- Department of Psychology and Sports Sciences, Institute of Psychology, Westfälische Wilhelms-Universität, Münster, Germany
Background: The vascular depression hypothesis emphasizes the significance of vascular lesions in late-life depression. At present, no meta-analytic model has investigated whether a difference in hyperintensity burden compared to controls between late-life and late-onset depression is evident. By including a substantial number of studies, focusing on a meaningful outcome measure, and considering several moderating and control variables, the present meta-analysis investigates the severity of hyperintensity burden in major depressive disorder (MDD) and bipolar disorder (BD). A major focus of the present meta-analysis refers to the role of age at illness onset. It is analyzed whether late-onset rather than late-life depression characterizes vascular depression.
Method: In total, 68 studies were included in the meta-analysis and a multilevel random effects model was calculated using Hedges' g as the effect size measure.
Results: The severity of hyperintensity burden was significantly greater in the patient group compared to the control group. This effect was evident regarding the whole patient group (g = 0.229) as well as both depression subgroups, with a significantly greater effect in BD (g = 0.374) compared to MDD (g = 0.189). Hyperintensity burden was more pronounced in late-onset depression than in early-onset depression or late-life depression. A considerable heterogeneity between the included studies was observed, which is reflected by the large variability in effects sizes.
Conclusion: In conclusion, the present meta-analysis underscores the association of hyperintensities with MDD and BD. Especially late-onset depression is associated with an increased hyperintensity burden, which is in line with the vascular depression hypothesis. The results suggest that it might be more feasible to confine the concept of vascular depression specifically to late-onset depression as opposed to late-life depression. Further research is needed to understand the causal mechanisms that might underlie the relation between hyperintensity burden and depression.
Introduction
Rationale
Major depressive disorder (MDD) and bipolar disorder (BD) have shown to be associated with hyperintense lesions in various MRI studies. Research in this area focuses predominantly on white matter hyperintensities, especially in later life (van Agtmaal et al., 2017). However, these lesions occur in both gray and white matter (Greenwald et al., 1996, 2001; Steffens et al., 2001; Beyer et al., 2009). There is a multitude of studies examining the relationship between hyperintensities and unipolar and bipolar depression, but findings reveal considerable inconsistencies with regard to whether this association exists at all (Lee et al., 2003; Sassi et al., 2003; Dalby et al., 2010), and whether it depends on lesion location (Krishnan et al., 2006). Furthermore, meta-analytic reviews do not consistently coincide and in several cases relevant moderators, e.g., lesion location or age, are not taken into account. To date, no meta-analytic model has investigated whether there is a difference in hyperintensity burden compared to controls between late-life and late-onset depression. In terms of the outcome measure, the majority of prior meta-analyses have focused on prevalence rates rather than severity of hyperintensities. In the case of BD, there is no meta-analysis that has investigated the difference in the severity of hyperintensity burden in comparison to healthy controls. Thus, the goal of the present work was to provide a meta-analytic update on the association of hyperintensities with MDD and BD by including a comprehensive number of studies, elucidating the role of age at illness onset and lesion location, and defining the severity of hyperintensities as an outcome measure.
Hyperintense Lesions
Hyperintensities are assumed to reflect silent lesions of vascular origin in the brain (Farkas et al., 2006). Their relaxation properties in magnetic resonance imaging (MRI) make hyperintensities appearing as particularly bright signals, i.e., areas of increased signal intensity, on T2-weighted MRI images (Pantoni and Garcia, 1997). These lesions are not restricted to pathologic conditions affecting the central nervous system such as Alzheimer's disease, Parkinson's disease, or small vessel disease (Radanovic et al., 2013; Compta et al., 2016; Foo et al., 2016; Li et al., 2016). It is a phenomenon that is frequently observed in aging (Hendrie et al., 1989; Breteler et al., 1994; Gattringer et al., 2012). The prevalence rates reported by several studies vary, which may be due to different MRI procedures or rating scales (Mäntylä et al., 1997), but altogether hyperintensities can be considered as a rather common phenomenon in the elderly. For example, a population-based study by de Leeuw et al. (2012) reported that only 5% of the study sample aged between 60 and 90 were free of any hyperintense white matter lesions. However, the clinical relevance of hyperintense lesions should not be underestimated. Particularly, white matter hyperintensities (WMH) are frequently associated with cognitive deficits in different domains. As demonstrated by the meta-analysis by Kloppenborg et al. (2014) the presence of white matter hyperintensities is related to cognitive deficits regarding general intelligence, memory, processing speed, attention, and executive functions. Moreover, they found that WMH progression was associated with greater cognitive decline over time in executive functions, attention, and general intelligence.
The origin of hyperintense lesions may vary across conditions, and the exact causes remain to be conclusively determined. However, the prevalence of hyperintensities increases in later life, which can be explained by the fact that old age is more commonly associated with various changes in the vascular system both on structural and functional levels (Marín, 1995; Kovacic et al., 2011), and considering that vascular or ischemic pathology is assumed to account for the majority of hyperintensities (Pantoni and Garcia, 1997; Bakker et al., 1999; Farkas et al., 2006). Furthermore, diseases with vascular impact such as hypertension and diabetes mellitus increase with age (Kearney et al., 2005; Shaw et al., 2010) and are associated with hyperintensities (Longstreth et al., 1996; Habes et al., 2016). Post-mortem studies reveal a variety of etiologic patterns for hyperintensities. Findings most often reported as being associated with hyperintense lesions include myelin rarefaction, arteriosclerosis, dilated perivascular spaces, vascular ectasia, ependymal loss, and cerebral ischemia (Awad et al., 1986; Kirkpatrick and Hayman, 1987; Fazekas et al., 1991; Scheltens et al., 1995). Similar relations were found in studies investigating the neuropathological substrates of hyperintensities in depressed subjects (Thomas et al., 2002, 2003). While there is evidence for WMH as preceding depression or depressive symptoms (Teodorczuk et al., 2007, 2012; Firbank et al., 2012; Qiu et al., 2017), other studies did not find such a pattern (Versluis et al., 2006; Dotson et al., 2013). Therefore, a potential causal relation between hyperintensities and depression remains unclear.
The Vascular Depression Hypothesis
Diseases involving cerebrovascular symptomatology are assumed to function as a predisposing, triggering or perpetuating factor for some depressive syndromes in the elderly (Alexopoulos et al., 1997). The vascular depression hypothesis is based on the association between depression and vascular pathology or vascular risk factors and their behavioral correlates. Vascular compared to non-vascular depression can be assumed to be associated, among others, with old age and old age at illness onset (Krishnan et al., 1997). More recently, Krishnan et al. (2004) introduced the term subcortical ischemic depression (SID) to describe vascular-related depression. The authors identified SID on the basis of deep white matter hyperintensity (DWMH) and subcortical gray matter hyperintensity (SCGMH) ratings. They found that age, lassitude, and a history of hypertension were associated with SID. A study investigating the internal validity of the vascular depression concept identified DWMH burden as the most specific and sensitive factor for distinguishing vascular from non-vascular late-life depression (Sneed et al., 2008). Further evidence regarding the external validity of vascular depression is established by studies which found that the vascular subtype is associated with a more severe psychomotor retardation (Pimontel et al., 2013) and lower response rates to antidepressant medication (Sneed et al., 2011) than the non-vascular subtype.
Objectives
The present meta-analysis aims to increase insight into the association of hyperintensities and depression mainly in view of two key aspects. Firstly, relevant moderators were examined, which is especially crucial with regard to age at illness onset, lesion location and investigating MDD as well as BD. The second key aspect drew attention to the severity (as opposed to the dichotomously defined presence vs. non-presence, i.e., frequency) of hyperintensities. The rationale for why these issues are emphasized is described below. In addition, several methodological aspects were considered during different research stages (i.e., exclusion criteria, potential confounders, publication bias), which have not consistently been applied by previous meta-analytic studies regarding this topic.
Notably, though age at illness onset has been shown to moderate the association between hyperintensities and depression disorders in several studies (Lesser et al., 1996; Lloyd et al., 2004; Tamashiro et al., 2008; Delaloye et al., 2010), in current meta-analytic research on late-life depression its moderating role is not consistently adhered to. A categorical distinction can be made between early-onset and late-onset depression according to the age at illness onset. Late-life depression as such which geriatric depression is commonly referred to can comprise both an early and a late illness onset. Cut-offs to define late-life depression or to differentiate between an early and a late illness onset usually vary from 50 to 65 years (Aizenstein et al., 2016). Alexopoulos et al. (1997) proposed the vascular depression hypothesis which is related to late-life depressive syndromes. While it focuses on late-life depression, it explicitly includes early-onset depression in later life (Taylor et al., 2013a). To investigate if late-onset rather than late-life depression might be more effective to determine vascular depression, the present meta-analysis defined late-onset depression as a distinct category in addition to late-life depression. This was done to account for the crucial role of age at illness onset, which the commonly used categorization of late-life depression does not take into consideration. Moreover, two depression types, unipolar and bipolar, were investigated. In the case of BD, the most recent meta-analysis is from 2009 (Beyer et al., 2009), which makes a meta-analytical update crucial. A further emphasis was placed on lesion location in terms of a possible moderator, as previous meta-analytic results show inconsistencies with regard to the association of hyperintensities with MDD or BD in different lesion locations.
The second major characteristic of the present meta-analysis is its focus on the severity of hyperintensities instead of the frequency of hyperintensities (i.e., dichotomous categorization as present or not). This is particularly relevant, since several studies found that an increase in the severity of white matter hyperintensities (WMH) is associated with more pronounced cognitive impairment (de Groot et al., 2001; Murata et al., 2001; Chen Y. F. et al., 2006). Therefore, the severity of hyperintensity burden seems to be of clinical significance with respect to depressive symptomatology. In addition, hyperintensities are frequently observed in aging irrespective of pathological conditions (Hendrie et al., 1989; Breteler et al., 1994; Gattringer et al., 2012). In this regard, comparing the severity rather than the frequency of hyperintensities between depressed patients and healthy controls seems more comprehensive.
With respect to methodological issues, the present meta-analysis aims at extending previous findings by including a substantial number of studies, applying an appropriate statistical model—namely a multilevel random effects model to account for dependencies between outcomes and evaluating heterogeneity of the effect—and by conducting statistical analyses to control for several demographic and methodological differences between studies. Furthermore, in order to account for clinical validity, only studies that provide explicit diagnoses of MDD or BD according to DSM or ICD criteria are included.
Review of the Meta-Analytic State of Research
To date, nine meta-analyses have investigated the association of hyperintensities with MDD and BD (Altshuler et al., 1995; Videbech, 1997; Herrmann et al., 2008; Kempton et al., 2008, 2011; Beyer et al., 2009; Anorne et al., 2012; Wang et al., 2014; van Agtmaal et al., 2017). Of these meta-analyses, five studies investigated the association with MDD (Herrmann et al., 2008; Kempton et al., 2011; Anorne et al., 2012; Wang et al., 2014; van Agtmaal et al., 2017), three with BD (Altshuler et al., 1995; Kempton et al., 2008; Beyer et al., 2009), and one with MDD and BD separately (Videbech, 1997). These prior studies provide important results on the association between hyperintensities and depression and are a good starting point for further meta-analytic research.
Each meta-analysis renders some evidence in favor of increased hyperintensities in unipolar and bipolar depression. Investigating lesion location, Kempton et al. (2008) and Beyer et al. (2009) found a significantly higher prevalence of hyperintensities in BD subjects compared to control subjects regarding deep white matter hyperintensities (DWMH) but not periventricular hyperintensities (PVH). For subcortical gray matter hyperintensities (SCGMH), only the meta-analysis by Beyer et al. (2009) found an increased prevalence in BD. Altshuler et al. (1995) did not differentiate between lesion locations, but found a higher frequency of hyperintensities in BD subjects compared to controls. Wang et al. (2014) report that DWMH, but not PVH or overall WMH, were associated with MDD. Kempton et al. (2011), on the contrary, found a significant association of MDD with PVH but not with DWMH. Focusing on late-life MDD, Herrmann et al. (2008) report significantly increased PVH and DWMH. van Agtmaal et al. (2017) demonstrated that overall WMH were more frequent in late-life depression than in controls. The meta-analysis by Anorne et al. (2012) reports an increased volume of WMH in MDD patients compared to controls. Videbech (1997) reports a higher risk for hyperintensities in both MDD and BD not specifying lesion location. In summary, in the case of BD, meta-analyses agree on a higher prevalence of DWMH compared to controls while no effect was observed regarding PVH. For SCGMH, meta-analyses do not coincide. In the case of MDD, results are diverging with respect to DWMH and PVH. The prevalence of SCGMH in MDD was only investigated by Kempton et al. (2011) who report a higher frequency of MDD patients with SCGMH than controls.
Importantly, with regard to examining the difference in the severity of hyperintensity burden between BD patients and controls (instead of the frequency), there is no meta-analytic research yet. For MDD, the severity of hyperintensity burden was investigated in three prior meta-analyses (Herrmann et al., 2008; Kempton et al., 2011; Anorne et al., 2012) but the number of studies included in the analyses is limited. The meta-analysis by Anorne et al. (2012) comprises four studies and the meta-analysis by Kempton et al. (2011) nine. In the meta-analysis by Herrmann et al. (2008) 13 studies on late-life depression and five on late-onset depression were included. Thus, the severity of hyperintensity burden in BD patients compared to controls needs to be scrutinized in meta-analytic research. In the case of MDD, the hyperintensity severity in different onset subgroups needs further evaluation, as the meta-analysis by Herrmann et al. (2008) is the only study that investigated late-onset depression as opposed to late-life depression. Taking the year of publication into consideration as well as the total number of included studies, a meta-analytic update is essential.
