- 1 Center for Mind/Brain Sciences, University of Trento, Trento, Italy
- 2 Laboratory of Integrative Neuroscience, School of Exact and Natural Sciences, University of Buenos Aires, Buenos Aires, Argentina
- 3 Neuroinformatics Laboratory, Bruno Kessler Foundation, Trento, Italy
- 4 Department of Cognitive Sciences, University of Trento, Rovereto, Italy
The operations and processes that the human brain employs to achieve fast visual categorization remain a matter of debate. A first issue concerns the timing and place of rapid visual categorization and to what extent it can be performed with an early feed-forward pass of information through the visual system. A second issue involves the categorization of stimuli that do not reach visual awareness. There is disagreement over the degree to which these stimuli activate the same early mechanisms as stimuli that are consciously perceived. We employed continuous flash suppression (CFS), EEG recordings, and machine learning techniques to study visual categorization of seen and unseen stimuli. Our classifiers were able to predict from the EEG recordings the category of stimuli on seen trials but not on unseen trials. Rapid categorization of conscious images could be detected around 100 ms on the occipital electrodes, consistent with a fast, feed-forward mechanism of target detection. For the invisible stimuli, however, CFS eliminated all traces of early processing. Our results support the idea of a fast mechanism of categorization and suggest that this early categorization process plays an important role in later, more subtle categorizations, and perceptual processes.
Introduction
The human brain continuously performs visual categorization of stimuli in everyday life. Studies of rapid visual categorization suggest that the first 100–200 ms are crucial to this process, consistent with categorization during the first pass of visual processing (Potter and Faulconer, 1975; VanRullen and Thorpe, 2001b; Liu et al., 2009). For go/no go tasks, for example, early event-related potentials (ERPs) at approximately 150 ms reflect the decision that there was a target present in a natural scene (Thorpe et al., 1996; VanRullen and Thorpe, 2001b). This first rapid categorization appears to be similar for diverse categories such as means of transportation or living objects (Thorpe and Fabre-Thorpe, 2001; VanRullen and Thorpe, 2001a).
An open issue regards the capacity of invisible stimuli to influence visual categorization and to activate different areas of the visual cortex. Experiments employing change blindness and inattentional blindness have clearly documented that important visual events that impinge on our retina can go widely unseen when attention is diverted from them (Mack and Rock, 1998; Simons and Chabris, 1999). On the other side, it has been also demonstrated that visual category detection can be rapidly achieved even in the near absence of visual attention (Fei-Fei et al., 2002). Psychophysical studies using interocular suppression as well as neuroimaging studies have given conflicting reports on the degree to which suppressed information activates areas of the brain (Blake and Logothetis, 2002; Alais and Melcher, 2007). Visual information arriving to the ventral stream appears to be deeply suppressed under interocular suppression, as shown by psychophysics (Zimba and Blake, 1983; Alais and Melcher, 2007), neurophysiological data in monkeys (Logothetis, 1998), and in single-cell recordings in humans (Kreiman et al., 2005). However, it has recently been proposed that there is a difference between the ventral and dorsal stream for the processing of invisible pictures of animals and tools (Fang and He, 2005; Almeida et al., 2008, 2010). It was suggested that while dorsal stream neurons responded to invisible tools that carried the characteristic of being “graspable” the categorization of invisible animals was widely suppressed in the ventral stream (Fang and He, 2005).
Even though the human and non-human primate brain can achieve visual categorization very fast, theories of visual awareness propose that a rapid feed-forward mechanism might not be sufficient for visual awareness, which might also require horizontal connections between different brain areas (Lamme, 2000) and/or late feedback projections from prefrontal areas (Sergent et al., 2005). The study of the neural correlates of invisible stimuli is valuable to disentangle the minimal set of processes that are necessary and sufficient for visual awareness to occur (Koch, 2003). Further investigation is needed to understand the relationship between the first, rapid feed-forward pass of information and the emergence of visual awareness.
We studied the timing of categorization of seen and unseen images of animals, tools, and scrambled control images employing continuous flash suppression (CFS; Tsuchiya and Koch, 2005), EEG recordings and single trial analysis. Based on the EEG signal, our classifiers were able to predict the visual category on single trials of seen but not unseen stimuli. Fast categorization of conscious images could be detected around 100 ms on the occipital electrodes, suggesting a fast, feed-forward mechanism responsible for the fast recognition of visual categories. For the unconscious images of animals and tools, however, no trace of a distinction between semantic categories was found in the EEG signal. The claim that processing of unseen tools but not of unseen animals can occur in the dorsal stream (Fang and He, 2005) was not be replicated with EEG recordings. Overall, these results provide further evidence that categorization occurs early in visual processing (VanRullen and Thorpe, 2001b; Hung et al., 2005) and that this early, initial and (perhaps) approximate categorization plays a role in later semantic processing and in conscious awareness.
Materials and Methods
Participants
We recruited 12 students – 2 female and 10 male, mean age 26.7 ranging from 21 to 31-year-old from the university of Buenos Aires for the experiment. All subjects had normal or corrected to normal visual acuity and were tested for ocular dominance before running the experiment. All participants gave written informed consent and were naive about the aims of the experiment. All the experiments described in this paper were reviewed and approved by the ethics committee of the Centre of Medical Education and Clinical Research “Norberto Quirno” (CEMIC), qualified by the Department of Health and Human Services (HHS, USA).
Stimuli
For the current experiments we used 50 images of animals, 50 tools (all downloaded from the Internet), 100 phase-scrambled control images (Figure 1) and a set of 40 Mondrians images (Tsuchiya and Koch, 2005; Figure 2A). All images of animals and tools were converted into gray-scale images with a maximum brightness intensity value of 0.8 for every pixel in the image, while background pixels were turned into a value of 0.5 pixel brightness in a scale from 0 = black to 1 = white. No images with strong emotional saliency were used for this study (such as spiders, snakes, or guns). We created 100 control phase-scrambled images (one scrambled image for each animal and tool image) with the same spatial frequencies and mean luminance values as the animal and tool images. In order to generate these images we applied the Fourier transform to each picture of an animal, tool, or Mondrian and obtained the respective magnitude and phase matrices. We then reconstructed each image by using the magnitudes of the animals and tools and the phases of the Mondrian images. Finally, we multiplied each single pixel of the scrambled images by an appropriate constant to correct for any differences in mean luminance values between the original images and their scrambled counterparts. A t-test comparing the mean luminance value of the group of original images and the group of scrambled images showed no significant difference between both groups (p = 0.6).
