extreme levels of symptoms on the BRIEF-A-SR- and self-report inventories in general” (Abeare et al., 2021, p. 9). Additionally, a reasonable number of studies have shown that the BRIEF-A can validly measure EF in various target groups such as deaf and hearing students (Hauser et al., 2013), students and procrastination (Rabin et al., 2011), and depression within students (Mohammadnia et al., 2022).
Nevertheless, both Schwartz et al. (2020) and Abeare et al. (2021) suggest that on the validity scale “negativity” a cut-off score of 4 (instead of ≥6) is more representative (i.e., essentially a frequency count of the extreme self-ratings on 10 items of the BRIEF-A). In our study, this would imply that 10 more students should have been disregarded as outliers. However, considering this small number of students, we do not expect different outcomes. To gain insight into the impact of assessment mode on outcomes, research is needed that includes both self-report measures of EF and SRL and objective measures, such as neuropsychological tests for EF or learning analytics for SRL (Yamada et al., 2017). Additionally, measurement instruments that can support screening for non-credible symptom reports can be used (Abeare et al., 2021), such as the MMPI-2 (Schwartz et al., 2020).
Subsequently, a CFI (just) below 0.9 indicates a reasonable but not good fit of the model with the dependent variable. That is, as argued, if the CFI scale is considered as a continuum and not, as is often incorrect, as a dichotomous scale. If our model had had a higher CFI, it would be easier to make statements about the relationship between the variables in the model and the outcome measure. However, this does not alter the fact that the correlations between the various SRL scales and the EF scales with study success have been reliably established. The lower CFI mainly concerns correlations between these (sub)scales, making it more difficult to see what their unique contribution is to study success. Further research will be required to investigate this further.
A final limitation might have been that this study was conducted during the COVID-19 pandemic. Research demonstrates that the lockdowns and other restrictive regulations impacted students’ lives considerably (e.g., Copeland et al., 2021; Ihm et al., 2021), and therefore we investigated the self-reported impact of COVID on how students completed the questionnaires. We found that this period negatively related to how students experience their EF and SRL. This was especially true for students reporting severe EF problems, which implies that the results might be colored because assessments were conducted during the pandemic. Appelhans et al. (2021) found a similar result: young adults with preexisting EF deficits have shown increased unhealthy behavior since COVID-19. We think this period especially challenged students’ EF because of the constant flow of new and complex issues they encountered. Nevertheless, although students’ response patterns might have deviated a bit due to COVID-19, we think that, in light of previous research, patterns would have been the same when assessed in regular times. However, it might be valuable to repeat the study in non-pandemic times.
Future research could further explore how EF and SRL impact study success in theory and practice. One aspect is that a large part of the variance in study success is still unaccounted for, and future research could focus on finding additional answers, for example, in personal and contextual regulatory factors, such as studied by De la Fuente et al. (2022) and Pachón-Basallo et al. (2022).
Another aspect is that EF and SRL have different yet complementary conceptual lenses on how students learn and achieve success [such as Dinsmore et al. (2008) suggest for metacognition and SRL]. Although our study is not about the conceptual lens of EF and SRL, further research into how we can learn from both ways of looking at things to understand student study behavior is desirable since the results confirm that, taken together, they better predict study success even though they do not measure the same thing. The findings of this study can be used to motivate improving learning environments in higher education. Since EF and SRL combined better explain the differences in study success, it makes sense to look at the available EF tools to expand the educational professional’s toolbox beyond the already available SRL tools (e.g., Theobald, 2021). Providing education of EF in addition to SRL probably increases the levels of success in students. Furthermore, metacognitive knowledge about SRL and EF leads to more motivation to use the learned strategies correctly (e.g., Veenman, 2011; Follmer, 2021). Additionally, in the design of (blended) learning environments, educational professionals can build a certain degree of adaptivity when considering different levels of students’ EF, knowing that individual differences are significant. For example, regarding problems with task initiation, one can think of a lesson structure with more intermediate moments during which a student can ask a supervisor for help, more formative tests, or additional (warm-up) assignments in a module that support and encourage the start-up. This way of working is not new and also falls under the intersection of educational science, psychology, and neuroscience, also called neuroeducation (e.g., Jolles and Jolles, 2021; Willingham, 2023).
