- 1 Center for Mind/Brain Sciences, University of Trento, Trento, Italy
- 2 Department of Cognitive Sciences, University of Trento, Trento, Italy
Many studies suggest a large capacity memory for briefly presented pictures of whole scenes. At the same time, visual working memory (WM) of scene elements is limited to only a few items. We examined the role of retroactive interference in limiting memory for visual details. Participants viewed a scene for 5 s and then, after a short delay containing either a blank screen or 10 distracter scenes, answered questions about the location, color, and identity of objects in the scene. We found that the influence of the distracters depended on whether they were from a similar semantic domain, such as “kitchen” or “airport.” Increasing the number of similar scenes reduced, and eventually eliminated, memory for scene details. Although scene memory was firmly established over the initial study period, this memory was fragile and susceptible to interference. This may help to explain the discrepancy in the literature between studies showing limited visual WM and those showing a large capacity memory for scenes.
Introduction
Real-world scenes tend to include a large number of different objects that are arranged in a variety of different configurations. A street scene, for example, would likely contain buildings, people, cars, and signposts, while an office might contain a desk, computer, telephone, bookshelves, and small objects such as cups, papers, or pens. A fundamental challenge for cognition is to reconcile the complexity of real-world environments, and our rich experience of the scene, with an extremely limited attention and working memory (WM) span (Melcher, 2001; Tatler, 2001). At any given point in time, we are attending to one, or at most a few, items in the scene. Is the information which is out of sight also out of mind?
One possibility would be that memory for the scene is quickly encoded into a relatively stable long-term memory (LTM) representation. Traditional models of memory posit that there is a long-term store that is effectively unlimited in capacity and duration. It has long been known that people are able to memorize a large set of photographs of visual scenes (Shepard, 1967; Standing, 1973; Vogt and Magnussen, 2007). Similarly, when subjects are asked to memorize a large set of pictures of objects (Brady et al., 2008), recognition memory remains high even after hundreds of intervening pictures. In contrast, visual short-term memory is limited to a period of seconds and its capacity is limited to at most a handful of attended objects. This short-term memory may be particularly useful in keeping in mind exact object details (Magnussen, 2000). The information gleaned in a single glance is limited and so the withdrawal of attention away from objects (as a result of a shift in attention and gaze) means that information must either be encoded in a more permanent store or else it will be forgotten. For example, it was shown that memory for identity of items in a nine object display dropped off rapidly after one or two fixations to subsequent items (Zelinsky and Loschky, 2005). Thus, there are conflicting reports regarding whether scene memory is relatively stable and long-lasting or fragile and of brief duration.
One complication in trying to characterize the representation underlying scene memory is that a scene can be viewed both as a collection of objects and as a unique entity whose “gist” can be quickly recognized and used to guide the processing of objects (Bar, 2004; Oliva and Torralba, 2007). For example, a picture of a beach can be discriminated from that of a street scene based on statistical differences in low-level visual properties. Since it is possible to recognize a scene without encoding the details of the location or visual features of specific objects, this raises the question of the roles of scene gist (statistical representations), and object recognition in perceiving and remembering scenes. It is possible, for instance, to induce participants to recall seeing a particular object in a scene, even when that item had not actually been present (Miller and Gazzaniga, 1998), suggesting that gist information can dominate object details in memory.
We tend to stay in the same scene for seconds, or even minutes at a time and even return often to the same location (see Tatler and Land, 2011 for a review on differences between pictures and real scenes). Thus, at any given point in time, performance might reflect a combination of LTM and WM. In other words, the on-line WM that is available while we interact within real scenes may be a combination of bottom-up information gained through individual fixations (traditionally thought of as visual short-term memory) and also representations recalled out of a long-term store (Melcher, 2001, 2006; Hollingworth, 2004). Together, this suggests that there are two different mechanisms involved in scene perception – gist and object perception – and two different memory types. We wanted to test the roles of the relatively “superficial” gist analysis and the detailed analysis of object details in scene memory, and to see which of these items were involved in a scene WM task over a period of seconds.
Previous studies of memory for pictures of complex scenes have shown that the ability to recall or recognize the details of objects in the scenes increases as a function of total looking time (Melcher, 2001, 2006; Tatler et al., 2003; Hollingworth, 2004; Tatler and Melcher, 2007; Pertzov et al., 2009). Upon first entering a new scene, there would be only a limited amount of information in working memory, but this information would quickly build up over time and across glances. Memory for object details seems to accumulate approximately linearly over time (Melcher, 2001, 2006; Tatler et al., 2003; Tatler and Melcher, 2007; Pertzov et al., 2009). Moreover, memory for details in a scene was not hampered by introducing a 1 min delay period between memorization and test during which time participants were occupied with a reading or another VWM task (Melcher, 2006). Scene memory remained above chance even 1 week later, as measured by an improvement in performance for scenes viewed briefly in a previous session (Melcher, 2010). Similarly, change detection for the replacement or rotation of an object in a picture has been shown to improve over time, probably because the participant had more time to fixate the various objects in the scene (Hollingworth, 2004, 2005). Even 24 h later, participants were able to detect these changes at above chance level, although performance was considerably worse than immediately after having viewed the initial scene (Hollingworth, 2005).
