- 1Instituto Federal de Educação Ciência e Tecnologia do Pará, Campus Bragança, Laboratório de Biologia Molecular e Neuroecologia, Bragança, Brazil
- 2Laboratório de Genética e Conservação, Instituto de Estudos Costeiros (IECOS), Universidade Federal do Pará, Bragança, Brazil
- 3Laboratório de Investigações em Neurodegeneração e Infecção, Instituto de Ciências Biológicas, Universidade Federal do Pará, Hospital Universitário João de Barros Barreto, Belém, Brazil
- 4Laboratório de Microscopia Eletrônica, Instituto Evandro Chagas, Belém, Brazil
- 5Department of Psychology, Advanced Facility for Avian Research, University of Western Ontario, London, ON, Canada
For the epic journey of autumn migration, long-distance migratory birds use innate and learned information and follow strict schedules imposed by genetic and epigenetic mechanisms, the details of which remain largely unknown. In addition, bird migration requires integrated action of different multisensory systems for learning and memory, and the hippocampus appears to be the integration center for this task. In previous studies we found that contrasting long-distance migratory flights differentially affected the morphological complexity of two types of hippocampus astrocytes. Recently, a significant association was found between the latitude of the reproductive site and the size of the ADCYAP1 allele in long distance migratory birds. We tested for correlations between astrocyte morphological complexity, migratory distances, and size of the ADCYAP1 allele in three long-distance migrant species of shorebird and one non-migrant. Significant differences among species were found in the number and morphological complexity of the astrocytes, as well as in the size of the microsatellites of the ADCYAP1 gene. We found significant associations between the size of the ADCYAP1 microsatellites, the migratory distances, and the degree of morphological complexity of the astrocytes. We suggest that associations between astrocyte number and morphological complexity, ADCYAP1 microsatellite size, and migratory behavior may be part of the adaptive response to the migratory process of shorebirds.
Introduction
Overall, long-distance navigation includes at least three different phases. The first involves long distance guidance based on global tracks (Able, 1991; Wiltschko R. and Wiltschko, 2012; Wiltschko W. and Wiltschko, 2012; Wiltschko and Wiltschko, 2019); the second includes the construction of a variety of local gradient maps based on learned information from all available sensory information (Bingman and MacDougall-Shackleton, 2017). The last involves identifying the target area, most likely based on local landmarks (Frost and Mouritsen, 2006; Bingman and MacDougall-Shackleton, 2017). In young birds on their first migratory journey, navigation is based on compass-CLOCK orientation that requires only an inherited migratory direction, a circannual CLOCK and at least one compass (van Toor et al., 2013; Mouritsen et al., 2016). In experienced birds, in addition to the compass-CLOCK system, learned maps become part of the navigation system (Mouritsen et al., 2016) and include olfactory cues (Gagliardo et al., 2013), landmarks (Mann et al., 2014), celestial tracks (Emlen, 1975), and geomagnetic signals (Dennis et al., 2007).
Specific genes or gene regions, and polymorphisms at candidate genes, may explain phenotypic variance in many aspects of migratory behavior (Bazzi et al., 2016b). Microsatellite loci show high mutation rates with a high level of polymorphism with significant changes in the number of repeats and allele size (Hardy et al., 2003) and have been widely used for investigation of genetic patterns among and within populations (Balloux and Lugon-Moulin, 2002; Song et al., 2011). Indeed, after investigate polymorphisms in the exon of six candidate genes for a relationship with behavioral migratory phenotypes a consistent association there was found a consistent association between microsatellite polymorphism at only one candidate gene: the ADCYAP1 (Mueller et al., 2011). Other studies on neotropical migratory passerines (Passerina ciris) however, have pointed out that the size of microsatellite alleles of the ADCYAP1 and CLOCK gene do not show correlation with the onset or duration of migration, making this a controversial issue (Contina et al., 2018). In contrast, in blackpoll warblers (Setophaga striata), Clock and ADCYAP1 allele lengths were correlated with migratory behaviors (Ralston et al., 2019). In addition, it has been suggested that potential interaction between ADCYAP1, wing morphology and sex predict spring migration arrival in blackcap (Sylvia atricapilla) populations (Mettler et al., 2015) and that both ADCYAP1 and CLOCK gene alleles increase in size with breeding latitude in trans-Saharan migratory birds (Bazzi et al., 2016a,b).
Thus, it emerges that we are still far from filling in the details of the functional contribution of the ADCYAP1 and CLOCK genes to the intricate interaction between the internal clock and environmental conditions regulating the annual navigation cycle of long-distance migratory birds (Åkesson et al., 2017).
The adenylate cyclase activating polypeptide 1 (ADCYAP1) is a dinucleotide microsatellite locus in the 3′ UTR (untranslated region) of avian chromosome 2a with polymorphism associated with phenotypic variance of migratory behavior (Bazzi et al., 2016b; Contina et al., 2018). It is a protein code gene with polymorphic profile, which encodes the pituitary adenylate cyclase activator peptide (PACAP), widely expressed in the central nervous system (CNS) and peripheral organs (Montero et al., 2000; Gahete et al., 2009; Toth et al., 2020). PACAP alters neurotransmitter release and contributes to regulation of energy homeostasis in the CNS acting within hypothalamic/hypophyseal system (Rudecki and Gray, 2016; Gastelum et al., 2021), and through limbic actions that contribute to cognition (Kirry et al., 2018; Ciranna and Costa, 2019; Gilmartin and Ferrara, 2021). In the periphery it causes increased insulin (Shao et al., 2013) and histamine (Schubert, 2003) secretions, controls vasodilation (Baliga et al., 2013), bronchodilation (Lindén et al., 1999; Kinhult et al., 2000), modulates innate and adaptive immunity (Ganea and Delgado, 2002), alters intestinal motility (Bornstein et al., 2004) and stimulates cellular proliferation as well as differentiation (Nowak and Zawilska, 2003; Vaudry et al., 2009; Prisco et al., 2019; Karpiesiuk and Palus, 2021). In the CNS, PACAP displays pleiotropic activity, including functions as a hypophysiotropic hormone (Vélez and Unniappan, 2020), neuromodulator, and neurotrophic factor (Sadanandan et al., 2021). PACAP is also involved in the rhythmicity of melatonin production and in the increase of cAMP in birds (Nakahara et al., 2002).
ADCYAP1 gene encodes pituitary adenylate cyclase-activating polypeptide (Bakalar et al., 2021) which contributes to energy homeostasis (Rudecki and Gray, 2016; Bozadjieva-Kramer et al., 2021; Gastelum et al., 2021), and astroglial functions are modulated by PACAP (Masmoudi-Kouki et al., 2007; Hansson et al., 2009; Nakamachi et al., 2011; Kong et al., 2016; Seo and Lee, 2016; Kambe et al., 2021), and respond to many metabolic demands regulating a wide array of physiological processes (Murat and García-Cáceres, 2021) including hippocampal-dependent behavioral functions (Johnson et al., 2020).
We previously explored how the contrasting navigation strategies of the semipalmated sandpiper (Calidris pusilla) and the semipalmated plover (Charadrius semipalmatus) during autumn migration were related to hippocampal astroglia morphology (Carvalho-Paulo et al., 2018; Mendes de Lima et al., 2019; Henrique et al., 2020). This comparative analysis of morphological features to classify astrocytes revealed there were two types of morphological astrocytes influenced in different ways by contrasting long-distance migratory flights suggesting distinct physiological roles for these cells (Carvalho-Paulo et al., 2018; Henrique et al., 2020).
Because the hippocampus integrates all information related to bird’s migratory behavior (Mouritsen et al., 2016; Bingman and MacDougall-Shackleton, 2017) we searched in this exploratory investigation for an association between astrocyte morphological complexity, size of microsatellites of the ADCYAP1 allele and migratory distances in four species: the spotted sandpiper (Actitis macularius), the semipalmated sandpiper (C. pusilla), the semipalmated plover (C. semipalmatus), and the non-migratory collared plover (Charadrius collaris).
We hypothesized that astrocyte morphological complexity, migratory distances, and size of the ADCYAP1 allele will be correlated and to test this hypothesis we selected three long-distance migrant species of shorebird and one non-migrant.
Materials and Methods
Sampling Area
For both ADCYAP1 analysis and astrocyte morphometry, we collected adult individuals with mist-nets during the wintering period in the mangroves of the Amazon River estuary. All individuals were collected between 2012 and 2017, in the northeast of Pará state, at the municipality of Bragança, Pará, Brazil, on Canela Isle (0° 47′33.52″ S 46° 43′ 8.55″ W), Lombo Grande Isle (0 ° 47′33.52″ S 46 ° 43′8.55″ W), Praia do Pilão (0 ° 47′46.08″ S 46 ° 40′29.64″ W), Baiacu Beach (0 ° 47′32.55″ S 46 ° 46′52.05″ W), Quatipuru Mirim Beach (0 ° 46′35.61″ S 46 ° 52′57.66″ W) and Otelina Isle (0 ° 45′42.57″ S 46 ° 55′ 51.86″ W). Captured wintering birds included C. semipalmatus, C. pusilla, and A. macularius. The non-migratory C. collaris, a resident of South America, was also captured and compared with the long-distance migratory species (Del Hoyo et al., 1992; Rodrigues, 2000, 2006). Immediately after capture, biometric data were obtained from all individuals.
Birds were captured in compliance with license No. 44551-2 of the Chico Mendes Institute for Biodiversity Conservation (ICMBio), minimizing discomfort during handling as much as possible.