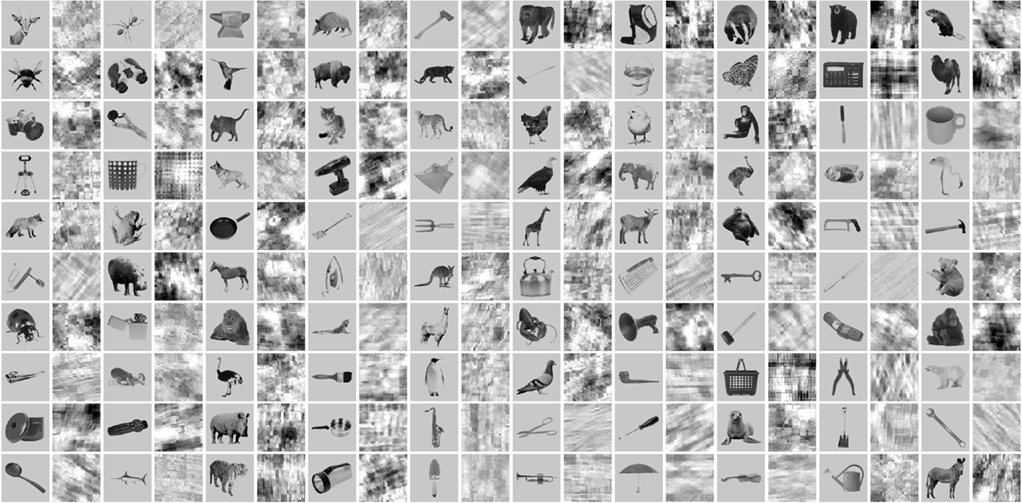
Figure 1. The set of 200 stimuli used in the experiments: 50 animals, 50 tools, and 100 phase-scrambled controls.
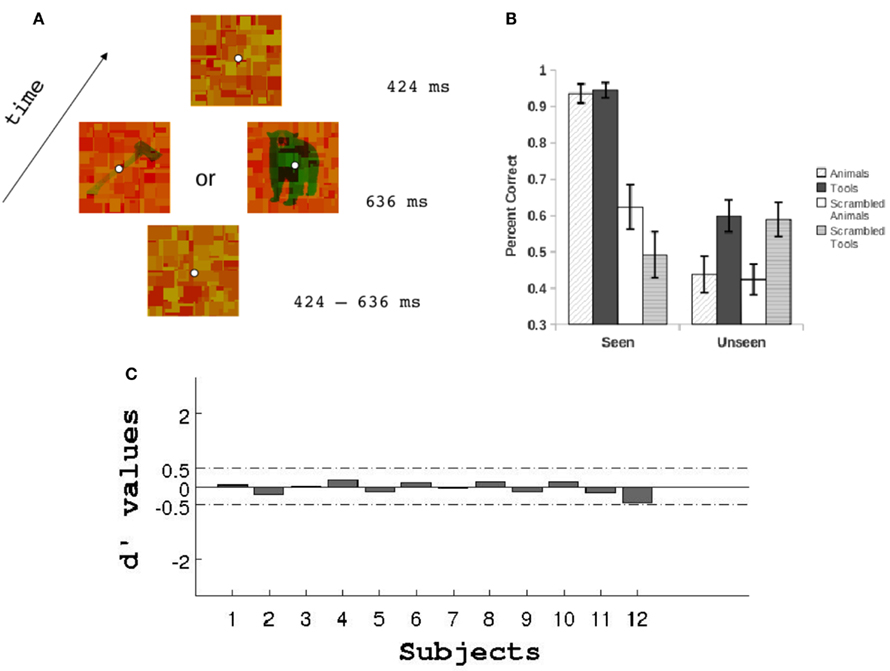
Figure 2. (A) Schematic trial representation. Mondrians were changed on the screen every 106 ms (see Materials and Methods). (B) Performance at discriminating the categories of stimuli for the seen and unseen conditions. (C) Objective assessment of invisibility. Prior to the main experiment we conducted a detection task with targets at different low luminances to be sure that subjects were completely unaware of the target stimuli under the unseen conditions (see Materials and Methods). This task was identical to that depicted in (A) but with half the trials presenting a blank screen instead of a target. Subjects had to report whether they had seen a target or nothing. Signal detection theory was used to estimate for each subject the target luminance values that yielded a d’ between −0.5 and 0.5. In addition to this objective measure of invisibility subjects reported to be completely unaware of any targets presented at low luminance during CFS.
Procedures
Stimuli were presented using a PC computer with a CRT display monitor (resolution = 1024 × 768, 75 Hz refresh rate) using Matlab Psychophysics toolbox (Brainard, 1997). Subjects were instructed to fixate on a central point while they viewed a series of pictures through a pair of red–green anaglyph glasses. These pictures were viewed from a distance of 70 cm at the center of the screen and subtended 8 by 8 degrees of visual angle. To allow competition between stimuli on each trial we randomly selected 10 Mondrians from the set of 40 Mondrians and presented them to the red RGB channel of the image every eight frames (10 Hz, each Mondrian presentation lasting 106 ms). Target animals, tools, and scrambled images were presented to the green RGB channel of the image. In this way, subjects using the anaglyph glasses saw the Mondrians with their dominant eye and the animals, tools, and scrambled images with their non-dominant eye.
Throughout a trial Mondrians were dynamically changed every 106 ms. Trials initiated with a period of 424–636 ms (4, 5, or 6 flashes) of Mondrian presentation followed by the targets plus the Mondrians for 636 ms. Once the targets disappeared, four more Mondrians were flashed on the screen to avoid afterimages (Figure 2A). For the seen conditions targets were presented at low luminance and Mondrians at high luminance whereas for the unseen condition targets were presented at a low luminance and Mondrians at high luminance. These luminance levels were chosen for each subject based on a visibility threshold detection task (see below). On each trial, subjects were asked to fixate at the center of the screen to avoid eye movements. Their task was to respond with their right hand index or middle fingers whether the picture of an animal or tool had appeared on the screen (2AFC). An inter-trial interval of either 1, 1.5, or 2 s was used in between trials to avoid attentional expectations. The EEG experiment comprised eight conditions: four stimuli types (animals, tools, scrambled animals, and scrambled tools) by two visibility levels (seen and unseen). The EEG experiment consisted of 800 trials (100 trials per condition by 8 condition) and lasted approximately 70 min.
