- 1Soft Matter Physics Laboratory, School of Advanced Materials Science and Engineering, SKKU Advanced Institute of Nanotechnology, Sungkyunkwan University, Suwon, South Korea
- 2Research Center for Advanced Materials Technology, Sungkyunkwan University, Suwon, South Korea
Clean foams tend to age with time through sequential coalescence events. This study evaluates aging dynamics in clean foams by measuring bubble populations from coalescence simulation experiments and adopting biological population dynamics analysis. The population dynamics of bubbles in clean foams during coalescence show that the mortality rates of individual bubbles change exponentially with time, regardless of initial simulation conditions, consistent with the Gompertz mortality law commonly observed in biological aging. This result would be beneficial in understanding the aging dynamics of clean foams.
1. Introduction
Foams have a cellular network structure between two immiscible phases. The structural dynamics of foams have attracted researchers in both scientific and industrial applications. Particularly, solid metallic foams are exciting thanks to combinations of physical and mechanical properties, such as high stiffness in conjunction with a low specific weight or high compressive strength combined with suitable energy absorption characteristics. Their network structure makes them proper for lightweight construction or crash energy absorption in automotive and aerospace industries [1]. A composite metal foam is appropriate for Li-ion batteries [2]. Fluid foams or cellular fluids consist of uniformly dispersed bubbles and a continuous liquid film. Bubbles inside fluid foams are usually unstable and evolve with time to minimize their surface energy [3]. In physics, foams are a model system for materials that minimize surface energy: soap foams, emulsions, magnetic garnets, and grain boundaries [3], because they eventually evolve into a stationary state of statistical equilibrium [4]. In mathematics, foams are a model system to study the isoperimetric problem relevant to the minimal perimeter with a fixed number of bubbles in an area [3]. Two-dimensional random cellular networks (2D foams) are ubiquitous such as soap froths, fragmentation patterns, and biological epidermis [4]. Initially unstable foams evolve with time toward equilibrium by reducing their total surface area as the average size of the bubbles grows over time by either rupture of the liquid films between bubbles (coalescence) or growth through the diffusive exchange of gas (coarsening) [5].
After an initial transient, film rupture initiates dynamic rearrangement of bubbles, where bubbles rapidly coalesce and slowly evolve toward a new quasi-equilibrium state. Slow dynamics and aging effects in out-of-equilibrium systems such as glasses, gels, and foams are a rich and fascinating topic, yet still poorly understood [6]. Cellular patterns like foams widely appear in nature, such as cells in biological tissues, crystalline grains in polycrystals, grain aggregates in colloidal materials, and bubbles on a pint of beer [7, 8]. Physicists have extensively studied collective statics and dynamics of foams theoretically and experimentally [7–15]. Foams have gained much attention not only in engineering but also in soft matter physics [7]. Clean foams spontaneously and rapidly coalesce with time unless they are stabilized by surfactants and stabilizing particles [10] under the volume constraint [3]. The sequential coalescence events in clean foams show the irreversible monotonic decay in the bubble population, analogous to the irreversible biological aging.
This study evaluates the temporal evolution of bubbles in clean foams by adopting biological population dynamics analysis. Clean foams are far-from-equilibrium dissipative systems like biological systems despite the detailed aging mechanisms are different in both systems. Taking the simulation data reproduced by Dr. Peter Stewart (personal communication, see the Datasets 1, 2) based on his simulation methods [see the details in [10]], we analyzed the clean foam coalescence curves. The network model for numerical simulations of clean foams was previously developed to simulate the rapid evolution of molten metallic foams [10]. The aging dynamics for a given bubble population emerged as a consequence of collective interactions among individual bubbles [10]. From the measurements of the total bubble numbers, we find that the mortality rate (μ) of individual bubbles in clean foams increases exponentially with time (x), indicating the Gompertz mortality law that truly holds commonly in biological aging [16, 17]. The exponential changes in the mortality rates for clean foams are attributable to a cumulative consequence of sequential coalescence events [3, 10], similar to progressive physiological changes in biology [16, 17]. We find that a Boltzmann sigmoidal function well describes the bubble population change, indicating that foam aging may be a kind of phase transition phenomena [18, 19]. The aging of clean foam cells occurs by the self-similar coalescence of bubbles regardless of initial conditions [10]. For the analysis of aging dynamics of clean foams, we suggest a new methodology for survival analysis by formulating the mortality probability, μ(x) = −d ln[s(x)]/dx, where s(x) is the survival probability of bubbles in clean foams that survive without coalescence from birth to age x.
