- 1Université Clermont Auvergne, CNRS, LAPSCO, Clermont-Ferrand, France
- 2Université Grenoble Alpes, CNRS, Grenoble INP, LJK, Grenoble, France
- 3CEREMA, Equipe Recherche STI, Agence de Clermont-Ferrand, Clermont-Ferrand, France
Smartphones are particularly likely to elicit driver distraction with obvious negative repercussions on road safety. Recent selective attention models lead to expect that smartphones might be very effective in capturing attention due to their social reward history. Hence, individual differences in terms of Fear of Missing Out (FoMO) – i.e., of the apprehension of missing out on socially rewarding experiences – should play an important role in driver distraction. This factor has already been associated with self-reported estimations of greater attention paid to smartphones while driving, but the potential link between FoMO and smartphone-induced distraction has never been tested empirically. Therefore, we conducted a preliminary study to investigate whether FoMO would modulate attentional capture by reward distractors displayed on a smartphone. First, participants performed a classical visual search task in which neutral stimuli (colored circles) were associated with high or low social reward outcomes. Then, they had to detect a pedestrian or a roe deer in driving scenes with various levels of fog density. The social reward stimuli were displayed as distractors on the screen of a smartphone embedded in the pictures. The results showed a significant three-way interaction between FoMO, social reward distraction, and task difficulty. More precisely, under attention-demanding conditions (i.e., high-fog density), individual FoMO scores predicted attentional capture by social reward distractors, with longer reaction times (RTs) for high rather than low social reward distractors. These results highlight the importance to consider reward history and FoMO when investigating smartphone-based distraction. Limitations are discussed, notably regarding our sample characteristics (i.e., mainly young females) that might hamper the generalization of our findings to the overall population. Future research directions are provided.
Introduction
Drivers’ attentional failures are considered among the major contributing factors in road accidents (Klauer et al., 2006; Dingus et al., 2016). According to the taxonomy proposed by Regan et al. (2011), distraction occurs when the driver’s attention is focused on driving-irrelevant information, resulting in insufficient attention toward driving-related stimuli that are critical for safety. Besides, driver distraction might be caused by external information like billboards (e.g., Chattington et al., 2009; Bendak and Al-Saleh, 2010; Dukic et al., 2013; Belyusar et al., 2016; Gitelman et al., 2019) but also by in-vehicle devices (e.g., Strayer, 2015; Arexis et al., 2017; Noy et al., 2018). In the latter case, smartphones are considered as a game changing technology regarding driver distraction, due to their particularly strong prevalence in our societies, and daily activities (Srivastava, 2005; Strayer, 2015; Ward et al., 2017; Pew Research Center, 2018; CREDOC, 2019).
State of the Art
Interacting with a smartphone1 (e.g., reading or writing a message) can quite obviously lead to visual/manual distraction that impairs drivers’ performance (Caird et al., 2008, 2014; Dumitru et al., 2018; Ortiz et al., 2018). But smartphones might also produce cognitive distraction with comparable effects on drivers’ performance and road safety (Strayer, 2015) although the driver’s eyes are on the roadway and his/her hands are on the steering wheel (Strayer et al., 2003; Strayer and Drews, 2007). Indeed, in laboratory settings, it has been demonstrated that receiving a notification on a smartphone significantly disrupted performance on attention-demanding tasks, even when the participants did not directly interact with their device (Stothart et al., 2015). Above the temporary attentional capture produced by the notification, the authors argued that it might place a new prospective memory demand (i.e., waiting to respond, willingness to do so promptly) that could give rise to task-irrelevant thoughts (see also Waddell and Wiener, 2014). In the same vein, because smartphones allow us to interact with social media and “infotainment” systems, others authors argued that their mere presence might serve as a constant reminder of the broader social network that is potentially available (Thornton et al., 2014). In their study, the mere presence of participants’ own phones on their desktops negatively affected performance on attentional tasks, even though no incoming call nor message reception occurred during data collection. Accordingly, such a constant reminder of social network availability might also trigger mind wandering (Thornton et al., 2014; Stothart et al., 2015). Conjointly, other authors claimed that smartphones could produce cognitive distraction by draining attentional resources out of the task for the purpose of attentional control (Ward et al., 2017). Cognitive resources would indeed be recruited to inhibit both attentional capture and the (resulting) distracting thoughts generated by one’s smartphone. Therefore, such resources would no longer be available for attentional tasks, leading to poorer performance (but see also Hartmann et al. (2020) for null effects of smartphone presence on short-term and prospective memory). In sum, beyond their potential for visual/manual distraction, smartphones may also produce cognitive distraction and drain attentional resources out of the on-going task. Therefore, understanding attentional capture by smartphones appears crucial to understand driver distraction.
Classically, selective attention models relied on the interplay between bottom-up (e.g., saliency) and top-down factors (e.g., task set) to predict attentional capture by irrelevant distractors (Corbetta and Shulman, 2002; Fecteau and Munoz, 2006; Theeuwes, 2010; Zelinsky and Bisley, 2015). However, a third factor named selection history has emerged in more recent models of selective attention (Awh et al., 2012; Belopolsky, 2015; Luck et al., 2021). Past episodes of attentional selection would endow some stimuli with a particular “value” (through explicit or implicit learning), leading to lingering biases that affect future selection episodes above and beyond bottom-up and top-down factors (Theeuwes, 2018). More particularly, one class of phenomena that belongs to selection history is related to reward history (Anderson, 2015; Failing and Theeuwes, 2018; Theeuwes, 2018). Indeed, many studies have shown that stimuli (previously) associated with reward outcomes could trigger attentional capture in spite of being neither salient nor relevant in the task at hand (e.g., Hickey et al., 2010; Anderson et al., 2011a, b, 2017; Bourgeois et al., 2015; Bucker et al., 2015; Le Pelley et al., 2015; Munneke et al., 2015; Pearson et al., 2015; Anderson, 2016; Failing and Theeuwes, 2017).
We contend that the influence of smartphones on attention falls into the scope of reward history, and more precisely, of social reward history. First, users actually perceive the high reward value of their smartphone (Wilmer and Chein, 2016; Johannes et al., 2019), with social aspects being of particular importance (Özcan and Koçak, 2003; Srivastava, 2005; Waddell and Wiener, 2014; Sherman et al., 2016; Johannes et al., 2019). Moreover, studies have shown that smartphone use generates positive reinforcement learning (Turel and Serenko, 2012; Chen et al., 2019) and relies on neural regions involved in reward processing, social cognition, and attention (Sherman et al., 2016). Additionally, a large body of work has shown how people are extremely attached to their smartphone, as revealed by symptoms of behavioral addiction to their device (e.g., Walsh et al., 2008; Cheever et al., 2014; Clayton et al., 2015). However, it is worth noting that individual differences (e.g., impulsivity, reward-seeking behavior) also play an important role in smartphones’ influence on behavior (Brown et al., 2021) as well as in reward-based distraction (see Bourgeois et al., 2016; Failing and Theeuwes, 2018). In this framework, Fear of Missing Out (FoMO) is an emerging factor that has been shown to be associated with problematic internet and smartphone use in several studies (e.g., Elhai et al., 2016; Dempsey et al., 2019; Rozgonjuk et al., 2020; Wolniewicz et al., 2020). FoMO is defined as the pervasive apprehension that others might be having rewarding experiences from which one is absent, and is characterized by the desire to stay continually connected with what others are doing (Przybylski et al., 2013). Individuals reporting high levels of FoMO are more likely to want to stay constantly connected with others and are, therefore, more likely to engage with social media and technology (Przybylski et al., 2013). Accordingly, they reckon they are more prone to react to push notifications whereas people with lower levels of FoMO would be more able to resist being distracted by such stimuli (Rozgonjuk et al., 2019). Additionally, some studies have revealed a positive correlation between FoMO and driver distraction (Przybylski et al., 2013; Brown et al., 2021), indicating that the greater the FoMO the more frequently participants declare paying attention to their smartphone and reading/sending messages while driving.