Invisibility assessment
On a previous session to running the EEG recordings subjects performed a visibility threshold detection task. We presented the targets at six different luminance levels while Mondrians were kept constant. The trial presentation was exactly the same as the sequence described above (see Figure 2A) with the only exception that instead of scrambled images a blank green screen of the same luminance as target images was presented on half the trials to the dominant eye. Subjects had to respond whether they had seen a target (animal or tool) or a blank screen. Using signal detection theory we calculated the d’ values for each luminance condition to obtain a measure of each subjects visibility threshold (see Figure 2C). Finally, we chose a luminance value that yielded a d’ between −0.5 and 0.5. This luminance value was then assigned to the targets in the unseen conditions and to the Mondrians in the seen conditions for the EEG experiment. For all subjects, the final targets were presented against a green background (CIE coordinates X = 0.414, Y = 0.391) with a maximum luminance of 4.8 cd/m2. When presented at low luminance the mean pixel intensities for the gray-scale targets was 0.26 (in a scale from 0 to 1) with an STD of 0.02. For the gray-scale high luminance targets and Mondrians the mean pixel intensities was 0.71 with an STD of 0.06. CFS allowed us to present constant stimuli to our subjects while they underwent two conditions: a visible condition in which pictures were consciously perceived and an invisible condition in which participants were not able to report the presence nor the identity of the suppressed stimuli.
Preprocessing of EEG Data
EEG activity was recorded on a dedicated PC at 1024 Hz, at 128 electrode positions on a standard 10–20 montage, using a BrainVision electrode system1. An additional electrode at the right ear lobe was used as reference. Datasets were bandpass filtered (1–120 Hz), down sampled at 300 Hz, and independent component analysis (ICA) was run on the continuous datasets to detect components associated with eye blinks, eye movements, electrical noise, and muscular noise. The resulting components from the ICA decomposition were visually inspected and the ones associated with eye blinks and eye movements were manually eliminated from the data. Channels containing artifact noise for long periods of time were interpolated (a maximum of four channels were interpolated for each dataset). The datasets were notch filtered at 50 Hz to clear out electrical noise, and the reference on the right ear was digitally transformed into an averaged reference. Once this process was finished the datasets were epoched in synchrony with the beginning of the target presentation and each epoch was corrected for baseline over a 400-ms window during fixation at the beginning of the trial. An automatic method was applied to discard those trials with voltage exceeding 200 mv, transients exceeding 80 mv, or oculogram activity larger than 80 mv. The remaining trials were then separated accordingly to the experimental conditions and averaged to create the ERPs. On average, 15% of trials were discarded after artifact removal. All the preprocessing steps were performed using EEGLAB (Delorme and Makeig, 2004) and custom made scripts in Matlab.
Statistical Analysis of ERPs
In order to assess the earliest time point in visual categorization we initially ran statistical comparisons between seen animals versus seen scrambled animals and seen tools versus seen scrambled tools (Figure 3). In all cases we submitted each (channel, time) sample of the ERP calculated for each subject to a non-parametric rank-sum test to compare the two conditions across all subjects. This implied over 5000 comparisons for each pair of conditions. We filtered these multiple comparisons across time samples and recording sites with the following criteria. (1) We kept only samples with p < 0.01. (2) For each channel, a given time point was considered significant if it was part of a cluster of six or more consecutive significant consecutive time points for a 19.5-ms time window (Thorpe et al., 1996; Dehaene et al., 2001). (3) Each sample was considered significant if for the same time point at least two neighboring channels were also significant.
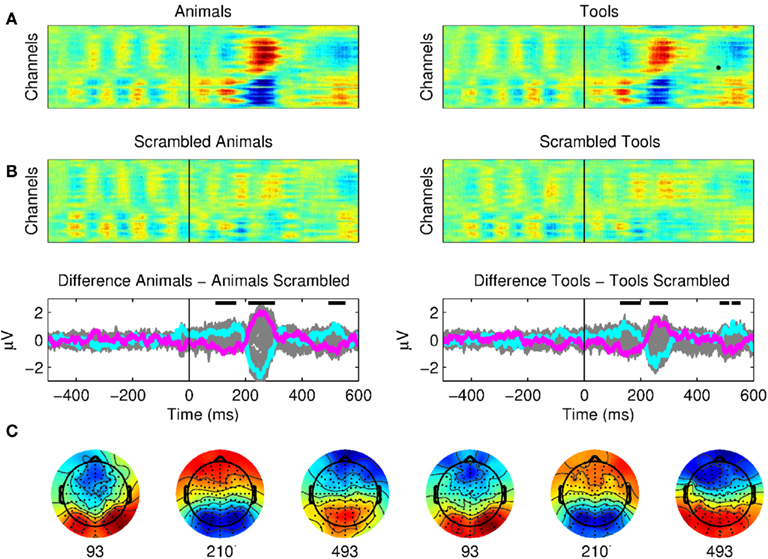
Figure 3. The earliest correlates of visual categorization. (A) Raster plots of seen animals, tools, and their scrambled control conditions. The presentation of meaningful stimuli (animal or tool) produced three distinctive components with respect to the scrambled pictures with onsets at 100, 200, and 500 ms. The signals prior to the stimuli onset correspond to the Mondrians presentation. These plots show the average of 12 subjects. (B) The difference between seen animals versus seen scrambled animals and seen tools versus seen scrambled tools are plotted for all channels (gray) and as particular examples for channel “OZ = cyan” and “FZ = magenta.” The activity of Mondrians is canceled by the subtraction between conditions. Upper dark bars indicate the time points where the signals showed statistical differences for both conditions (see Results). (C) Topographical maps show brain activity at the beginning of each period of significance.
Single Trial Classification
We employed multivariate pattern analysis (Hanke et al., 2009) to decode visual information from the EEG recordings in single trials. All the analysis were performed in Python language adopting the software library PyMVPA2. For each channel we assessed the time and frequency intervals within the EEG signals that carried the biggest amount of stimuli-related information and maximized the separability between the stimuli categories. The amount of information was estimated as the accuracy of a classifier trained on single trials at predicting the stimuli of future trials. A dimensionality reduction step was performed before each classification process via a variable selection step, and for this study the selected variables were time intervals. Variable selection was conducted in order to discard irrelevant information for classification and improve the signal-to-noise ratio.
For each subject, the dataset consisted in 3D matrices of 300 sample points by 128 channels by 800 trials containing voltage values. For all the analysis we used a sixfold cross-validation procedure in order to estimate the accuracy of classification. We refer to “classification performance” of a subject as the average of the six classification accuracies obtained on the test set of each fold. At each one of the iterations of the cross-validation scheme the following variable selection procedure was applied to the training dataset. First, we computed the mean and SD for each timestep and class over all training trials. Then, for each timestep and each channel we ran a one-way ANOVA between the two classes to compare the differences in signal amplitude. Next, we obtained a vector with 300 p-values (one for each sample point). The 300 time points correspond to the time range 0–1000 ms after stimuli presentation. The first 100 timesteps with the lowest p-value were selected for each channel and used as feature values to set up a final dataset where each trial is a vector of 100 features. Finally, the resulting dataset was used to train a classifier with a support vector machine (SVM) algorithm (Schlkopf and Smola, 2001) and linear kernel.