With respect to methodological issues, the number of included studies generally ranges from four (Anorne et al., 2012) to 38 studies in the meta-analysis by van Agtmaal et al. (2017). Here, however, in the analysis of studies where an explicit MDD diagnosis was provided, only 16 studies were included (as opposed to defining depression as the mere presence of depressive symptoms assessed by questionnaires). Strikingly, a depression diagnosis was not necessarily part of the inclusion criteria of previous meta-analyses. For example, the meta-analysis conducted by Wang et al. (2014) investigated hyperintensities in patients with MDD diagnosis and subjects exhibiting depressive symptoms without distinguishing between these two conditions. Another aspect regarding inclusion criteria concerns the selectivity of the patient sample according to comorbidities. The meta-analysis by van Agtmaal et al. (2017), for instance, comprises patient samples selected according to specific disease factors (e.g., post-stroke depression). In such highly selected samples it cannot be excluded that the association between depression and hyperintensities is confounded with the effect of this underlying factor. Concerning statistical analysis, a random effects model seems reasonable and, in addition, a multilevel analysis would be appropriate to account for dependencies between outcomes within the same study or sample. This heterogeneity, however, was not accounted for in the meta-analyses by Altshuler et al. (1995), Beyer et al. (2009), and Videbech (1997), and none of the meta-analyses applied a multilevel model.
Taken together, the different meta-analyses elucidate important aspects of the association between hyperintensities and depression, for example, with regard to the role of different lesion locations. Further strengths of these meta-analyses refer, among others, to the careful handling of different outcome measures in the primary studies. That is, accounting for differences in lesion assessment, for example, by selectively excluding inappropriate outcomes or pooling primary studies according to methodological aspects (e.g., van Agtmaal et al., 2017). Therefore, these meta-analyses represent a meaningful starting point for a meta-analytic update on the association of hyperintensities with MDD and BD. Further meta-analytic investigation seems reasonable since prior meta-analyses differ in overall effect sizes and partly report contradicting results with respect to lesion location. Moreover, taking into account the heterogeneity between primary studies, several demographic and methodological variables should be controlled for to exclude confounding. Concerning moderating factors, investigating the role of late-onset depression as opposed to late-life depression might increase insight into the feasibility of the current conceptualization of vascular depression. In addition, the above delineated methodological issues might potentially limit the validity of previous findings, which can be resolved by a meta-analytic update.
Hypotheses
The severity of hyperintensity burden in MDD and BD compared to healthy controls has been explored. Possible confounders and moderators of this association have been derived from the research outlined above and from methodological considerations. Age at illness onset defined by group (early-onset vs. late-onset) has been assumed to act as a moderating variable not only in MDD (Herrmann et al., 2008), but also in BD (Tamashiro et al., 2008). Further, the role of lesion location has been examined. There is evidence for hyperintensities to occur in the deep white matter (DWMH), in periventricular areas (PVH) and in subcortical gray matter structures (SCGMH) in both MDD and BD (Greenwald et al., 1996; McDonald et al., 1999; Beyer et al., 2009). Thus, the hypotheses drawn from considerations on the current state of research were as follows:
1. Hyperintensity burden is higher in subjects with depression (MDD and BD) than in healthy control subjects. This association is evident in both MDD and BD and in different lesion locations (DWMH, PVH, SCGMH) in both disorders.
2. Late-onset depression is associated with a higher hyperintensity burden than early-onset depression in both MDD and BD.
Method
Search Strategy
The starting point for the present meta-analysis was an unpublished diploma thesis by Isabelle D. Wilden (Mokwa, 2013). To also include newer publications, a database literature search for studies published since January 2012 was conducted using the search term: (Leukoaraiosis OR Hyperintens* OR Lesion* OR Leukoencephalopath*) AND (Hypoman* OR Depress* OR Unipolar* OR Bipolar* OR Mania OR Manic OR Affectiv*) AND (mri OR “Magnetic Resonance Imaging”). The databases which were included in this search were: MEDLINE (PubMed), PsycINFO, Academic Search Premier, PsycARTICLES, PsycCritiques, PsycBOOKS, PSYNDEX, and ProQuest. A total of 1,393 titles were screened, and if regarded as relevant, abstracts were reviewed. Of all potentially relevant studies, full texts were examined. In addition, backward and forward search was conducted for 37 reviews including the prior meta-analyses. Database search was terminated in March 2017. Backward and forward search was completed in July 2017. Figure 1 displays the result of the search procedure. In total, 68 studies were included in the meta-analysis.
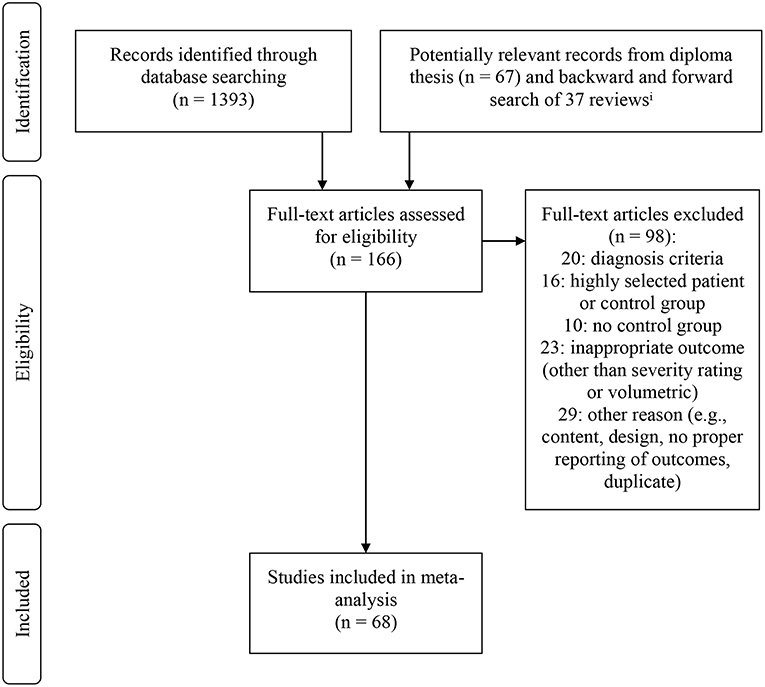
Figure 1. Flow chart displaying inclusion and exclusion of studies in meta-analysis. iAll identified titles were screened for relevance. If titles were regarded as relevant, abstracts were screened. If studies were regarded as potentially eligible on the basis of the abstract, full-text articles were retrieved.
Inclusion and Exclusion Criteria
The predefined inclusion criteria were as follows: Firstly, diagnosis of either MDD or BD must be established in the patient sample. Diagnosis must be made according to either Diagnostic and Statistical Manual of Mental Disorders (DSM) or International Classification of Diseases (World Health Organization, 1992), excluding classification systems older than DSM-III-R (American Psychiatric Association, 1987) to ensure clinical validity. The diagnosis criterion therefore excludes studies in which diagnosis is either based on self-report of prior MDD or BD diagnosis, based on self-report of having been in psychotherapeutic treatment due to depressive or manic symptomatology, based on the prescription of antidepressants or mood stabilizing medication, or based on cut-off scores of inventories assessing depressive or bipolar symptomatology. Secondly, the severity of hyperintensity burden must be assessed in the patient sample and in a healthy control group. A life-time history of affective disorders must be excluded in control subjects to avoid confounding. Thirdly, hyperintensity burden must be assessed via MRI in both groups. Lastly, the studies must be published in English.
Furthermore, studies were excluded if, firstly, the patient or control group was selected according to a specific disease factor (e.g., post-stroke depression and migraine) or otherwise highly selected (e.g., craniocerebral injury), and, secondly, if neurodegenerative or neuroinflammatory diseases (e.g., Alzheimer's disease and multiple sclerosis) were included in either the patient or control group since hyperintensities are common among these pathological conditions as well.
Coding Scheme
The coding scheme was developed on the basis of the current state of research (Data Sheet 1) and methodological considerations on possible confounders. The variables were assigned to four categories. The first category comprised variables that refer to the main effects. These were the variables disorder, hyperintensity burden, lesion location and illness onset defined by group (i.e., early-onset, late-onset, late-life, and not specified). The second category included several demographic control variables. These were age, sex (%), smoking (%), antidepressant medication (%), severity of depression, age at illness onset, hypertension (%), and diabetes mellitus (%). Methodological control variables were assigned to the third category which comprised the variables matching, blinding, year of publication, hyperintensities as primary vs. secondary research interest, assessment of hyperintensities (i.e., visual rating scale, automated, semi-automated), type of rating scale used, and outcome measure (i.e., severity rating vs. lesion volume). In short, automated lesion assessment describes the fully automated assessment of lesion volume via different adapted algorithms applied to the MRI scans. An automated segmentation process is operated using individually designed or at hand software. Predefined segmentation criteria are incorporated into the algorithms to identify lesion volume by differentiating between different kinds of brain tissue. In semi-automated processes, automatically pre-labeled MRI scans are additionally inspected by a researcher who manually outlines relevant lesions. Either lesion volume or severity ratings according to visual rating scales can be inferred. The fourth category of control variables incorporated differences in MRI procedures, namely the variables tesla, plane, slice thickness, and interslice gap.
Since the outcome measures of hyperintensity burden (e.g., volumetric vs. rating scale) were not homogenous between studies, the influence of the demographic variables on lesion load in the patient and the control sample could not directly be calculated. Furthermore, to investigate possible confounding it is necessary to determine if a difference in these variables between the patient and the control group moderates the association between depression and hyperintensity burden. For example, with increasing age the prevalence of hyperintensities increases. Therefore, it has to be excluded that differences in age between the patient and the control group might influence the difference in hyperintensity burden between patients and controls. Thus, to investigate the impact of these variables on the effect size measure (i.e., difference in hyperintensity burden), the differences between the patient and the control group were calculated. Concretely, the variables age, sex, smoking, hypertension, and diabetes were transformed into a new variable defined as the difference between the patient and the control group. For age, the years were subtracted. In the case of prevalence data (sex, smoking, hypertension, and diabetes), the percentages were subtracted. The influence of the difference in these variables between patients and controls on the standardized mean difference (hyperintensity burden) was analyzed. Taken together, investigating the influence of the differences in these demographic variables on the differences in lesion load (i.e., the effect size measure) allows controlling for possible confounding irrespective of different outcome measures between the studies.
In cases where samples overlapped between studies these studies were coded as one study. In this way, it was controlled for dependencies between outcomes due to dependencies between samples. Consequently, the studies by Potter et al. (2007), Taylor et al. (2007), and Taylor et al. (2008) were coded as different samples from the same study. In addition, the samples from the studies by McDonald et al. (1991) and McDonald et al. (1999) were coded as belonging to the same study.
Concerning lesion location, the different subcortical structures or white matter lesion locations were assigned to the categories OWMH, DWMH, PVH, and SCGMH.
All studies included in the meta-analysis were coded according to the above delineated coding scheme. If data on moderator or control variables was not directly reported, it was inferred from the given information where possible. Either lesion volume or severity ratings assessed via visual rating scales (Fazekas et al., 1987; Coffey et al., 1990; Scheltens et al., 1993) were regarded as indicators for the severity of hyperintensity burden. When outcome data for hyperintensities was not provided in an applicable form, authors of eligible studies were contacted. One of six authors replied and the study was included in the analyses.
As the meta-analysis represents an investigation of cross-sectional data and no intervention was examined (which excludes selection bias, performance bias, and attrition bias), blinding of the researcher in cases of visual severity ratings or semi-automated lesion assessment procedures was regarded as the primary control variable for assessing potential risk of bias (i.e., detection bias). Reporting bias is considered minimal, as highly selected samples were not included in the analyses (see inclusion and exclusion criteria). In addition, the risk of publication bias has been examined in multiple ways (see statistical analyses).
Effect Size Measure
Hedges' g was chosen as the effect size measure since it represents an appropriate calculation to determine the standardized mean difference between two groups. Hedges' g has the advantage of applying a correction term J to Cohen's d so that it is corrected for possible overestimation of the effect size due to small sample size. In the present meta-analysis, Hedges' g displays the difference in hyperintensity burden between the patient and the control group. Hedges' g is defined by the following formula (Hedges, 1981):
In this case, represents the mean hyperintensity burden of the control group and that of the patient group within one study, s02 and s12 are the corresponding variances and n0 and n1 represent the number of subjects in each group. For the exact definition of J see Hedges (1981).
Statistical Analyses
All statistical analyses were carried out using RStudio, version 1.0.153. Meta-analytic models were calculated using the R package metafor (Viechtbauer, 2010). Statistical analysis of heterogeneity and inspection of data strongly suggested applying a random-effects model (Cooper, 2009) since the Q-statistic revealed a significant heterogeneity between studies (p < 0.001) and study characteristics (design, demographics, and methods) were considerably diverging. A multilevel model (Van den Noortgate et al., 2013) was calculated to control for dependencies between outcomes with random effects on the levels outcome nested in sample nested in study taking into consideration that one study may include several samples or several outcome measures within one sample. Thus, it is accounted for that different outcomes from the same sample or study do not represent independent data. The influence of moderating variables on the effect size was investigated in subgroup analyses by including it in the model and testing subgroups against each other via linear hypothesis. Metric variables were investigated by calculating a meta-regression analysis of Hedges' g. The significance level for all statistical analyses was set α = 0.05. It is referred to two-sided p-values in every case to determine significance.
Outcomes were regarded as outliers if the value deviated more than three standard deviations from the arithmetic mean of Hedges' g, as such a deviation is considered highly unlikely under normal distribution (cf. three-sigma rule, Pukelsheim, 1994).
Publication bias was analyzed in three different ways. Firstly, the funnel plot was visually inspected to detect asymmetry. Secondly, as suggested by Sterne and Egger (2005), a meta-regression of the effect size against its variance was conducted, that is, a multilevel random effects meta-regression of Hedges' g against its variance was calculated. Thirdly, it was investigated if the binary control variable research interest (i.e., whether hyperintensities were part of the primary research question or not) acted as a moderating factor. This was controlled for by conducting a subgroup analysis.
Interrelation of variables was investigated by computing an interrelation matrix using adequate effect sizes for each interrelation (i.e., Pearson's r for two metric variables, Cramer's V for two factors, and η2 if one variable was metric and the other a factor). Interrelations were regarded as substantial if r ≥ 0.05 or if the correlation was significant at α = 0.05 significance level, if V ≥ 0.40, and if η2 ≥ 0.14 (Cohen, 1977). Each pair of highly confounded variables was included in a multilevel random effects model to test if the effect of one variable is explained by confounding with another variable.
