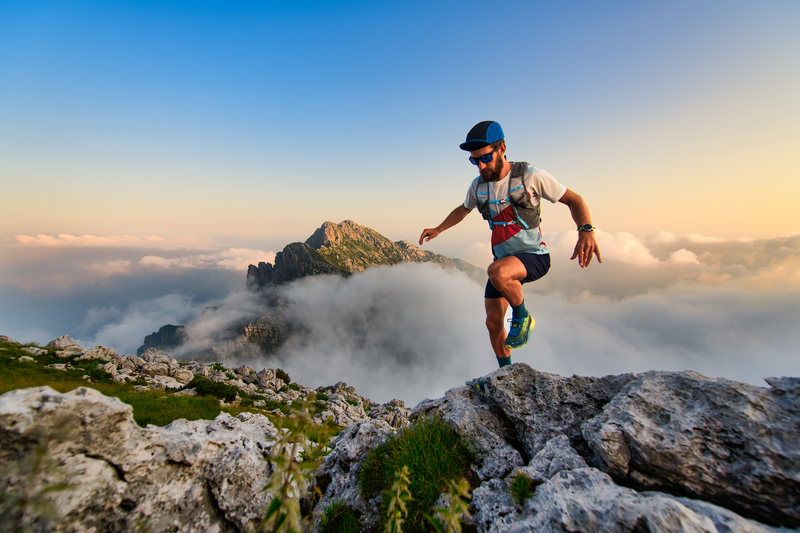
95% of researchers rate our articles as excellent or good
Learn more about the work of our research integrity team to safeguard the quality of each article we publish.
Find out more
ORIGINAL RESEARCH article
Front. Psychol. , 31 January 2024
Sec. Performance Science
Volume 15 - 2024 | https://doi.org/10.3389/fpsyg.2024.1324100
This article is part of the Research Topic Motivation in Learning and Performance in the Arts and Sports View all 11 articles
Introduction: This study aimed to investigate the Self-Regulated Learning behaviors of advanced Brazilian and Portuguese musicians and how these processes vary in terms of gender, nationality, musical instrument, quantity of practice, expertise, and professional experience.
Methods: 300 participants fully completed the 22-item questionnaire “Attitudes in music practice”. The sample comprised of 54.3% males, 44.0% females, and 1% non-binary; 0.7% did not respond. 68.0% (n = 204) were Brazilian, and 32.0% (n = 96) were Portuguese. The mean age was 32.70 years old (SD = 11.261), the mode was 22 years old, with a range of 18 to 66 years. Data analysis procedure included exploratory factor analysis, internal consistency, independent sample t test, analysis of variance (ANOVA), and chi-square tests.
Results: Exploratory Factor Analysis generated three factors: Practice Organization, Personal Resources, and External Resources. The results report there are no differences in SRL scores in terms of gender, nationality, and musical instrument. However, One-way ANOVA test results convey differences in SRL scores and the quantity of practice and expertise with those musicians who reported practicing for longer periods scoring more highly than participants who declared spending less time on daily practice.
Discussion: The results for the expertise variables suggest that more experienced and older musicians scored higher in Personal Resources and lower in External Resources indicating that, as musicians gain in experience, their metacognitive processes become more evident than the social factors of their performance.
Musicians who achieve high levels of proficiency invest a significant amount of their time practicing their instrument and continue to train in order to maintain excellent performance levels. However, the quality of this practice is of utmost importance as the outcomes do not solely depend on the quantity of hours invested (Ericsson et al., 1993; Williamon and Valentine, 2000; Byo and Cassidy, 2008; Bonneville-Roussy and Bouffard, 2015). Research into musical practice has focused on investigating the factors rendering practice efficiency beyond quantity of practice, discussing processes such as how to set personal goals, sustain focus, and persevere in error correction as well as how best to address challenging musical sections (Ericsson et al., 1993; Araújo, 2016; How et al., 2022).
A comprehensive review of music practice research from 1928 to 2020, conducted by How et al. (2022), demonstrates the influence of psychological methodologies on this domain. Their analysis reveals that popular topics and extensively cited articles revolve around psychological constructs such as deliberate practice, motivation, and self-regulation. The role of self-regulation, defined by Zimmerman (2000), p. 14 as “self-generated thoughts, feelings, and actions that are planned and cyclically adapted to the attainment of personal goals” in learning processes, may be temporally distributed as described in Zimmerman’s cyclical model (Zimmerman, 2000; Zimmerman and Campillo, 2003). Zimmerman’s model comprises three phases: Forethought, Performance/Volitional Control, and Reflection. In the Forethought phase, learners employ various actions and strategies for task analysis and goal setting. This is motivated by their self-efficacy beliefs, outcome expectations, and perceived value of the task. Self-control and self-observation are essential during the Performance/Volitional Control phase for the proper employment of learning strategies. This includes focus maintenance, self-monitoring, and other metacognitive subprocesses. During the third phase, Self-Reflection, the learner conducts self-evaluation and manages self-reactions. These self-reflective conclusions feed into the next learning cycle, influencing the subsequent Forethought phase of the model (Zimmerman, 2000).
The model was adapted to music learning and performance by McPherson and Zimmerman (2002, 2011) and extensively applied to music research in order to investigate metacognitive aspects of music practice and performance (How et al., 2022). Even advanced musicians can benefit from SRL strategies and improve their musical performance and daily practice (Clark and Williamon, 2011; Pike, 2017; López-Íñiguez and McPherson, 2020).
From a cognitive point of view, the most conventional definition of a musician is someone who has the ability to play an instrument or sing, this ability being acquired through years of practice (Hallam, 2010; Zhang et al., 2020). Thus, most studies consider the amount of deliberate practice, which is formal and supervised by a teacher, as a primary factor in the development of musical expertise. In Western music, this type of practice occurs mainly in conservatories and higher education music institutions.
According to previous literature (Ericsson et al., 1993), advanced musicians were defined as those who had at least 10 years of experience with their main instrument. However, as research in musical practice has increased, musicians enrolled in higher education institutions, such as universities, professional conservatories and other tertiary institutions, who are preparing to become professional musicians or already working professionally, have been also designated as advanced musicians (Papageorgi et al., 2010; Araújo, 2016). In this study, we will use “advanced musicians” to refer to these individuals. Advanced musicians exhibit practice skills similar to deliberate practice, engage in a focused manner during practice, and have received adequate training to organize their practice based on the technical, theoretical and interpretative aspects of music (Williamon and Valentine, 2000; Miksza, 2015). These musicians have also been described as elite musicians (De Bruin, 2019; Kegelaers and Oudejans, 2020; Mornell et al., 2020), expert musicians (De Bruin, 2017; Fasano et al., 2020), undergraduate musicians (Clark and Williamon, 2011; Zhukov, 2012; McPherson et al., 2019), professional musicians (Dos Santos and Gerling, 2011; Pike, 2017), and college musicians (Kim, 2010; Boucher et al., 2020, 2021).
Regarding the development of musical expertise, it is possible to consider it in relationship with the training period in higher music education. Before entering the higher education course, there is a specific test to assess the minimum musical skills required to enter the course, skills usually developed through years of practice in music schools and conservatories prior to university. Placement in the professional job market usually occurs after completing an undergraduate course in musical performance (Creech et al., 2008).
Developing musical skills is a comprehensive journey involving a combination of theoretical and practical experiences, with relevant aspects such as a first solid foundation in the theoretical and practical foundations of music, including the study of music theory, sheet music reading, counterpoint, harmony, musical analysis, history of music and constant auditory training through music perception classes. During this time, students also have constant instrument lessons and opportunities to play solo and in an ensemble, a period that culminates with the first public audition. Up to this point, we consider naming the category as students, being those who have not yet performed the mid-course recital. The mid-course recital already has a public character and is normally the first public performance of repertoire prepared under the guidance of a specialist teacher.