The corresponding test dataset for the given iteration of the cross-validation scheme was reduced to the 100 features selected on the training dataset. In order to avoid circularity analysis during variable selection we performed the feature selection process jointly with the cross-validation process for each step of the multivariate analysis (Olivetti et al., 2010). For each fold of the cross-validation process the SVM algorithm produced a classifier for each channel and the related accuracy on the test set was used to evaluate the informativeness of the channel. The result of these classification processes yielded a measure of the information contained in each channel to discriminate stimuli categories, a “single-channel based decoding.” To obtain a global measure of classification or “all-channel based decoding” (see Results), we performed the same procedure as before with the exception that during training the 128 channels were concatenated and 12800 features were selected, i.e., the best 100 features for each of the 128 channels. The classification performance of an SVM classifier was estimated by means of cross-validation.
Results
Behavioral Responses
We ran eight Bonferroni corrected one-sample t-tests (one for each condition, Figure 2B) against the null hypothesis that subjects were performing at chance level. Subjects were above 90% accuracy at discriminating animals and tools on the seen condition (corrected p < 0.05). For the remaining six conditions none of the tests rejected the null hypothesis that participants were responding differently from chance level.
ERP Analysis
First of all, we found three main components that distinguished targets (animals or tools) from scrambled images (Figure 3; see Materials and Methods for details on statistical criteria for ERPs comparisons). The earliest differences between ERPs of seen animals and seen scrambled animals, and seen tools and seen scrambled tools were observed for a P1 component at occipital electrodes. These components had a statistically significant onset starting at 93 ms for animals and 127 ms for tools. These results are in agreement with previous studies showing early categorization around 100 ms (Thorpe et al., 1996; Fabre-Thorpe et al., 2001; VanRullen and Thorpe, 2001b; Rousselet et al., 2007). We also observed a second N2 component with a peak starting at ∼230 ms and a third late component with a peak starting at ∼490 ms. The pictures of seen animals or seen tools produced a widespread activation throughout the cortex as compared to the seen scrambled controls.
Second, we assessed the EEG signal associated with the presentation of unseen pictures of animals or tools (Figure 4). We observed no EEG correlates of unseen stimuli as compared with their unseen scrambled controls. Even the earliest P1 components were eliminated under CFS, suggesting that under interocular suppression unseen stimuli were completely suppressed before 100 ms or that they were too weak to be detected with ERPs.
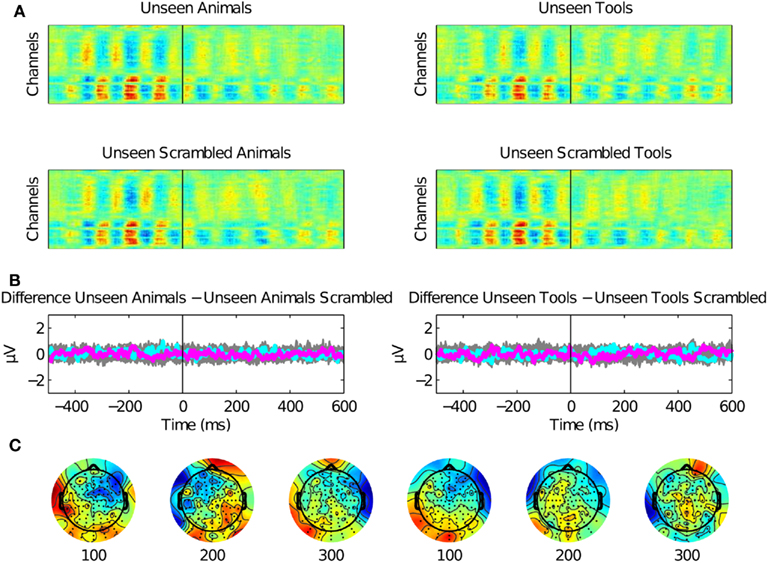
Figure 4. Rapid categorization is suppressed for unseen stimuli. (A) Raster plots for stimuli presented under invisibility conditions. These plots show the average of 12 subjects. (B) No ERP components were present in the data except for the activity associated to the Mondrians presentation. The difference between unseen animals versus unseen scrambled animals and unseen tools versus unseen scrambled tools are plotted for all channels (gray) and as particular examples for channel “OZ = cyan” and “FZ = magenta.” The activity of Mondrians is canceled by the subtraction between conditions. (C) Topographical maps show no particular information regarding unseen stimuli. CFS eliminated even the earliest EEG correlates of image categorization.
Third, we found no differences in the ERPs for the subtler semantic categorization of animals versus tools (Figure 5). For the seen targets the EEG activity related to the two categories was almost identical, with only an N2 component that (even though not statistically significant) could be still observed around 200 ms after the subtraction of the two categories. For the unseen targets even the earliest components were nor present.
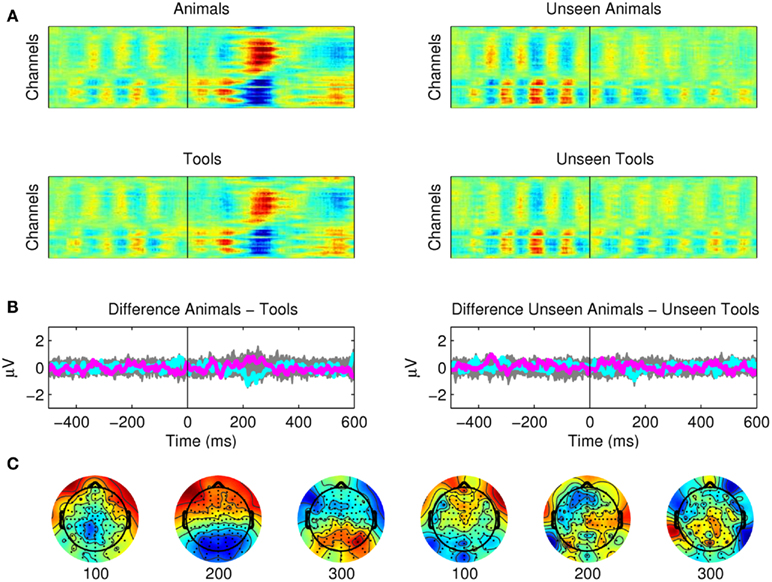
Figure 5. Semantic categorization between animals and tools. (A) Raster plots showing activations for seen animals and seen tools. These plots show the average of 12 subjects. (B) The subtler semantic categorization of animals versus tools could not be observed from the EEG recordings. The difference between seen animals versus seen tools and unseen animals versus unseen tools are plotted for all channels (gray) and as particular examples for channel “OZ = cyan” and “FZ = magenta.” The activity of Mondrians is canceled by the subtraction between conditions. (C) However, even if it was not statistically significant, some type of small potential was still present after the subtraction around 200 ms. We investigated this activity employing a multi pattern analysis approach (Figure 7).