Actitis macularius presents a pattern of migration on broad fronts with many stopover sites and a broad dispersion on spring and summer grounds, whereas C. pusilla has a narrow band of migration and moderate dispersion on spring and summer sites (Reed and Oring, 1993; Billerman et al., 2020). Migration timing for these species is very similar but greater migratory distance is performed by C. pusilla compared to A. macularius (Skagen et al., 1999). C. semipalmatus flies towards coastal areas in the southern United States, the Caribbean and much of South America. Like A. macularius, C. semipalmatus travels long distances with flights interrupted for resting and feeding (Campos et al., 2008; Serrano, 2010; Nóbrega et al., 2015).
ADCYAP1: Microsatellite Genotyping
Microsatellites have been used in ecological and conservation studies since the 1990s (Moura et al., 2017). The comparison between the length of the alleles and the nucleotide composition of the base of the sequence makes it possible to identify variation in the microsatellites (Ellegren, 2004). Allele size variation is identified by polymerase chain reaction (PCR) amplification (Primmer et al., 1996). In the present report we carried out DNA isolation and purification from stored blood samples of 53 individuals with distinct migratory behaviors: A. macularius (n = 12), C. pusilla (n = 14), C. semipalmatus (n = 13), and C. collaris (n = 14). After blood collection (less than 100 μL), all captured animals were released back into the wild, except for five individuals of each species used in morphometric studies. We followed the recommendations of the DNA extraction protocol of Wizard® Genomic Purification Kit (PROMEGA).
For PCR, specific primers were used that flank microsatellite repetitions of the ADCYAP1 locus (Steinmeyer et al., 2009). To amplify the ADCYAP1 loci, the M13 tail technique proposed by Schuelke (2000) was used. The principle of the technique is to use primer pairs that flank repetitive DNA sequences to amplify samples of genomic DNA and to examine the size of the amplified alleles on a sequencing device (Boutin et al., 1997; Boutin-Ganache et al., 2001). The PCR reaction was performed using a total volume of 13 μL containing 5 ng of DNA, 10 μL Buffer, 1.5 mM MgCl2, 1.2 mM dNTP, 8 pM M13 probe and reverse primer, 0.5 pM of primer forward and 1 unit (U) of Taq DNA polymerase. To find the best hybridization temperature for the studied species, PCRs were performed with temperature gradients between 50 and 60°C. The PCR reaction consisted of an initial denaturation of 94°C for 5min, followed by 30 cycles of 94°C for 30s, 51°C for 45s and 72°C for 45s, followed later by 8 cycles of 94°C for 30s, 53°C for 45s and 72°C for 45s, with a final extension of 72°C for 10min [for more details on PCR see Schuelke (2000)]. We used the standard microsatellite genotyping method in the ABI 3500XL fragment analyzer (GeneMapper, Applied Biosystems). Subsequently, peak patterns were analyzed by the Fragment Profiler 1.2 program (Amersham Biosciences) and organized in Microsoft Excel 2019.
To detect and identify genotyping errors resulting from null alleles, allele drop out and stuttering related to ADCYAP1 locus, we used the software MICROCHECKER (Barros et al., 2020). We used ARLEQUIN v3.5 (Excoffier et al., 2007) to measure genetic diversity in terms of number of alleles per locus (A), observed (HO), and expected (HE) heterozygosity (Nei, 1978). We tested for Hardy–Weinberg Equilibrium (Mayo, 2008; Meirmans et al., 2018) and analyzed population differentiation at gene ADCYAP1 through FST and RST statistics (Meirmans, 2020) with a significance level α < 0.05.
Immunohistochemistry
Five individuals of each species were used for morphometric astrocyte studies. All birds were anesthetized with Isoflurane (Olkowski and Classen, 1998), euthanized with an anesthetic overdose, and perfused transcardially with 0.1% heparinized phosphate buffered saline (PBS) for 10 min, followed by 4% paraformaldehyde pH 7.2–7.4 for another 30 min. After craniotomy, brains were removed and stored in 9% phosphate buffer (Sigma Aldrich - S3264) and then cut in the coronal plane. Eighty micrometer thick sections were obtained using a vibrating blade microtome (LeicaVibratomeVT1000S). Serial anatomical sections (1:6 interval) were subjected to immunohistochemical reactions using GFAP selective marker for astrocytes.
Three-Dimensional Reconstruction
In previous reports we used three-dimensional microscopic reconstructions and applied hierarchical cluster analysis to classify astrocytes based on morphometric features using the largest Euclidean distance between the groups. We found two large morphological families (Type I and Type II). These families were differentially affected by contrasting migratory patterns (Carvalho-Paulo et al., 2018; Henrique et al., 2020). Here, we performed hierarchical clustering on variance-shrunk logarithmized values of multimodal morphometrical features to define the number of morphological families and compared hippocampal astrocyte morphologies of long-distance migratory birds with contrasting migratory flights (C. semipalmatus, C. pusilla, and A. macularius) with a non-migratory specie (C. collaris).
For the three-dimensional reconstruction of positive GFAP astrocytes, an optical microscope (Eclipse Ci, NIKON) with motorized stage and analog-digital converters (MAC6000 System, Ludl Electronic Products, Hawthorne, NY, United States) was used. This system was coupled to a microprocessor that controlled the movements of the microscopic stage with the aid of a specialized program (Neurolucida, MBF Bioscience, Williston, VT, United States) to store the spatial information (X, Y, Z coordinates) of each digitized point of interest. Three types of cells were identified: protoplasmic, fibrous, and radial astrocytes similar to previous descriptions (Carvalho-Paulo et al., 2017; Mendes de Lima et al., 2019; Henrique et al., 2020). In this study, we used only protoplasmic astrocytes.
The contours of the hippocampal formation (Atoji and Wild, 2004; Atoji et al., 2016) were determined on a low power objective (4× lens). To identify astrocyte morphological details and to ensure greater detail in 3D reconstructions, the low power lens was replaced by a high-power oil immersion 100× lens PLANFLUOR (NA 1.3; DF = 0.2 μm; Nikon, Japan).
A total of 264 astrocytes from the hippocampal formation of A. macularius, 251 of C. pusilla, 302 of C. semipalmatus, and 260 of C. collaris were reconstructed in three dimensions. To select astrocytes for reconstruction a random and systematic stereological sampling approach was adopted (West, 2002). For this, we used squared probes (50 μm × 50 μm) separated from each other by a 900 μm × 900 μm grid interval (Figure 1). The grid interval was estimated to obtain a minimum of 50 reconstructed astrocytes per animal and it was selected based on the total area of the hippocampal formation. The number of probes per section was proportional to the area of the hippocampal formation of each section.
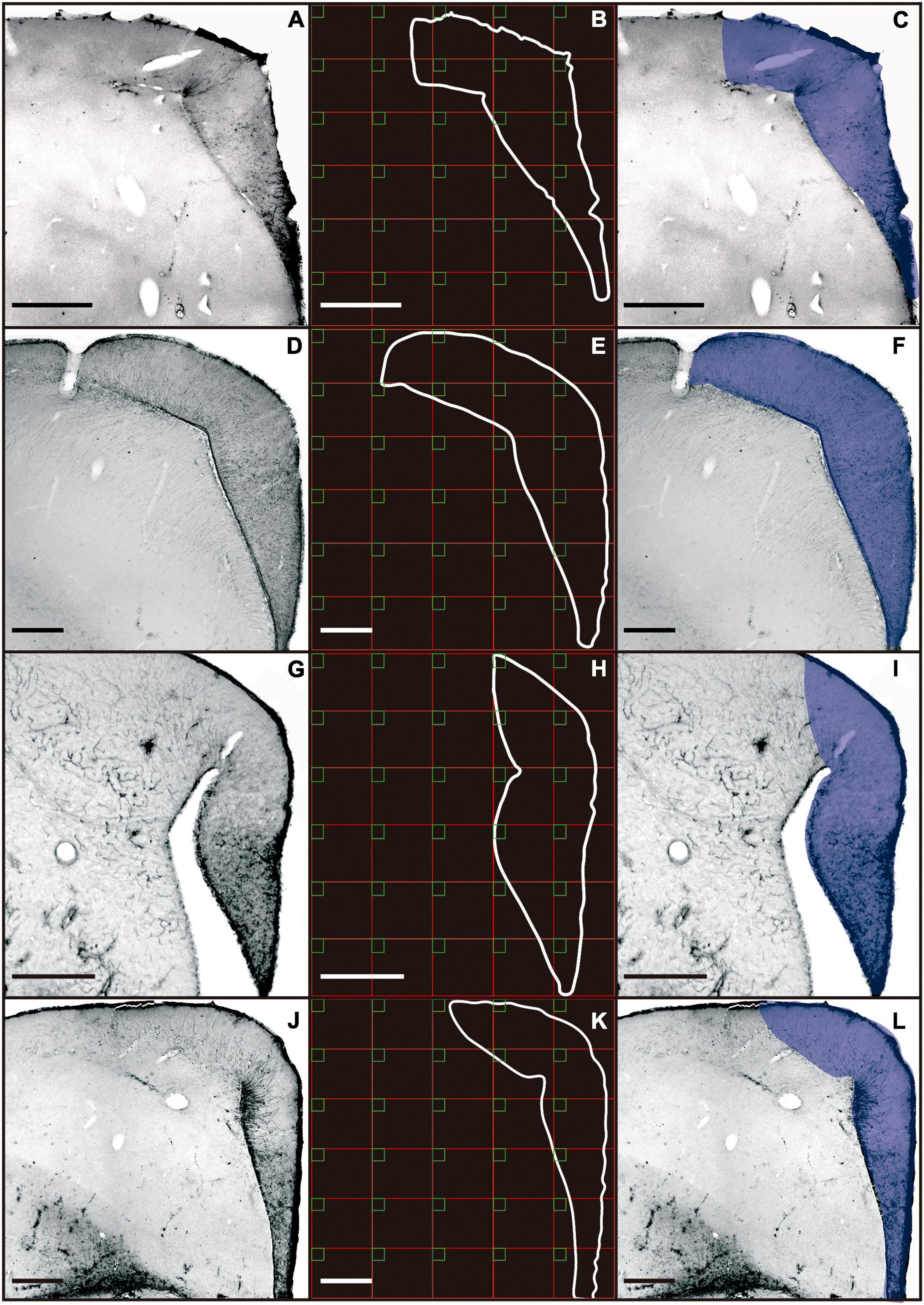
Figure 1. Photomicrographs of coronal sections of the telencephalon of C. collaris (A), C. semipalmatus (D), C. pusilla (G), and A. macularius (J). Outline of the hippocampal formation, and sampling grid (B,E,H,K). Borders of the hippocampal formation are defined by blue line (C,F,I,L). Scale bars: (A) 250 μm; (D) 500 μm; (G) 500 μm; (J) 500 μm.
