- Centre for Mental Health, Swinburne University of Technology, Hawthorn, VIC, Australia
Sleep quality, circadian phase, and mood are highly interdependent processes. Remarkably, there is currently no self-report questionnaire that measures all three of these clinically significant functions: The aim of this project was to address this deficit. In Study 1, 720 participants completed a set of potential items was generated from existing questionnaires in each of the three domains and refined to follow a single presentation format. Study 2 used an independent sample (N = 498) to interrogate the latent structure. Exploratory factor analysis was used to identify a parsimonious, three-factor latent structure. Following item reduction, the optimal representation of sleep quality, circadian phase, and mood was captured by a questionnaire with three 5-item scales: Depressed Mood, Morningness, and Good Sleep. Confirmatory factor analysis found the three-scale structure provided adequate fit. In both samples, Morningness and Good Sleep were positively associated, and each was negatively associated with the Depressed Mood scale. Further research is now required to quantify the convergent and discriminant validity of its three face-valid and structurally replicated scales. The new sleep, circadian rhythms, and mood (SCRAM) questionnaire is the first instrument to conjointly measure sleep quality, circadian phase, and mood processes, and has significant potential as a clinical tool.
Introduction
A range of evidence in normative and clinical populations demonstrates that sleep quality, circadian phase, and mood are highly interdependent (Mason and Harvey, 2014; Soehner et al., 2014, 2016). In clinical settings this generates a common problem: When a patient presents with some combination of sleep quality, circadian phase, and mood problems, where should treatment be targeted (Harvey, 2015)? At least in part, this clinical problem arises from the fact that, while well-validated measures of sleep quality, circadian phase, and mood exist, there is no self-report instrument that quantifies function in all three domains. Such an instrument would provide a quantum advance over existing single-construct measures that, while validly capturing the individual construct of interest, pay no attention to independent and overlapping variance arising from the mechanistic interplay between the three processes. The aim of this project was to take the first step toward addressing this problem by developing a novel self-report questionnaire to measure sleep quality, circadian phase, and mood processes.
There are strong reasons to consider the interaction of sleep quality, circadian phase, and mood problems in treatment. Circadian phase refers to the timing of endogenous circadian rhythms relative to 24-h clock time (Czeisler et al., 1989; Kripke et al., 2007). Circadian phase has reliable associations with diurnal preference of activity and rest, or chronotype (Duffy et al., 2001; Mongrain et al., 2004). Chronotype is anchored by extremes of “morningness” and “eveningness.” Morningness being the preference for earlier activity and sleep times, eveningness the preference for later activity and sleep times (Duffy et al., 1999; Baehr et al., 2000). Sleep quality involves a subjective assessment of total sleep duration, sleep latency, wake after sleep onset, and qualitative interpretation of the depth and restfulness of the sleep period (Buysse et al., 1989). Sleep quality and circadian phase are interdependent processes with individuals high on morningness reporting better sleep quality (Wittmann et al., 2006; Barclay et al., 2016). Moreover, a stable circadian phase, i.e., having regular sleep times, has been found to improve sleep quality (Gruber et al., 2011; Harvey et al., 2011), and the circadian system regulates antidepressant and mood stabilizing medication pathways in the brain (McClung, 2013). Data suggests that cognitive behavioral therapy for insomnia can alleviate symptoms of depression (Manber et al., 2008), and in turn Chan et al. (2014) found high levels of eveningness and insomnia were independent risk factors of non-remitting depression. It is clear therefore, that comprehensive consideration of all three processes has the potential to improve case formulation and treatment planning.
Clinical interactions between sleep quality, circadian phase, and mood processes limit current self-report measures. For example, self-report measures of sleep quality (such as the Pittsburgh Sleep Quality Index) may have difficulty in reliably differentiating individuals with depression from primary insomnia (Buysse et al., 1989). Grandner et al. (2006) hypothesized that self-report measures of sleep quality may in part reflect negative cognitive processes and pessimism rather than sleep quality alone. Similarly, individuals with insomnia scored in the mild symptomology bracket of the Beck Depression Inventory-II (Carney et al., 2009). In a study by Abe et al. (2011), 64% of individuals who had a delayed circadian phase (as measured by the Morningness-Eveningness Questionnaire, Horne and Östberg, 1976) had moderate to severe depressive symptoms. Suggesting a bidirectional link, Robillard et al. (2013) observed that a delayed evening sleep phase is common in those with both unipolar and bipolar depression. Finally, shifts in circadian phase (such as those experienced in jetlag and shift-work) leads to sleep quality complaints (Rajaratnam and Arendt, 2001), and poor sleep quality can also impact circadian function (Morin, 2013).
The overarching aim of this project was to develop a single questionnaire to measure the three interconnected processes of sleep quality, circadian phase, and mood. Such a questionnaire has the potential to guide assessment and treatment given the important clinical conundrum that arises when a client presents with a combination of complaints in the three domains. The present project is a critical first step in an ongoing program of research. The aim of Study 1 was to develop a brief three-factor self-report measure that provides maximal measurement separation between the domains of sleep quality, circadian phase and mood. Depressed mood in physical illnesses is common and leads to an increased mortality rate (Katon, 2003); autonomic function (Grimaldi et al., 2016) and obesity (Haus et al., 2016) have both been associated with sleep and circadian disruption. These processes may be linked by fundamental physiological dysregulation (see, for example, Kemp and Quintana, 2013; Beauchaine and Thayer, 2015; Gruber et al., 2015). As part of a preliminary external validation of the measure, we predicted that scale scores from the new instrument would demonstrate intelligible patterns of convergent and divergent validity with single-item measures of sleep, physical, and mental health problems. Study 2 aimed to confirm the measure's theoretical three-factor structure using confirmatory factor analysis.
