- 1Department of Emergency Medicine, Inselspital, Bern University Hospital, University of Bern, Bern, Switzerland
- 2Graduate School for Health Sciences, University of Bern, Bern, Switzerland
- 3Center for Educational Measurement (CEMO) and Unit for Health Sciences Education, University of Oslo, Oslo, Norway
- 4FHNW School of Applied Psychology, University of Applied Sciences and Arts, Northwestern Switzerland, Olten, Switzerland
- 5Division of Anesthesiology and Intensive Care, Department of Clinical Sciences, Intervention and Technology, Karolinska Institutet, Huddinge, Sweden
- 6Department of Social and Communication Psychology, University of Göttingen, Göttingen, Germany
Introduction: Effective teamwork plays a critical role in achieving high-performance outcomes in healthcare. Consequently, conducting a comprehensive assessment of team performance is essential for providing meaningful feedback during team trainings and enabling comparisons in scientific studies. However, traditional methods like self-reports or behavior observations have limitations such as susceptibility to bias or being resource consuming. To overcome these limitations and gain a more comprehensive understanding of team processes and performance, the assessment of objective measures, such as physiological parameters, can be valuable. These objective measures can complement traditional methods and provide a more holistic view of team performance. The aim of this study was to explore the potential of the use of objective measures for evaluating team performance for research and training purposes. For this, experts in the field of research and medical simulation training were interviewed to gather their opinions, ideas, and concerns regarding this novel approach.
Methods: A total of 34 medical and research experts participated in this exploratory qualitative study, engaging in semi-structured interviews. During the interview, experts were asked for (a) their opinion on measuring team performance with objective measures, (b) their ideas concerning potential objective measures suitable for measuring team performance of healthcare teams, and (c) their concerns regarding the use of objective measures for evaluating team performance. During data analysis responses were categorized per question.
Results: The findings from the 34 interviews revealed a predominantly positive reception of the idea of utilizing objective measures for evaluating team performance. However, the experts reported limited experience in actively incorporating objective measures into their training and research. Nevertheless, they identified various potential objective measures, including acoustical, visual, physiological, and endocrinological measures and a time layer. Concerns were raised regarding feasibility, complexity, cost, and privacy issues associated with the use of objective measures.
Discussion: The study highlights the opportunities and challenges associated with employing objective measures to assess healthcare team performance. It particularly emphasizes the concerns expressed by medical simulation experts and team researchers, providing valuable insights for developers, trainers, researchers, and healthcare professionals involved in the design, planning or utilization of objective measures in team training or research.
1. Introduction
1.1. Team significance and measures
Collaborative efforts are undeniably essential in providing healthcare. Health-care teams operate in situations that require making high-risk and high-stakes decisions while facing time constraints (Teuma Custo and Trapani, 2020). Empirical research demonstrates that the performance of such teams relies not only on their medical expertise and technical skills but also on their teamwork, that is, their ability to work together effectively (Manser, 2009; Schmutz and Manser, 2013; Schmutz et al., 2019). Furthermore, effective teamwork within health-care teams significantly impacts patient outcomes, as well as staff satisfaction, well-being, and overall organizational success (Heinemann and Zeiss, 2002; Pronovost et al., 2006; Schmutz and Manser, 2013; Rosenman et al., 2018).
Teamwork is a collaborative process in which team members interact and pool their collective resources to meet task requirements such as resuscitating a patient (Fernandez et al., 2008). To ensure high performance, Salas et al. (2008b) have highlighted the importance of various elements of effective teamwork, such as high team cohesion, adaptability, flexibility, and problem-solving skills. However, effective teamwork is often hindered by communication failures, coordination problems, and interprofessional stereotypes (Kozlowski and Klein, 2000; Devine and Philips, 2001; Dietz et al., 2014). Numerous reviews suggest that team trainings and interprofessional education activities can mitigate these obstacles and improve teamwork (Chakraborti et al., 2008; Salas et al., 2008a; Weaver et al., 2014; Fox et al., 2018; Hughes et al., 2019).
In order to identify areas for improvement and to provide feedback to team members in such trainings, it is essential to evaluate team performance in a reliable and valid way (Edmondson, 1999). Establishing appropriate methods for assessing and evaluating team performance is also essential for measuring and monitoring medical teams in their working environment, understanding how to develop and maintain “good” teamwork, and identifying the criteria for “good” teams and outcomes (Jeffcott and Mackenzie, 2008). Measuring team performance is similarly relevant for research purposes, such as when investigating the components of successful teamwork (Murray and Enarson, 2007). However, assessing team performance can be challenging due to the complexity of team dynamics, lack of clear metrics, and limited data and resources (Marlow et al., 2018).
Currently, a multitude of different measures of teamwork is used in the context of team trainings and research. While self-reports and peer assessments are well established, including physiological measures such as the team members’ heart rate variability (HRV) or electrodermal activity (EDA) as indicators of their arousal or stress level are relatively new and still unexplored ways of teamwork assessment. Yet, they deserve a closer exploration as they can potentially mitigate some of the limitations associated with traditional measures such as susceptibility to self-reporting bias (Kozlowski et al., 2013). With our study, we aim to capture the opinions of experts in the fields of medical team training and research on the potentials and challenges associated with integrating physiological and, more generally, objective measures of teamwork into the evaluation of healthcare teamwork. By seeking insights from key stakeholders, this study endeavors to contribute to the theoretical discourse on healthcare teamwork assessment, while also highlighting practical implications for medical training and research.
1.2. Traditional and novel evaluation approaches
For a comprehensive evaluation of team performance, it is essential to assess both team processes and outcomes. Team processes include the strategies, steps, and procedures used by the team to accomplish a task (Salas and Cannon-Bowers, 1997). Team performance outcomes focus on results, such as treatment and patient condition. To assess training benefits, medical training studies usually focus on reporting outcomes such as triage accuracy, time to triage, and occasionally administer the perceived benefits from participants (e.g., Luigi Ingrassia et al., 2015; Dittmar et al., 2018; Baetzner et al., 2022). Team researchers typically use the same measures, often assessing time intervals during medical processes, such as decision or execution latency (Burtscher et al., 2011), percentage of hands-on time during resuscitations (Tschan et al., 2009), or durations required to complete a specific task (Tschan et al., 2009). Adherence to institutional standards (Kolbe et al., 2012) or the diagnostic process itself (Tschan et al., 2009) are also considered as measures of teamwork quality.
Traditional data sources for assessing team performance have their advantages and disadvantages (Salas and Cannon-Bowers, 2001; Marlow et al., 2018). Self-reports and peer-assessments can provide access to unobservable reactions, attitudes, and emotions, but may suffer from biases, particularly if individuals are motivated to present themselves in a favorable light. Expert observations based on standardized tools, such as the Team Emergency Assessment Measure (TEAM; Cooper et al., 2010) or Medi-StuNTS (Hamilton et al., 2019), can provide reliable assessment of relevant attributes, but are time- and resource-intensive. On the positive side, measuring these observable behaviors provides actionable guidance for team members to improve their future performance (Rosen et al., 2010).