Objective and Hypothesis
So far, studies that aimed at linking FoMO with distraction (e.g., Przybylski et al., 2013; Rozgonjuk et al., 2019; Al-Furaih and Al-Awidi, 2020; Brown et al., 2021) have relied exclusively on self-reported estimations of distraction. To our knowledge, no study has yet experimentally tested whether FoMO would predict the behavioral distraction elicited by a smartphone, notably in a driving context. Our main objective was therefore to put more directly to the test the influence of FoMO on smartphone-based distraction while driving. More precisely, our hypothesis was that FoMO would predict distraction by smartphones but only for information that have a high social reward value. Therefore, participants first performed a classic association phase (Anderson, 2016) in which the attentional selection of target neutral stimuli (i.e., colored circles with no particular selection history before the experiment) was paired with a high or low social reward (i.e., more or less positive social feedback manipulated through different ratios of neutral/smiling faces). Subsequently, they performed a visual search task on pictures of driving scenes containing a target (i.e., a child pedestrian or a roe deer) that had to be accurately and quickly identified. Simultaneously, stimuli previously associated with social reward could appear as distractors on a smartphone, irrelevantly displayed in one corner of the pictures, as if put on the car dashboard, next to the wheel (e.g., as when used as a GPS device). Previous studies have reported that cognitive distraction triggered by a smartphone mainly occurred during attentional-demanding tasks (Thornton et al., 2014; Stothart et al., 2015). Accordingly, considering attention as a limited resource process (Kahneman, 1973; Shiffrin and Schneider, 1977), performance deficits due to smartphone distraction might be minimal with simple tasks that can be done with little or no attentional resources. However, greater attentional demands placed on the primary task should increase the potential for performance deficits. Consequently, we manipulated the task difficulty by increasing the fog density on the driving scenes as contrast reduction (Liu et al., 2009), so that prolonged evidence accumulation from visual inputs to reduce decision-making uncertainty (Quétard et al., 2015; Quétard, 2018), would increase attentional demands.
Thereafter, we first describe the main methodological aspects of our study, including the material used (i.e., FoMO scale, pictures …) and the procedure followed by the volunteers. Note that the methodological details for the reward association phase can be found as Supplementary Material. Then, we provide the statistical analyses of the behavioral results for both the association phase and the driving visual search task. Finally, theoretical implications and limitations of the present preliminary study are discussed and future research directions are provided.
Materials and Methods
Participants
Twenty-nine college students (seven males; M = 20 years old, SD = 2, min = 17, and max = 26) from the Université Clermont Auvergne were recruited in exchange of course credits. Volunteer sampling among psychology students explains why the sex ratio in our sample was biased toward female participants. All participants were right-handed and all reported normal or corrected-to-normal visual acuity, as well as normal color perception. They were naive to the experiment purpose but, prior to their participation, they were told that a 10€ gift card could be earned according to their performance. The study was ethically approved by an institutional review board (IRB00011540-2018-12).
Apparatus
The participants were tested individually in a quiet room with constant ambient illumination, in front of a 14-inch VGA monitor (1,024 × 1,280 resolution, 60 Hz) at a distance of approximately 50 cm. The presentation of the stimuli, timing operations and data collection were controlled by E-Prime 2.0 software (Psychology Software Tools, Pittsburgh, PA, United States).
Materials and Procedure
After giving their informed consent, the participants first completed the FoMO scale and then performed the association phase, followed by the driving visual search task. They were not explicitly informed about the role of the association phase nor about its relation with the following driving visual search task. The two tasks were presented as independent of each other. The experiment took approximately 60 min.
Fear of Missing Out Scale
The volunteers completed the French translation (see Michot et al., 2016) of the 10-items FoMO scale (Przybylski et al., 2013; see Supplementary Figure 1). Possible responses to the affirmations (e.g., “I fear my friends have more rewarding experiences than me”) ranged from 1 (“Not at all true for me”) to 5 (“Perfectly true for me”). The scale produces an average score ranging from 1 to 5, with higher scores indicating higher levels of FoMO.
Social Reward Association Phase
The social reward association task was adapted from a classic visual search task (Anderson, 2016) in which the correct selection of different targets (i.e., circles of different colors) led to different social reward outcomes through the presentation of neutral or happy faces displayed in each trial with the performance feedback (see Figure 1). Because only minor changes were made to its original version (Anderson, 2016), the materials and procedure of the association phase are available as Supplementary Material.
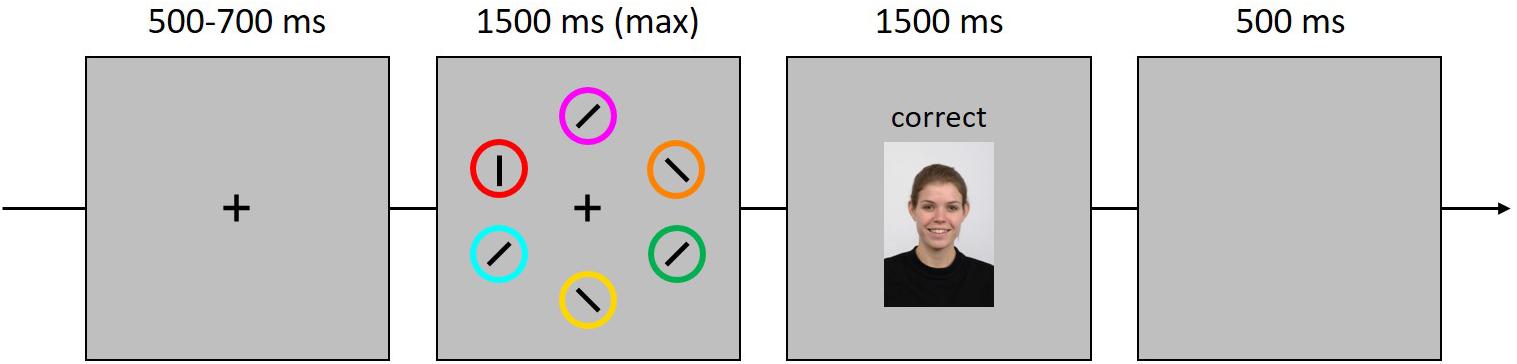
Figure 1. Example trial in the social reward association phase. Participants reported with a keypress the orientation of the bar within targets defined by their color (e.g., red in this case). Correct responses were followed by a feedback consisting of the presentation of a neutral or happy face (here, happy) from the Radboud Faces database (Langner et al., 2010). One target color was associated with a greater probability of a happy face vs. a neutral face (80–20%), while for the other target color this ratio was reversed.
Driving Visual Search Task
All stimuli were displayed on a black background. A central gray fixation cross (192, 192, 192) preceded the visual search display consisting of a centrally presented picture of a driving scene [from Quétard (2018); Figure 2]2. The pictures (29.1 × 21.8 dva), taken from a driver point-of-view, represented a foggy driving scene on a road at night (Colomb et al., 2008). On each picture, the rear lights of a preceding vehicle could be seen (at approximatively 14 m from the driver point-of-view). On some pictures, a child pedestrian (1.4 × 5.1 dva) or a roe deer (3.4 × 3.4 dva) could be present as a target (at approximatively 12 m from the driver point-of-view). The target could be at four possible locations: two external positions (off road, at 10.9 dva to the left or right from the screen center) and two internal positions (on road, at 5.1 dva to the left or right from the center), and was always heading toward the left side of the scene. Half of the pictures were taken under low fog density, while the other half was taken under high fog density (see Quétard, 2018). Each picture contained a smartphone (3.4 × 5 dva) with a gray screen (192, 192, 192; 2.6 × 4.2 dva), displayed at the bottom right corner of the picture, as if put on the car dashboard for being used as a GPS device (the distance between the smartphone center and the right and bottom edges of the picture was, respectively, 3.5 and 2.4 dva). When present, the social reward distractors (i.e., the target circles of the social reward association phase; diameter = 2.4 dva) were displayed at the center of the smartphone’ screen.