One important feature of most laboratory studies of visual short-term memory is that they typically use many different displays (memory sets that must be remembered) in a relatively short period of time. One of the most typical measures of visual memory uses a limited number of colored shapes re-arranged in different locations across trials, and studies using naturalistic stimuli have often used a limited set of objects and potential locations (Triesch et al., 2003; Zelinsky and Loschky, 2005). In real-life scenes, multiple fixations could be integrated over time into a coherent scene representation. In contrast, in experiments with colored squares any LTM would likely cause interference on subsequent trials. An interesting study, in this regard, examined LTM for photographs of doors (Vogt and Magnussen, 2007). When extra cues were included, such as signs, lamp posts, or plants, recognition memory was good. Removing these details, however, dramatically reduced performance. This suggests that increasing the similarity between different memory stimuli can dramatically reduce memory performance. Similarly, Konkle et al. (2010a) reported that large capacity memory for object exemplars decreased when there were a larger number of objects from the same category in the memory set. However, the effect of doubling the number of exemplars within the same category was relatively small (Konkle et al., 2010a,b).
The goal of this experiment was to examine why we forget the details of visual scenes. Previous reports have shown a decrease in performance over time, but the reason for this decrease has not been explained. To this end, we investigated the role of retroactive interference in visual scene memory. As stated above, many of the studies showing limits in visual memory repeated similar stimuli across trials. We studied interference effects using a simple scene memory task in which participants viewed a scene for 5 s and then, about 10 s later, were tested on their memory for the visual details. Based on similar studies, we would expect good performance in answering detailed questions about the items in the scene as well as in recognizing objects from the memorized scene (Tatler and Melcher, 2007). The novel aspect of this study was the introduction of a set of 10 distracter images on some trials. Participants had to pay attention to the distracters because they knew that there would be an old–new recognition test for the distracter items at the end of the session. The distracters were chosen so that they either belonged to the same topical category (such as “kitchen,” “bedroom,” “city street,” or “beach scene”) or to completely different categories. Given that semantics in one of the main organizing principles of LTM (Warrington and Shallice, 1984; Caramazza, 1998; Gabrieli et al., 1998; Hutchison, 2003; Baroni et al., 2010), our hypothesis was that the influence of the distracters would depend on the number of semantically related items.
Materials and Methods
Participants
A group of 40 participants took part in the experiment for course credit. Informed consent was obtained from all participants, who were also fully debriefed after their participation. There were a total of 25 female participants and 15 male participants (mean age = 26).
Stimuli
There were 40 target pictures of natural or human-made scenes, which had been normed for difficulty and for the effects of guessing (e.g., general knowledge, without having remembered the scene, would not be sufficient for answering the questions at about chance level) in previous studies (Melcher, 2006, 2010; Tatler and Melcher, 2007). The pictures contained an average of 11.4 unique objects. Examples of the scene categories include: an airport tarmac with planes; a bedroom; English tea and sandwiches; a desk with computer, papers, and objects; English breakfast; a sunroom with furniture and plants; a kitchen; a living room with couch; children playing with a dog; a playroom with billiard table; bathroom; a toy train set.
The distracter items were photographs chosen among copyright-free images available on the World Wide Web. There were two types of distracter images: similar and dissimilar category images. Similar images were pre-selected by the experimenters based on satisfying the same description as the target item (e.g., kitchen or playground), while the dissimilar items showed a completely different subject matter (see Figure 1). The similar pictures were not identical visually, but were chosen to differ based on overall range of colors, object locations, and object identities. At the same time, similar distracter images were more likely to contain the same types of objects as the target image, when compared to the distracter images. However, for any given question type, the number of distracter images which shared that object, in the same versus different condition, varied across trials. This allowed for some ability to separately measure the influences of the similar scene topic (e.g., two images of a kitchen) versus shared objects (e.g., the two images both contained a bowl of fruit).
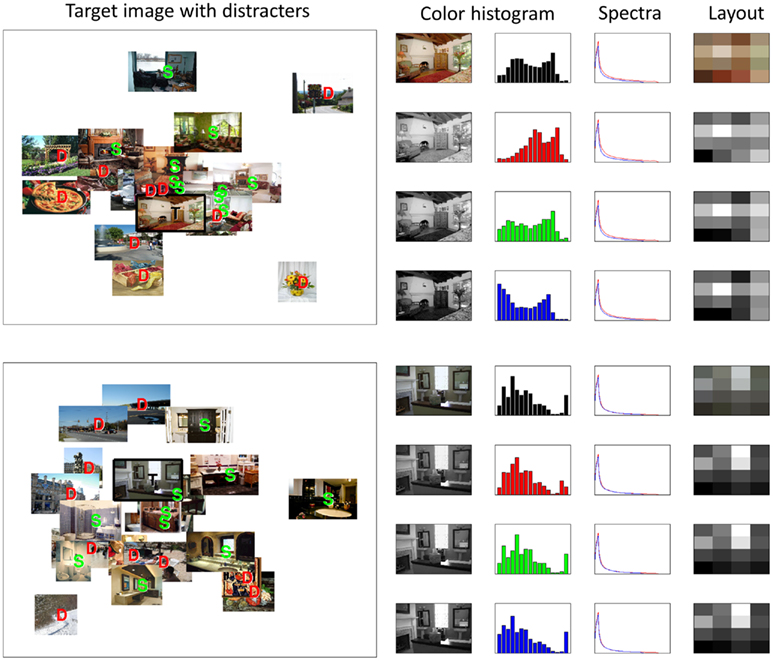
Figure 1. Multidimensional scaling plots showing two examples of target images (outlined in black rectangle and marked with “T”), similar distracters (marked with “S”), and different semantic category distracters (labeled with “D”). Distances represent similarity in terms of low-level visual features (see Materials and Methods). The rightmost columns show the color channels, color histograms, spectral frequency, and layout of the representative target images.