After the mid-course recital, musicians can be considered pre-professionals because they are already in the final year of their undergraduate course, in preparation for the final recital. Following the rationale, individuals who finished their undergraduate music course were considered professionals, as they were, by definition, ready for the job market. This categorization is justified as a way of expanding the current notion of musical expertise development, and it is the one we used in the present study.
The literature has extensively studied the quantitative measuring of SRL behaviors in advanced musicians, especially in the last decade (Ritchie and Williamon, 2013; Bonneville-Roussy and Bouffard, 2015; Miksza and Tan, 2015; Araújo, 2016; Ersozlu et al., 2017; Hatfield et al., 2017; Volioti and Williamon, 2017; Topoğlu and Topoğlu, 2018; Boon, 2020; Peistaraite and Clark, 2020; Nusseck and Spahn, 2021; Liu, 2023a). A recent review on musical practice by How et al. (2022) reports that 66.2% of the retrieved articles applied quantitative methods, with questionnaires emerging as the most common instrument type (47.4%). Initially, descriptive-correlational studies in this field employed instruments developed for general education or adaptations of these instruments, such as the Pintrich and de Groot Motivated Strategies for Learning Questionnaire (MSLQ) (Pintrich and De Groot, 1990), applied by McPherson and McCormick (1999, 2000), and Nielsen (2004, 2012) or the Self-Regulated Learning Interview Schedule (Zimmerman and Martinez-Pons, 1986), used by Clark and Williamon (2011) and Ritchie and Williamon (2013). However, music practice also involves specific behaviors and task-related demands, which prompted the need to develop a measurement instrument tailored to SRL processes related to musical learning (Miksza, 2012; Araújo, 2016).
The Self-Regulated Practice Behavior scale was designed and validated (α = 0.76 to 0.90) for measuring the self-regulatory behaviors of beginner and intermediate musicians (Miksza, 2012). This scale has since been translated and validated in Turkish (Ersozlu and Miksza, 2015; α =0.62 to 0.90), Portuguese (Madeira et al., 2018; α = 0.71 to 0.84), and Chinese (Zhang et al., 2023; α = 0.77 to 0.86). Subsequently, Araújo (2016) designed the “Attitudes and Sensations in Music Practice” questionnaire for advanced musicians to assess SRL behaviors and Flow sensations in advanced musicians, which has since been validated and applied in both Portuguese and English (α = 0.86). However, considering the best practices based on recent evidence (Costello and Osborne, 2005; Worthington and Whittaker, 2006; Howard, 2016; Rogers, 2022), we deem it necessary to replicate Araújo’s study, updating the validation procedures of the “Attitudes in Music Practice” scale (Araújo, 2016) as regards Exploratory Factorial Analysis, before conducting Confirmatory Factor Analysis (CFA), to avoid model specification errors. These updates will be described in section 2.4.
Quantitative studies have investigated if there are differences or relationships between SRL processes and variables like quantity of practice (Ritchie and Williamon, 2013; Bonneville-Roussy and Bouffard, 2015; Araújo, 2016; Topoğlu and Topoğlu, 2018; Boon, 2020; Zhang et al., 2023), gender (Topoğlu and Topoğlu, 2018; Nusseck and Spahn, 2021; Liu, 2023a), musical instrument (Nielsen, 2004; Liu, 2023a), expertise (Boon, 2020; Kaleli, 2021), and age (Bonneville-Roussy and Bouffard, 2015). We did not find evidence of correlations or differences between SRL scores and nationality in the reviewed quantitative studies, besides Araújo’s (2016).
Thus, the purpose of the study is to explore the SRL behaviors of advanced musicians from Brazil and Portugal and how these processes vary according to gender, musical instrument, quantity of practice (measured by hours of practice per day and days of practice per week), expertise (determined from information about the participants’ formal music education), professional experience (measured by years since first public music performance), age, and nationality.
In light of previous findings in the SRL literature, we formulated the following four hypotheses. First, Exploratory Factorial Analysis will identify the same dimensions as the first study by Araújo (2016); second, similar to the first application of the questionnaire (Araújo, 2016), the SRL scores will report no significant differences regarding gender, nationality, and musical instrument; third, advanced musicians who declare spending more hours per day in music practice will score higher in SRL processes; fourth, more experienced participants will score higher in SRL processes.
The Attitudes in Music Practice questionnaire (Araújo, 2016) applied in this study comprises 22 items (see Supplementary material) and was validated both in Portuguese and English. These items were designed to assess various aspects of self-regulated practice behaviors, such as the management and evaluation of practice goals (e.g., ‘I set specific goals for my practice sessions’), time management and physical environment structuring (e.g., ‘I plan the time of my practice sessions’), strategy selection (e.g., ‘I am aware of the strategies that I use during practice’), self-efficacy beliefs (e.g., ‘I am able to achieve my practice goals satisfactorily’), external causal attributions (e.g., ‘I cannot reach my practice goals without the support of some external factors - peers, teachers, materials, environment’), help-seeking (e.g., ‘I request help from others [teachers, peers, composers, musicologists and specialists]’) and external resources (e.g., ‘I seek information from several sources - books, CDs, videos, internet, biographies, arts, etc. to support my study’).
Participants were required to rate their self-regulated practice behaviors on a 5-point Likert-type scale, based on the frequency of behaviors, ranging from 1-never to 5-always in some items, and level of agreement ranging from 1-completely disagree to 5-completely agree in other items (see Appendix for the complete questionnaire). The questionnaire also included a demographic data section, which requested information about the participants’ age, gender, nationality, formal education, musical instrument, practice time (hours of practice per day and days of practice per week), and how many years from their first public musical performance.
The questionnaire and the Formal Consent form (approved by the local Ethics Committee) were hosted by the Lime Survey platform, managed by the University of Aveiro, and with completed responses submitted through a hyperlink. Prior to its dissemination, we asked 9 SRL researchers and advanced musicians to answer the questionnaire between January 8 and January 9, 2021 to identify eventual difficulties in understanding experienced by participants. The responses returned by the pilot study were removed from the platform to protect the final data.
Data collection occurred during the periods of lockdown in Portugal and Brazil and all contact with participants was therefore virtual. The researchers prepared two invitations to participate in this research project, one addressed to teachers from higher education institutions, distributed by email, and the other for musicians in general and for students attending these institutions, which was disseminated by e-mail and social media in order to attract a wide range of participants.
The questionnaire link was active from January 10, 2021 until May 7, 2021. 476 people answered the questionnaire; only 306 answered the complete questionnaire correctly.
The inclusion criteria consisted of Brazilian and Portuguese advanced musicians. Based on this criteria, we removed six participants because they were under 18 years old and had limited musical instrument experience. The final sample consisted of 300 participants (54.3% male, 44.0% female, 1% non-binary, and 0.7% did not respond). 68.0% (n = 204) of the participants were Brazilian, and 32.0% (n = 96) were Portuguese.
Their ages ranged from 18 to 66 years old, with a mean of 32.70 years old (SD = 11.261), mode 22 years old; with most participants in the age range between 26 and 35 years of age (n = 104, more in Table 1).
Since the educational system in Brazil differs from Portugal, we organized this information from participants into categories of expertise: participants in their first 2 years of professional music education (undergraduate studies) were allocated to the Student category. Participants in their final year of undergraduate studies were classified as Pre-professionals, and participants who had completed undergraduate studies and/or undertook graduate studies were allocated to the Professional category.
The descriptive results identify how 54% of participants fell into the Professional category. Table 2 presents the results for this variable.
Regarding years of experience since their first public concert, the majority of participants (76.0%) declared having 10 or more years of professional performance experience and with the majority beginning their instrument lessons when they were 12 years old or younger (56.7%).