Research on the unobtrusive measurement of team members’ physiological parameters (biosignals) suggests that an additional source of data can provide valuable information about team processes and unobservable states, such as stress levels, and allow objective assessments of relevant parameters in real time: team physiological dynamics (Kazi et al., 2021; Hałgas et al., 2022). This endeavor is in line with the growing recognition of the multidimensional nature of effective teamwork, highlighting the benefits of considering both visible behaviors and underlying physiological responses (Rojo López et al., 2021). By monitoring physiological signals such as HRV, researchers can assess the arousal, attention, and emotional states of team members during training or real-life scenarios. This information can complement traditional measures to provide a more comprehensive and objective picture of team performance. Moreover, the use of objective measures offers the possibility to shift the focus from an outcome-based assessment toward a process-oriented assessment (Salas et al., 2017; Hałgas et al., 2022). They include specific and measurable data obtained through standardized measurements that are not influenced by personal biases or subjective interpretations. The crucial advantage of objective measures is their ability to capture data at a fine resolution over long periods of time, which cannot be achieved with conventional measures. However, the strategic implementation of objective measures such as physiological data requires a user-friendly methodology that simplifies the analysis and interpretation of data. Furthermore, the collection and analysis of physiological data, for instance, still incurs inherent costs in terms of time and resources.
Despite these potential benefits, the effective use of physiological data in team training and its relationship to higher-order constructs such as successful coordination is still poorly understood. To date, most practical studies in the field of physiological team dynamics have been conducted using simulations of work-related tasks (Kazi et al., 2021; Hałgas et al., 2022). In addition to simulation studies, there are also laboratory studies that investigate physiological team dynamics in video games, simple tasks, or similar (Chanel et al., 2012; Järvelä et al., 2014; Fusaroli et al., 2016). In the medical field, however, there are only a handful of studies, which have investigated only one or two physiological measures like direction of gaze and pupillometry (He et al., 2021) or EDA (Misal et al., 2020). The complexity of the topic and the challenges associated with significant and appropriate implementation could be possible explanations. Therefore, research in this area is essential for the future use of objective data to capture team performance indicators.
1.3. Research questions
Our proposed vision is to use objective measures to assess team performance during team training and research to complement traditional team performance assessment. Hereby, objective measures are understood as factual and quantifiable information obtained through standardized measurement and free from personal bias or interpretation, including bio-signals, time stamps, checklists, and the like. By integrating these measures into team assessments, layers of team interactions that often remain concealed may be unveiled, representing new dimensions for the analysis and comprehension of teamwork.
With our study, we aim to contribute to the discussion and ultimately the effective implementation of objective measures into teamwork assessment in training and research contexts. Thereby, we follow the principles of participatory action research, with active engagement of stakeholders who will play a role in its implementation. The primary focus is thus to assess the views of medical team coaches and researchers, key stakeholders in healthcare teamwork, on the viability of integrating objective measures, with particular reference to physiological data, and to identify the potential benefits, challenges and acceptability associated with this approach. We have three main research questions (RQ):
RQ 1: What do experts think about the vision of evaluating team performance with objective measures?
RQ 2: Which objective measures could be used to evaluate medical team performance?
RQ 3: What could be obstacles with the approach of using objective measures to evaluate team performance during team training and research?
In summary, our approach envisions the harmonious integration of objective measures, including physiological indicators, to holistically assess team performance. The aim of this study was to find out what experts in medical education and team research think and know about the opportunities and barriers to evaluating medical team performance using objective measures. In doing so, we aim to provide insights that will shape medical education, research and the wider understanding of teamwork in healthcare.
2. Methods
2.1. Study design
An exploratory qualitative study design was utilized. We conducted a semi-structured interview study with two expert-groups, followed by a brief on-line survey.
2.2. Participants
To answer the three research questions, medical team training and scientific team experts were recruited and interviewed. The inclusion criterion for participation in the interviews was thus either team training expertise as a trainer in medical team training or expertise as a team researcher. Experience in the field of physiological data collection was not required. In addition, we aimed to include an equal number of women and men in the sample.
To identify relevant experts in the field, we used the snowball sampling procedure (Parker et al., 2019). The medical experts were solicited with the help of recommendations from the co-authors, after which they were in turn asked for recommendations at the end of the interviews. For the team researchers, researchers with publications in the field of team research were sought, who were then also asked for recommendations.
2.3. Material
The interview questions were developed by the authors in line with the research questions. The complete interview guide can be found in the Supplementary material 1.1. The following analysis will focus on the interview questions from the third block: (a) What comes to your mind when you hear about our vision/goal? (b) Have you considered using objective measures such as biosignals in team training/research to evaluate team performance, and if so, which ones? (c) What are the factors and challenges in assessing team performance using physiological parameters? Where can objective measures be used?
In order to keep the interviews as short as possible, an on-line questionnaire was sent to the interviewees after the interview (via www.soscisurvey.de). It consisted of three questions on age, gender, and expertise (i.e., number of years of experience in training/research context).
2.4. Procedure
Identified experts were invited via email to participate in a 30–60 min interview. They were informed that (a) the interview would be recorded, transcribed, and analyzed, (b) their identity would remain confidential, and (c) that participation was voluntary and could be withdrawn at any time.
Interviews were conducted in German or English, according to the preference of the interviewee. At the beginning of an interview, consent was again obtained for the interview to be recorded. The interview procedure was then explained and the interview conducted. Finally, the experts were thanked for their participation, the literature on objective measures of performance was briefly explained, and open questions were answered. They were also offered the opportunity to receive news about the project by email.
After the interviews, participants were sent the on-line survey on demographics. If this was not completed after 7 days, the participants were reminded by email.
2.5. Setting
All interviews and questionnaires were collected between June and August in 2022 and were conducted by the first author of this paper. All interviews were conducted using Zoom (Zoom Video Communications Inc., 2016) and recorded using the integrated tool.
2.6. Analysis
The transcription of interviews was carried out verbatim by the first author with the help of a speech recognition software (Dragon NaturallySpeaking, Nuance Communications Inc.).
The content analysis was based on the approach of Kuckartz, 2019, which is a rigorous and systematic method used in qualitative research to analyze textual data. It involves the identification and categorization of specific content patterns, themes or codes within the data, providing valuable insights and interpretations for the research study.
All categorizations were carried out by one rater, checked by another rater, and then aggregated into categories by two raters using a consensus procedure. We used MAXQDA 2022.2 (VERBI Software, 2022) for the process of data analysis.
For RQ1 (i.e., opinion on vision), responses were categorized into three categories (positive, neutral, and negative) according to their valence. The positive category included responses that were predominantly positive about the vision presented. The neutral category included all responses that did not have a clear value or where the question was not answered. The negative category included responses where experts expressed a negative or hesitant view such as when they could not relate to the vision or pointed to unsurmountable obstacles.
For RQ2 (i.e., possible measures), responses were categorized based on the measures mentioned. Higher order categories were created to group related measures together such as EDA and electrocardiogram (ECG) together to electrophysiological measures.
For RQ3 (i.e., obstacles), responses were categorized according to the named obstacles identified. Similarly, higher-order categories were established based on the source of these obstacles such as whether they originated from an individual, the model or concerned the implementation.
We decided not to weight the identified categories by their frequency but to treat all responses equally in order to receive a comprehensive overview.
In order to explore how familiar interviewees were with objective measures in the context of team training and research, all responses were examined to determine whether participants had reported personal experience or had undertaken projects or experiments involving objective measures (categorized as “having experience”) or not (categorized as “not having experience).
Demographic data was collected and are presented as means and standard deviations. In addition, t-tests were conducted with the software “R,” version 4.3.0, for the variables age and expertise to check whether the two expert-groups differed from each other.
2.7. Ethics
The studies involving human participants were reviewed and approved by The Bern Cantonal Ethics Committee (CEC, BASEC Nr: Req-2022-00684). All methods were carried out in accordance with relevant guidelines and regulations. Informed Consent to participate was recorded in writing, at the beginning of the interview and in the survey by each participant. The data were collected, analyzed and stored in pseudonymised form.