In addition to the target and distracter images, the other stimuli shown in the main experiment (see Figure 2) were the two types of test question displays: questions on image content, and a pictorial recognition test (Melcher, 2006; Tatler and Melcher, 2007). The questions regarded the location, color, and identity of objects in the picture and there were three alternative answers from which to choose for each question. An example of a location question is: “Where is the tea cup? (1) bottom right (2) bottom left (3) center.” An example of a color question is: “What color are the towels on the left of the picture? (1) cream (2) navy blue (3) yellow.” An example of an identity question is: “What is sitting on top of the computer monitor? (1) photo of a dog (2) computer cables (3) wooden bowl.”
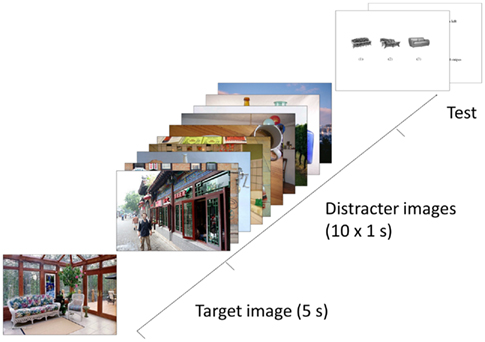
Figure 2. Illustration of the procedure used in the experiment. The target image was presented for 5 s, followed by 10 distracter images (or a blank screen) for a period of 10 s. Then participants answered a series of multiple choice (three alternative) questions about the identity, location, and color objects in the scene.
The recognition test contained three pictures of similar objects: one object taken directly from that image and two similar objects (see Figure 2, showing three similar sofa/couches). Neither of the two foil objects was taken from the distracter images used in this study, but were instead taken from pictures that were not used in this study. Correct response for the object picture recognition test required detailed visual information about the objects shown in the target image. The target, distracter, and question stimuli were resized to have the same maximum height or width (22.7° of visual angle) when shown on the screen. Each picture was displayed at the center of the display (21″ monitor) against a clear white background and viewed from a distance of about 65 cm.
Evaluating Topical and Visual Relatedness
The topical similarity of target–distractor pairs was then normed on a seven-point likert scale by a group of anonymous raters using Amazon’s Mechanical Turk (www.mturk.com). Mechanical Turk is widely used for norming and annotation tasks, and has been demonstrated to give data that in aggregate is of similar quality to that collected in more controlled settings. Its economy and effectiveness has been demonstrated for a range of semantic tagging tasks (Kittur et al., 2008; Snow et al., 2008; Sorokin and Forsyth, 2008). The on-line task was entitled “How similar are these two images?” Pairs of images were shown along with the instructions: “Rate to what extent these images are about the same thing or topic. You should consider only the subject matter, not simple features like color and brightness.” Similarity was judged on a seven-point likert scale from “identical” to “different.” Each pair of images was evaluated by three participants, with a total of 67 different participants across the entire study. For each target image an aggregate score of relatedness was computed for its “similar category” distracters, for its “dissimilar category” distracters (each a mean of 10 distracters × 3 judgments), and for its “mixed category” distracters (the mean of similar and dissimilar aggregate figures). The results corresponded very closely to the categories assigned by the experimenters. The nominal category of distracter sets (coded as 1 for similar, 0.5 for mixed, and 0 for dissimilar) and the normed judgments of distracter similarity were found to be almost perfectly correlated, with r = 0.993.
The low-level visual properties of each target and distracter image were also quantified, so that any interference effects in terms of visual similarity versus topical similarity could be interpreted. Such measures have been shown to correlate with elicited judgments of visual similarity (Rorissa et al., 2008) and are used for finding related pictures in image retrieval systems (Deselaers et al., 2008). Four low-level image features that correspond to perceptual properties were considered: color histograms, image layout, and vertical and horizontal spectral amplitudes. These four features together capture the type of low-level information that computer vision models and computational models of early visual processing suggest are involved in low-level vision (Lee et al., 2000). The color brightness histograms split the image into four channels (gray, red, green, blue) and counted the frequencies in 16 equally spaced bins. This measured reflected the global distribution of shades and tones in each image. The measure of image layout was calculated by resampling the image to 4 × 4 pixels and then measuring average brightness for each pixel on each channel, giving a measure of the gross configuration of the image (e.g., blue sky at top, green field at base). The horizontal and vertical spectral amplitudes capture form and texture in an image, and were also calculated separately for each channel using a Fast Fourier Transform (using a zero-padded 1024 point FFT, smoothed with a 20-point moving average, unit normalized). The perceptual similarity of target–distracter pairs was computed by taking the mean of the z-score normalized Euclidean distances on each of these four measures.