The failure in categorizing unseen stimuli could have been simply a matter of insufficient signal-to-noise ratio: the target images could have been too weak to produce a reliable EEG signal. In order to rule out this possibility we ran a control experiment (n = 6) with two conditions. We presented the same target and scrambled images as in the unseen condition: (1) without presenting any Mondrian and (2) with low luminance Mondrians presented to the dominant eye. We found that the targets animals and tools produced a significant cortical response at P1 and N2 components (Figure 6). This result implies that the lack of signal under the unseen conditions (Figure 4) cannot be attributed to weak visual stimulation. On the other hand, it remains possible that some form of interaction between the CFS Mondrian sequence and our already weak target stimuli could have wiped out the corresponding signals at an early cortical stage. While this possibility is, to some extent, compatible with our conclusions, it would be useful in future studies to observe the ERP signals (or lack thereof) generated by unseen stimuli that are physically matched to the consciously seen ones (e.g., in a condition where the same target stimulus sometimes becomes conscious and generates an ERP and sometimes remains unconscious with no associated ERP).
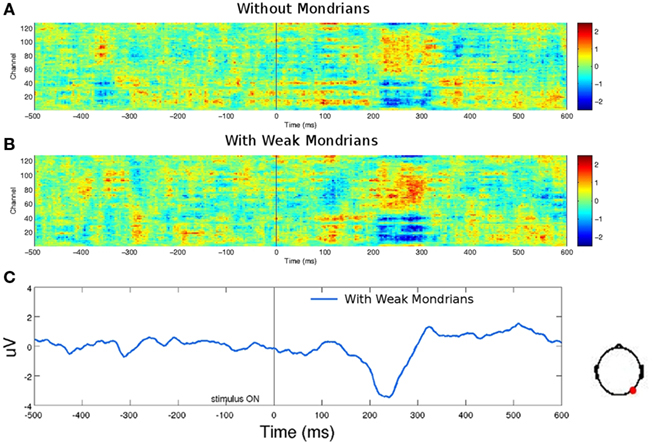
Figure 6. EEG signal-to-noise ratio of targets. (A) Low luminance targets (animals and tools together) generated a reliable EEG response when presented to one eye without Mondrians to the other eye, and (B) with low luminance Mondrians in the dominant eye. Six subjects participated in this control experiment. (C) One occipital channel from (B) is shown as an example of the components generated by low luminance targets. For both conditions (A,B), subjects were above 95% accuracy at discriminating the category of the stimuli and reported seeing the stimuli without difficulty. The absence of an EEG response to unseen targets (Figure 4) cannot be explained by low signal-to-noise ratio of low luminance targets.
EEG Single Trial Analysis
We employed single trial multivariate analysis for all the conditions in our data (Figure 7). We designed our classification approach with an emphasis on avoiding biases in the process of feature extraction and parameter estimation implementing a nested cross-validation scheme (Olivetti et al., 2010). For the seen conditions the classifiers were able to discriminate well above chance animals from scrambled animals with an accuracy of 72% and tools from scrambled tools with an accuracy of 66% (p < 0.0001). A 55% of accuracy was obtained for the comparison of seen animals versus seen tools, which was statistically suggestive (p = 0.0518) but not significant at the confidence level of 0.01 that we adopted in this work. This suggestive classification performance could not be attributed to low-level image statistics, as the comparison seen scrambled animals versus seen scrambled tools was at chance level. The most important channels contributing to the classification performance can be observed in Figure 8. For the discrimination between seen animals, tools, and their scrambled controls the occipital electrodes conveyed the highest discriminative information. For the seen animal versus tool classification performance our results were lower than in previous EEG and MEG studies (Murphy et al., 2009; Simanova et al., 2010; Chan et al., 2011). We speculate that our results might differ from these studies due to two reasons. First, the relative small number of trials used in our study (100 per category) compared to previous works [i.e., Murphy et al. (2009, 2010)] could have made the classifier and the estimation of its accuracy less precise. Second, the additive noise effect of the low luminance Mondrians in the seen conditions might have reduced the signal-to-noise ratio thus decreasing the accuracy of the classifier.
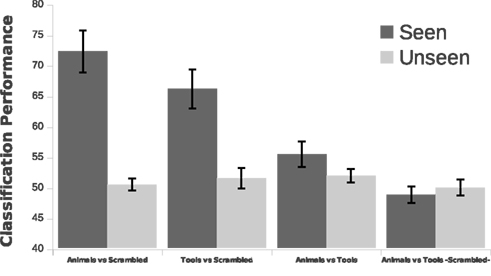
Figure 7. Single trial classification performance. Multivariate pattern analysis was used to discriminate between experimental conditions. The classifier was well above chance level for the discrimination of seen animals and seen tools against their scrambled controls (p < 0.0001). Also, the classifier found suggestive evidence of a discrimination between seen animals and seen tools categories but the final classification performance was not significant at the confidence level of 0.01 that we adopted in this work (p = 0.0518). The classifier was at chance level at discriminating scrambled animals from scrambled tools (p > 0.05). Seen animals versus seen tools was higher than the discrimination of seen scrambled animals versus seen scrambled tools (p < 0.01). This implies that low-level image statistics cannot account for the decoding of animals versus tools. For all the unseen stimuli comparisons the classification performance was at chance level. The classifier was run on each subject separately and the final classification performance was obtained from the average across subjects. Error bars depict one SEM.
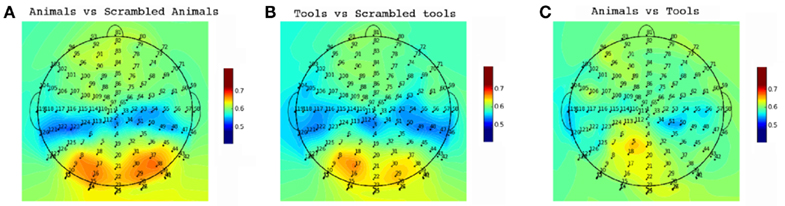
Figure 8. Single Trial Channel Sensitivity. Classification accuracy for each channel for the conditions with seen targets. Each color represents the cross-validated accuracy of the classifier specific to each channel. For the comparisons of meaningful pictures against meaningless pictures (A,B) the occipital electrodes were of most importance. These occipital electrodes were not determinant for subtler semantic categorizations (C).
