- 1Agriculture and Food, CSIRO, Canberra, ACT, Australia
- 2Synthetic Biology Future Science Platform, CSIRO, Canberra, ACT, Australia
- 3The John Curtin School of Medical Research, Australian National University College of Health and Medicine, Canberra, ACT, Australia
- 4Data61, CSIRO, Canberra, ACT, Australia
Traditional functional genetic studies in crops are time consuming, complicated and cannot be readily scaled up. The reason is that mutant or transformed crops need to be generated to study the effect of gene modifications on specific traits of interest. However, many crop species have a complex genome and a long generation time. As a result, it usually takes several months to over a year to obtain desired mutants or transgenic plants, which represents a significant bottleneck in the development of new crop varieties. To overcome this major issue, we are currently establishing a versatile plant genetic screening platform, amenable to high throughput screening in almost any crop species, with a unique workflow. This platform combines protoplast transformation and fluorescence activated cell sorting. Here we show that tobacco protoplasts can accumulate high levels of lipid if transiently transformed with genes involved in lipid biosynthesis and can be sorted based on lipid content. Hence, protoplasts can be used as a predictive tool for plant lipid engineering. Using this newly established strategy, we demonstrate the major role of ABI3 in plant lipid accumulation. We anticipate that this workflow can be applied to numerous highly valuable metabolic traits other than storage lipid accumulation. This new strategy represents a significant step toward screening complex genetic libraries, in a single experiment and in a matter of days, as opposed to years by conventional means.
Introduction
Modern societies have slowly transitioned, over the past couple of centuries, from a bio-based economy to a petrol-based economy. Agriculture consequently transitioned to a food/feed focus whereas it used to meet all demands for energy and materials too. Driven by the threat of climate change and a need for more sustainability, re-establishing a bio-based economy is currently a major global challenge. To compete with established petrochemical industries, crops need to be re-engineered to have new traits or produce new compounds. However, current crop metabolic engineering approaches are slow and difficult, and this creates a significant bottleneck in the development of new crop varieties that are tailored to meet future agricultural needs in an ever changing environment.
As reflected by the late Richard Feynman’s statement: “What I cannot create, I do not understand,” the major challenge in crop metabolic engineering is to find the right set of genetic components necessary to obtain a desired trait. This is particularly due to traditional functional genetic studies in crops being time consuming, complicated and not usually compatible with high throughput. Indeed, it usually takes several months to over a year to obtain desired mutants or transgenic plants (Ishida et al., 2007; Yadava et al., 2017) necessary to study the effect of single genetic components on specific traits of interest. The testing of complex metabolic or genetic circuits thus seems like a herculean task. The use of model plants, such as Arabidopsis thaliana, with fully annotated genomes and much faster and cheaper gene delivery systems (Clough and Bent, 1998), had an undeniable impact on crop improvement (Provart et al., 2016), but still has limitations regarding time, scale and technology transfer between species.
While more promising, few transient transformation systems have been developed to test genetic components for crop re-engineering strategies. Currently, the most widely used transient transformation system for rapid gene testing is agroinfiltration of Nicotiana benthamiana leaves (Yang et al., 2000), which allows for the delivery of one or multiple genetic components to test multigene circuits in a few days (Wood et al., 2009). Pioneering studies have already demonstrated the power of such efficient transient transformation systems. For example, the “Leaf Oil” platform technology, which allows plants to accumulate oil in all vegetative organs (Vanhercke et al., 2017) with yields potentially exceeding those of canola and oil palm, was quickly developed thanks to an extensive number of design-built-test-learn cycles (Vanhercke et al., 2013, 2014; El Tahchy et al., 2017) that strongly relied on an efficient use of the agroinfiltration-based system. The technology is continuously being upgraded by further cycles to create tailor-made products for biofuel applications (Reynolds et al., 2015; Reynolds et al., 2017), and is also being transferred to a range of major crops such as sugarcane (Zale et al., 2016), maize (Alameldin et al., 2017) and Sorghum bicolor (Vanhercke et al., 2019). Such high biomass crops have the potential to become a future sustainable and abundant supply of plant oils for food, feed, biofuel and oleo-chemical applications (Rahman et al., 2016; Weselake, 2016). This type of assay is not limited to studying leaf specific metabolic pathways. Petrie et al. (2010) demonstrated that co-infiltration of the tested components in combination with the A. thaliana LEAFY COTYLEDON2 (LEC2) gene results in a leaf with a seed-like gene regulation profile, thus allowing for the testing of seed-specific components. A modified version of this assay was also developed in transgenic LEC2 induced Brassica napus somatic embryos for testing seed-specific components with increased reliability to predict seed traits (Belide et al., 2013). These assays allowed for the rapid gene testing and assembly of the ω3 fish-like oil synthetic seed biosynthesis pathway that was first deployed in A. thaliana as proof of concept (Petrie et al., 2012), and then in oilseed crops such as Camelina sativa (Petrie et al., 2014) and canola (Walsh et al., 2016), with ω3 production at industrially relevant levels. Nevertheless, these transient transformation systems are still limited in terms of scale, species and tissue specificity, and other transient assays are required to overcome these limitations.
Another type of transient system based on protoplast transfection has been available for more than 50 years, and now represents a very promising system for rapid testing of genetic components in most plants, and for several reasons. First, protoplasts can be isolated from almost any tissue of any crop (Burris et al., 2016), thus allowing better predictability through more species-targeted approaches than the agroinfiltration-based system that is established only in tobacco and few other species. Second, protoplasts are single cells, thus allowing more precise studies than multicellular systems to address tissue and cell-type specific questions. Thirdly, a very large number of protoplasts can be isolated from one preparation, allowing testing of many variables simultaneously in one assay. Finally, protoplast transformation is now well established (Yoo et al., 2007), with a higher efficiency than any other plant transformation system (Jiang et al., 2013), and is therefore very well suited for high throughput platforms. The use of protoplast-based systems also allows access to other types of high throughput approaches, such as flow cytometry and cell sorting, which open a wide new range of screening possibilities. Protoplasts have long been extensively used with fluorescence activated cell sorting for several different applications. Such methods were developed from the early 1980s for applications such as protoplast viral infection level monitoring (van Klaveren et al., 1983) and chlorophyll content and size distribution characterization (Galbraith et al., 1988). More recently, similar approaches have been used for more complex studies such as protein accumulation detection (Cronjé et al., 2004), overexpression analyses (Bargmann and Birnbaum, 2009), sorting of particular cell types for RNA isolation and transcriptome-wide analyses (Birnbaum et al., 2005; Bargmann and Birnbaum, 2010), or to study cell-type specific expression during both development and stress (Grønlund et al., 2012). Such techniques allow for the direct screening of millions of variants in a very short amount of time, and ongoing improvements (You et al., 2014) allow for the development of increasingly powerful high throughput screening methods. For example, recently, cell sorting based on the lipid content of thousands of mutant lines of a green unicellular algae was achieved in a few hours, followed by a few additional days for cellular multiplication and additional lipid analyses to confirm the phenotype (Terashima et al., 2015), representing one of the most powerful mutant screens ever reported for green vegetal cells. While random mutagenesis is not available for protoplasts, transient transformation is very efficient, and transformation of expression libraries could be carried out to generate large numbers of transformed cells for rapid trait evaluation by flow cytometry. However, sorting of plant protoplasts based on a high value trait, such as lipid accumulation, has not yet been reported.
The regulation of lipid biosynthesis and storage in plants has been extensively studied. The highly conserved plant transcription factors ABSCISIC ACID INSENSITIVE 3 (ABI3) (Giraudat et al., 1992), FUSCA3 (FUS3) (Luerssen et al., 1998), LEAFY COTYLEDON1 (LEC1) (Meinke et al., 1994; West et al., 1994), and LEC2 (Chen et al., 1997) are the major master regulators that control the gene regulation networks governing most seed developmental mechanisms (Suzuki et al., 2003; Braybrook et al., 2006; To et al., 2006; North et al., 2010; Wang and Perry, 2013). The abi3, fus3, lec1, and lec2 mutants share common phenotypes such as reduced storage protein accumulation and anthocyanin accumulation (To et al., 2006). FUS3, LEC1, and LEC2 also have been reported to trigger triacylglycerol accumulation in seeds, leaves and liquid cell culture (Lotan et al., 1998; Zhang et al., 2002, 2016; Santos Mendoza et al., 2005; Tan et al., 2011; Vanhercke et al., 2017). The major action of LEC1, LEC2, and FUS3 on lipid accumulation is mostly through regulating directly or indirectly the expression of the Wrinkled1 (WRI1) transcription factor (Casson and Lindsey, 2006; Baud et al., 2007; Santos-Mendoza et al., 2008; Yamamoto et al., 2010; Wang and Perry, 2013; Marchive et al., 2014), but the complete picture of WRI1 regulation is still unclear (Kim et al., 2016). WRI1 function is highly conserved amongst plants. WRI1 is a node acting at the interplay between these master regulatory elements and fatty acid metabolism. WRI1 directly regulates the transcription level of many genes essential for fatty acid synthesis to trigger seed oil production (Baud et al., 2007; Pouvreau et al., 2011). After it was demonstrated that WRI1 overexpression increases oil accumulation without undesirable side effects (Shen et al., 2010; Pouvreau et al., 2011), WRI1 became a major target of metabolic engineering approaches to increase oil content (Vanhercke et al., 2013, 2014, 2017). By contrast, even though many of ABI3 targeted genes are known to function in seed oil storage (Wang et al., 2007; Monke et al., 2012), to date, little is known about the direct effect of ABI3 on lipid content in plants.
In this study, we demonstrate that protoplasts from 15-day-old tobacco leaf can accumulate high levels of lipid if transiently transformed with genes involved in lipid biosynthesis. We observed a direct correlation between protoplast lipid content and protoplast fluorescence intensity after lipid-specific fluorescent staining. Furthermore, we show that protoplasts can be sorted based on lipid content and further used for downstream processing and analyses. Transient gene expression in protoplasts produced metabolic results similar to those reported for stably transformed plants, in terms of lipid accumulation. We demonstrate that combinatorial gene testing is possible in protoplasts and we observed a similar synergistic effect of the genes WRI1 and DGAT1 on increasing lipid accumulation in protoplasts, compared with previously published studies conducted in transiently and stably transformed leaves (Vanhercke et al., 2013, 2014). Taken together, our results demonstrate that tobacco leaf protoplasts are a reliable model for plant lipid engineering. This platform constitutes a new high throughput tool to develop plant lipid engineering strategies, but also an alternative strategy to study plant lipid metabolism in general, such as the relative effects of major known elicitors of lipid accumulation in plants. We compared the effect of ABI3, FUS3, LEC1, and LEC2 on lipid accumulation in protoplasts. Our results confirm that ABI3 is an important regulator of oil accumulation and demonstrate for the first time the direct correlation between ABI3 overexpression and increased lipid content in plants. These results also suggest that ABI3 might trigger lipid accumulation partly through pathways independent from WRI1 and LEC2 regulation.
Materials and Methods
Plant Material and Growth Conditions
Plants used in this study all originated from the Nicotiana tabacum cultivar Wisconsin 38, including wild-type (WT) and a T4 generation WRI1-DGAT1-OLEOSIN transgenic line, referred to as High Oil (HO), for the high levels of triglycerides accumulating in its leaves (Vanhercke et al., 2014). Seeds were surface-sterilized by 2 min incubation in 70% ethanol, followed by 10 min incubation in 1.8% bleach and five washes in sterile water, all with frequent agitation. Seeds were sown on half MS media, supplemented with 3% sucrose, in petri dishes and placed in the dark at 4°C for 4 days. Plants were grown in a growth cabinet under 16 h day/8 h night light conditions (100 μmol m–2 s–1) at 22°C/18°C, respectively.