As regards musical instruments, 49.7% of participants declared playing plucked string instruments (n = 149), 15.3% wind instruments (n = 46), 12.7% bowed strings (n = 38), 10.7% keyboards (n = 32), 8% voice (n = 24), 1.7% percussion (n = 5) and 1.7% conductors (n = 5). Due to analysis requirements regarding the minimum number of participants in each category (Field, 2018), percussionists and conductors were not included in the inferential analysis. One participant did not register information about the instrument (Table 3).
When asked about the practice quantity, participants answered how many days per week they practiced, and how much time per day (in hours). 13.3% declared practicing 1 to 2 days per week, 23.2% practiced 3 to 4 times per week, 37.3% of participants (n = 112) declared practicing 5 to 6 days a week, and 26.0% (n = 78) practiced daily. The majority of participants declared practicing between 1 and 2 h per day (27.7%). Table 4 reports the length of daily practice hours.
Exploratory Factor Analysis (EFA) served to explore the structure of the scale and assess its internal reliability. The dispersion matrix was generated by polychoric correlations (Muthén and Kaplan, 1985, 1992; Baglin, 2014). Researchers suggest employing polychoric correlations when conducting EFA on data derived from ordinal variables (Baglin, 2014). We assessed sampling adequacy and factorability according to the Kaiser-Meyer-Olkin (KMO) index and significance by Bartlett’s test of sphericity (Rogers, 2022). The extraction method adopted was Robust Diagonally Weighted Least Squares (RDWLS–Asparouhov and Muthen, 2010). This estimator is most suitable for categorical data and is robust in handling deviations from normality (DiStefano and Morgan, 2014). To determine the appropriate number of factors, we deployed parallel analysis with random permutation of observed data (Timmerman and Lorenzo-Seva, 2011; Baglin, 2014) that has proven to be more effective than traditional methods in accurately determining the actual number of dimensions (Timmerman and Lorenzo-Seva, 2011; Baglin, 2014). Items with factor loadings ≥0.30 were considered relevant and included in the model. We implemented the Robust Promin rotation method (Lorenzo-Seva and Ferrando, 2019). It is advisable to select oblique rotation methods for multidimensional scales, as most factors within these scales tend to have some level of interrelation, and orthogonal rotations presume that the factors are independent (Fabrigar et al., 1999; Fabrigar and Weneger, 2011; Baglin, 2014; Lloret-Segura et al., 2014; Howard, 2016). Model adequacy was assessed by the Root Mean Square Error of Approximation (RMSEA), Comparative Fit Index (CFI), and Non-Normed Fit Index - Tucker-Lewis Index (NNFI). According to the literature (Fabrigar et al., 1999; Brown, 2015), the RMSEA values should be less than 0.08, and the CFI and NNFI results should be above 0.90. EFA was performed using the software FACTOR version 12 (Lorenzo-Seva and Ferrando, 2006; Ferrando and Lorenzo-Seva, 2017; Rogers, 2022).
We tested the internal consistency of each factor by McDonald’s Omega coefficients (Hayes and Coutts, 2020) and the Composite Reliability Index (Raykov, 1997; Valentini and Damásio, 2016; with ≥0.60 considered satisfactory). These calculations were performed by Jasp software (version 0.16.4) for McDonald’s Omega, and the Composite Reliability Calculator for the Composite Reliability Index. We did not apply Cronbach’s alpha coefficient due to current discussions questioning its suitability for the types of data and models deployed in psychological research, which often violate the assumptions made by this coefficient (McNeish, 2018; Hayes and Coutts, 2020). Especially in scales with a smaller number of items, Cronbach’s alpha may report reliability lower than the scale actually attains (Zinbarg et al., 2006; McNeish, 2018).
An independent sample t-test was conducted to investigate how the “Attitudes in Music Practice” scale scores differed between groups, according to Gender and Nationality. The assumption of homogeneity of variance was evaluated using Levene’s test and, when violated, we applied Welch’s statistic. Bootstrapping procedures (1,000 re-samples; 95% IC BCa) were performed to correct for the non-normality of the sample distribution and to increase the reliability of the results (Tan and Tan, 2010). We calculated the effect size according to Hedges g to account for bias in unbalanced samples in keeping with Cohen’s benchmarks (Cohen, 1988); g = 0.2, 0.5, and 0.8 correspond to small, medium, and large effects (Lakens, 2013). This data analysis made recourse to IBM SPSS Statistics software (Version 28).
One-way ANOVA evaluated the potential differences in musical practice attitudes based on the scores of the three dimensions (Practice Organization, Personal Resources, External Resources) within group variables: instrument, practice hours per day, days of practice per week, number of concerts per year, expertise, and age. The assumption of variance homogeneity was assessed using Levene’s test, and post-hoc evaluation was performed using the Bonferroni correction for multiple comparisons (Field, 2018). We then applied bootstrapping procedures (1,000 re-samplings; 95% IC BCa) to obtain a higher level of result reliability, correcting any normality deviations from the sample distribution and returning more robust confidence intervals for the mean differences (Haukoos and Lewis, 2005; Tan and Tan, 2010). The coefficient ω2 represented effect size, according to Cohen’s benchmarks (Cohen, 1988, 0.01 = small; 0.06 = medium; 0.14 = large). We made recourse to IBM SPSS Statistics (Version 28) as the statistical software for this analysis.
We assessed the relationships among the categorical variables by the chi-square test of association. These relationships were deemed significant when the adjusted residuals were > 1.96 (regardless of sign).
We examined the dataset to identify any inconsistent and/or missing values related to participant responses to scale items with no such inconsistencies detected. However, we excluded from this analysis participants who did not respond to all the scale items. Therefore, for analyses related to the internal structure of the instrument, the total sample size was n = 297. Table 5 presents the univariate descriptive analysis for the 22 original items of the “Attitudes in Musical Practice” scale.
The first stage of analysis included all the 22 scale items. Bartlett’s sphericity test returned a significant result [χ2 = 3219.2 (df = 231; p = 0.000010)] and the KMO measure (0.85772) was also above that recommended (Howard, 2016). The robust goodness of fit statistics were satisfactory (RMSEA = 0.062; NNFI; Tucker and Lewis = 0.963; CFI = 0.970) with the parallel analysis indicating the extraction of two factors. According to the two-factor model, all items achieved a relevant factor loading (≥ 0.30); however, items 6 (“I use specific strategies related to my practice goals”) and 17 (“I request help from others teachers, peers, composers, musicologists and specialists”) reported cross loading (see Supplementary Table S1).
We carried out further analysis to undertake the extraction of the three dimensions based on Araújo’s preliminary study from 2016. The three-factor model maintained the Bartlett [χ2 = 3219.2 (df = 231; p = 0.000010)] and KMO (0.85772) results, and the robust goodness of fit indices returned improvements (RMSEA = 0.043; NNFI; Tucker and Lewis = 0.982; CFI = 0.987). Item 17 (“I request help from others - teachers, peers, composers, musicologists and specialists”) loaded above 0.30 on only one dimension. However, item 6 still presented cross loading (Supplementary Table S2).
The model also reflects theoretical inconsistency in the distribution of items across factors. Item 5 (“I understand that my goals are challenging”) was allocated to factor 3, which groups items related to the External Factors dimension. This distribution would thus hinder the discussion of this dimension as a whole. Therefore, items 5 and 6 (“I use specific strategies related to my practice goals”) were excluded and we performed a new EFA.
Both Bartlett’s test of sphericity and the KMO results were acceptable [χ2 = 2851.7 (df = 210; p = 0.000010); KMO = 0.85718]. The robust goodness of fit indices demonstrated a better fit than the previous models: (RMSEA = 0.037; NNFI; Tucker and Lewis = 0.986; CFI = 0.990). Table 6 displays the factorial loadings of this updated model.