2. Methods
We study the coalescence-driven aging dynamics of clean foam cells from the coalescence data taken by numerical simulations with a network model for clean foam coalescence events [10]. The details of the network model for clean foams are well-described in the original work [10]. In the absence of stabilizing particles or surfactants, bubbles inside clean foams coalesce rapidly after film rupture typically occurs on time scales of milliseconds in experiments [20]. Van der Waals intermolecular attractions drive film rupture [21]. The resulting film thinning over two distinct phases induces bubble coalescence in clean foams [10]. In the first phase, film rupture triggers a cascade of rapid topological rearrangement of bubbles through bubble coalescence, and finally, the foam reaches a new quasi-equilibrium state [10]. Typically, bubble coalescence is a fundamental consequence of the surface energy minimization of the whole liquid films [22, 23]. In the simulation work, bubble coalescence occurs by film rupture in clean foams driven by capillary viscous suction [10]. The second phase proceeds by isolated rupture events, which occur intermittently over much longer time scales [10].
Based on a planar gas−liquid foam with low liquid fraction without any surfactants and stabilizing particles, the network model for clean foams treated the gas bubbles as polygons, the accumulation of liquid at the bubble vertices (Plateau borders) as dynamic nodes, and the liquid bridges separating the bubbles as uniformly thinning free films [10]. The clean foam system started from a periodic array of equally pressurized bubbles, with the initial film thicknesses sampled from a normal distribution [10]. After an initial transient, the first film rupture induces a dynamic rearrangement, where the bubbles rapidly coalesce, evolving toward a new quasi-equilibrium [10]. Through the Monte Carlo simulations of the coalescence process, it was possible to measure the total bubble population as a function of the model parameters [10]. We received the simulation data (see the Datasets 1, 2) reproduced by Dr. Peter Stewart (personal communication) and analyzed the bubble coalescence curves with the Origin software (OriginLab Corp., Origin 2021).
Figure 1 shows representative examples of a typical soap foam and a collective bubble number (population) dynamics with sequential coalescence events in clean foams: the initial number of bubbles gradually decreases with age. As seen here, the bubble population decreases as time goes on from the initial bubbles. We evaluate the foam population dynamics from the total number of bubbles for each simulation, denoted as sb(x). From each simulation, we adopt three quantities: the first rupture time, denoted tr, the time scale of the first phase of coalescence, denoted x = t − tr, and the temporal change in the total number of bubbles over the first phase of coalescence, denoted s(x). To analyze the bubble survival curves at which the bubbles coalesce for different initial foam sizes, the current number of bubbles sb(x) is normalized by the initial bubble number sb(0) as s(x) = sb(x)/sb(0), so that 0 ≤ s(x) ≤ 1. The bubble total numbers with the rescaled time for four initial foam sizes of 72, 128, 200, and 512 are self-similar despite initial various simulation parameters. All the bubble survival curves monotonically decrease with age regardless of their initial conditions.
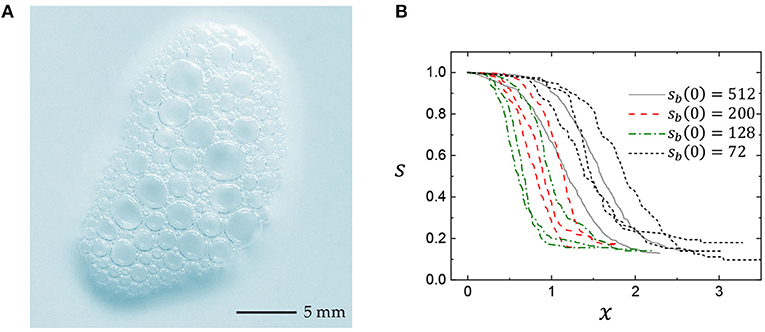
Figure 1. Foam coalescence. (A) Soap foam taken in a kitchen. (B) Bubble population dynamics of sequential coalescence events in clean foams from simulation results, reproduced by Dr. Peter Stewart (personal communication, see the Dataset 1) based on a network model [10] (see details on data collection and analysis in the Supplementary Note): showing the time-dependent changes in bubble numbers. The current number of bubbles is normalized by the initial number as s(x) = sb(x)/sb(0), so that 0 ≤ s(x) ≤ 1. The time after the first rupture time (tr) is rescaled as x = t − tr. The data for sb(0) = 72, 128, 200, and 512 were taken from a range of models: the datasets for 200 and 512 bubbles were generated using a simpler model than those for 72 and 128.
In the simulation work, the base-line pressure in the bubbles (P0), the liquid fraction (ϕ), the van der Waals attraction (S), and the lamellae thickness (h0) are the main parameters to reproduce the simulation results [10]. Importantly, the normalized simulation results are self-similar and almost invariant regardless of the simulation parameters in the range of 1.0 < P0 < 50.0 and 0.01 < ϕ < 0.05 for S = 10−10 and h0 ≪ 1 [10] (discussed with Dr. Peter Stewart). In this analysis study, we ignore the initial condition variances because the analysis results are almost invariant by the self-similarity of the coalescence events.