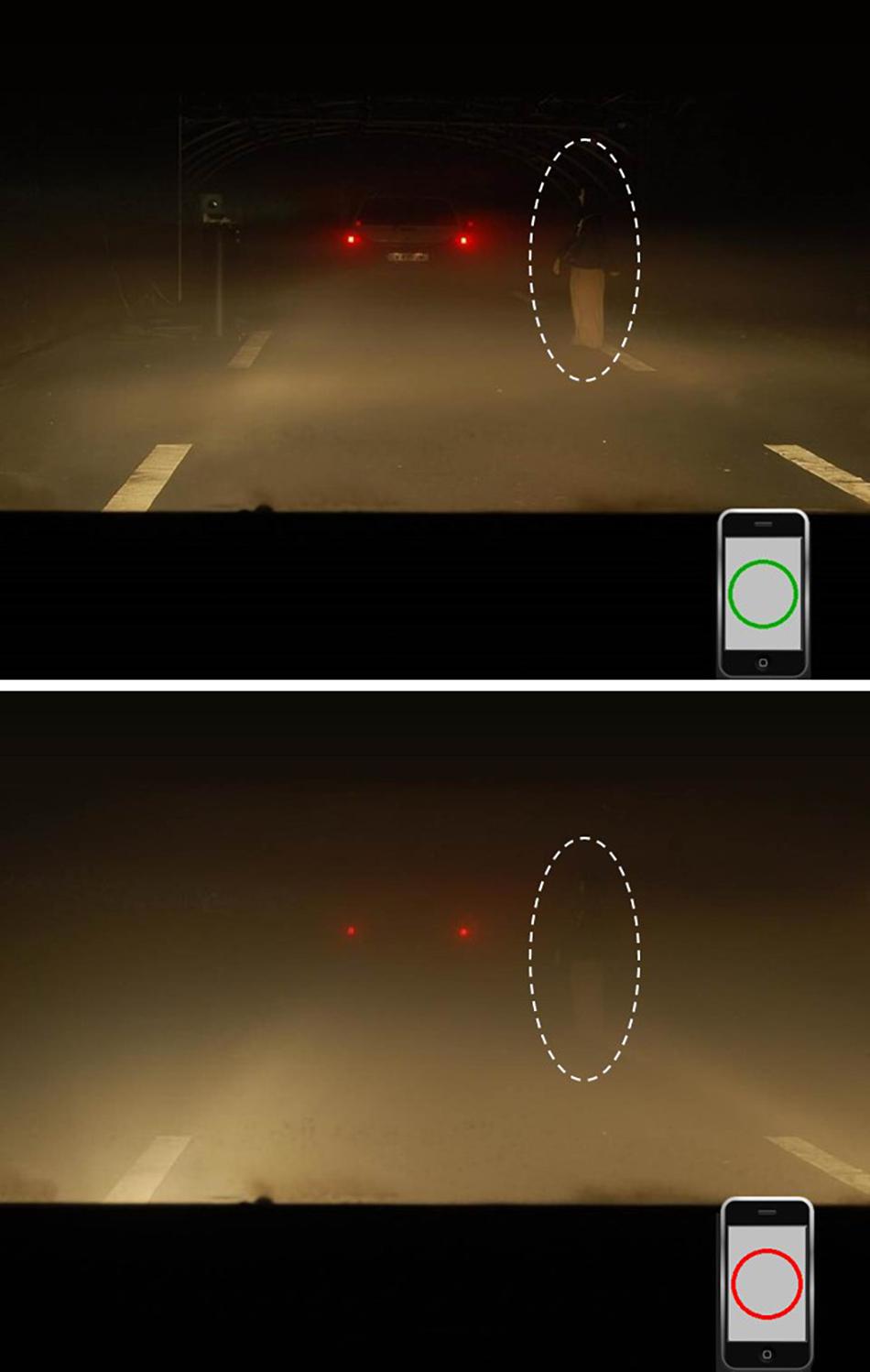
Figure 2. Examples of driving scene pictures used in the driving visual search task. Participants were required to identify as fast as possible the target (e.g., a child pedestrian, surrounded here by a dotted line for clarity, or a roe deer, not represented here) with pictures taken under low (top) or high fog density (bottom). In 75% of the trials, an irrelevant low (e.g., green – top) or high (e.g., red – bottom) social reward distractor could appear on the screen of a smartphone embedded in each picture (the smartphone displayed an empty gray screen in the remaining trials - not represented here).
Participants performed 384 trials3 split equally in 12 experimental blocks, with six consecutive blocks for each fog density level, the order of the fog density levels being counterbalanced across participants. In each block, a target (equiprobably a pedestrian or a roe deer) appeared in 75% of trials. Each target appeared equally often at each of the four possible locations. No target was displayed on the remaining 25% of the trials. Moreover, one-third of the trials contained a high-reward distractor, one-third a low-reward distractor and no distractor was displayed in the remaining third (distractor presence/absence was counterbalanced with target type – child pedestrians, roe deer, none - and position, as well as fog density). Trials order was randomly determined within each block and a short break was offered between each block. Before performing the experimental task proper, participants completed a training block of 12 trials (i.e., stimuli were in same proportion as experimental blocks except that a target was displayed on 50% of the training trials).
Each trial began with a fixation cross displayed for 500, 600, or 700 ms (randomly determined), followed by the driving scene that remained on the screen until the participant’s response (or up to 1,500 ms). He/she had to indicate as fast as possible whether a pedestrian or a roe deer was present on the picture, by pressing “2” or “5” on the numeric keypad with his/her forefinger or middle finger, respectively (the association between the buttons and the target types was counterbalanced across participants). The aim of this discrimination task was to mirror classic attentional task where participants usually have to indicate the orientation of a line (two choices) inside a target (e.g., Anderson et al., 2011b), but also to make the task more difficult and thus introduce more variability in the collected data. No response was required when the target was absent. Consecutively, a feedback screen informed the participants about their accuracy on the current trial (“correct,” “error,” or “missed” – when no response had been given whereas a target was actually present) during 1,500 ms. Finally, a black screen separated two consecutive trials for 500 ms.
Results
Reaction times (RTs) for incorrect trials or shorter than 200 ms have been excluded from the following analyses. Moreover, for each participant, RTs plus/minus 2.5 absolute deviations around the median (Leys et al., 2013) were also discarded (altogether, less than 5% of the data for both the social reward association and the driving visual search tasks). All analyses relied on linear models, following aggregation at the condition per participant level to make observations independent, hence the need to eliminate extreme RTs that may bias mean estimates. All results were nevertheless confirmed by fitting linear mixed models at trial level, alleviating the need for filtering outlier RTs. The more parsimonious models were reported for readability purpose.
Social Reward Association Phase
A paired t-test comparison revealed that the difference on RTs between high (M = 746 ms; SE = 17 ms) and low reward target (M = 758 ms; SE = 20 ms) was not significant [t(28) = 1.15, p = 0.259]. In the same way, error rates were not significantly different for high (M = 6.7%; SE = 0.92%) and low reward target (M = 7.6%; SE = 1.03%) [t(28) = 1.40, p = 0.171].
One could expect that an efficient stimulus-reward association should lead to significantly faster and more accurate responses for high reward stimuli. However, although our results were numerically in line with this expectation, our participants were not significantly faster nor more accurate for high versus low reward targets. Nonetheless, as repeatedly observed, significant differences in the association phase are not a necessary pre-requisite to observe value-driven attentional capture in the subsequent search task (e.g., Anderson et al., 2011a; Roper et al., 2014; Anderson, 2016; Anderson and Halpern, 2017; Anderson and Kim, 2019). Non-significant differences could suggest that participants searched for both target colors with roughly equal priority but it does not preclude from investigating whether the experience of these stimulus-reward associations would further influence attention in the test phase, when those stimuli were presented as irrelevant distractors (Anderson, 2016).