Only astrocytes located within the limits of the probe box were selected for analysis. In cases where there were no cells within the probe box that met the pattern of complete immunostaining and branch integrity, the closest astrocyte outside the probe box border was chosen for reconstruction. Due to minimal shrinkage in the X/Y axes, the correction for shrinkage induced by histological processing was applied exclusively for the z axis, and corresponded to 1.75×, as previously recommended by Carlo and Stevens (2011).
Supplementary Table 1 shows the definition of all morphometric features of hippocampal astrocytes. The following equation adapted from previous neuronal dendritic reconstruction (Pillai et al., 2012) was used to estimate morphological complexity:
Complexity =[Sum of the terminal orders + Number of terminals] × [Total branch length/Number of primary branches]
Thus, 20 morphological variables were used for morphometry: total branch length, branch surface area, tortuosity, total branch volume, base diameter of primary branches, total number of segments, segments/mm, number of branching points, tree surface area (μm2), planar angle, complexity, convex hull perimeter (μm), convex hull area (μm2) 2-D, convex hull surface area (μm2), convex hull volume (μm3), and Vertex: Va, Vb, Vc, K-dim (fractal dimension) (see Supplementary Table 1 for details).
Statistical Analysis
Morphometry
With a total of 1,077 reconstructed astrocytes from groups of migrant and non-migrant birds, we performed a multivariate statistical analysis using 20 morphological parameters. This procedure was used to identify possible morphological clusters within each species (Schweitzer and Renehan, 1997; Yamada and Jinno, 2013). Morphometric data for all astrocytes were obtained using Neuroexplorer software (MicroBright Field Inc.). To search for morphological characteristics shared by astrocytes, only quantitative morphometric variables with multimodality indices (MMI) greater than 0.55 were selected, to identify which variables were multimodal or at least bimodal. MMI was estimated based on the parameters of asymmetry and kurtosis of each morphometric variable, MMI = [M32+1]/[M4+3(n−1)2/(n−2)n−3)] in which M3 is asymmetry, M4 is kurtosis and n is the sample size (Schweitzer and Renehan, 1997).
Hierarchical cluster analysis using Ward’s method or Ward’s Minimum Variance Clustering Method (Ward, 1963) was applied to multimodal variables to classify cells (Schweitzer and Renehan, 1997). Variance-shrunk logarithmized values of multimodal morphometrical features were then submitted to cluster analysis. The morphometric variables used in the cluster analysis (MMI > 0.55) were subjected to discriminant analysis, using Statistica 12.0 software. This procedure identifies which variables contribute most to the formation of clusters. The software compares matrices of total variances and covariances using multivariate F tests to determine if there are significant differences between groups (for all variables). In the analysis of the step-forward discriminant function, the program builds a step-by-step discrimination model. In this model, at each test stage, all variables are reviewed and evaluated to determine which variable contributes the most to discrimination between groups. If any variable did not have a p-value below 0.05 or did not occur in all studied species, it was disregarded in the subsequent analyses so that we could detect the morphometric variables that provided the best separation between the astrocyte morphological classes suggested by the cluster analysis.
Following discriminant analysis, we found morphological complexity and convex hull volume to be the variables shared by all species that contributed most to cluster formation. We did an initial test of normality (Shapiro–Wilk) (Shapiro and Wilk, 1965) and homogeneity of variances (Levene, 1960) (Supplementary Table 2) and then transformed the scale of complexity values into decimal log units to perform an independent univariate General Linear Model (GLM) test (Nelder and Wedderburn, 1972). We used the Sidak test (Salkind, 2007) for paired tests, and for effect size used the Cohen d test (Thalheimer and Cook, 2002).
For a priori multivariate comparison tests, data with continuous variables were transformed into values of Log (X + 1) (Clarke and Warwick, 2001) and normalized (Anderson et al., 2008) to correct additivity effects of factor and scale diversity, respectively. Then we generated Euclidean similarity matrix where the data were exchanged to verify significant differences (setting α = 0.05) through dispersion homogeneity tests (PERMIDISP) (Anderson et al., 2006) and the Analysis of Variance by Multivariate Permutation (PERMANOVA) (Anderson et al., 2008).
In PERMIDISP, we verified whether the differences found were associated with sample dispersion using the distance protocol between the centroids with 9,999 permutations and paired tests. In order to have the values of pseudo-F in PERMANOVA, we verified possible differences in the location of the samples by treating the distance matrices using the residual permutation method under a reduced model (“residuals under a reduced model”) with 9,999 repetitions, sum of squares “type III” (McArdle and Anderson, 2001) and paired tests (pseudo-t). The tests were considered two factor (“species” and “type”) and treated as fixed. All tests were generated using the PRIMER E software (Anderson et al., 2008).
Analysis of ADCYAP1 Microsatellites
The migration distance traveled by each migrant species was estimated using information available on the departure of birds from North America (breeding site) to their arrival at the isles of Bragança estuarine region in South America (wintering site) (Reed et al., 2013; Nóbrega et al., 2015; Billerman et al., 2020; Hicklin and Gratto-Trevor, 2020). C. semipalmatus, C. pusilla, and A. macularius are long-distance migratory birds whereas C. collaris is classified as a resident species of South America (Piersma and Wiersma, 1996). C. semipalmatus travels around 8,039 km, while C. pusilla travels 9,309 km and A. macularius around 13,139 km. We adopted 0 (zero) for the migratory route of C. collaris (Reed et al., 2013; Nóbrega et al., 2015; Billerman et al., 2020; Hicklin and Gratto-Trevor, 2020) (see Figure 2).
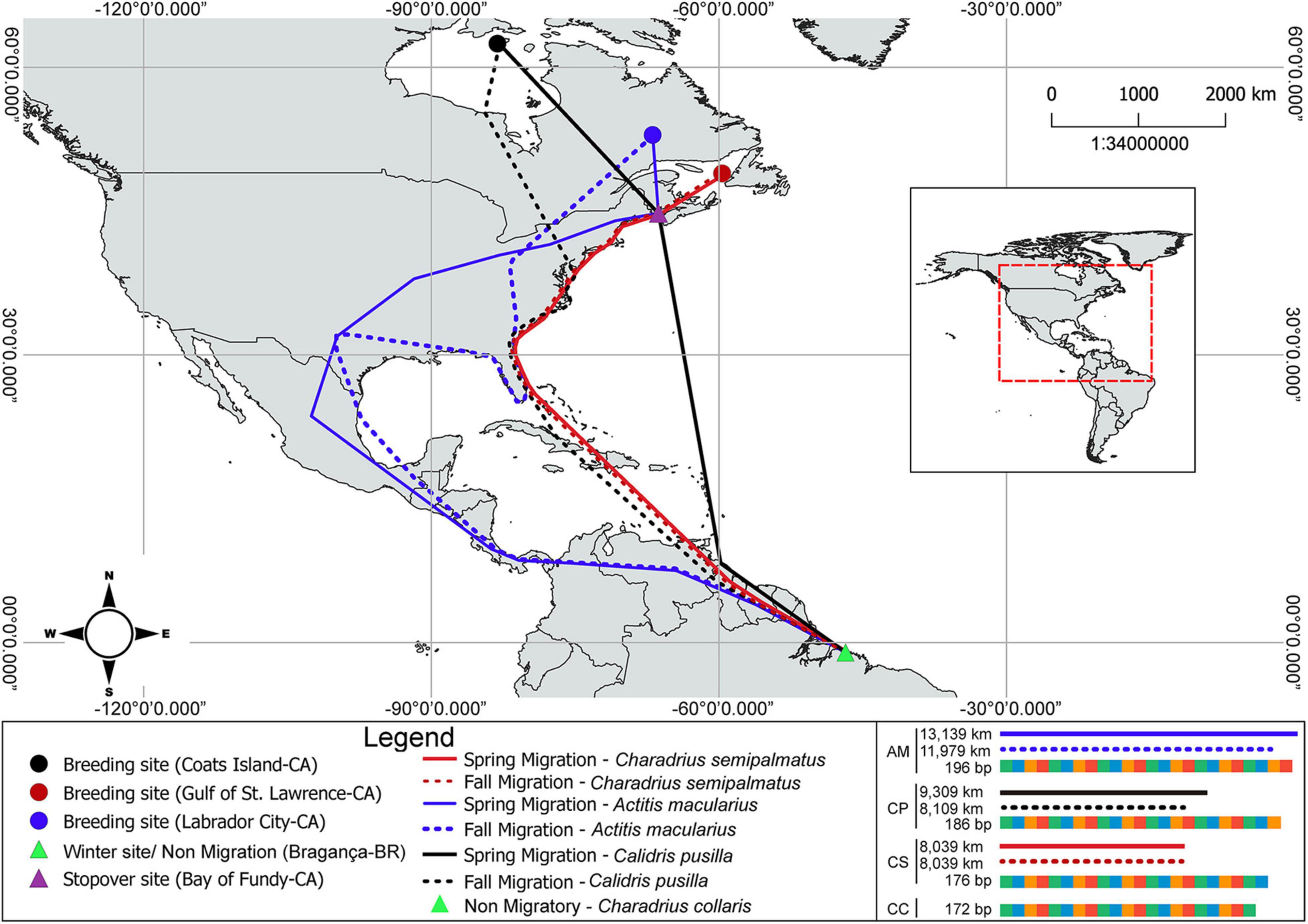
Figure 2. Breeding sites and migratory routes to the wintering area of C. semipalmatus, C. pusilla, and A. macularius. Lower right panel shows the size of the ADCYAP1 gene.