Plasmids DNA Construction and Preparation
Vectors were designed as minimal plant expression constructs for production in large quantity in Escherichia coli and strong transient expression in tobacco protoplasts. A modified pENTR11 vector (Invitrogen) was used as a minimal backbone after removal of the ccdB operon, conserving only kanamycin resistance and the E. coli origin of replication. The chemically synthesized (GeneArt) CaMV35S promoter, coding sequence (A. thaliana WRI1, DGAT1, ABI3, FUS3, LEC1, or LEC2, respectively AT3G54320.1, AT2G19450.1, AT3G24650.1, AT3G26790.1, AT1G21970.1, or AT1G28300, codon optimized for tobacco) and NOS terminator were ligated in this minimal vector. For protoplast transient transformation, plasmid DNA was prepared using the NucleoBond Xtra Midi Kit (MACHEREY-NAGEL, Germany) according to the manufacturer’s recommended protocol, and resuspended in ultrapure DNAse/RNAse-free distilled water (Invitrogen) at 1 μg/μl.
Protoplasts Preparation, Transient Transformation and Staining
The whole procedure was conducted under sterile conditions. Plants were collected 15 days after germination. Leaf tissues were harvested and thinly sliced in protoplast buffer (400 mM mannitol, 10 mM MES hydrate, 20 mM KCl, 20 mM CaCl2, 2 mM MgCl2, pH 5.7, 0.1% bovine serum albumin). Leaf strips were rinsed with fresh protoplast buffer, placed in enzyme solution (protoplast buffer supplemented with 1.2% cellulase Onozuka R-10, 0.6% cellulase Onozuka RS, and 0.4% macerozyme R-10), subjected to vacuum for 10 min and incubated in enzyme solution for 16 h in the dark at 22°C. After incubation, protoplasts were released with 5 min agitation on a rotating plate at 50 rpm, filtered through a 40 μm cell strainer, washed twice with fresh protoplast buffer, counted on a hemocytometer, and then resuspended in fresh protoplast buffer at 0.25 M cells per ml. For transformation, protoplast samples were incubated for 1 h on ice, the buffer was then removed, pelleted protoplasts were resuspended in an identical volume of MMg solution (0.4 M mannitol, 15 mM MgCl2, 4 mM MES, pH 5.6), supplemented with 25 μg/ml of each plasmid and then mixed with an identical volume of PEG solution (40% PEG4000, 0.2 M mannitol, 300 mM CaCl2). After 5 min incubation, protoplasts were washed twice with fresh protoplast buffer, resuspended in fresh protoplast buffer at 0.25 M cells per ml, placed in culture plates and incubated in a growth cabinet under constant light conditions (150 μmol m–2 s–1) at 22°C. For lipid staining, protoplasts in protoplast buffer at 0.25 M cells per ml were supplemented with 1 μg/ml BODIPYTM 493/503, incubated for 15 min at room temperature in the dark, spun down (300 × g for 5 min) and resuspended in the same volume of fresh protoplast buffer.
Lipid Extractions and Analyses
For total lipid extractions, fresh leaves were cut as for protoplast isolation and freeze-dried whereas freshly isolated protoplasts were spun down (for 5 min) to remove all buffer and then snap-frozen in liquid nitrogen. Samples were ground with a ball bearing in TissuLyser II (QIAGEN, Germany) and homogenized in 36% methanol, 36% chloroform, 20 mM acetic acid, 1 mM EDTA. Total lipid fraction (lower phase) was separated by 2 min centrifugation at 4000 × g, dried under nitrogen and resuspended in chloroform. Total lipid extracts were fractionated by thin layer chromatography fractionation as previously described (El Tahchy et al., 2017), followed by fatty acid methyl ester quantification and analysis by gas chromatography, also conducted as previously described (Vanhercke et al., 2013; El Tahchy et al., 2017). Unless otherwise stated, analyses were conducted on a minimum of three individual samples for each genotype, condition or cell population.
Cell Imaging by Microscopy
Glass slides were prepared by placing four drops of petroleum jelly in a square arrangement and 50 μl of protoplasts at 1 M cells per ml in protoplast buffer within that square. A coverslip was then gently pressed down on the solution and jelly drops. Protoplasts were captured (Figure 3A) using a 40× objective on a Zeiss Axio Imager M1 fluorescence compound microscope fitted with an AxioCam HR Rev3 camera (Carl Zeiss Microscopy GmbH, Germany), with bright field and no filter or with UV light and a Filter Set 38 HE (Excitation filter BP 450–490 nm, Beam splitter FT 495 HE, emission BP 500–550 nm) to visualize lipid bodies stained with BODIPYTM 493/503. Images were acquired using the Zeiss Zen imaging software (blue edition, Carl Zeiss Microscopy GmbH, Germany).
Protoplast Flow Cytometry and Fluorescence Activated Cell Sorting
Cell sorting was performed on a BD FACS Aria III (BDIS, San Jose) with a 100 μm nozzle and data were acquired and analyzed using the DIVA 8.0 software. Protoplasts suspended in the protoplast buffer at a concentration of 1 million/ml were acquired at an approximate flow rate of 10 μl/min with mild agitation. Data were acquired for 30,000 particles and after exclusion of doublets, the samples were analyzed for BODIPYTM 493/503 (Excitation 488 nm, Emission 530/30 nm) and chlorophyll auto fluorescence (Excitation 640 nm, Emission 670/30 nm). Cells were sorted based on BODIPY/chlorophyll signal ratio. For each gated population, three independent samples of 0.25 M cells were collected in protoplast buffer.
Flow cytometric analyses were conducted on an Invitrogen Attune NxT Flow Cytometer and analyzed with Invitrogen Attune NxT Software. Cells suspended in the protoplast buffer at a concentration of 0.25 M cells per ml were analyzed at a flow rate of 250 μl/min and data were acquired for 300 μl. BODIPYTM 493/503 fluorescence was excited with a 488-nm laser and emission was captured by a 530/30-nm bandpass filter. Unless otherwise stated, analyses were conducted on a minimum of three individual samples for each condition.
Statistical Analysis
For the statistical analysis presented in Figure 5, data were analyzed via a linear mixed model fitted using the “lmer” linear mixed modeling function from the “lme4” package (Bates et al., 2015) of the R statistical software system (R Core Team., 2014). This allowed the analysis to properly account for the impacts of the grouping of processed samples together on plates (via a factor “Plate”), and also recognize that the full dataset is formed from two individual repeats of the same experiment (via a factor “Experiment”). Hence, the model incorporated a (random effects) “blocking” structure consisting of “Sample”, nested within Plate, nested within “Experiment”. Treatment (fixed effects) structure was modeled as a two-way factorial, WRI1 crossed with DGAT1, where factors WRI1 (having levels WRI1+ and WRI1−) and DGAT1 (levels DGAT1+ and DGAT1−) represent the presence/absence of the corresponding genes in the samples. Hence, the WT treatment corresponds to the combination “WRI1−:DGAT1−” and the samples transformed with DGAT1, WRI1, or both, correspond to “WRI1−:DGAT1+,” “WRI1+:DGAT1−” and “WRI1+:DGAT1+,” respectively. The model allows to test for the “main effect” of WRI1 (the average effect of WRI1 status, regardless of DGAT1 status), the main effect of DGAT1 (the average effect of DGAT1 status, regardless of WRI1 status), and the interaction effect between WRI1 and DGAT1 (the combined effects of WRI1 and DGAT1 after having removed effects of the two main effects terms).
In fitting any statistical model, it is important to assess various residual diagnostic plots to ensure that the assumptions underlying the model are met and that predictions and tests derived from the model are reliable. Residual diagnostic plots from the fit of the model to the raw data suggested that the random scatter in the data increases as the mean of the data increases; a common property of such assay data. To model the data appropriately, a log10 (log to base 10) transformation of the data was required to ensure that the model assumption of constant variance was met. The statistical model was therefore applied to the log10 transformed data, and predicted means were back-transformed as required to obtain predictions on the original data scale. Treatment predicted means and associated statistics were obtained using the R “predictmeans” package (Luo et al., 2018). Tests for the statistical significance of terms in the factorial treatment structure were obtained using the R “lmerTest” (Kuznetsova et al., 2017) package.
The errors bars and P-values presented in Figures 2, 3, 4 and 6 correspond to the standard deviation and a two-tailed t-test with unequal variance.
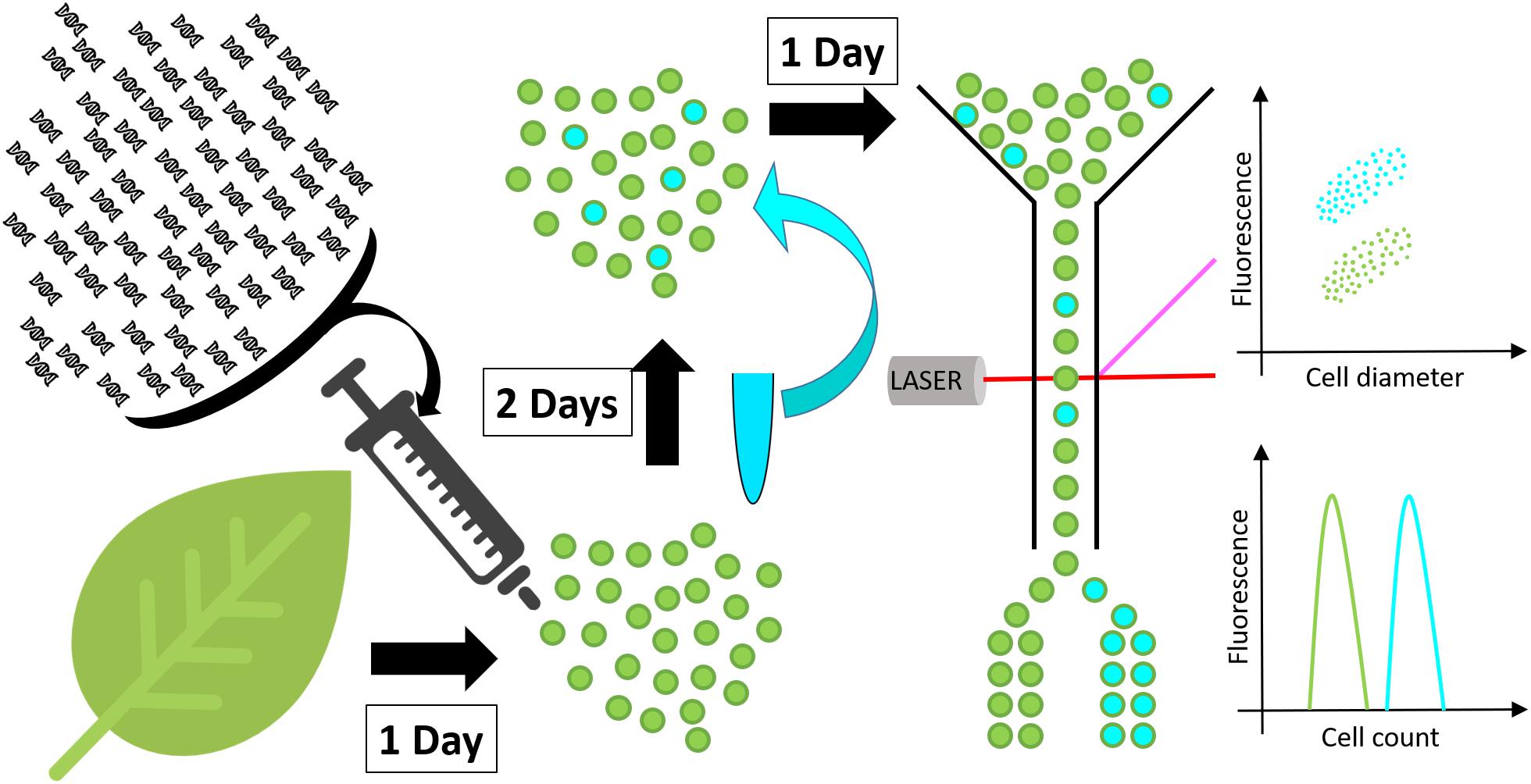
Figure 1. Schematic representation of the high throughput screen developed in this work. Protoplasts (green spheres) are isolated from a leaf and transformed with DNA constructs. After 48 h incubation, to allow for transgene expression and metabolite accumulation, protoplasts are stained with a neutral lipid specific fluorescent stain and screened for fluorescence by flow cytometry or fluorescence activated cell sorting.