3. Results
3.1. Sample
3.1.1. Demographics
In total, N = 34 interviews were conducted (44.1% women, Age: M = 48.8 years, SD = 11.1, Expertise: M = 17.2 years, SD = 8.4). One person from the medical group did not respond to the survey. Interviews were conducted with n = 21 medical experts who trained medical staff as simulation trainers in Switzerland, Germany, and Austria (38.1% women, Age: M = 46.8 years, SD = 9.3, Expertise: M = 15.5 years, SD = 7.2), and interviews with n = 13 team research experts conducting research in Europe and the United States (53.9% women, Age: M = 51.9 years, SD = 13.2, Expertise: M = 19.7 years, SD = 9.9). The expert groups did not differ significantly from each other in terms of age [t(32) = −1.309, p = 0.1] and expertise [t(32) = −1.435, p = 0.080].
3.1.2. Experience with objective measures in simulation training and research
In total, 10 of the 34 experts (29.4%) stated that they had experience with physiological measurements in the context of simulation training and research such as with heart rate or examinations of volume. Of these, eight were from the group of team researchers.
3.2. Interview responses
3.2.1. RQ1: experts’ opinion about vision
Of the 34 responses, 19 were positive, 13 were neutral, and three were negative. Both neutral and positive responses were consistently constructive, with curious and skeptical elements. In terms of content, the responses of the expert groups did not differ meaningfully from each other. All three negative responses came from the medical experts.
An example of a positive response:
“[…] In principle, I don't think it's a bad idea. If it were possible to measure stress levels before, during and after a task in a less annoying, less invasive and relatively chic way that would certainly be a good addition to self-reported levels. And I can also see us getting to the point where fitness trackers alone can tell me how well I slept, which means they can certainly tell me how much stress I was under”—(Medical Expert, CM).
An example of a neutral response:
“[…] So what I don't know at the moment is how to do this better in an automated way, although there are certainly people who are extracting data from measurements, whether it's videos, coding or something like that to make it objective but I haven't seen any implementation of that in science yet”—(Research Expert, SK).
An example of a negative response:
“I think it's very challenging, very difficult. It seems very complex and the scenarios are often difficult even for the instructors because you have to be very flexible. Just because we've thought about something in the planning and we know which way it's going to go, doesn't mean it's going to work that way. It is insanely difficult to somehow get a standardized evaluation out of it”—(Medical Expert, DH).
3.2.2. RQ2: possible measures
Answers to the second research question were categorized into five main categories: visual, acoustical, physiological, and endocrinological measures, and a time layer (see Figure 1 and Supplementary Table 1 for a list of exemplary answers).
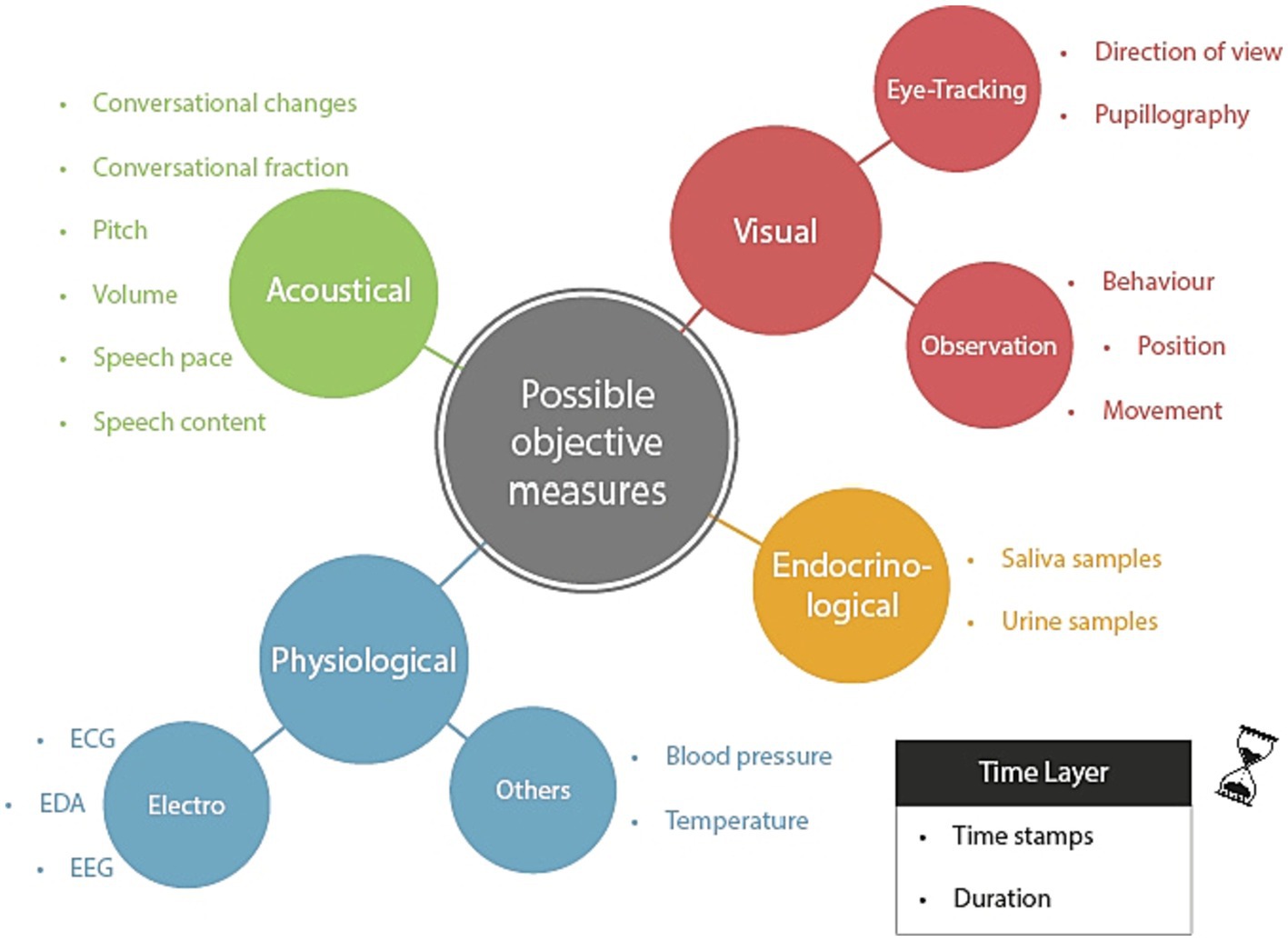
Figure 1. Overview of main and sub-categories concerning the question for potential objective measures. The time layer is to be understood as a meta-layer, which may be integrated with the other layers so that the measured values can be located in their time and duration. EEG, Electroencephalography; EDA, Electrodermal activity; and ECG, Electrocardiogram.
Concerning the time layer, our interview partners highlighted the importance of utilizing time stamps and duration tracking to gain a better understanding of the temporal aspects of social interactions. They emphasized the need for capturing social dynamics in various contexts, particularly in medical settings, and the potential benefits of data-driven approaches for analyzing them.
In terms of physiological data, the experts suggested measuring blood pressure, temperature, electroencephalogram (EEG) signals, and EDA and ECG variables (heart rate and heart rate variability) as potential indicators of stress levels or other relevant factors. Endocrinological measurements, such as analyzing saliva or urine samples, were also named. As they are usually evaluated using laboratory analyses after a training session, rather than in real time, they are depicted as a separate category in Figure 1.
Visual methods named involved observing behavior and movements to indicate the actions taken and the stage of a process. In addition, eye-tracking measures such as pupillography to measure cognitive load, as changes in pupil width can reflect this, and tracking the direction of view to enhance situational awareness and interface design were proposed. Experts acknowledged that careful preparation and interpretation of the data are essential to avoid misinterpretation.