To confirm that these four dimensions capture salient but distinct attributes of images, we calculated their mutual correlation over 120 conditions (40 targets × 3 distracter categories: similar, dissimilar, mixed). While there was a moderate correlation between the horizontal/vertical spectral amplitude measures (r = 0.38), other pairwise correlations were low (r < 0.2), indicating that these measures represent independent low-level properties of the images. To further validate the perceptual grounding of the measures, we noted that for 33 for the 40 target images, their “similar category” distracters were nearer in the space of low-level attributes than their “dissimilar category ones.” The MDS plots in Figure 1 also illustrates this point, with visually similar images tending to cluster closely to the target, relative to dissimilar images. Of course since the target images are natural scenes, they varied greatly in their general characteristics and complexity. The images were not controlled in terms of overall number of objects, amount of texture, complexity of forms, distribution of colors, etc. Since these variables were be expected to affect the general perceptual processing, encoding, and retrieval load, our goal in these analyses was not to explain all of the variation in performance. Rather we aimed to see what correspondences between target and distractors had an effect on memory performance.
In addition, we also included a list of relevant image characteristics in the regression analyses. These included the total number of objects in the target scene and the fine and coarse scale visual detail of the scene. The visual complexity of the target image was estimated by the size of the file after a jpeg compression of the stimulus at two different image scales (90% quality setting of image dimensions of 60 or 480 pixels square). We used two different image sizes for this analysis in order to capture both coarse and fine scale complexity of the image (Forsythe et al., 2008). We expected that participants might be worse overall at responding to questions about scenes which were highly complex visually (lots of clutter and details) and contained a large number of objects, since this would decrease the likelihood that they would have encoded information about the specific objects referred to in the questions. In addition, we also calculated the similarity of the target image to other images across the entire stimulus set (not just the distracters shown on that particular image). This “target uniqueness” measure counted the number of images in the stimulus set that matched the same topic. For example, an image of a tent outdoors had a value of zero, since there were not similar target images in the experiment, while the scenes showing a bathroom had a value of four since there were several target scenes which included elements from a bathroom somewhere in the image.
Procedure
The first display showed a fixation cross at the center of the screen. Each trial began when the participant pressed a button on the keyboard. First, the target image was shown for 5 s (Figure 2). Then either a fixation cross (in the no distracter condition) or 10 distracter images (1 s per image) was presented for a total of 10 s. On trials with 10 distracters, the number of similar distracters was varied (0, 5, or 10 similar distracters). Then the memory test questions and the recognition test were displayed as text (and pictures in the case of the recognition test) on the screen. For each test item, three multiple choice answers were below the question, numbered from 1 to 3. Participants answered at their own pace by pressing “1,” “2,” or “3” on the keyboard. The experiment was run using SUPERLAB version 2.0 software. The pairing of a particular image to a particular condition was counterbalanced across participants. Across the participants, each image appeared an equal number of trials in each condition. The order of the test questions and recognition test was randomized across trials.
After completing all 40 trials, participants were given a sheet of paper containing 20 images, half of which were new, and half had been presented as distracters during the experiment. Participant’s performance on this final control task was not used for any statistical analysis, but rather to ensure that they paid attention to the distracter images during the experiment. In total, the experiment took about 40 min to complete.
Analyses
The main effects of distracter type (no distracters, 10 similar, 10 dissimilar, mix of 5 similar and 5 dissimilar) and question type (color, location, identity, and object recognition) on percentage correct were analyzed using a repeated measures, within-subject ANOVA. In addition, the main effect of distracter was tested separately for the four different question types with repeated measures ANOVA tests.
Results
In the no distracter condition, participants were good at answering the object detail questions and in recognizing the correct object from the display (Figure 3). However, the presence of distracters led to decreased performance. This reduction in performance depended on the number of similar distracters within the set of 10 distracter images [main effect of distracter type: F(3,37) = 55.91, p < 0.001]. The full group of 10 similar distracters effectively eliminated detailed memory for the items in the target photograph, with performance near chance (37.8% correct, with chance level of 33.3%). The distracter set containing an equal mix of similar and dissimilar distracters caused an intermediate amount of interference, consistent with our hypothesis that the degree of interference would depend on the number of similar distracters.
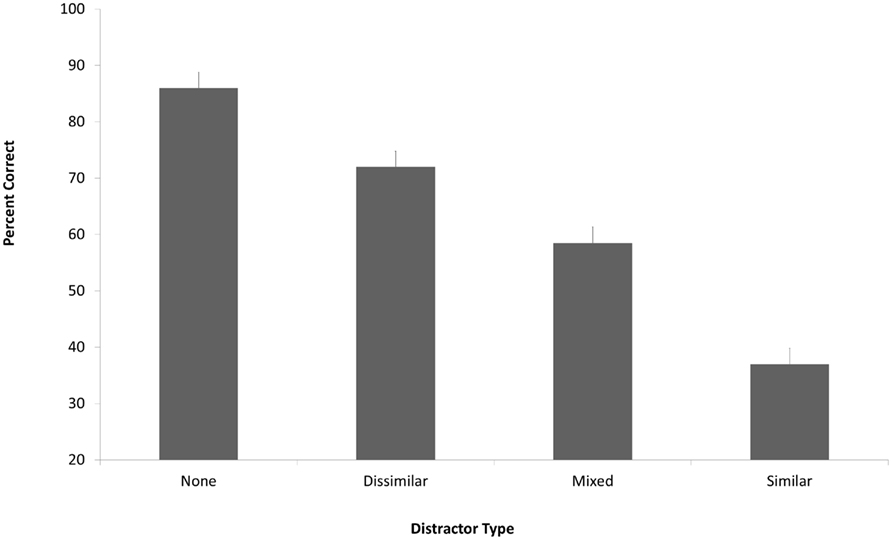
Figure 3. Percent correct in the memory test, with performance shown separately for the different distracter conditions. The number of distracters shown during the 10-s delay between test image and memory test was either zero (leftmost bar) or 10 (other bars). The distracters were made up of 10 topically dissimilar images, 10 topically similar images, or an equal mixture of the two (5 similar and 5 dissimilar). Error bars show 1 SE.