5 Conclusion
In conclusion, this study highlights that while EF and SRL cohere and are related to study success, they do not measure the same. This is also reflected in that each separately explains less variance in study success than taken together. Nonetheless, combined they provide more information about how student achieve study success. Generally, a student reporting EF deficits will likely report less effective SRL and achieve less study success in the long-term. This suggests that attention to EF alongside SRL in education is justified and valuable. Future theoretical research on both the working mechanisms of EF and SRL is needed, as well as the more practical application of the knowledge associated with EF and SRL.
Data availability statement
The raw data supporting the conclusions of this article will be made available by the authors, without undue reservation.
Ethics statement
The studies involving humans were approved by Ellis van Dooren-Wissebom, chairperson of the LLM Saxion Ethical Advice Committee (SEAC). The studies were conducted in accordance with the local legislation and institutional requirements. Written informed consent for participation in this study was provided by the participants.
Author contributions
DM secured funding for the study, conceptualized the study, oversaw, and conducted the data collection, analyzed the data, and led the writing of the manuscript. MS-D managed the data collection in SPSS and contributed to drafting the method and result sections of the manuscript. JD supervised the design, data collection and analyses, and manuscript writing. JF supervised the writing of the manuscript. AJ provided feedback on the latest versions of the article. All authors contributed to the article and approved the submitted version.
Funding
This study was supported by a grant from Saxion University of Applied Sciences.
Conflict of interest
The authors declare that the research was conducted in the absence of any commercial or financial relationships that could be construed as a potential conflict of interest.
Publisher’s note
All claims expressed in this article are solely those of the authors and do not necessarily represent those of their affiliated organizations, or those of the publisher, the editors and the reviewers. Any product that may be evaluated in this article, or claim that may be made by its manufacturer, is not guaranteed or endorsed by the publisher.
Supplementary material
The Supplementary Material for this article can be found online at: https://www.frontiersin.org/articles/10.3389/fpsyg.2023.1229518/full#supplementary-material
References
Abeare, K., Razvi, P., Sirianni, C. D., Giromini, L., Holcomb, M., Cutler, L., et al. (2021). Introducing alternative validity cutoffs to improve the detection of non-credible symptom report on the BRIEF. Psychol. Inj. Law 14, 2–16. doi: 10.1007/s12207-021-09402-4
Appelhans, B. M., Thomas, A. S., Roisman, G. I., Booth-LaForce, C., and Bleil, M. E. (2021). Preexisting executive function deficits and change in health behaviors during the COVID-19 pandemic. Int. J. Behav. Med. 28, 813–819.
Ato, M., López, J. J., and Benavente, A. (2013). A classification system for research designs in psychology. Anal. Psicol. 29, 1038–1059. doi: 10.6018/analesps.29.3.178511
Baars, M. A., Nije Bijvank, M., Tonnaer, G. H., and Jolles, J. (2015). Self-report measures of executive functioning are a determinant of academic performance in first-year students at a university of applied sciences. Front. Psychol. 6:1131. doi: 10.3389/fpsyg.2015.01131
Baggetta, P., and Alexander, P. A. (2016). Conceptualization and operationalization of executive function. Mind Brain Educ. 10, 10–33. doi: 10.1111/mbe.12100
Barkley, R. A. (2012). Executive functions. What they are, how they work, and why they evolved. New York, NY: Guilford Press.
Barkley, R. A., and Fischer, M. (2011). Predicting impairment in major life activities and occupational functioning in hyperactive children as adults: Self-reported executive function (EF) deficits versus EF tests. Dev. Neuropsychol. 36, 137–161. doi: 10.1080/87565641.2010.549877
Bollen, K. A. (1989). Structural equation models with latent variables. New York, NY: Wiley Online Library, doi: 10.1002/9781118619179
Broadbent, J., and Poon, W. L. (2015). Self-regulated learning strategies and academic achievement in online higher education learning environments: A systematic review. Internet High. Educ. 27, 1–13. doi: 10.1016/j.iheduc.2015.04.007
Byrne, B. M. (2006). Structural equation modeling with EQS and EQS/Windows: Basic concepts, application and programming. Mahwah, NJ: Lawrence Erlbaum Associates.