Driving Visual Search Task
Reaction times were submitted to a FoMO × Fog Density (low vs. high) × Distractor Type (high-reward, low-reward, and no distractor) linear model analysis with FoMO as a continuous predictor and Fog Density and Distractor Type as within-participant predictors. Relying on linear models to test the combined effects of factors and continuous predictor, distractor Type was coded using orthogonal Helmert contrasts with the first contrast (C1) opposing RTs on high-reward distractor trials (coded + 1) to RTs on low-reward and no distractor trials (both coded −0.5), and the second (C2) opposing RTs on low-reward trials (coded + 0.5) to RTs on no distractor trials (coded −0.5). Fog was also contrast-coded (low density as −1 and high density as + 1). FoMO scores (M = 2.01, SD = 0.32) were mean-centered. We predicted that RTs would be significantly longer in the condition of high fog density (indicating that the fog increased attentional demands) and that this effect would be amplified by the interaction of high-reward distractor and FoMO (indicating that distraction occurred).
The expected three-way interaction between FoMO, Fog, and Distractor Type (C1) was significant, b = 22, 95% CI [3, 41], t(108) = 2.31, p = 0.023, and R2 = 0.0474. This interaction was decomposed into simple interactions for each level of Fog density. When Fog density was low, the interaction of FoMO and Distractor Type (C1) was non-significant [b = −5 ms, 95% CI [−28, 17], t(27) = 0.5, and p = 0.629]. Thus, under low fog density, FoMO played no significant role in the distraction produced by the high-reward distractor. However, and as expected, when the fog density was high (i.e., when attentional demands increased), the interaction of FoMO and Distractor Type (C1) was significant, b = 28 ms, 95% CI [7, 48], t(27) = 2.83, and p = 0.009. Indeed, in conditions of high-fog density, high social reward distractors resulted in an increased of RTs by 28 ms for each point on the FoMO scale (Figure 3; r = 0.48). In other words, when the fog density was high, higher levels of FoMO were associated with a larger distraction effect for high compared to low reward distractors. The analysis also revealed a main effect of Fog, b = 48 ms, 95% CI [33, 63], t(27) = 6.61, p < 0.001, and R2 = 0.62, with slower RTs under high (M = 731 ms; SE = 18 ms) rather than low fog density (M = 683 ms; SE = 17 ms). All other effects and interactions were non-significant. Finally, a similar generalized linear model analysis performed on error rates revealed no significant effect.
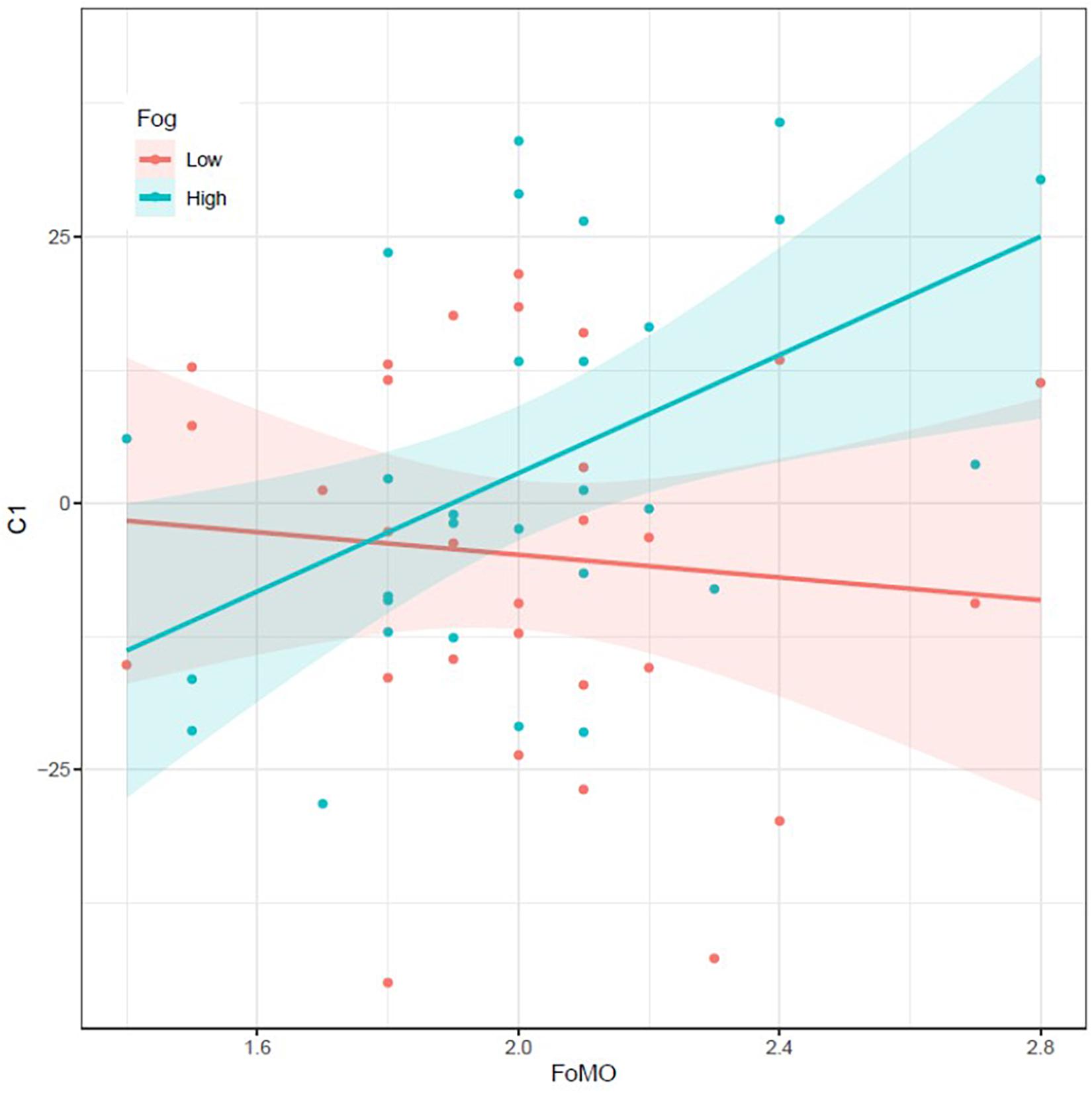
Figure 3. Distraction effect (in ms; with standard error bands) triggered by the high vs. low/no social reward distractor (contrast code C1) under low (red line) and high (green line) fog density for each participant, as a function of Fear of Missing Out (FoMO) scores.
Discussion
The results from the present study revealed that individuals’ level of FoMO can predict the distraction triggered by high social reward stimuli, in a driving context. This observation is in line with previous works showing that participants who are high in FoMO are also those who report paying the most attention to their smartphone when driving (Przybylski et al., 2013; Brown et al., 2021). However, to our knowledge, the present study is the first that relied on direct behavioral observations of distraction, instead of potentially biased self-reported estimations (see Boase and Ling, 2013; Vanden Abeele et al., 2013; Lee et al., 2017).
Theoretical Implications
To be precise, the link between FoMO and distraction was evident only when the primary task was difficult (i.e., high-fog density). This result is consistent with previous studies showing that smartphones are more likely to elicit distraction under attentional-demanding conditions (Thornton et al., 2014; Stothart et al., 2015). The rationale is that the target search and identification task could have been performed with very little resources in the low fog density. Therefore, attentional capture by reward distractor, if/when present, could not have produced significant performance deficits. One could argue that such interpretation was not strictly supported by our data, as increasing fog density did not significantly increased error rates. However, RTs were significantly longer under high rather than low fog density, suggesting a prolonged accumulating evidence process from visual inputs to reduce decision-making uncertainty (Quétard et al., 2015; Quétard, 2018). This prolonged delay before the participant’s response might offer a larger time window for distractor intrusion (Lavie and de Fockert, 2003). Alternatively, because the fog degraded visual information only outside the vehicle, we could argue that the smartphone and reward distractors were actually relatively more salient in the high rather than low fog density condition. Consequently, along with reward history, higher distractor’s relative salience under high fog density could also have led to larger distraction effect in this condition (see Theeuwes, 1991, 1992; Koch et al., 2013; Zehetleitner et al., 2013).