This overall pattern of results was consistent across each of the four memory measures tested (Figure 4). There was a main effect of question type [F(3,37) = 29.45, p < 0.001], indicating that the question types were not equated for difficulty. The interaction between question type and distracter types was significant [F(9,31) = 3.44, p = 0.005]. Despite this interaction, the distracters influenced all of the measures of memory as shown by a significant main effect of distracter type on color questions [F(3,37) = 32.21, p < 0.001], identity questions [F(3,37) = 29.18, p < 0.001], location questions [F(3,37) = 56.83, p < 0.001], and object recognition [F(3,37) = 63.45, p < 0.001]. Thus, the interference from distracters was not limited to a single type of question, but rather influenced a range of the aspects of the visual object representation.
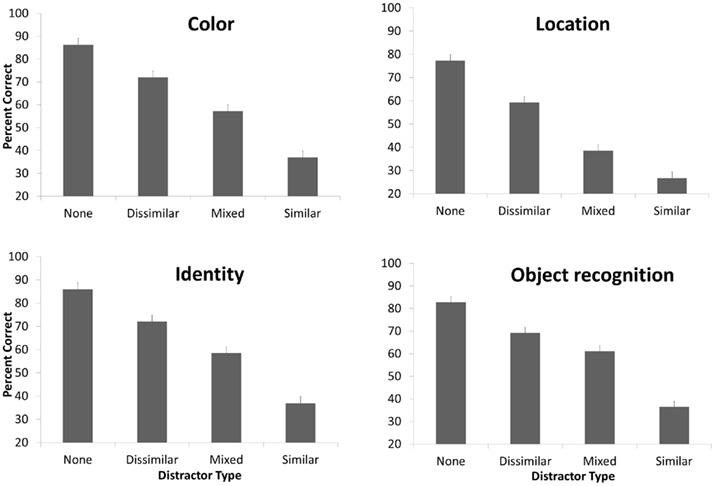
Figure 4. Memory performance for each of the four question types, for each of the four experimental conditions. See the caption of Figure 3 for details of the conditions. Error bars show 1 SE.
As shown in Figures 3 and 4, the effect of the distracters on performance appears to be driven by topical similarity to the target image. The materials were chosen with semantic similarity of the topic in mind, meaning that the images either matched, or did not match, the same topic or subject matter. However semantic topic is likely to be confounded, at least to some extent, with low-level visual properties of the image and with the presence of the same characteristic objects. For example, a “beach” scene will likely have a large upper region colored blue (of sea and sky), and a lower region in gray or beige tones, and is likely to contain objects such as palm trees or beach umbrellas. To determine which aspect of similarity (topical, visual, or similar objects) was responsible for the behavioral effects, we used linear regression models to see which individual explanatory variables or combination thereof could provide the best fit of the data. Topical similarity was described using the norms of topical relatedness between target and distracter pairs, elicited from multiple informants over the internet (see Materials and Methods). Visual similarity was quantified using the four low-level image features described in the Section “Materials and Methods”: distribution of colors (color layer histograms of intensity), visual texture and form (spectral amplitudes in vertical and horizontal orientations of color layers), and image layout as captured by a low-resolution 4 × 4 pixel thumbnail of image layers. Object similarity (number of shared objects) was measured by counting the number of distracter images (same or different) which also contained the object which was the subject of one of the probe questions. For example, if the test question asked about the color of the boat, and 5 of the 10 similar distracter images contained a boat, then the object similarity score for similar distracters for that scene was calculated to be five.
Aggregate relatedness measures were calculated between each target and its similar, dissimilar, and mixed distracter sets, from the mean of the individual target–distractor distances. All other things being equal, we expect that targets having larger differentials between their similar and dissimilar distracter sets would see a larger differential in performance between conditions. The question is whether topical, visual, or object similarity distance gives a better account of this differential.
Four regression models were run, one for each of the four behavioral tasks (color, identity, recognition, location). There were 120 cases in each regression model, representing 40 target images, by three distracter conditions (similar, dissimilar, mixed). The dependent variable was the mean memory performance over participants for that combination of task, target, and distracter category. The independent variables were the aggregate judgments of target–distracter relatedness (see Materials and Methods), the uniqueness of the topic of the image across the entire stimulus set (see Materials and Methods), the four low-level visual measures of similarity and the number of similar objects. All variables were scale normalized using z-scores so that the beta weights assigned to each variable would be comparable.