Carragher, J., and McCaughey, J. (2022). The effectiveness of peer mentoring in promoting a positive transition to higher education for first-year undergraduate students: A mixed methods systematic review protocol. Syst. Rev. 5:68. doi: 10.1186/s13643-016-0245
Copeland, W. E., McGinnis, E., Bai, Y., Adams, Z., Nardone, H., Devadanam, V., et al. (2021). Impact of COVID-19 pandemic on college student mental health and wellness. J. Am. Acad. Child Adolesc. Psychiatry 60, 134–141. doi: 10.1016/j.jaac.2020.08.466
De la Fuente, J., Martinez-Vicente, J. M., Pachón-Basallo, M., Peralta-Sánchez, F. J., Vera-Martinez, M. M., and Andrés-Romero, M. P. (2022). Differential predictive effect of self-regulation beahvior and the combination of self- vs. external regulation behavior on executive dysfunctions and emotion regulation difficulties, in university students. Front. Psychol. 13:876292. doi: 10.3389/fpsyg.2022.876292
Demetriou, C., Ozer, B. U., and Essau, C. A. (2015). “Self-report questionnaires,” in The encyclopedia of clinical psychology, 1st Edn, eds R. Cautin and S. Lilienfeld (New York, NY: Wiley Online Library), doi: 10.1002/9781118625392.wbecp507
Denckla, M. B., and Mahone, E. M. (2018). “Executive function. Binding together the definitions of attention-deficit/hyperactivity disorder and learning disabilities,” in Executive function in education. From theory to practice, 2nd Edn, ed. L. Meltzer (New York, NY: The Guilford Press), 5–24.
Dent, A. L., and Koenka, C. (2016). The relation between self-regulated learning and academic achievement across childhood and adolescence: A meta-analysis. Educ. Psychol. Rev. 28, 425–474. doi: 10.1007/s10648-015-9320-8
Diamond, A. (2013). Executive functions. Annu. Rev. Psychol. 64, 135–168. doi: 10.1146/annurev-psych-113011-143750
Diamond, A., and Ling, D. S. (2016). Conclusions about interventions, programs, and approaches for improving executive functions that appear justified and those that, despite much hype, do not. Dev. Cogn. Neurosci. 18, 34–48. doi: 10.1016/j.dcn.2015.11.005
Dijkhuis, R., De Sonneville, L., Ziermans, T., Staal, W., and Swaab, H. (2020). Autism symptoms, executive functioning and academic progress in higher education students. J. Autism Dev. Disord. 50, 1353–1363. doi: 10.1007/s10803-019-04267-8
Dinsmore, D. L., Alexander, P. A., and Loughlin, S. M. (2008). Focusing the conceptual lens on metacognition, self-regulation, and self-regulated learning. Educ. Psychol. Rev. 20, 391–409. doi: 10.1007/s10648-008-9083-6
Duncan, T., and McKeachie, W. J. (2015). Motivated Strategies for Learning Questionnaire (MSLQ) Manual. Available online at: https://www.researchgate.net/publication/280741846_Motivated_Strategies_for_Learning_Questionnaire_MSLQ_Manual (accessed June 5, 2019).