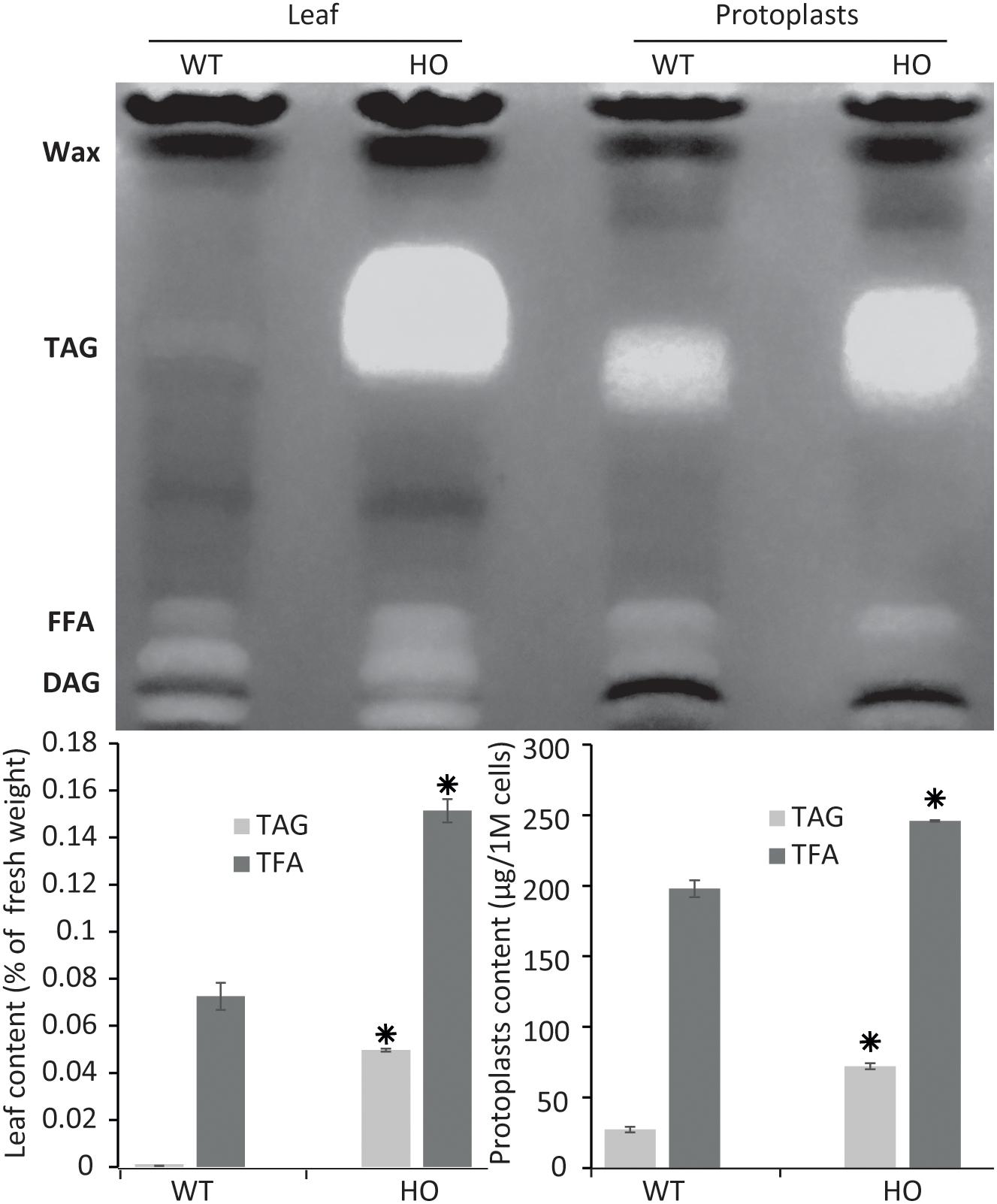
Figure 2. Protoplast lipid quantification results mimic total tissue lipid analysis results. Thin layer chromatography plate separation of total fatty acids (TFA) extracted from leaves or from isolated protoplasts of WT or HO lines and total lipid and triacylglycerol (TAG) quantification of the same samples quantified by gas chromatography. Concentrations are given in percent relative to leaf fresh weight or in micrograms per million cells. Bars represent standard deviation. Stars indicate statistically relevant variation compared to WT (P-values < 0.05). FFA, free fatty acids; DAG, diacylglycerol. See Supplementary Figure S1 for standards run next to samples.
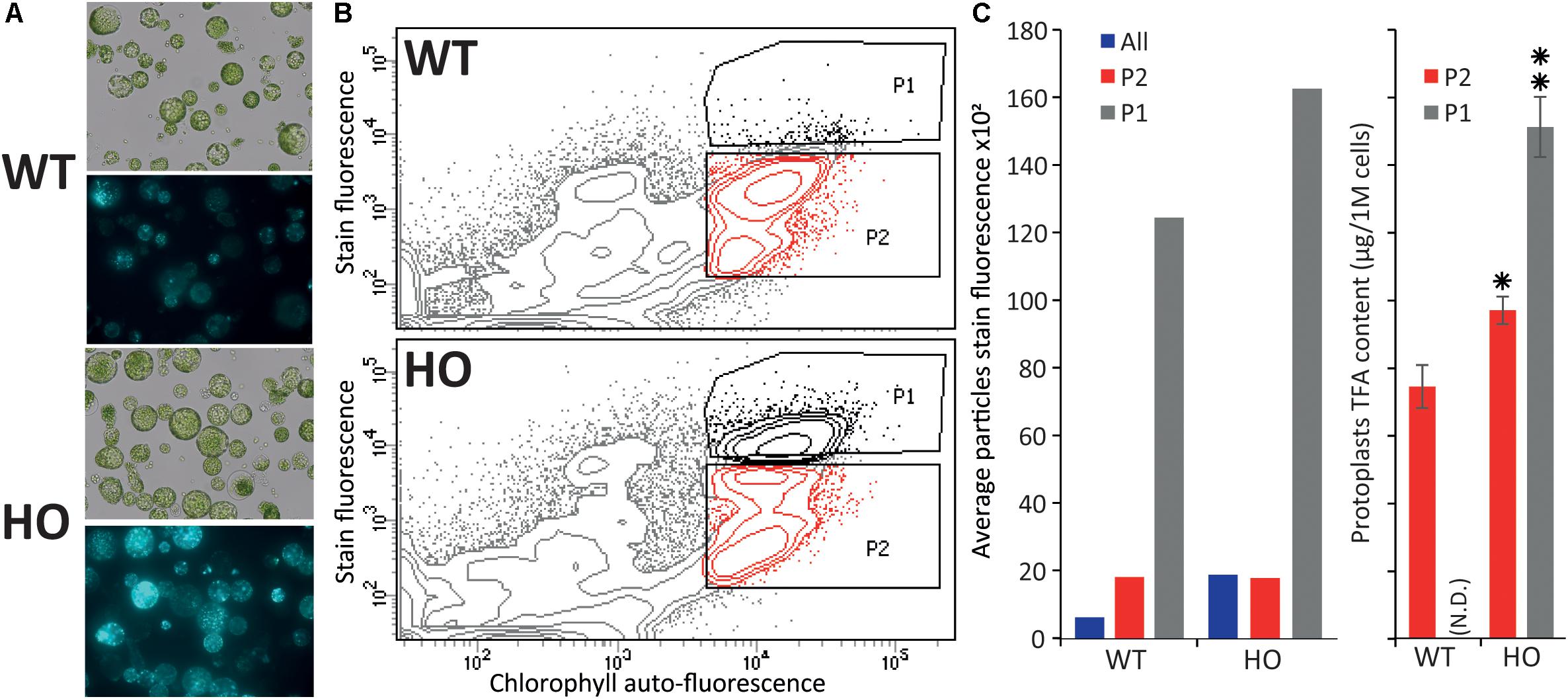
Figure 3. Fluorescence activated cell sorting of lipid rich tobacco leaf protoplasts. (A) Microscopy images of protoplasts extracted from WT or HO (line with high leaf oil content) tobacco leaves in bright field (top) and their lipid content (bottom), visualized under fluorescent light after BODIPYTM 493/503 staining. (B) Cell sorting of the same protoplast samples, with the P1 population defined as the protoplasts with the highest stain fluorescence to chlorophyll auto-fluorescence ratio, and P2 defined as the remainder of the intact cells (based on previous data not show). (C) Average stain fluorescence (left) and lipid content (right) for each sorted population. Average stain fluorescence of each whole sample is also indicated (blue bars). Where N.D. is in brackets, this indicates not determined. Error bars represent the standard deviation. Asterisks represent statistically significant differences (P-value < 0.05) compared with WT (one asterisk) or with both WT and HO P2 population (two asterisks).
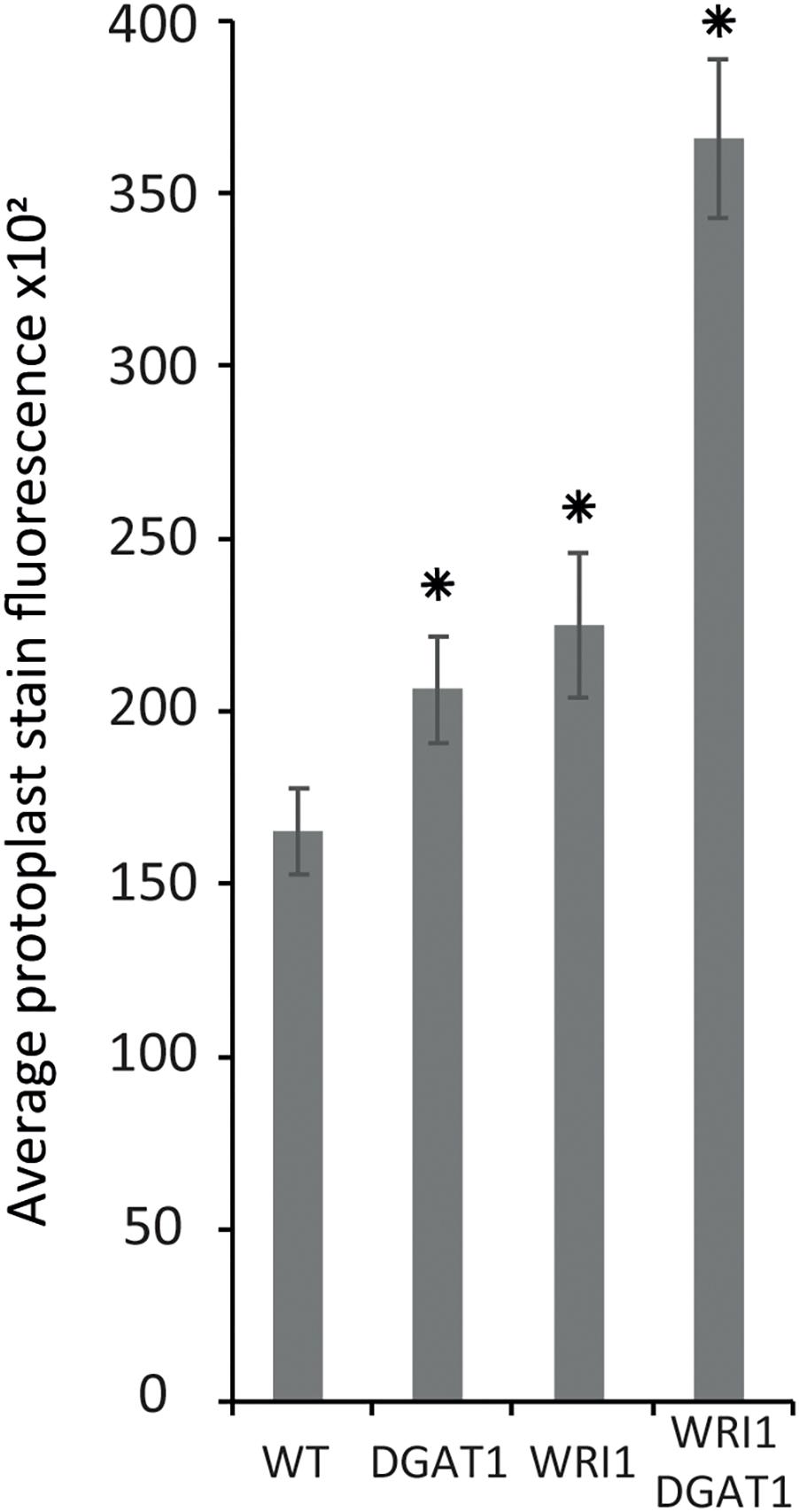
Figure 4. Lipid accumulation in tobacco leaf transiently transformed protoplasts. Lipid accumulation in WT tobacco leaf protoplasts compared to WT protoplasts transiently transformed with WRI1 or DGAT1 or both, was measured with BODIPYTM staining and flow cytometry recording of the average particle stain fluorescence for the whole sample. Error bars represent the standard deviation. Asterisks represent statistically significant differences (P-value < 0.05) compared with WT.