We find that the total number of bubbles monotonically decreases with age x, independent on the initial conditions, which can be fitted by an arbitrary sigmoidal function. To compare physical and biological survival curves with an identical sigmoidal function, the Boltzmann sigmoidal function is adopted as , where a1 and a2 are the initial and the final values, x0 is the critical value of the stimulus, and ω is a coefficient associated with the slope of the process during the transition [18]. The Boltzmann sigmoidal function, based on the logistic sigmoidal equation of s(x) = 1/[1 + ex], is an appropriate decay function for phase transition phenomena [18]. The sigmoidal patterns in s(x) are common in [8–11]. The mortality probability, μ(x) = −d ln[s(x)]/dx, is estimated from the fitting curves with the Boltzmann sigmoidal function for the survival probability s(x) of the bubbles in clean foams (see the fitting methods in the Supplementary Note).
3. Results and Discussion
The foam survival curves taken from Figure 1B (from the Dataset 1, reproduced by Dr. Peter Stewart) show good agreements between the data (solid lines) and the sigmoidal fits (dashed lines) (Figure 2A): the average values of a1 and a2 are almost identical as a1 ≈ 1.002568 and a2 ≈ 0.144341, whereas the other two parameters have a linear proportion as seen in Figure 2B. Therefore, a master survival curve (red line in Figure 2C) for clean foams could be obtained by adopting the average value of x0/ω ~ 6.254547 and by rescaling the age by (x − x0)/ω. All the bubble survival curves look to follow very well the master curve, indicating the self-similarity of the coalescence events, which was found by normalizing x/Δx (Δx = the length of the first phase of coalescence) [10], while by (x − x0)/ω in this study. From the fitted survival curves (dashed lines) in Figure 2A, the mortality curves (μ) are calculated with the rescaled age in Figure 2D. This result clearly shows that the mortality rates in clean foams increase exponentially with age, consistent with the Gompertz mortality law, regardless of the initial condition variances.
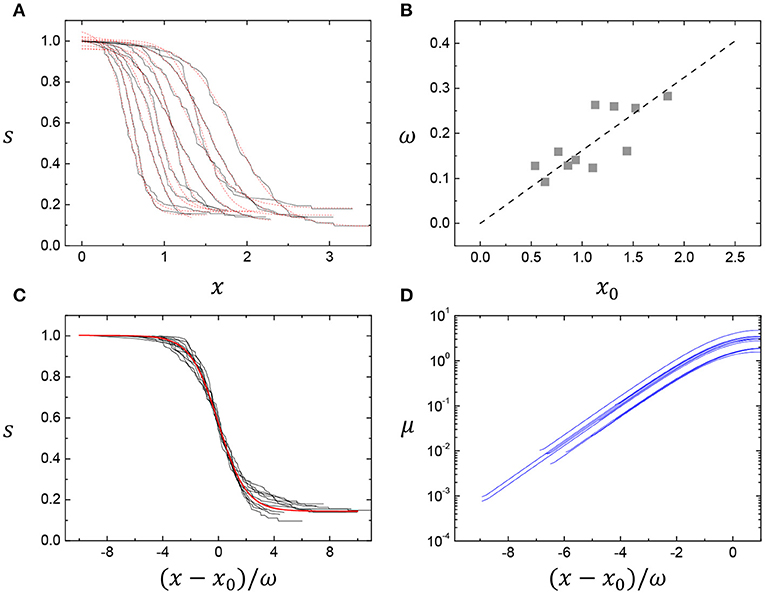
Figure 2. Sigmoidal and demographic analyses of bubble coalescence events in clean foams. (A) The normalized current number of bubbles with the rescaled time [from the Dataset 1, reproduced by Dr. Peter Stewart (personal communication) (solid lines), identical to Figure 1B] agrees well the Boltzmann sigmoidal function (dashed lines, see details on data collection and analysis in the Supplementary Note). (B) In the Boltzmann sigmoidal fits, two parameters, x0 and ω, have a linear proportion. (C) A master survival curve (solid red line) is achieved. (D) The death (mortality) curves, μ, are calculated from the fitted survival curves. This result clearly shows that the death rates in clean foams increase exponentially with age, consistent with the Gompertz mortality law, regardless of the initial conditions.
