- 1Department of Educational Psychology and Counseling, National Taiwan Normal University, Taipei, Taiwan
- 2State Key Laboratory of Cognitive Neuroscience and Learning and IDG/McGovern Institute for Brain Research, Beijing Normal University, Beijing, China
- 3School of Systems Science, Beijing Normal University, Beijing, China
- 4Institute of Learning Sciences, National Tsing Hua University, Hsinchu, Taiwan
- 5Department of Psychology and Institute for CyberScience, Pennsylvania State University, University Park, PA, USA
Most research into the neural mechanisms of humor has not explicitly focused on the association between emotion and humor on the brain white matter networks mediating this connection. However, this connection is especially salient in gelotophobia (the fear of being laughed at), which is regarded as the presentation of humorlessness, and two related traits, gelotophilia (the enjoyment of being laughed at) and katagelasticism (the enjoyment of laughing at others). Here, we explored whether the topological properties of white matter networks can account for the individual differences in the laughter-related traits of 31 healthy adults. We observed a significant negative correlation between gelotophobia scores and the clustering coefficient, local efficiency and global efficiency, but a positive association between gelotophobia scores and path length in the brain's white matter network. Moreover, the current study revealed that with increasing individual fear of being laughed at, the linking efficiencies in superior frontal gyrus, anterior cingulate cortex, parahippocampal gyrus, and middle temporal gyrus decreased. However, there were no significant correlations between either gelotophilia or katagelasticism scores or the topological properties of the brain white matter network. These findings suggest that the fear of being laughed at is directly related to the level of local and global information processing of the brain network, which might provide new insights into the neural mechanisms of the humor information processing.
Introduction
Laughter is an innate human emotional expression (Ruch and Ekman, 2001). Different types of laughter have different social consequences: while warm smiles facilitate social interactions, scornful laughing or ridiculing often leaves the target of the laughter having anxiety and pain (Chen et al., 2011). Different people also experience laughter differently: while some people enjoy mocking others, a few enjoy being laughed at. Long-term clinical observations suggest that early and repeated experiences of being mocked and laughed at in childhood and youth are causally associated with the fear of being laughed at, a symptom called gelotophobia (Titze, 2009). Continued experience of gelotophobia may lead to an individual's lack of liveliness, spontaneity, and joy (Titze, 2009). Moreover, individuals with a high gelotophobia score have difficulty perceiving and interpreting their own emotions (Papousek et al., 2009; Weiss et al., 2012); they do not discriminate between playful teasing and nature teasing (Platt, 2008). In addition, individuals with gelotophobia reported experiencing shame and fear more frequently, feeling happy more seldom during a typical week (Platt and Ruch, 2009). Hence, they would less likely enjoy their positive emotions while having high degrees of social anxiety, social phobia, and shame-bound anxiety. EEG research indicated loose functional coupling of prefrontal and posterior cortex in individuals with gelotophobia when expressing anger and aggression (Papousek et al., 2016).
In terms of the personality, gelotophobia positively correlated with introversion and neuroticism and negatively correlated with agreeableness and openness (Ruch et al., 2008; Chen et al., 2011, 2013). Individual with high gelotophobia would be introvert and emotionally instable, while not open to new experiences and be friendly with people.
Ruch et al. (2009) and Ruch and Proyer (2009) examined the psychometric properties of gelotophobia, and identified two related characters and their correlations with gelotophobia: gelotophilia (the enjoyment of being laughed at) and katagelasticism (the enjoyment of laughing at others). So far, research has shown that gelotophobia negatively correlated with gelotophilia but positively associated with katagelasticism (Ruch and Proyer, 2009; Chen et al., 2011).
Previous studies of humor processing have identified the cognitive components of humor processing and their associated neural mechanisms (Ozawa et al., 2000; Goel and Dolan, 2001). In two recent fMRI studies, Chan et al. (2012, 2013) divided the cognitive processes of humor comprehension into three stages: the incongruity stage, which engages the right middle temporal gyrus (MTG) and right medial frontal gyrus (MFG); the solution stage, which is anchored in the left superior frontal gyrus (SFG) and the left inferior parietal lobule (IPL); and the elaboration stage, which includes the left ventromedial prefrontal cortex (vmPFC), the bilateral amygdala, and the bilateral parahippocampal gyri. To date, however, very few studies have specifically examined the emotional functions of humor processing, or applied this knowledge of laughter-related brain networks to investigate the underlying neural mechanisms of laughter-related behavioral traits (i.e., gelotophobia, gelotophilia, and katagelasticism). This is surprising given the observed relationship between gelotophobia and humor processing.
The human brain is a complex network, both structurally and functionally, and consists of densely connected neural units (Sporns et al., 2005; Sporns, 2011). Advances in diffusion imaging techniques have made it possible to clearly delineate white matter (WM) tracts, which allows the modeling of the human brain as a complex network with graph-theoretical analytic tools (Hagmann et al., 2008; Bullmore and Sporns, 2009; Gong et al., 2009). Graph theoretical approaches have been applied effectively to characterize the topological architectures of whole-brain WM networks (Rubinov and Sporns, 2010). Using such approaches, recent studies have made significant progresses in describing the relationship between brain topological characteristics and cognitive function (Li et al., 2009; Wen et al., 2011). Researchers have also begun investigating the relationship between WM structure and individual traits. Specifically, the technique of diffusion tensor imaging (DTI) has enabled researchers to correlate WM structure with aspects of personality, creativity, and other psychological traits (Takeuchi et al., 2010, 2012; Xu and Potenza, 2012). However, no work in this direction that has examined structural or functional brain properties in terms of network models and their potential relationship to laughter-related performances (i.e., gelotophobia, gelotophilia, and katagelasticism). In this study, we attempt to fill this gap in the literature by using DTI and graph-theoretical network analyses.