Discussion
The primate visual system can accomplish complex visual stimuli processing in a fraction of a second. Fast visual categorization can occur in human and non-human primates as fast as 100 ms after stimuli presentation (Perrett et al., 1982; Oram and Perrett, 1992; Liu et al., 2009). The nature of connections among neurons from the retina to the inferotemporal cortex (Felleman and Van Essen, 1991) and the spikes needed for the information to step through all these areas suggest that fast categorization is performed in a single feed-forward pass of information (Thorpe et al., 1996; VanRullen and Thorpe, 2001a,b).
Our results give support to this view as they show a first early categorization of meaningful pictures starting at 90–120 ms. Consistent with studies showing that a visual scene can be rapidly detected (Thorpe et al., 1996; Fei-Fei et al., 2002), the presentation of a meaningful picture produced a rapid widespread activation throughout the cortex as compared to the meaningless scrambled pictures. In our data identifiable targets of animals or tools generated an early event-related component as early as 100 ms, suggesting that initial visual categorization might originate from the first pass of processing in visual cortex. These early processes could not be attributed in our data to spatial frequency or mean luminance differences between targets and scrambled controls.
The absence of a difference in ERPs between seen animals and tools along with the low single trial classification performance suggests that finer semantic categorization takes place at a later stage of the processing hierarchy. This idea is in accordance with the latency of the N400 ERP component, usually in the time window of 200–500 ms after stimuli onset, associated with semantic processing as reported in previous studies (Kutas and Hillyard, 1980; Dehaene, 1995; Pulvermuller et al., 1996; Kiefer, 2001). Recent studies employing single trial analysis have shown better classification performances at discriminating between seen animal and tool categories (Murphy et al., 2009, 2010; Simanova et al., 2010; Chan et al., 2011) and animals versus vehicles (VanRullen and Thorpe, 2001b). Our results are slightly different from these previous studies as our classifier only found suggestive evidence of a discrimination between these two categories but a classification performance at the limit of chance level. We can only speculate that these differences might have occurred due to the additive noise effect of the low luminance Mondrians accompanying the targets, to the lower number of trials or to possible biases in the feature selection and classification process.
Some theories state that visual awareness is linked to late stages of processing in the ventral stream (Milner and Goodale, 1995; Koch, 1996; Bar and Biederman, 1999). If this is the case, is there a preliminary categorization process for unseen stimuli? Backward masking studies have shown that for unseen stimuli the second stage of processing of information around 250 ms is eliminated while the first pass of information survives suppression (Schiller and Chorover, 1966; Dehaene et al., 2001; Bacon-Macé et al., 2005; Melloni et al., 2007).
Models of binocular suppression propose that neural competitive interactions occur at several levels of the visual processing hierarchy (Tong, 2001; Blake and Logothetis, 2002; Tong et al., 2006). The idea that interocular suppression starts very early in visual processing (Tong and Engel, 2001; Haynes et al., 2005; Wunderlich et al., 2005) and that there exists an almost complete suppression of the information conveyed by the non-dominant stimuli in ventral areas of the visual cortex is supported by psychophysics (Moradi et al., 2005; Alais and Melcher, 2007), fMRI (Tong et al., 1998; Pasley et al., 2004; Hesselmann and Malach, 2011), single-cell recordings in monkeys (Sheinberg and Logothetis, 1997), and single-cell recordings in human beings (Kreiman et al., 2002, 2005).
On the other side, recent experiments have shown that during interocular competition complex suppressed stimuli can nonetheless generate behavioral effects, suggesting invisible processing beyond striate cortex (Kovács et al., 1996; Andrews and Blakemore, 1999; Alais and Parker, 2006; Jiang et al., 2006; Stein et al., 2011). Also, it has been proposed that weak category-specific neural activity could be detected during CFS using MEG/EEG (Jiang et al., 2009; Sterzer et al., 2009) and multivariate analysis of fMRI data (Sterzer et al., 2008).
Our results suggest that CFS suppresses information even for the first pass and that unseen animal and tool categories are suppressed early in visual cortex. Previous reports on CFS have found residual processing of information in the dorsal stream for unseen tool pictures but not for unseen animal pictures (Fang and He, 2005; Almeida et al., 2008). In these studies the results were explained in terms of the “graspability” nature of tool pictures and by the difference in interocular suppression for the ventral and the dorsal stream (Almeida et al., 2010). We were not able to corroborate this hypothesis as we did not observe any evidence of cortical activity in the parietal channels associated with the perception of manipulable objects, a result in agreement with a recent study by Hesselmann and Malach (2011).
An important reason for the discrepancies between previous studies and our results might be related to differences in depth of suppression (Tsuchiya et al., 2006; Alais and Melcher, 2007). In the studies by Fang and He (2005) and Almeida et al. (2008) the authors presented the target stimuli on the screen for 200 ms. Target pictures were made invisible by presenting two flashes of Mondrians in each trial, the minimal set of Mondrian flashes for a paradigm to be considered CFS. This fact might have bring their paradigms closer to backward masking than to classical CFS (Tsuchiya and Koch, 2005) in terms of depth of suppression (Tsuchiya et al., 2006). In our study, however, we presented a continuum of flashes before and after picture targets appeared on the screen, which rendered suppression stronger (but see Jiang et al. (2006) for a design with several Mondrian presentation). Under these conditions both unseen animal and tool targets were equally suppressed. Alternatively, it could also be argued that the our results arise from the differences in nature and temporal dynamics of EEG and fMRI recordings, that the small changes in BOLD signal generated by unseen tools in the parietal cortex that were reported by Fang and He (2005) might not have any correlate in any ERP component and would therefore go undetected.
Conclusion
Our results suggest that rapid categorization is suppressed under CFS. The fact that these processes can be canceled by deep binocular suppression suggests a competition even within the first pass of visual recognition. Under some conditions, with only minimal competition, some weak categorization might occur, but our results question the robustness of these unconscious processes to strong suppression. Thus, even target detection is not completely automatic, but can be intercepted by competitive interactions in early vision.
Conflict of Interest Statement
The authors declare that the research was conducted in the absence of any commercial or financial relationships that could be construed as a potential conflict of interest.
Acknowledgments
We are very grateful to Mariano Sigman for allowing us to access the EEG facilities at the Laboratory of Integrative Neuroscience of the University of Buenos Aires. We are also thankful to Marco Buiatti who helped us to automatize the process of EEG artifact removal and to Guido Hesselmann for his comments on an early version of the manuscript. This work has been realized also thanks to the support from the Provincia Autonoma di Trento and the Fondazione Cassa di Risparmio di Trento e Rovereto.