Results
The demographic characteristics of the included studies (n = 68) and samples (n = 87) are summarized in Table 1. Patients and controls were of similar age (61.2 and 61.0 years, respectively) and sex (38.5 and 38.0% male, respectively). The characteristics of each sample are listed in Table 2. The data of 3,096 patients and 4,071 controls was included in the present meta-analysis. Table 3 shows the random effects model using Hedges' g, which displays the difference in hyperintensity burden between patients and controls across all lesion locations. In accordance with hypothesis 1, the patient group (MDD and BD) exhibited a significantly greater mean severity of hyperintensity burden than the control group (g = 0.229, SE = 0.031, p < 0.001). In this model, three outliers were excluded. This affected the study by Rej et al. (2014) and two outcomes of the study by Tighe et al. (2012) with effect sizes of g = −0.918, g = 2.293, and g = 3.058, respectively. The random effects model including all outcomes (g = 0.238, SE = 0.042, p < 0.001) is shown in Table 4. For all following analyses, outliers were excluded.
Visual inspection of the forest plot revealed a substantial heterogeneity between outcomes, which was also reflected by the estimated total standard deviation of the true effect from the multilevel random effects model as τ = 0.225 (Table 3).
Subgroup Analyses
Table 5 displays the subgroup analyses. The mean severity of hyperintensity burden was greater in either patient sample compared with the control group when including the subgroups MDD and BD in the model (p < 0.001 for both subgroups). The BD subgroup exhibited a significantly greater effect than the MDD subgroup (p = 0.012).
As displayed in Table 5, a late illness onset (late-onset depression) was associated with a greater hyperintensity burden than early-onset depression (p < 0.001), which is in line with hypothesis 2. Further, the late-onset depression group exhibited a significantly greater effect size than the late-life depression group (p < 0.001). These effects were also evident when investigating the interaction model including disorder (subgroups MDD and BD) and onset (subgroups early-onset, late-onset, and late-life). That is, both in unipolar and bipolar depression, late-onset depression was associated with a greater difference in hyperintensity burden between patients and controls than early-onset or late-life depression (Table 6).
Investigating lesion location (DWMH, PVH, and SCGMH), Hedges' g turned out significant in each subgroup regarding the whole patient sample (p < 0.001 for each subgroup). The interaction model including lesion location and disorder (MDD and BD) revealed that Hedges' g was significant in all lesion location subgroups in MDD. In BD, however, the effect size for SCGMH did not reach significance (p = 0.071). All other p-values for lesion location subgroups in MDD and BD ranged from p < 0.001 to p = 0.014. In subgroup analysis, no significant differences between DWMH, PVH, and SCGMH in effect size were evident regarding the whole patient group, as well as regarding MDD. In BD, on the contrary, Hedges' g was significantly greater for DWMH than for PVH (p = 0.033) and SCGMH (p = 0.005).
Control Variables
Three control variables turned out significant. Firstly, subgroup analysis revealed that the type of lesion assessment had a significant influence (Table 5). Semi-automated lesion assessment was associated with a significantly greater effect size than automated lesion assessment (p = 0.027). However, no difference in effect size was observed between volumetric outcome measures and severity ratings using semi-quantitative rating scales (p = 0.559).
Secondly, meta-regression analysis revealed a significant influence of age (p < 0.001) and age at illness onset (p = 0.037). Age was defined as the difference in years between the patient and the control sample, thus, a higher age in the patient group compared to the control group was significantly associated with a more pronounced difference in hyperintensity burden. An increase in age at illness onset was also significantly associated with a greater effect size. No other control variable included in the coding scheme turned out significant in meta-regression or subgroup analysis. The p-values ranged from p = 0.059 (hypertension) to p = 0.854 (smoking).
Interrelations Between Variables
Table 7 displays the measures of interrelation between variables. Substantial interrelations were found between onset and disorder, onset and age, as well as between lesion assessment and lesion location, which means that MDD and BD were not equally distributed across the onset groups, that the age difference between patients and controls differed between the onset groups, and that the lesion locations were not equally distributed across the lesion assessment categories. Each pair of interrelated variables was included in a multilevel random effects model. None of the models did differ from the above-mentioned outcome patterns, which means that interrelation of variables did not confound effect sizes, with one exception: Table 5 displays a significant difference between early-onset and late-life depression (p = 0.008). When including age in the model, this difference is no longer significant (p = 0.125). Notably, the differences between late-onset and early-onset depression, as well as between late-onset and late-life depression remain significant when controlling for age (p < 0.001 in both cases).
Publication Bias
Visual inspection of the funnel plot allows for a first evaluation regarding the existence of publication bias (Egger et al., 1997). The absence of publication bias is assumed to reflect the following pattern: The effect sizes of samples with a large sample size (and therefore small SE) are distributed near the average, while effect sizes of small samples (and therefore large SE) reveal a more widespread distribution, which leads to a symmetric, funnel-shaped distribution of effect sizes around the average. In this case, visual inspection of the funnel plot (Figure 2) suggested a slight asymmetry with missing outcomes in the lower left sections, that is, outcomes with an effect size that is smaller than the average and with a larger SE (i.e., smaller sample size) seemed to be underrepresented.
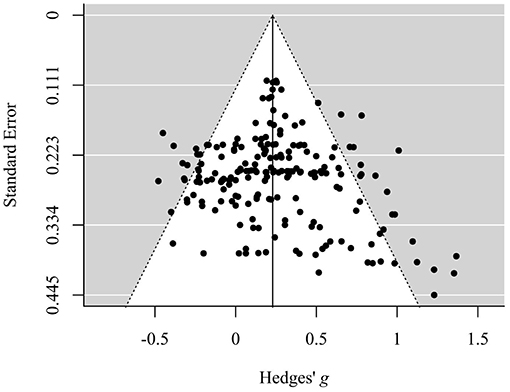
Figure 2. Funnel plot displaying the distribution of standard errors in dependence of corresponding standardized mean differences (Hedges' g). Data is shown on outcome level.
However, the meta-regression analysis of Hedges' g against its variance revealed a small and non-significant intercept (p = 0.910), which indicates that there is no evidence for funnel plot asymmetry as the regression line runs through zero. More precisely, on the basis of this model a “small study effect” is unlikely. This indicates that the meta-analytic model is not biased by a selective publication of studies in dependence of effect size and SE in view of the fact that studies with a small number of participants (and therefore a larger SE) are commonly more likely to be publicized if they show a greater effect size in the desired direction.
Another aspect of possible publication bias refers to the study aims. The binary control variable research interest differentiated whether hyperintensity burden was part of the primary research question. Hedges' g was significantly greater in studies in which hyperintensity burden was part of the primary research question (p = 0.029). This might hint at a publication bias in the sense that studies in which hyperintensity burden is the core object of investigation are more likely to be publicized if the effect size is substantial. This would not affect studies that regard hyperintensity burden as a secondary outcome with respect to study aims. Collectively, the presence of a publication bias cannot be conclusively excluded.
Discussion
Summary of Main Findings
Including 68 studies, the meta-analysis represents the most extensive overview of the state of research regarding the severity of hyperintensity burden in MDD (n = 54) and BD (n = 17) in comparison to healthy controls so far. To a large extent, both hypotheses were confirmed by the statistical analyses: Hyperintensity burden was higher in the entire depression group compared to the control group, which was also evident within each depression group (MDD and BD) and within the categories DWMH, PVH, and SCGMH (hypothesis 1), though the analyses did not confirm an association of SCGMH burden with BD. The association between hyperintensity burden and depression was moderated by age at illness onset in terms of late-onset depression being associated with a more pronounced difference in hyperintensity burden between patients and controls in comparison to early-onset depression in both MDD and BD (hypotheses 2). Notably, it is the first meta-analysis to demonstrate a significant difference between late-life and late-onset depression and, in terms of the outcome measure, this is the first meta-analysis that investigated the association between BD and hyperintensity severity burden instead of frequency. Regarding MDD, this was conducted by three prior meta-analyses (Herrmann et al., 2008; Kempton et al., 2011; Anorne et al., 2012). However, the present meta-analysis provides a more comprehensive overview of the current state of research on hyperintensity severity in MDD as a substantially greater number of studies were included in the analyses. Taken together, the results underscore the association of hyperintensities with unipolar and bipolar depression, which is in line with prior meta-analyses (Beyer et al., 2009; Wang et al., 2014; van Agtmaal et al., 2017).
The results reveal a substantial heterogeneity with τ = 0.225 in relation to g = 0.229, which has to be taken into account when interpreting the results. This heterogeneity is reduced to τ = 0.127 by including the moderators disorder, onset, and age, which shows that these variables explain heterogeneity to some degree. However, its magnitude suggests that the association of MDD and BD with hyperintensities varies across conditions and cannot be assumed to predict lesion load on an individual level unrestrictedly.
Linking Hyperintensities and Depression
Hyperintensities Displaying Disruption in White Matter Tracts
Hyperintense lesions are linked to decreased fractional anisotropy (FA) and an increased apparent diffusion coefficient (ADC), which is evident in healthy as well as in depressed subjects (Taylor et al., 2001; Vernooij et al., 2008). For example, impaired integrity in tracts that connect prefrontal regions and subcortical gray matter structures might thus be presented as DWMH on MRI scans. Abnormalities in these tracts are assumed to interrupt brain circuitries involved in emotion processing and are therefore assumed to play a role in MDD and BD symptomatology (Mahon et al., 2010).
Hyperintensity-Related Correlates on Molecular Level
Recent approaches explaining the association between lesion load and depression depict a less deterministic perspective than the vascular depression hypothesis. However, these are closely related to the vascular depression hypothesis, since they likewise refer to the association between vascular changes, lesion load, and depression. On the molecular level, three interrelated mechanisms are suggested (Santos et al., 2012). Firstly, an elevated plasma homocysteine level is associated with hyperintense lesions and is assumed to be a risk factor for depression in later life (Almeida et al., 2007; Kim et al., 2008; Tseng et al., 2009). One of the possible mechanisms explaining this association is that elevated homocysteine levels can lead to endothelial dysfunction (Tawakol et al., 1997; Lentz, 2005). Endothelial dysfunction, in turn, is proposed to represent the second mechanism linking hyperintensities and depression. Several markers of endothelial dysfunction are associated with unipolar and bipolar depression (Rybakowski et al., 2006) as well as with white matter hyperintensities (Zupan et al., 2015). There is evidence for a bidirectional relationship between depressive symptoms and endothelial dysfunction (Kim et al., 2010). The third mechanism linking hyperintensities and depression considers inflammatory activity. For example, Interleukin 6 (IL-6) was shown to be associated with late-life depression (Penninx et al., 2003; Bremmer et al., 2008) as well as with DWMH and PVH (Nagai et al., 2011). IL-6 is, like homocysteine, assumed to induce endothelial dysfunction (Wassmann et al., 2004). Therefore, these models presume an interaction between several hyperintensity-related correlates of depression on molecular level and do not exclude a bidirectional relation of depression and lesion load. For a more detailed overview, see Santos et al. (2012).
Hyperintensity Burden in MDD vs. BD
Several studies indicate that the frequency of subjects with hyperintensities is higher in BD compared to MDD (Dupont et al., 1995; Kieseppä et al., 2014), while other studies did not find such a difference (Sassi et al., 2003). Here it was shown that subjects with BD exhibit a more severe hyperintensity burden than subjects with MDD. Specifically, the significant role of DWMH in BD compared to PVH and SCGMH seems to distinguish hyperintensity burden in BD from that of MDD, as in MDD no difference in lesion load regarding DWMH, PVH, and SCGMH was observed. The more pronounced DWMH burden in BD compared to MDD might be explained by differences in the extent of decreased FA between MDD and BD. The meta-analysis by Nortje et al. (2013) demonstrated a broad white matter involvement in BD patients regarding changes in diffusion properties. One study compared whole-brain FA between MDD subjects, BD subjects, and healthy controls (Versace et al., 2010). In BD, bilateral changes in white matter connectivity (i.e., decreased FA) were found in regions involved in emotion regulation and sensory processing, while in MDD, only unilateral changes were evident. It is suggested that these more global abnormalities in white matter tracts in BD compared to MDD patients might account for the characteristic mood lability in BD (Cardoso de Almeida and Phillips, 2013).
Another possible explanation is that the more pronounced hyperintensity burden in BD, compared to MDD, does not cause bipolar symptomatology, but rather reflects the presence of hyperintensity-related correlates that are more prevalent in BD than in MDD, for example, obesity, metabolic syndrome, and cardiovascular risk factors such as hypertension (Fiedorowicz et al., 2008). This assumption seems plausible, as these risk factors are associated with hyperintensities (Portet et al., 2012; Yin et al., 2014; Habes et al., 2016). According to this view, hyperintense lesions are indicators for the presence of comorbidities which frequently occur in the course of BD (Gunde et al., 2011).
Furthermore, the more pronounced lesion load regarding DWMH compared to PVH in BD might be explained on molecular level. Permoda-Osip et al. (2013) demonstrated that hyperhomocysteinemia frequently occurs in BD subjects during an acute affective episode. In addition, elevated homocysteine levels are associated with common comorbidities of BD such as hypertension or metabolic syndrome (Sutton-Tyrrell et al., 1997; Hajer et al., 2007). Regarding lesion location, there are several studies showing that elevated homocysteine levels are rather linked to DWMH than to PVH (Hogervorst et al., 2002; Sachdev, 2004; Sachdev et al., 2004). Therefore, a high prevalence of hyperhomocysteinemia in BD patients might to some degree account for the difference between DWMH and PVH burden which was found in the present meta-analysis.
Theoretical Implications: Confining the Vascular Depression Hypothesis
The present meta-analysis underscores the relevance of age at illness onset with regard to lesion load. Consistent with prior findings (Herrmann et al., 2008), hyperintensity burden is more severe in late-onset depression in comparison to early-onset depression, that is, in the present study, the difference in hyperintensity burden between patients and controls is more pronounced in late-onset depression compared to early-onset depression. The findings demonstrate that this pattern is evident in both unipolar and bipolar depression. Therefore, the results can be interpreted as underlining the significance of cerebrovascular correlates when it comes to depression in the elderly.