For the color, identity, and location questions, the only independent variable that had predictive power was semantic relatedness (Figure 5A), which was highly significant in all cases (p < 0.001). The semantic relatedness could be measured in terms of topical relatedness (e.g., “kitchen”) or number of shared objects since these two variables co-varied (r = 0.91). Of the low-level visual variables, color similarity (brightness histograms across the various color channels) was significant in the recognition task, and vertical spectral amplitude approached significance on the location task (p < 0.1). For the recognition questions, the similarity in topic between target and similar distracters was again the main factor, although the estimated amount of coarse visual complexity (see Materials and Methods) also contributed to the regression analysis. This finding is interesting, since this question required the most detailed visual information about the shape and textures of the objects which would have been difficult to encode at a more abstract semantic level.
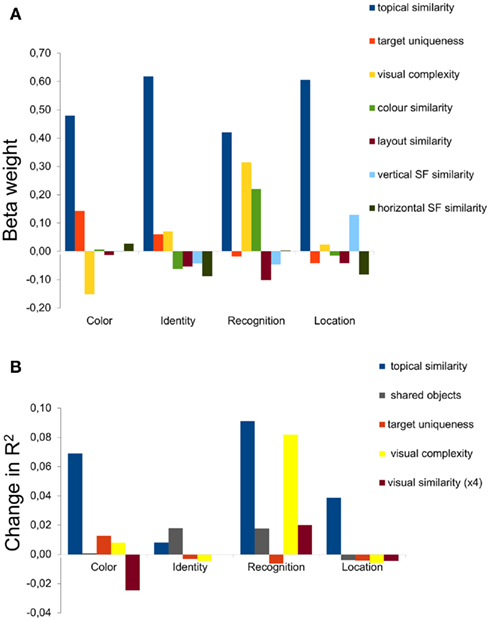
Figure 5. Explanatory power of semantic versus low-level visual properties in a regression model, for the four types of questions. (A) The top panel shows the beta weight, a measure of explanatory power, for the semantic properties and the low-level visual properties (color brightness histogram, brightness layout, vertical spectral amplitude, and horizontal spectral amplitude, from left to right). Number of similar objects is not included in this panel due to the strong correlation (r = 0.91) between topical similarity and number of shared objects. (B) The bottom row shows the change in the regression model from removing one of the factors, in order to show the relative contribution of semantic factors related to similarity between the target scene and the distracters (topic similarity and shared objects), target uniqueness of topic compared to other target images in the entire stimulus set, visual complexity of the target image and the low-level visual similarity between target and distracter images. The bars show the added advantage of including that variable in the regression model in terms of changes in adjusted R2.
To better understand the roles of each explanatory variable, in particular the relationship between target similarity and number of shared objects, we individually removed factors from the model to see which factors would have the largest effect (Figure 5B). This analysis confirmed the preeminence of semantic similarity, with topical similarity being most important for color, recognition, and location questions but the number of shared objects providing a better estimation of participants’ behavior on the identity question. As described above, visual complexity helped to predict performance in the recognition task. In all four cases, it is interesting to note that the low-level visual features contributed little or nothing.
Discussion
The main finding was that retroactive interference from the similar distracter images was able to reduce or even eliminate memory for the details of the objects in the scenes. This interference effect was based on the semantic similarity between the distracter and the target, rather than low-level visual similarity (see Konkle et al., 2010a,b for similar conclusions). Our findings fit well with recent studies of visual search in complex scenes, which have demonstrated that a preview of the background of the scene, even without any objects, can aid participants to quickly find a target objects in the scene (Vo and Schneider, 2010).
One of the fundamental mysteries of visual scene memory is how the pictorial-like information that subjects report (and seem to use in many visual tasks) can be reconciled with the semantic organization of concepts. For example, participants were good, in the baseline condition, at recognizing which object exemplar had been presented in the scene compared to semantically similar items. Similarly, the naïve intuition is that recalling the location of objects in the scene is based on spatial–temporal information, and many participants reported imagining the scene in order to answer the location questions. According to memory construction theories (Schacter, 1996; Schacter et al., 1998), however, the scene is not represented as a single, intact picture but instead as a collection of pieces of information which are used to construct an incomplete memory. This point is illustrated well by studies of change blindness, in which participants are not able to memorize and compare a metric, pixel-based representation in memory to what they see on the screen. Our results provide strong evidence that the semantic coding of the visual scene in memory, based on its topic and the identity of the objects in the scene, plays a role in organizing the memory of the scene. In other words, even pictorial memory is based on a semantic, rather than an exclusively visual, representation. It may be the case that our ability to recognize large numbers of scenes as same/different is more strongly influenced by visual similarity and statistical representations (such as gist), while our limited memory for object details is tied to the semantic organization of the scene memory.
The current results fit well with previous studies showing a rapid accumulation of memory for scene details over a period of seconds (Melcher, 2001, 2006; Tatler et al., 2003; Hollingworth, 2004; Tatler and Melcher, 2007; Pertzov et al., 2009). Performance in the color, location, and object identity questions was generally quite good, and remained high even after a blank delay. The addition of 10 dissimilar distracters caused only a moderate drop in performance, consistent with prior studies suggesting a relatively unlimited capacity for remembering scenes. Most investigations of LTM capacity have tested recognition of the entire stimulus, rather than the questions about object details used here. If, as suggested by those studies, the memory for the general gist and layout of the scenes is relatively long-lasting and unlimited in capacity, then this scene structure could be used to help remember the scene details (Melcher, 2006, 2010). In other words, the representation of the scene in LTM might work, metaphorically, like a coat rack on which to hang the details of the scene.