The present study first attempt to investigate the connection between fear to be laughed at and network topological properties within few direct references. Based on the close relationship between fear to be laughed at and personality (Ruch et al., 2008), we refer to the openness personality positively connect to integrated efficiency of default mode network (Beaty et al., 2016) which includes the dorsolateral and ventral medial prefrontal cortex, posterior cingulate cortex, inferior parietal lobule, lateral temporal cortex, hippocampal formation (Buckner et al., 2008); it means when individual was more opened to new things, and the regions of default mode network worked better, and vice versa.
To address these issues, the present study makes a first attempt to explore the correlations between topological properties of the structural brain network underlying laughter-related traits, including gelotophobia, gelotophilia, and katagelasticism. Previous research has indicated significant relations among the laughter-related traits and personality traits (Chen et al., 2011); gelotophobia positively correlated to neuroticism but was negatively associated to extraversion, agreeableness and openness whereas for gelotophilia, it was positive correlated to extraversion and openness. With regard to the negative relation between katagelasticism and agreeableness. In additions, past research on the relation between five-factor personality (Xu and Potenza, 2012) and white matter integrity also pointed out that, fractional anisotropy was negatively related to neuroticism but positively related to openness and agreeableness. In particular, according to the positive relationship between openness and global efficiency of default mode network (Beaty et al., 2016); we hypothesized that the WM network efficiency would differ significantly between individuals with distinct profiles of gelotophobia, gelotophilia, and katagelasticism characteristics. Given the different characteristics of laughter toward oneself and others, we further expected that the network efficiency would decrease with the fear of being laughed at and the enjoyment of laughing at others, but increase with the enjoyment of being laughed at.
Methods
Subjects
Thirty-one neurologically healthy volunteers (18 females; 24.74 ± 2.48 years old; range, 20–30 years old) were included in this study (see Table 1). All participants were recruited from National Taiwan Normal University and had no history of neurological or psychiatric disorders. Participants were asked to refrain from ingesting caffeine and alcohol for the 24 h preceding the experiment. The study was approved by the Research Ethics Committee of National Taiwan University Hospital. All of the subjects gave their written informed consent to participate before the study.
PhoPhiKat Score
Before the experiment, participants completed the Chinese version of the PhoPhiKat-45 (gelotophobia, gelotophilia, and katagelasticism; Chen et al., 2011), a questionnaire translated from the German version (Ruch and Proyer, 2009) that has been successfully used to assess gelotophobia (e.g., “When strangers laugh in my presence I often relate it to me personally”), gelotophilia (e.g., “When I am with other people, I enjoy making jokes at my own expense to make the others laugh.”), and katagelasticism (e.g., I enjoy exposing others and I am happy when they get laughed at). The questionnaire had 45 items, with 15 items devoted to each category of gelotophobia, gelotophilia, and katagelasticism. A 4-point scale was used (1 = “strongly disagree”; 2 = “disagree”; 3 = “agree”; 4 = “strongly agree”) for each item, and the larger the accumulated points under a category, the higher the participant has the corresponding characteristic. The Cronbach α consistency coefficient was 0.85, indicating a high degree of internal consistency. Further, the mild correlations with criterions (i.e., humor-style, aggressive behavior, personality, and self-esteem) were found (rs > 0.50), suggesting a moderate validity of the measurement (Chen et al., 2011).
MRI Acquisition
Images were acquired with a 3T scanner (Siemens Trio, Siemens Medical Solutions USA) at National Taiwan University Hospital, Taiwan. DTI were acquired by using a single-shot echo planar imaging-based sequence with sensitivity encoding and the following parameters: a parallel imaging factor of 2.0; coverage of the whole brain; 2.5-mm slice thickness with no inter-slice gap; 60 axial slices; TR = 11,000 ms; TE = 98 ms; 30 optimal non-linear diffusion weighting directions with b = 1000 s/mm2 and five additional images without diffusion weighting (i.e., b = 0 s/mm2); average, 3; acquisition matrix, 96 × 96; field of view (FOV), 256 × 248 mm2. A T1-weighted MPRAGE sequence was used to acquire high-resolution anatomical images of the entire brain with the following parameters: TR = 1560 ms, TE = 3.68 ms, flip angle = 15⋅, field of view = 256 × 256 mm2, and matrix size = 256 × 256; 192 sagittal slices; 1 × 1 × 1 mm3 resolution.
Data Preprocessing
The preprocessing pipeline for each subject is composed of the following steps: brain extraction, correction for eddy-current distortion and simple head-motion, correction for b-matrix (Leemans and Jones, 2009), and computation for diffusion tensor and fractional anisotropy. All of the image preprocessing was implemented by a pipeline tool for diffusion MRI (PANDA; Cui et al., 2013) that has utilized the FMRIB Software Library (FSL; Smith et al., 2004), Pipeline System for Octave and Matlab (PSOM; Bellec et al., 2012), Diffusion Toolkit (Wang et al., 2007) and MRIcron (http://www.mccauslandcenter.sc.edu/mricro/mricron/).
Construction of Binary White Matter Connectivity Networks
Figure 1 illustrates the flowchart of WM brain network construction. The first step is to determine two basic network elements, nodes and edges, as defined below.