The association of vascular lesions with depressive symptomatology in older age was first described in 1905 (Gaupp, 1905) and referred mainly to arteriosclerotic phenomena. By introducing the vascular depression hypothesis, Alexopoulos et al. (1997) presented a more elaborate concept which involves cerebrovascular changes. According to this theory, disruption in brain circuits that subserve mood regulation due to cerebrovascular lesions is the core mechanism in the etiology of vascular depression in later life. In the case of BD, some authors refer to this concept using the term vascular mania (Steffens and Krishnan, 1998). As mentioned before, the vascular depression hypothesis is not restricted to late-onset depressive syndromes, arguing that depression in earlier life increases the risk for vascular diseases which, in turn, can lead to vascular depression in later life (Taylor et al., 2013a). In view of the present findings, however, it seems reasonable to link the concept of vascular depression specifically to late-onset rather than late-life depression. Although, late-life depression with an early illness onset might in some cases likewise be of a vascular type, this phenomenon can be assumed to be significantly less common—at least when referring to hyperintensities as the hallmark of vascular depression—as demonstrated by the findings of the present meta-analysis: Firstly, it is shown that hyperintensity burden is significantly more severe in late-onset depression than in late-life depression, which was observed in the entire patient sample, as well as in MDD and BD. This difference might be due to the circumstance that late-life depression can comprise both early- and late-onset depression which are in many cases not distinguished between when investigating late-life depression (van Agtmaal et al., 2017), while late-onset depression is restricted to late-life depression with a late illness onset (Aizenstein et al., 2016). It can therefore not be excluded that the association of late-life depression with hyperintensities might rather be explained, i.e., mediated by late-onset depression. Secondly, when controlling for age, there was no longer a significant difference between late-life and early-onset depression, while the differences between late-onset and late-life depression as well as between late-onset and early-onset depression remained significant. Vascular depression among early-onset patients is unlikely, as there was no significant difference regarding hyperintensity burden in the early-onset subgroup compared to the control group. In addition, age at illness onset turned out to be a significant moderator. Taken together, these findings provide evidence that the vascular depression subtype is more likely when the first episode occurs in older age.
In conclusion, it might be more feasible to focus on late-onset depression when it comes to defining vascular depression. Confining the concept on the basis of the above delineated evidence might allow for more precise theoretical elaboration and research on vascular depression. With respect to specifying diagnostic criteria for the vascular subtype of depression, a stricter definition (i.e., a late illness onset) helps to increase its internal and external validity and might, on that account, subserve its diagnostic implementation in clinical context. Why identifying a vascular subtype of depression might be of practical relevance concerning individualized treatment planning, is discussed below. Taken together, vascular depression might in some cases have an early illness onset, but this circumstance does not justify the rather vague focus on late-life depression, which is currently more common in research—as also displayed by the number of studies included in the different onset subgroups of the present meta-analysis. In this view, the present results strongly suggest confining the concept of vascular depression to late-onset depression in terms of its feasibility in research as well as in clinical context.
Although the present meta-analysis is in line with the vascular depression hypothesis regarding the association of hyperintensities with depression in the elderly, it should not be mistaken as fostering this theory taking into account the cross-sectional nature of the meta-analysis. No conclusions on causal directions can be drawn. Therefore, strictly speaking, the findings should not be construed as proving evidence for the vascular depression hypothesis as such, viz. that cerebrovascular disease may entail depressive disorders in old age (predispose, precipitate, or perpetuate, cf. (Alexopoulos et al., 1997), which would at least to some degree imply causality.
Neuropathological Correlates of Late-Onset Depression
A broader understanding of neuropathological changes in late-onset depression is essential to determine its etiopathogenetic factors. In addition to hyperintensity burden, there is a variety of abnormalities associated with late-onset depression, as demonstrated by a substantial number of studies. This underlines the clinical validity of distinguishing late-onset depression from depression in earlier life. With regard to vascular burden, Smith et al. (2009) found that a later age at illness onset in depressed subjects were associated with higher levels of intima-media thickness. Intima-media thickness, in turn, was shown to correlate with white matter hyperintensities in late-onset depression (Chen C. S. et al., 2006). Further, Liao et al. (2017) found that late-onset depression is characterized by greater abnormalities in cerebral blood flow compared to early-onset depression. Regarding neurostructural changes, Xekardaki et al. (2012) report in their review that volume reductions in several brain regions occur in late-onset depression in comparison to both healthy control subjects and subjects with early-onset depression. Specifically, studies on structural brain changes found that late-onset depression was associated with right frontal lobe atrophy (Almeida et al., 2003), gray matter reductions in parahippocampal area, parietal inferior area, cingulum and putamen (Andreescu et al., 2008), and decrease of volume in the right rostral hippocampus, the right amygdala, and the medial orbito-frontal cortex (Egger et al., 2008). Comparing late-onset depression with late-life-early-onset depression, Disabato et al. (2014) found that late-onset depression was associated with a smaller left anterior cingulate thickness. Further neuroanatomical changes in the limbic system are described by Choi et al. (2017) who demonstrated total hippocampal and hippocampal subfield volume reductions in subjects with late-onset depression in comparison to healthy controls. Interestingly, hippocampal subfield volumes were partly correlated with white matter hyperintensity volume. Concerning neurofunctional changes, studies using functional MRI (fMRI) or positron-emission tomography (PET) reveal several abnormalities in patients with late-onset depression. Fujimoto et al. (2008) describe widespread changes in the distribution of metabolism in subjects with treatment-resistant late-onset depression compared to age-matched healthy controls. Alterations were not only evident in limbic circuits, but also in a wider range of thalamo-cortical circuits. By measuring regional homogeneity in resting-state fMRI signals in depressed, treatment-naive subjects, Chen et al. (2012) found differences in regional brain activity between late-onset and early-onset depression in the right precuneus and bilateral superior frontal gyrus. Recently, Liu et al. (2018) identified abnormal functional connectivity in anterior and posterior sub-networks of the default mode network as a potential risk factor for late-onset depression. Taken together, neuropathological correlates of late-onset depression seem to comprehend multiple cerebrovascular, neurostructural and neurofunctional changes compared to both healthy control subjects and subjects with early-onset depression.
However, there are several studies that do not confirm that late-onset depression is characterized by distinct neuropathological phenomena (Santos et al., 2010; Jellinger, 2011; Dols et al., 2017). As a possible explanation for the non-significant association between late-onset depression and microvascular disease shown in several post-mortem studies, Xekardaki et al. (2012) point out the relevance of psychosocial determinants in late-onset depression. The contradictory findings could also be interpreted as demonstrating that late-onset depression is not necessarily of a vascular subtype. This would be in line with the substantial heterogeneity found in the present study. In view of the multiple neuropathological phenomena that are found in late-onset depression it remains to be investigated how these are related to vascular depression. The diverse abnormalities on cerebrovascular, neurostructural, and neurofunctional level indicate that late-onset depression represents a heterogeneous disease pattern, within which vascular depression may be one possible subtype.
Limitations
There are some limiting factors that should be taken into account when interpreting the results. Above all, the cross-sectional nature of the analyses must be considered when referring to implications of the findings on theoretical and practical level. Therefore, on the basis of the present meta-analysis, no conclusions on causality regarding the association between depression and hyperintensity burden can be drawn. All explanations and implications of findings that are depicted in this article display only a few of many possible other mechanisms and consequences.
Several control variables that can be assumed to be associated with hyperintensities were included. Except for age, none of these turned out significant. The non-significant findings, however, may be the consequence of inappropriate or insufficient data provided by the primary studies. This affects the variables hypertension, diabetes mellitus, depression severity, smoking, and medication. Therefore, the analyses on control variables do not represent the whole dataset, and confounding by such variables that, in most cases, were not reported in primary studies can—despite of the non-significant findings—not be excluded.
In addition, the interaction model for disorder and onset includes only a limited number of studies in the BD onset-subgroups. This might limit the validity of the depicted outcome pattern.
A bias due to selective publication is unlikely, but cannot be excluded. Controlling for the role of hyperintensities regarding the research question(s) of the primary studies revealed that studies in which the focus lies on investigating hyperintensities show a greater effect size. In view of this, a publication bias might exist in the sense that studies with smaller effect sizes were less likely to be publicized if hyperintensity burden was the main outcome measure.
A further limitation regards the database literature search. Even though the present meta-analysis comprises a substantial number of studies and, therefore, is likely to represent a comprehensive overview of the present state of research, it cannot be excluded that a more elaborate literature search including further databases (e.g., Scopus) might have yielded even more potentially relevant studies. However, due to limited resources, the literature search was restricted to the above mentioned databases.
Future Research
The present meta-analysis focuses on severity ratings and volumetric measurements as indicators of the extent of hyperintensity burden in patients and controls, while prior meta-analyses predominantly compared the frequency of subjects with hyperintensities between both groups. As hyperintensities are a common phenomenon of old age (de Leeuw et al., 2012), rather the extent of hyperintensity burden than the number of subjects with hyperintensities might represent a meaningful parameter when investigating depression in later life. Future research might directly compare severity ratings with frequency ratings to examine potential differences between these two outcome measures.
Regarding age at illness onset, the studies included in the present meta-analysis use different cut-offs to differentiate between early-onset and late-onset depression. It seems unlikely that a single cut-off age can be determined. However, future research regarding late-onset depression or vascular depression, respectively, could engage in identifying an age at onset range which is most likely to indicate the presence of a vascular subtype of depression.
The role of hyperintensity assessment requires further research. While there was no significant difference in effect size between volumetric outcomes and severity measurements using semi-quantitative rating scales, fully-automated vs. semi-automated lesion assessment did make a difference within volumetric measurements. Hyperintensity burden was significantly higher when lesion volume was determined using semi-automated procedures compared to automated (Table 5). At first glance, this might hint at some kind of researcher bias. Yet, the binary control variable blinding did not turn out significant (p = 0.885). Thus, the reason for the effect of automated vs. semi-automated hyperintensity assessment remains unclear. Aside from bias, it is possible that this difference reflects a distinctive characteristic of depression-related hyperintensities. The nature of hyperintense lesions that are associated with depression might be specific in such a way that they cannot be adequately detected using fully automated assessment processes, e.g., because they are too small or the sensitivity of automated algorithms is too low. In this respect, it should be considered to which extent, i.e., limits, segmentation criteria applied by a radiologist or expert rater can be incorporated into fully automated segmentation algorithms. This further questions the feasibility of fully automated lesion assessment procedures in general. These and other possible explanations need to be scrutinized in future research.
Clinical Implications
The pronounced difference in hyperintensity burden comparing late-onset to early-onset depression in MDD and BD suggests a clinical differentiation according to age at illness onset. Nevertheless, this does not mean that late-onset depression is accompanied by cerebrovascular pathology in every case. A more reasonable explanation would be that among late-onset depression the vascular subtype is more common, that is, late-onset depression is more frequently but not necessarily associated with a substantial lesion load.
There is evidence that vascular MDD is associated with increased functional impairment relative to non-vascular MDD (González et al., 2012), which underscores the clinical relevance of distinguishing between vascular and non-vascular depression. This differentiation in clinical context gains importance when it comes to treatment planning. For example, it might be worth considering a co-treatment of cerebrovascular pathology in addition to treating the mood disorder alone. In this respect, a thorough examination of vascular risk factors that possibly underlie hyperintense lesions can facilitate individualized treatment options for vascular depression. First of all, this affects medication. In late-onset depressive syndromes, not only psychoactive drugs, but also those that might prevent or reduce hyperintensity progression should be examined for possible benefits regarding depression treatment, e.g., cilostazol (Takahashi and Mikuni, 2012) or other substances that improve cerebral blood flow. Moreover, hyperintensity-related correlates on molecular level could be taken into account when considering broader medical treatment options, for example, with respect to inflammatory processes (Müller et al., 2006). In clinical context, however, side-effects of such additional medical treatment must be considered.
In addition, the benefits of different classes of antidepressant drugs should be taken into account. As mentioned above, vascular depression is characterized, among others, by a reduced response rate to antidepressant medication and by cognitive decline. This raises the question if antidepressant drugs that show effects on neurostructural and neurofunctional level can enhance response rates to medical treatment of vascular depression. For example, Pompili et al. (2013) report in their review that agomelatine, an atypical antidepressant drug targeting melatonergic receptors, is not only effective with regard to its antidepressant action and side-effect profile, but also promotes neurogenesis in the hippocampus and the prefrontal cortex as well as enhancing neuroplasticity mechanisms. Whether these neurofunctional and neurostructural effects can improve treatment response in vascular depression would thus be an interesting question regarding medical treatment options.
Aside from medical conditions, lifestyle aspects can lead to or increase cerebrovascular lesion burden, such as smoking or obesity (Zhu et al., 2014; Habes et al., 2016). Therefore, treatment of vascular depression might profit from an approach tackling behavioral patterns that are associated with progressing cerebrovascular pathology.
In the present meta-analysis, age (i.e., the difference in years between the patient and the control sample) turned out to be a significant moderator. This underlines the general association of age with hyperintensities (de Leeuw et al., 2012), which might be explained by vascular changes associated with aging (Marín, 1995; Kovacic et al., 2011) and the higher prevalence of vascular-related diseases in the elderly (Kearney et al., 2005; Shaw et al., 2010). Nevertheless, even though hyperintensities are a common condition in later life, they might represent a premorbid phenotype of depression. For example, the meta-analysis by Kloppenborg et al. (2014) demonstrated that WMH progression was associated with decline in several cognitive domains which, in turn, represents a characteristic symptomatology in geriatric depression, especially in late-onset depression (Lesser et al., 1996; Rapp et al., 2005). The association of hyperintensities with age and depression as well as with abnormalities in cognitive functioning (as shown by Kloppenborg et al., 2014) underlines their clinical relevance in geriatric healthcare. For example, depression screenings in later life that give attention to the symptom classes of late-onset depression might be useful to detect premorbid vascular depression.
Final Conclusions
To conclude, there is an association between hyperintensities and depression which seems to be strongly dependent on age at illness onset. On the basis of the present findings it seems reasonable to assume that hyperintensity-related depression is most likely to be characterized by a late illness onset. This association should be taken into account when investigating etiologic models for MDD and BD in later life. Specifically, it suggests to confine the concept of vascular depression to late-onset depression. This confinement seems feasible in a research as well as in a clinical context. Within a research context it might enhance internal and external validity when it comes to elaborating the concept of vascular depression. In a clinical context, the confinement gains importance regarding diagnostic criteria and the detection of vascular depression. A depression diagnosis that differentiates vascular from non-vascular depression might allow for a more individualized treatment plan. This, finally, might reduce the rate of treatment-resistant depression in later life. On the one hand, this would affect the choice of antidepressant medication as well as considering other potentially adjuvant drugs. Aside from medication, behavioral patterns associated with hyperintensities likewise merit consideration in the context of vascular depression treatment. The moderating role of age regarding hyperintensity burden suggests that the risk for vascular depression is increased in the elderly, at least when assuming that hyperintensities represent a preceding or interacting risk factor for vascular depression. Thus, treating vascular diseases and reducing vascular risk factors that are associated with hyperintensities could help to prevent depressive syndromes in the elderly.