Acoustical measures from the field of communication analysis were suggested to provide useful insights into interpersonal dynamics. These measurements consist of conversational changes that track interaction frequency and nature to assess leadership roles and psychological safety. Conversation fraction analysis captures patterns of interaction and communication frequency among team members. Speech content analysis focuses on the quality of communication. In addition, acoustical indicators like pitch, volume, and speech pace could be studied to comprehend how they impact responses and interactions between individuals.
The list of answers given by the two groups of experts differed only slightly. For example, “pupillography” and “temperature” were mentioned by the medical experts but not by the research experts. On the other hand, the research experts mentioned EEG, which was not mentioned by the medical experts.
3.2.3. RQ3: obstacles
Answers to the third research question were categorized into four main categories: individual, implementation, doubts, and model/concept. The last category consisted of two sub-categories: bias and situation-dependent output. Each category comprised several obstacles, which are summarized in Figure 2.
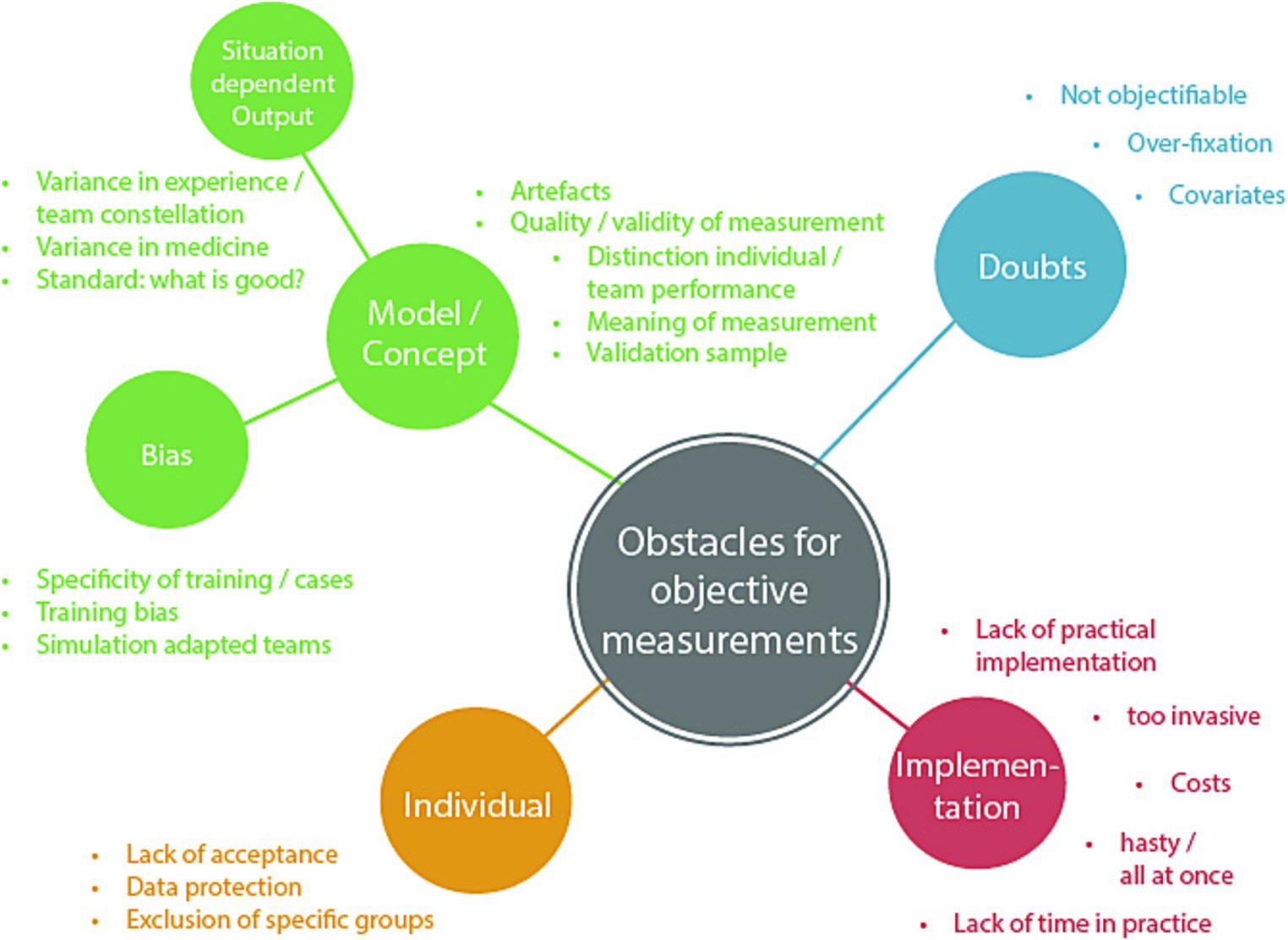
Figure 2. Overview of main and sub-categories concerning the question for potential obstacles of objective measures.
The interviews disclosed integrated themes that covered the model, bias and situation-dependent output in evaluating the performance of medical teams. Experts acknowledged the importance of artifacts, illustrating how they could both improve and impede training. This encouraged an analysis of the quality and legitimacy of measuring techniques, as well as the difficulty in differentiating individual from collective performance within a convoluted team context. As experts explored the meaning of measurements, they emphasized the importance of validation samples to enhance the reliability of objective evaluation. The discussion broadened to encompass prejudices that arose from training specificity, where particular case requirements and the participants’ consciousness of simulation conditions could influence the outcomes. The experts considered the potential influences of training-induced biases and the impact of team adaptation in simulated scenarios. Moreover, the experts dealt with the situation-specific aspects of output, recognizing the complexities of different medical contexts and the impact of proficiency levels on performance deviation. The efforts to establish the criteria for “good” team performance were emphasized, highlighting the need for flexible measurement standards.
In the interviews, concerns were raised regarding the complete objectification of measuring team performance. Experts acknowledged the complex relationship between factors that influence team dynamics. There were queries about the hurdles of achieving objectivity and separating training effects from a variety of covariates. Worries were expressed about the potential peril of overly fixating on certain metrics, which may overshadow subtle aspects of team interactions. The complexity of assessing and interpreting objective measurements of team performance was recognized, highlighting the multidimensional nature of this subject.
Experts emphasized the delicate balance between obtaining objective data and maintaining the authenticity of the simulation environment. Challenges related to the invasiveness of measurement devices, associated costs, and time constraints were named. Several experts emphasized the importance of a gradual, step-by-step approach to implementation, and ensuring effective navigation of challenges. In parallel, experts recognized the need to address issues related to individual acceptance, data protection, and privacy to ensure the successful integration of objective measurement methods.
The importance of individual factors in measuring the performance of medical teams was identified as a critical theme. Experts acknowledged the need to consider inclusivity and potential exclusions of groups while developing measurement approaches. They considered the complexities involved in ensuring that assessment methods support varied team compositions while accounting for roles and levels of expertise. In addition, they discussed the importance of participants accepting measurement devices and protocols. They raised concerns regarding the potential violation of data protection and privacy while considering the collection of sensitive physiological data. The experts stressed the significance of creating an environment where individual rights and sensitivities are upheld while enabling the thorough measurement of team performance.
Additional obstacles mentioned included technical challenges and biases in the training process as well as the difficulty to distinguish between individual and team performance. To get a more nuanced understanding of the stated obstacles, see Table 1.
The list of answers given by the two groups of experts differed only slightly from each other. The points “Bias: simulation-adapted teams,” “hasty/all at once,” “costs,” and “lack of time in practice” were only mentioned by the medical experts, while all other points were mentioned by both expert groups.