Study 1: Method
Study Design and Data Analysis
Instrument development was guided by the PROMIS standards (Cella et al., 2007). Item generation involved drawing items from existing questionnaires in the three domains, standardizing them to the same response format and making small changes to wording where necessary. Item reduction was achieved by conducting an Exploratory Factor Analysis (EFA) on responses from a predominantly student sample to this draft set of items. Preliminary investigation of external validity was conducted via associations with self-reported physical and mental health outcomes.
Item Generation
To develop a comprehensive list of items measuring the three domains we examined relevant topic areas from which we could generate newer items. Draft items were identified from highly cited self-report measures of sleep quality, circadian phase, and pathological mood (symptoms of depression and also hypo/mania). Nine sleep scales (e.g., PSQI Buysse et al., 1989 and Insomnia Severity Index Bastien et al., 2001); five circadian rhythm questionnaires [e.g., Munich Chronotype Questionnaire (Roenneberg et al., 2003) and Morningness-Eveningness Questionnaire (Horne and Östberg, 1976)], and 20 mood questionnaires [e.g., BDI-II (Beck et al., 1996), Centre for Epidemiological Studies Depression Scale (Radloff, 1977), and The Hospital Anxiety and Depression Scale (Zigmond and Snaith, 1983)] were used. As part of this generative process we created items that had purified wording, with the specific aim of decreasing overlap between the three domains. Items were selected for being face-valid as relatively pure measures of only one of the three processes. Draft items were then reworded to permit a shared response format (below) and/or to improve clarity and brevity. The preliminary item pool contained 170 items, n = 58 measuring sleep quality, n = 60 measuring circadian phase, and n = 52 measuring problems with mood (full list included in the Supplementary Materials).
The given question prompt was: “The following questions ask about your sleep, mood and timing of daily activities. Pick the answer which best describes you over the past two weeks.” A 2-week timeframe was deemed appropriate, given the nature of the processes under investigation (e.g., 2 weeks is the minimum timeframe required for DSM-5 diagnosis of Major Depressive Episode (American Psychiatric Association, 2013). A 6-point Likert-type response scale (Strongly Disagree to Strongly Agree) was selected for the new questionnaire. We chose not to have a middle response option to avoid ambiguity, as middle response options can reflect socially desirable responding, indifference, uncertainty, or non-applicability (Garland, 1991; Nowlis et al., 2002). Six response categories was considered an optimal balance between the higher validity associated with increasing response options and participant response burden (Chang, 1994; Preston and Colman, 2000).
Exploratory Factor Analysis
The expected three factor structure was forced onto the item set. For completeness, a range of empirical methods was also used to explore the number of factors to extract (O'Connor, 2000). The scree plot (Tabachnick and Fidell, 2013), minimum average partial test (Velicer, 1976), parallel analysis (Horn, 1965), and Kaiser-Guttman's criteria (Tabachnick and Fidell, 2013) were all examined. Using an orthogonal rotation (varimax), the adequacy of all factor solutions was compared against the following criteria: item communality magnitudes of over 0.40, cross-loadings less than 0.32 and at least five strongly loading (>0.50) items on each factor (Costello and Osborne, 2005).
Item Reduction
To generate the three scale questionnaire, two item reduction steps were applied. First, items were removed if they failed to load with sufficient strength (< 0.32) on any factor, had low communalities (<0.2), or had high cross-loading (>0.3) (Tabachnick and Fidell, 2013). The item content of the final three-scale questionnaire was developed from the remaining 98 items using the following principles: (1) each scale should have the minimum number of items for internal reliability, (2) each scale should contain reverse-coded items, and equal numbers of reverse-coded items were required for each scale, and (3) heterogeneity of meaning across items was sought to cover the breadth of the domain being measured. When items with comparable loadings were semantically similar, brevity and face validity were preferred.
Preliminary Investigation of External Correlates
Participants completed standard demographic questions including age and gender, and were asked dichotomous (Yes/No) questions about physical and mental health problems. For example, “Have you ever been diagnosed with a mental disorder”? Affirmative responses were followed by a request for participants to describe the mental disorder in an open response format. Age, gender, physical health problems, history of mental illness, and self-reported difficulties sleeping were investigated as external correlates of the final questionnaire.
Participants
A predominantly university student sample (18 years or older) was recruited. A total of 890 individuals commenced the questionnaire package online, with 783 (88%) complete responses recorded (see Table 1 for sample characteristics). Participants were largely first-year university students who were participating in a research experience program (which included on-campus and online students). Other recruitment methods included advertisements on social media and through contacts of the researchers.
Materials and Procedures
A questionnaire package was developed and delivered online using Qualtrics software (https://www.qualtrics.com/au/), Version 15. Participants were given a direct link to the survey. Order of items in the 170-item pool was randomized prior to participant administration. A single item measuring mindless responding was included: “Some participants don't read questionnaires carefully, please select ‘agree’ for this question.” Ninety-two percent of participants selected the correct response for this item. The 62 incorrect responses were excluded leaving a final sample of 720 participants for item reduction analyses [adequate for an EFA of 170 items Tabachnick and Fidell, 2013]. The university's internal ethics review board approved study procedures.