Thus, Factor 1 comprises the items related to Practice Organization processes that incorporate the behavioral determinants of SRL (Zimmerman, 1989). Factor 2, designated Personal Resources by Araújo (2016), gathers those processes associated with personal determinants, and Factor 3, External Resources, refers to influences from the surrounding environment, which is the third determinant described by Zimmerman (1989). This analysis returns extraction scores similar to those of Araújo’s (2016) primary study. Thus, analyses below will not contain items 5 and 6.
Factor 1 internal consistency resulted in McDonald’s ω = 0.90; [CI 95% (0.88–0.91)], composite reliability = 0.918; Factor 2 ω = 0.78 [CI 95% (0.75–0.88)], composite reliability = 0.841; and Factor 3 ω = 0.93 [CI 95% (0.93–0.94)], composite reliability = 0.953.
Participants obtained a mean score of 30.13 (SD = 5.93) in Practice Organization, 28.69 (SD = 3.93) in Personal Resources, and 18.98 (SD = 3.22) in External Resources. Supplementary Table S17 contains this data and total scores.
The T test results for gender return no significant differences in the SRL scores between groups across any of the dimensions [Practice Organization: t (291.802) = −1.509 p = 0.132 IC 95% Bca: −2.36; 0.343; Personal Resources: t (292) = 1.069, p = 0.286 (IC 95% Bca: −0.401; 1.424); External Resources: t (291.366) = −0.937, p = 0.350 (IC 95% Bca: −1.023; 0.345)]. These descriptive statistics are made available in Supplementary Table S3. Considering the low sample size of participants in the non-binary category, we were compelled to conduct the test only with the male and female categories.
The SRL scores when organized by nationality also presented no significant differences according to the T test results [Practice Organization: t (295) = −0.155 p = 0.877 (IC 95% Bca: −1.505; 1.251), Personal Resources: t (295) = −1.257, p = 0.210 (IC 95% Bca: −1.550; 0.302); External Resources: t (223.718) = 0.818, p = 0.415(IC 95% Bca: −0.475; 1.021)]. In turn, Supplementary Table S4 sets out these descriptive statistics.
Levene’s tests describe a homogeneity of variance across every dimension: Practice Organization [Levene (4, 284) = 0.253, p = 0.908], Personal Resources [Levene (4, 283) = 0.624, p = 646], External Resources [Levene (4, 284) = 1.744, p = 140].
The ANOVA results for differences between musical instruments and SRL were also statistically non-significant [Practice Organization: F (4, 284) = 0.469, p = 0.758; Personal Resources: F (4, 283) = 1.24, p = 0.290; External Resources: F (4, 284) = 1.10, p = 0.354]. These descriptive statistics are available in Supplementary Table S5.
Levene’s tests report a homogeneity of variance across all dimensions: Practice Organization [Levene, (4, 294) = 0.795, p = 0.529]; Personal Resources [Levene, (4, 292) = 1.053, p = 0.380]; External Resources [Levene, (4, 294) = 1.554, p = 0.187]. The descriptive statistics for all groups and dimensions are set out in Supplementary Table S6 in the Supplementary Material.
The ANOVA results demonstrate significant differences with small size effects between the groups in Practice Organization [F (4, 294) = 3.818, p = 0.005, ω2 = 0.04]. Specifically, there are differences in the mean scores between musicians who practice less than 1 h per day (M = 28.55, SD = 6.05) and those who practice more than 4 h per day (M = 32.77, SD = 5.69) as well as between participants practicing between 1 and 2 h per day (M = 29.17, SD = 6.26) and those practicing more than 4 h per day (M = 32.77, SD = 5.69).
Regarding the External Resources dimension, there are medium size effect differences (F(4, 294) = 7.608, p < 0.001, ω2 = 0.08). These differences emerge when comparing musicians who practice less than 1 h per day (M = 17.09, SD = 3.49) with those who practice within the range of 2 to 3 h (M = 19.44, SD = 3.34) and between 3 and 4 h (M = 20.14, SD = 2.70), as well as with participants practicing over 4 h per day (M = 19.60, SD = 2.52). Additionally, the results also detail differences between participants practicing between 1 and 2 h per day (M = 18.55, SD = 3.03) and those practicing for between 3 and 4 h per day (M = 20.14, SD = 2.70). Supplementary Table S7 describes the post-hoc tests deploying Bonferroni correction and CI through Bootstrapping.
All dimensions display homogeneity of variance: Practice Organization [Levene, (3, 295) = 0.324, p = 0.808]; Personal Resources [Levene, (3, 293) = 2.465, p = 0.062]; External Resources [Levene, (3, 295) = 0.254, p = 0.859] with the complete descriptive statistics set out in Supplementary Table S8 (see Supplementary Material).
The ANOVA results point to small effect differences in the Practice Organization dimension [F(3, 295) = 6.157, p < 0.001, ω2 = 0.049]. In particular, there are significant differences between the categories of musicians who practice between 1 and 2 days per week (M = 26.88, SD = 6.51) and musicians who practice between 5 and 6 days per week (M = 30.83, SD = 5.74) as well as musicians practicing daily (M = 31.35, SD = 5.39).
In the External Resources dimension [F (3, 295) = 9.810, p < 0.001, ω2 = 0.081], there are significant medium size differences between musicians practicing between 1 and 2 days per week (M = 16.95, SD = 3.08) and musicians practicing between 5 and 6 days per week (M = 19.28, SD = 3.04) as well as musicians practicing daily (M = 20.05, SD = 3.37). Differences also appear between musicians practicing between 3 and 4 days per week (M = 18.47, SD = 2.82) and their peers practicing daily (M = 20.05, SD = 3.37). These post-hoc tests appear in Supplementary Table S9.
After excluding the possibility of non-homogeneity of variance across all dimensions [Practice Organization: Levene (2, 296) = 0.412, p = 0.663; Personal Resources: Levene (2, 294) = 0.186, p = 0.831; External Resources: Levene (2, 296) = 1.225 p = 0.295], we conducted a one-way ANOVA test to examine the differences between levels of expertise. The corresponding descriptive results are available in Supplementary Table S10.
The ANOVA results reveal significant differences with a small size effect in the Personal Resources Dimension [F(2, 294) = 5.659, p = 0.004; ω2 = 0.030] between the Pre-Professionals (M = 27.65; SD = 3.62) and the Professionals (M = 29.36; SD = 3.87). In terms of the External Resources dimension, the results convey significant differences with small size effects [F(2, 296) = 8.085, p < 0.001; ω2 = 0.045] between Students (M = 20.21; SD = 3.31) and Professionals (M = 18.35; SD = 2.98). Supplementary Table S11 (in the Supplementary Material) details the post-hoc tests for this variable.
Levene’s test confirms the homogeneity of variance across every dimension [Practice Organization: Levene (2, 296) = 2.475, p = 0.086; Personal Resources: Levene (2, 294) = 1.319, p = 0.269; External Resources: Levene (2, 296) = 0.232 p = 0.793] with the descriptive results provided in Supplementary Table S12 (Supplementary Material).
The ANOVA results report small size differences in the Practice Organization dimension [F(2, 296) = 3.697; p = 0.26; ω2 = 0.018] between participants aged 26 to 35 years (M = 29.03, SD = 6.48) and those aged 36 years or older (M = 31.27, SD = 5.84). The Personal Resources dimension returned differences with a medium size effect [F (2, 294) = 12.333, p < 0.001; ω2 = 0.071] between musicians aged 18 to 25 years (M = 27.88, SD = 4.00) and those aged 36 years or older (M = 30.24, SD = 3.20), as well as between participants aged 26 to 35 years (M = 27.95, SD = 4.09) and those aged 36 years or older (M = 30.24, SD = 3.20). The External Resources dimension displays differences with a medium size effect between participants [F (2, 296) = 12.690, p < 0.001; ω2 = 0.073] aged 18 to 25 years (M = 20.28, SD = 2.89) and those aged 36 years or older (M = 18.21, SD = 3.12). Supplementary Table S13 presents the post-hoc tests.