It therefore appears that FoMO should be taken into consideration when investigating attentional capture by social reward stimuli as it might provide a finer-grained understanding of this phenomenon. Indeed, so far, most studies investigating reward history have associated neutral stimuli with monetary outcomes or points converted into real cash (e.g., Anderson et al., 2011b; Le Pelley et al., 2015). Experimental social reward manipulations are scarcer, probably because they are less meaningful for subjects as they do not have a concrete incidence on their (social) life. We contend that not considering individual characteristics such as FoMO might also account for some null effects (e.g., Johannes et al., 2019). In line with this view, some authors (Wilmer and Chein, 2016) have failed to identify a relationship between mobile engagement and reward sensitivity measured through the classic Behavioral Inhibition/Activation System scale (BIS/BAS: Carver and White, 1994). However, the BIS/BAS scale is not specifically designed for social reward sensitivity whereas social aspects are of particular importance when considering smartphone use (Özcan and Koçak, 2003; Srivastava, 2005; Waddell and Wiener, 2014; Sherman et al., 2016). Therefore, we suggest that the FoMO scale would be better suited for such investigations.
Limitations and Future Research Directions
One important limitation of our study is related to its design. Indeed, our participants were not engaged in a truly realistic nor simulated driving task and thus had no risk of collision with the child pedestrian or the roe deer. As a consequence, the current experimental settings could have been insufficient to incite the participants to strengthen their attentional control settings thereby preventing attentional capture (Gaspelin and Luck, 2018b). Additionally, we did not ask our participants about their driving experience. Because our experimental context was actually relatively different from a real driving activity, one could argue that the participants’ performance might not rely on their driving experience. However, this factor can obviously influence driver behavior and notably, experienced drivers would be more able than novices to respond to hazard and adapt their driving in situation of degraded visibility (e.g., Mueller and Trick, 2012). Therefore, along with FoMO, driver’s experience could be a critical factor to understand smartphone-based distraction and driver’s attentional control under degraded conditions, but more realistic driving scenario and tasks would be necessary. Also, due to our sample characteristics (i.e., mainly young females), it is difficult to generalize our findings to the overall population. Further research is thus needed to clarify the role of FoMO into a more ecological context to offer a better comprehensive model of driver distraction.
Finally, to our knowledge, distractors’ reward history and their motivational values are still underinvestigated in driver distraction studies, with most of the works focusing on salience-based attentional capture [e.g., Chattington et al. (2009), Dukic et al. (2013), but see Walker and Trick (2019) for the impact of emotional irrelevant billboards]. Yet, whereas salience-based distraction can be prevented under some conditions (e.g., Lavie, 1995; Belopolsky and Theeuwes, 2010; Cosman and Vecera, 2010; Gaspelin et al., 2017; Gaspelin and Luck, 2018a, c), attentional capture caused by reward distractors seems to be “automatic” and thus likely to occur in any condition (e.g., Gupta et al., 2016; Munneke et al., 2016; Wang et al., 2018; Matias et al., 2021). Besides, reward-based distraction seems to be qualitatively different from salience-based distraction, as processing reward stimuli hampers a wider range of cognitive process (Anderson, 2017). Hence, attentional capture by smartphone (socially rewarding) notifications is likely to produce effects that persist longer over time (Thornton et al., 2014; Stothart et al., 2015), with more detrimental consequences on drivers’ performances (He et al., 2011; Lemercier et al., 2014). Building more bridges between studies on reward-based and smartphone-based distraction therefore appears of primary importance to improve road safety.
Conclusion
This preliminary study is the first, to our knowledge, that provides experimental evidences of the link between individuals’ FoMO and social-reward distraction in a driving context. Indeed, we showed that, under difficult (i.e., foggy) driving situations, higher levels of FoMO are associated with larger distraction produced by high social reward stimuli. Therefore, this research emphasizes the necessity for models of road safety to take into account a broader range of drivers’ sources of distraction such as socio-psychological needs, and to go beyond salience-based distraction.
Data Availability Statement
The raw data supporting the conclusions of this article will be made available by the authors, without undue reservation.
Ethics Statement
The studies involving human participants were reviewed and approved by CER-IRB UCA. The patients/participants provided their written informed consent to participate in this study.
Author Contributions
All authors contributed to the study conception, design, statistical analysis, commented on previous versions of the manuscript, read, and approved the final manuscript. JM performed the material preparation, data collection, wrote the first draft of the manuscript.
Funding
This work was funded by grants from the French program “investissement d’avenir” managed by the National Research Agency (ANR), from the European Union (Auvergne European Regional Development Funds – ERDF – of Auvergne region) and from the “Région Auvergne” in the framework of the IMobS3 LabEx (ANR-10-LABX-16-01).
Conflict of Interest
The authors declare that the research was conducted in the absence of any commercial or financial relationships that could be construed as a potential conflict of interest.
Acknowledgments
The authors expressed their heartfelt thanks to Boris Quétard for sharing its experimental material.
Supplementary Material
The Supplementary Material for this article can be found online at: https://www.frontiersin.org/articles/10.3389/fpsyg.2021.688157/full#supplementary-material
Footnotes
- ^ Note that early studies generally referred to cell phone or mobile phone instead of smartphone, which is, basically, a mobile phone with more advanced features (e.g., browsing the web, emailing, social networking, playing personal media, or games).
- ^ Original pictures (3,006 × 2,000 pixels) were just trimmed in order to remove irrelevant stimuli for our study (e.g., road signs). To this end, the left, right, and lower edges were, respectively trimmed by 410, 605, and 210 pixels.
- ^ See https://osf.io/tay95/
- ^ Partial R2 standardized effect sizes were estimated using Kenward-Roger approximation of degrees of freedom, following recommendations from Edwards et al. (2008), since we relied on a mixed design with a continuous predictor (FoMO).