4. Discussion
The aim of this study was to explore together with relevant stakeholders the potentials and challenges of a novel approach for evaluating team performance for research and training purposes, namely the use of objective measures, by asking experts in the field of research and medical simulation training for their opinions, ideas, and concerns.
To the best of our knowledge, this study is the first to explore the opportunities and challenges of objectively measuring team performance by consulting experts in the relevant fields. Given the increasing feasibility of automated solutions (Kazi et al., 2021; Hałgas et al., 2022), our work thus provides insights to help implement the use of objective measures in the fields of medical simulation training and research, and hints to aspects deemed relevant for the development of such measures from the perspective of its future users.
4.1. Reflections on the results
We found that the use of objective measures to assess medical team performance was met with a combination of interest, goodwill, and a degree of skepticism by the participating experts. Responses included a variety of proposed measurement modalities and potential challenges associated with collecting objective data on team performance. Importantly, the responses from both research and medical experts showed a remarkable level of agreement, reinforcing the consistency within the categories and responses identified.
With respect to RQ1 (vision), we found that the approach to use objective measures to evaluate team performance was received largely positively from the experts. However, it must be acknowledged that only a minority of experts had previous practical experience of measuring objective measures such as physiological data. Consequently, the majority of the experts lacked extensive expertise in the specific area under investigation, which is to be expected given the novelty of the topic. It is important to consider this limitation when interpreting the data collected and drawing conclusions from the study.
With respect to RQ2 (measures), experts saw potential in a variety of measurement methods for assessing the performance of medical teams including acoustical, visual, physiological, and endocrinological measures as well as a time layer. All of the listed measures (Figure 1) have already received some attention in research on team performance assessment in different domains (Elkins et al., 2009; Guastello and Peressini, 2017). For example, there is evidence suggesting that team performance correlates with movement patterns (Calabrese et al., 2021) and several physiological measures such as EDA (Pijeira Díaz et al., 2016), ECG (Rojo López et al., 2021), eye-tracking (He et al., 2021), as well as attention (Mahanama et al., 2022). In the majority of these papers, team performance has been inferred using 1–2 measures, although the use of multiple modalities in one measurement would likely add value to the evaluation of team performance (Hałgas et al., 2022). Moreover, most of the existing studies that have attempted to assess team performance using physiological data have focused on simple tasks that may not be directly relevant to medical procedures, and have been conducted under conditions of low movement, which may mask potential artifacts (Stuldreher et al., 2023; van Eijndhoven et al., 2023). Therefore, it is crucial to address these limitations and ensure that future studies properly account for movement and stress artifacts to ensure the validity of performance evaluations.
Various measures have been used to assess not only overall team performance, but also specific elements of team performance, such as shared cognitive load (Collins et al., 2019; Dias et al., 2019; Dindar et al., 2020), shared attention (Stuldreher et al., 2020; He et al., 2021; Pérez et al., 2021), and stress (Cao et al., 2019; Bhoja et al., 2020; Misal et al., 2020). Existing research has largely focused on assessing team performance using measures such as heart rate and EDA, while limited attention has been paid to using motion and voice data for this purpose.
With respect to RQ3 (obstacles), a number of potential barriers to assessing team performance using objective measures were identified. From a research perspective, one of the most relevant obstacles likely is that it is difficult to define a standard for “good” team performance that takes into account the different contexts and preconditions of teams. In fact, the lack of a gold standard for measuring team performance is a widely acknowledged problem (Heinemann and Zeiss, 2002). A standard of team performance should be established via consensus with relevant stakeholders to enable the development and research of objective measures as a solution to this problem. When conceptualizing an objective approach, it is crucial to consider that, depending on the training and its associated learning objectives, various aspects of a teamwork may be emphasized. Thus, a thorough task analysis will be detrimental for establishing standards for “good” teamwork (Tschan et al., 2011).
Another crucial challenge is the question of how to distinguish between team and individual performance, which needs further theoretical work. Challenges specific to the objective measurement approach included the fear of impaired results due to (e.g., movement) artifacts or low measurement quality, which need to be taken into account by developers and users. This concern points to the need for further development and research efforts to optimize the use of physiological data and ensure their reliability and validity in the context of medical team training. Further research is needed to address the remaining obstacles, including the development of a user-friendly measurement process and the establishment of reliable performance assessment models.
From a medical trainer-centered perspective, the most relevant challenges included concerns about trainee privacy and data handling. Ensuring self-determination and privacy were considered crucial for a positive working environment. According to the experts, implementing an objective measurement approach in real-life settings will require considerations of cost effectiveness and smooth integration. Especially limited resources in the health-professions education sector require a simple and reliable measurement system (Maloney and Haines, 2016). Moreover, the approach must be designed such that it is user-friendly and the data output is easily interpretable to generate enhanced values. Therefore, collaborative development of objective indicators along with simulation trainers and medical educators is not only recommended, but also crucial.
4.2. Outlook
Objective measures can complement traditional methods and, together, offer a more comprehensive perspective on team performance, although the extent of their impact is currently uncertain. The hope connected with this approach is that objective measures may provide more fine-grained process data and thus enable a greater focus on team dynamics, leading to novel training and research insights. They may thus mitigate shortcomings of, for example, behavior observations that typically result in an average rating per dimension for an entire scenario. Moreover, besides training and research settings, it is possible to gather such data in routine clinical practice to assess team dynamics, improve processes, and identify critical issues. Nevertheless, it is crucial to exercise great caution as this approach should not create any sense of control or supervision among medical personnel at any point.
In particular, training concepts using Virtual Reality (Bracq et al., 2019) provide the opportunity to automatically collect numerous objective measures (e.g., eye tracking-, acoustical-, movement tracking-, and behavior data) without much effort since these sensors are part of a classical head mounted display, which may benefit their training outcomes. Moreover, gathering and integrating physiological data with additional devices are also possible, and unlikely to cause significant disruption during routine simulation training. It is worth noting that virtual, augmented, and mixed reality are relatively new in medical education; nevertheless, they are currently used for this purpose, and extensive research exists that attest to their usefulness and effectiveness in various settings (Barteit et al., 2021; Birrenbach et al., 2021). Consequently, the inclusion of various objective parameters in the assessment of these tools in different contexts is the next logical step. This could also provide benefits for new training and evaluation approaches in the domain of health care education (Collins et al., 2019).
It is important to note that the objective approach to team performance evaluation is not intended to supplement traditional performance evaluation, but rather to focus on the processes and thus enrich the overall evaluation. We acknowledge that each measure alone provides only limited insight into team performance. Therefore, Salas et al. (2017, p. 25) proposed to measure team performance in a comprehensive way by triangulating data in terms of (a) collecting data from diverse sources, including self-reports, peer ratings, and observations, in addition to objective outcomes, (b) measuring performance at the individual and team levels, and (c) measuring both processes and outcomes. Such a triangulation approach also promises a rich basis for debriefing, an essential part of medical team training.
4.3. Limitations
Our study comes with some limitations. One limitations is that the majority of the experts we interviewed had no expertise in the specific area of physiological measurement in simulation training or objective assessment using physiological measures. Consequently, their responses were primarily based on subject-specific knowledge or personal beliefs. Yet, the selected experts were key stakeholders in the fields of team research and training and thus representative of the “end users” of objective measures, making it relevant to explore their opinions. It is also worth noting that the field of objective performance assessment, particularly in relation to physiological measures, is still in its infancy and as a result, there are only few experts in this area. To progress, collaboration with experts from relevant adjunct fields is required. Participatory-based model and approach development, based on data, must be continued and consistently improved. Furthermore, it is necessary to involve specialists in the field of measurement technique to prevent issues like unreliable data.