Levene’s test results convey a homogeneity of variance in each of the three factors [Practice Organization: Levene (2, 296) = 0.030, p = 0.970; Personal Resources: Levene (2, 294) = 1.618, p = 0.200; External Resources: Levene (2, 296) = 0.319, p = 0.727]. These descriptive results are available in Supplementary Table S14 (Supplementary Material).
The ANOVA results display medium sized differences in the Personal Resources dimension [F (2, 294) = 10.412, p < 0.001; ω2 = 0.060] between the “1 to 9 years” group (M = 26.75; SD = 4.47) and the “10 to 29 years” group (M = 28.74; SD = 3.78); between the “1 to 9 years” group and the “30 years or more” group (M = 30.18; SD = 3.29); and between the “10 to 29 years” group and the “30 years or more” group. In the External Resources dimension, we may report medium sized differences [F (2, 296) = 10.547, p < 0.001; ω2 = 0.060] between the “1 to 9 years” category (M = 20.73; SD = 2.88) and the “10 to 29 years” category (M = 18.83; SD = 3.15); and between the “1 to 9 years” category and the “30 years or more” category (M = 17.98; SD = 3.23). The complete post-hoc tests may be found in Supplementary Table S15 (Supplementary Material).
We then carried out a chi-square test of independence (3×5) to investigate the relationship between hours of study and participant expertise levels (student, pre-professional, and professional). The results identify a significant association between practice hours and expertise [χ2(8) = 16.812, p = 0.032, Cramer’s V = 0.16]. Analyses of the adjusted standardized residuals demonstrate that the student category statistically associated with more hours of study (1 to 2 h and 3 to 4 h of study per day). On the other hand, professionals were statistically associated with fewer hours of study (up to 1 h of study per day). Pre-professional participants did not show a significant relationship with any of the practice time categories. Supplementary Table S16 presents these estimates (see Supplementary Material).
This study set out to investigate the differences in the SRL scores achieved by advanced musicians according to age, gender, nationality, musical instrument, quantity of practice, expertise and quantity of professional experience. As described above, 300 participants completed a survey with the resulting data analyzed through parametric statistical tests. The findings report no statistically significant differences among the categories of gender, nationality and musical instrument. These results are consistent with those obtained in the first application of the same instrument (Araújo, 2016). Other studies of a similar design and sample, produced by Bonneville-Roussy and Bouffard (2015), Liu (2023a), Nusseck and Spahn (2021), and Topoğlu and Topoğlu (2018), also failed to encounter any gender differences.
Similar to our study, Nielsen (2004) reports no significant differences between instrument categories. In Liu’s (2023a) survey, brass players reported employing more learning strategies than keyboard players. In our study, woodwind and brass players were included in the same category due to the small sample size. Our results may not point out any significant differences existing as almost half of our sample were plucked string players.
The quantity of practice was measured according to two variables: the number of practice hours per day, and the practice days per week. Regarding the Practice Organization dimension, musicians that declared practicing more hours per day and more days per week obtained higher scores. In the Personal Resources dimension, there are no differences between the SRL scores and the categories of quantity of practice similar to the first application of this scale by Araújo (2016). However, the results for the External Resources dimension did contrast: while musicians who practiced for more than 4 h a day registered lower scores in this dimension (in comparison to participants who practiced between 3 and 4 h), and those who practiced every day of the week reported increased scores (in comparison with participants who practiced less days per week).
The literature has explored the correlation between the quantity of practice time and the self-regulatory behaviors exhibited by advanced musicians. Previous descriptive-correlational studies by Miksza and Tan (2015), Topoğlu and Topoğlu (2018), and Ritchie and Williamon (2013), as well as observational studies by Boon (2020) and the microanalysis study by Miksza et al. (2018), which measured the quantity of practice hours per day, also found that a greater number of practice hours corresponds to higher SRL scores.
However, when relating the quantity of practice hours per day to the expertise variable, the chi-square test of independence (3×5) here demonstrates that students were associated with more hours of practice per day than professionals. Furthermore, professionals were statistically associated with fewer hours of practice (up to 1 h of practice per day). Pre-professional participants did not show a significant relationship with any of the practice time categories.
These results are similar to those of Bonneville-Roussy and Bouffard (2015), Araújo (2016), and Dos Santos and Gerling (2011), suggesting that, as musicians gain more experience, their practice becomes more self-regulated, thereby reducing the practice time necessary to achieving goals. While musicians who practice for extended periods report greater recourse to SRL processes, more experienced musicians claim to practice for less time. Students, who spend more time in the practice room, may simply have more information to report about their strategies. By contrast, professional musicians may spend more time in activities such as rehearsals, performances or teaching (Vellacott and Ballantyne, 2022). Thus, self-regulation eventually serves as a determining factor for individuals to be able to sustain their artistic activities. Studies that measure SRL and time management in advanced musicians portray improvements in time management and enhanced practice efficiency as the main outcomes (Kim, 2010; Clark and Williamon, 2011; Miksza, 2015; Pike, 2017; López-Íñiguez and McPherson, 2020).
The Expertise variable categorized participants into Students, Pre-Professionals, and Professionals. ANOVA analysis identifies significant differences in the Personal Resources dimension, with Professionals obtaining higher scores than Pre-professionals. On the other hand, in the External Resources dimension, Students scored higher than Professionals.
The same differences emerged when musicians were asked to report the number of years since their first public performance. Musicians reporting more years since their first public performance scored higher in the Personal Resources dimension and lower in the External Resources dimension. Similarly, in terms of age, older musicians scored higher in the Personal Resources dimension and lower in the External Resources dimension. Bonneville-Roussy and Bouffard (2015) describe how older participants deploy deliberate practice strategies more frequently than younger participants. These findings suggest that, as musicians gain in experience, their metacognitive processes become more relevant than the social factors of their performance.
The items included in the External Resources dimension encompass processes such as seeking help from others (teachers, peers, composers, musicologists, and specialists) as well as actively searching for other sources of information able to support daily practice, such as books, recordings, videos, the Internet, and social media. In the 2002 article by McPherson and Zimmerman, the first to adapt Zimmerman’s cyclical model to music learning, the authors state they did not find any mention of seeking external resources in the literature on musical practice (McPherson and Zimmerman, 2002). Two decades later, music practice research has advanced (How et al., 2022) and therefore enables discussion of these results. Over the years, other studies have reported that professional musicians rely less on external assistance when preparing for performances, in comparison with students, even among advanced musicians from diverse cultural backgrounds who not only received different musical education but also face different job markets (Nielsen, 2004; Dos Santos and Gerling, 2011; Araújo, 2016; Volioti and Williamon, 2017).
On the other hand, when approaching undergraduate students, studies examining the practices of pre-service music teachers obtain results that portray how more advanced students on this study program employ more help-seeking processes than their peers during the early years of the program (Boon, 2020; Kaleli, 2021). Similarly, microanalysis studies register the greater use of external resources among undergraduate participants with higher music performance scores (Miksza et al., 2018; McPherson et al., 2019; Osborne et al., 2021). This suggests that, even though professionals report minimal usage of strategies related to social factors, this behavior is adopted by students with higher levels of performance evaluation in keeping with how aspiring musicians can benefit from seeking external resources during their practice sessions. This proactive behavior enables them to engage in “modeling, listening, and critical appraisal” (Ritchie and Williamon, 2013) and engage in positive reinforcement through exchanging knowledge with peers (Dos Santos Silva et al., 2023; Liu, 2023b), which are crucial for their growth and attainment of performance excellence, particularly during the learning phase.
The results of this study should be considered in light of its limitations. The sample consisted of volunteers, which may affect the generalizability of the findings and may not fully encompass the variety existing in the population studied. Nevertheless, data anonymity may have mitigated potential sample bias.