Because our samples were small (n < 30) and the data did not follow a normal distribution, a Spearman rank correlation coefficient was calculated to assess the degree of correlation between migratory distance and size of the microsatellites.
To detect differences in the size of the microsatellites between the species, PERMANOVA was performed, as an analogue to univariate ANOVA. We followed the same criteria described above in the morphometric analyses. For this test, however, we used the residual exchange method under an unrestricted model with 9,999 repetitions with sum of squares for “Type III” (McArdle and Anderson, 2001) in the PRIMER E software (Anderson et al., 2008).
Photomicrographs and Post-Processing
For photomicrographs, a digital camera (Microfire, Optronics, CA, United States) coupled with a Nikon microscope (Eclipse Ci, NIKON) was used, and acquired images were post-processed for brightness and contrast with Adobe Photoshop software (Adobe Inc San José, CA, United States). We selected images of the most representative astrocytes of each cell type indicated by the hierarchical cluster analysis. For the choice of the representative cell of each group (“average cell”), the distance matrix was used to obtain the sum of the distances of each cell relative to all others. It is assumed that the cell that best represents a group has the smallest sum of distances. The matrices were constructed with the combination of all cells of a given group taken pairwise, followed by the weighted calculation of a scalar Euclidean distance between cells using all morphometric variables (Henrique et al., 2020).
Results
Size of Microsatellites in ADCYPA1
We identified 17 alleles for the ADCYAP1 locus, varying in size from 168 to 204 base pairs (bp). The most common alleles found were 172 and 174 bp. The ADCYAP1 locus was polymorphic in all four species with the number of alleles varying from 5 to 6, and number of exclusive alleles varying from 1 to 4 across populations (Supplementary Table 3). Analysis showed no evidence of null alleles and genotype errors such as stuttering, or allele drop out. However, two out of the four populations exhibited significant deviation (p < 0.01) from Hardy–Weinberg equilibrium and higher numbers of homozygotes compared to the other populations. Observed and expected heterozygosity ranged from 0.417 to 0.786 and from 0.712 to 0.792, respectively (Supplementary Table 4).
All pairwise Fst and Rst values based on ADCYAP1 allele frequencies presented significant differentiation across species, which was expected since all are distinct and known species (Supplementary Table 5). Higher levels of genetic differentiation were observed across species pairwise comparisons and low levels were observed between species in the Charadrius genus, corroborating the evolutionary relations of these groups.
The association of size of microsatellites with migratory distance showed a positive and strongly supported association (Spearman correlation test, Rho = 0.915; p = 0.000; Figure 3 and Supplementary Table 6).
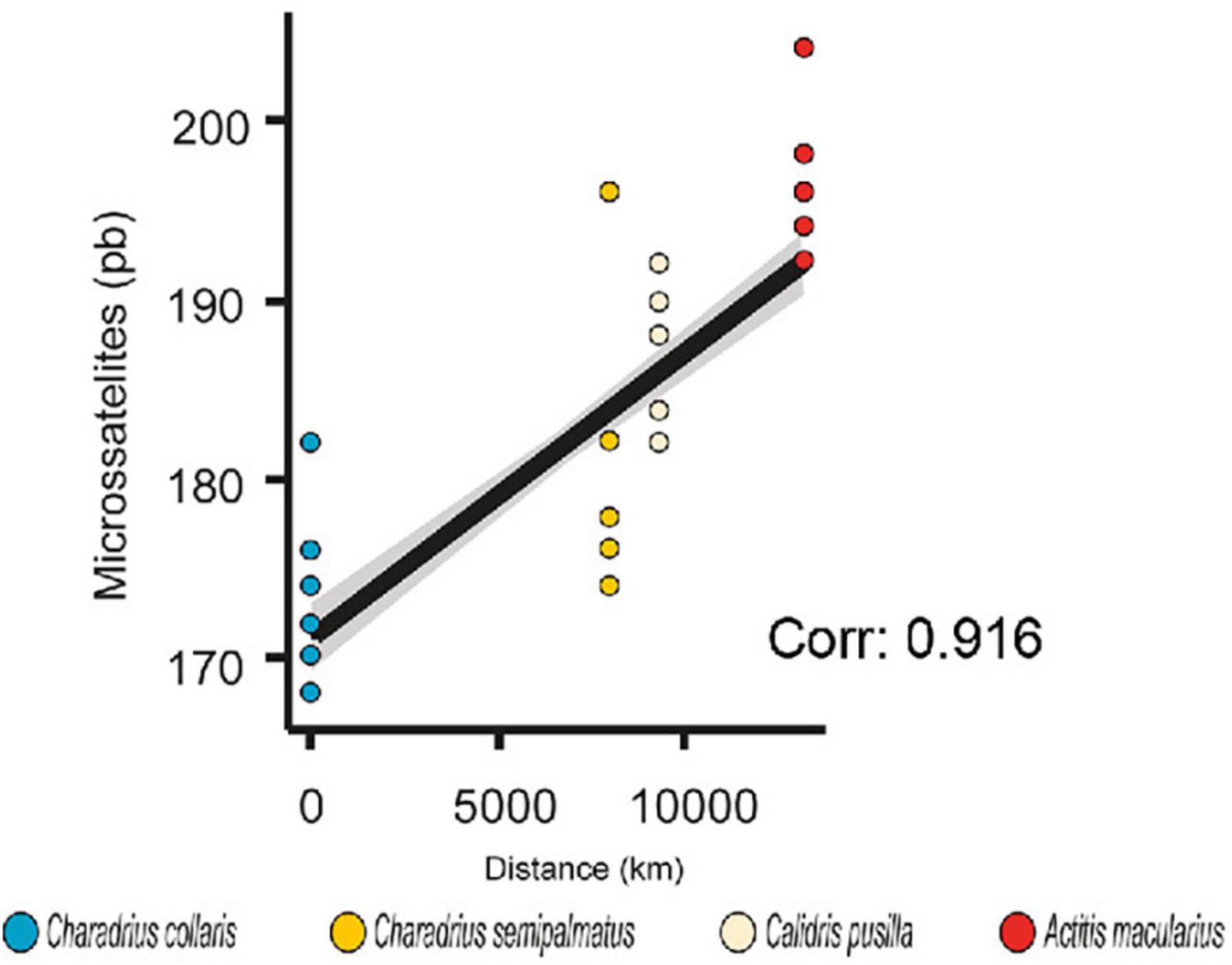
Figure 3. Comparison and correlation tests in microsatellites with migratory distance. Linear regression (R = 0.840) and Spearman’s correlation (Rho = 0.916) showing positive association between size of ADCYAP1 alleles as pair of bases (pb) and migratory distance.
We also performed a comparison between the size of the microsatellites in the four species (Supplementary Table 7). We found significant differences (PERMANOVA, F = 180.98, p = 0.0001) in all possible comparisons (C. semipalmatus vs C. collaris, p < 0.026; C. semipalmatus vs C. pusilla, p < 0.025; C. semipalmatus vs A. macularius, p < 0.0001; C. collaris vs C. pusilla, p < 0.0001; C. collaris vs C. pusilla, p < 0.0001; A. macularius vs C pusilla, p < 0.0127).
Three-Dimensional Reconstruction of Astrocytes in Hippocampal Formation
Stellate astrocytes in the hippocampal formation of A. macularius, C. pusilla, C. semipalmatus, and C. collaris show glial fibrillary acidic protein expression in the cell body, from which GFAP positive primary thicker branches emerge and progressively ramify, terminating as tiny branches (Figure 4).