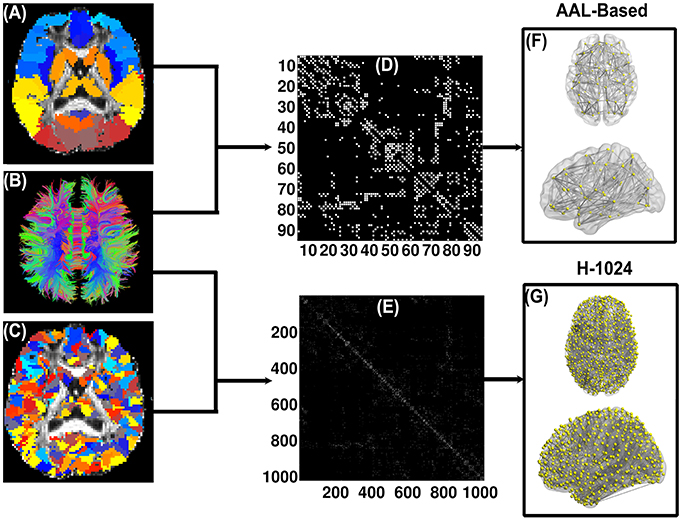
Figure 1. Flow chart of the DTI-based WM brain network construction. (A) and (C) are the subject-specific AAL-based parcellation and H-1024 parcellation in the diffusion native space, respectively; (B) shows the white matter fibers reconstructed by deterministic tractography; (D) and (E) are the AAL-based and H-1024 binary network matrix, respectively; (F) and (G) are the 3D representation of the anatomical brain network, which was shown using the in-house BrainNet Viewer package (http://www.nitrc.org/projects/bnv/; Xia et al., 2013).
Network Node Definition
In this study, the automated anatomical labeling (AAL, Tzourio-Mazoyer et al., 2002) atlas was used to segment the cerebral cortex of each subject into 90 regions (45 for each hemisphere) without the cerebellum. Each region represents a node of the DTI-based WM network. The detailed parceling processes were implemented according to the procedure proposed by Gong and colleagues (Gong et al., 2009). Briefly, the T1-weighted image was first non-linearly normalized to the MNI space by FMRIB's Linear Image Registration Tool (FNIRT, FSL, http://www.fmrib.ox.ac.uk/fsl/). Next, the fractional anisotropy image of each subject was co-registered to the individual T1-weighted image. Finally, the inverse transformations from the previous two steps were applied to the atlas, resulting in native-space GM parcellations for each subject.
Network Edge Definition
In this study, the deterministic fiber assignment continuous tracking (FACT) algorithm was applied to reconstruct whole-brain WM tracts (Mori et al., 1999) by the Diffusion toolkit (http://trackvis.org), which is embedded in PANDA (Cui et al., 2013). Specifically, the tracking procedure terminated if the turn angle of the fiber was greater than 45⋅ or the fiber entered a voxel with the fractional anisotropy less than 0.2. Two region pairs, A and B, were considered structurally connected (i.e., having an edge) if there existed at least three tracts with terminal points in both regions A and B (Bai et al., 2012). Combining the above definitions of the nodes and edges, we attained for each subject a 90 × 90 binary network whose elements indicated the existence/absence of an edge between any pair-wise regions.
High-Resolution Brain Network
Previous research showed that brain graph metrics are dependent on the resolution of the network (i.e., network size) (van den Heuvel et al., 2008; Wang et al., 2009; Zalesky et al., 2010; Bai et al., 2012). To explore the validity of our results, we further subdivided the AAL template into 1024 ROIs with equal size [i.e., high-resolution (H-1024)] (Zalesky et al., 2010; Bai et al., 2012) and constructed the brain networks accordingly. Similar to the low-resolution AAL (L-AAL) networks, the H-1024 networks were examined with respect to the relationships between PhoPhiKat scores and network metrics.
Network Analysis
Graph theoretical measures were used to characterize the topological architecture of the WM brain networks derived above. In the current study, both global network metrics and nodal metrics were computed. The global network metrics were computed for the mean clustering coefficient (Cp), the characteristic path length (Lp), the normalized Cp (γ), the normalized Lp (λ), small-worldness (ζ), global efficiency (Eglob), and local efficiency (Eloc). The nodal network metric was computed only for the nodal efficiency (Enodal). These graph-theoretical network metrics were calculated by using the GRETNA package (http://www.nitrc.org/projects/gretna/).
Clustering Coefficient Cp
The clustering coefficient of a network characterizes the segregation ability of the network by calculating the global mean of the clustering coefficients over all nodes, where the clustering coefficient of a node is defined as the ratio of the number of existing connections among the node's neighbors over all of their possible connections (Bullmore and Sporns, 2009).
Characteristic Path Length Lp
The characteristic path length is used to characterize the optimal routing for information transmission. The characteristic path length of a graph refers to the averaged shortest path lengths across all nodes, where the shortest path length of a node i is computed as the average number of distinct edges along the shortest path between nodal i and all other nodes in the networks. The characteristic path length of a network is computed as follows:
where N is the number of nodes in the graph G, and Lij is the shortest path length between nodes i and j.
Small-Worldness ζ
The concept of small-worldness in network science was originally proposed by Watts and Strogatz (Watts and Strogatz, 1998). Specifically, a network is considered a small-world network if it has similar shortest path lengths but higher clustering coefficients than degree-matched random networks. Typically, a small-world network should meet the following criteria: ζ = γ/λ > 1, where γ = Cpreal/Cprandom > 1, λ = Lpreal/Lprandom ≈1, where Cpreal and Lpreal are clustering coefficient and characteristic path length, respectively, of the real brain network, and Cprandom and Lprandom are the averaged values of the 100 matched random networks, which are the same as the real network in the number of nodes, edges, and degree distribution. The random rewiring procedure depicted by Maslov and Sneppen (Maslov and Sneppen, 2002) was used here to produce the 100 matched random networks for each subject.
Global Efficiency Eglob
Global efficiency is a global measure of the parallel information transfer ability of the whole network. It is computed as the average of the inverse of the “harmonic mean” of the characteristic path length (Latora and Marchiori, 2001):
where N is the number of nodes in the graph G, and Lij is the shortest path length between nodes i and j.
Local Efficiency Eloc
Local efficiency quantifies the network's ability to tolerate faults, corresponding to the efficiency of the information flow between the nearest neighbors of the node i (Latora and Marchiori, 2001). The local efficiency of a network is computed as follows:
where Gi is the sub-graph composed of the nearest neighbors of node i and the connections among them.