Footnotes
References
Alais, D., and Melcher, D. (2007). Strength and coherence of binocular rivalry depends on shared stimulus complexity. Vision Res. 47, 269–279.
Alais, D., and Parker, A. (2006). Independent binocular rivalry processes for motion and form. Neuron 52, 911–920.
Almeida, J., Mahon, B. Z., and Caramazza, A. (2010). The role of the dorsal visual processing stream in tool identification. Psychol. Sci. 21, 772–778.
Almeida, J., Mahon, B. Z., Nakayama, K., and Caramazza, A. (2008). Unconscious processing dissociates along categorical lines. Proc. Natl. Acad. Sci. U.S.A. 105, 15214–15218.
Andrews, T. J., and Blakemore, C. (1999). Form and motion have independent access to consciousness. Nat. Neurosci. 2, 405–406.
Bacon-Macé, N., Macé, M. J. M., Fabre-Thorpe, M., and Thorpe, S. J. (2005). The time course of visual processing: backward masking and natural scene categorisation. Vision Res. 45, 1459–1469.
Bar, M., and Biederman, I. (1999). Localizing the cortical region mediating visual awareness of object identity. Proc. Natl. Acad. Sci. U.S.A. 96, 1790–1793.
Chan, A. M., Halgren, E., Marinkovic, K., and Cash, S. S. (2011). Decoding word and category-specific spatiotemporal representations from MEG and EEG. Neuroimage 54, 3028–3039.
Dehaene, S. (1995). Electrophysiological evidence for category-specific word processing in the normal human brain. Neuroreport 6, 2153–2157.
Dehaene, S., Naccache, L., Cohen, L., Bihan, D. L., Mangin, J.-F., Poline, J.-B., and Riviere, D. (2001). Cerebral mechanisms of word masking and unconscious repetition priming. Nat. Neurosci. 4, 752–758.
Delorme, A., and Makeig, S. (2004). EEGLAB: an open source toolbox for analysis of single-trial EEG dynamics including independent component analysis. J. Neurosci. Methods 134, 9–21.
Fabre-Thorpe, M., Delorme, A., Marlot, C., and Thorpe, S. (2001). A limit to the speed of processing in ultra-rapid visual categorization of novel natural scenes. J. Cogn. Neurosci. 13, 171–180.
Fang, F., and He, S. (2005). Cortical responses to invisible objects in the human dorsal and ventral pathways. Nat. Neurosci. 8, 1380–1385.
Fei-Fei, L., Vanrullen, R., Koch, C., and Perona, P. (2002). Rapid natural scene categorization in the near absence of attention. Proc. Natl. Acad. Sci. U.S.A. 99, 9596–9601.
Felleman, D. J., and Van Essen, D. C. (1991). Distributed hierarchical processing in the primate cerebral cortex. Cereb. Cortex 1, 1–47.
Hanke, M., Halchenko, Y. O., Sederberg, P. B., Olivetti, E., Fründ, I., Rieger, J. W., Herrmann, C. S., Haxby, J. V., Hanson, S. J., and Pollmann, S. (2009). PyMVPA: a unifying approach to the analysis of neuroscientific data. Front. Neuroinform. 3:3. doi: 10.3389/neuro.11.003.2009
Haynes, J.-D., Deichmann, R., and Rees, G. (2005). Eye-specific effects of binocular rivalry in the human lateral geniculate nucleus. Nature 438, 496–499.
Hesselmann, G., and Malach, R. (2011). The link between fMRI-BOLD activation and perceptual awareness is â stream-invariantâ in the human visual system. Cereb. Cortex.. doi: 10.1093/cercor/bhr085. [Epub ahead of print].
Hung, C. P., Kreiman, G., Poggio, T., and DiCarlo, J. J. (2005). Fast readout of object identity from macaque inferior temporal cortex. Science 310, 863–866.
Jiang, Y., Costello, P., Fang, F., Huang, M., and He, S. (2006). A gender- and sexual orientation-dependent spatial attentional effect of invisible images. Proc. Natl. Acad. Sci. U.S.A. 103, 17048–17052.
Jiang, Y., Shannon, R. W., Vizueta, N., Bernat, E. M., Patrick, C. J., and He, S. (2009). Dynamics of processing invisible faces in the brain: automatic neural encoding of facial expression information. Neuroimage 44, 1171–1177.
Kiefer, M. (2001). Perceptual and semantic sources of category-specific effects: event-related potentials during picture and word categorization. Mem. Cognit. 29, 100–116.
Koch, C. (1996). Towards the neuronal correlate of visual awareness. Curr. Opin. Neurobiol. 6, 158–164.
Koch, C. (2003). The Quest for Consciousness: A Neurobiological Approach. Englewood, CO: Roberts and Company Publishers.
Kovács, I., Papathomas, T. V., Yang, M., and Fehér, A. (1996). When the brain changes its mind: interocular grouping during binocular rivalry. Proc. Natl. Acad. Sci. U.S.A. 93, 15508–15511.
Kreiman, G., Fried, I., and Koch, C. (2002). Single-neuron correlates of subjective vision in the human medial temporal lobe. Proc. Natl. Acad. Sci. U.S.A. 99, 8378–8383.
Kreiman, G., Fried, I., and Koch, C. (2005). “Responses of single neurons in the human brain during flash suppression,” in Binocular Rivalry, eds D. Alais, and R. Blake (Cambridge, MA: MIT Press), 213–230.
Kutas, M., and Hillyard, S. A. (1980). Reading senseless sentences: brain potentials reflect semantic incongruity. Science 207, 203–205.
Lamme, V. (2000). The distinct modes of vision offered by feedforward and recurrent processing. Trends Neurosci. 23, 571–579.
Liu, H., Agam, Y., Madsen, J. R., and Kreiman, G. (2009). Timing, timing, timing: fast decoding of object information from intracranial field potentials in human visual cortex. Neuron 62, 281–290.
Logothetis, N. K. (1998). Single units and conscious vision. Philos. Trans. R. Soc. Lond. B Biol. Sci. 353, 1801–1818.
Melloni, L., Molina, C., Pena, M., Torres, D., Singer, W., and Rodriguez, E. (2007). Synchronization of neural activity across cortical areas correlates with conscious perception. J. Neurosci. 27, 2858–2865.
Milner, D., and Goodale, M. (1995). The Visual Brain in Action, 2nd Edn. Oxford: Oxford University Press.