Results
Protoplasts Can Be Used as a Predictive Tool for Plant Lipid Engineering
To confirm that protoplasts can be used as a predictive tool for plant lipid engineering, we tested if the HO phenotype of a previously established stably transformed line could be reproduced with protoplasts isolated from the same line. The surprising lack of studies regarding lipid accumulation in protoplasts to date suggested that such approaches might not be possible to be conducted. We previously reported the establishment of a HO line of tobacco (henceforth referred to as HO) accumulating in its leaves up to 24 times more total lipid, and particularly 76 times more triglycerides, at maturity, compared to WT (Vanhercke et al., 2014). We also reported that this increased lipid accumulation was gradual during development, thus potentially making it difficult to measure the increase in very young tissue. Leaf tissues from 15 days old WT and HO plants were harvested and partly used for protoplast isolation. The remaining leaf tissues as well as protoplasts isolated from the same leaf samples were then analyzed for their triglycerides and total lipid content (Figure 2). The analysis revealed that at this early developmental stage and under the specific growth conditions of this assay, HO plantlets had accumulated about two times more total lipid and almost 90 times more triglycerides than WT, while HO protoplasts had accumulated about 25% more total lipid and more than two times more triglycerides than WT. Thus, despite some variation in intensity, the analysis confirmed that the increase can still be measured at the protoplast level. We also confirmed that even though the variation between the HO line and WT would be easier to measure by focusing on the triglycerides level, it is still quite feasible to measure it at the total lipid level. This will allow for the use of generalist neutral lipid stains, which stain any neutral lipid and not only triglycerides, to measure the variation in oil content.
We can observe a disparity at 15 days between the lipid contents of fresh tissues and protoplasts, indicating that the variation between HO and WT is reduced in the protoplast samples. One reason could be that fresh tissue samples are mostly constituted of fibres, which contains no lipid, and are not present in the protoplast sample. Another explanation is that the total leaf sample contains multiple types of cells, whereas protoplasts isolation methods are notorious to mostly enrich mesophyll cells. It could be that mesophyll cell contains more TAG than other types of leaf cells, but this was not tested in this study. This could explain why the WT protoplast sample appears to have a richer lipid composition than the fresh tissue it was prepared from, because it concentrates a certain type of cells only. Finally, a recent study demonstrated that the protoplast isolation itself could trigger the accumulation of some lipid species (Barnes et al., 2019), which could also explain why the WT protoplast sample appears to have a richer lipid composition than the WT leaf tissue sample.
A New and Rapid Method for Testing DNA Constructs for Plant Lipid Engineering
The new protoplast-based platform for high throughput plant lipid engineering presented in this study allows for the testing of DNA constructs in regard to their ability to induce lipid accumulation in plant cells within only 72 h. Using BODIPYTM 493/503, a fluorescent neutral lipid stain, lipid quantity in protoplasts is evaluated by measuring cell fluorescence by flow cytometry or alternatively by fluorescence activated cell sorting if specific cell populations need to be separated for further analyses (Figure 1). Protoplasts with high lipid accumulation consequently exhibit higher fluorescence and can be identified and eventually sorted based on the relative intensity of the fluorescent stain per cell.
Tobacco Leaf Protoplasts Can Be Sorted Based on Lipid Content
In the previous part, we demonstrated that we can measure variation in protoplast oil accumulation by conducting the analysis at the total lipid level instead of focusing on triglyceride accumulation only, which should allow for the use of a generalist neutral lipid stain, which stains triglycerides and all other neutral lipid, to measure the variation in oil content. We next sought to confirm that protoplasts can be sorted based on storage lipid content. Protoplasts were isolated from the leaves of 15-day-old WT and HO plantlets as described above, and stained with BODIPYTM 493/503, a generalist neutral lipid stain, prior to microscopy (Figure 3A) or flow cytometric analysis (Figure 3B). WT and HO stained protoplasts were consecutively sorted, based on their intensity of BODIPYTM 493/503 fluorescence relative to chlorophyll auto-fluorescence. Previous iterations of sorting coupled with microscopy allowed us to determine where the intact green cells are situated in the graph generated during cell sorting (data not shown). Two distinct populations of intact green cells were gated, with P1 defined as the most intensively stained cells and P2 as the rest of the intact green cells (Figure 3B). Average stain fluorescence per cell was calculated for each population (Figure 3C left). For each population, three samples of 250,000 cells were collected and then subjected to the same total lipid analysis as described above (Figure 3C right). This was not done for the WT-P1 population because it was not possible to retrieve enough cells to conduct the analysis. Several conclusions could be drawn for this assay.
First, we confirmed that BODIPYTM 493/503 staining allows for measuring of the oil content difference that we previously measured between WT and HO protoplasts. The whole HO sample had a higher average protoplast stain fluorescence intensity than the whole WT sample (blue bars in Figure 3C left). This correlates with the results presented in the previous part demonstrating that HO protoplasts accumulate more total lipid than WT and illustrates the direct correlation between lipid content and BODIPYTM 493/503 fluorescence intensity.
Secondly, the flow cytometric analysis showed that the HO sample had a distinct P1 population that segregated from the rest of the cells, while the WT cells attributed to the P1 population corresponded to the top cells of the P2 population rather than being a distinct cell population (Figure 3B). For both WT and HO samples, cells of the P1 population exhibited higher average stain fluorescence intensity compared to cells of the P2 population (Figure 3C left). Lipid analysis revealed that the HO-P2 population was significantly more lipid-rich than the WT-P2 population and that the HO-P1 population was significantly more lipid-rich than both the HO-P2 and WT-P2 populations (Figure 3C right). The variation in total lipid quantified in the HO-P2 and WT-P2 populations, while these two populations present a very similar level of fluorescence can be explained by the strong disparity between the ratios of different lipid species present in WT and in HO samples (Supplementary Table S1). It is possible that BODIPYTM 493/503 has different affinity depending on lipid species, which was not investigated in this study but could explain why the HO-P2 and WT-P2 populations present an identical fluorescence intensity after staining, while containing a slightly different amount of total lipid. Additionally, BODIPYTM 493/503, while being the most appropriate lipophilic dye available for this study, only binds to neutral lipid, while the total lipid quantification would quantify a much larger spectra of lipid species.
Overall, this assay confirmed that protoplasts can be sorted based on lipid content and that measuring the whole sample BODIPYTM 493/503 fluorescence signal (green bars in Figure 3C left) is enough to evaluate the effect of a gene combination on the overall lipid content in protoplasts. We also note that the variation measured between the WT and HO sample is amplified when using BODIPYTM 493/503 staining coupled with flow cytometry (compared with lipid analysis methods), suggesting that this alternative screening strategy could be more powerful than traditional ones.
Transiently Transformed Tobacco Leaf Protoplasts Can Accumulate Lipid
We have established that leaf protoplasts from a stable transgenic line that accumulates more oil in its leaves than WT also accumulates on average more oil per protoplast compared to WT and that the difference can be measured by traditional lipid analysis or measured by flow cytometry after protoplast isolation and appropriate staining. However, to date, it was unclear if and how leaf protoplasts would produce an increased quantity of oil compared to WT, after isolation and transient transformation, reflected by the absence of any similar study in the literature.
The stable transgenic line used in the study over-expresses a combination of genes coding for the A. thaliana WRI1 transcription factor, a regulator of the late glycolysis and de novo fatty acid biosynthesis pathways, the A. thaliana DGAT1 acyl-CoA: diacylglycerol acyltransferase, a major lipid metabolism enzyme, and an OLEOSIN oil body protein from Sesamum indicum, which coats oil droplets and protects them from degradation. The reasoning for including OLEOSIN was that achieving greater oil accumulation in leaves in a long gene-expression-window (stable expression) might have required reducing oil degradation in this non-seed tissue. However, we previously demonstrated that the combined transient overexpression of WRI1 and DGAT1 only in N. benthamiana leaves was enough to produce a measurable and significant synergistic effect on leaf oil content (Vanhercke et al., 2013).
Based on the results presented above, next we tested transient transformation of leaf protoplasts with genetic constructs carrying expression cassettes for WRI1 or DGAT1 or both and measured the difference in protoplast total neutral lipid content, using BODIPYTM 493/503 staining and flow cytometry recording of the average protoplast stain fluorescence for the whole sample (Figure 4). Compared to the non-transformed control, leaf protoplasts transiently transformed with WRI1 or DGAT1 or both exhibited increased average stain fluorescence, indicating an increase in total neutral lipid content.
We also observed that when co-transformed, the combined effect of WRI1 and DGAT1 on lipid accumulation is greater than the additive effect of each individual transformations, illustrating a synergistic effect of WRI1 and DGAT1 (Figure 4) toward lipid accumulation in N. tabacum leaf protoplast, consistent with previous reports from N. benthamiana leaf transient assay (Vanhercke et al., 2013) and stably transformed N. tabacum plants (Vanhercke et al., 2014). The significance of this observation was accessed by a statistical analysis, which is further discussed below.
Combinatorial Gene Testing in Protoplasts Mimics Results Obtained With Leaf Transient Assay
The statistical analysis of the data confirms the statistical significance of the synergistic effect, with a P-value for the interaction effect on the log10-transformed scale of p < 5e-6. An interaction plot of the WRI1 by DGAT1 predicted means is given in Figure 5A. The significant P-value confirms that the lack of parallelism of the lines (and hence the presence of the synergistic effect) joining the pairs of means is genuine. Figure 5B plots the predicted means back-transformed to the scale of the data, with associated back-transformed 95% confidence interval limits for the means indicated by the error bars.