Effeney, G., Carroll, A., and Bahr, N. (2013). Self-regulated learning and executive function: Exploring the relationships in a sample of adolescent males. Educ. Psychol. 33, 773–796. doi: 10.1080/01443410.2013.785054
Follmer, D. J. (2021). Examining the rol of calibration of executive function performance in college learners’ regulation. Appl. Cogn. Psychol. 35, 646–658. doi: 10.1002/acp.3787
Follmer, D. J., and Sperling, R. A. (2016). The mediating role of metacognition in the relationship between executive function and self-regulated learning. Br. J. Educ. Psychol. 86, 559–575. doi: 10.1111/bjep.12123
Garner, J. (2009). Conceptualizing the relations between executive functions and self-regulated learning. J. Psychol. Interdiscip. Appl. 143, 405–426. doi: 10.3200/JRLP.143.4.405-426
Giedd, J. N., and Rapoport, J. L. (2010). Structural MRI of pediatric brain development: What have we learned and where are we going? Neuron 67, 728–734. doi: 10.1016/j.neuron.2010.08.040
Gioia, G. A., Isquith, P. K., Guy, S. C., and Kenworthy, L. (2000). Test review. Behavior rating inventory of executive function. Child Neuropsychol. 6, 235–238. doi: 10.1076/chin.6.3.235.3152
Hajjar, S. (2018). Statistical analysis: Internal-consistency reliability and construct validity. Int. J. Quant. Qual. Res. Methods 6, 46–57.
Hauser, P. C., Lukomski, J., and Samar, V. (2013). Reliability and validity of the BRIEF-A for assessing deaf college students’ executive function. J. Psychoeduc. Assess. 31, 363–374. doi: 10.1177/0734282912464466
Hayat, A. A., Shateri, K., Amini, M., and Shokrpour, N. (2020). Relationships between academic self-efficacy, learning-related emotions, and metacognitive learning strategies with academic performance in medical students: A structural equation model. BMC Med. Educ. 20:76. doi: 10.1186/s12909-020-01995-9
Honicke, T., and Broadbent, J. (2016). The influence of academic self-efficacy on academic performance: A systematic review. Educ. Res. Rev. 17, 63–84. doi: 10.1016/j.edurev.2015.11.002
Hu, L., and Bentler, P. M. (1999). Cutoff criteria for fit indexes in covariance structure analysis: Conventional criteria versus new alternatives. Struct. Equat. Model. 6, 1–55. doi: 10.1080/10705519909540118
Huizinga, M., and Schmidts, D. P. (2012). Age-related changes in executive function: A normative study with the Dutch version of the Behavior Rating Inventory of Executive Function (BRIEF). Child Neuropsychol. 17, 51–66. doi: 10.1080/09297049.2010.509715
Ihm, L., Zhang, H., Van Vijfeijken, A., and Waugh, M. G. (2021). Impacts of the COVID-19 pandemic on the health of university students. Int. J. Health Plann. Manag. 36, 618–627. doi: 10.1002/hpm.3145
Jolles, J., and Jolles, D. D. (2021). On neuroeducation: Why and how to improve neuroscientific literacy in educational professionals. Front. Psychol. 12:752151. doi: 10.3389/fpsyg.2021.752151
Kline, R. B. (2016). Principles and practice of structural equation modeling, 4th Edn. New York, NY: The Guilford Press.
Knouse, L. E., Feldman, G., and Blevins, E. J. (2014). Executive functioning difficulties as predictors of academic performance: Examining the role of grade goals. Learn. Individ. Differ. 36, 19–26. doi: 10.1016/j.lindif.2014.07.001
Larrabee, G. J. (2012). Performance validity and symptom validity in neuropsychological assessment. J. Int. Neuropsychol. Soc. 18, 625–630. doi: 10.1017/S1355617712000240
Li, J., Ye, H., Tang, Y., Xhou, Z., and Hu, X. (2018). What are the effects of self-regulation phases and strategies for Chinese students? A meta-analysis of two decades research of the association between self-regulation and academic performance. Front. Psychol. 9:2434. doi: 10.3389/fpsyg.2018.02434
Lowe, H. and Cook, A. (2003). Mind the Gap: Are students prepared for higher education? J. Furth. High. Educ. 27, 53–76. doi: 10.1080/03098770305629
Marsh, H. W. (2007). “Application of confirmatory factor analysis and structural equation modeling in sport/exercise psychology,” in Handbook of sport psychology, 3rd Edn, eds G. Tenenbaum and R. C. Eklund (New York, NY: Wiley Online Library), 774–798.