Moradi, F., Koch, C., and Shimojo, S. (2005). Face adaptation depends on seeing the face. Neuron 45, 169–175.
Murphy, B., Baroni, M., and Poesio, M. (2009). “EEG responds to conceptual stimuli and corpus semantics,” in Proceedings of the 2009 Conference on Empirical Methods in Natural Language Processing: Volume 2 – Volume 2, EMNLP ’09 (Stroudsburg, PA: Association for Computational Linguistics), 619–627.
Murphy, B., Poesio, M., Bovolo, F., Bruzzone, L., Dalponte, M., and Lakany, H. (2010). EEG decoding of semantic category reveals distributed representations for single concepts. Brain Lang. 117, 12–22.
Olivetti, E., Mognon, A., Greiner, S., and Avesani, P. (2010). Brain decoding: biases in error estimation. First Workshop on Brain Decoding: Pattern Recognition Challenges in Neuroimaging, Istanbul, 40–43.
Oram, M. W., and Perrett, D. I. (1992). Time course of neural responses discriminating different views of the face and head. J. Neurophysiol. 68, 70–84.
Pasley, B. N., Mayes, L. C., and Schultz, R. T. (2004). Subcortical discrimination of unperceived objects during binocular rivalry. Neuron 42, 163–172.
Perrett, D. I., Rolls, E. T., and Caan, W. (1982). Visual neurones responsive to faces in the monkey temporal cortex. Exp. Brain Res. 47, 329–342.
Potter, M. C., and Faulconer, B. A. (1975). Time to understand pictures and words. Nature 253, 437–438.
Pulvermuller, F., Preissl, H., Lutzenberger, W., and Birbaumer, N. (1996). Brain rhythms of language: nouns versus verbs. Eur. J. Neurosci. 8, 937–941.
Rousselet, G. A., Macé, M. J., Thorpe, S. J., and Fabre-Thorpe, M. (2007). Limits of event-related potential differences in tracking object processing speed. J. Cogn. Neurosci. 19, 1241–1258.
Schiller, P. H., and Chorover, S. L. (1966). Metacontrast: its relation to evoked potentials. Science 153, 1398–1400.
Schlkopf, B., and Smola, A. J. (2001). Learning with Kernels: Support Vector Machines, Regularization, Optimization, and Beyond (Adaptive Computation and Machine Learning), 1st Edn. Cambridge, MA: The MIT Press.
Sergent, C., Baillet, S., and Dehaene, S. (2005). Timing of the brain events underlying access to consciousness during the attentional blink. Nat. Neurosci. 8, 1391–1400.
Sheinberg, D. L., and Logothetis, N. K. (1997). The role of temporal cortical areas in perceptual organization. Proc. Nat. Acad. Sci. U.S.A. 94, 3408–3413.
Simanova, I., van Gerven, M., Oostenveld, R., and Hagoort, P. (2010). Identifying object categories from event-related EEG: toward decoding of conceptual representations. PLoS ONE 5, e14465. doi: 10.1371/journal.pone.0014465
Simons, D. J., and Chabris, C. F. (1999). Gorillas in our midst: sustained inattentional blindness for dynamic events. Perception 28, 1059–1074.
Stein, T., Senju, A., Peelen, M. V., and Sterzer, P. (2011). Eye contact facilitates awareness of faces during interocular suppression. Cognition 119, 307–311.
Sterzer, P., Haynes, J.-D. D., and Rees, G. (2008). Fine-scale activity patterns in high-level visual areas encode the category of invisible objects. J. Vis. 8, 1–12.
Sterzer, P., Jalkanen, L., and Rees, G. (2009). Electromagnetic responses to invisible face stimuli during binocular suppression. Neuroimage 46, 803–808.
Thorpe, S., Fize, D., and Marlot, C. (1996). Speed of processing in the human visual system. Nature 381, 520–522.
Tong, F. (2001). Competing theories of binocular rivalry: a possible resolution. Brain Mind 2, 55–83.
Tong, F., and Engel, S. A. (2001). Interocular rivalry revealed in the human cortical blind-spot representation. Nature 411, 195–199.
Tong, F., Meng, M., and Blake, R. (2006). Neural bases of binocular rivalry. Trends Cogn. Sci. (Regul. Ed.) 10, 502–511.
Tong, F., Nakayama, K., Vaughan, J. T., and Kanwisher, N. (1998). Binocular rivalry and visual awareness in human extrastriate cortex. Neuron 21, 753–759.
Tsuchiya, N., and Koch, C. (2005). Continuous flash suppression reduces negative afterimages. Nat. Neurosci. 8, 1096–1101.
Tsuchiya, N., Koch, C., Gilroy, L. A., and Blake, R. (2006). Depth of interocular suppression associated with continuous flash suppression, flash suppression, and binocular rivalry. J. Vis. 6, 1068–1078.
VanRullen, R., and Thorpe, S. J. (2001a). Is it a bird? Is it a plane? Ultra-rapid visual categorisation of natural and artifactual objects. Perception 30, 655–668.
VanRullen, R., and Thorpe, S. J. (2001b). The time course of visual processing: from early perception to decision-making. J. Cogn. Neurosci. 13, 454–461.
Wunderlich, K., Schneider, K. A., and Kastner, S. (2005). Neural correlates of binocular rivalry in the human lateral geniculate nucleus. Nat. Neurosci. 8, 1595–1602.
Keywords: visual awareness, rapid categorization, continuous flash suppression, EEG
Citation: Kaunitz LN, Kamienkowski JE, Olivetti E Murphy B Avesani P and Melcher DP (2011) Intercepting the first pass: rapid categorization is suppressed for unseen stimuli. Front. Psychology 2:198. doi: 10.3389/fpsyg.2011.00198
Received: 27 February 2011; Accepted: 04 August 2011;
Published online: 23 August 2011.
Edited by:
Rufin VanRullen, Centre de Recherche Cerveau et Cognition, FranceReviewed by:
Thomas Carlson, University of Maryland, USAThomas Serre, Brown University, USA
Naotsugu Tsuchiya, RIKEN, Japan
Copyright: © 2011 Kaunitz, Kamienkowski, Olivetti, Murphy, Avesani and Melcher. This is an open-access article subject to a non-exclusive license between the authors and Frontiers Media SA, which permits use, distribution and reproduction in other forums, provided the original authors and source are credited and other Frontiers conditions are complied with.
*Correspondence: Lisandro Nicolas Kaunitz, Center for Mind/Brain Sciences (CIMeC), University of Trento, Via delle Regole 101, 38060 Mattarello (TN), Italy. e-mail:bGthdW5pdHpAZ21haWwuY29t