Study 1: Results
Exploratory Factor Analysis
Preliminary analyses showed the data were amenable to factor analysis. Inspection of the correlation matrix at the item generation stage showed numerous correlations >0.3 with sampling adequacy meeting criteria (Kaiser-Meyer-Olkin = 0.94). Bartlett's test of sphericity indicated that the observed correlation matrix deviated significantly from the identity matrix [ = 84692.54, p < 0.001].
The enforced orthogonal three-factor solution (explaining 30% of the total variance) was robust against Costello and Osborne's [35] criteria. Inspection of item content and factor loadings in the forced 3-factor solution generated three robust factors with item content of depressed mood, morningness, and sleep quality.
Data-driven approaches to factor extraction generated a wide range of number of factors to extract. Inspection of the scree plot indicated five factors, the minimum average partial test indicated 26 factors, parallel analysis indicated 26 factors, and Kaiser-Guttman's criteria indicated 35 components with eigenvalues greater than 1. None of the empirically derived solutions were robust against the criteria of Costello and Osborne (2005). The 35-factor solution had five stable factors, the 26-factor solutions had four stable factors and the five-factor solution had three stable factors all explored for item content. All factor solutions indicated factor content of: depressed mood, morningness, and sleep quality; the fourth factor in the 26- and 35-factor solution included item content of value and beliefs about sleep, and the 35-factor model had a fifth stable factor with items pertaining to hypomania.
Item Reduction
After initial item removal using the above criteria, 36 items remained on a provisional depressed mood scale, 24 items on a provisional sleep quality scale, and 38 items on a provisional morningness scale. Applying the principles outlined above to select the final items, the scales could be unambiguously named Depressed Mood, Morningness, and Good Sleep on the basis of their item content.
The 15-item Sleep, Circadian Rhythm and Mood (SCRAM) questionnaire had three 5-item scales (one reverse-scored item per scale) (Appendix). As shown in Table 2, these items had moderate-large loadings on their respective factors, and the resultant scales had adequate internal reliabilities, and interpretable intercorrelations in this sample (Table 3).
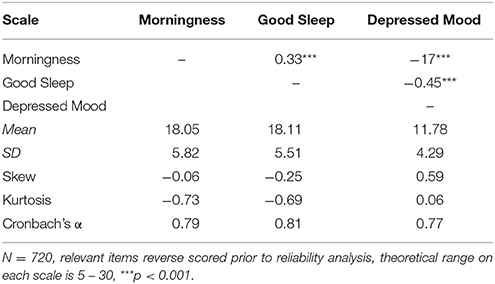
Table 3. Correlations, Descriptive Statistics and Cronbach's Alpha for the Morningness, Good Sleep and Mood Scales.
Figure 1 shows that scores on the SCRAM Morningness and Good Sleep scales were relatively normally distributed, while a non-significant positive skew was observed for scores on the Depressed Mood scale.
External Correlates
Women scored significantly higher on scores of Morningness than men [t(717) = 2.77, p = 0.005, Hedge's g = 0.27]; and a non-significant trend was observed for women reporting lower levels of Good Sleep [t(717) = 1.78, p = 0.075]. Scores on Depressed Mood did not differ by gender [t(717) = 0.053, p = 0.96]. Age was associated positively with Morningness [r(719) = 0.21, p < 0.001], and negatively with Depressed Mood [r(719) = −0.13, p = 0.001].
Preliminary External Validation of the SCRAM Factors
Independent samples t-tests were performed to investigate differences in Morningness, Good Sleep, and Depressed Mood in dichotomous self-reported health outcomes for mental illnesses (31% of the sample), reported physical complaints (13%) and sleep problems (36%) (see Table 4).
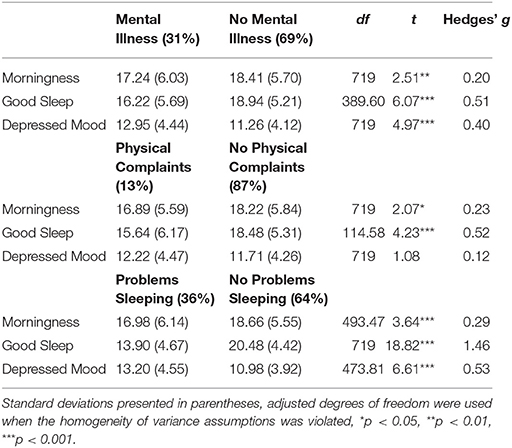
Table 4. Differences in Mean Good Sleep, Depressed Mood and Morningness Scores Across Self-Reported Mental Illness Status, Physical Complaints, and Sleep Problems.
Self-reporting a diagnosed mental illness was associated with lower scores on Morningness [t(718) = 2.55, p = 0.011, Hedge's g = 0.21] and Good Sleep [t(390.22) = 6.07, p < 0.001, Hedge's g = 0.51], and higher scores on Depressed Mood [t(718) = 4.97, p < 0.001, Hedge's g = 0.40]. Self-reported presence of physical complaints was associated with lower scores on Good Sleep [t(114.65) = 4.23, p < 0.001, Hedge's g = 0.52] and Morningness [t(718) = 2.10, p = 0.036, Hedge's g = 0.23], but was unrelated to Depressed Mood [t(718) = 1.07, p = 0.28]. Finally, self-reported problems sleeping were associated with lower scores on Good Sleep [t(718) = 18.84, p < 0.001, Hedge's g = 1.46] and Morningness [t(491.89) = 3.57 p < 0.001, Hedge's g = 0.29] and higher scores on Depressed Mood [t(470.83) = 6.62, p < 0.001, Hedge's g = 0.54].