Overall, future research should consider different perspectives regarding possible mechanisms underlying the relationship between hyperintensities and depression in order to achieve a broader understanding of illness predisposition, triggering, perpetuation, and consequences. This, essentially, might allow for more individualized and potent treatment options regarding depressive disorders.
Author Contributions
KS and IW conducted the literature search. KS coded the studies. KS and JS performed the data analysis. KS, JS, RS, HH, and IW wrote the article.
Conflict of Interest Statement
The authors declare that the research was conducted in the absence of any commercial or financial relationships that could be construed as a potential conflict of interest.
Supplementary Material
The Supplementary Material for this article can be found online at: https://www.frontiersin.org/articles/10.3389/fpsyg.2019.01241/full#supplementary-material
References
Agid, R., Levin, T., Gomori, J. M., Lerer, B., and Bonne, O. (2003). T2-weighted image hyperintensities in major depression: focus on the basal ganglia. Int. J. Neuropsychopharmacol. 6, 215–224. doi: 10.1017/S146114570300347X
Ahn, K. H., Lyoo, I. K., Lee, H. K., Song, I. C., Oh, J. S., Hwang, J., et al. (2004). White matter hyperintensities in subjects with bipolar disorder. Psychiatry Clin. Neurosci. 58, 516–521. doi: 10.1111/j.1440-1819.2004.01294.x
Aizenstein, H. J., Baskys, A., Boldrini, M., Butters, M. A., Diniz, B. S., Jaiswal, M. K., et al. (2016). Vascular depression consensus report - a critical update. BMC Med. 14:161. doi: 10.1186/s12916-016-0720-5
Alexopoulos, G. S., Meyers, B. S., Young, R. C., Campbell, S., Silbersweig, D., and Charlson, M. (1997). 'Vascular depression' hypothesis. Arch. Gen. Psychiatry 54, 915–922.
Allan, C. L. (2014). Brain MRI Correlates of Depression and Vascular Risk: Whitehall Imaging Sub-Study. Dissertation, University College, London.
Almeida, O. P., Burton, E. J., Ferrier, N., McKeith, I. G., and O'Brien, J. T. (2003). Depression with late onset is associated with right frontal lobe atrophy. Psychol. Med. 33, 675–681. doi: 10.1017/S003329170300758X
Almeida, O. P., Flicker, L., Norman, P., Hankey, G. J., Vasikaran, S., van Bockxmeer, F. M., et al. (2007). Association of cardiovascular risk factors and disease with depression in later life. Am. J. Geriatr. Psychiatry 15, 506–513. doi: 10.1097/01.JGP.0000246869.49892.77
Altshuler, L. L., Curran, J. G., Hauser, P., Mintz, J., Denicoff, K., and Post, R. (1995). T2 hyperintensities in bipolar disorder. Magnetic resonance imaging comparison and literature meta-analysis. Am. J. Psychiatry 152, 1139–1144.
Alves, G. S., Karakaya, T., Fußer, F., Kordulla, M., O'Dwyer, L., Christl, J., et al. (2012). Association of microstructural white matter abnormalities with cognitive dysfunction in geriatric patients with major depression. Psychiatry Res. 203, 194–200. doi: 10.1016/j.pscychresns.2011.12.006
American Psychiatric Association (1987). Diagnostic and Statistical Manual of Mental Disorders, 3rd Edn. Washington DC: American Psychiatric Association.
Andreescu, C., Butters, M. A., Begley, A., Rajji, T., Wu, M., Meltzer, C. C., et al. (2008). Gray matter changes in late life depression–a structural MRI analysis. Neuropsychopharmacology 33, 2566–2572. doi: 10.1038/sj.npp.1301655
Anorne, D., McIntosh, A., Ebmeier, K., Munafò, M., and Andersen, I. (2012). Magnetic resonance imaging studies in unipolar depression: systematic review and meta-regression analysis. Eur. Neuropsychopharmacol. 22, 1–16. doi: 10.1016/j.euroneuro.2011.05.003
Awad, I. A., Johnson, P. C., Spetzler, R. F., and Hodak, J. A. (1986). Incidental subcortical lesions identified on magnetic resonance imaging in the elderly. II. Postmortem pathological correlations. Stroke 17, 1090–1097.
Bakker, S. L., de Leeuw, F. E., de Groot, J. C., Hofman, A., Koudstaal, P. J., and Breteler, M. M. (1999). Cerebral vasomotor reactivity and cerebral white matter lesions in the elderly. Neurology 52, 578–583.
Beyer, J. L., Young, R., Kuchibhatla, M., and Krishnan, K. R. (2009). Hyperintense MRI lesions in bipolar disorder: a meta-analysis and review. Int. Rev. Psychiatry 21, 394–409. doi: 10.1080/09540260902962198
Birner, A., Seiler, S., Lackner, N., Bengesser, S. A., Queissner, R., Fellendorf, F. T., et al. (2015). Cerebral white matter lesions and affective episodes correlate in male individuals with bipolar disorder. PLoS ONE 10:e0135313. doi: 10.1371/journal.pone.0135313
Bremmer, M. A., Beekman, A. T. F., Deeg, D. J. H., Penninx, B. W. J. H., Dik, M. G., Hack, C. E., et al. (2008). Inflammatory markers in late-life depression: results from a population-based study. J. Affect. Disord. 106, 249–255. doi: 10.1016/j.jad.2007.07.002
Breteler, M. M. B., van Swieten, J. C., Bots, M. L., Grobbee, D. E., Claus, J. J., van den Hout, J. H. W., et al. (1994). Cerebral white matter lesions, vascular risk factors, and cognitive function in a population-based study: the Rotterdam Study. Neurology 44, 1246–1252.
Brown, F. W., Lewine, R. J., Hudgins, P. A., and Risch, S. C. (1992). White matter hyperintensity signals in psychiatric and nonpsychiatric subjects. Am. J. Psychiatry 149, 620–625.
Cardoso de Almeida, J. R., and Phillips, M. L. (2013). Distinguishing between unipolar depression and bipolar depression. Curr. Fut. Clin. Neuroimaging Perspect. Biol. Psychiatry 73, 111–118. doi: 10.1016/j.biopsych.2012.06.010
Chen, C. S., Chen, C. C., Kuo, Y. T., Chiang, I. C., Ko, C. H., and Lin, H. F. (2006). Carotid intima-media thickness in late-onset major depressive disorder. Int. J. Geriatr. Psychiatry 21, 36–42. doi: 10.1002/gps.1420
Chen, J.-D., Liu, F., Xun, G.-L., Chen, H.-F., Hu, M.-R., Guo, X.-F., et al. (2012). Early and late onset, first-episode, treatment-naive depression. Same clinical symptoms, different regional neural activities. J. Affect. Disord. 143, 56–63. doi: 10.1016/j.jad.2012.05.025
Chen, Y. F., Wang, H., Chu, Y., Huang, Y. C., and Su, M. Y. (2006). Regional quantification of white matter hyperintensity in normal aging, mild cognitive impairment, and Alzheimer's disease. Dement. Geriatr. Cogn. Disord. 22, 177–184. doi: 10.1159/000094785
Choi, W. H., Jung, W. S., Um, Y. H., Lee, C. U., Park, Y. H., and Lim, H. K. (2017). Cerebral vascular burden on hippocampal subfields in first-onset drug-naïve subjects with late-onset depression. J. Affect. Disord. 208, 47–53. doi: 10.1016/j.jad.2016.08.070
Coffey, C. E., Figiel, G. S., Djang, W. T., and Weiner, R. D. (1990). Subcortical hyperintensity on magnetic resonance imaging. A comparison of normal and depressed elderly subjects. Am. J. Psychiatry 147, 187–189. doi: 10.1176/ajp.147.2.187
Cohen, J. (1977). Statistical Power Analysis for the Behavioural Sciences. 2. Aufl. New York, NY: Academic Press.
Colloby, S. J., Vasudev, A., O'Brien, J. T., Firbank, M. J., Parry, S. W., and Thomas, A. J. (2011). Relationship of orthostatic blood pressure to white matter hyperintensities and subcortical volumes in late-life depression. Br. J. Psychiatry 199, 404–410. doi: 10.1192/bjp.bp.110.090423
Compta, Y., Buongiorno, M., Bargalló, N., Valldeoriola, F., Muñoz, E., Tolosa, E., et al. (2016). White matter hyperintensities, cerebrospinal amyloid-β and dementia in Parkinson's disease. J. Neurol. Sci. 367, 284–290. doi: 10.1016/j.jns.2016.06.009
Cooper, H. (2009). The Handbook of Research Synthesis and Meta-Analysis. New York, NY: Russell Sage Foundation.
Dalby, R. B., Chakravarty, M. M., Ahdidan, J., Sørensen, L., Frandsen, J., Jonsdottir, K. Y., et al. (2010). Localization of white-matter lesions and effect of vascular risk factors in late-onset major depression. Psychol. Med. 4, 1389–1399. doi: 10.1017/S0033291709991656
Davis, K. A., Kwon, A., Cardenas, V. A., and Deicken, R. F. (2004). Decreased cortical gray and cerebral white matter in male patients with familial bipolar I disorder. J. Affect. Disord. 82, 475–485. doi: 10.1016/j.jad.2004.03.010
De Asis, J. M., Greenwald, B. S., Alexopoulos, G. S., Kiosses, D. N., Ashtari, M., Heo, M., et al. (2006). Frontal signal hyperintensities in mania in old age. Am. J. Geriatr. Psychiatry 14, 598–604. doi: 10.1097/01.JGP.0000200603.70504.d5
de Groot, J. C., de Leeuw, F. E., Oudkerk, M., Hofman, A., Jolles, J., and Breteler, M. M. (2001). Cerebral white matter lesions and subjective cognitive dysfunction. The Rotterdam Scan Study. Neurology 56, 1539–1545. doi: 10.1212/wnl.56.11.1539
de Leeuw, F. E., de Groot, J. C., Achten, E., Oudkerk, M., Ramos, L. M., Heijboer, R., et al. (2012). Prevalence of cerebral white matter lesions in elderly people: a population based magnetic resonance imaging study. The Rotterdam Scan Study. J. Neurol. Neurosurg. Psychiatr. 70, 2–3. doi: 10.1136/jnnp.70.1.9
Delaloye, C., Moy, G., Bilbao, F., Baudois, S., Weber, K., Hofer, F., et al. (2010). Neuroanatomical and neuropsychological features of elderly euthymic depressed patients with early- and late-onset. J. Neurol. Sci. 299, 19–23. doi: 10.1016/j.jns.2010.08.046
Disabato, B. M., Morris, C., Hranilovich, J., D'Angelo, G. M., Zhou, G., Wu, N., et al. (2014). Comparison of brain structural variables, neuropsychological factors, and treatment outcome in early-onset versus late-onset late-life depression. Am. J. Geriatr. Psychiatry 22, 1039–1046. doi: 10.1016/j.jagp.2013.02.005
Dols, A., Bouckaert, F., Sienaert, P., Rhebergen, D., Vansteelandt, K., Ten Kate, M., et al. (2017). Early- and late-onset depression in late life. A prospective study on clinical and structural brain characteristics and response to electroconvulsive therapy. Am. J. Geriatr. Psychiatry 25, 178–189. doi: 10.1016/j.jagp.2016.09.005
Dotson, V. M., Zonderman, A. B., Kraut, M. A., and Resnick, S. M. (2013). Temporal relationships between depressive symptoms and white matter hyperintensities in older men and women. Int. J. Geriatr. Psychiatry 28, 66–74. doi: 10.1002/gps.3791
Dupont, R. M., Butters, N., Schafer, K., Wilson, T., Hesselink, J., and Gillin, J. C. (1995). Diagnostic specificity of focal white matter abnormalities in bipolar and unipolar mood disorder. Biol. Psychiatry 38, 482–486.
Egger, K., Schocke, M., Weiss, E., Auffinger, S., Esterhammer, R., Goebel, G., et al. (2008). Pattern of brain atrophy in elderly patients with depression revealed by voxel-based morphometry. Psychiatry Res. 164, 237–244. doi: 10.1016/j.pscychresns.2007.12.018
Egger, M., Smith, G. D., Schneider, M., and Minder, C. (1997). Bias in meta-analysis detected by a simple, graphical test. BMJ 315, 629–634. doi: 10.1136/bmj.315.7109.629
El-Badri, S. M., Cousins, D. A., Parker, S., Ashton, H. C., McAllister, V. L., Ferrier, I. N., et al. (2006). Magnetic resonance imaging abnormalities in young euthymic patients with bipolar affective disorder. Br. J. Psychiatry 189, 81–82. doi: 10.1192/bjp.bp.105.011098
Emsell, L., Adamson, C., Winter, F.-L., de Billiet, T., Christiaens, D., Bouckaert, F., et al. (2017). Corpus callosum macro and microstructure in late-life depression. J. Affect. Disord. 222, 63–70. doi: 10.1016/j.jad.2017.06.063
Farkas, E., de Vos, R. A., Donka, G., Jansen Steur, E. N., Mihály, A., and Luiten, P. G. (2006). Age-related microvascular degeneration in the human cerebral periventricular white matter. Acta Neuropathol. 111, 150–157. doi: 10.1007/s00401-005-0007-y
Fazekas, F., Chawluk, J. B., Alavi, A., Hurtig, H. I., and Zimmerman, R. A. (1987). MR signal abnormalities at 1.5 T in Alzheimer's dementia and normal aging. Am. J. Roentgenol. 149, 351–356. doi: 10.2214/ajr.149.2.351
Fazekas, F., Kleinert, R., Offenbacher, H., Payer, F., Schmidt, R., Kleinert, G., et al. (1991). The morphologic correlate of incidental punctate white matter hyperintensities on MR images. Am. J. Neuroradiol. 12, 915–921.