At the same time, memory for scene details was extremely susceptible to interference. This retroactive interference worked in a relatively straightforward and linear fashion: the more similar the distracters, the worse the performance in remembering the details of the target scene. However, even the semantically dissimilar distracters, which would have occupied VWM, decreased memory for scene details (consistent with the hypothesis than on-line visual memory combines working and LTM). Our results are consistent with previous reports showing some decrease in memory for scenes after a long delay, and suggest that at least part of this effect may come from interference from similar stimuli or even from interference from real-world scenes viewed between study and test (Hollingworth, 2005; Melcher, 2010). Many studies of WM for scenes have involved repeating the same set of objects and backgrounds (Hayhoe et al., 1998; Melcher, 2001; Triesch et al., 2003; Zelinsky and Loschky, 2005) or, in the case of change detection, involved comparing two identical scenes at the level of object details. Such an experimental design would create a great deal of interference within trials and/or blocks of trials, helping to explain why memory would seem to be so limited in such studies. The choice of using complex scenes and objects, rather than stimuli like colored circles, should already lead to an improvement in memory since more distinct items are more resistant to interference from similar items in other trials. However, the present results suggest that using natural objects and scenes is not sufficient to maximize scene memory, since even scenes from the same category cause greater interference than scenes from different categories. This interference would likely occur both at the level of scene gist, where the participant might fail to correctly bind together objects to a particular scene, and also at the level of individual objects (since scenes sharing similar semantics would likely also share the same categories of objects).
The effect of the semantically similar distracters was stronger than might have been expected based on recent studies by Konkle et al. (2010a,b), even if both studies agree on a central role for conceptual distinctiveness in LTM. They reported that doubling the number of distracters resulted in a 2% decrease in performance, whereas we found that doubling the number of similar distracters caused a more dramatic loss (around 20%). In their study, the effect of similar exemplars ranged from about 12% (4 similar exemplars) to 16% (16 similar exemplars) for scenes, and for isolated objects only 7% (4 similar exemplars) to 11% (16 similar exemplars). However, the paradigms used in our study and theirs were quite different. First, participants in their experiment had to learn nearly 3,000 scenes all at once, encouraging a different memorization strategy than in our experiment. Second, Konkle and colleagues used a two alternative forced choice test between one of the many memorized stimuli and a foil (which could be either similar or dissimilar). Participants in their study could have used any number of different strategies to answer these tasks, whereas our participants had to answer specific questions about particular details of the color, location, or identity of the items in the scene. Overall, our results confirm and extend the conclusions of the work of Konkle and colleagues, showing that under some conditions the role of conceptual similarity can be even greater than previously reported.
In conclusion, the current findings help to reconcile the seemingly incompatible claims of high-capacity scene memory and limited-capacity working memory. With longer viewing time, scene details can be accumulated into memory. At least initially, however, these scene details are only weakly linked to the scene representation and can be easily lost. The visual scene representation that survives interference depends on a semantic encoding of the scene, in terms of objects and categories, rather than a purely pictorial representation.
Conflict of Interest Statement
The authors declare that the research was conducted in the absence of any commercial or financial relationships that could be construed as a potential conflict of interest.
Acknowledgments
Special thanks to Laila Rashid for assistance in data collection. This work has been realized thanks to the support from the Autonomous Province of Trento, the Fondazione Cassa di Risparmio di Trento e Rovereto and the Italian Ministry of Universities and Research (MIUR – PRIN 2007).
References
Baroni, M., Murphy, B., Barbu, E., and Poesio, M. (2010). Strudel: a corpus-based semantic model based on properties and types. Cogn. Sci. 34, 222–254.
Brady, T. F., Konkle, T., Alvarez, G. A., and Oliva, A. (2008). Visual long-term memory has a massive storage capacity for object details. Proc. Natl. Acad. Sci. U.S.A. 105, 14325–14329.
Caramazza, A. (1998). The interpretation of semantic category-specific deficits: what do they reveal about the organization of conceptual knowledge in the brain? Neurocase, 4, 265–272.
Deselaers, T., Keysers, D., and Ney, H. (2008). Features for image retrieval: an experimental comparison. Inf. Retr. Boston 11, 77–107.
Forsythe, A., Mulhern, G., and Sawey, M. (2008). Confounds in pictorial sets: the role of complexity and familiarity in basic-level picture processing. Behav. Res. Methods 40, 116–129.
Gabrieli, J. D., Poldrack, R. A., and Desmond, J. E. (1998). The role of left prefrontal cortex in language and memory. Proc. Natl. Acad. Sci. U.S.A. 95, 906–913.
Hayhoe, M. M., Bensinger, D. G., and Ballard, D. H. (1998). Task constraints in visual working memory. Vision Res. 38, 125–137.
Hollingworth, A. (2004). Constructing visual representations of natural scenes: the roles of short- and long-term visual memory. J. Exp. Psychol. Hum. Percept. Perform. 30, 519–537.
Hollingworth, A. (2005). The relationship between online visual representation of a scene and long-term scene memory. J. Exp. Psychol. Learn. Mem. Cogn. 31, 396–411.