Another limitation is inherent in the interview method, including the potential for respondents to engage in socially acceptable behavior, thereby not fully expressing their true thoughts. To mitigate this risk, participants were assured anonymity to encourage open and honest feedback. Further, it is important to acknowledge that the conclusions drawn from these studies may be limited by the selection of experts (Parker et al., 2019) and the specific questions asked (Halbig et al., 2022).
4.4. Conclusion
In conclusion, this study represents an advance in the exploration of objective measures for evaluating medical team performance by providing insights into the opportunities and challenges observed by the relevant stakeholders. The study provides relevant insights for the future development of objective measurement methods in medical simulation training, research, and beyond. Although challenges related to privacy concerns, resource limitations, and complexity may arise, they should be viewed as opportunities for further research and development. Proactively, addressing these challenges will refine and optimize the use of objective measures and provide a robust framework for assessing team performance in healthcare settings. Future research should focus on expanding the scope of physiological data, designing measures with teams, and collecting data to achieve a comprehensive assessment of team dynamics and build a measurement model. By harnessing the potential of objective measures in close collaboration with experts from relevant fields, this study informs future investigations, developments and utilization, ultimately contributing to the advancement of medical education and training practices, leading to improved patient outcomes. However, it is important to note that this is only the first step in a long journey that will continue to rely on close collaboration with medical simulation trainers and team researchers to further develop and implement team assessment using objective measures.
Data availability statement
The datasets presented in this article are not readily available because consent to share the interviews has not been obtained. Requests to access the datasets should be directed to RW, cmFmYWVsLndlc3BpQGV4dGVybi5pbnNlbC5jaA==.
Ethics statement
The studies involving humans were approved by The Bern Cantonal Ethics Committee. The studies were conducted in accordance with the local legislation and institutional requirements. The participants provided their written informed consent to participate in this study.
Author contributions
RW served as the main author and led each part of the working progress. JK and TS made equal contributions in the planning, data acquisition, analysis, and writing stages. TB, SS, and TM contributed to the planning, analysis, and writing steps. All authors contributed to the article and approved the submitted version.
Funding
This project has received funding from the European Union’s Horizon 2020 Research and Innovation Program under grant agreement No 101021775 and “Medical First Responder Training using a Mixed Reality approach with haptic feedback for enhanced realism” (MED1stMR).
Acknowledgments
We would like to express our sincere gratitude to all the interviewees for generously giving their time and sharing their valuable knowledge and insights. Their contributions have enabled this study and provided important perspectives on the topic of objective performance assessment in medical team training. Special thanks to Marcus Rall for his assistance in identifying and contacting suitable interviewees. His support was crucial to the successful completion of this research project.
Conflict of interest
The authors declare that the research was conducted in the absence of any commercial or financial relationships that could be construed as a potential conflict of interest.
Publisher’s note
All claims expressed in this article are solely those of the authors and do not necessarily represent those of their affiliated organizations, or those of the publisher, the editors and the reviewers. Any product that may be evaluated in this article, or claim that may be made by its manufacturer, is not guaranteed or endorsed by the publisher.
Author disclaimer
The content reflects only authors’ view. Research Executive Agency and European Commission is not liable for any use that may be made of the information contained herein.
Supplementary material
The Supplementary material for this article can be found online at: https://www.frontiersin.org/articles/10.3389/fpsyg.2023.1232628/full#supplementary-material
References
Baetzner, A. S., Wespi, R., Hill, Y., Gyllencreutz, L., Sauter, T. C., Saveman, B.-I., et al. (2022). Preparing medical first responders for crises: a systematic literature review of disaster training programs and their effectiveness. Scand. J. Trauma Resusc. Emerg. Med. 30:76. doi: 10.1186/s13049-022-01056-8
Barteit, S., Lanfermann, L., Bärnighausen, T., Neuhann, F., and Beiersmann, C. (2021). Augmented, mixed, and virtual reality-based head-mounted devices for medical education: systematic review. JMIR Serious Games 9:e29080. doi: 10.2196/29080
Bhoja, R., Guttman, O. T., Fox, A. A., Melikman, E., Kosemund, M., and Gingrich, K. J. (2020). Psychophysiological stress indicators of heart rate variability and electrodermal activity with application in healthcare simulation research. Simulat. Healthcare J. Soc. Simulat. Healthcare 15, 39–45. doi: 10.1097/SIH.0000000000000402
Birrenbach, T., Zbinden, J., Papagiannakis, G., Exadaktylos, A. K., Müller, M., Hautz, W. E., et al. (2021). Effectiveness and utility of virtual reality simulation as an educational tool for safe performance of COVID-19 diagnostics: prospective randomized pilot trial. JMIR Serious Games 9:e29586. doi: 10.2196/29586
Bracq, M.-S., Michinov, E., and Jannin, P. (2019). Virtual reality simulation in nontechnical skills training for healthcare professionals: a systematic review. Simulat. Healthcare J. Soc. Simulat. Healthcare 14, 188–194. doi: 10.1097/SIH.0000000000000347
Burtscher, M. J., Manser, T., Kolbe, M., Grote, G., Grande, B., Spahn, D. R., et al. (2011). Adaptation in anaesthesia team coordination in response to a simulated critical event and its relationship to clinical performance. Br. J. Anaesth. 106, 801–806. doi: 10.1093/bja/aer039
Calabrese, C., Lombardi, M., Bollt, E., De Lellis, P., Bardy, B. G., and di Bernardo, M. (2021). Spontaneous emergence of leadership patterns drives synchronization in complex human networks. Sci. Rep. 11:18379. doi: 10.1038/s41598-021-97656-y
Cao, X., MacNaughton, P., Cadet, L. R., Cedeno-Laurent, J. G., Flanigan, S., Vallarino, J., et al. (2019). Heart rate variability and performance of commercial airline pilots during flight simulations. Int. J. Environ. Res. Public Health 16:237. doi: 10.3390/ijerph16020237
Chakraborti, C., Boonyasai, R. T., Wright, S. M., and Kern, D. E. (2008). A systematic review of teamwork training interventions in medical student and resident education. J. Gen. Intern. Med. 23, 846–853. doi: 10.1007/s11606-008-0600-6
Chanel, G., Kivikangas, J. M., and Ravaja, N. (2012). Physiological compliance for social gaming analysis: Cooperative versus competitive play. Interact. Comput. 24, 306–316. doi: 10.1016/j.intcom.2012.04.012
Collins, J., Regenbrecht, H., Langlotz, T., Can, Y., Ersoy, C., and Butson, R. (2019). Measuring Cognitive Load and Insight: A Methodology Exemplified in a Virtual Reality Learning Context. 2019 IEEE International Symposium on Mixed and Augmented Reality (ISMAR), 351–362.