Feng, C., Fang, M., Xu, Y., Hua, T., and Liu, X.-Y. (2014). Microbleeds in late-life depression: comparison of early- and late-onset depression. Biomed Res. Int. 2014:682092. doi: 10.1155/2014/682092
Fiedorowicz, J. G., Palagummi, N. M., Forman-Hoffman, V. L., Miller, D. D., and Haynes, W. G. (2008). Elevated prevalence of obesity, metabolic syndrome, and cardiovascular risk factors in bipolar disorder. Ann. Clin. Psychiatry 20, 131–137. doi: 10.1080/10401230802177722
Firbank, M. J., Lloyd, A. J., Ferrier, N., and O'Brien, J. T. (2004). A volumetric study of MRI signal hyperintensities in late-life depression. Am. J. Geriatr. Psychiatry 12, 606–612. doi: 10.1176/appi.ajgp.12.6.606
Firbank, M. J., Teodorczuk, A., van der Flier, W. M., Gouw, A. A., Wallin, A., Erkinjuntti, T., et al. (2012). Relationship between progression of brain white matter changes and late-life depression: 3-year results from the LADIS study. Br. J. Psychiatry 201, 40–45. doi: 10.1192/bjp.bp.111.098897
Foo, H., Mak, E., Yong, T. T., Wen, M. C., Chander, R. J., Au, W. L., et al. (2016). Progression of small vessel disease correlates with cortical thinning in Parkinson's disease. Parkinsonism Relat. Disord. 31, 34–40. doi: 10.1016/j.parkreldis.2016.06.019
Fujimoto, T., Takeuchi, K., Matsumoto, T., Fujita, S., Honda, K., Higashi, Y., et al. (2008). Metabolic changes in the brain of patients with late-onset major depression. Psychiatry Res. 164, 48–57. doi: 10.1016/j.pscychresns.2007.03.010
Gattringer, T., Enzinger, C., Ropele, S., Gorani, F., Petrovic, K. E., Schmidt, R., et al. (2012). Vascular risk factors, white matter hyperintensities and hippocampal volume in normal elderly individuals. Dement. Geriatr. Cogn. Disord. 33, 29–34. doi: 10.1159/000336052
Gaupp, R. (1905). Die depressionszustände des höheren lebensalters. Münch. Med. Wochenschr. 1531, 216–225.
Gildengers, A. G., Butters, M. A., Aizenstein, H. J., Marron, M. M., Emanuel, J., Anderson, S. J., et al. (2015). Longer lithium exposure is associated with better white matter integrity in older adults with bipolar disorder. Bipolar Disord. 17, 248–256. doi: 10.1111/bdi.12260
González, H. M., Tarraf, W., Whitfield, K., and Gallo, J. J. (2012). Vascular depression prevalence and epidemiology in the United States. J. Psychiatr. Res. 46, 456–461. doi: 10.1016/j.jpsychires.2012.01.011
Greenwald, B. S., Kramer-Ginsberg, E., Krishnan, K. R. R., Ashtari, M., Auerbach, C., and Patel, M. (1998). Neuroanatomic localization of magnetic resonance imaging signal hyperintensities in geriatric depression. Stroke 29, 613–617. doi: 10.1161/01.STR.29.3.613
Greenwald, B. S., Kramer-Ginsberg, E., Krishnan, K. R. R., Hu, J., Ashtari, M., Wu, H., et al. (2001). A controlled study of MRI signal hyperintensities in older depressed patients with and without hypertension. J. Am. Geratr. Soc. 49, 1218–1225. doi: 10.1046/j.1532-5415.2001.49240.x
Greenwald, B. S., Kramer-Ginsberg, E., Krishnan, R. R., Ashtari, M., Aupperle, P. M., and Patel, M. (1996). MRI signal hyperintensities in geriatric depression. Am. J. Psychiatry 153, 1212–1215.
Gunde, E., Blagdon, R., and Hajek, T. (2011). White matter hyperintensities. From medical comorbidities to bipolar disorders and back. Ann. Med. 43, 571–580. doi: 10.3109/07853890.2011.595733
Gunning-Dixon, F. M., Walton, M., Cheng, J., Acuna, J., Klimstra, S., Zimmerman, M. E., et al. (2010). MRI signal hyperintensities and treatment remission of geriatric depression. J. Affect. Disord. 126, 395–401. doi: 10.1016/j.jad.2010.04.004
Habes, M., Erus, G., Toledo, J. B., Zhang, T., Bryan, N., Launer, L. J., et al. (2016). White matter hyperintensities and imaging patterns of brain ageing in the general population. Brain 139, 1164–1179. doi: 10.1093/brain/aww008
Hajer, G. R., van der Graaf, Y., Olijhoek, J. K., Verhaar, M. C., and Visseren, F. L. J. (2007). Levels of homocysteine are increased in metabolic syndrome patients but are not associated with an increased cardiovascular risk, in contrast to patients without the metabolic syndrome. Heart 93, 216–220. doi: 10.1136/hrt.2006.093971
Harada, K., Matsuo, K., Nakashima, M., Hobara, T., Higuchi, N., Higuchi, F., et al. (2016). Disrupted orbitomedial prefrontal limbic network in individuals with later-life depression. J. Affect. Disord. 204, 112–119. doi: 10.1016/j.jad.2016.06.031
Hedges, L. V. (1981). Distribution theory for glass's estimator of effect size and related estimators. J. Educ. Behav. Stat. 6, 107–128.
Hendrie, H. C., Farlow, M. R., Austrom, M. G., Edwards, M. K., and Williams, M. A. (1989). Foci of increased T2 signal intensity on brain MR scans of healthy elderly subjects. J. Neuroradiol. 10, 703–707.
Herrmann, L. L., Le Masurier, M., and Ebmeier, K. P. (2008). White matter hyperintensities in late life depression: a systematic review. J. Neurol. Neurosurg. Psychiatr. 79, 619–624. doi: 10.1136/jnnp.2007.124651
Hickie, I., Naismith, S., Ward, P. B., Scott, E., Mitchell, P., Wilhelm, K., et al. (2005). Vascular risk and low serum B12 predict white matter lesions in patients with major depression. J. Affect. Disord. 85, 327–332. doi: 10.1016/j.jad.2004.11.003
Hogervorst, E., Ribeiro, H. M., Molyneux, A., Budge, M., and Smith, A. D. (2002). Plasma homocysteine levels, cerebrovascular risk factors, and cerebral white matter changes (leukoaraiosis) in patients with Alzheimer disease. Arch. Neurol. 59, 787–793. doi: 10.1001/archneur.59.5.787
Hong, E. D., Taylor, W. D., McQuoid, D. R., Potter, G. G., Payne, M. E., Ashley-Koch, A., et al. (2009). Influence of the MTHFR C677T polymorphism on magnetic resonance imaging hyperintensity volume and cognition in geriatric depression. Am. J. Geriatr. Psychiatry 17, 847–855. doi: 10.1097/JGP.0b013e3181aad5b2
Iosifescu, D. V., Papakostas, G. I., Lyoo, I. K., Lee, H. K., Renshaw, P. F., Alpert, J. E., et al. (2005). Brain MRI white matter hyperintensities and one-carbon cycle metabolism in non-geriatric outpatients with major depressive disorder (Part I). Psychiatry Res. 140, 291–299. doi: 10.1016/j.pscychresns.2005.09.003
Iosifescu, D. V., Renshaw, P. F., Lyoo, I. K., Lee, H. K., Perlis, R. H., Papakostas, G. I., et al. (2006). Brain white-matter hyperintensities and treatment outcome in major depressive disorder. Br. J. Psychiatry 188, 180–185. doi: 10.1192/bjp.188.2.180
Jellinger, K. A. (2011). Neuropathological analysis of brain lesions in late-onset depression. Neuropathol. Appl. Neurobiol. 37, 560–562. doi: 10.1111/j.1365-2990.2011.01191.x
Johnson, A. D., McQuoid, D. R., Steffens, D. C., Payne, M. E., Beyer, J. L., and Taylor, W. D. (2017). Effects of stressful life events on cerebral white matter hyperintensity progression. Int. J. Geriatr. Psychiatry 32, e10–e17. doi: 10.1002/gps.4644
Kearney, P. M., Whelton, M., Reynolds, K., Muntner, P., Whelton, P. K., and He, J. (2005). Global burden of hypertension: analysis of worldwide data. Lancet 365, 217–223. doi: 10.1016/S0140-6736(05)17741-1
Kempton, M. J., Geddes, J. R., Ettinger, U., Williams, S. C., and Grasby, P. M. (2008). Meta-analysis, database, and meta-regression of 98 structural imaging studies in bipolar disorder. Arch. Gen. Psychiatry 65, 1017–1032. doi: 10.1001/archpsyc.65.9.1017
Kempton, M. J., Salvador, Z., Munafò, M. R., Geddes, J. R., Simmons, A., Frangou, S., et al. (2011). Structural neuroimaging studies in major depressive disorder. meta-analysis and comparison with bipolar disorder. Arch. Gen. Psychiatry 68, 675–690. doi: 10.1001/archgenpsychiatry.2011.60
Keshavan, M. S., Mulsant, B. H., Sweet, R. A., Pasternak, R., Zubenko, G. S., and Krishnan, R. R. (1996). MRI changes in schizophrenia in late life: a preliminary controlled study. Psychiatry Res. 60, 117–123. doi: 10.1016/0165-178102867-3
Kieseppä, T., Mäntylä, R., Tuulio-Henriksson, A., Luoma, K., Mantere, O., Ketokivi, M., et al. (2014). White matter hyperintensities and cognitive performance in adult patients with bipolar I, bipolar II, and major depressive disorders. Eur. Psychiatry 29, 226–232. doi: 10.1016/j.eurpsy.2013.08.002
Kim, J. H., Kim, J. W., Ko, Y. H., Choi, C. U., Na, J. O., Kim, E. J., et al. (2010). Coronary endothelial dysfunction associated with a depressive mood in patients with atypical angina but angiographically normal coronary artery. Int. J. Cardiol. 143, 154–157. doi: 10.1016/j.ijcard.2009.02.004
Kim, J. M., Stewart, R., Kim, S. W., Yang, S. J., Shin, I. S., and Yoon, J. S. (2008). Predictive value of folate, vitamin B12 and homocysteine levels in late-life depression. Br. J. Psychiatry 192, 268–274. doi: 10.1192/bjp.bp.107.039511
Kirkpatrick, J. B., and Hayman, L. A. (1987). White-matter lesions in MR imaging of clinically healthy brains of elderly subjects: possible pathologic basis. Radiology 162, 509–511.
Kloppenborg, R. P., Nederkoorn, P. J., Geerlings, M. I., and van den Berg, E. (2014). Presence and progression of white matter hyperintensities and cognition: a meta-analysis. Neurology 82, 2127–2138. doi: 10.1212/WNL.0000000000000505
Köhler, S., Ashton, C. H., Marsh, R., Thomas, A. J., Barnett, N. A., and O'Brien, J. T. (2011). Electrophysiological changes in late life depression and their relation to structural brain changes. Int. Psychogeriatr. 23, 141–148. doi: 10.1017/S1041610210001250
Köhler, S., Thomas, A. J., Lloyd, A., Barber, R., Almeida, O. P., and O'Brien, J. T. (2010). White matter hyperintensities, cortisol levels, brain atrophy and continuing cognitive deficits in late-life depression. Br. J. Psychiatry 196, 143–149. doi: 10.1192/bjp.bp.109.071399
Kovacic, J. C., Moreno, P., Nabel, E. G., Hachinski, V., and Fuster, V. (2011). Cellular senescence, vascular disease, and aging. Part 2 of a 2-part review: clinical vascular disease in the elderly. Circulation 123, 1900–1910. doi: 10.1161/CIRCULATIONAHA.110.009118
Kramer-Ginsberg, E., Greenwald, B. S., Krishnan, K. R. R., Christiansen, B., Hu, J., Ashtari, M., et al. (1999). Neuropsychological functioning and MRI signal hyperintensities in geriatric depression. Am. J. Psychiatry 156, 438–444.
Krishnan, K. R., Hays, J. C., and Blazer, D. G. (1997). MRI-defined vascular depression. Am. J. Psychiatry 154, 497–501.
Krishnan, K. R., Taylor, W. D., McQuoid, D. R., MacFall, J. R., Payne, M. E., Provenzale, J. M., et al. (2004). Clinical characteristics of magnetic resonance imaging-defined subcortical ischemic depression. Biol. Psychiatry 55, 390–397. doi: 10.1016/j.biopsych.2003.08.014
Krishnan, M. S., O'Brien, J. T., Firbank, M. J., Pantoni, L., Carlucci, G., Erkinjuntti, T., et al. (2006). Relationship between periventricular and deep white matter lesions and depressive symptoms in older people. The LADIS study. Int. J. Geriatr. Psychiatry 21, 983–989. doi: 10.1002/gps.1596
Kumar, A., Bilker, W., Jin, Z., and Udupa, J. (2000). Atrophy and high intensity lesions complementary: neurobiological mechanisms in late-life major depression. Neuropsychopharmacology 22, 264–274. doi: 10.1016/S0893-133X(99)00124-4
Lee, S. H., Payne, M. E., Steffens, D. C., McQuoid, D. R., Lai, T. J., Provenzale, J. M., et al. (2003). Subcortical lesion severity and orbitofrontal cortex volume in geriatric depression. Biol. Psychiatry 54, 529–533. doi: 10.1016/S0006-3223(03)00063-5
Lentz, S. R. (2005). Mechanisms of homocysteine-induced atherothrombosis. J. Thromb. Haemost. 3, 1646–1654. doi: 10.1111/j.1538-7836.2005.01364.x
Lenze, E., Cross, D., McKeel, D., Neuman, R. J., and Sheline, Y. I. (1999). White matter hyperintensities and gray matter lesions in physically healthy depressed subjects. Am. J. Psychiatry 156, 1602–1607. doi: 10.1176/ajp.156.10.1602
Lesser, I. M., Boone, K. B., Mehringer, C. M., Wohl, M. A., Miller, B. L., and Berman, N. G. (1996). Cognition and white matter hyperintensities in older depressed patients. Am. J. Psychiatry 15, 1280–1287.