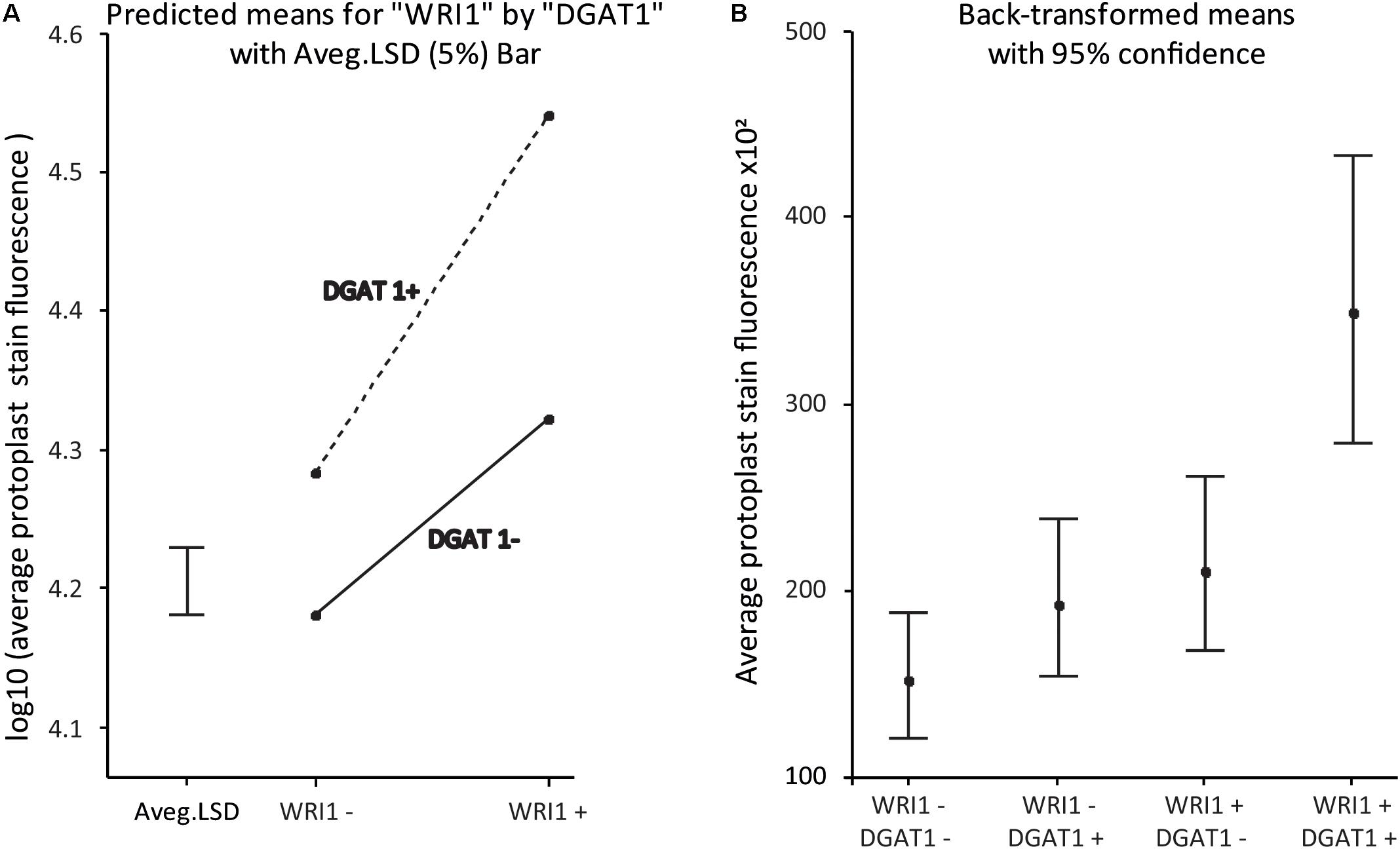
Figure 5. WRI1 and DGAT1 have a synergistic positive effect on protoplast lipid biosynthesis. (A) Interaction plot of predicted treatment means with 5% Least Significant Difference (LSD) bar on the log10-transformed scale and (B) Plot of the back-transformed predicted means (with back-transformed 95% confidence interval limits) from the analysis of the data previously presented in Figure 4B. WT corresponds to “WRI1−:DGAT1−” and the samples transformed with DGAT1, WRI1 and both, correspond to WRI1−:DGAT1+, “WRI1+:DGAT1−” and “WRI1+:DGAT1+,” respectively.
Overall our results confirm that protoplast systems are robust models that can be used to predict and inform plant phenotype and are compatible with combinatorial gene testing. This finding is particularly important for coupling transient protoplast expression with high throughput combinatorial gene screening.
ABI3 Induces Lipid Accumulation in Tobacco Leaf Protoplasts
Our new screening strategy constitutes a new high throughput tool to fast-track plant lipid engineering strategies and offers an alternative method to study plant lipid metabolism. We therefore wanted to use this newly developed strategy to investigate the relative effect of the major known seed specific developmental regulators. ABI3, FUS3, LEC1, and LEC2 are master regulators that control the gene regulation networks governing most seed developmental mechanisms. FUS3, LEC1, and LEC2 also have been reported to trigger oil accumulation through regulation of WRI1, which controls the expression of many genes essential for fatty acid synthesis. However, while many targets of ABI3 were identified to function in seed oil storage, the direct effect of ABI3 on lipid content in plants remained undocumented.
Tobacco leaf protoplasts were transiently transformed with WRI1 and DGAT1 only or with one of the master regulators, being ABI3, FUS3, LEC1, or LEC2. The effect on protoplast total neutral lipid content was then measured and compared as described above (Figure 6A). This first assay indicated that in addition to the combined effect of WRI1 and DGAT1, only co-expression with FUS3 and ABI3 significantly (P-value < 0.05) further increased protoplast total neutral lipid content. This was particularly surprising due to the lack of a previously reported direct effect of ABI3 overexpression on plant lipid accumulation. To date, the most efficient gene combination reported for lipid accumulation enhancement involved WRI1 with DGAT1 and LEC2 or SDP1 silencing (Vanhercke et al., 2017). Therefore, in a second similar analysis we investigated in more detail the individual effect of individual genes (WRI1, DGAT1, LEC2, and ABI3) and of several related combinations on total neutral lipid content in transiently transformed protoplasts (Figure 6B). This second assay confirmed that ABI3 overexpression alone resulted in the highest accumulation of total neutral lipid in protoplasts. In addition, unlike LEC2, co-expression with ABI3 further increased the lipid content of protoplasts transiently transformed with WRI1 and DGAT1. This difference supports the previous reports that LEC2 regulates fatty acid biosynthesis mainly through the regulation of WRI1 (Baud et al., 2007; Santos-Mendoza et al., 2008). Thus, the addition of LEC2 might be ineffective due to the expression level of exogenous WRI1 in the co-transformed protoplasts being already so high that triggering the expression of native WRI1 would make a negligible effect on the overall WRI1 expression levels in protoplasts. Overall, our results suggest that ABI3 control over plant lipid metabolism and storage might involve mechanisms that act independently from the previously described LEC2 and WRI1 regulation networks. Our new screening approach also suggests that ABI3 could be an important factor triggering oil accumulation in plants and suppose that ABI3 should be of prime interest in future plant lipid engineering approaches.
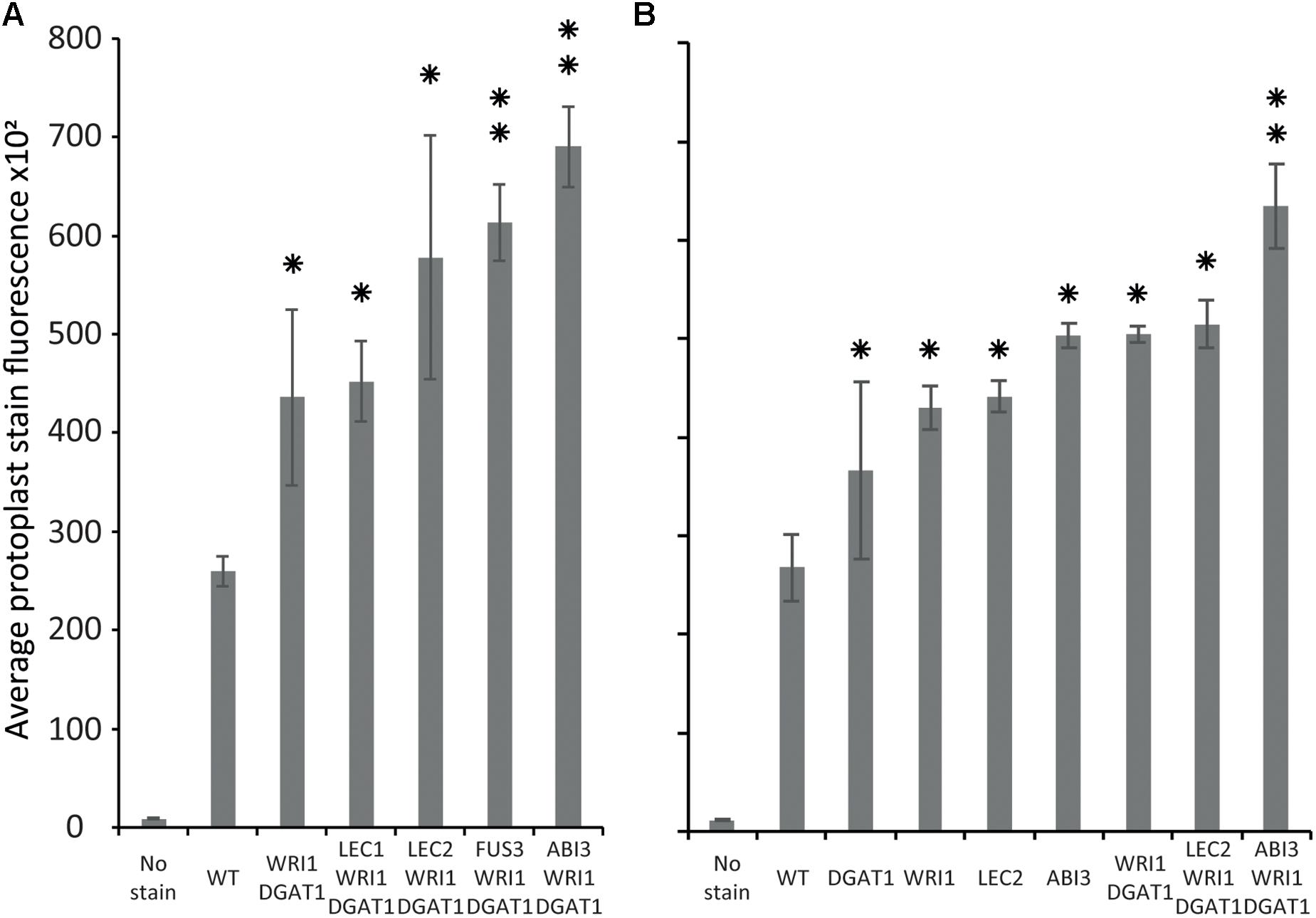
Figure 6. Comparison of the relative effect of various transiently transformed effectors on lipid accumulation in tobacco leaf protoplasts. Lipid accumulation, compared to WT, in tobacco leaf protoplasts transiently transformed with WRI1, DGAT1, ABI3, FUS3, LEC1, or LEC2 and various combinations of these constructs, was measured by flow cytometry and based on the average particle stain fluorescence for the whole sample following BODIPYTM staining. Bars represent standard deviation. (A) Comparative additional effect of ABI3, FUS3, LEC1, or LEC2 on lipid content in protoplasts co-transformed with WRI1 and DGAT1. (B) Individual effect of WRI1, DGAT1, ABI3, LEC2 and of various combinations of these constructs on lipid content in co-transformed protoplasts. A single star represents statistically significant difference (P-value < 0.05) compared with WT, while the double stars represent additional statistically significant difference (P-value < 0.05) compared with WRI1 and DGAT1 combination (shown for samples with significant increase only).