Study 2: Method
Participants, Materials, and Procedure
A predominantly student population was recruited with 498 complete survey responses (95% completion rate). A further 36 participants incorrectly answered the validity question (see Study 1 Method) and were deleted from further analyses. Administration of the questionnaire was identical to Study 1 with participants answering demographic questions and then the draft item pool. From these items the 15 items selected in Study 1 for the SCRAM questionnaire were screened for univariate outliers with 32 participants deleted across the scales to leave a final sample of 430 for the CFA (see Table 1 for sample characteristics).
Data Analyses
Using Mplus version 7.11 (Muthén and Muthén, 2013), a CFA with a maximum likelihood estimation was used to investigate the three-factor model found in Study 1. Multiple methods were used to assess model fit (Hu and Bentler, 1999) including: the chi square test of model fit (divided by the degrees of freedom given the sensitivity to sample size), root mean square error of approximation (RMSEA), standardized root mean square residual (SRMR), comparative goodness-of-fit index (CFI), and Tucker-Lewis index (TLI). The chi square statistic divided by the degrees of freedom should be less than 3 for an acceptable fit (Schermelleh-Engel et al., 2003), RMSEA scores less than 0.05 reflect a good fit, scores less than 0.08 an adequate model fit; and if the upper limit of the 90% confidence interval is 0.08 this adds additional support of model adequacy (Browne and Cudeck, 1992). SRMR scores should be below 0.08 (Hu and Bentler, 1999; Brown, 2015). CFI and TLI values should preferably be above 0.95, however indices in the range of 0.90–0.95 are acceptable (Hu and Bentler, 1999).
Study 2: Results
The results of the CFA are displayed in Figure 2.
The analyses indicated the following fit indices: χ2/df = 2.93, CFI = 0.93, TLI = 0.92, RMSEA = 0.067 (90% CI [0.058,0.077]), and SRMR = 0.052. Given the reference criteria, model fit was acceptable across all fit indices. As displayed in Figure 2, the resultant model supported the latent structure of the SCRAM as scored. In each case, the five items of the SCRAM scale loaded significantly on a single latent variable. The Morningness scale had a moderate positive relationship to Good Sleep (r = 0.38) and a weak negative association with Depression (r = −0.24); Good Sleep and Depression had a strong negative relationship (r = −0.65).
General Discussion
The aim of this project was to develop the first self-report instrument to reliably measure individual differences in sleep quality, circadian phase, and mood. Three distinct but correlated latent factors of Depressed Mood, Good Sleep and Morningness were identified using exploratory factor analyses. Data-driven factor structures supported the theoretically-based three-factor solution, and systematic item reduction led to the 15-item SCRAM questionnaire—a face-valid instrument maximally separating the measurement of sleep quality, circadian phase and mood. The three-factor latent structure of the SCRAM was confirmed in a second large student sample.
Relationship between Morningness, Good Sleep and Depressed Mood Scales
Relationships amongst the three SCRAM scales were found to be congruent with existing literature. First, Study 1 and Study 2 data showed that Depressed Mood was correlated with lower levels of Good Sleep. Sleep disturbances affect mood (Pilcher and Huffcutt, 1996), and mood problems have been associated with lowered sleep quality through increased rumination and subsequent arousal at night (Schmidt et al., 2011). The negative correlation between Morningness and Depressed Mood aligns with data suggesting that evening-type individuals are more likely to experience depression, and report more severe depressive symptomatology (Abe et al., 2011; Chan et al., 2014). Morningness and Good Sleep had a moderate positive correlation, consistent with evidence that eveningness is associated with higher levels of insomnia complaints (Wittmann et al., 2006; Chan et al., 2014; Barclay et al., 2016). Consistent with some previous research (e.g., Roenneberg et al., 2003), Morningness on the SCRAM questionnaire was positively associated with older age and female gender (cf. Merikanto et al., 2012, for evidence that men may have higher levels of morningness relative to women).
In a preliminary assessment of convergent and divergent validity (Study 1), the SCRAM scales showed interpretable relationships to self-reported medical, sleep and psychiatric diagnoses. Previous or current mental illness were associated with lower scores on Morningness and Good Sleep scales, and higher scores on the Depressed Mood scale, consistent with evidence for sleep and circadian rhythm disturbances in a range of mental health disorders, (e.g., Harvey et al., 2011; Gershon et al., 2012, 2015; Pritchett et al., 2012; Soehner et al., 2016; Wong et al., 2016). Self-report of mental illness was associated with higher levels of Depressed Mood; aligning with previous data showing mood complaints to be common in mood and anxiety disorders, and schizophrenia (Levinson et al., 1999; Ohayon and Roth, 2003; Taylor et al., 2005; Pritchett et al., 2012). Finally, consistent with links between physical illnesses and biological rhythm function (e.g., Katon, 2003; Ancoli-Israel et al., 2006; Musiek et al., 2015; Grimaldi et al., 2016; Haus et al., 2016), self-reported physical illness was associated lower scores on the Good Sleep and Morningness scales.