Nodal Efficiency Enodal
Nodal efficiency is a measure of the nodal capacity to communicate with other nodes of the network. The nodal efficiency for a given node (Enodal) was defined as the inverse of the harmonic mean of the shortest path length between this node and all other nodes in the network (Achard and Bullmore, 2007):
where Lij is the characteristic path length between node i and node j.
Statistical Analysis
To explore the relationship between the topological parameters (Cp, Lp, ζ, Eloc, Eglob, and Enodal) of WM brain networks and PhoPhiKat scores, general linear models (GLM) were applied with age, gender and years of education as covariates. Specifically, the GLM is as follows: Y = β0 + β1 × X + β2 × Age + β3 × Gender + β4 × Education, where Y is the topological parameter and X the PhoPhiKat score. The correlation was determined by examining the null hypothesis of β1 = 0. The threshold value for establishing the significance of correlation was set at p < 0.05 for the global metrics, p < 1/N (corrected for multiple comparisons) for the nodal metrics of the AAL-based networks.
Results
Small-World Properties of Brain Networks
The structural brain networks of all subjects showed a small-world architecture. More specifically, when compared with a matched random network, the WM networks had comparably shortest path lengths but higher clustering coefficients (ζ = 3.54 ± 0.32), suggesting that the overall topological properties were preserved regardless of the scores from the scales for gelotophobia, gelotophilia, and katagelasticism.
PhoPhiKat Scores and Network Properties
The GLM analysis showed a significant negative association between gelotophobia scores and the clustering coefficient (r = −0.40, p = 0.037), local efficiency (r = −0.48, p = 0.01), and global efficiency (r = −0.42, p = 0.027) after controlling for age, gender, and years of education, and a significant positive association with the characteristic path length (r = 0.41, p = 0.029, see Figure 2). However, there were no significant correlations between gelotophilia or katagelasticism scores and the topological properties of the WM network.
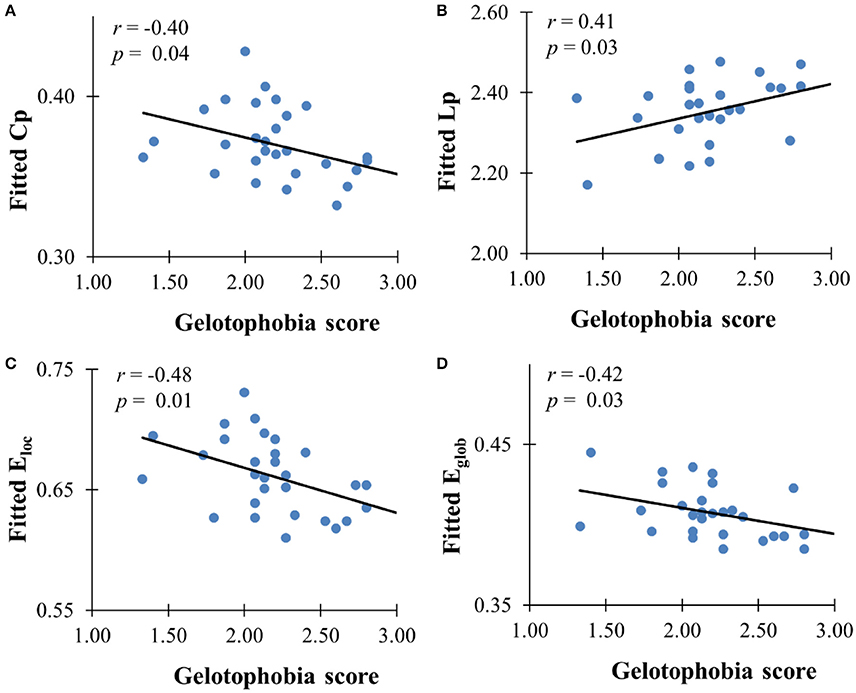
Figure 2. The correlation between gelotophobia score and global metrics derived from AAL-based WM network. (A) Clustering coefficient, (B) path length, (C) local efficiency, and (D) global efficiency. For all plots, we used linear regression to remove the influence of age, gender and years of education from brain network metrics.
We further investigated the specific brain regions associated with the tendency of an individual to fear being laughed at. Figure 3 shows the 3D surface visualizations of the results implemented using the Brain Net Viewer (www.nitrc.org/projects/bnv; Xia et al., 2013). As can be seen, significant correlations between gelotophobia scores and nodal efficiency were found in the inferior occipital gyrus (r = −0.62, p < 0.0001). Notably, we corrected for age, gender and years of education when computing the correlation, and we used p < 0.05/90 to correct for multiple comparisons.
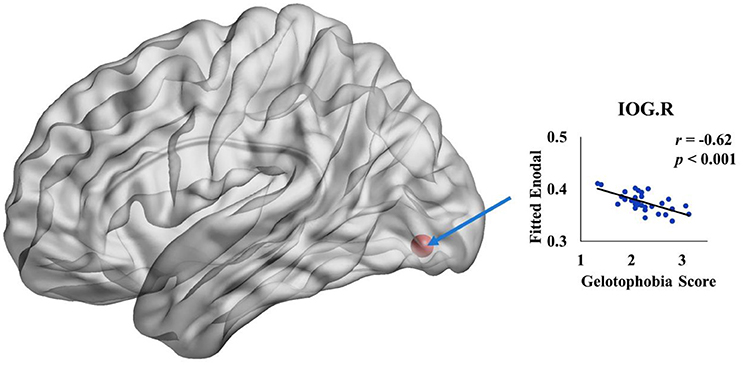
Figure 3. The spatial distribution of cortical region showing a significant association between nodal efficiency in the right inferior occipital gyrus and gelotophobia score (p < 0.05/90).