We repeatedly find the Gompertz mortality patterns in clean foams for the second simulation datasets (from the Dataset 2, reproduced by Dr. Peter Stewart) with the different sets for the larger initial numbers of bubbles as illustrated in Figure 3. The bubble population dynamics (s(x)) with age (x) shows the validity of the Boltzmann sigmoidal function fitting (Figure 3A) and the Gompertz mortality growth in the mortality curves (μ) with the rescaled age for clean foams (Figure 3B), independent on the initial condition variances. In particular, the mortality curves are almost identical regardless of the initial conditions (Figures 2D, 3B), suggesting the universality of the Gompertz mortality patterns in clean foams.
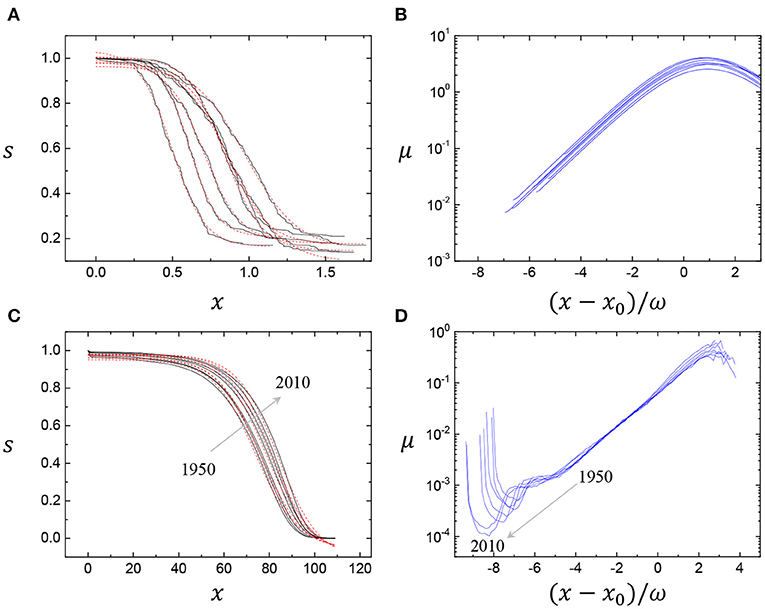
Figure 3. Gompertz mortality patterns for clean foams (A,B) and for humans (C,D). The clean foam coalescence data were analyzed for the Dataset 2 (reproduced by Dr. Peter Stewart). The human survival and mortality data were taken for the USA female period life tables from 1950 to 2010 from the Human Mortality Database (www.mortality.org). The Boltzmann sigmoidal function fits in (A) for clean foams and (C) for humans produce the Gompertz mortality growths in the mortality curves (μ) with the rescaled age in (B) for clean foams and (D) for humans (see details on data collection and analysis in the Supplementary Note).
We discuss the analogy of physical and biological survival curves. The human survival curves are characteristic biological survival curves. Biological aging includes a progressive deterioration of physiological function with age and an increase of vulnerability to disease and death [24, 25]. The Gompertz mortality law shows the exponential growth of the mortality rate commonly observed in many organisms, including mammals, flies, worms, plants, and yeast cells [24–26]. We obtain the survival and mortality data for humans from reliable statistical data in the Human Mortality Database (www.mortality.org), taking (in life tables, lx means the number of survivors at age normalized by 100,000 people). The Boltzmann sigmoidal function well fits the survival curves for humans (solid lines) (dashed lines) (Figure 3C). The mortality curves (μ) for humans (Figure 3D) were rescaled by (x − x0)/ω for comparisons with those for clean foams (Figures 2D, 3B). As well-known in the demography community, the survival curves shift upward from 1950 to 2010, and the mortality curves shift downward [24, 25, 27], because of the survival-mortality link as μ(x) = −d ln[s(x)]/dx. The exponential growth in μ in most adult ages, except infant and oldest ages, supports the validity of the Gompertz mortality law (Figure 3D). Here we can find similarities in the mortality curves between clean foam aging (Figures 2D, 3B) and human aging (Figure 3D), reproducing the Gompertz mortality law, without any adjustments. The slopes for both cases are identical as μ/[(x − x0)/ω] ≈ 0.5 during the exponential mortality growth. Here, the identical slope of μ is attributable to the similarity in the shape of the survival curves for clean foams and humans. Both cases originate from the irreversible monotonic decay dynamics of the survival probability. This similarity may imply the presence of a universal law in physical and biological aging dynamics.