Cooper, S., Cant, R., Porter, J., Sellick, K., Somers, G., Kinsman, L., et al. (2010). Rating medical emergency teamwork performance: development of the team emergency assessment measure (TEAM). Resuscitation 81, 446–452. doi: 10.1016/j.resuscitation.2009.11.027
Devine, D. J., and Philips, J. L. (2001). Do smarter teams do better: a meta-analysis of cognitive ability and team performance. Small Group Res. 32, 507–532. doi: 10.1177/104649640103200501
Dias, R. D., Zenati, M., Stevens, R., Gabany, J., and Yule, S. (2019). Physiological synchronization and entropy as measures of team cognitive load. J. Biomed. Inform. 96:103250. doi: 10.1016/j.jbi.2019.103250
Dietz, A. S., Pronovost, P. J., Mendez-Tellez, P. A., Wyskiel, R., Marsteller, J. A., Thompson, D. A., et al. (2014). A systematic review of teamwork in the intensive care unit: What do we know about teamwork, team tasks, and improvement strategies? J. Crit. Care 29, 908–914. doi: 10.1016/j.jcrc.2014.05.025
Dindar, M., Järvelä, S., and Haataja, E. (2020). What does physiological synchrony reveal about metacognitive experiences and group performance? Br. J. Educ. Technol. 51, 1577–1596. doi: 10.1111/bjet.12981
Dittmar, M. S., Wolf, P., Bigalke, M., Graf, B. M., and Birkholz, T. (2018). Primary mass casualty incident triage: Evidence for the benefit of yearly brief re-training from a simulation study. Scand. J. Trauma Resusc. Emerg. Med. 26:35. doi: 10.1186/s13049-018-0501-6
Edmondson, A. (1999). Psychological safety and learning behavior in work teams. Adm. Sci. Q. 44:2. doi: 10.2307/2666999
Elkins, A. N., Muth, E. R., Hoover, A. W., Walker, A. D., Carpenter, T. L., and Switzer, F. S. (2009). Physiological compliance and team performance. Appl. Ergon. 40, 997–1003. doi: 10.1016/j.apergo.2009.02.002
Fernandez, R., Kozlowski, S. W. J., Shapiro, M. J., and Salas, E. (2008). Toward a definition of teamwork in emergency medicine. Acad. Emerg. Med. 15, 1104–1112. doi: 10.1111/j.1553-2712.2008.00250.x
Fox, L., Onders, R., Hermansen-Kobulnicky, C. J., Nguyen, T.-N., Myran, L., Linn, B., et al. (2018). Teaching interprofessional teamwork skills to health professional students: A scoping review. J. Interprof. Care 32, 127–135. doi: 10.1080/13561820.2017.1399868
Fusaroli, R., Bjørndahl, J. S., Roepstorff, A., and Tylén, K. (2016). A heart for interaction: Shared physiological dynamics and behavioral coordination in a collective, creative construction task. J. Exp. Psychol. Hum. Percept. Perform. 42, 1297–1310. doi: 10.1037/xhp0000207
Guastello, S. J., and Peressini, A. F. (2017). Development of a synchronization coefficient for biosocial interactions in groups and teams. Small Group Res. 48, 3–33. doi: 10.1177/1046496416675225
Halbig, A., Babu, S. K., Gatter, S., Latoschik, M. E., Brukamp, K., and von Mammen, S. (2022). Opportunities and challenges of virtual reality in healthcare—a domain experts inquiry. Front. Virtual Reality 3:837616. doi: 10.3389/frvir.2022.837616
Hałgas, E., van Eijndhoven, K., Gevers, J., Wiltshire, T., Westerink, J., and Rispens, S. (2022). A review of using wearable technology to assess team functioning and performance. Small Group Res. 54, 41–76. doi: 10.1177/10464964221125717
Hamilton, A. L., Kerins, J., MacCrossan, M. A., and Tallentire, V. R. (2019). Medical Students’ Non-Technical Skills (Medi-StuNTS): Preliminary work developing a behavioural marker system for the non-technical skills of medical students in acute care. BMJ Simulat. Technol. Enhanc. Learn. 5, 130–139. doi: 10.1136/bmjstel-2018-000310
He, W., Jiang, X., and Zheng, B. (2021). Synchronization of pupil dilations correlates with team performance in a simulated laparoscopic team coordination task. Simulat. Healthcare J. Soc. Simulat. Healthcare 16, e206–e213. doi: 10.1097/SIH.0000000000000548
Heinemann, G., and Zeiss, A. (Eds.) (2002). “A model of team performance,” in Team performance in health care: Assessment and development. (Kluwer Academic/Plenum Publishers), 29–42.
Hughes, A. M., Hancock, G. M., Marlow, S. L., Stowers, K., and Salas, E. (2019). Cardiac measures of cognitive workload: a meta-analysis. Hum. Factors 61, 393–414. doi: 10.1177/0018720819830553
Järvelä, S., Kivikangas, J. M., Kätsyri, J., and Ravaja, N. (2014). Physiological linkage of dyadic gaming experience. Simul. Gaming 45, 24–40. doi: 10.1177/1046878113513080
Jeffcott, S. A., and Mackenzie, C. F. (2008). Measuring team performance in healthcare: review of research and implications for patient safety. J. Crit. Care 23, 188–196. doi: 10.1016/j.jcrc.2007.12.005
Kazi, S., Khaleghzadegan, S., Dinh, J. V., Shelhamer, M. J., Sapirstein, A., Goeddel, L. A., et al. (2021). Team physiological dynamics: a critical review. Hum. Factors 63, 32–65. doi: 10.1177/0018720819874160
Kolbe, M., Burtscher, M. J., Wacker, J., Grande, B., Nohynkova, R., Manser, T., et al. (2012). Speaking up is related to better team performance in simulated anesthesia inductions: An observational study. Anesth. Analg. 115, 1099–1108. doi: 10.1213/ANE.0b013e318269cd32
Kozlowski, S., Chao, G. T., Grand, J. A., Braun, M. T., and Kuljanin, G. (2013). Advancing multilevel research design: capturing the dynamics of emergence. Organ. Res. Methods 16, 581–615. doi: 10.1177/1094428113493119
Kozlowski, S., and Klein, K. (Eds.) (2000). “A multilevel approach to theory and research in organizations: Contextual, temporal, and emergent processes” in Multi-Level Theory, Research, and Methods in Organizations: Foundations, Extensions, and New Directions. (San Francisco, CA: Jossey-Bass), 3–90.
Kuckartz, U. (2019). “Qualitative text analysis: a systematic approach” in Compendium for Early Career Researchers in Mathematics Education. eds. G. Kaiser and N. Presmeg (Cham: Springer International Publishing), 181–197.
Luigi Ingrassia, P., Ragazzoni, L., Carenzo, L., Colombo, D., Ripoll Gallardo, A., and Della Corte, F. (2015). Virtual reality and live simulation: a comparison between two simulation tools for assessing mass casualty triage skills. Eur J Emerg Med 22, 121–127. doi: 10.1097/MEJ.0000000000000132
Mahanama, B., Jayawardana, Y., Rengarajan, S., Jayawardena, G., Chukoskie, L., Snider, J., et al. (2022). Eye movement and pupil measures: a review. Front. Comput. Sci. 3:733531. doi: 10.3389/fcomp.2021.733531
Maloney, S., and Haines, T. (2016). Issues of cost-benefit and cost-effectiveness for simulation in health professions education. Adv. Simul 1:13. doi: 10.1186/s41077-016-0020-3
Manser, T. (2009). Teamwork and patient safety in dynamic domains of healthcare: a review of the literature. Acta Anaesthesiol. Scand. 53, 143–151. doi: 10.1111/j.1399-6576.2008.01717.x
Marlow, S., Bisbey, T., Lacerenza, C., and Salas, E. (2018). Performance measures for health care teams: a review. Small Group Res. 49, 306–356. doi: 10.1177/1046496417748196
Misal, V., Akiri, S., Taherzadeh, S., McGowan, H., Williams, G., Jenkins, J. L., et al. (2020). “Physiological synchrony, stress and communication of paramedic trainees during emergency response training” in ICMI 2020 Companion—Companion Publication of the 2020 International Conference on Multimodal Interaction, 82–86.