Discussion
Transient Protoplast Transformation as an Alternative Predictive Tool for Plant Lipid Engineering
In this study, we demonstrated that protoplasts from 15-day-old tobacco leaves are a reliable model to study plant lipid engineering. This protoplast-based screening assay takes about three days to complete, as compared to the minimum of two weeks required for a traditional transient leaf transformation assays based on agroinfiltration and GC analysis. We confirmed the direct correlation between protoplast lipid content and protoplast fluorescence intensity after lipid specific fluorescent staining. Furthermore, we show that protoplasts can be sorted based on lipid content and further used for downstream processing and lipid or molecular analyses. Our results demonstrate that transient gene expression in protoplasts produces lipid accumulation results similar to those obtained in stably transformed plants. We confirm that combinatorial gene testing is possible in protoplasts, with synergistic effect of the genes WRI1 and DGAT1 on increasing lipid accumulation in protoplasts, comparable to previously published studies conducted in transiently and stably transformed leaves (Vanhercke et al., 2013, 2014).
ABI3 Is a Major Regulator of Lipid Accumulation in Plant Cell
Using our newly developed workflow, we demonstrated that ABI3 has a significant effect on protoplast total neutral lipid content. ABI3 super-transformation significantly further increased the lipid content of protoplasts transiently transformed with WRI1 and DGAT1, to a higher level than other known major effectors.
This result suggests that ABI3 control over plant lipid metabolism and storage might at least partially involve mechanisms that are independent from the previously described LEC2 and WRI1 regulation networks. Our new approach also suggests that ABI3 is a major regulator of oil accumulation in plants and reveal ABI3 as a new target for future plant lipid engineering approaches.
A New High Throughput Platform for Gene Function and Plant Metabolic Engineering Study
The protoplast-based workflow presented in this study is very versatile and should be compatible with many crop species and for a wide range of future output traits. Indeed, protoplasts can nowadays be isolated from almost any plants and any tissue. A profusion of methods were developed during the 1970s and 1980s for many major crops and model plants, including tobacco (N. tabacum) (Takebe and Otsuki, 1969; Guerche et al., 1987a), cowpea (Vigna unguiculata) (Hibi et al., 1975), tomato (Solanum lycopersicum) (Motoyoshi and Oshima, 1979), turnip (Brassica rapa) (Howell and Hull, 1978), barley (Hordeum vulgare) (Loesch-Fries and Hall, 1980), cucumber (Cucumis sativus) (Maule et al., 1980) great millet (Sorghum bicolor), rice (Oryza sativa), wheat (Triticum monococcum) (Ou-Lee et al., 1986; Bart et al., 2006), canola (B. napus) (Guerche et al., 1987b), A. thaliana (Damm et al., 1989), and maize (Zea mays) (Sheen, 1990). Such approaches then fell into disuse probably due to the very high technical skills required to perform high quality protoplast isolation, transformation and culture. However, new methods were sporadically released for other crops of interest, such as carrot (Daucus carota) (Liu et al., 1994). Recently, in the wake of a multitude of new high throughput cutting-edge technologies becoming easily accessible, protoplast-based systems have regained in their popularity and a profusion of improved methods have been released. Protoplast systems are the most suited for rapid screening of gene silencing (siRNA and miRNA) and genome editing (e.g., CRISPR) targets (Zhai et al., 2009; Cao et al., 2014; Maćkowska et al., 2014; You et al., 2014; Schapire and Lois, 2016). In addition, protoplast-based systems are now available for almost any plant of commercial interest, including lettuce (Lactuca sativa) (Sasamoto and Ashihara, 2014), grape (Vitis vinifera) (Wang et al., 2015) and bean (Phaseolus vulgaris) (Nanjareddy et al., 2016) and even the less expected, such as oil palm (Elaeis guineensis) (Masani et al., 2014), poplar (Populus euphratica) (Guo et al., 2015) and switchgrass (Panicum virgatum) (Burris et al., 2016).
In addition, our strategy should allow similar approaches for a broad variety of other metabolic traits since many fluorescent dyes, specific to particular metabolites are available. In the absence of a specific stain, genetic switches or biosensors can be developed for the specific detection of small molecules such as toluene (Behzadian et al., 2011), metabolites (Liu et al., 2017), and even specific proteins of interest (Abe et al., 2011; Wongso et al., 2017). For example, a new phosphatidic acid (PA) biosensor was recently developed to track PA cellular concentration and dynamics (Li et al., 2019). PA is the precursor of most phospholipid and triglyceride and represents around 1% of all lipid. PA acts as a key signaling lipid that predominantly accumulates at the plasma membrane. This biosensor was based on the use of Förster resonance energy transfer (FRET) and was used to study the spatio-temporal complexity of PA accumulation in plant tissues and its contribution to mediate plant response to salt stress. Numerous other biosensors have been also developed based on FRET to study protein-protein, protein-DNA or DNA-DNA interactions (Lymperopoulos et al., 2010; Crawford et al., 2012). Alternatively, riboswitches have been engineered as nucleotide-based biosensors with great potential (Hallberg et al., 2017; Villa et al., 2018).
The new screening strategy presented in this study constitute the first step toward high throughput screening of complex genetic libraries in a plant system by using transient protoplast transformation and rapid cell sorting based on a fluorescent signal that is linked to a trait of interest. The workflow can be completed as a single experiment in a matter of days as opposed to several years that are needed by conventional means. Such novel synthetic biology inspired high throughput screens are paving the way for rapid identification of new unreported genes or gene combinations that significantly improve traits of interest in crops and thereby greatly improve the timelines associated with crop improvement (Zhang et al., 2019).
Data Availability Statement
All datasets generated for this study are included in the article/Supplementary Material, excepted raw flow cytometry data.
Author Contributions
BP, TV, and SS conceived the study. BP and CB performed the experimental work with assistance from HV for fluorescence activated cell sorting. BP analyzed the data with assistance from AZ for statistical analysis. BP wrote the manuscript with contributions from all other authors.
Funding
This work was partly funded through the CSIRO Synthetic Biology Future Science Platform and the CSIRO Research Office CERC Postdoctoral Fellowship scheme.
Conflict of Interest
The authors declare that the research was conducted in the absence of any commercial or financial relationships that could be construed as a potential conflict of interest.
Acknowledgments
Thanks to Nathalie Niesner for cloning the DGAT1 fragment into the “CaMV35S promoter/NOS terminator” expression cassette of the modified pENTR11 vector. Thanks also to Lauren Venugoban for aiding with microscopy.
Supplementary Material
The Supplementary Material for this article can be found online at: https://www.frontiersin.org/articles/10.3389/fpls.2020.00288/full#supplementary-material
FIGURE S1 | Repeat of thin layer chromatography plate presented in Figure 2. Thin layer chromatography plate separation of total fatty acids (TFA) extracted from leaves or from isolated protoplasts of WT or HO lines. STD stands for standards run in parallel of the samples to validate the location of the lipid.
TABLE S1 | Raw data for lipid quantifications presented in Figures 2, 3. Note: TAG/TFA content in tobacco leaf is presented in % of fresh weight leaf and in protoplasts in μg/1 M cells.
References
Abe, R., Ohashi, H., Iijima, I., Ihara, M., Takagi, H., Hohsaka, T., et al. (2011). “Quenchbodies”: quench-based antibody probes that show antigen-dependent fluorescence. J. Am. Chem. Soc. 133, 17386–17394. doi: 10.1021/ja205925j
Alameldin, H., Izadi-Darbandi, A., Smith, S. A., Balan, V., Jones, A. D., and Sticklen, M. (2017). Production of seed-like storage lipids and increase in oil bodies in corn (Maize; Zea mays L.) vegetative biomass. Ind. Crops Products 108, 526–534. doi: 10.1016/j.indcrop.2017.07.021
Bargmann, B. O., and Birnbaum, K. D. (2009). Positive fluorescent selection permits precise, rapid, and in-depth overexpression analysis in plant protoplasts. Plant Physiol. 149, 1231–1239. doi: 10.1104/pp.108.133975
Bargmann, B. O., and Birnbaum, K. D. (2010). Fluorescence activated cell sorting of plant protoplasts. J. Vis. Exp. 18:1673.
Barnes, A. C., Elowsky, C. G., and Roston, R. L. (2019). An Arabidopsis protoplast isolation method reduces cytosolic acidification and activation of the chloroplast stress sensor SENSITIVE TO FREEZING 2. Plant Signal. Behav. 14:1629270. doi: 10.1080/15592324.2019.1629270
Bart, R., Chern, M., Park, C.-J., Bartley, L., and Ronald, P. C. (2006). A novel system for gene silencing using siRNAs in rice leaf and stem-derived protoplasts. Plant Methods 2:13.
Bates, D., Mächler, M., Bolker, B., and Walker, S. (2015). Fitting linear mixed-effects models using lme4. 67:48.
Baud, S., Mendoza, M. S., To, A., Harscoet, E., Lepiniec, L., and Dubreucq, B. (2007). WRINKLED1 specifies the regulatory action of LEAFY COTYLEDON2 towards fatty acid metabolism during seed maturation in Arabidopsis. Plant J. 50, 825–838. doi: 10.1111/j.1365-313x.2007.03092.x
Behzadian, F., Barjeste, H., Hosseinkhani, S., and Zarei, A. R. (2011). Construction and characterization of Escherichia coli whole-cell biosensors for toluene and related compounds. Curr. Microbiol. 62, 690–696. doi: 10.1007/s00284-010-9764-5
Belide, S., Zhou, X.-R., Kennedy, Y., Lester, G., Shrestha, P., Petrie, J. R., et al. (2013). Rapid expression and validation of seed-specific constructs in transgenic LEC2 induced somatic embryos of Brassica napus. Plant Cell Tissue Organ Culture (PCTOC) 113, 543–553. doi: 10.1007/s11240-013-0295-1
Birnbaum, K., Jung, J. W., Wang, J. Y., Lambert, G. M., Hirst, J. A., Galbraith, D. W., et al. (2005). Cell type–specific expression profiling in plants via cell sorting of protoplasts from fluorescent reporter lines. Nat. Method 2, 615–619. doi: 10.1038/nmeth0805-615
Braybrook, S. A., Stone, S. L., Park, S., Bui, A. Q., Le, B. H., Fischer, R. L., et al. (2006). Genes directly regulated by LEAFY COTYLEDON2 provide insight into the control of embryo maturation and somatic embryogenesis. Proc. Natl. Acad. Sci. U.S.A. 103, 3468–3473. doi: 10.1073/pnas.0511331103
Burris, K. P., Dlugosz, E. M., Collins, A. G., Stewart, C. N., and Lenaghan, S. C. (2016). Development of a rapid, low-cost protoplast transfection system for switchgrass (Panicum virgatum L.). Plant Cell Rep. 35, 693–704. doi: 10.1007/s00299-015-1913-7
Cao, J., Yao, D., Lin, F., and Jiang, M. (2014). PEG-mediated transient gene expression and silencing system in maize mesophyll protoplasts: a valuable tool for signal transduction study in maize. Acta Physiol. Plant 36, 1271–1281. doi: 10.1007/s11738-014-1508-x
Casson, S. A., and Lindsey, K. (2006). The turnip mutant of Arabidopsis reveals that LEAFY COTYLEDON1 expression mediates the effects of auxin and sugars to promote embryonic cell identity. Plant Physiol. 142, 526–541. doi: 10.1104/pp.106.080895
Chen, D., Kini, R. M., Yuen, R., and Khoo, H. E. (1997). Haemolytic activity of stonustoxin from stonefish (Synanceja horrida) venom: pore formation and the role of cationic amino acid residues. Biochem. J. 325, 685–691. doi: 10.1042/bj3250685
Clough, S. J., and Bent, A. F. (1998). Floral dip: a simplified method for Agrobacterium-mediated transformation of Arabidopsis thaliana. Plant J. 16, 735–743. doi: 10.1046/j.1365-313x.1998.00343.x
Crawford, R., Kelly, D. J., and Kapanidis, A. N. (2012). A protein biosensor that relies on bending of single DNA molecules. Chemphyschem 13, 918–922. doi: 10.1002/cphc.201100881
Cronjé, M. J., Snyman, M., Bornman, L., and Weir, I. E. (2004). A rapid and reliable flow cytometric method for determining Hsp70 levels in tobacco protoplasts. Methods Cell Sci. 25, 237–246. doi: 10.1007/s11022-004-2878-z
Damm, B., Schmidt, R., and Willmitzer, L. (1989). Efficient transformation of Arabidopsis thaliana using direct gene transfer to protoplasts. Molec. Gen. Genet. 217, 6–12. doi: 10.1007/bf00330935
El Tahchy, A., Reynolds, K. B., Petrie, J. R., Singh, S. P., and Vanhercke, T. (2017). Thioesterase overexpression in Nicotiana benthamiana leaf increases the fatty acid flux into triacylgycerol. FEBS Lett. 591, 448–456. doi: 10.1002/1873-3468.12539
Galbraith, D. W., Harkins, K. R., and Jefferson, R. A. (1988). Flow cytometric characterization of the chlorophyll contents and size distributions of plant protoplasts. Cytometry 9, 75–83. doi: 10.1002/cyto.990090112
Giraudat, J., Hauge, B. M., Valon, C., Smalle, J., Parcy, F., and Goodman, H. M. (1992). Isolation of the Arabidopsis ABI3 gene by positional cloning. Plant Cell 4, 1251–1261. doi: 10.1105/tpc.4.10.1251
Grønlund, J. T., Eyres, A., Kumar, S., Buchanan-Wollaston, V., and Gifford, M. L. (2012). Cell specific analysis of Arabidopsis leaves using fluorescence activated cell sorting. J. Visual. Exp. 68:4214.