High-Resolution Structural Brain Networks
In addition to the analyses of AAL-based networks, we further analyzed the relationship between the high resolution-based H-1024 network and the PhoPhiKat scores. Consistent with findings from the AAL-based networks, there were no relationships between gelotophilia or katagelasticism and brain network properties, but there were significant negative associations between gelotophobia scores and local efficiency (r = −0.36, one-tailed p = 0.03) and global efficiency (r = −0.41, p = 0.028; see Figure 4). As shown in Figure 5, significant correlation between the nodal efficiency and gelotophobia score was found in right cuneus (r = −0.70, p < 0.001, uncorrected), parahippocampal gyrus (r = −0.67, p < 0.001, uncorrected), anterior cingulate and paracingulate gyri (r = −0.61, p < 0.001, uncorrected), and middle temporal gyrus (r = −0.50, p = 0.007, uncorrected). Furthermore, a significant positive association was also found between the characteristic path length and gelotophobia score (r = 0.41, p = 0.032). The only difference between the high resolution-based networks and the AAL-based networks seems to be that gelotophobia score showed no correlation with the clustering coefficient (r = −0.24, p = 0.214).
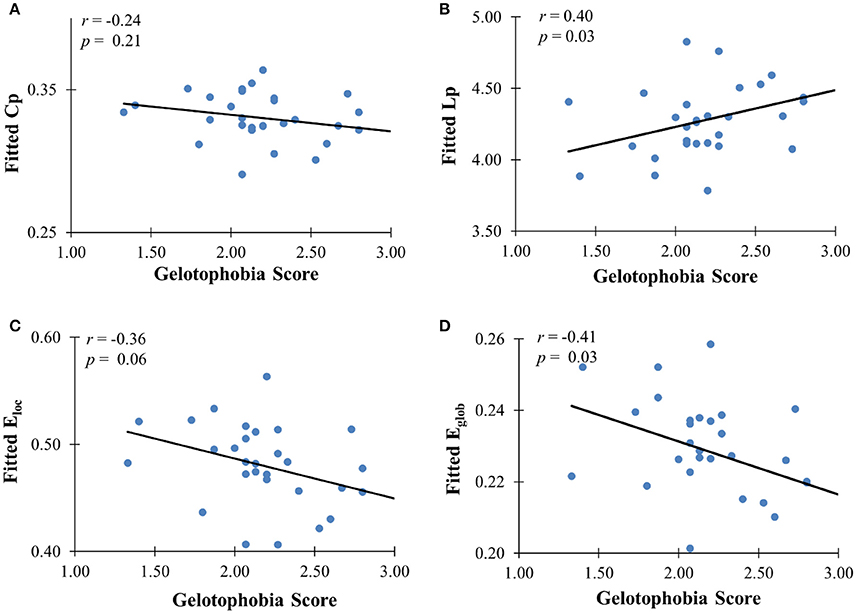
Figure 4. The correlation between gelotophobia score and H-1024 WM network metrics. (A) Clustering coefficient, (B) path length, (C) local efficiency, and (D) global efficiency; For all plots, we used linear regression to remove the influence of age, gender and years of education from brain network metrics.
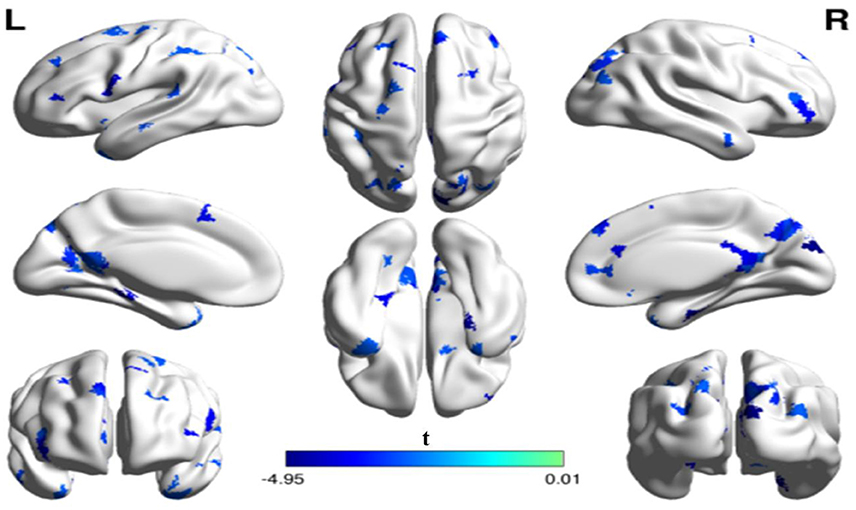
Figure 5. The spatial distribution of cortical regions showing a significant association between nodal efficiency and gelotophobia score (p < 0.01, uncorrected) in an H-1024 network. Note that three regions (the ACC, PHG, MTG) appeared in a bilateral manner and the CUN. R, right hemisphere; ACC, anterior cingulate cortex; CUN, Cuneus; PHG, parahippocampal gyrus; MTG, middle temporal gyrus.
Discussion
Gelotophobia refers to the experience of intense fear felt by an individual when being laughed at (Titze, 1996, 1997). Gelotophobia can cause significant problems in people's lives, leading them to be socially inept, cold, mean-spirited, or depressed. However, the neural mechanisms underlying gelotophobia are not well-understood, as there has been no research designed to address this question. The present study is a first attempt to uncover the neuro-cognitive mechanisms underlying gelotophobia and related laughter-related traits from the perspective of brain networks. Specifically, we aim at understanding how WM structure in the human brain subserves human laughter-related processing, given the role of WM in facilitating neural processing and communication across areas of the cortex and between cortical and subcortical regions.