References
Al-Furaih, S. A. A., and Al-Awidi, H. M. (2020). Fear of missing out (FoMO) among undergraduate students in relation to attention distraction and learning disengagement in lectures. Educ. Inform. Technol. 26, 2355–2373. doi: 10.1007/s10639-020-10361-7
Anderson, B. A. (2015). The attention habit: how reward learning shapes attentional selection. Ann. N. Y. Acad. Sci. 1369, 24–39. doi: 10.1111/nyas.12957
Anderson, B. A. (2016). Social reward shapes attentional biases. Cogn. Neurosci. 7, 30–36. doi: 10.1080/17588928.2015.1047823
Anderson, B. A. (2017). Going for it: the economics of automaticity in perception and action. Curr. Direct. Psychol. Sci. 26, 140–145. doi: 10.1177/0963721416686181
Anderson, B. A., Chiu, M., DiBartolo, M. M., and Leal, S. L. (2017). On the distinction between value-driven attention and selection history: evidence from individuals with depressive symptoms. Psychon. Bull. Rev. 24, 1636–1642. doi: 10.3758/s13423-017-1240-9
Anderson, B. A., and Halpern, M. (2017). On the value-dependence of value-driven attentional capture. Attent. Percept. Psychophys. 79, 1001–1011. doi: 10.3758/s13414-017-1289-6
Anderson, B. A., and Kim, H. (2019). On the relationship between value-driven and stimulus-driven attentional capture. Attent. Percept. Psychophys. 81, 607–613. doi: 10.3758/s13414-019-01670-2
Anderson, B. A., Laurent, P. A., and Yantis, S. (2011a). Learned value magnifies salience-based attentional capture. PLoS One 6:e27926. doi: 10.1371/journal.pone.0027926
Anderson, B. A., Laurent, P. A., and Yantis, S. (2011b). Value-driven attentional capture. Proc. Natl. Acad. Sci. U.S.A. 108, 10367–10371. doi: 10.1073/pnas.1104047108
Arexis, M., Maquestiaux, F., Gaspelin, N., Ruthruff, E., and Didierjean, A. (2017). Attentional capture in driving displays. Br. J. Psychol. 108, 259–275. doi: 10.1111/bjop.12197
Awh, E., Belopolsky, A. V., and Theeuwes, J. (2012). Top-down versus bottom-up attentional control: a failed theoretical dichotomy. Trends Cogn. Sci. 16, 437–443. doi: 10.1016/j.tics.2012.06.010
Belopolsky, A. V. (2015). Common priority map for selection history, reward and emotion in the oculomotor system. Perception 44, 920–933. doi: 10.1177/0301006615596866
Belopolsky, A. V., and Theeuwes, J. (2010). No capture outside the attentional window. Vision Res. 50, 2543–2550. doi: 10.1016/j.visres.2010.08.023
Belyusar, D., Reimer, B., Mehler, B., and Coughlin, J. F. (2016). A field study on the effects of digital billboards on glance behavior during highway driving. Accident Anal. Prevent. 88, 88–96. doi: 10.1016/j.aap.2015.12.014
Bendak, S., and Al-Saleh, K. (2010). The role of roadside advertising signs in distracting drivers. Int. J. Indust. Ergon. 40, 233–236. doi: 10.1016/J.ERGON.2009.12.001
Boase, J., and Ling, R. (2013). Measuring mobile phone use: self-report versus log data. J. Comp. Mediated Commun. 18, 508–519. doi: 10.1111/jcc4.12021
Bourgeois, A., Chelazzi, L., and Vuilleumier, P. (2016). How motivation and reward learning modulate selective attention. Prog. Brain Res. 229, 325–342. doi: 10.1016/bs.pbr.2016.06.004
Bourgeois, A., Neveu, R., Bayle, D. J., and Vuilleumier, P. (2015). How does reward compete with goal-directed and stimulus-driven shifts of attention? Cogn. Emot. 9931, 1–10. doi: 10.1080/02699931.2015.1085366
Brown, P. M., George, A. M., and Rickwood, D. J. (2021). Rash impulsivity, reward seeking and fear of missing out as predictors of texting while driving: indirect effects via mobile phone involvement. Pers. Individ. Differ. 171:110492. doi: 10.1016/j.paid.2020.110492
Bucker, B., Belopolsky, A. V., and Theeuwes, J. (2015). Distractors that signal reward attract the eyes. Visual Cogn. 23, 1–24. doi: 10.1080/13506285.2014.980483
Caird, J. K., Johnston, K. A., Willness, C. R., Asbridge, M., and Steel, P. (2014). A meta-analysis of the effects of texting on driving. Accident Anal. Prevent. 71, 311–318. doi: 10.1016/j.aap.2014.06.005
Caird, J. K., Willness, C. R., Steel, P., and Scialfa, C. (2008). A meta-analysis of the effects of cell phones on driver performance. Accident Anal. Prevent. 40, 1282–1293. doi: 10.1016/j.aap.2008.01.009
Carver, C. S., and White, T. L. (1994). Behavioral inhibition, behavioral activation, and affective responses to impending reward and punishment: the BIS/BAS Scales. J. Pers. Soc. Psychol. 67, 319–333. doi: 10.1037/0022-3514.67.2.319
Chattington, M., Reed, N., Basacik, D., Flint, A., and Parkes, A. (2009). Investigating Driver Distraction: The Effects of Video and Static Advertising (Issue RPN 256 PO 3100173332). Crowthorne: TRL Limited.
Cheever, N. A., Rosen, L. D., Carrier, L. M., and Chavez, A. (2014). Out of sight is not out of mind: the impact of restricting wireless mobile device use on anxiety levels among low, moderate and high users. Comp. Hum. Behav. 37, 290–297. doi: 10.1016/j.chb.2014.05.002
Chen, C., Zhang, K. Z. K., Gong, X., and Lee, M. (2019). Dual mechanisms of reinforcement reward and habit in driving smartphone addiction: the role of smartphone features. Internet Res. 29, 1551–1570. doi: 10.1108/INTR-11-2018-0489
Clayton, R. B., Leshner, G., and Almond, A. (2015). The extended iself: the impact of iphone separation on cognition, emotion, and physiology. J. Comp. Mediated Commun. 20, 119–135. doi: 10.1111/jcc4.12109
Colomb, M., Hirech, K., André, P., Boreux, J. J., Lacôte, P., and Dufour, J. (2008). An innovative artificial fog production device improved in the European project “FOG.” Atmos. Res. 87, 242–251. doi: 10.1016/j.atmosres.2007.11.021
Corbetta, M., and Shulman, G. L. (2002). Control of goal-directed and stimulus-driven attention in the brain. Nat. Rev. Neurosci. 3, 201–215. doi: 10.1038/nrn755
Cosman, J. D., and Vecera, S. P. (2010). Attentional capture by motion onsets is modulated by perceptual load. Attent. Percept. Psychophys. 72, 2096–2105. doi: 10.3758/APP.72.8.2096
CREDOC (2019). Baromètre du Numérique 2019. Available online at: https://www.credoc.fr/publications/barometre-du-numerique-2019 (accessed January, 2021).