A collection of radioactive atoms shows a simple exponential decay, which has a constant decay rate [28]. On the contrary, complex systems may show a form of non-exponential decays: most viscous materials close to the glass-transition temperature exhibit non-exponential relaxation [29, 30]. Non-exponentiality of physical aging is frequently described by the stretched exponential or Kohlrausch-Williams-Watts function [31, 32] or the Weibull function [33] as s(x) = exp[−(t/α)β] and 0 < β < 1 [34]. The biological survival curves are typically more complicated than the physical survival curves and can be fitted by a modified stretched exponential function [24, 25, 27], described as s(x) = exp(−uβ(u)), where the rescaled age u = x/α is relevant to the characteristic life (α) to make s(α) = exp(−1) and the stretched exponent β(u) = ln[−ln(s(x))]/ln(u) is an age-dependent variable, which differs from the classical stretched exponential function with a fixed β. In a survival curve, α indicates the scale effect and β indicates the shape effect in the curvature of a survival curve [24, 25]. Healthy biological populations strive to achieve the ideal rectangular survival curve [35], where β(u) tends to shift toward an ideal rectangular curve of β(u) = 7|ln(u)|−1 that corresponds to s(x) ≈ 1 at u < 1 and s(x) ≈ 0 at u > 1 [24, 25]. The upward (downward) shift in β at u < 1 (at u > 1) (Figure 4) is a typical feature of biological aging [24, 25].
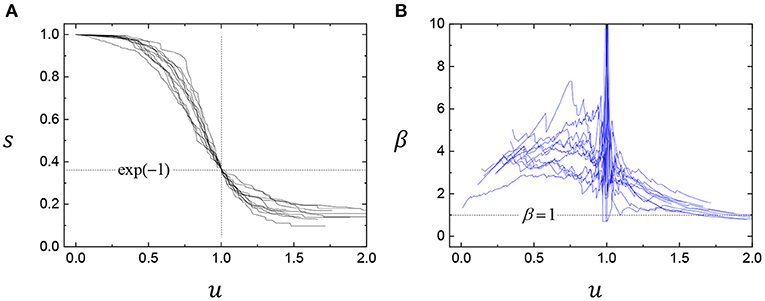
Figure 4. Nonexponentiality of survival curves in clean foams. (A) The survival curves of clean foams (from Figure 1B) are analyzed by the modified stretched exponential function, s(u) = exp(−uβ(u)), where the rescaled age u = x/α is relevant to the characteristic life (α) at s(α) = exp(−1) and the stretched exponent β(u) is age-dependent. (B) The age-dependence in β(u) is a typical feature found in biological aging [24, 25].
Clean foam models successfully reproduce the Gompertz mortality law, suggesting that clean foam aging resembles biological aging. For clean foams, each bubble would have different lifespans from many-body effects, and death (coalescence) occurs as stochastic events. Clean foams show collective interactions between bubbles: single events would affect the entire system's stability as a many-body effect. To provide insights into biological aging, physicists have suggested physical models imitate biological aging. The Penna model, where bit strings represent individual genomes [36], is one of the successful models for biological aging [37–39]. This model is suitable for testing evolutionary aging mechanisms through mutation accumulations, reproducing the Gompertz mortality law [38]. A recent study took living systems as large networks and tested how large networks can age [28]. This network model suggests that aging is a many-body effect, and mortality curves for different organisms can be similar because of strong interactions between individuals [28].
We evaluate the experimental results taken from coalescing and coarsening foams in the literature [[40, 41] for coarsening foams and [42] for coalescing foams] with the Boltzmann sigmoidal function, rescaled as in Figure 5A (see details on data collection and analysis in the Supplementary Note). The collapse of all data indicates the validity of the Boltzmann sigmoidal function. We find in Figure 5B that the mortality rates of the bubble population (μ) decrease exponentially with age at the positive (x − x0)/ω, which is equivalent with the exponential increase in μ at the negative (x − x0)/ω in Figures 2D, 3B, indicating the Gompertz law. The experimental results seem to reflect only the positive (x − x0)/ω dynamics.
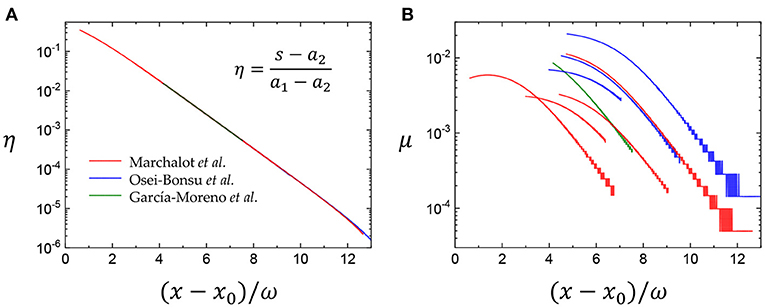
Figure 5. Comparison with experimental results in foams. (A) The Boltzmann sigmoidal function for the experimental data [[40, 41] for coarsening foams and [42] for coalescing foams, see details on data collection and analysis in the Supplementary Note] is rescaled as . The collapse of all data indicates the validity of the Boltzmann sigmoidal function. (B) The mortality rates μ decrease exponentially at the positive (x − x0)/ω, equivalent with the exponential increase at the negative (x − x0)/ω (Figures 2D, 3B), indicating the Gompertz law.