Marsh, H. W., Hau, K., and Grayson, D. (2005). “Goodness of fit evaluation in structural equation modeling,” in Psychometrics: A festschrift to Roderick P. McDonald, eds A. Maydeu-Olivares and J. McArdle (Mahwah, NJ: Erlbaum), 275–340.
Marsh, H. W., Tau, K., and Wen, Z. (2004). In search of golden rules: Comment on hypothesis-testing approaches to setting cutoff values for fit indexes and dangers in overgeneralizing Hu and Bentler’s (1999) Findings. Struct. Equ. Model. 11, 320–341. doi: 10.1207/s15328007sem1103_2
McDonald, J. D. (2008). Measuring personality constructs: The advantages and disadvantages of self-reports, informant reports and behavioural assessments. Enquire 1, 75–94.
Meltzer, L., Dunstan-Brewer, J., and Krishnan, K. (2018). “Learning differences and executive function,” in Executive function in education. From theory to practice, 2nd Edn, ed. L. Meltzer (New York, NY: The Guilford Press), 109–141.
Miller, E. K., and Cohen, J. D. (2001). An integrative theory of prefrontal cortex functio. Annu. Rev. Neurosci. 24, 167–202. doi: 10.1146/annurev.neuro.24.1.167
Moghadari-Koosha, M., Moghadasi-Amiri, M., Cheraghi, F., Mozafari, H., Imani, B., and Zandieh, M. (2020). Self-efficacy, self-regulated learning, and motivation as factors influencing academic achievement among paramedical students. J. Allied Health 49, 221–228.
Mohammadnia, S., Bigdeli, I., Mashhadi, A., Ghanaei Chamanabad, A., and Roth, R. M. (2022). Behavior Rating Inventory of Executive Function – adult version (BRIEF-A) in Iranian university students: Factor structure and relationship to depressive symptom severity. Appl. Neuropsychol. Adult 29, 786–792. doi: 10.1080/23279095.2020.1810689
Moran, S., and Gardner, H. (2018). “Hill, skill, and will: Executive function from a multiple-intelligences perspective,” in Executive function in education. From theory to practice, 2nd Edn, ed. L. Meltzer (New York, NY: The Guilford Press), 29–56.
Musso, M. F., Boekaerts, M., Segers, M., and Cascallar, E. C. (2019). Individual differences in basic cognitive processes and self-regulated learning: Their interaction effects on math performance. Learn. Individ. Differ. 71, 58–70. doi: 10.1016/j.lindif.2019.03.003
Naglieri, J. A., and Otero, T. M. (2014). “The assessment of executive function using the cognitive assessment system,” in Handbook of executive functioning, 2nd Edn, eds S. Goldstein and J. Naglieri (New York, NY: Springer), 191–208.
Nigg, J. T. (2017). Annual research review: On the relations among self-regulation, self-control, executive functioning, effortful control, cognitive control, impulsivity, risk-taking, and inhibition for developmental psychology. J. Child Psychol. Psychiatry 58, 361–383. doi: 10.1111/jcpp.12675
Pachón-Basallo, M., De la Fuente, J., Gonzáles-Torres, M. C., Martinez-Vicente, J. M., Peralta-Sánchez, F. J., and Vera-Martinez, M. M. (2022). Effects of self-regulation vs. factors of external regulation of learning in self-regulated study. Front. Psychol. 13:968733. doi: 10.3389/fpsyg.2022.968733
Panadero, E. (2017). A review of self-regulated learning: Six models and four directions of research. Front. Psychol. 8:422. doi: 10.3389/fpsyg.2017.00422