Dempsey, A. E., O’Brien, K. D., Tiamiyu, M. F., and Elhai, J. D. (2019). Fear of missing out (FoMO) and rumination mediate relations between social anxiety and problematic Facebook use. Addict. Behav. Rep. 9:100150. doi: 10.1016/j.abrep.2018.100150
Dingus, T. A., Guo, F., Lee, S., Antin, J. F., Perez, M., Buchanan-King, M., et al. (2016). Driver crash risk factors and prevalence evaluation using naturalistic driving data. Proc. Natl. Acad. Sci. U.S.A. 113, 2636–2641. doi: 10.1073/pnas.1513271113
Dukic, T., Ahlstrom, C., Patten, C., Kettwich, C., and Kircher, K. (2013). Effects of electronic billboards on driver distraction. Traffic Injury Prevent. 14, 469–476. doi: 10.1080/15389588.2012.731546
Dumitru, A. I., Girbacia, T., Boboc, R. G., Postelnicu, C. C., and Mogan, G. L. (2018). Effects of smartphone based advanced driver assistance system on distracted driving behavior: A simulator study. Comp. Hum. Behav. 83, 1–7. doi: 10.1016/j.chb.2018.01.011
Edwards, L. J., Muller, K. E., Wolfinger, R. D., Qaqish, B. F., and Schabenberger, O. (2008). An R 2 statistic for fixed effects in the linear mixed model. Statistics Med. 27, 6137–6157. doi: 10.1002/sim.3429
Elhai, J. D., Levine, J. C., Dvorak, R. D., and Hall, B. J. (2016). Fear of missing out, need for touch, anxiety and depression are related to problematic smartphone use. Comp. Hum. Behav. 63, 509–516. doi: 10.1016/j.chb.2016.05.079
Failing, M., and Theeuwes, J. (2017). Don’t let it distract you: how information about the availability of reward affects attentional selection. Attent. Percept. Psychophys. 79, 2275–2298. doi: 10.3758/s13414-017-1376-8
Failing, M., and Theeuwes, J. (2018). Selection history: how reward modulates selectivity of visual attention. Psychon. Bull. Rev. 25, 514–538. doi: 10.3758/s13423-017-1380-y
Fecteau, J., and Munoz, D. (2006). Salience, relevance, and firing: a priority map for target selection. Trends Cogn. Sci. 10, 382–390. doi: 10.1016/j.tics.2006.06.011
Gaspelin, N., Leonard, C. J., and Luck, S. J. (2017). Suppression of overt attentional capture by salient-but-irrelevant color singletons. Attent. Percept. Psychophys. 79, 45–62. doi: 10.3758/s13414-016-1209-1
Gaspelin, N., and Luck, S. J. (2018c). The role of inhibition in avoiding distraction by salient stimuli. Trends Cogn. Sci. 22, 79–92. doi: 10.1016/J.TICS.2017.11.001
Gaspelin, N., and Luck, S. J. (2018a). Combined electrophysiological and behavioral evidence for the suppression of salient distractors. J. Cogn. Neurosci. 30, 1265–1280. doi: 10.1162/jocn_a_01279
Gaspelin, N., and Luck, S. J. (2018b). Inhibition as a potential resolution to the attentional capture debate. Curr. Opin. Psychol. 29, 12–18. doi: 10.1016/J.COPSYC.2018.10.013
Gitelman, V., Doveh, E., and Zaidel, D. (2019). An examination of billboard impacts on crashes on a suburban highway: comparing three periods—billboards present, removed, and restored. Traffic Injury Prevent. 20(Suppl. 2), S69–S74. doi: 10.1080/15389588.2019.1645330
Gupta, R., Hur, Y.-J., and Lavie, N. (2016). Distracted by pleasure: effects of positive versus negative valence on emotional capture under load. Emotion 16, 328–337. doi: 10.1037/emo0000112
Hartmann, M., Martarelli, C. S., Reber, T. P., and Rothen, N. (2020). Does a smartphone on the desk drain our brain? No evidence of cognitive costs due to smartphone presence in a short-term and prospective memory task. Consciousness Cogn. 86:103033. doi: 10.1016/j.concog.2020.103033
He, J., Becic, E., Lee, Y. C., and McCarley, J. S. (2011). Mind wandering behind the wheel: performance and oculomotor correlates. Hum. Factors 53, 13–21. doi: 10.1177/0018720810391530
Hickey, C., Chelazzi, L., and Theeuwes, J. (2010). Reward changes salience in human vision via the anterior cingulate. J. Neurosci. 30, 11096–11103. doi: 10.1523/JNEUROSCI.1026-10.2010
Johannes, N., Dora, J., and Rusz, D. (2019). Social smartphone apps do not capture attention despite their perceived high reward value. Collabra Psychol. 5:14. doi: 10.1525/collabra.207
Klauer, S. G., Dingus, T. A., Neale, V. L., Sudweeks, J. D., and Ramsey, D. J. (2006). The Impact of Driver Inattention on Near Crash/Crash Risk: An Analysis Using the 100-Car Naturalistic Driving Study Data. Analysis, April. Report No.DOT HS 810 594.
Koch, A. I., Müller, H. J., and Zehetleitner, M. (2013). Distractors less salient than targets capture attention rather than producing non-spatial filtering costs. Acta Psychol. 144, 61–72. doi: 10.1016/j.actpsy.2013.04.023
Langner, O., Dotsch, R., Bijlstra, G., Wigboldus, D. H. J., Hawk, S. T., and van Knippenberg, A. (2010). Presentation and validation of the radboud faces database. Cogn. Emot. 24, 1377–1388. doi: 10.1080/02699930903485076
Lavie, N. (1995). Perceptual load as a necessary condition for selective attention. J. Exp. Psychol. Hum. Percept. Perform. 21, 451–468. doi: 10.1037//0096-1523.21.3.451
Lavie, N., and de Fockert, J. W. (2003). Contrasting effects of sensory limits and capacity limits in visual selective attention. Percept. Psychophys. 65, 202–212. doi: 10.3758/BF03194795
Le Pelley, M. E., Pearson, D., Griffiths, O., and Beesley, T. (2015). When goals conflict with values: Counterproductive attentional and oculomotor capture by reward-related stimuli. J. Exp. Psychol. Gen. 144, 158–171. doi: 10.1037/xge0000037
Lee, H., Ahn, H., Nguyen, T. G., Choi, S. W., and Kim, D. J. (2017). Comparing the self-report and measured smartphone usage of college students: a pilot study. Psychiatry Invest. 14, 198–204. doi: 10.4306/pi.2017.14.2.198
Lemercier, C., Pêcher, C., Berthié, G., Valéry, B., Vidal, V., Paubel, P. V., et al. (2014). Inattention behind the wheel: how factual internal thoughts impact attentional control while driving. Safety Sci. 62, 279–285. doi: 10.1016/j.ssci.2013.08.011
Leys, C., Ley, C., Klein, O., Bernard, P., and Licata, L. (2013). Detecting outliers: do not use standard deviation around the mean, use absolute deviation around the median. J. Exp. Soc. Psychol. 49, 764–766. doi: 10.1016/j.jesp.2013.03.013
Liu, T., Abrams, J., and Carrasco, M. (2009). Voluntary attention enhances contrast appearance. Psychol. Sci. 20, 354–362. doi: 10.1111/j.1467-9280.2009.02300.x
Luck, S. J., Gaspelin, N., Folk, C. L., Remington, R. W., and Theeuwes, J. (2021). Progress toward resolving the attentional capture debate. Visual Cogn. 29, 1–21. doi: 10.1080/13506285.2020.1848949
Matias, J., Quinton, J.-C., Colomb, M., Izaute, M., and Silvert, L. (2021). Reward history modulates perceptual load effects. Acta Psychol. 212:103217. doi: 10.1016/j.actpsy.2020.103217
Michot, D., Blancot, C., and Bourdon Baron Munoz, B. (2016). Relationship Between Fear of Missing Out and Social Media En-Gagement in a French population Sample. Available online at: https://www.spotpink.com/spotpink/wp-content/uploads/Michot-Blancot-Bourdon-Baron-Munoz-2016-VF.pdf (accessed January 31, 2018).
Mueller, A. S., and Trick, L. M. (2012). Driving in fog: the effects of driving experience and visibility on speed compensation and hazard avoidance. Accident Anal. Prevent. 48, 472–479. doi: 10.1016/J.AAP.2012.03.003
Munneke, J., Belopolsky, A. V., and Theeuwes, J. (2016). Distractors associated with reward break through the focus of attention. Attent. Percept. Psychophys. 78, 2213–2225. doi: 10.3758/s13414-016-1075-x
Munneke, J., Hoppenbrouwers, S. S., and Theeuwes, J. (2015). Reward can modulate attentional capture, independent of top-down set. Attent. Percept. Psychophys. 77, 2540–2548. doi: 10.3758/s13414-015-0958-6
Noy, I. Y., Shinar, D., and Horrey, W. J. (2018). Automated driving: safety blind spots. Safety Sci. 102, 68–78. doi: 10.1016/j.ssci.2017.07.018
Ortiz, C., Ortiz-Peregrina, S., Castro, J. J., Casares-López, M., and Salas, C. (2018). Driver distraction by smartphone use (WhatsApp) in different age groups. Accident Anal. Prevent. 117, 239–249. doi: 10.1016/j.aap.2018.04.018
Özcan, Y. Z., and Koçak, A. (2003). Research note: a need or a status symbol? Eur. J. Commun. 18, 241–254. doi: 10.1177/0267323103018002004
Pearson, D., Donkin, C., Tran, S. C., Most, S. B., and Le Pelley, M. E. (2015). Cognitive control and counterproductive oculomotor capture by reward-related stimuli. Visual Cogn. 6285, 1–26. doi: 10.1080/13506285.2014.994252
Pew Research Center (2018). Social Media Use Continues to Rise in Developing Countries. Washington, DC: Pew Research Center.