The potential clinical significance of distinguishing between sleep quality, circadian phase, and mood is underscored by the SCRAM scores of participants who self-reported sleeping problems in Study 1. Self-reported sleep problems, not surprisingly, were associated with lower scores on the SCRAM Good Sleep scale but were also associated with higher scores on the SCRAM Depressed Mood scale. This is consistent with sleep disturbance being part of the diagnostic constellation of mood disorders in the DSM-5 (American Psychiatric Association, 2013) and higher negative mood levels observed following sleep disturbance in healthy individuals (Tempesta et al., 2015; Raniti et al., 2016). Self-reported sleep problems were also associated with lower scores on the SCRAM Morningness scale, consistent with an association between morningness and better sleep quality (e.g., Wittmann et al., 2006). The significance of this is that when a patient presents with sleep complaints mood problems and circadian phase alignment are critical in both the remittance of sleep problems and maintenance of sleep quality (Harvey et al., 2005; Carney et al., 2009; Crowe et al., 2016). Sleep quality may be effectively treated but if the patient continues to have a delayed sleep cycle or depressed mood these may serve as independent risk factors for future problems with sleep quality, circadian phase, and/or mood.
The SCRAM Questionnaire as a Clinical and Research Tool
With additional validation (see below) the SCRAM questionnaire could improve clinical assessment of symptomatology across the three domains. By distinguishing these three strongly interrelated processes, the SCRAM questionnaire may also be a useful research tool. Malhi and Kuiper (2013) highlight that the substrates of sleep quality, circadian phase, and mood are likely to have different configurations and relationships across psychopathologies. The well-recognized biobehavioral interplay between sleep, circadian function and mood may speak to an under-appreciated superordinate construct or biobehavioral process that could be measured by the SCRAM questionnaire: Murray and colleagues have proposed the Circadian Reward Rhythm as a fundamental motivational process with circadian, sleep and mood manifestations (Murray et al., 2009; see also Alloy et al., 2017).
Limitations
A number of limitations should be noted. The samples of both studies were predominantly women (82 and 79% for Study 1 and 2 respectively) and students (94 and 98%), so findings may not generalize to the general population. Test-retest reliability was not investigated here, and the SCRAM's sensitivity to change in clinical settings is unknown.
Conclusions
The present project is a necessary first step in an ongoing program of research. Here, we have developed a psychometrically sound, face-valid brief measure that separates three intrinsically correlated processes for measurement/assessment purposes—the SCRAM questionnaire. To confirm the expected clinical utility of the instrument, more research is required. A critical next phase is to develop SCRAM profiles (pre- and post-treatment) for clinical samples with primary presenting problems in the sleep, circadian phase or mood domains. Subsequently our core prediction—that use of such profiles to drive treatment decisions is superior to current practice based on single-construct instruments—can be tested. With further investigation, the SCRAM questionnaire holds promise as a tool for assessing clinically-significant patterns of disturbance across these three processes.
Ethics Statement
This study was given ethics approval by the Swinburne Human Research Ethics Committee. Participants completed an online survey with completion of the survey implying consent, as detailed in the information statement at the beginning of the survey.
Author Contributions
JB had the original idea and contributed to the study design, collected the data, undertook the statistical analyses, and wrote the first draft of the manuscript. GM and BB contributed to the study design and drafting of the manuscript. All authors contributed to and have approved the final manuscript.
Funding
The study was funded by a Barbara Dicker Brain Sciences Foundation grant. The funding agency had no further role in study design, in the collection, analysis, and interpretation of data, in the writing of the report, and in the decision to submit the paper for publication.
Conflict of Interest Statement
The authors declare that the research was conducted in the absence of any commercial or financial relationships that could be construed as a potential conflict of interest.
Supplementary Material
The Supplementary Material for this article can be found online at: https://www.frontiersin.org/articles/10.3389/fpsyg.2017.02105/full#supplementary-material
References
Abe, T., Inoue, Y., Komada, Y., Nakamura, M., Asaoka, S., Kanno, M., et al. (2011). Relation between morningness–eveningness score and depressive symptoms among patients with delayed sleep phase syndrome. Sleep Med. 12, 680–684. doi: 10.1016/j.sleep.2010.12.017
Alloy, L. B., Ng, T. H., Titone, M. K., and Boland, E. M. (2017). Circadian rhythm dysregulation in bipolar spectrum disorders. Curr. Psychiatry Rep. 19, 21. doi: 10.1007/s11920-017-0772-z
American Psychiatric Association (2013). Diagnostic and Statistical Manual of Mental Disorders. Washington, DC: American Psychiatric Association.
Ancoli-Israel, S., Liu, L., Marler, M. R., Parker, B. A., Jones, V., Sadler, G. R., et al. (2006). Fatigue, sleep, and circadian rhythms prior to chemotherapy for breast cancer. Support. Care Cancer 14, 201–209. doi: 10.1007/s00520-005-0861-0
Baehr, E. K., Revelle, W., and Eastman, C. I. (2000). Individual differences in the phase and amplitude of the human circadian temperature rhythm: with an emphasis on morningness–eveningness. J. Sleep Res. 9, 117–127. doi: 10.1046/j.1365-2869.2000.00196.x
Barclay, N. L., Rowe, R., O'Leary, R., Bream, D., and Gregory, A. M. (2016). Longitudinal stability of genetic and environmental influences on the association between diurnal preference and sleep quality in young adult twins and siblings. J. Biol. Rhythms. 31, 375–386. doi: 10.1177/0748730416653533
Bastien, C. H., Vallières, A., and Morin, C. M. (2001). Validation of the insomnia severity Index as an outcome measure for insomnia research. Sleep Med. 2, 297–307. doi: 10.1016/S1389-9457(00)00065-4
Beauchaine, T. P., and Thayer, J. F. (2015). Heart rate variability as a transdiagnostic biomarker of psychopathology. Int. J. Psychophysiol. 98, 338–350. doi: 10.1016/j.ijpsycho.2015.08.004
Beck, A. T., Steer, R. A., and Brown, G. K. (1996). Beck Depression Inventory-II. San Antonio, TX: Psychological Corporation, b9.