The main finding of this study is the existence of a strong association between the topological metrics of the WM brain network and gelotophobia scores at both the global and the nodal level. For the global metrics, our results revealed a significant negative association between gelotophobia scores and the clustering coefficient, local efficiency and global efficiency; for the nodal metric, we observed a strong positive association between gelotophobia scores and the path length of the structural brain networks. Given that both global and local efficiency metrics significantly and negatively associated with gelotophobia scores, we suggest that an individual's fear of being laughed at related to the level of local and global information processing of the brain network. In other words, less efficiently connected brain structures show a higher level of gelotophobia. Part of our finding is also supported by a further analysis with High-resolution structural brain networks. Present study is a first attempt for discussing the connection between fear to be laughed at and WM network topological properties. Individual with fear to be laughed at had higher hostility and less stable emotion status, less interest to new things (Ruch et al., 2008). Our results are similar with the findings between openness personality and integrated efficiency of default mode network. Although the AAL network that we use is different from default mode network, but the finding still supports a decrease global efficiency of WM network among individual with gelotophobia which would not intend to open to new experience.
In addition to the overall correlations between brain network metrics and the gelotophobia score, our data also revealed that the more an individual fears being laughed at, the lower the connecting efficiencies the individual displays in right inferior occipital gyrus (IOG). IOG is relevant to emotions recognition; functional MRI result indicated a higher activation in right IOG during the process of identifying an anger face (Fusar-Poli et al., 2009). On the other hand, DTI results found a positive correlation between the mean diffusion (MD) of inferior frontal occipital fasciculusçŽĎmean diffusion (MD) and openness (Xu and Potenza, 2012). In particular, individuals with fear to be laughed at had less interest to trying something new (Chen et al., 2011), it was consistent to the finding that IOG and fear to be laughed at had negative connection. Although EEG research result could not provide detail information of brain regions, but it indicated the weakness of brain function for people with fear to be laughed at, which supports our finding, a negative connection between nodal efficiency and trait of fear to be laughed at. However, present study did not collect data about personality of participants; we could not investigate moderate effect of personality between fear to be laughed at and brain network. It's worthwhile for further study.
The present findings suggest that gelotophilia and katagelasticism were not significantly correlated with nodal efficiency of the WM network. It is possible that clearly defined neural networks exist only for gelotophobia because this condition was defined based upon rigorous long-term observations among patients by Titze (2009) and was a clinical syndrome concluded by qualitative analysis. In contrast, gelotophilia and katagelasticism were developed as expanded and ancillary concepts by theoretical generalizations about gelotophobia. The correlations between gelotophobia and mental traits are much stronger than the ones between gelotophilia, katagelasticism and mental traits (Ruch and Proyer, 2009; Chen et al., 2011); thus, significant associations between topological properties of the WM network and gelotophilia or katagelasticism could not be found.
There remain two methodological issues that we should consider for future follow-up research. First, we used the number of fibers as the weighting factor in the construction of the graphs, rather than other measures such as average fractional anisotropy (FA), mean diffusivity (MD), or a combination of such measures. FA and MD are measures of different aspects of the fibers; e.g., FA is relevant to fiber “integrity (magnitude of diffusion of water molecules in the brain)” whereas number of fibers is relevant to fiber “quantity.” Whether analyses based on FA, MD, and similar measures will provide similar brain network patterns as we showed in this study needs further investigation. Second, we built two spatial resolutions of the WM network in the present study, L-AAL and H-1024, to analyze the relationship between typological properties of the networks and the PhoPhiKat laughter-processing scores. There were some discrepancies in the regions that were correlated with gelotophobia between the L-AAL and H-1024 WM networks. Such discrepancies may be moderated by differences in the graph properties under the subregions of some anatomical structures. It revealed that constructing high-resolution WM network can provide a useful way to support or validate L-AAL-based WM network analyses. Therefore, graph analyses with different spatial resolutions in the future should be conducted with an aim to provide a more comprehensive picture of the topological properties of brain networks in the clinically normal and disordered populations.
Conclusion
The present study revealed significant correlations between gelotophobia and the underlying organization of the cortical anatomical network within the superior frontal gyrus, anterior cingulate cortex, parahippocampal gyrus, and middle temporal gyrus. Our study provides new insights into the structural substrates that underlie the individual personality trait of fear of being laughed at. These findings reveal patterns and efficiency of brain networks in gelotophobic individuals and at the same time provide significant implications for evaluating clinical populations with reference to altered connectivity in the neuroanatomy of the relevant populations.
Author Contributions
C-LW and SZ collected and analyzed the data and wrote the initial draft of the manuscript. Y-CC assisted in literature review and discussion. H-CC designed this study. YH, GG, and PL monitored and supervised all aspects of the study. All authors approved the final version of the paper.
Conflict of Interest Statement
The authors declare that the research was conducted in the absence of any commercial or financial relationships that could be construed as a potential conflict of interest.
Acknowledgments
We wish to thank the National Science Council for funding this project via the following grants: Neuropsychological Traits of Mathematically and Scientifically Talented Student with and without Asperger's Syndrome and Training Effect of Sense of Humor on Them in Taiwan (NSC100-2511-S-003-059-MY3). The work was also supported by the Ministry of Education, Taiwan, under the Aiming for the Top University Plan at National Taiwan Normal University. Finally, we would like to thank the International Research Intensive Center of Excellence of National Taiwan Normal University and the National Science Council, Taiwan, R.O.C. for their support (NSC104-2911-I-003-301).