Murray, D., and Enarson, C. (2007). Communication and teamwork: essential to learn but difficult to measure. Anesthesiology 106, 895–896. doi: 10.1097/01.anes.0000265145.40825.ac
Parker, C., Scott, S., and Geddes, A. (2019). Snowball Sampling. SAGE Research Methods Foundations. Available at: http://methods.sagepub.com/foundations/snowball-sampling
Pérez, P., Madsen, J., Banellis, L., Türker, B., Raimondo, F., Perlbarg, V., et al. (2021). Conscious processing of narrative stimuli synchronizes heart rate between individuals. Cell Rep. 36:109692. doi: 10.1016/j.celrep.2021.109692
Pijeira Díaz, H. J., Drachsler, H., Järvelä, S., and Kirschner, P. (2016). Investigating Collaborative Learning Success With Physiological Coupling Indices Based on Electrodermal Activity. New York, NY, United States: Association for Computing Machinery, 73.
Pronovost, P., Needham, D., Berenholtz, S., Sinopoli, D., Chu, H., Cosgrove, S., et al. (2006). An intervention to decrease catheter-related bloodstream infections in the ICU. N. Engl. J. Med. 355:26. doi: 10.1056/NEJMoa061115
Rojo López, A. M., Foulquié-Rubio, A.-I., Espín López, L., and Martínez Sánchez, F. (2021). Analysis of speech rhythm and heart rate as indicators of stress on student interpreters. Perspectives 29, 591–607. doi: 10.1080/0907676X.2021.1900305
Rosen, M. A., Weaver, S. J., Lazzara, E. H., Salas, E., Wu, T., Silvestri, S., et al. (2010). Tools for evaluating team performance in simulation-based training. J. Emerg. Trauma Shock 3, 353–359. doi: 10.4103/0974-2700.70746
Rosenman, E. D., Dixon, A. J., Webb, J. M., Brolliar, S., Golden, S. J., Jones, K. A., et al. (2018). A Simulation-based Approach to Measuring Team Situational Awareness in Emergency Medicine: A Multicenter, Observational Study. Academic Emergency Medicine. Off. J. Soc. Acad. Emerg. Med. 25, 196–204. doi: 10.1111/acem.13257
Salas, E., and Cannon-Bowers, J. A. (1997). “Methods, tools, and strategies for team training” in Training for a Rapidly Changing Workplace: Applications of Psychological Research. Eds. M. A. Quiñones and A. Ehrenstein (American Psychological Association), 249–279.
Salas, E., and Cannon-Bowers, J. (2001). The science of training: a decade of progress. Annu. Rev. Psychol. 52, 471–499. doi: 10.1146/annurev.psych.52.1.471
Salas, E., DiazGranados, D., Klein, C., Burke, C. S., Stagl, K. C., Goodwin, G. F., et al. (2008a). Does team training improve team performance? A meta-analysis. Hum. Factors 50, 903–933. doi: 10.1518/001872008X375009
Salas, E., Reyes, D., and Woods, A. (2017). “The assessment of team performance: Observations and needs,” in Innovative assessment of collaboration. Eds. A. A. von Davier, M. Zhu, and P. C. Kyllonen (Springer International Publishing), 21–36.
Salas, E., Rosen, M. A., Burke, C. S., and Goodwin, G. F. (2008b). “The wisdom of collectives in organizations: an update of the teamwork competencies” in Team effectiveness in complex organizations: Cross-disciplinary perspectives and approaches. Eds. E. Salas, G. F. Goodwin, and C. S. Burke (Routledge/Taylor & Francis Group), 39–79.
Schmutz, J., and Manser, T. (2013). Do team processes really have an effect on clinical performance? A systematic literature review. Br. J. Anaesth. 110, 529–544. doi: 10.1093/bja/aes513
Schmutz, J., Meier, L. L., and Manser, T. (2019). How effective is teamwork really? The relationship between teamwork and performance in healthcare teams: a systematic review and meta-analysis. BMJ Open 9:e028280. doi: 10.1136/bmjopen-2018-028280
Stuldreher, I. V., Thammasan, N., van Erp, J. B. F., and Brouwer, A.-M. (2020). Physiological Synchrony in EEG, Electrodermal Activity and Heart Rate Detects Attentionally Relevant Events in Time. Front. Neurosci. 14:575521. doi: 10.3389/fnins.2020.575521
Stuldreher, I. V., van Erp, J. B. F., and Brouwer, A.-M. (2023). Robustness of physiological synchrony in wearable electrodermal activity and heart rate as a measure of attentional engagement to movie clips. Sensors 23. doi: 10.3390/s23063006
Teuma Custo, R., and Trapani, J. (2020). The impact of rapid response systems on mortality and cardiac arrests – A literature review. Intens. Critic. Care Nurs. 59:102848. doi: 10.1016/j.iccn.2020.102848
Tschan, F., Semmer, N. K., Gurtner, A., Bizzari, L., Spychiger, M., Breuer, M., et al. (2009). Explicit reasoning, confirmation bias, and illusory transactive memory: a simulation study of group medical decision making. Small Group Res. 40, 271–300. doi: 10.1177/1046496409332928
Tschan, F., Semmer, N., Vetterli, M., Gurtner, A., Hunziker, S., and Marsch, S. (2011). “Developing observational categories for group process research based on task and coordination requirement analysis: examples from research on medical emergency-driven teams,” in Coordination in Human and Primate Groups. Eds. M. Boos, M. Kolbe, P. Kappeler, and T. Ellwart (Berlin, Heidelberg: Springer), 93–115.
van Eijndhoven, K. H. J., Wiltshire, T. J., Hałgas, E. A., and Gevers, J. M. P. (2023). A computational approach to examining team coordination breakdowns during crisis situations. J. Cogn. Engineer. Decis. Making 17, 256–278. doi: 10.1177/15553434231156417
Weaver, S. J., Dy, S. M., and Rosen, M. A. (2014). Team-training in healthcare: a narrative synthesis of the literature. BMJ Qual. Saf. 23, 359–372. doi: 10.1136/bmjqs-2013-001848
Zoom Video Communications Inc. (2016). Security guide. Zoom Video Communications Inc. Available at: https://d24cgw3uvb9a9h.cloudfront.net/static/81625/doc/Zoom-Security-White-Paper.pdf
Keywords: team performance, objective measures, healthcare, medical simulation training, performance assessment
Citation: Wespi R, Birrenbach T, Schauber SK, Manser T, Sauter TC and Kämmer JE (2023) Exploring objective measures for assessing team performance in healthcare: an interview study. Front. Psychol. 14:1232628. doi: 10.3389/fpsyg.2023.1232628
Edited by:
Evangelos Himonides, University College London, United KingdomReviewed by:
Katelynn A. Kapalo, National Fire Academy, United StatesElizabeth Austin, Macquarie University, Australia
Copyright © 2023 Wespi, Birrenbach, Schauber, Manser, Sauter and Kämmer. This is an open-access article distributed under the terms of the Creative Commons Attribution License (CC BY). The use, distribution or reproduction in other forums is permitted, provided the original author(s) and the copyright owner(s) are credited and that the original publication in this journal is cited, in accordance with accepted academic practice. No use, distribution or reproduction is permitted which does not comply with these terms.
*Correspondence: Rafael Wespi, cmFmYWVsLndlc3BpQGV4dGVybi5pbnNlbC5jaA==
‡ORCID: Rafael Wespi, https://orcid.org/0000-0002-3416-3938
Tanja Birrenbach, https://orcid.org/0000-0002-3046-0900
Stefan K. Schauber, https://orcid.org/0000-0002-1832-2732
Tanja Manser, https://orcid.org/0000-0002-5852-8735
Thomas C. Sauter, https://orcid.org/0000-0002-6646-5789
Juliane E. Kämmer, https://orcid.org/0000-0001-6042-8453
†These authors have contributed equally to this work and share last authorship