Brown, T. A. (2015). Confirmatory Factor Analysis for Applied Research. New York, NY: Guilford Publications.
Browne, M. W., and Cudeck, R. (1992). Alternative ways of assessing model fit. Sociol. Methods Res. 21, 230–258. doi: 10.1177/0049124192021002005
Buysse, D. J., Reynolds, Iii, C. F., Monk, T. H., Berman, S. R., and Kupfer, D. J. (1989). The pittsburgh sleep quality index: a new instrument for psychiatric practice and research. Psychiatr. Res. 28, 193–213. doi: 10.1016/0165-1781(89)90047-4
Carney, C. E., Ulmer, C., Edinger, J. D., Krystal, A. D., and Knauss, F. (2009). Assessing depression symptoms in those with insomnia: an examination of the beck depression inventory second edition (BDI-II). J. Psychiatr. Res. 43, 576–582. doi: 10.1016/j.jpsychires.2008.09.002
Cella, D., Yount, S., Rothrock, N., Gershon, R., Cook, K., Reeve, B., et al. (2007). The patient-reported outcomes measurement information system (PROMIS): progress of an NIH roadmap cooperative group during its first two years. Med. Care 45, S3–S11. doi: 10.1097/01.mlr.0000258615.42478.55
Chan, J. W., Lam, S. P., Li, S. X., Yu, M., Chan, N. Y., Zhang, J., et al. (2014). Eveningness and insomnia: independent risk factors of nonremission in major depressive disorder. Sleep 37, 911–917. doi: 10.5665/sleep.3658
Chang, L. (1994). A psychometric evaluation of 4-point and 6-point Likert-type scales in relation to reliability and validity. Appl. Psychol. Meas. 18, 205–215. doi: 10.1177/014662169401800302
Costello, A. B., and Osborne, J. W. (2005). Best practices in exploratory factor analysis: four recommendations for getting the most from your analysis. Pract. Asses. Res. Eval. 10, 1–9. Available online at: http://pareonline.net/getvn.asp?v=10&n=7
Crowe, M., Beaglehole, B., and Inder, M. (2016). Social rhythm interventions for bipolar disorder: a systematic review and rationale for practice. J. Psychiatr. Ment. Health Nurs. 23, 3–11. doi: 10.1111/jpm.12271
Czeisler, C. A., Kronauer, R. E., Allan, J. S., Duffy, J. F., Jewett, M. E., Brown, E. N., et al. (1989). Bright light induction of strong (type 0) resetting of the human circadian pacemaker. Science 244, 1328–1333. doi: 10.1126/science.2734611
Duffy, J. F., Dijk, D. J., Hall, E. F., and Czeisler, C. A. (1999). Relationship of endogenous circadian melatonin and temperature rhythms to self-reported preference for morning or evening activity in young and older people. J. Investig. Med. 47, 141–150.
Duffy, J. F., Rimmer, D. W., and Czeisler, C. A. (2001). Association of intrinsic circadian period with morningness–eveningness, usual wake time, and circadian phase. Behav. Neurosci. 115, 895–899. doi: 10.1037/0735-7044.115.4.895
Gershon, A., Ram, N., Johnson, S. L., Harvey, A. G., and Zeitzer, J. M. (2015). Daily actigraphy profiles distinguish depressive and interepisode states in bipolar disorder. Clin. Psychol. Sci. 4, 641–650. doi: 10.1177/2167702615604613
Gershon, A., Thompson, W. K., Eidelman, P., Mcglinchey, E. L., Kaplan, K. A., and Harvey, A. G. (2012). Restless pillow, ruffled mind: sleep and affect coupling in interepisode bipolar disorder. J. Abnorm. Psychol. 121, 863–873. doi: 10.1037/a0028233
Grandner, M. A., Kripke, D. F., Yoon, I.-Y., and Youngstedt, S. D. (2006). Criterion validity of the pittsburgh sleep quality index: investigation in a non-clinical sample. Sleep Biol. Rhythms 4, 129–136. doi: 10.1111/j.1479-8425.2006.00207.x
Grimaldi, D., Carter, J. R., Van Cauter, E., and Leproult, R. (2016). Adverse impact of sleep restriction and circadian misalignment on autonomic function in healthy young adults. Hypertension 68, 243–250. doi: 10.1161/HYPERTENSIONAHA.115.06847
Gruber, J., Mennin, D. S., Fields, A., Purcell, A., and Murray, G. (2015). Heart rate variability as a potential indicator of positive valence system disturbance: a proof of concept investigation. Int. J. Psychophysiol. 98, 240–248. doi: 10.1016/j.ijpsycho.2015.08.005
Gruber, J., Miklowitz, D. J., Harvey, A. G., Frank, E., Kupfer, D., Thase, M. E., et al. (2011). Sleep matters: sleep functioning and course of illness in bipolar disorder. J. Affect. Disord. 134, 416–420. doi: 10.1016/j.jad.2011.05.016
Harvey, A. G. (2015). A transdiagnostic intervention for youth sleep and circadian problems. Cogn. Behav. Pract. 23, 341–355. doi: 10.1016/j.cbpra.2015.06.001
Harvey, A. G., Murray, G., Chandler, R. A., and Soehner, A. (2011). Sleep disturbance as transdiagnostic: consideration of neurobiological mechanisms. Clin. Psychol. Rev. 31, 225–235. doi: 10.1016/j.cpr.2010.04.003
Harvey, A. G., Schmidt, D. A., Scarnà, A., Semler, C. N., and Goodwin, G. M. (2005). Sleep-related functioning in euthymic patients with bipolar disorder, patients with insomnia, and subjects without sleep problems. Am. J. Psychiatr. 162, 50–57. doi: 10.1176/appi.ajp.162.1.50
Haus, E., Reinberg, A., Mauvieux, B., Le Floc'h, N., Sackett-Lundeen, L., and Touitou, Y. (2016). Risk of obesity in male shift workers: a chronophysiological approach. Chronobiol. Int. 33, 1–19. doi: 10.3109/07420528.2016.1167079
Horn, J. L. (1965). A rationale and test for the number of factors in factor analysis. Psychometrika 30, 179–185. doi: 10.1007/BF02289447
Horne, J. A., and Östberg, O. (1976). A self-assessment questionnaire to determine morningness-eveningness in human circadian rhythms. Int. J. Chronobiol. 4, 97–110.