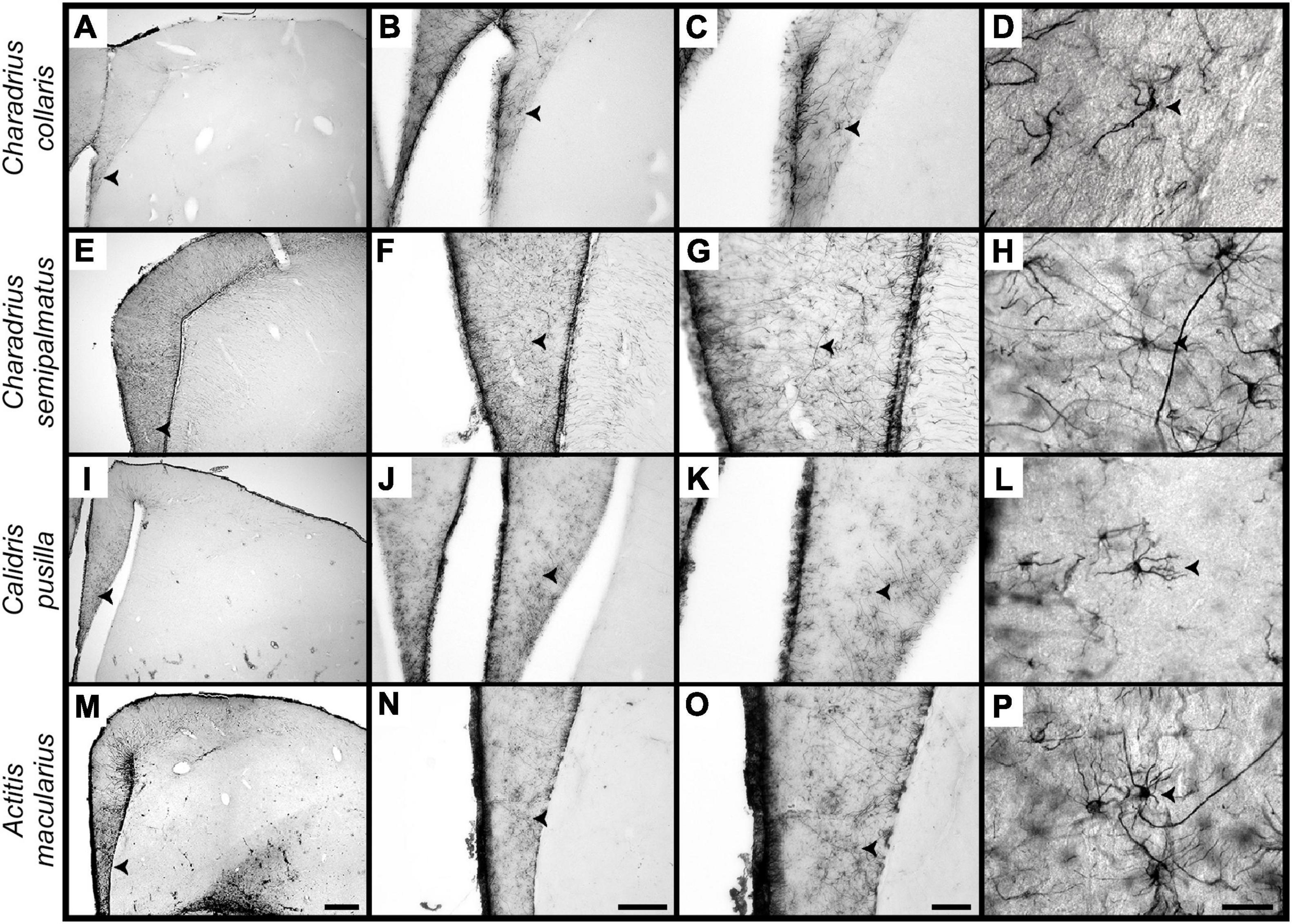
Figure 4. Photomicrographs of brain sections to illustrate stellate astrocytes of the hippocampus V region of non-migrant (C. collaris) and migrant (C. semipalmatus, C. pusilla, and A. macularius) species. From top to bottom rows correspond to C. collaris, C. semipalmatus, C. pusilla, and A. macularius. (A–P) Are photomicrographs from hippocampal sections of C. collaris, C. semipalmatus, C. pusilla and A. macularius, respectively, to illustrate GFAP stellate astrocytes of each species at different magnifications. Detailed three-dimensional reconstructions of these cells were done using high-power (100x) microscope lens. Scale bars: (A,B,G,L) = 250 mm; (F,K,P) = 500 mm; (C,H,M) = 120 mm and (D,I,N) = 60 mm; (E,J,O) = 25 mm.
From hierarchical cluster and discriminant function analysis emerged that in the non-migratory species, the morphological complexity, the volume of the convex hull and the number of segments were the multimodal variables that most contributed to the formation of clusters (Figures 5A,B). In the analysis of the canonical discriminant function, it was shown that the complexity and volume of the convex hull accounted for 99.4% of the variance (Figures 5C,D). Wilks’s lambda value correspondent to 1 and 2 canonical discriminant functions was the most important for group separation (Wilks’s lambda = 0.185; p = 0.000) (Figure 5E) with 95.4% of the group data classified correctly (Figure 5F).
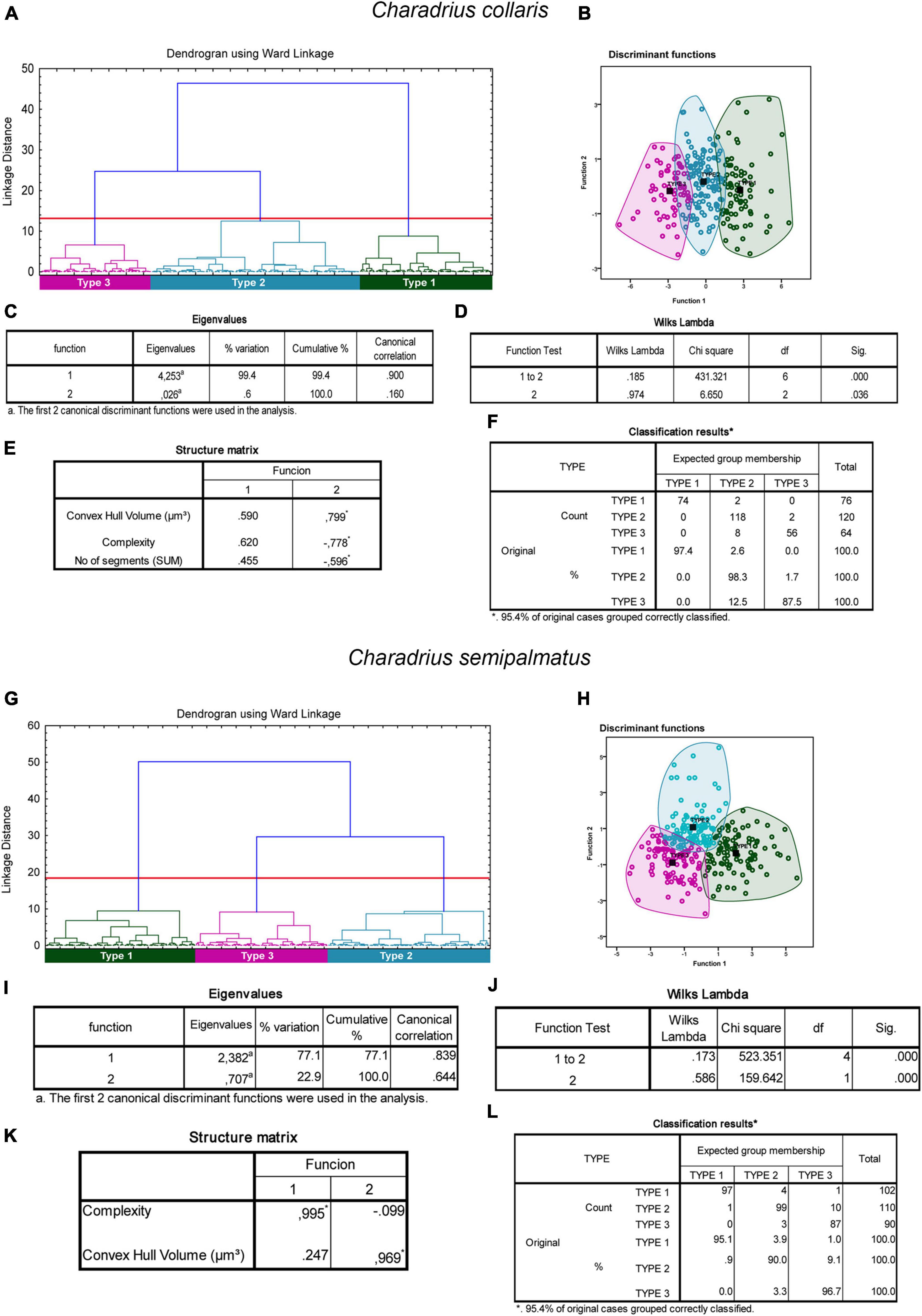
Figure 5. Cluster analysis of the morphology of astrocytes from rostral, intermediate, and caudal regions of the hippocampal formation of Charadrius collaris (A–F) and Charadrius semipalmatus (G–L). Dendrogram representation of the hierarchical cluster analysis (Ward’s method) of the 260 cells of five individual C. collaris (A). Notice three main morphological phenotypes named Type I (green), Type II (blue), and Type III (magenta). Graphic representation of the canonical discriminant function analysis illustrates the distribution of the three main clusters of astrocytes in the Euclidean space (B). Colored circles green, blue, and magenta identify individual astrocytes of Type I, Type II, and Type III morphotypes. The variables that most contributed to the cluster’s formation were morphological complexity and convex hull volume. The eigenvalues output (C) indicates that function 1 explains 99.4% of the variance. Wilks’s lambda values in the function test 1–2, rejected the null hypothesis of no differences between the groups for these 2 variables (0.185; p = 0.000). In the structure matrix panel (E) is displayed the relative contributions of the variables to the cluster formation of functions 1 and 2. (G) Dendrogram representation of hierarchical cluster analysis (Ward’s method) of 302 reconstructed astrocytes of C. semipalmatus (n = 5). Three main morphological phenotypes named Type I (green), Type II (blue), and Type III (magenta) were found. Graphic representation of the canonical discriminant function analysis illustrates the distribution of the three main clusters of astrocytes in the Euclidean space (H). Colored circles green, blue, and magenta identify individual astrocytes of Type I, Type II, and Type III morphotypes. The variables that most contributed to the cluster’s formation were morphological complexity and convex hull volume. The eigenvalues output (I) indicates that function 1 explains 77.1% of the variance. Wilks’s lambda values in the function test 1–2 (J), rejected the null hypothesis of no differences between the groups for these 2 variables (0.173; p = 0.000). In the structure matrix panel (K) is displayed the relative contributions of the variables to the cluster formation of functions 1 and 2. Classification results for the expected group membership (F). Black squared dots indicate de centroid of each cluster. (*) indicates statistically significant difference.