Li, J. Q., Tan, L., Wang, H. F., Tan, M. S., Tan, L., Xu, W., et al. (2016). Risk factors for predicting progression from mild cognitive impairment to Alzheimer's disease: a systematic review and meta-analysis of cohort studies. J. Neurol. Neurosurg. Psychiatr. 87, 476–484. doi: 10.1136/jnnp-2014-310095
Liao, W., Wang, Z., Zhang, X., Shu, H., Wang, Z., Liu, D., et al. (2017). Cerebral blood flow changes in remitted early- and late-onset depression patients. Oncotarget 8, 76214–76222. doi: 10.18632/oncotarget.19185
Lin, H. F., Kuo, Y. T., Chiang, I. C., Chen, H. M., and Chen, C. S. L. (2005). Structural abnormality on brain magnetic resonance imaging in late-onset major depressive disorder. Kaohsiung J. Med. Sci. 21, 405–411. doi: 10.1016/S1607-551X(09)70142-1
Liu, R., Yue, Y., Hou, Z., Yuan, Y., and Wang, Q. (2018). Risk factors associated with cognitions for late-onset depression based on anterior and posterior default mode sub-networks. J. Affect. Disord. 235, 544–550. doi: 10.1016/j.jad.2018.04.065
Lloyd, A. J., Ferrier, I. N., Barber, R., Gholkar, A., Young, A. H., and O'Brien, J. T. (2004). Hippocampal volume change in depression. Late- and early-onset illness compared. Br. J. Psychiatry 184, 488–495. doi: 10.1192/bjp.184.6.488
Lloyd, A. J., Moore, P. B., Cousins, D. A., Thompson, J. M., McAllister, V. L., Hughes, J. H., et al. (2009). White matter lesions in euthymic patients with bipolar disorder. Acta Psychiatr. Scand. 120, 481–491. doi: 10.1111/j.1600-0447.2009.01416.x
Longstreth, W. T., Manolio, T. A., Arnold, A., Burke, G. L., Bryan, N., Jungreis, C. A., et al. (1996). Clinical correlates of white matter findings on cranial magnetic resonance imaging of 3301 elderly people. The Cardiovascular Health Study. Stroke 27, 1274–1282. doi: 10.1161/01.STR.27.8.1274
Madsen, K., Hasselbalch, B. J., Frederiksen, K. S., Haahr, M. E., Gade, A., Law, I., et al. (2012). Lack of association between prior depressive episodes and cerebral 11CPiB binding. Neurobiol. Aging 33, 2334–2342. doi: 10.1016/j.neurobiolaging.2011.11.021
Mahon, K., Burdick, K. E., and Szeszko, P. R. (2010). A role for white matter abnormalities in the pathophysiology of bipolar disorder. Neurosci. Biobehav. Rev. 34, 533–554. doi: 10.1016/j.neubiorev.2009.10.012
Mak, E., Colloby, S. J., Thomas, A., and O'Brien, J. T. (2016). The segregated connectome of late-life depression: a combined cortical thickness and structural covariance analysis. Neurobiol. Aging 48, 212–221. doi: 10.1016/j.neurobiolaging.2016.08.013
Mäntylä, R., Erkinjuntti, T., Salonen, O., Aronen, H. J., Peltonen, T., Pohjasvaara, T., et al. (1997). Variable agreement between visual rating scales for white matter hyperintensities on MRI. Comparison of 13 rating scales in a poststroke cohort. Stroke 28, 1614–1623.
Marín, J. (1995). Age-related changes in vascular responses. A review. Mech. Ageing Dev. 79, 71–114. doi: 10.1016/0047-6374(94)01551-V
McDonald, W. M., Krishnan, K. R. R., Doraiswamy, P. M., and Blazer, D. G. (1991). Occurrence of subcortical hyperintensities in elderly subjects with mania. Psychiatry Res. Neuroimaging 40, 211–220. doi: 10.1016/0925-4927(91)90013-G
McDonald, W. M., Tupler, L. A., Marsteller, F. A., Figiel, G. S., DiSouza, S., Nemeroff, C. B., et al. (1999). Hyperintense lesions on magnetic resonance images in bipolar disorder. Biol. Psychiatry 45, 965–971. doi: 10.1016/S0006-3223(98)00341-2
Miller, D. S., Kumar, A., Yousem, D. M., and Gottlieb, G. L. (1994). MRI high-intensity signals in late-life depression and Alzheimer's disease: a comparison of subjects without major vascular risk factors. Am. J. Geriatr. Psychiatry 2, 332–337. doi: 10.1097/00019442-199402040-00008
Mokwa, I. D. (2013). Hyperintensien bei Depression, Bipolarer Störung und Schizophrenie: Eine Metaanalyse. Diploma thesis. Westfälische Wilhelms-Universität, Münster.
Moore, P. B., Shepherd, D. J., Eccleston, D., Macmillan, I. C., Goswami, U., McAllister, V. L., et al. (2001). Cerebral white matter lesions in bipolar affective disorder: relationship to outcome. Br. J. Psychiatry 178, 172–176. doi: 10.1192/bjp.178.2.172
Müller, N., Schwarz, M. J., Dehning, S., Douhe, A., Cerovecki, A., Goldstein-Müller, B., et al. (2006). The cyclooxygenase-2 inhibitor celecoxib has therapeutic effects in major depression: results of a double-blind, randomized, placebo controlled, add-on pilot study to reboxetine. Mol. Psychiatry 11, 680–684. doi: 10.1038/sj.mp.4001805
Murata, T., Kimura, H., Omori, M., Kado, H., Kosaka, H., Iidaka, T., et al. (2001). MRI white matter hyperintensities, (1)H-MR spectroscopy and cognitive function in geriatric depression: a comparison of early- and late-onset cases. Int. J. Geriatr. Psychiatry 16, 1129–1135. doi: 10.1002/gps.501
Nagai, K., Kozaki, K., Sonohara, K., Akishita, M., and Toba, K. (2011). Relationship between interleukin-6 and cerebral deep white matter and periventricular hyperintensity in elderly women. Geriatr. Gerontol. Int. 11, 328–332. doi: 10.1111/j.1447-0594.2010.00686.x
Nortje, G., Stein, D. J., Radua, J., Mataix-Cols, D., and Horn, N. (2013). Systematic review and voxel-based meta-analysis of diffusion tensor imaging studies in bipolar disorder. J. Affect. Disord. 150, 192–200. doi: 10.1016/j.jad.2013.05.034
O'Brien, J., Desmond, P., Ames, D., Schweitzer, I., Harrigan, S., and Tress, B. (1996). A magnetic resonance imaging study of white matter lesions in depression and Alzheimer's disease. Br. J. Psychiatry 168, 477–485. doi: 10.1192/bjp.168.4.477
Oh, B. H., and Cheon, J. S. (2004). White matter lesions on magnetic resonance imaging in late-life depression. Psychogeriatrics 4, 72–81. doi: 10.1111/j.1479-8301.2004.00054.x
Paranthaman, R., Greenstein, A. S., Burns, A. S., Cruickshank, J. K., Heagerty, A. M., Jackson, A., et al. (2010). Vascular function in older adults with depressive disorder. Biol. Psychiatry, 68, 133–139. doi: 10.1016/j.biopsych.2010.04.017
Patel, M. J., Boada, F. E., Price, J. C., Sheu, L. K., Tudorascu, D. L., Reynolds Iii, C. F., et al. (2012). Association of small vessel ischemic white matter changes with BOLD fMRI imaging in the elderly. Psychiatry Res. 204, 117–122. doi: 10.1016/j.pscychresns.2012.09.006
Payne, M. E., Pierce, C. W., McQuoid, D. R., Steffens, D. C., and Anderson, J. J. B. (2013). Serum ionized calcium may be related to white matter lesion volumes in older adults: a pilot study. Nutrients 5, 2192–2205. doi: 10.3390/nu5062192
Penninx, B. W., Kritchevsky, S. B., Yaffe, K., Newman, A. B., Simonsick, E. M., Rubin, S., et al. (2003). Inflammatory markers and depressed mood in older persons: results from the health, aging and body composition study. Biol. Psychiatry 54, 566–572. doi: 10.1016/S0006-3223(02)01811-5
Permoda-Osip, A., Dorszewska, J., Skibinska, M., Chlopocka-Wozniak, M., and Rybakowski, J. K. (2013). Hyperhomocysteinemia in bipolar depression: clinical and biochemical correlates. Neuropsychobiology 68, 193–196. doi: 10.1159/000355292
Pillai, J. J., Friedman, L., Stuve, T. A., Trinidad, S., Jesberger, J. A., Lewin, J. S., et al. (2002). Increased presence of white matter hyperintensities in adolescent patients with bipolar disorder. Psychiatry Res. Neuroimaging 114, 51–56. doi: 10.1016/S0925-4927(01)00129-9
Pimontel, M. A., Reinlieb, M. E., Johnert, L. C., Garcon, E., Sneed, J. R., and Roose, S. P. (2013). The external validity of MRI-defined vascular depression. Am. J. Geriatr. Psychiatry 21, S66–S67. doi: 10.1016/j.jagp.2012.12.086
Pompili, M., Serafini, G., Innamorati, M., Venturini, P., Fusar-Poli, P., Sher, L., et al. (2013). Agomelatine, a novel intriguing antidepressant option enhancing neuroplasticity: a critical review. World J. Biol. Psychiatry 14, 412–431. doi: 10.3109/15622975.2013.765593
Portet, F., Brickman, A. M., Stern, Y., Scarmeas, N., Muraskin, J., Provenzano, F. A., et al. (2012). Metabolic syndrome and localization of white matter hyperintensities in the elderly population. Alzheimer's Dement. 8(Suppl. 5), S88–S95.e1. doi: 10.1016/j.jalz.2011.11.007
Potter, G. G., Blackwell, A. D., McQuoid, D. R., Payne, M. E., Steffens, D. C., Sahakian, B. J., et al. (2007). Prefrontal white matter lesions and prefrontal task impersistence in depressed and nondepressed elders. Neuropsychopharmacology 32, 2135–2142. doi: 10.1038/sj.npp.1301339
Qiu, W. Q., Himali, J. J., Wolf, P. A., DeCarli, D. C., Beiser, A., and Au, R. (2017). Effects of white matter integrity and brain volumes on late life depression in the Framingham Heart Study. Int. J. Geriatr. Psychiatry 32, 214–221. doi: 10.1002/gps.4469
Rabins, P. V., Pearlson, G. D., Aylward, E., Kumar, A. J., and Dowell, K. (1991). Cortical magnetic resonance imaging changes in elderly inpatients with major depression. Am. J. Psychiatry 148, 617–620.
Radanovic, M., Pereira, F. R., Stella, F., Aprahamian, I., Ferreira, L. K., Forlenza, O. V., et al. (2013). White matter abnormalities associated with Alzheimer's disease and mild cognitive impairment: a critical review of MRI studies. Expert Rev. Neurother. 13, 483–493. doi: 10.1586/ern.13.45
Rapp, M. A., Dahlman, K., Sano, M., Grossman, H. T., Haroutunian, V., and Gorman, J. M. (2005). Neuropsychological differences between late-onset and recurrent geriatric major depression. Am. J. Psychiatry 162, 691–698. doi: 10.1176/appi.ajp.162.4.691
Rej, S., Butters, M. A., Aizenstein, H. J., Begley, A., Tsay, J., Reynolds, C. F., et al. (2014). Neuroimaging and neurocognitive abnormalities associated with bipolar disorder in old age. Int. J. Geriatr. Psychiatry 29, 421–427. doi: 10.1002/gps.4021
Rolstad, S., Abé, C., Olsson, E., Eckerström, C., and Landén, M. (2016). Cognitive reserve lessens the burden of white matter lesions on executive functions in bipolar disorder. Psychol. Med. 46, 3095–3104. doi: 10.1017/S0033291716001641
Rybakowski, J. K., Wykretowicz, A., Heymann-Szlachcinska, A., and Wysocki, H. (2006). Impairment of endothelial function in unipolar and bipolar depression. Biol. Psychiatry 60, 889–891. doi: 10.1016/j.biopsych.2006.03.025
Sachdev, P. (2004). Homocysteine, cerebrovascular disease and brain atrophy. J. Neurol. Sci. 226, 25–29. doi: 10.1016/j.jns.2004.09.006
Sachdev, P., Parslow, R., Salonikas, C., Lux, O., Wen, W., Kumar, R., et al. (2004). Homocysteine and the brain in midadult life: evidence for an increased risk of leukoaraiosis in men. Arch. Neurol. 61, 1369–1376. doi: 10.1001/archneur.61.9.1369
Santos, M., Gold, G., Kövari, E., Herrmann, F. R., Hof, P. R., Bouras, C., et al. (2010). Neuropathological analysis of lacunes and microvascular lesions in late-onset depression. Neuropathol. Appl. Neurobiol. 36, 661–672. doi: 10.1111/j.1365-2990.2010.01101.x
Santos, M., Xekardaki, A., Kövari, E., Gold, G., Bouras, C., and Giannakopoulos, P. (2012). Microvascular pathology in late-life depression. J. Neurol. Sci. 322, 46–49. doi: 10.1016/j.jns.2012.05.048
Sassi, R. B., Brambilla, P., Nicoletti, M., Mallinger, A. G., Frank, E., Kupfer, D. J., et al. (2003). White matter hyperintensities in bipolar and unipolar patients with relatively mild-to-moderate illness severity. J. Affect. Disord. 77, 237–245. doi: 10.1016/S0165-0327(02)00170-2
Scheltens, P., Barkhof, F., Leys, D., Pruvo, J. P., Nauta, J. J., Vermersch, P., et al. (1993). A semiquantative rating scale for the assessment of signal hyperintensities on magnetic resonance imaging. J. Neurol. Sci. 114, 7–12. doi: 10.1016/0022-510X(93)90041-V
Scheltens, P., Barkhof, F., Leys, D., Wolters, E. C., Ravid, R., and Kamphorst, W. (1995). Histopathologic correlates of white matter changes on MRI in Alzheimer's disease and normal aging. Neurology 45, 883–888.