Hu, L. T., and Bentler, P. M. (1999). Cutoff criteria for fit indexes in covariance structure analysis: Conventional criteria vs. new alternatives. Struct. Equ. Model. Multidiscipl. J. 6, 1–55. doi: 10.1080/10705519909540118
Katon, W. J. (2003). Clinical and health services relationships between major depression, depressive symptoms, and general medical illness. Biol. Psychiatr. 54, 216–226. doi: 10.1016/S0006-3223(03)00273-7
Kemp, A. H., and Quintana, D. S. (2013). The relationship between mental and physical health: insights from the study of heart rate variability. Int. J. Psychophysiol. 89, 288–296. doi: 10.1016/j.ijpsycho.2013.06.018
Kripke, D. F., Elliott, J. A., Youngstedt, S. D., and Rex, K. M. (2007). Circadian phase response curves to light in older and young women and men. J. Circadian Rhythms 5, 1–13. doi: 10.1186/1740-3391-5-4
Levinson, D. F., Umapathy, C., and Musthaq, M. (1999). Treatment of schizoaffective disorder and schizophrenia with mood symptoms. Am. J. Psychiatr. 156, 1138–1148.
Malhi, G. S., and Kuiper, S. (2013). Chronobiology of mood disorders. Acta Psychiatr. Scand. 128, 2–15. doi: 10.1111/acps.12173
Manber, R., Edinger, J. D., Gress, J. L., San Pedro-Salcedo, M. G., Kuo, T. F., and Kalista, T. (2008). Cognitive behavioral therapy for insomnia enhances depression outcome in patients with comorbid major depressive disorder and insomnia. Sleep 31:489. doi: 10.1093/sleep/31.4.489
Mason, E. C., and Harvey, A. G. (2014). Insomnia before and after treatment for anxiety and depression. J. Affect. Disord. 168, 415–421. doi: 10.1016/j.jad.2014.07.020
McClung, C. A. (2013). How might circadian rhythms control mood? let me count the ways. Biol. Psychiatr. 74, 242–249. doi: 10.1016/j.biopsych.2013.02.019
Merikanto, I., Kronholm, E., Peltonen, M., Laatikainen, T., Lahti, T., and Partonen, T. (2012). Relation of chronotype to sleep complaints in the general finnish population. Chronobiol. Int. 29, 311–317. doi: 10.3109/07420528.2012.655870
Mongrain, V., Lavoie, S., Selmaoui, B., Paquet, J., and Dumont, M. (2004). Phase relationships between sleep-wake cycle and underlying circadian rhythms in morningness-eveningness. J. Biol. Rhythms 19, 248–257. doi: 10.1177/0748730404264365
Morin, L. P. (2013). Neuroanatomy of the extended circadian rhythm system. Exp. Neurol. 243, 4–20. doi: 10.1016/j.expneurol.2012.06.026
Murray, G., Nicholas, C. L., Kleiman, J., Dwyer, R., Carrington, M. J., Allen, N. B., et al. (2009). Nature's clocks and human mood: the circadian system modulates reward motivation. Emotion 9, 705–716. doi: 10.1037/a0017080
Musiek, E. S., Xiong, D. D., and Holtzman, D. M. (2015). Sleep, circadian rhythms, and the pathogenesis of Alzheimer disease. Exp. Mol. Med. 47, e148. doi: 10.1038/emm.2014.121
Nowlis, S. M., Kahn, B. E., and Dhar, R. (2002). Coping with ambivalence: the effect of removing a neutral option on consumer attitude and preference judgments. J. Consum. Res. 29, 319–334. doi: 10.1086/344431
O'Connor, B. P. (2000). SPSS and SAS programs for determining the number of components using parallel analysis and Velicer's MAP test. Behav. Res. Methods Instrum. Comp. 32, 396–402. doi: 10.3758/BF03200807
Ohayon, M. M., and Roth, T. (2003). Place of chronic insomnia in the course of depressive and anxiety disorders. J. Psychiatr. Res. 37, 9–15. doi: 10.1016/S0022-3956(02)00052-3
Pilcher, J. J., and Huffcutt, A. I. (1996). Effects of sleep deprivation on performance: a meta-analysis. Sleep 19, 318–326. doi: 10.1093/sleep/19.4.318
Preston, C. C., and Colman, A. M. (2000). Optimal number of response categories in rating scales: reliability, validity, discriminating power, and respondent preferences. Acta Psychol. 104, 1–15. doi: 10.1016/S0001-6918(99)00050-5
Pritchett, D., Wulff, K., Oliver, P. L., Bannerman, D. M., Davies, K. E., Harrison, P. J., et al. (2012). Evaluating the links between schizophrenia and sleep and circadian rhythm disruption. J. Neural Transm. 119, 1061–1075. doi: 10.1007/s00702-012-0817-8