Przybylski, A. K., Murayama, K., DeHaan, C. R., and Gladwell, V. (2013). Motivational, emotional, and behavioral correlates of fear of missing out. Comp. Hum. Behav. 29, 1841–1848. doi: 10.1016/J.CHB.2013.02.014
Quétard, B. (2018). Anticipation et Accumulation Active D’information Sensorielle Dans la Prise de Décision en Situations de Vision Normale et Dégradée. Clermont-Ferrand: Université Clermont Auvergne].
Quétard, B., Quinton, J.-C., Colomb, M., Pezzulo, G., Barca, L., Izaute, M., et al. (2015). Combined effects of expectations and visual uncertainty upon detection and identification of a target in the fog. Cogn. Process. 16, 343–348. doi: 10.1007/s10339-015-0673-1
Regan, M. A., Hallett, C., and Gordon, C. P. (2011). Driver distraction and driver inattention: definition, relationship and taxonomy. Accident Anal. Prevent. 43, 1771–1781. doi: 10.1016/j.aap.2011.04.008
Roper, Z. J. J., Vecera, S. P., and Vaidya, J. G. (2014). Value-driven attentional capture in adolescence. Psychol. Sci. 25, 1987–1993. doi: 10.1177/0956797614545654
Rozgonjuk, D., Elhai, J. D., Ryan, T., and Scott, G. G. (2019). Fear of missing out is associated with disrupted activities from receiving smartphone notifications and surface learning in college students. Comp. Educ. 140:103590. doi: 10.1016/j.compedu.2019.05.016
Rozgonjuk, D., Sindermann, C., Elhai, J. D., and Montag, C. (2020). Fear of Missing Out (FoMO) and social media’s impact on daily-life and productivity at work: do whatsapp, facebook, instagram, and snapchat use disorders mediate that association? Addict. Behav. 110:106487. doi: 10.1016/j.addbeh.2020.106487
Sherman, L. E., Payton, A. A., Hernandez, L. M., Greenfield, P. M., and Dapretto, M. (2016). The power of the like in adolescence: effects of peer influence on neural and behavioral responses to social media. Psychol. Sci. 27, 1027–1035. doi: 10.1177/0956797616645673
Shiffrin, R. M., and Schneider, W. (1977). Controlled and automatic human information processing: II. Perceptual learning, automatic attending and a general theory. Psychol. Rev. 84, 127–190. doi: 10.1037/0033-295X.84.2.127
Srivastava, L. (2005). Mobile phones and the evolution of social behaviour. Behav. Inform. Technol. 24, 111–129. doi: 10.1080/01449290512331321910
Stothart, C. R., Mitchum, A., and Yehnert, C. (2015). The attentional cost of receiving a cell phone notification. J. Exp. Psychol. Hum. Percept. Perform. 41, 893–897. doi: 10.1037/xhp0000100
Strayer, D. L. (2015). Is the technology in your car driving you to distraction? Policy Insights Behav. Brain Sci. 2, 157–165. doi: 10.1177/2372732215600885
Strayer, D. L., and Drews, F. A. (2007). Cell-phone–induced driver distraction. Curr. Direct. Psychol. Sci. 16, 128–131. doi: 10.1111/j.1467-8721.2007.00489.x
Strayer, D. L., Drews, F. A., and Johnston, W. A. (2003). Cell phone-induced failures of visual attention during simulated driving. J. Exp. Psychol. Appl. 9, 23–32. doi: 10.1037/1076-898X.9.1.23
Theeuwes, J. (1991). Cross-dimensional perceptual selectivity. Percept. Psychophys. 50, 184–193. doi: 10.3758/BF03212219
Theeuwes, J. (1992). Perceptual selectivity for color and form. Percept. Psychophys. 51, 599–606. doi: 10.3758/BF03211656
Theeuwes, J. (2010). Top-down and bottom-up control of visual selection. Acta Psychol. 135, 77–99. doi: 10.1016/j.actpsy.2010.02.006
Theeuwes, J. (2018). Visual selection: usually fast and automatic; seldom slow and volitional. J. Cogn. 1, 1–15. doi: 10.5334/joc.13
Thornton, B., Faires, A., Robbins, M., and Rollins, E. (2014). The mere presence of a cell phone may be distracting. Soc. Psychol. 45, 479–488. doi: 10.1027/1864-9335/a000216
Turel, O., and Serenko, A. (2012). The benefits and dangers of enjoyment with social networking websites. Eur. J. Inform. Syst. 21, 512–528. doi: 10.1057/ejis.2012.1
Vanden Abeele, M., Beullens, K., and Roe, K. (2013). Measuring mobile phone use: gender, age and real usage level in relation to the accuracy and validity of self-reported mobile phone use. Mobile Media Commun. 1, 213–236. doi: 10.1177/2050157913477095
Waddell, L. P., and Wiener, K. K. K. (2014). What’s driving illegal mobile phone use? Psychosocial influences on drivers’ intentions to use hand-held mobile phones. Transport. Res. Part F Traffic Psychol. Behav. 22, 1–11. doi: 10.1016/j.trf.2013.10.008
Walker, H. E. K., and Trick, L. M. (2019). How the emotional content of roadside images affect driver attention and performance. Safety Sci. 115, 121–130. doi: 10.1016/j.ssci.2019.02.004
Walsh, S. P., White, K. M., and Young, R. M. (2008). Over-connected? A qualitative exploration of the relationship between Australian youth and their mobile phones. J. Adolesc. 31, 77–92. doi: 10.1016/j.adolescence.2007.04.004
Wang, L., Li, S., Zhou, X., and Theeuwes, J. (2018). Stimuli that signal the availability of reward break into attentional focus. Vision Res. 144, 20–28. doi: 10.1016/J.VISRES.2017.10.008
Ward, A. F., Duke, K., Gneezy, A., and Bos, M. W. (2017). Brain drain: the mere presence of one’s own smartphone reduces available cognitive capacity. J. Assoc. Consumer Res. 2, 140–154. doi: 10.1086/691462
Wilmer, H. H., and Chein, J. M. (2016). Mobile technology habits: patterns of association among device usage, intertemporal preference, impulse control, and reward sensitivity. Psychon. Bull. Rev. 23, 1607–1614. doi: 10.3758/s13423-016-1011-z
Wolniewicz, C. A., Rozgonjuk, D., and Elhai, J. D. (2020). Boredom proneness and fear of missing out mediate relations between depression and anxiety with problematic smartphone use. Hum. Behav. Emerging Technol. 2, 61–70. doi: 10.1002/hbe2.159
Zehetleitner, M., Koch, A. I., Goschy, H., and Müller, H. J. (2013). Salience-based selection: attentional capture by distractors less salient than the target. PLoS One 8:e52595. doi: 10.1371/journal.pone.0052595
Keywords: distraction, Fear of Missing Out, smartphone, social reward, driving, fog
Citation: Matias J, Quinton J-C, Colomb M, Normand A, Izaute M and Silvert L (2021) Fear of Missing Out Predicts Distraction by Social Reward Signals Displayed on a Smartphone in Difficult Driving Situations. Front. Psychol. 12:688157. doi: 10.3389/fpsyg.2021.688157
Received: 30 March 2021; Accepted: 24 June 2021;
Published: 16 July 2021.
Edited by:
Guillaume Chanel, Université de Genève, SwitzerlandReviewed by:
Cesar Collazos, University of Cauca, ColombiaFernando Moreira, Portucalense University, Portugal
George Yannis, National Technical University of Athens, Greece
Copyright © 2021 Matias, Quinton, Colomb, Normand, Izaute and Silvert. This is an open-access article distributed under the terms of the Creative Commons Attribution License (CC BY). The use, distribution or reproduction in other forums is permitted, provided the original author(s) and the copyright owner(s) are credited and that the original publication in this journal is cited, in accordance with accepted academic practice. No use, distribution or reproduction is permitted which does not comply with these terms.
*Correspondence: Jérémy Matias, amVyZW15Lm1hdGlhc0B1Y2EuZnI=