Schwichtenberg, J., Al-Zghloul, M., Kerl, H. U., Wenz, H., Hausner, L., Frölich, L., et al. (2017). Late-onset major depression is associated with age-related white matter lesions in the brainstem. Int. J. Geriatr. Psychiatry 32, 446–454. doi: 10.1002/gps.4487
Sexton, C. E., Allan, C. L., Le Masurier, M., McDermott, L. M., Kalu, U. G., Herrmann, L. L., et al. (2012). Magnetic resonance imaging in late-life depression: multimodal examination of network disruption. Arch. Gen. Psychiatry 69, 680–689. doi: 10.1001/archgenpsychiatry.2011.1862
Shaw, J. E., Sicree, R. A., and Zimmet, P. Z. (2010). Global estimates of the prevalence of diabetes for 2010 and 2030. Diabetes Res. Clin. Pract. 87, 4–14. doi: 10.1016/j.diabres.2009.10.007
Silverstone, T., McPherson, H., Li, Q., and Doyle, T. (2003). Deep white matter hyperintensities in patients with bipolar depression, unipolar depression and age-matched control subjects. Bipolar Disord. 5, 53–57. doi: 10.1034/j.1399-5618.2003.01208.x
Smith, P. J., Blumenthal, J. A., Babyak, M. A., Doraiswamy, P. M., Hinderliter, A., Hoffman, B. M., et al. (2009). Intima-media thickness and age of first depressive episode. Biol. Psychol. 80, 361–364. doi: 10.1016/j.biopsycho.2008.11.001
Sneed, J. R., Culang-Reinlieb, M. E., Brickman, A. M., Gunning-Dixon, F. M., Johnert, L., Garcon, E., et al. (2011). MRI signal hyperintensities and failure to remit following antidepressant treatment. J. Affect. Disord. 135 , 315–320. doi: 10.1016/j.jad.2011.06.052
Sneed, J. R., Rindskopf, D., Steffens, D. C., Krishnan, K. R., and Roose, S. P. (2008). The vascular depression subtype: evidence of internal validity. Biol. Psychiatry 64, 491–497. doi: 10.1016/j.biopsych.2008.03.032
Steffens, D. C., Conway, C. R., Dombeck, C. B., Wagner, H. R., Tupler, L. A., and Weiner, R. D. (2001). Severity of subcortical gray matter hyperintensity predicts ECT response in geriatric depression. J. ECT 17, 45–49. doi: 10.1097/00124509-200103000-00009
Steffens, D. C., and Krishnan, K. R. (1998). Structural neuroimaging and mood disorders. Recent findings, implications for classification, and future directions. Biol. Psychiatry 43, 705–712. doi: 10.1016/S0006-3223(98)00084-5
Sterne, J. A. C., and Egger, M. (2005). “Regression methods to detect publication and other bias in meta-analysis,” in Publication Bias in Meta-Analysis – Prevention, Assessment and Adjustments, eds H. R. Rothstein, A. J. Sutton, and M. Borenstein (Chichester: John Wiley & Sons Ltd.) 99–110. doi: 10.1002/0470870168.ch6
Sutton-Tyrrell, K., Bostom, A., Selhub, J., and Zeigler-Johnson, C. (1997). High homocysteine levels are independently related to isolated systolic hypertension in older adults. Circulation 96, 1745–1749. doi: 10.1161/01.CIR.96.6.1745
Takahashi, K., and Mikuni, M. (2012). Augmentation therapy with cilostazol for the intractable geriatric major depressive disorder patients with deep white matter hyperintensities on T2-weighted brain MRI. Psychiatr. Neurol. Jap. 114, 297–302.
Tamashiro, J. H., Zung, S., Zanetti, M. V., de Castro, C. C., Vallada, H., Busatto, G. F., et al. (2008). Increased rates of white matter hyperintensities in late-onset bipolar disorder. Bipolar Disord. 10, 765–775. doi: 10.1111/j.1399-5618.2008.00621.x
Tawakol, A., Omland, T., Gerhard, M., Wu, J. T., and Creager, M. A. (1997). Hyperhomocyst(e)inemia is associated with impaired endothelium-dependent vasodilation in humans. Circulation 95, 1119–1121. doi: 10.1161/01.CIR.95.5.1119
Taylor, W. D., Aizenstein, H. J., and Alexopoulos, G. S. (2013a). The vascular depression hypothesis: mechanisms linking vascular disease with depression. Mol. Psychiatry 18, 963–974. doi: 10.1038/mp.2013.20
Taylor, W. D., MacFall, J. R., Payne, M. E., McQuoid, D. R., Steffens, D. C., Provenzale, J. M., et al. (2005). Greater MRI lesion volumes in elderly depressed subjects than in control subjects. Psychiatry Res. 139, 1–7. doi: 10.1016/j.pscychresns.2004.08.004
Taylor, W. D., MacFall, J. R., Payne, M. E., McQuoid, D. R., Steffens, D. C., Provenzale, J. M., et al. (2007). Orbitofrontal cortex volume in late life depression: influence of hyperintense lesions and genetic polymorphisms. Psychol. Med. 37, 1763–1773. doi: 10.1017/S0033291707000128
Taylor, W. D., McQuoid, D. R., Payne, M. E., Zannas, A. S., MacFall, J. R., and Steffens, D. C. (2014). Hippocampus atrophy and the longitudinal course of late-life depression. Am. J. Geriatr. Psychiatry 22, 1504–1512. doi: 10.1016/j.jagp.2013.11.004
Taylor, W. D., Payne, M. E., Krishnan, K. R., Wagner, H. R., Provenzale, J. M., Steffens, D. C., et al. (2001). Evidence of white matter tract disruption in MRI hyperintensities. Biol. Psychiatry 50, 179–183. doi: 10.1016/S0006-3223(01)01160-X
Taylor, W. D., Zhao, Z., Ashley-Koch, A., Payne, M. E., Steffens, D. C., Krishnan, R. R., et al. (2013b). Fiber tract-specific white matter lesion severity Findings in late-life depression and by AGTR1 A1166C genotype. Hum. Brain Mapp. 34, 295–303. doi: 10.1002/hbm.21445
Taylor, W. D., Züchner, S., McQuoid, D. R., Payne, M. E., MacFall, J. R., Steffens, D. C., et al. (2008). The brain-derived neurotrophic factor VAL66MET polymorphism and cerebral white matter hyperintensities in late-life depression. Am. J. Geriatr. Psychiatry 16, 263–271. doi: 10.1097/JGP.0b013e3181591c30
Teodorczuk, A., Firbank, M. J., Pantoni, L., Poggesi, A., Erkinjuntti, T., Wallin, A., et al. (2012). Relationship between baseline white-matter changes and development of late-life depressive symptoms: 3-year results from the LADIS study. Psychol. Med. 40, 603–610. doi: 10.1017/S0033291709990857
Teodorczuk, A., O'Brien, J. T., Firbank, M. J., Pantoni, L., Poggesi, A., Erkinjuntti, T., et al. (2007). White matter changes and late-life depressive symptoms: longitudinal study. Br. J. Psychiatry 91, 212–217. doi: 10.1192/bjp.bp.107.036756
Thomas, A. J., O'Brien, J. T., Barber, R., McMeekin, W., and Perry, R. (2003). A neuropathological study of periventricular white matter hyperintensities in major depression. J. Affect. Disord. 76, 49–54. doi: 10.1016/S0165-0327(02)00064-2
Thomas, A. J., Perry, R., Barber, R., Kalaria, R. N., and O'Brien, J. T. (2002). Pathologies and pathological mechanisms for white matter hyperintensities in depression. Ann. N. Y. Acad. Sci. 977, 333–339. doi: 10.1111/j.1749-6632.2002.tb04835.x
Tighe, S. K., Reading, S. A., Rivkin, P., Caffo, B., Schweizer, B., Pearlson, G., et al. (2012). Total white matter hyperintensity volume in bipolar disorder patients and their healthy relatives. Bipolar Disord. 14, 888–893. doi: 10.1111/bdi.12019
Tseng, Y.-L., Chang, Y.-Y., Liu, J.-S., Su, C.-S., Lai, S.-L., and Lan, M.-Y. (2009). Association of plasma homocysteine concentration with cerebral white matter hyperintensity on magnetic resonance images in stroke patients. J. Neurol. Sci. 284, 36–39. doi: 10.1016/j.jns.2009.03.030
Tupler, L. A., Krishnan, K. R. R., McDonald, W. M., Dombeck, C. B., D'Souza, S., and Steffens, D. C. (2002). Anatomic location and laterality of MRI signal hyperintensities in late-life depression. J. Psychosom. Res. 53, 665–676. doi: 10.1016/S0022-3999(02)00425-7
van Agtmaal, M. J. M., Houben, J. H. M., Pouwer, F., Stehouwer, C. D. A., and Schram, M. T. (2017). Association of microvascular dysfunction with late-life depression. a systematic review and meta-analysis. JAMA Psychiatry 4, 729–739. doi: 10.1001/jamapsychiatry.2017.0984
Van den Noortgate, W., López-López, J. A., Marín-Martínez, F., and Sánchez-Meca, J. (2013). Three-level meta-analysis of dependent effect sizes. Behav. Res. Methods 45, 576–594. doi: 10.3758/s13428-012-0261-6
Vasudev, A., Saxby, B. K., O'Brien, J. T., Colloby, S. J., Firbank, M. J., Brooker, H., et al. (2012). Relationship between cognition, magnetic resonance white matter hyperintensities, and cardiovascular autonomic changes in late-life depression. Am. J. Geriatr. Psychiatry 20, 691–699. doi: 10.1097/JGP.0b013e31824c0435
Vernooij, M. W., de Groot, M., van der Lugt, A., Ikram, M. A., Krestin, G. P., Hofman, A., et al. (2008). White matter atrophy and lesion formation explain the loss of structural integrity of white matter in aging. NeuroImage 43, 470–477. doi: 10.1016/j.neuroimage.2008.07.052
Versace, A., Almeida, J. R., Quevedo, K., Thompson, W. K., Terwilliger, R. A., Hassel, S., et al. (2010). Right orbitofrontal corticolimbic and left corticocortical white matter connectivity differentiate bipolar and unipolar depression. Biol. Psychiatry 68, 560–567. doi: 10.1016/j.biopsych.2010.04.036
Versluis, C. E., van der Mast, R. C., van Buchem, M. A., Bollen, E. L. E. M., Blauw, G. J., Eekhof, J. A. H., et al. (2006). Progression of cerebral white matter lesions is not associated with development of depressive symptoms in elderly subjects at risk of cardiovascular disease. The PROSPER study. Int. J. Geriatr. Psychiatry 21, 375–381. doi: 10.1002/gps.1477
Videbech, P. (1997). MRI findings in patients with affective disorder: a meta-analysis. Acta Psychiatr. Scand. 96, 157–168.
Viechtbauer, W. (2010). Conducting meta-analyses in R with the metafor package. J. Stat. Soft. 36, 5–15. doi: 10.18637/jss.v036.i03.
Wang, L., Leonards, C. O., Sterzer, P., and Ebinger, M. (2014). White matter lesions and depression: a systematic review and metaanalysis. J. Psychiatr. Res. 56, 56–64. doi: 10.1016/j.jpsychires.2014.05.005
Wassmann, S., Stumpf, M., Strehlow, K., Schmid, A., Schieffer, B., Böhm, M., et al. (2004). Interleukin-6 induces oxidative stress and endothelial dysfunction by overexpression of the angiotensin II type 1 receptor. Circ. Res. 94, 534–541. doi: 10.1161/01.RES.0000115557.25127.8D
Weber, K., Giannakopoulos, P., Delaloye, C., de Bilbao, F., Moy, G., Moussa, A., et al. (2010). Volumetric MRI changes, cognition and personality traits in old age depression. J. Affect. Dis. 124, 275–282. doi: 10.1016/j.jad.2009.11.016
World Health Organization (1992). The ICD-10 Classification of Mental and Behavioural Disorders: Clinical Descriptions and Diagnostic Guidelines. Geneva: World Health Organization.
Wu, R H, Feng, C., Xu, Y., Hua, T., Liu, X. Y., and Fang, M. (2014). Late-onset depression in the absence of stroke. Associated with silent brain infarctions, microbleeds and lesion locations. Int. J. Med. Sci. 11, 587–592. doi: 10.7150/ijms.8025
Xekardaki, A., Santos, M., Hof, P., Kövari, E., Bouras, C., and Giannakopoulos, P. (2012). Neuropathological substrates and structural changes in late-life depression. The impact of vascular burden. Acta Neuropathol. 124, 453–464. doi: 10.1007/s00401-012-1021-5
Yin, Z. G., Cui, M., Zhou, S. M., Yu, M. M., Li, R., and Zhou, H. D. (2014). Association between metabolic syndrome and white matter lesions in middle-aged and elderly patients. Eur. J. Neurol. 21, 1032–1039. doi: 10.1111/ene.12433
Zhu, G., Chu, S., Ding, D., and Zee, C.-S. (2014). White matter hyperintensities and related risk factors in Chinese normal aging: a community based study. Healthmed 8, 275–281.
Keywords: white matter hyperintensities (WMH), leukoaraiosis, major depressive disorder, bipolar disorder, depression, cerebrovascular pathology, hyperintense lesions
Citation: Salo KI, Scharfen J, Wilden ID, Schubotz RI and Holling H (2019) Confining the Concept of Vascular Depression to Late-Onset Depression: A Meta-Analysis of MRI-Defined Hyperintensity Burden in Major Depressive Disorder and Bipolar Disorder. Front. Psychol. 10:1241. doi: 10.3389/fpsyg.2019.01241
Received: 27 November 2018; Accepted: 10 May 2019;
Published: 31 May 2019.
Edited by:
Éric Laurent, Université Bourgogne Franche-Comté, FranceReviewed by:
Jin Li, Chinese Academy of Sciences, ChinaPanteleimon Giannakopoulos, Université de Genève, Switzerland
Copyright © 2019 Salo, Scharfen, Wilden, Schubotz and Holling. This is an open-access article distributed under the terms of the Creative Commons Attribution License (CC BY). The use, distribution or reproduction in other forums is permitted, provided the original author(s) and the copyright owner(s) are credited and that the original publication in this journal is cited, in accordance with accepted academic practice. No use, distribution or reproduction is permitted which does not comply with these terms.
*Correspondence: Katharina I. Salo, a2F0aGFyaW5hLnNhbG9AZ21haWwuY29t