Guerche, P., Bellini, C., Le Moullec, J. M., and Caboche, M. (1987a). Use of a transient expression assay for the optimization of direct gene transfer into tobacco mesophyll protoplasts by electroporation. Biochimie 69, 621–628. doi: 10.1016/0300-9084(87)90181-7
Guerche, P., Charbonnier, M., Jouanin, L., Tourneur, C., Paszkowski, J., and Pelletier, G. (1987b). Direct gene transfer by electroporation in Brassica napus. Plant Sci. 52, 111–116. doi: 10.1016/0168-9452(87)90112-9
Guo, Y., Song, X., Zhao, S., Lv, J., and Lu, M. (2015). A transient gene expression system in Populus euphratica Oliv. Protoplasts prepared from suspension cultured cells. Acta Physiol. Plant 37:160.
Hallberg, Z. F., Su, Y., Kitto, R. Z., and Hammond, M. C. (2017). Engineering and in vivo applications of riboswitches. Annu. Rev. Biochem. 86, 515–539. doi: 10.1146/annurev-biochem-060815-014628
Hibi, T., Rezelman, G., and Van Kammen, A. (1975). Infection of cowpea mesophyll protoplasts with cowpea mosaic virus. Virology 64, 308–318. doi: 10.1016/0042-6822(75)90107-5
Howell, S. H., and Hull, R. (1978). Replication of cauliflower mosaic virus and transcription of its genome in turnip leaf protoplasts. Virology 86, 468–481. doi: 10.1016/0042-6822(78)90086-7
Ishida, Y., Hiei, Y., and Komari, T. (2007). Agrobacterium-mediated transformation of maize. Nat. Protoc. 2, 1614–1621. doi: 10.1038/nprot.2007.241
Jiang, F., Zhu, J., and Liu, H. L. (2013). Protoplasts: a useful research system for plant cell biology, especially dedifferentiation. Protoplasma 250, 1231–1238. doi: 10.1007/s00709-013-0513-z
Kim, M. J., Jang, I. C., and Chua, N. H. (2016). The mediator complex MED15 subunit mediates activation of downstream lipid-related genes by the WRINKLED1 transcription factor. Plant Physiol. 171, 1951–1964. doi: 10.1104/pp.16.00664
Kuznetsova, A., Brockhoff, P. B., and Christensen, R. H. B. (2017). lmerTest package: tests in linear mixed effects models. 82:26.
Li, W., Song, T., Wallrad, L., Kudla, J., Wang, X., and Zhang, W. (2019). Tissue-specific accumulation of pH-sensing phosphatidic acid determines plant stress tolerance. Nat. Plants 5, 1012–1021. doi: 10.1038/s41477-019-0497-6
Liu, Y., Liu, Y., and Wang, M. (2017). Design, optimization and application of small molecule biosensor in metabolic engineering. Front. Microbiol. 8:2012. doi: 10.3389/fmicb.2017.02012
Liu, Z. B., Ulmasov, T., Shi, X., Hagen, G., and Guilfoyle, T. J. (1994). Soybean GH3 promoter contains multiple auxin-inducible elements. Plant Cell 6, 645–657. doi: 10.1105/tpc.6.5.645
Loesch-Fries, L. S., and Hall, T. C. (1980). Synthesis, accumulation and encapsidation of individual brome mosaic virus RNA components in barley protoplasts. J. Gen. Virol. 47, 323–332. doi: 10.1099/0022-1317-47-2-323
Lotan, T., Ohto, M., Yee, K. M., West, M. A., Lo, R., Kwong, R. W., et al. (1998). Arabidopsis LEAFY COTYLEDON1 is sufficient to induce embryo development in vegetative cells. Cell 93, 1195–1205. doi: 10.1016/s0092-8674(00)81463-4
Luerssen, H., Kirik, V., Herrmann, P., and Misera, S. (1998). FUSCA3 encodes a protein with a conserved VP1/AB13-like B3 domain which is of functional importance for the regulation of seed maturation in Arabidopsis thaliana. Plant J. 15, 755–764. doi: 10.1046/j.1365-313x.1998.00259.x
Luo, D., Ganesh, S., and Koolaard, J. (2018). Predictmeans: Calculate Predicted Means for Linear Models. R package version 1.0.1. Available online at: https://CRANR-projectorg/package=predictmeans.
Lymperopoulos, K., Crawford, R., Torella, J. P., Heilemann, M., Hwang, L. C., Holden, S. J., et al. (2010). Single-molecule DNA biosensors for protein and ligand detection. Angew. Chem. Int. Ed. 49, 1316–1320. doi: 10.1002/anie.200904597
Maćkowska, K., Jarosz, A., and Grzebelus, E. (2014). Plant regeneration from leaf-derived protoplasts within the Daucus genus: effect of different conditions in alginate embedding and phytosulfokine application. Plant Cell Tissue Organ Culture (PCTOC) 117, 241–252. doi: 10.1007/s11240-014-0436-1
Marchive, C., Nikovics, K., To, A., Lepiniec, L., and Baud, S. (2014). Transcriptional regulation of fatty acid production in higher plants: molecular bases and biotechnological outcomes. Eur. J. Lipid Sci. Technol. 116, 1332–1343. doi: 10.1002/ejlt.201400027
Masani, M. Y., Noll, G. A., Parveez, G. K., Sambanthamurthi, R., and Prufer, D. (2014). Efficient transformation of oil palm protoplasts by PEG-mediated transfection and DNA microinjection. PLoS One 9:e96831. doi: 10.1371/journal.pone.0096831
Maule, A. J., Boulton, M. I., Edmunds, C., and Wood, K. R. (1980). Polyethylene glycol-mediated infection of cucumber protoplasts by cucumber mosaic virus and virus RNA. J. Gen. Virol. 47, 199–203. doi: 10.1099/0022-1317-47-1-199
Meinke, D. W., Franzmann, L. H., Nickle, T. C., and Yeung, E. C. (1994). Leafy cotyledon mutants of Arabidopsis. Plant Cell 6, 1049–1064. doi: 10.1105/tpc.6.8.1049
Monke, G., Seifert, M., Keilwagen, J., Mohr, M., Grosse, I., Hahnel, U., et al. (2012). Toward the identification and regulation of the Arabidopsis thaliana ABI3 regulon. Nucleic Acids Res. 40, 8240–8254. doi: 10.1093/nar/gks594
Motoyoshi, F., and Oshima, N. (1979). Standardization in inoculation procedure and effect of a resistance gene on infection of tomato protoplasts with tobacco mosaic virus RNA. J. Gen. Virol. 44, 801–806. doi: 10.1099/0022-1317-44-3-801
Nanjareddy, K., Arthikala, M. K., Blanco, L., Arellano, E. S., and Lara, M. (2016). Protoplast isolation, transient transformation of leaf mesophyll protoplasts and improved Agrobacterium-mediated leaf disc infiltration of Phaseolus vulgaris: tools for rapid gene expression analysis. BMC Biotechnol. 16:53. doi: 10.1186/s12896-016-0283-8
North, H., Baud, S., Debeaujon, I., Dubos, C., Dubreucq, B., Grappin, P., et al. (2010). Arabidopsis seed secrets unravelled after a decade of genetic and omics-driven research. Plant J. 61, 971–981. doi: 10.1111/j.1365-313x.2009.04095.x
Ou-Lee, T. M., Turgeon, R., and Wu, R. (1986). Expression of a foreign gene linked to either a plant-virus or a Drosophila promoter, after electroporation of protoplasts of rice, wheat, and sorghum. Proc. Natl. Acad. Sci. U.S.A. 83, 6815–6819. doi: 10.1073/pnas.83.18.6815
Petrie, J. R., Shrestha, P., Belide, S., Kennedy, Y., Lester, G., Liu, Q., et al. (2014). Metabolic engineering camelina sativa with fish oil-like levels of DHA. PLoS ONE 9:e85061. doi: 10.1371/journal.pone.0085061
Petrie, J. R., Shrestha, P., Liu, Q., Mansour, M. P., Wood, C. C., Zhou, X. R., et al. (2010). Rapid expression of transgenes driven by seed-specific constructs in leaf tissue: DHA production. Plant Methods 6:8. doi: 10.1186/1746-4811-6-8
Petrie, J. R., Shrestha, P., Zhou, X. R., Mansour, M. P., Liu, Q., Belide, S., et al. (2012). Metabolic engineering plant seeds with fish oil-like levels of DHA. PLoS ONE 7:e49165. doi: 10.1371/journal.pone.0049165
Pouvreau, B., Baud, S., Vernoud, V., Morin, V., Py, C., Gendrot, G., et al. (2011). Duplicate maize Wrinkled1 transcription factors activate target genes involved in seed oil biosynthesis. Plant Physiol. 156, 674–686. doi: 10.1104/pp.111.173641
Provart, N. J., Alonso, J., Assmann, S. M., Bergmann, D., Brady, S. M., Brkljacic, J., et al. (2016). 50 years of Arabidopsis research: highlights and future directions. New Phytol. 209, 921–944. doi: 10.1111/nph.13687
R Core Team. (2014). R: A Language and Environment for Statistical Computing. Vienna: R Foundation for Statistical Computing.
Rahman, M. M., Divi, U. K., Liu, Q., Zhou, X., Surinder, S., and Aruna, K. (2016). Oil-rich nonseed tissues for enhancing plant oil production. CAB Rev. 11, 1–11.