References
Achard, S., and Bullmore, E. (2007). Efficiency and cost of economical brain functional networks. PLoS Comput Biol. 3:e17. doi: 10.1371/journal.pcbi.0030017
Bai, F., Shu, N., Yuan, Y., Shi, Y., Yu, H., Wu, D., et al. (2012). Topologically convergent and divergent structural connectivity patterns between patients with remitted geriatric depression and amnestic mild cognitive impairment. J. Neurosci. 32, 4307–4318. doi: 10.1523/JNEUROSCI.5061-11.2012
Beaty, R. E., Kaufman, S. B., Benedek, M., Jung, R. E., Kenett, Y. N., Jauk, E., et al. (2016). Personality and complex brain networks: the role of openness to experience in default network efficiency. Hum. Brain Mapp. 37, 773–779. doi: 10.1002/hbm.23065
Bellec, P., Lavoie-Courchesne, S., Dickinson, P., Lerch, J. P., Zijdenbos, A. P., and Evans, A. C. (2012). The pipeline system for Octave and Matlab (PSOM): a lightweight scripting framework and execution engine for scientific workflows. Front. Neuroinform. 6:7. doi: 10.3389/fninf.2012.00007
Buckner, R. L., Andrews-Hanna, J. R., and Schacter, D. L. (2008). The brain's default network: anatomy, function, and relevance to disease. Ann. N. Y. Acad. Sci. 1124, 1–38. doi: 10.1196/annals.1440.011
Bullmore, E., and Sporns, O. (2009). Complex brain networks: graph theoretical analysis of structural and functional systems. Nat. Rev. Neurosci. 10, 186–198. doi: 10.1038/nrn2575
Chan, Y., Chou, T., Chen, H., and Liang, K. (2012). Segregating humor comprehension and elaboration process of verbal jokes: an fMRI study. NeuroImage 61, 899–906. doi: 10.1016/j.neuroimage.2012.03.052
Chan, Y. C., Chou, T. L., Chen, H., Yeh, Y., Lavallee, J., Liang, K. C., et al. (2013). Towards a neural circuit model of verbal humor processing: an fMRI study of the neural substrates of incongruity detection and resolution. NeuroImage 66, 169–176. doi: 10.1016/j.neuroimage.2012.10.019
Chen, H., Chan, Y., Ruch, W., and Proyer, R. (2011). Evaluating the reliability and validity of a traditional Chinese version of the PhoPhiKat-45. Psychol. Testing 58, 119–145.
Chen, H., Chan, Y., Ruch, W., and Proyer, R. (2013). “Laughing at others and being laughed at in Taiwan and Switzerland: a cross-cultural perspective,” in Humour in Chinese life and culture: Resistance and control in modern Times, Vol. 2, eds J. M. Davis and J. Chey (Hong Kong: Hong Kong University Press), 215–229.
Cui, Z., Zhong, S., Xu, P., He, Y., and Gong, G. (2013). PANDA: a pipeline toolbox for analyzing brain diffusion images. Front. Hum. Neurosci. 7:42. doi: 10.3389/fnhum.2013.00042
Fusar-Poli, P., Placentino, A., Carletti, F., Landi, P., Allen, P., Surguladze, S., et al. (2009). Functional atlas of emotional faces processing: a voxel-based meta-analysis of 105 functional magnetic resonance imaging studies. J. Psychiatry Neurosci. 34, 418–432.
Goel, V., and Dolan, R. (2001). The functional anatomy of humor: segregating cognitive and affective components. Nat. Neurosci. 4, 237–238. doi: 10.1038/85076
Gong, G., Rosa-Neto, P., Carbonell, F., Chen, Z., He, Y., and Evans, A. C. (2009). Age and gender-related differences in the cortical anatomical network. J. Neurosci. 29, 15684–15693. doi: 10.1523/JNEUROSCI.2308-09.2009
Hagmann, P., Cammoun, L., Gigandet, X., Meuli, R., Honey, C. J., Wedeen, V. J., et al. (2008). Mapping the structural core of human cerebral cortex. PLoS Biol. 6:e159. doi: 10.1371/journal.pbio.0060159
Latora, V., and Marchiori, M. (2001). Efficient behavior of small-world networks. Phys Rev Lett. 87, 198701–198704. doi: 10.1103/PhysRevLett.87.198701
Leemans, A., and Jones, D. K. (2009). The B-matrix must be rotated when correcting for subject motion in DTI data. Magn Reson Med. 61, 1336–1349. doi: 10.1002/mrm.21890
Li, Y. H., Liu, Y., Qin, W., Li, K. C., Yu, C. S., and Jiang, T. Z. (2009). Brain anatomical network and intelligence. PLoS Comput. Biol. 5:e1000395. doi: 10.1371/journal.pcbi.1000395
Maslov, S., and Sneppen, K. (2002). Specificity and stability in topology of protein networks. Science 296, 910–913. doi: 10.1126/science.1065103
Mori, S., Crain, B. J., Chacko, V. P., and van Zijl, P. C. (1999). Three-dimensional tracking of axonal projections in the brain by magnetic resonance imaging. Ann. Neurol. 45, 265–269.
Ozawa, F., Matsuo, K., Kato, C., Nakai, T., Isoda, H., Takehara, Y., et al. (2000). The effects of listening comprehension of various genres of literature on response in the linguistic area: an fMRI study. NeuroReport 11, 1141–1143. doi: 10.1097/00001756-200004270-00001
Papousek, I., Ruch, W., Freudenthaler, H., Kogler, E., Lang, B., and Schulter, G. (2009). Gelotophobia, emotion-related skills and responses to the affective states of others. Pers. Indiv. Differ. 47, 58–63. doi: 10.1016/j.paid.2009.01.047
Papousek, I., Schulter, G., Rominger, C., Fink, A., and Weiss, E. M. (2016). The fear of other persons' laughter: Poor neuronal protection against social signals of anger and aggression. Psychiatry Res. 235, 61–68. doi: 10.1016/j.psychres.2015.11.049
Platt, T. (2008). Emotional responses to ridicule and teasing: should gelotophobes react differently? Humor 21, 105–128. doi: 10.1515/HUMOR.2008.005
Platt, T., and Ruch, W. (2009). The emotions of gelotophobes: shameful, fearful and joyless? Humor 22, 91–110. doi: 10.1515/humr.2009.005
Rubinov, M., and Sporns, O. (2010). Complex network measures of brain connectivity: uses and interpretations. Neuroimage 52, 1059–1069. doi: 10.1016/j.neuroimage.2009.10.003
Ruch, W., Beermann, U., and Proyer, R. (2009). Investigating the humor of gelotophobes: does feeling ridiculous equal being humorless? Humor 22, 111–143. doi: 10.1515/humr.2009.006
Ruch, W., and Ekman, P. (2001). “The expressive pattern of laughter,” in Emotion, Qualia, and Consciousness, ed A. W. Kaszniak (Tokyo: World Scientific Publisher), 426–433.