Furthermore, SRL processes in descriptive research may reach a broader population but may not accurately reflect actual practice behaviors. Recent studies employing the same questionnaire in structured interviews have indicated that conceptions of SRL processes, such as goal-setting and environment structuring, diverge considerably among advanced musicians (Silva and Fiorini, 2021). Other studies have combined quantitative scales with the observation of SRL processes as they occur, for example in microanalysis studies (Miksza et al., 2018; McPherson et al., 2019; Osborne et al., 2021). Future research might combine large sample surveys with observation applied to a sample subset. Moreover, follow-up studies using quantitative scales could efficiently measure the maintenance of SRL behaviors learned through intervention over time.
The purpose of our study was to evaluate the reliability of a self-regulation measurement scale in Portuguese. Additionally, we sought to gather current information about the practice habits of advanced musicians who study and work in two Portuguese-speaking countries. Our two first hypotheses were confirmed: the EFA results organized the same items into the three dimensions as Araújo (2016) first study, suggesting that the questionnaire is robust for assessing SRL processes. We may also report that there were no significant differences in SRL scores based on gender, nationality or musical instrument. Participants who declared practicing for more time scored higher in the Practice Organization dimension across both variables (hours per day and days per week). In the External Resources dimension, musicians who declared practicing every day of the week scored higher (than all the other categories). However, participants who reported practicing more hours per day then scored lower in this dimension, and that partially confirms hypothesis 3. Lastly, the fourth hypothesis was also partially confirmed. More experienced musicians scored higher in the Personal Resources dimension but lower in the External Resources dimension, based on expertise, age, and years since their first public performance.
The results of this research suggest that SRL constitutes a set of processes that musicians acquire throughout their learning journey and that these interlink with a significant amount of practice time. As these processes become internalized, practicing becomes more efficient and the time required to achieve performance goals decreases. Similarly, the search for assistance and external resources is an expected behavior in the professional development of musicians. As they attain higher levels of professional performance, personal resources surpass their recourse to external factors.
Developing and validating questionnaires tailored to the specificities of music practice should be encouraged as this may improve music teacher diagnosis of just which SRL dimensions their students need to consider most as well as keeping track of positive changes in SRL behaviors. In the present study, we provided psychometric evidences to this instrument; therefore, new studies should be conducted to establish norms that will be used to contextualize individual scores on this test. Future studies may use the questionnaire to collect empirical results and compare scores with new samples in the context of Brazil and Portugal, concerning the total table.
In small samples, from which data may not be generalized, the questionnaire is especially helpful as it may encourage advanced students to stop and reflect on their practice habits (Silva and Fiorini, 2021). Furthermore, future research might further assess the consistency of this SRL measuring scale for the learning processes undertaken by beginner and intermediate musicians.
The datasets presented in this article are not readily available because the raw data supporting the conclusions of this article will be available after the full research is completed. Requests to access the datasets should be directed to Y2FtaWxsYXNpbHZhbXVzaWNhQGdtYWlsLmNvbQ==.
The studies involving humans were approved by UNICAMP–PRÓ-REITORIA DE PESQUISA DA UNIVERSIDADE ESTADUAL DE CAMPINAS–Comitê de Ética em Pesquisa em Ciências Humanas–CHS/UNICAMP. CAAE: 09319219.8.0000.8142. The studies were conducted in accordance with the local legislation and institutional requirements. The participants provided their on-line informed consent to participate in this study.
CS: Data curation, Formal analysis, Funding acquisition, Investigation, Methodology, Software, Validation, Writing – original draft, Writing – review & editing, Conceptualization. MA: Conceptualization, Data curation, Investigation, Methodology, Software, Writing – review & editing, Writing – original draft. HM: Funding acquisition, Supervision, Writing – review & editing, Writing – original draft.
The author(s) declare financial support was received for the research, authorship, and/or publication of this article. This study is part of a larger research funded by the São Paulo Research Foundation (FAPESP), grant #2018/20809-2 and #2019/21481-3 held by CS. Thanks are due to FCT/MCTES for the financial support to INET-md (UIDB/00472/2020), through national funds.
The authors thank Dr. Alex França for providing statistical consultation.
The authors declare that the research was conducted in the absence of any commercial or financial relationships that could be construed as a potential conflict of interest.
All claims expressed in this article are solely those of the authors and do not necessarily represent those of their affiliated organizations, or those of the publisher, the editors and the reviewers. Any product that may be evaluated in this article, or claim that may be made by its manufacturer, is not guaranteed or endorsed by the publisher.
The Supplementary material for this article can be found online at: https://www.frontiersin.org/articles/10.3389/fpsyg.2024.1324100/full#supplementary-material
Araújo, M. V. (2016). Measuring self-regulated practice behaviours in highly skilled musicians. Psychol. Music 44, 278–292. doi: 10.1177/0305735614567554
Asparouhov, T., and Muthen, B. (2010). Simple second order chi-square correction. Mplus Technical Appendix. Available at: https://www.statmodel.com/download/WLSMV_new_chi21.pdf (Accessed September 5, 2023).
Baglin, J. (2014). Improving your exploratory factor analysis for ordinal data: a demonstration using FACTOR. Pract. Assess. Res. Eval. 19, 1–15. doi: 10.7275/DSEP-4220
Bonneville-Roussy, A., and Bouffard, T. (2015). When quantity is not enough: disentangling the roles of practice time, self-regulation and deliberate practice in musical achievement. Psychol. Music 43, 686–704. doi: 10.1177/0305735614534910
Boon, E. T. (2020). Self-regulated learning skills of prospective music teachers in Turkey. Int. J. Music. Educ. 38, 415–430. doi: 10.1177/0255761420909919
Boucher, M., Creech, A., and Dubé, F. (2020). Video feedback and the choice of strategies of college-level guitarists during individual practice. Music. Sci. 24, 430–448. doi: 10.1177/1029864918817577
Boucher, M., Creech, A., and Dubé, F. (2021). Video feedback and the self-evaluation of college-level guitarists during individual practice. Psychol. Music 49, 159–176. doi: 10.1177/0305735619842374
Brown, T. A. (2015). Confirmatory factor analysis for applied research. New York: Guilford Publications.
Byo, J. L., and Cassidy, J. W. (2008). An exploratory study of time use in the practice of music majors. Education 27, 33–40. doi: 10.1177/8755123308322272
Clark, T., and Williamon, A. (2011). Evaluation of a mental skills training program for musicians. J. Appl. Sport Psychol. 23, 342–359. doi: 10.1080/10413200.2011.574676
Costello, A. B., and Osborne, J. (2005). Best practices in exploratory factor analysis: four recommendations for getting the most from your analysis. Pract. Assess. Res. Eval. 10, 1–9. doi: 10.7275/JYJ1-4868
Creech, A., Papageorgi, I., Duffy, C., Morton, F., Haddon, E., Potter, J., et al. (2008). From music student to professional: the process of transition. Br. J. Music Educ. 25, 315–331. doi: 10.1017/S0265051708008127
De Bruin, L. R. (2017). Expert voices in learning improvisation: shaping regulation processes through experiential influence. Music Educ. Res. 19, 384–397. doi: 10.1080/14613808.2016.1204279
De Bruin, L. R. (2019). Improvising musicians’ self-regulation and distributed creativities: A phenomenological investigation. Think Skills Creat. 32, 30–41. doi: 10.1016/j.tsc.2019.03.004
DiStefano, C., and Morgan, G. B. (2014). A comparison of diagonal weighted least squares robust estimation techniques for ordinal data. Struct. Equ. Model. Multidiscip. J. 21, 425–438. doi: 10.1080/10705511.2014.915373
Dos Santos, R. A. T., and Gerling, C. C. (2011). (Dis)similarities in music performance among self-regulated learners: an exploratory study. Music. Educ. Res. 13, 431–446. doi: 10.1080/14613808.2011.632085
Dos Santos Silva, C., Marinho, H., and Fiorini, C. (2023). #100daysofpractice: selection and adaptation of self-regulated learning strategies in an online music performance challenge. Psychol. Music 51, 667–681. doi: 10.1177/03057356221108762
Ericsson, K. A., Krampe, R. T., and Tesch-Römer, C. (1993). The role of deliberate practice in the acquisition of expert performance. Psychol. Rev. 100, 363–406. doi: 10.1037/0033-295X.100.3.363
Ersozlu, Z. N., and Miksza, P. (2015). A Turkish adaptation of a self-regulated practice behavior scale for collegiate music students. Psychol. Music 43, 855–869. doi: 10.1177/0305735614543283
Ersozlu, Z. N., Nietfeld, J. L., and Huseynova, L. (2017). Predicting preservice music teachers’ performance success in instrumental courses using self-regulated study strategies and predictor variables. Music. Educ. Res. 19, 123–132. doi: 10.1080/14613808.2015.1092508
Fabrigar, L. R., and Wegener, D. T. (2011). Exploratory factor analysis. Oxford, UK: Oxford University Press.