The current understanding of the coalescence dynamics in clean foams is still incomplete: further attention is required for the many-body effects for individual bubbles during sequential coalescence events. We test the Gaussian distributions of rupture times for clean foams and death rates for humans, as seen in Figure 6. The histogram of rupture times for clean forms follows the Gaussian distribution very well (Figure 6A). Similarly, the distribution of age-specific death (mortality) rates for humans shows the Gaussian distribution well (Figure 6B). The Gaussian distributions found in both systems would be a credible reason for the analogous survival curves. The analogy of the survival curves is restricted as: while the human survival curves tend toward zero as x increases, the bubble population in the clean foams evolves toward a constant value (s ~ 0.15) by the end of the first phase (Figure 3). The Gompertz mortality law of bubbles in clean foams appears for the first phase of bubble coalescence. In practice, the coalescence process would continue to much longer times (and further avalanches) [10]. Further study is required for the second phase of long-time bubble coalescence events [10].
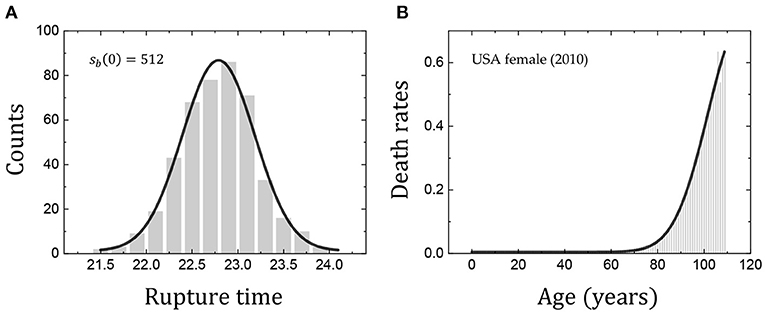
Figure 6. Gaussian distributions of rupture times taken from sb(0) = 512 (in the Dataset 1) (from Figure 1B) for clean foams (A) and of death rates taken from the USA female (2010, period 1x1) (from Figure 3D) for humans (B). The Gauss fits were carried out with the Origin software and described by the solid lines (adj. R2 = 0.9851 in A and adj. R2 = 0.9961 in B). The Gaussian distributions found in both systems would be a reason for the analogous survival curves.
4. Conclusion
To conclude, this study presents that clean foam aging is analogous to biological aging: the bubble mortality rate changes exponentially with time, demonstrating the Gompertzian aging dynamics. Presumably, the mortality curve universality is attributable to the Gaussian distributions of rupture times in clean foams and of death rates in humans. Both cases originate from the irreversible monotonic decay dynamics of the survival probability despite the differences in physical and biological aging mechanisms. This similarity may imply the presence of a universal law in physical and biological aging dynamics. For clean foams, aging occurs through sequential coalescence events. The bubble population dynamics in clean foams are well-described by a sigmoidal function, indicating that foam aging is a phase transition from non-equilibrium (life) to equilibrium (death) states. This result would help understand the structure and stability of clean foams.
Data Availability Statement
The original contributions presented in the study are included in the article/Supplementary Material, further inquiries can be directed to the corresponding author/s.
Author Contributions
BW conceived the study and collected the data. GO, MG, and BW analyzed the data and interpreted the results, and wrote and reviewed the manuscript. All authors contributed to the article and approved the submitted version.
Funding
This research was supported by Basic Science Research Program through the National Research Foundation of Korea (NRF) funded by the Ministry of Education (NRF-2016R1D1A1B01007133, 2019R1A6A1A03033215), and also supported by the Korea Evaluation Institute of Industrial Technology funded by the Ministry of Trade, Industry and Energy (20000423, Developing core technology of materials and processes for control of rheological properties of nanoink for printed electronics).
Conflict of Interest
The authors declare that the research was conducted in the absence of any commercial or financial relationships that could be construed as a potential conflict of interest.
Acknowledgments
We thank Dr. Peter Stewart for the simulation results and comments.