Reynolds, K. B., Taylor, M. C., Cullerne, D. P., Blanchard, C. L., Wood, C. C., Singh, S. P., et al. (2017). A reconfigured Kennedy pathway which promotes efficient accumulation of medium-chain fatty acids in leaf oils. Plant Biotechnol. J. 15, 1397–1408. doi: 10.1111/pbi.12724
Reynolds, K. B., Taylor, M. C., Zhou, X.-R., Vanhercke, T., Wood, C. C., Blanchard, C. L., et al. (2015). Metabolic engineering of medium-chain fatty acid biosynthesis in Nicotiana benthamiana plant leaf lipids. Front. Plant Sci. 6:164. doi: 10.3389/fpls.2015.00164
Santos Mendoza, M., Dubreucq, B., Miquel, M., Caboche, M., and Lepiniec, L. (2005). LEAFY COTYLEDON 2 activation is sufficient to trigger the accumulation of oil and seed specific mRNAs in Arabidopsis leaves. FEBS Lett. 579, 4666–4670. doi: 10.1016/j.febslet.2005.07.037
Santos-Mendoza, M., Dubreucq, B., Baud, S., Parcy, F., Caboche, M., and Lepiniec, L. (2008). Deciphering gene regulatory networks that control seed development and maturation in Arabidopsis. Plant J. 54, 608–620. doi: 10.1111/j.1365-313x.2008.03461.x
Sasamoto, H., and Ashihara, H. (2014). Effect of nicotinic acid, nicotinamide and trigonelline on the proliferation of lettuce cells derived from protoplasts. Phytochem. Lett. 7, 38–41. doi: 10.1016/j.phytol.2013.09.008
Schapire, A. L., and Lois, L. M. (2016). A simplified and rapid method for the isolation and transfection of Arabidopsis leaf mesophyll protoplasts for large-scale applications. Methods Mol. Biol. 1363, 79–88. doi: 10.1007/978-1-4939-3115-6_8
Sheen, J. (1990). Metabolic repression of transcription in higher plants. Plant Cell 2, 1027–1038. doi: 10.1105/tpc.2.10.1027
Shen, B., Allen, W. B., Zheng, P., Li, C., Glassman, K., Ranch, J., et al. (2010). Expression of ZmLEC1 and ZmWRI1 increases seed oil production in maize. Plant Physiol. 153, 980–987. doi: 10.1104/pp.110.157537
Suzuki, M., Ketterling, M. G., Li, Q.-B., and McCarty, D. R. (2003). Viviparous1 alters global gene expression patterns through regulation of abscisic acid signaling. Plant Physiol. 132, 1664–1677. doi: 10.1104/pp.103.022475
Takebe, I., and Otsuki, Y. (1969). Infection of tobacco mesophyll protoplasts by tobacco mosaic virus. Proc. Natl. Acad. Sci. U.S.A. 64, 843–848. doi: 10.1073/pnas.64.3.843
Tan, H., Yang, X., Zhang, F., Zheng, X., Qu, C., Mu, J., et al. (2011). Enhanced seed oil production in canola by conditional expression of Brassica napus LEAFY COTYLEDON1 and LEC1-LIKE in developing seeds. Plant Physiol. 156, 1577–1588. doi: 10.1104/pp.111.175000
Terashima, M., Freeman, E. S., Jinkerson, R. E., and Jonikas, M. C. (2015). A fluorescence-activated cell sorting-based strategy for rapid isolation of high-lipid Chlamydomonas mutants. Plant J. 81, 147–159. doi: 10.1111/tpj.12682
To, A., Valon, C., Savino, G., Guilleminot, J., Devic, M., Giraudat, J., et al. (2006). A network of local and redundant gene regulation governs arabidopsis seed maturation. Plant Cell 18, 1642–1651. doi: 10.1105/tpc.105.039925
van Klaveren, P., Slats, J., Roosien, J., and van Vloten-Doting, L. (1983). Flow cytometric analysis of tobacco and cowpea protoplasts infected in vivo and in vitro with alfalfa mosaic virus. Plant Mol. Biol. 2, 19–25. doi: 10.1007/bf00187572
Vanhercke, T., Belide, S., Taylor, M. C., El Tahchy, A., Okada, S., Rolland, V., et al. (2019). Up-regulation of lipid biosynthesis increases the oil content in leaves of Sorghum bicolor. Plant Biotechnol. J. 17, 220–232. doi: 10.1111/pbi.12959
Vanhercke, T., Divi, U. K., El Tahchy, A., Liu, Q., Mitchell, M., Taylor, M. C., et al. (2017). Step changes in leaf oil accumulation via iterative metabolic engineering. Metabol. Eng. 39, 237–246. doi: 10.1016/j.ymben.2016.12.007
Vanhercke, T., El Tahchy, A., Liu, Q., Zhou, X. R., Shrestha, P., Divi, U. K., et al. (2014). Metabolic engineering of biomass for high energy density: oilseed-like triacylglycerol yields from plant leaves. Plant Biotechnol. J 12, 231–239. doi: 10.1111/pbi.12131
Vanhercke, T., El Tahchy, A., Shrestha, P., Zhou, X.-R., Singh, S. P., and Petrie, J. R. (2013). Synergistic effect of WRI1 and DGAT1 coexpression on triacylglycerol biosynthesis in plants. FEBS Lett. 587, 364–369. doi: 10.1016/j.febslet.2012.12.018
Villa, J. K., Su, Y., Contreras, L. M., and Hammond, M. C. (2018). Synthetic biology of small RNAs and riboswitches. Microbiol. Spectr. 6. doi: 10.1128/microbiolspec.RWR-0007-2017
Walsh, T. A., Bevan, S. A., Gachotte, D. J., Larsen, C. M., Moskal, W. A., Merlo, P. A., et al. (2016). Canola engineered with a microalgal polyketide synthase-like system produces oil enriched in docosahexaenoic acid. Nat. Biotechnol. 34, 881–887. doi: 10.1038/nbt.3585
Wang, F., and Perry, S. E. (2013). Identification of direct targets of FUSCA3, a key regulator of Arabidopsis seed development. Plant Physiol. 161, 1251–1264. doi: 10.1104/pp.112.212282
Wang, H., Guo, J., Lambert, K. N., and Lin, Y. (2007). Developmental control of Arabidopsis seed oil biosynthesis. Planta 226, 773–783. doi: 10.1007/s00425-007-0524-0
Wang, H., Wang, W., Zhan, J., Huang, W., and Xu, H. (2015). An efficient PEG-mediated transient gene expression system in grape protoplasts and its application in subcellular localization studies of flavonoids biosynthesis enzymes. Sci. Horticult. 191, 82–89. doi: 10.1016/j.scienta.2015.04.039
Weselake, R. J. (2016). “Chapter 15 – engineering oil accumulation in vegetative tissue,” in Industrial Oil Crops, eds T. McKeon, D. Hayes, D. Hildebrand, and R. Weselake (Urbana, IL: AOCS Press), 413–434. doi: 10.1016/b978-1-893997-98-1.00015-4
West, M., Yee, K. M., Danao, J., Zimmerman, J. L., Fischer, R. L., Goldberg, R. B., et al. (1994). LEAFY COTYLEDON1 is an essential regulator of late embryogenesis and cotyledon identity in Arabidopsis. Plant Cell 6, 1731–1745. doi: 10.1105/tpc.6.12.1731
Wongso, D., Dong, J., Ueda, H., and Kitaguchi, T. (2017). Flashbody: a next generation fluobody with fluorescence intensity enhanced by antigen binding. Anal. Chem. 89, 6719–6725. doi: 10.1021/acs.analchem.7b00959
Wood, C. C., Petrie, J. R., Shrestha, P., Mansour, M. P., Nichols, P. D., Green, A. G., et al. (2009). A leaf-based assay using interchangeable design principles to rapidly assemble multistep recombinant pathways. Plant Biotechnol. J. 7, 914–924. doi: 10.1111/j.1467-7652.2009.00453.x
Yadava, P., Abhishek, A., Singh, R., Singh, I., Kaul, T., Pattanayak, A., et al. (2017). Advances in maize transformation technologies and development of transgenic maize. Front. Plant Sci. 7:1949. doi: 10.3389/fpls.2016.01949
Yamamoto, A., Kagaya, Y., Usui, H., Hobo, T., Takeda, S., and Hattori, T. (2010). Diverse roles and mechanisms of gene regulation by the Arabidopsis seed maturation master regulator FUS3 revealed by microarray analysis. Plant Cell Physiol. 51, 2031–2046. doi: 10.1093/pcp/pcq162
Yang, Y., Li, R., and Qi, M. (2000). In vivo analysis of plant promoters and transcription factors by agroinfiltration of tobacco leaves. Plant J. 22, 543–551. doi: 10.1046/j.1365-313x.2000.00760.x
Yoo, S. D., Cho, Y. H., and Sheen, J. (2007). Arabidopsis mesophyll protoplasts: a versatile cell system for transient gene expression analysis. Nat. Protoc. 2, 1565–1572. doi: 10.1038/nprot.2007.199
You, M. K., Lim, S. H., Kim, M. J., Jeong, Y. S., Lee, M. G., and Ha, S. H. (2014). Improvement of the fluorescence intensity during a flow cytometric analysis for rice protoplasts by localization of a green fluorescent protein into chloroplasts. Int. J. Mol. Sci. 16, 788–804. doi: 10.3390/ijms16010788
Zale, J., Jung, J. H., Kim, J. Y., Pathak, B., Karan, R., Liu, H., et al. (2016). Metabolic engineering of sugarcane to accumulate energy-dense triacylglycerols in vegetative biomass. Plant Biotechnol. J. 14, 661–669. doi: 10.1111/pbi.12411
Zhai, Z., Jung, H. I., and Vatamaniuk, O. K. (2009). Isolation of protoplasts from tissues of 14-day-old seedlings of Arabidopsis thaliana. J. Vis. Exp. 1149.
Zhang, M., Cao, X., Jia, Q., and Ohlrogge, J. (2016). FUSCA3 activates triacylglycerol accumulation in Arabidopsis seedlings and tobacco BY2 cells. Plant J. 88, 95–107. doi: 10.1111/tpj.13233
Zhang, S., Wong, L., Meng, L., and Lemaux, P. G. (2002). Similarity of expression patterns of knotted1 and ZmLEC1 during somatic and zygotic embryogenesis in maize (Zea mays L.). Planta 215, 191–194. doi: 10.1007/s00425-002-0735-3
Keywords: high throughput screening, protoplast, metabolic engineering, lipid accumulation, fluorescence activated cell sorting
Citation: Pouvreau B, Blundell C, Vohra H, Zwart AB, Arndell T, Singh S and Vanhercke T (2020) A Versatile High Throughput Screening Platform for Plant Metabolic Engineering Highlights the Major Role of ABI3 in Lipid Metabolism Regulation. Front. Plant Sci. 11:288. doi: 10.3389/fpls.2020.00288
Received: 27 October 2019; Accepted: 26 February 2020;
Published: 17 March 2020.
Edited by:
John Sedbrook, Illinois State University, United StatesReviewed by:
Timothy Patrick Durrett, Kansas State University, United StatesMichael Marks, University of Minnesota Twin Cities, United States
Copyright © 2020 Pouvreau, Blundell, Vohra, Zwart, Arndell, Singh and Vanhercke. This is an open-access article distributed under the terms of the Creative Commons Attribution License (CC BY). The use, distribution or reproduction in other forums is permitted, provided the original author(s) and the copyright owner(s) are credited and that the original publication in this journal is cited, in accordance with accepted academic practice. No use, distribution or reproduction is permitted which does not comply with these terms.
*Correspondence: Benjamin Pouvreau, benjamin_pouvreau@yahoo.fr