Fabrigar, L. R., Wegener, D. T., MacCallum, R. C., and Strahan, E. J. (1999). Evaluating the use of exploratory factor analysis in psychological research. Psychol. Methods 4, 272–299. doi: 10.1037/1082-989X.4.3.272
Fasano, M. C., Glerean, E., Gold, B. P., Sheng, D., Sams, M., Vuust, P., et al. (2020). Inter-subject Similarity of Brain Activity in Expert Musicians After Multimodal Learning: A Behavioral and Neuroimaging Study on Learning to Play a Piano Sonata. Neuroscience 441, 102–116. doi: 10.1016/j.neuroscience.2020.06.015
Ferrando, P. J., and Lorenzo-Seva, U. (2017). Program FACTOR at 10: origins, development and future directions. Psicothema 29, 236–240. doi: 10.7334/psicothema2016.304
Field, A. (2018). Discovering statistics using IBM SPSS statistics. 5th Edn. Thousand Oaks, CA: Sage.
Hallam, S. (2010). 21st century conceptions of musical ability. Psychol. Music 38, 308–330. doi: 10.1177/0305735609351922
Hatfield, J. L., Halvari, H., and Lemyre, P.-N. (2017). Instrumental practice in the contemporary music academy: a three-phase cycle of self-regulated learning in music students. Music. Sci. 21, 316–337. doi: 10.1177/1029864916658342
Haukoos, J. S., and Lewis, R. J. (2005). Advanced statistics: bootstrapping confidence intervals for statistics with “difficult” distributions. Acad. Emerg. Med. 12, 360–365. doi: 10.1197/j.aem.2004.11.018
Hayes, A. F., and Coutts, J. J. (2020). Use omega rather than Cronbach’s alpha for estimating reliability. But…. Commun. Methods Meas. 14, 1–24. doi: 10.1080/19312458.2020.1718629
How, E. R., Tan, L., and Miksza, P. (2022). A PRISMA review of research on music practice. Music. Sci. 26, 675–697. doi: 10.1177/10298649211005531
Howard, M. C. (2016). A review of exploratory factor analysis decisions and overview of current practices: what we are doing and how can we improve? Int. J. Hum. Comput. Interact. 32, 51–62. doi: 10.1080/10447318.2015.1087664
Kaleli, Y. S. (2021). An investigation of pre-service music teachers’ self-regulatory skills. Int. J. Soc. Educ. Sci. 3, 548–562. doi: 10.46328/ijonses.226
Kegelaers, J., and Oudejans, R. R. D. (2020). A Process Evaluation of a Performance Psychology Intervention for Transitioning Elite and Elite Musicians. Front. Psychol. 11:1090. doi: 10.3389/fpsyg.2020.01090
Kim, S. J. (2010). A study of self-regulated learning in college string majors. String Res. J. 1, 39–54. doi: 10.1177/194849921000100103
Lakens, D. (2013). Calculating and reporting effect sizes to facilitate cumulative science: a practical primer for t-tests and ANOVAs. Front. Psychol. 4:863. doi: 10.3389/fpsyg.2013.00863
Liu, Y. (2023a). Differences in instrumental practice time and strategies among Chinese music majors. Res. Stud. Music Educ. 45, 127–140. doi: 10.1177/1321103X211038839
Liu, Y. (2023b). Practice habits and motivation: a qualitative descriptive study of graduate instrumentalists. Int. J. Music. Educ. 41, 443–454. doi: 10.1177/02557614221121171
Lloret-Segura, S., Ferreres-Traver, A., Hernández-Baeza, A., and Tomás-Marco, I. (2014). El análisis factorial exploratorio de los ítems: una guía práctica, revisada y actualizada. Psicol Spain 30, 1151–1169. doi: 10.6018/analesps.30.3.199361
López-Íñiguez, G., and McPherson, G. E. (2020). Applying self-regulated learning and self-determination theory to optimize the performance of a concert cellist. Front. Psychol. 11:385. doi: 10.3389/fpsyg.2020.00385
Lorenzo-Seva, U., and Ferrando, P. J. (2006). FACTOR: a computer program to fit the exploratory factor analysis model. Behav. Res. Methods 38, 88–91. doi: 10.3758/bf03192753
Lorenzo-Seva, U., and Ferrando, P. J. (2019). Robust Promin: a method for diagonally weighted factor rotation. Liberabit 25, 99–106. doi: 10.24265/liberabit.2019.v25n1.08
Madeira, L. R. B., Araújo, M. V., Hein, C. F., and Marinho, H. (2018). Adaptation of a self-regulated practice behaviour scale for portuguese music students. Psychol. Music 46, 795–812. doi: 10.1177/0305735617724884
McNeish, D. (2018). Thanks coefficient alpha, we’ll take it from here. Psychol. Methods 23, 412–433. doi: 10.1037/met0000144
McPherson, G. E., and McCormick, J. (1999). Motivational and self-regulated learning components of musical practice. Champaign, IL: University of Illinois Press.
McPherson, G. E., and McCormick, J. (2000). The contribution of motivational factors to instrumental performance in a music examination. Res. Stud. Music Educ. 15, 31–39. doi: 10.1177/1321103X0001500105
McPherson, G. E., Osborne, M. S., Evans, P., and Miksza, P. (2019). Applying self-regulated learning microanalysis to study musicians’ practice. Psychol. Music 47, 18–32. doi: 10.1177/0305735617731614
McPherson, G. E., and Zimmerman, B. J. (2002). “Self-regulation of musical learning: a socialcognitive perspective” in The new handbook of research on music teaching and learning. eds. R. Colwell and P. Webster (New York: Oxofrd University Press), 327–347.
McPherson, G. E., and Zimmerman, B. J. (2011). “Self-regulation of musical learning” in MENC handbook of research on music learning. eds. R. Colwell and P. Webster (Oxford: Oxford University Press), 130–175.