Supplementary Material
The Supplementary Material for this article can be found online at: https://www.frontiersin.org/articles/10.3389/fphy.2021.620568/full#supplementary-material
References
1. Banhart J, Stanzick H, Helfen L, Baumbach T. Metal foam evolution studied by synchrotron radioscopy. Appl Phys Lett. (2001) 78:1152–4. doi: 10.1063/1.1350422
2. Yang GF, Song KY, Joo SK. A metal foam as a current collector for high power and high capacity lithium iron phosphate batteries. J Mater Chem A. (2014) 2:19648–52. doi: 10.1039/C4TA03890H
3. Graner F, Jiang Y, Janiaud E, Flament C. Equilibrium states and ground state of two-dimensional fluid foams. Phys Rev E. (2000) 63:011402. doi: 10.1103/PhysRevE.63.011402
4. Miri M, Rivier N. The equilibrium state of 2D foams. Europhys Lett. (2001) 54:112. doi: 10.1209/epl/i2001-00236-0
5. Hilgenfeldt S, Koehler SA, Stone HA. Dynamics of coarsening foams: accelerated and self-limiting drainage. Phys Rev Lett. (2001) 86:4704. doi: 10.1103/PhysRevLett.86.4704
6. Cipelletti L, Ramos L. Slow dynamics in glasses, gels and foams. Curr Opin Colloid Interface Sci. (2002) 7:228–34. doi: 10.1016/S1359-0294(02)00051-1
7. Ishimoto Y, Morishita Y. Bubbly vertex dynamics: a dynamical and geometrical model for epithelial tissues with curved cell shapes. Phys Rev E. (2014) 90:052711. doi: 10.1103/PhysRevE.90.052711
8. Glazier JA, Gross SP, Stavans J. Dynamics of two-dimensional soap froths. Phys Rev A. (1987) 36:306. doi: 10.1103/PhysRevA.36.306
9. Ritacco H, Kiefer F, Langevin D. Lifetime of bubble rafts: cooperativity and avalanches. Phys Rev Lett. (2007) 98:244501. doi: 10.1103/PhysRevLett.98.244501
10. Stewart PS, Davis SH. Self-similar coalescence of clean foams. J Fluid Mech. (2013) 722:645. doi: 10.1017/jfm.2013.145
11. Herdtle T, Aref H. Numerical experiments on two-dimensional foam. J Fluid Mech. (1992) 241:233–60. doi: 10.1017/S0022112092002027
12. Drenckhan W, Langevin D. Monodisperse foams in one to three dimensions. Curr Opin Colloid Interface Sci. (2010) 15:341–58. doi: 10.1016/j.cocis.2010.06.002
13. Duplat J, Bossa B, Villermaux E. On two-dimensional foam ageing. J Fluid Mech. (2011) 673:147–79. doi: 10.1017/S0022112010006257
14. Roth A, Jones C, Durian DJ. Bubble statistics and coarsening dynamics for quasi-two-dimensional foams with increasing liquid content. Phys Rev E. (2013) 87:042304. doi: 10.1103/PhysRevE.87.042304
15. Roth A, Chen B, Durian DJ. Structure and coarsening at the surface of a dry three-dimensional aqueous foam. Phys Rev E. (2013) 88:062302. doi: 10.1103/PhysRevE.88.062302
16. Partridge L, Mangel M. Messages from mortality: the evolution of death rates in the old. Trends Ecol Evol. (1999) 14:438–42. doi: 10.1016/S0169-5347(99)01646-8
17. Gompertz B. XXIV. On the nature of the function expressive of the law of human mortality, and on a new mode of determining the value of life contingencies. In a letter to Francis Baily, Esq. FRS &c. Philos Trans R Soc Lond. (1825) 115:513–83. doi: 10.1098/rstl.1825.0026
18. Navarro-Verdugo AL, Goycoolea FM, Romero-Meléndez G, Higuera-Ciapara I, Argüelles-Monal W. A modified Boltzmann sigmoidal model for the phase transition of smart gels. Soft Matter. (2011) 7:5847–53. doi: 10.1039/c1sm05252g
19. Schwartzkopf M, Buffet A, Körstgens V, Metwalli E, Schlage K, Benecke G, et al. From atoms to layers: in situ gold cluster growth kinetics during sputter deposition. Nanoscale. (2013) 5:5053–62. doi: 10.1039/c3nr34216f
20. García-Moreno F, Rack A, Helfen L, Baumbach T, Zabler S, Babcsán N, et al. Fast processes in liquid metal foams investigated by high-speed synchrotron x-ray microradioscopy. Appl Phys Lett. (2008) 92:134104. doi: 10.1063/1.2905748
21. Anderson AM, Brush LN, Davis SH. Foam mechanics: spontaneous rupture of thinning liquid films with Plateau borders. J Fluid Mech. (2010) 658:63. doi: 10.1017/S0022112010001527