Hutchison, K. A. (2003). Is semantic priming due to association strength or feature overlap? A microanalytic review. Psychon. Bull. Rev. 10, 785–813.
Kittur, A., Chi, E., and Suh, B. (2008). “Crowdsourcing user studies with Mechanical Turk,” in CHI 2007: Proceedings of the ACM Conference on Human-factors in Computing Systems (New York, NY: ACM Press). 453–456.
Konkle, T., Brady, T. F., Alvarez, G. A., and Oliva, A. (2010a). Scene memory is more detailed than you think: the role of scene categories in visual long-term memory. Psychol. Sci. 21, 1551–1556.
Konkle, T., Brady, T. F., Alvarez, G. A., and Oliva, A. (2010b). Conceptual distinctiveness supports detailed visual long-term memory. J. Exp. Psychol. Gen. 139, 558–758.
Lee, S. W., Bülthoff, H. H., and Poggio, T. (eds). (2000). “Biologically motivated computer vision,” in Proceedings of the First IEEE International Workshop, BMVC 2000, Seoul, Korea, May 15–17, 2000 (Oxford: Springer).
Melcher, D. (2010). Accumulating and remembering the details of neutral and emotional scenes. Perception 39, 1011–1025.
Miller, M. B., and Gazzaniga, M. S. (1998). Creating false memories for visual scenes. Neuropsychologia 36, 513–520.
Oliva, A., and Torralba, A. (2007). The role of context in object recognition. Trends Cogn. Sci. (Regul. Ed.) 11, 520–527.
Pertzov, Y., Avidan, G., and Zohary, E. (2009). Accumulation of visual information across multiple fixations. J. Vis. 9, 2 1–12.
Rorissa, A., Clough, P., and Deselaers, T. (2008). Exploring the relationship between feature and perceptual visual spaces. J. Am. Soc. Inform. Sci. Tech. 59, 770–784.
Schacter, D. L. (1996). Illusory memories: a cognitive neuroscience analysis. Proc. Natl. Acad. Sci. U.S.A. 93, 13527–13533.
Schacter, D. L., Norman, K. A., and Koutstaal, W. (1998). The cognitive neuroscience of constructive memory. Annu. Rev. Psychol. 49, 289–318.
Shepard, R. N. (1967). Recognition memory for words, sentences and pictures. J. Verbal Learn. Verbal Behav. 6, 156–163.
Snow, R., O’Connor, B., Jurafsky, D., and Ng, A. (2008). “Cheap and fast – but is it good? Evaluating non-expert annotations for natural language tasks,” in Proceedings of the Conference on Empirical Methods in Natural Language Processing (Honolulu, HI: Association for Computational Linguistics), 254–256.
Sorokin, A., and Forsyth, D. (2008). “Utility data annotation with Amazon Mechanical Turk,” in Proceedings of the IEEE Computer Society Conference on Computer Vision and Pattern Recognition Workshops IEEE, Anchorage, Alaska, 1–8.
Tatler, B. W. (2001). Characterising the visual buffer: real-world evidence for overwriting early in each fixation. Perception 30, 993–1006.
Tatler, B. W., Gilchrist, I. D., and Rusted, J. (2003). The time course of abstract visual representation. Perception 32, 579–592.
Tatler, B. W., and Land, M. F. (2011). Vision and the representation of the surroundings in spatial memory. Philos. Trans. R. Soc. Lond. B Biol. Sci. 366, 596–610.
Tatler, B. W., and Melcher, D. (2007). Pictures in mind: initial encoding of object properties varies with the realism of the scene stimulus. Perception 36, 1715–1729.
Triesch, J., Ballard, D. H., Hayhoe, M. M., and Sullivan, B. T. (2003). What you see is what you need. J. Vis. 3, 86–94.
Vo, M. L.-H., and Schneider, W. X. (2010). A glimpse is not a glimpse: differential processing of flashed scene previews leads to differential target search benefits. Vis. Cogn. 18, 171–200.
Vogt, S., and Magnussen, S. (2007). Long-term memory for 400 pictures on a common theme. Exp. Psychol. 54, 298–303.
Warrington, E. K., and Shallice, T. (1984). Category-specific semantic impairments. Brain 107, 829–854.
Keywords: visual memory, working memory, scene perception
Citation: Melcher D and Murphy B (2011) The role of semantic interference in limiting memory for the details of visual scenes. Front. Psychology 2:262. doi: 10.3389/fpsyg.2011.00262
Received: 14 April 2011; Accepted: 21 September 2011;
Published online: 14 October 2011.
Edited by:
Anna M. Borghi, University of Bologna and Institute of Cognitive Sciences and Technologies, ItalyReviewed by:
Ken McRae, University of Western Ontario, CanadaMark E. Wheeler, University of Pittsburgh, USA
Ben Tatler, University of Dundee, UK
Copyright: © 2011 Melcher and Murphy. This is an open-access article subject to a non-exclusive license between the authors and Frontiers Media SA, which permits use, distribution and reproduction in other forums, provided the original authors and source are credited and other Frontiers conditions are complied with.
*Correspondence: David Melcher, Center for Mind/Brain Sciences, University of Trento, Corso Bettini 31, 38068 Rovereto, Trento, Italy. e-mail:ZGF2aWQubWVsY2hlckB1bml0bi5pdA==