In migratory species, the morphological complexity, volume, surface, area, and perimeter of the convex hull were the multimodal variables that contributed most to the cluster formation in the different species (Figures 5, 6). The morphological complexity and volume of the convex hull were the morphometric features that contributed most for cluster formation in all species (Figures 5I,J, 6C,D,I,J). Function 1 explained 77.1, 98.4, and 94.7% of the sample variance in the species C. semipalmatus, C. pusilla, and A. macularius, respectively (Figures 5C,D). Wilks’s lambda value correspondent to 1 and 2 canonical discriminant functions was the most important for groups separation in the species C. semipalmatus and C. pusilla (Wilks lambda: C. semipalmatus = 0.185; p = 0.000; C. pusilla = 0.211; p = 0.000) (Figures 5K, 6E) and Wilks’s lambda value correspondent to 1 and 3 canonical discriminant functions was the most important for groups separation in A. macularius (Wilks lambda = 0.141; p = 0.000) (Figure 6K) with 95.4% of the group data correctly classified in the three species (Figures 5H, 6F,H).
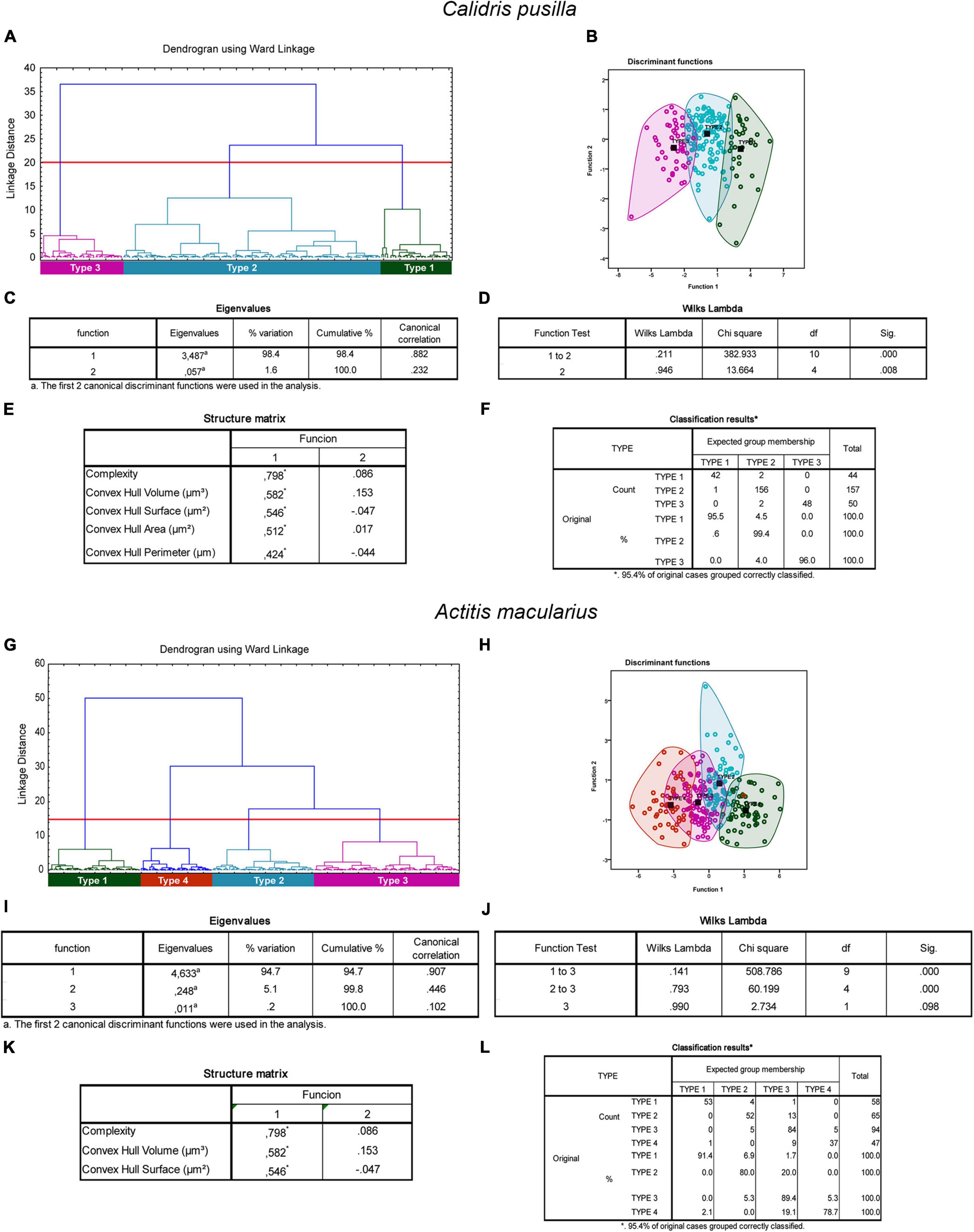
Figure 6. Cluster analysis of the morphology of astrocytes from rostral, intermediate, and caudal regions of the hippocampal formation of Calidris pusilla (A–F) and Actitis macularius (G–L). Dendrogram representation of the hierarchical cluster analysis (Ward’s method) of the 251 cells of five individual C. pusilla (A). Notice three main morphological phenotypes named Type I (green), Type II (blue), and Type III (magenta). Graphic representation of the canonical discriminant function analysis illustrates the distribution of the three main clusters of astrocytes in the Euclidean space (B). Colored circles green, blue, and magenta identify individual astrocytes of Type I, Type II, and Type III morphotypes. The variables that most contributed to the cluster’s formation were morphological complexity and convex hull volume. The eigenvalues output (C) indicates that function 1 explains 98.4% of the variance. Wilks’s lambda values in the function test 1–2, rejected the null hypothesis of no differences between the groups for these 2 variables (0.211; p = 0.000). In the structure matrix panel (E) is displayed the relative contributions of the variables to the cluster formation of functions 1 and 2. Classification results for the expected group membership (F). Dendrogram representation of hierarchical cluster analysis (Ward’s method) of 264 reconstructed astrocytes of Actitis macularius (n = 5) (G). Four main morphological phenotypes named Type I (green), Type II (blue), Type III (magenta), and Type 4 (orange) were found. Graphic representation of the canonical discriminant function analysis illustrates the distribution of the four main clusters of astrocytes in the Euclidean space (H). Colored circles green, blue, magenta, and orange identify individual astrocytes of Type I, Type II, Type III, and Type IV morphotypes respectively. The variables that most contributed to the cluster’s formation were morphological complexity and convex hull volume. The eigenvalues output (I) indicates that function 1 explains 94.7%% of the variance. Wilks’s lambda values in the function test 1–3, and 2–3 (J), rejected the null hypothesis of no differences between the classified groups (Wilks Lambda = 0.141 and 0.793; p = 0.000). In the structure matrix panel (K) is displayed the relative contributions of the variables to the cluster formation of functions 1 and 2. Classification results for the expected group membership (L). Black squared dots indicate de centroid of each cluster. (*) indicates statistically significant difference.
After evaluation of the data to select multimodal variables for hierarchical cluster analysis using variance-shrunk (logarithmized) parameters, we identified the groups in the dendrogram with statistically significant differences, followed by canonical discriminant analysis (Wiltschko W. and Wiltschko, 2012) (see, Figures 5–7). The canonical discriminant function analysis performed very well demonstrating its ability to predict astrocyte morphology, with high classification accuracy of individuals in the three different groups in C. collaris (97.4% for type I, 98.3% for type II and 87.5% for type III), and C. semipalmatus (95% for type I, 90% for type II and 96.7% for type III) and C. pusilla (95.5% for type I, 99.4% for type II and 96% for type III) and in four different groups in A. macularius (91.4% for type I, 80% for type 2, 89.4% for type III and 78.7% for type IV), confirming the existence of three distinct groups in C. collaris, C. semipalmatus, and C. pusilla and four groups in A. macularius. See Figures 5, 6 for details.