Ruch, W., and Proyer, R. T. (2009). Extending the studying of gelotophobia: on gelotophilies and katagelasticsits. Humor 22, 183–212. doi: 10.1515/HUMR.2009.009
Ruch, W., Proyer, R. T., and Popa, D. E. (2008). “The fear of being laughed at (gelotophobia) and personality,” in Series Humanistica, ed G. Baritiu (Cluj-Napoca: Romania), 53–68.
Smith, S. M., Jenkinson, M., Woolrich, M. W., Beckmann, C. F., Behrens, T. E., Johansen-Berg, H., et al. (2004). Advances in functional and structural MR image analysis and implementation as FSL. NeuroImage 23(Suppl 1), S208–S219. doi: 10.1016/j.neuroimage.2004.07.051
Sporns, O. (2011). The human connectome: a complex network. Ann. N. Y. Acad. Sci. 1224, 109–125. doi: 10.1111/j.1749-6632.2010.05888.x
Sporns, O., Tononi, G., and Kötter, R. (2005). The human connectome: a structural description of the human brain. PLoS Comput. Biol. 1:e42. doi: 10.1371/journal.pcbi.0010042
Takeuchi, H., Taki, Y., Sassa, Y., Hashizume, H., Sassa, Y., Nagase, T., et al. (2012). The association between resting functional connectivity and creativity. Cereb. Cortex. 22, 2921–2929. doi: 10.1093/cercor/bhr371
Takeuchi, H., Taki, Y., Sassa, Y., Hashizume, H., Sekiguchi, A., Fukushima, A., et al. (2010). White matter structures associated with creativity: evidence from diffusion tensor imaging. NeuroImage 51, 11–18. doi: 10.1016/j.neuroimage.2010.02.035
Titze, M. (1997). “The comical as a shame-generating factor,” in Shame: A Humor Emotion, eds R. Kühn, M. Raub and M. Titze (Opladen: Westdeutscher Verlag), 169–178.
Titze, M. (2009). Gelotophobia: the fear of being laughed at. Humor 22, 27–48. doi: 10.1515/HUMR.2009.002
Tzourio-Mazoyer, N., Landeau, B., Papathanassiou, D., Crivello, F., Etard, O., Delcroix, N., et al. (2002). Automated anatomical labeling of activations in SPM using a macroscopic anatomical parcellation of the MNI MRI single-subject brain. NeuroImage 15, 273–289. doi: 10.1006/nimg.2001.0978
van den Heuvel, M. P., Stam, C. J., Boersma, M., and Hulshoff Pol, H. E. (2008). Smallworld and scale-free organization of voxel-based resting-state functional connectivity in the human brain. NeuroImage 43, 528–539. doi: 10.1016/j.neuroimage.2008.08.010
Wang, J., Wang, L., Zang, Y., Yang, H., Tang, H., Gong, Q., et al. (2009). Parcellation-dependent small-world brain functional networks: a resting-state fMRI study. Hum. Brain Mapp. 30, 1511–1523. doi: 10.1002/hbm.20623
Wang, R., Benner, T., Sorensen, A. G., and Wedeen, V. J. (2007). Diffusion toolkit: a software package for diffusion imaging data processing and tractography. Proc. Intl Soc. Mag. Reson. Med. 15:3720.
Watts, D. J., and Strogatz, S. H. (1998). Collective dynamics of 'small-world' networks. Nature. 393, 440–442. doi: 10.1038/30918
Weiss, E. M., Schulter, G., Freudenthaler, H. H., Hofer, E., Pichler, N., and Papousek, I. (2012). Potential markers of aggressive behavior: the fear of other persons' laughter and its overlaps with mental disorders. PLoS ONE 7:e38088. doi: 10.1371/journal.pone.0038088
Wen, W., Zhu, W., He, Y., Kochan, N., Reppermund, S., Slavin, N., et al. (2011). Discrete neuroanatomical networks are associated with specific cognitive abilities in old age. J. Neurosci. 31, 1204–1212. doi: 10.1523/JNEUROSCI.4085-10.2011
Xia, M., Wang, J., and He, Y. (2013). BrainNet Viewer: a network visualization tool for human brain connectomics. PLoS ONE 8:e68910. doi: 10.1371/journal.pone.0068910
Xu, J., and Potenza, M. (2012). White matter integrity and five-factor personality measures in healthy adults. NeuroImage 59, 800–807. doi: 10.1016/j.neuroimage.2011.07.040
Keywords: gelotophobia, diffusion tensor imaging, graph theory, brain network, connectome
Citation: Wu C-L, Zhong S, Chan Y-C, Chen H-C, Gong G, He Y and Li P (2016) White-Matter Structural Connectivity Underlying Human Laughter-Related Traits Processing. Front. Psychol. 7:1637. doi: 10.3389/fpsyg.2016.01637
Received: 30 September 2015; Accepted: 06 October 2016;
Published: 27 October 2016.
Edited by:
Alain Morin, Mount Royal University, CanadaCopyright © 2016 Wu, Zhong, Chan, Chen, Gong, He and Li. This is an open-access article distributed under the terms of the Creative Commons Attribution License (CC BY). The use, distribution or reproduction in other forums is permitted, provided the original author(s) or licensor are credited and that the original publication in this journal is cited, in accordance with accepted academic practice. No use, distribution or reproduction is permitted which does not comply with these terms.
*Correspondence: Hsueh-Chih Chen, chcjyh@gmail.com
†Shared first authorship.