Panadero, E., Klug, J., and Järvelä, S. (2016). Third wave of measurement in the self-regulated learning field: When measurement and intervention come hand in hand. Scand. J. Educ. Res. 60, 723–735. doi: 10.1080/00313831.2015.1066436
Pascual, A. C., Muñoz, N. M., and Robres, A. Q. (2019). The relationship between executive functions and academic performance in primary education: Review and meta-analysis. Front. Psychol. 10:1582. doi: 10.3389/fpsyg.2019.01582
Pat-El, R. J., Tillema, H., Segers, M., and Vedder, P. (2013). Validation of assessment for learning questionnaires for teachers and students. Br. J. Educ. Psychol. 83, 98–113. doi: 10.1111/j.2044-8279.2011.02057.x
Pinochet-Quiroz, P., Lepe-Martínez, N., Gálvez-Gamboa, F., Ramos-Galarza, C., Del Valle Tapia, M., and Acosta-Rodas, P. (2022). Relationship between cold executive functions and self-regulated learning management in college students. Estudios Sobre Educ. 43, 93–113. doi: 10.15581/004.43.005
Pintrich, P. R. (2004). A conceptual framework for assessing motivation and self-regulated learning in college students. Educ. Psychol. Rev. 16, 385–407. doi: 10.1007/s10648-004-0006-x
Rabin, L. A., Fogel, J., and Nutter-Upham, K. E. (2011). Academic procrastination in college students: The role of self-reported executive function. J. Clin. Exp. Neuropsychol. 33, 344–357. doi: 10.1080/13803395.2010.518597
Ramos-Galarza, C., Acosat-Rodas, P., Bolaňos-Pasquel, M., and Lepe-Martínez, N. (2020). The role of executive functions in academic performance and behaviour of university students. J. Appl. Res. High. Educ. 12, 444–455. doi: 10.1108/JARHE-10-2018-0221
Roth, R. M., Isquith, P. K., and Gioia, G. A. (2005). BRIEF-A: behavior rating inventory of executive function–adult Version: Professional manual. Lutz, FL: Psychological Assessment Resources.
Roth, R. M., Lance, C. E., Isquith, P. K., Fischer, A. S., and Giancola, P. R. (2013). Confirmatory factor analysis of the behavior rating inventory of executive function®-adult version in healthy adults and application to attention-deficit/hyperactivity disorder. Arch. Clin. Neuropsychol. 28, 425–434. doi: 10.1093/arclin/act031
Rovers, S. F. E., Clarebout, G., Savelberg, H. H. C. M., De Bruin, A. B. H., and Van Merrïenboer, J. J. G. (2019). Granularity matters: Comparing different ways of measuring self-regulated learning. Metacogn. Learn. 14, 1–19. doi: 10.1007/s11409-019-09188-6
Rutherford, T., Buschkuehl, M., Jaeggi, S. M., and Farkas, G. (2018). Links between achievement, executive functions, and self-regulated learning. Appl. Cogn. Psychol. 32, 763–774. doi: 10.1002/acp.3462
Schellings, G. L. M. (2011). Applying learning strategy questionnaires: Problems and possibilities. Metacogn. Learn. 6, 91–109. doi: 10.1007/s11409-011-9069-5
Scholte, E., and Noens, I. (2011). BRIEF-A. Vragenlijst executieve functies voor volwassenen: Handleiding. Amsterdam: Hogrefe.
Schumacker, R. E., and Lomax, R. G. (2015). Structural equation modeling, 4th Edn. New York, NY: Routledge Taylor and Francis Group.
Schunk, D. H., and Greene, J. A. (2018). “Historical, contemporary, and future perspectives on self-regulated learning and performance,” in Handbook of self-regulation of learning and performance, eds D. H. Schunk and J. A. Greene (New York, NY: Routledge Taylor and Francis Group), 1–15.