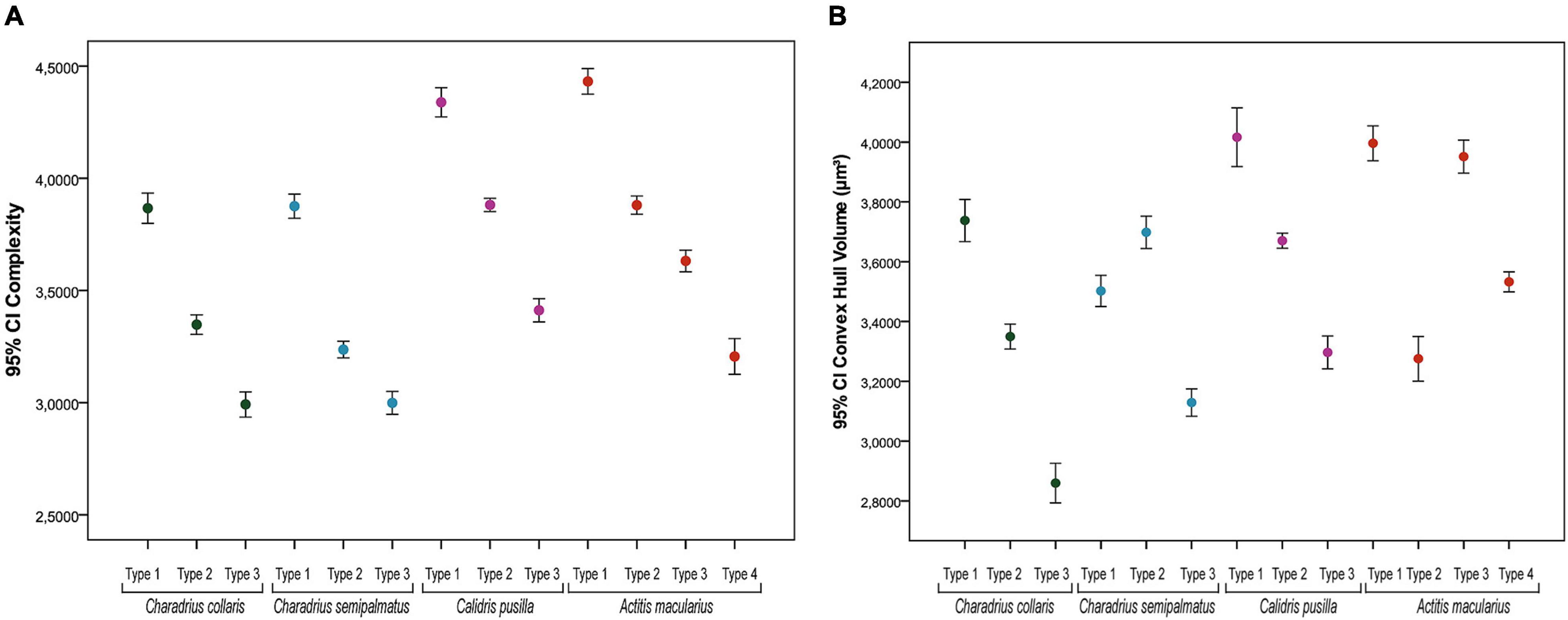
Figure 7. Mean values of morphological complexity (A) and of the convex hull volume (B) of astrocytes from the hippocampal formation of migratory (C. semipalmatus = blue, C. pusilla = magenta, and A. macularius = orange) and non-migratory (C. collaris = green dots) bird species and their confidence intervals (CI). Except for Actitis macularius which shows four groups of morphotypes (types I–IV) the dots indicate mean values for three morphotypes (types I–III). Whiskers show 95% CI. All species showed statistically significant differences in the mean values of morphological complexity for all comparisons between morphotypes. Intervals that do not overlap represent statistically significant differences (p < 0.05).
The comparative analysis between species, with all morphotypes revealed significant differences in all comparisons for morphological complexity [PERMANOVA: C. collaris—F (3;260) = 190.33, p = 0.0001; C. semipalmatus—F (3;302) = 226.65, p = 0.0001; C. pusilla—F (3;251) = 164.06, p = 0.0001; A. macularius—F (4;264) = 216.8, p = 0.0001] and significant differences in pairwise comparisons within all species (p = 0.0001) (Supplementary Tables 8–11).
Figure 7 is a graphic representation for these findings of morphological complexity and convex hull volume. As mentioned before these are the variables that contributed most to cluster formation in all species. It is important to highlight that there was no linear correspondence between morphotype mean values of convex hull volume in C. pusilla (with three morphotypes) and A. macularius (with four morphotypes) in the graphic representation of this variable in Figure 7.
The percent distribution of each morphotype in each species is shown in Figure 8. The semipalmated sandpiper C. pusilla had a higher percentage of type II (62.55%) than type I (17.53%) or type III (19.92%), and these values contrast with the percentual distributions of type II, type I, and type III astrocytes of C. semipalmatus (36.42, vs 33.47 vs 29.8%), A. macularius (24.62 vs 21.97 vs 35.61 vs 18% of type IV), or C. collaris (45.98 vs 29.12 vs 24.52%) (Figure 8). Thus, except for A. macularius where type III showed higher frequency, astrocytes of intermediate morphological complexity (Type II morphotype) are more frequent in all other species. Please remember that Type I designates the morphotype with greater morphological complexity mean value in all species.
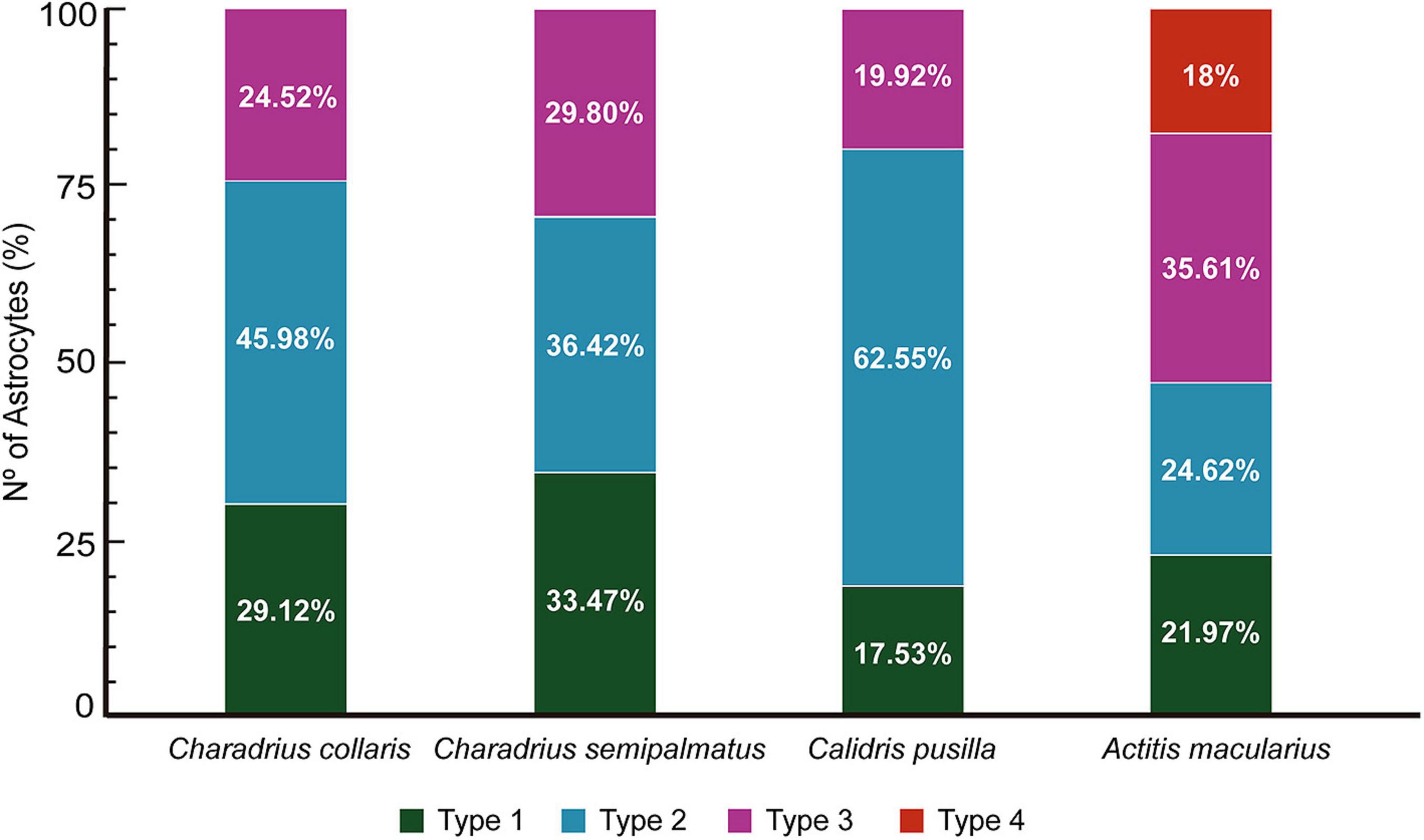
Figure 8. Relative percentage of Type I, Type II, Type III and Type IV astrocytes in the hippocampal formation of C. collaris, C. semipalmatus, C. pusilla, and A. macularius. Note that except by A. macularius where type III appears in greater proportion, astrocytes of intermediate morphological complexity (Type II) are more frequent in all other three species. Please remember that we named astrocytes based on morphological complexity as previously described in shorebirds (Carvalho-Paulo et al., 2018; da Costa et al., 2020; Henrique et al., 2020). Type I designates the morphotype with greater morphological complexity mean value in all species and that a progressive reduction in the mean values of morphological complexity is observed from type I to type IV.
Permutational analysis of multivariate dispersions of 17 morphometric features showed significant interaction between species and astrocyte type [PERMIDISP (7,1069) F: 5,277; p = 0.0001). In the paired tests for species there were differences in dispersion for almost all species (p < 0.05), except between non-migratory C. collaris and the species with the longest migratory distance A. macularius (t = 0.711; p = 0.477). Considering only the factor “type,” however, there was no significant dispersion (type I × type II; t = 0.973; p = 0.338) (see Supplementary Table 12).
Regarding the differences in Euclidean space, we found significant differences in the interaction of the factors [PERMANOVA; F (3.1069) = 5,479; p = 0.0001], “species” [F (3.1069) = 106.82; p = 0.0001] and “type” [F (3.1069) = 186.69; p = 0.0001]. All pairwise comparisons showed significant differences, including comparisons between species, between types and interactions between the two factors (p < 0.001). Thus, when we analyze the results of PERMIDISP and PERMANOVA, we can say that only the factor “type” showed differences in the location of the samples in the Euclidean space. Regarding the factors “species and “species × type” the differences found were due to the dispersion of the samples.