Radloff, L. S. (1977). The CES-D scale: a self-report depression scale for research in the general population. Appl. Psychol. Meas. 1, 385–401. doi: 10.1177/014662167700100306
Rajaratnam, S. M., and Arendt, J. (2001). Health in a 24-h society. Lancet 358, 999–1005. doi: 10.1016/S0140-6736(01)06108-6
Raniti, M. B., Allen, N. B., Schwartz, O., Waloszek, J. M., Byrne, M. L., Woods, M. J., et al. (2016). Sleep duration and sleep quality: associations with depressive symptoms across adolescence. Behav. Sleep Med. 15, 198–215. doi: 10.1080/15402002.2015.1120198
Robillard, R., Naismith, S. L., Rogers, N. L., Ip, T. K. C., Hermens, D. F., Scott, E. M., et al. (2013). Delayed sleep phase in young people with unipolar or bipolar affective disorders. J. Affect. Disord. 145, 260–263. doi: 10.1016/j.jad.2012.06.006
Roenneberg, T., Wirz-Justice, A., and Merrow, M. (2003). Life between clocks: daily temporal patterns of human chronotypes. J. Biol. Rhythms 18, 80–90. doi: 10.1177/0748730402239679
Schermelleh-Engel, K., Moosbrugger, H., and Müller, H. (2003). Evaluating the fit of structural equation models: tests of significance and descriptive goodness-of-fit measures. Methods Psychol. Res. Online 8, 23–74.
Schmidt, R. E., Harvey, A. G., and Van der Linden, M. (2011). Cognitive and affective control in insomnia. Front. Psychol. 2:349. doi: 10.3389/fpsyg.2011.00349
Soehner, A. M., Bertocci, M. A., Manelis, A., Bebko, G., Ladouceur, C. D., Graur, S., et al. (2016). Preliminary investigation of the relationships between sleep duration, reward circuitry function, and mood dysregulation in youth offspring of parents with bipolar disorder. J. Affect. Disord. 205, 144–153. doi: 10.1016/j.jad.2016.03.074
Soehner, A. M., Kaplan, K. A., and Harvey, A. G. (2014). Prevalence and clinical correlates of co-occurring insomnia and hypersomnia symptoms in depression. J. Affect. Disord. 167, 93–97. doi: 10.1016/j.jad.2014.05.060
Taylor, D. J., Lichstein, K. L., Durrence, H. H., Reidel, B. W., and Bush, A. J. (2005). Epidemiology of insomnia, depression, and anxiety. Sleep 28, 1457–1464. doi: 10.1093/sleep/28.11.1457
Tempesta, D., De Gennaro, L., Natale, V., and Ferrara, M. (2015). Emotional memory processing is influenced by sleep quality. Sleep Med. 16, 862–870. doi: 10.1016/j.sleep.2015.01.024
Velicer, W. F. (1976). Determining the number of components from the matrix of partial correlations. Psychometrika 41, 321–327. doi: 10.1007/BF02293557
Wittmann, M., Dinich, J., Merrow, M., and Roenneberg, T. (2006). Social jetlag: misalignment of biological and social time. Chronobiol. Int. 23, 497–509. doi: 10.1080/07420520500545979
Wong, M. M., Brower, K. J., and Craun, E. A. (2016). Insomnia symptoms and suicidality in the national comorbidity survey – adolescent supplement. J. Psychiatr. Res. 81, 1–8. doi: 10.1016/j.jpsychires.2016.06.004
Zigmond, A. S., and Snaith, R. P. (1983). The hospital anxiety and depression scale. Acta Psychiatr. Scand. 67, 361–370. doi: 10.1111/j.1600-0447.1983.tb09716.x
Appendix
SCRAM Questionnaire
Instructions
Keywords: sleep, circadian phase, mood, depression, measure, questionnaire
Citation: Byrne JEM, Bullock B and Murray G (2017) Development of a Measure of Sleep, Circadian Rhythms, and Mood: The SCRAM Questionnaire. Front. Psychol. 8:2105. doi: 10.3389/fpsyg.2017.02105
Received: 18 August 2017; Accepted: 17 November 2017;
Published: 01 December 2017.
Edited by:
Nuno Conceicao, Universidade de Lisboa, PortugalReviewed by:
Timo Partonen, National Institute for Health and Welfare, FinlandTanja Legenbauer, Ruhr University Bochum, Germany
Copyright © 2017 Byrne, Bullock and Murray. This is an open-access article distributed under the terms of the Creative Commons Attribution License (CC BY). The use, distribution or reproduction in other forums is permitted, provided the original author(s) or licensor are credited and that the original publication in this journal is cited, in accordance with accepted academic practice. No use, distribution or reproduction is permitted which does not comply with these terms.
*Correspondence: Jamie E. M. Byrne, amFtaWVieXJuZUBzd2luLmVkdS5hdQ==