Schwartz, S. K., Roper, B. L., Arentsen, T. J., Crouse, E. M., and Adler, M. C. (2020). The behavior rating inventory of executive function§-adult version is related to emotional distress, not executive dysfunction, in a veteran sample. Arch. Clin. Neuropsychol. 35, 701–716. doi: 10.1093/arclin/acaa024
Sun, J. C., Liu, Y., Lin, X., and Hu, X. (2023). Temporal learning analytics to explore traces of self-regulated learning behaviors and their associations with learning performance, cognitive load, and student engagement in an asynchronous online course. Front. Psychol. 13:1096337. doi: 10.3389/fpsyg.2022.1096337
Sun, Z., Xie, K., and Anderman, L. H. (2018). The role of self-regulated learning in students’ success in flipped undergraduate math courses. Internet High. Educ. 36, 41–53. doi: 10.1016/j.iheduc.2017.09.003
Theobald, M. (2021). Self-regulated learning training programs enhance university students’ academic performance, self-regulated learning strategies, and motivation: A meta-analysis. Contemp. Educ. Psychol. 66:101976. doi: 10.1016/j.cedpsych.2021.101976
Tomarken, A. J., and Waller, N. G. (2005). Structural equation modeling: Strengths, limitations, and misconceptions. Annu. Rev. Clin. Psychol. 1, 31–65. doi: 10.1146/annurev.clinpsy.1.102803.144239
Toplak, M. E., West, R. F., and Stanovich, K. E. (2013). Practitioner review: Do performance-based measures and ratings of executive function assess the same construct? J. Child Psychol. Psychiatry 54, 131–143. doi: 10.1111/jcpp.12001
Van Laar, S., and Braeken, J. (2021). Understanding the comparative fit index: It’s all about the base! Pract. Assess. Res. Eval. 26, 1–23. doi: 10.7275/23663996
Veenman, M. V. J. (2005). “The assessment of metacognitive skills: What can be learned from multi-method designs?,” in Lernstrategien und metakognition: Implikationen für forschung und praxis, eds C. Artelt and B. Moschner (Münster: Waxmann), 77–99.
Veenman, M. V. J. (2011). “Learning to self-monitor and self-regulate,” in Handbook of research on learning and instruction, eds R. Mayer and P. Alexander (New York, NY: Routledge), 197–218.
Virtanen, P., Niemi, H. M., and Nevgi, A. (2017). Active learning and self-regulation enhance student teachers’ professional competences. Austr. J. Teach. Educ. 42, 1–20. doi: 10.14221/ajte.2017v42n12.1
Willingham, D. (2023). Outsmart your brain. Why learning is hard and how you can make it easy. New York, NY: Gallery Books.
Wirth, J., Stebner, F., Trypke, M., Schuster, C., and Leutner, D. (2020). An interactive layers model of self-regulated learning and cognitive load. Educ. Psychol. Rev. 32, 1127–1149. doi: 10.1007/s10648-020-09568-4
Yamada, M., Shimada, A., Okubo, F., Oi, M., Kojima, K., and Ogata, H. (2017). Learning analytics of the relationships among self-regulated learning, learning behaviors, and learning performance. Res. Pract. Technol. Enhanced Learn. 12:13. doi: 10.1186/s41039-017-0053-9
Zelazo, P. D., and Carlson, S. M. (2020). The neurodevelopment of executive function skills: Implications for academic achievement gaps. Psychol. Neurosci. 13, 273–298. doi: 10.1037/pne0000208
Keywords: executive functions, self-regulated learning, study success, academic success, higher education, student, structural equation modeling
Citation: Manuhuwa DM, Snel-de Boer M, Jaarsma ADC, Fleer J and De Graaf JW (2023) The combined value of executive functions and self-regulated learning to predict differences in study success among higher education students. Front. Psychol. 14:1229518. doi: 10.3389/fpsyg.2023.1229518
Received: 26 May 2023; Accepted: 30 October 2023;
Published: 18 November 2023.
Edited by:
Maria Carmen Pichardo, University of Granada, SpainReviewed by:
Melissa T. Buelow, The Ohio State University, United StatesInmaculada Méndez, University of Murcia, Spain
Copyright © 2023 Manuhuwa, Snel-de Boer, Jaarsma, Fleer and De Graaf. This is an open-access article distributed under the terms of the Creative Commons Attribution License (CC BY). The use, distribution or reproduction in other forums is permitted, provided the original author(s) and the copyright owner(s) are credited and that the original publication in this journal is cited, in accordance with accepted academic practice. No use, distribution or reproduction is permitted which does not comply with these terms.
*Correspondence: Diane Marcia Manuhuwa, ZC5tLm1hbnVodXdhQHNheGlvbi5ubA==