22. Weon B, Kim J, Je J, Yi J, Wang S, Lee WK. Colloid coalescence with focused x rays. Phys Rev Lett. (2011) 107:018301. doi: 10.1103/PhysRevLett.107.018301
23. Weon BM, Je JH. Coalescence preference depends on size inequality. Phys Rev Lett. (2012) 108:224501. doi: 10.1103/PhysRevLett.108.224501
24. Weon BM, Je JH. Plasticity and rectangularity in survival curves. Sci Rep. (2011) 1:1–5. doi: 10.1038/srep00104
25. Weon BM, Je JH. Trends in scale and shape of survival curves. Sci Rep. (2012) 2:1–7. doi: 10.1038/srep00504
26. Vaupel JW, Carey JR, Christensen K, Johnson TE, Yashin AI, Holm NV, et al. Biodemographic trajectories of longevity. Science. (1998) 280:855–60. doi: 10.1126/science.280.5365.855
27. Weon BM, Je JH. Theoretical estimation of maximum human lifespan. Biogerontology. (2009) 10:65–71. doi: 10.1007/s10522-008-9156-4
28. Vural DC, Morrison G, Mahadevan L. Aging in complex interdependency networks. Phys Rev E. (2014) 89:022811. doi: 10.1103/PhysRevE.89.022811
29. Angell CA. Formation of glasses from liquids and biopolymers. Science. (1995) 267:1924–35. doi: 10.1126/science.267.5206.1924
30. Debenedetti PG, Stillinger FH. Supercooled liquids and the glass transition. Nature. (2001) 410:259–67. doi: 10.1038/35065704
31. Kohlrausch R. Theorie des elektrischen Rückstandes in der Leidener Flasche. Ann Physik. (1854) 167:179–214. doi: 10.1002/andp.18541670203
32. Williams G, Watts DC. Non-symmetrical dielectric relaxation behaviour arising from a simple empirical decay function. Trans Faraday Soc. (1970) 66:80–5. doi: 10.1039/tf9706600080
33. Weibull W. A statistical distribution function of wide applicability. J Appl Mech. (1951) 18:293–7. doi: 10.1115/1.4010337
34. Hodge IM. Physical aging in polymer glasses. Science. (1995) 267:1945–7. doi: 10.1126/science.267.5206.1945
35. Fries JF. Aging, natural death, and the compression of morbidity. N Engl J Med. (1980) 303:130–250. doi: 10.1056/NEJM198007173030304
36. Penna T. A bit-string model for biological aging. J Stat Phys. (1995) 78:1629–33. doi: 10.1007/BF02180147
37. Schwämmle V, de Oliveira SM. Simulations of a mortality plateau in the sexual Penna model for biological aging. Phys Rev E. (2005) 72:031911. doi: 10.1103/PhysRevE.72.031911
38. Coe J, Mao Y, Cates M. Solvable senescence model showing a mortality plateau. Phys Rev Lett. (2002) 89:288103. doi: 10.1103/PhysRevLett.89.288103
39. Coe J, Mao Y. Gompertz mortality law and scaling behavior of the Penna model. Phys Rev E. (2005) 72:051925. doi: 10.1103/PhysRevE.72.051925
40. Marchalot J, Lambert J, Cantat I, Tabeling P, Jullien MC. 2D foam coarsening in a microfluidic system. Europhys Lett. (2008) 83:64006. doi: 10.1209/0295-5075/83/64006
41. Osei-Bonsu K, Shokri N, Grassia P. Foam stability in the presence and absence of hydrocarbons: from bubble-to bulk-scale. Colloids Surf A Physicochem Eng Asp. (2015) 481:514–26. doi: 10.1016/j.colsurfa.2015.06.023
Keywords: foam, bubble, coarsening, coalescence, aging, Gompertz law of mortality
Citation: Oh G, Gonçalves M and Weon BM (2021) Gompertz Law in Clean Foam Coalescence. Front. Phys. 9:620568. doi: 10.3389/fphy.2021.620568
Received: 23 October 2020; Accepted: 13 April 2021;
Published: 14 May 2021.
Edited by:
Giancarlo Ruocco, Italian Institute of Technology (IIT), ItalyReviewed by:
Peter Stewart, University of Glasgow, United KingdomNeda Ghofraniha, National Research Council (CNR), Italy
Copyright © 2021 Oh, Gonçalves and Weon. This is an open-access article distributed under the terms of the Creative Commons Attribution License (CC BY). The use, distribution or reproduction in other forums is permitted, provided the original author(s) and the copyright owner(s) are credited and that the original publication in this journal is cited, in accordance with accepted academic practice. No use, distribution or reproduction is permitted which does not comply with these terms.
*Correspondence: Byung Mook Weon, bmweon@skku.edu
†These authors have contributed equally to this work