Miksza, P. (2012). The development of a measure of self-regulated practice behavior for beginning and intermediate instrumental music students. J. Res. Music. Educ. 59, 321–338. doi: 10.1177/0022429411414717
Miksza, P. (2015). The effect of self-regulation instruction on the performance achievement, musical self-efficacy, and practicing of advanced wind players. Psychol. Music 43, 219–243. doi: 10.1177/0305735613500832
Miksza, P., Blackwell, J., and Roseth, N. E. (2018). Self-regulated music practice: microanalysis as a data collection technique and inspiration for pedagogical intervention. J. Res. Music. Educ. 66, 295–319. doi: 10.1177/0022429418788557
Miksza, P., and Tan, L. (2015). Predicting collegiate wind players’ practice efficiency, flow, and self-efficacy for self-regulation: an exploratory study of relationships between teachers’ instruction and students’ practicing. J. Res. Music. Educ. 63, 162–179. doi: 10.1177/0022429415583474
Mornell, A., Osborne, M. S., and McPherson, G. E. (2020). Evaluating practice strategies, behavior and learning progress in elite performers: An exploratory study. Music. Sci. 24, 130–135. doi: 10.1177/1029864918771731
Muthén, B., and Kaplan, D. (1985). A comparison of some methodologies for the factor analysis of non-normal Likert variables. Br. J. Math. Stat. Psychol. 38, 171–189. doi: 10.1111/j.2044-8317.1985.tb00832.x
Muthén, B., and Kaplan, D. (1992). A comparison of some methodologies for the factor analysis of non-normal Likert variables: a note on the size of the model. Br. J. Math. Stat. Psychol. 45, 19–30. doi: 10.1111/j.2044-8317.1992.tb00975.x
Nielsen, S. G. (2004). Strategies and self-efficacy beliefs in instrumental and vocal individual practice: a study of students in higher music education. Psychol. Music 32, 418–431. doi: 10.1177/0305735604046099
Nielsen, S. G. (2012). Epistemic beliefs and self-regulated learning in music students. Psychol. Music 40, 324–338. doi: 10.1177/0305735610385509
Nusseck, M., and Spahn, C. (2021). Musical practice in music students during COVID-19 lockdown. Front. Psychol. 12:643177. doi: 10.3389/fpsyg.2021.643177
Osborne, M. S., McPherson, G. E., Miksza, P., and Evans, P. (2021). Using a microanalysis intervention to examine shifts in musicians’ self-regulated learning. Psychol. Music 49, 972–988. doi: 10.1177/0305735620915265
Papageorgi, I., Haddon, E., Creech, A., Morton, F., De Bezenac, C., Himonides, E., et al. (2010). Institutional culture and learning I: perceptions of the learning environment and musicians’ attitudes to learning. Music Educ. Res. 12, 151–178. doi: 10.1080/14613801003746550
Peistaraite, U., and Clark, T. (2020). Emotion regulation processes can benefit self-regulated learning in classical musicians. Front. Psychol. 11:568760. doi: 10.3389/fpsyg.2020.568760
Pike, P. D. (2017). Exploring self-regulation through a reflective practicum: a case study of improvement through mindful piano practice. Music. Educ. Res. 19, 398–409. doi: 10.1080/14613808.2017.1356813
Pintrich, P. R., and De Groot, E. V. (1990). Motivated Strategies for Learning Questionnaire (MSLQ). APA PsycTests. doi: 10.1037/t09161-000
Raykov, T. (1997). Estimation of composite reliability for congeneric measures. Appl. Psychol. Meas. 21, 173–184. doi: 10.1177/01466216970212006
Ritchie, L., and Williamon, A. (2013). Measuring musical self-regulation: linking processes, skills, and beliefs. J. Educ. Train. Stud. 1, 106–117. doi: 10.11114/jets.v1i1.81
Rogers, P. (2022). Best practices for your exploratory factor analysis: a factor tutorial. Rev. Adm. Contemp. 26:e210085. doi: 10.1590/1982-7849rac2022210085.en
Silva, C., and Fiorini, C. (2021). Daily instrument practice among music undergraduates: Self-Regulated Learning strategies. Opus 27, 1–20. doi: 10.20504/opus2021c2702
Tan, S. H., and Tan, S. B. (2010). The correct interpretation of confidence intervals. Proc. Singapore Healthcare 19, 276–278. doi: 10.1177/201010581001900316
Timmerman, M. E., and Lorenzo-Seva, U. (2011). Dimensionality assessment of ordered polytomous items with parallel analysis. Psychol. Methods 16, 209–220. doi: 10.1037/a0023353
Topoğlu, O., and Topoğlu, E. (2018). Examining perceived self-regulation beliefs of pre-service music teachers in musical instrument practice. HumanSciences 15:2180. doi: 10.14687/jhs.v15i4.5535
Valentini, F., and Damásio, B. F. (2016). Variância média extraída e confiabilidade composta: indicadores de precisão. Psicologia 32:225. doi: 10.1590/0102-3772e322225
Vellacott, C., and Ballantyne, J. (2022). An exploration of the practice habits and experiences of professional musicians. Music. Educ. Res. 24, 312–326. doi: 10.1080/14613808.2022.2053513
Volioti, G., and Williamon, A. (2017). Recordings as learning and practising resources for performance: exploring attitudes and behaviours of music students and professionals. Music. Sci. 21, 499–523. doi: 10.1177/1029864916674048
Williamon, A., and Valentine, E. (2000). Quantity and quality of musical practice as predictors of performance quality. Br. J. Psychol. 91, 353–376. doi: 10.1348/000712600161871
Worthington, R. L., and Whittaker, T. A. (2006). Scale development research: a content analysis and recommendations for best practices. Couns. Psychol. 34, 806–838. doi: 10.1177/0011000006288127
Zhang, C., Kwan, J. L. Y., and Leung, B.-W. (2023). Adaptation of a self-regulated practice behavior scale for chinese music majors. J. Res. Music. Educ. 71:147. doi: 10.1177/00224294221147008
Zhang, J. D., Susino, M., McPherson, G. E., and Schubert, E. (2020). The definition of a musician in music psychology: a literature review and the six-year rule. Psychol. Music 48, 389–409. doi: 10.1177/0305735618804038
Zhukov, K. (2012). Teaching strategies and gender in higher education instrumental studios. Int. J. Music. Educ. 30, 32–45. doi: 10.1177/0255761411431392
Zimmerman, B. J. (1989). A social cognitive view of self-regulated academic learning. J. Educ. Psychol. 81, 329–339. doi: 10.1037/0022-0663.81.3.329
Zimmerman, B. J. (2000). “Attaining self-regulation” in Handbook of self-regulation. eds. M. Boekaerts, P. R. Pintrich, and M. Zeidner (San Diego, CA: Academic Press), 13–39.
Zimmerman, B. J., and Campillo, M. (2003). “Motivating self-regulated problem solvers” in The psychology of problem solving. eds. J. E. Davidson and J. R. Sternberg (Cambridge: Cambridge University Press), 233–262.
Zimmerman, B. J., and Martinez-Pons, M. (1986). Development of a structured interview for assessing student use of self-regulated learning strategies. Am. Educ. Res. J. 23, 614–628. doi: 10.3102/00028312023004614
Keywords: self-regulated learning, music practice, survey, advanced musicians, exploratory factor analysis
Citation: dos Santos Silva C, Araújo MV and Marinho H (2024) Attitudes in music practice: a survey exploring the self-regulated learning processes of advanced Brazilian and Portuguese musicians. Front. Psychol. 15:1324100. doi: 10.3389/fpsyg.2024.1324100
Received: 19 October 2023; Accepted: 02 January 2024;
Published: 31 January 2024.
Edited by:
Adina Mornell, University of Music and Performing Arts Munich, GermanyReviewed by:
Alan Gumm, Central Michigan University, United StatesCopyright © 2024 dos Santos Silva, Araújo and Marinho. This is an open-access article distributed under the terms of the Creative Commons Attribution License (CC BY). The use, distribution or reproduction in other forums is permitted, provided the original author(s) and the copyright owner(s) are credited and that the original publication in this journal is cited, in accordance with accepted academic practice. No use, distribution or reproduction is permitted which does not comply with these terms.
*Correspondence: Camilla dos Santos Silva, Y2FtaWxsYXNpbHZhbXVzaWNhQGdtYWlsLmNvbQ==
Disclaimer: All claims expressed in this article are solely those of the authors and do not necessarily represent those of their affiliated organizations, or those of the publisher, the editors and the reviewers. Any product that may be evaluated in this article or claim that may be made by its manufacturer is not guaranteed or endorsed by the publisher.
Research integrity at Frontiers
Learn more about the work of our research integrity team to safeguard the quality of each article we publish.