Figure 9 exhibits a Kernel density plot of the distribution of morphological complexity values of distinct morphotypes of hippocampal astrocytes from the dataset of C. collaris, C. semipalmatus, C. pusilla, and A. macularius. As expected, except for A. macularius where we identified four peaks, all other species showed three peaks, confirming that morphological complexity can be used in isolation to distinguish the morphotypes of astrocytes of the hippocampal formation.
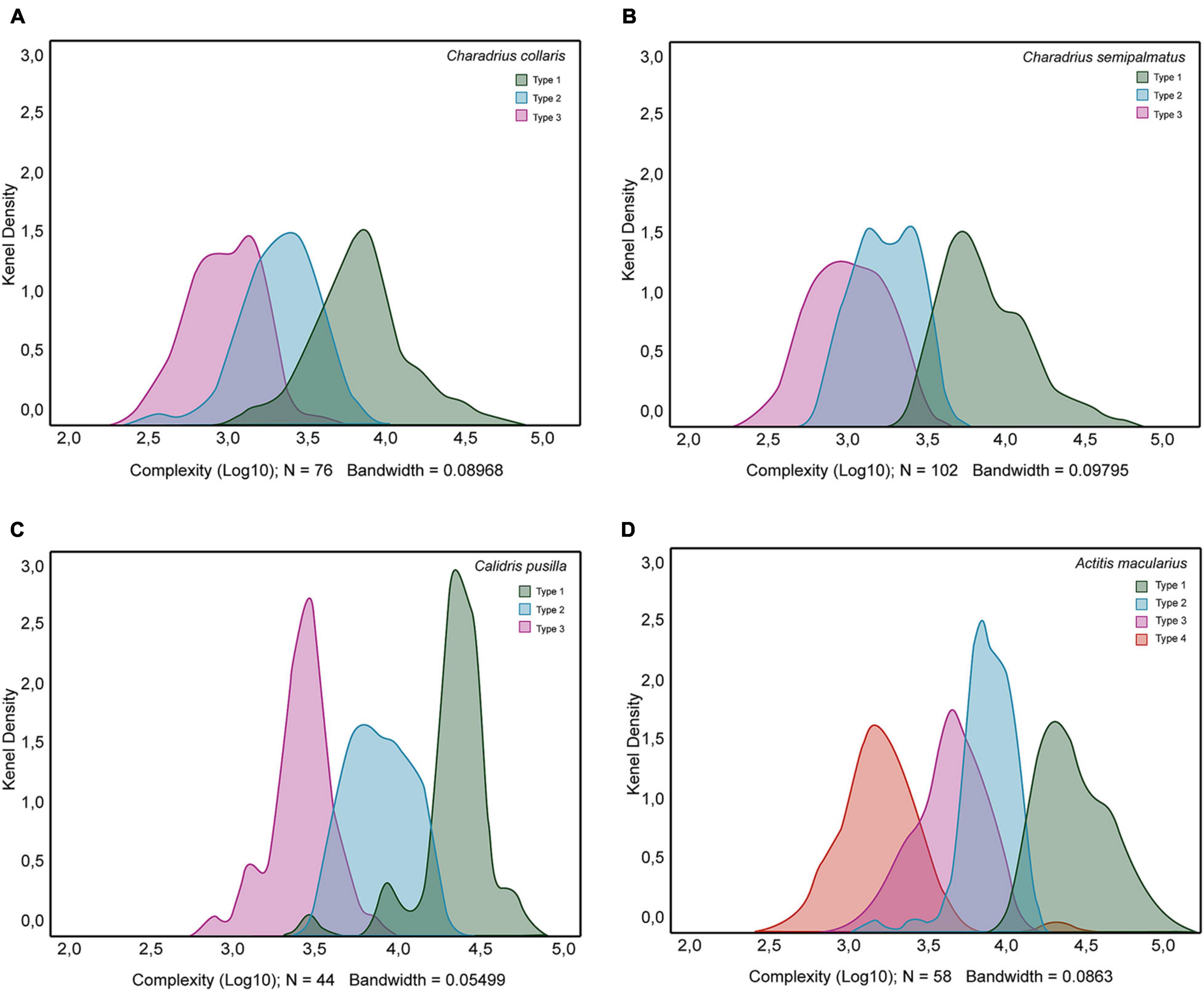
Figure 9. Kernel density plots to display where values of morphological complexity are concentrated over the interval covered by the dataset of Charadrius collaris (A), Charadrius semipalmatus (B), Calidris pusilla (C), and Actitis macularius (D). Notice that except by A. macularius where we found four peaks, in all other density plots we identified three peaks. Green, blue, magenta, and orange colors identify distribution of morphological complexity values of morphotypes I, II, III. and IV, respectively.
Another way of visualizing the influence of migratory behavior on the morphology of astrocytes is to observe the representative 3D mean cells of each group (Figure 10). While in A. macularius we named type IV the hippocampal astrocytes with the lowest complexity, in species with three morphotypes (C. collaris, C. semipalmatus, and C. pusilla) the lowest complexity astrocytes corresponded to type III. Notice the greater morphological complexity mean values of hippocampal astrocytes in migratory species as compared to the non-migratory C. collaris.
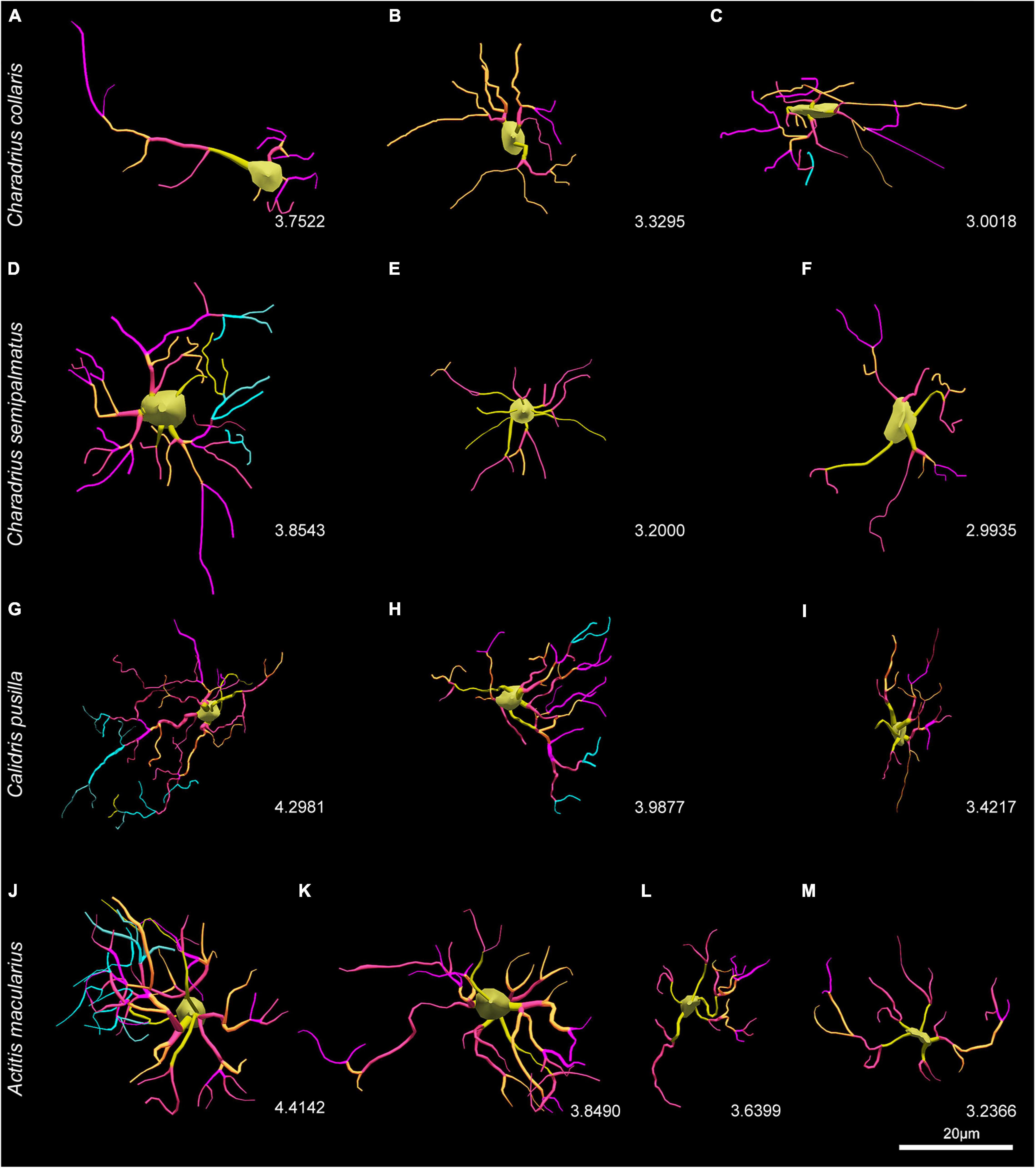
Figure 10. Three-dimensional reconstructions of representative cells of C. collaris (A–C), C. semipalmatus (D–F), C. pusilla (G–I), and A. macularius (J–M) indicating correspondent logarithmized values of morphological complexity of each morphotype. Note that the values of the decimal logarithm of the morphological complexity of the representative mean cell of each morphotype are displayed for comparison purposes between the species. Scale bars = 25 μm.
Two raw astrocytes images overlayed with branch reconstructions used to illustrate contrasting morphological values are exhibited on Figure 11. They are examples of higher and lower morphological complexity astrocytes from the hippocampal formation of shorebirds which were microscopically reconstructed in 3D.
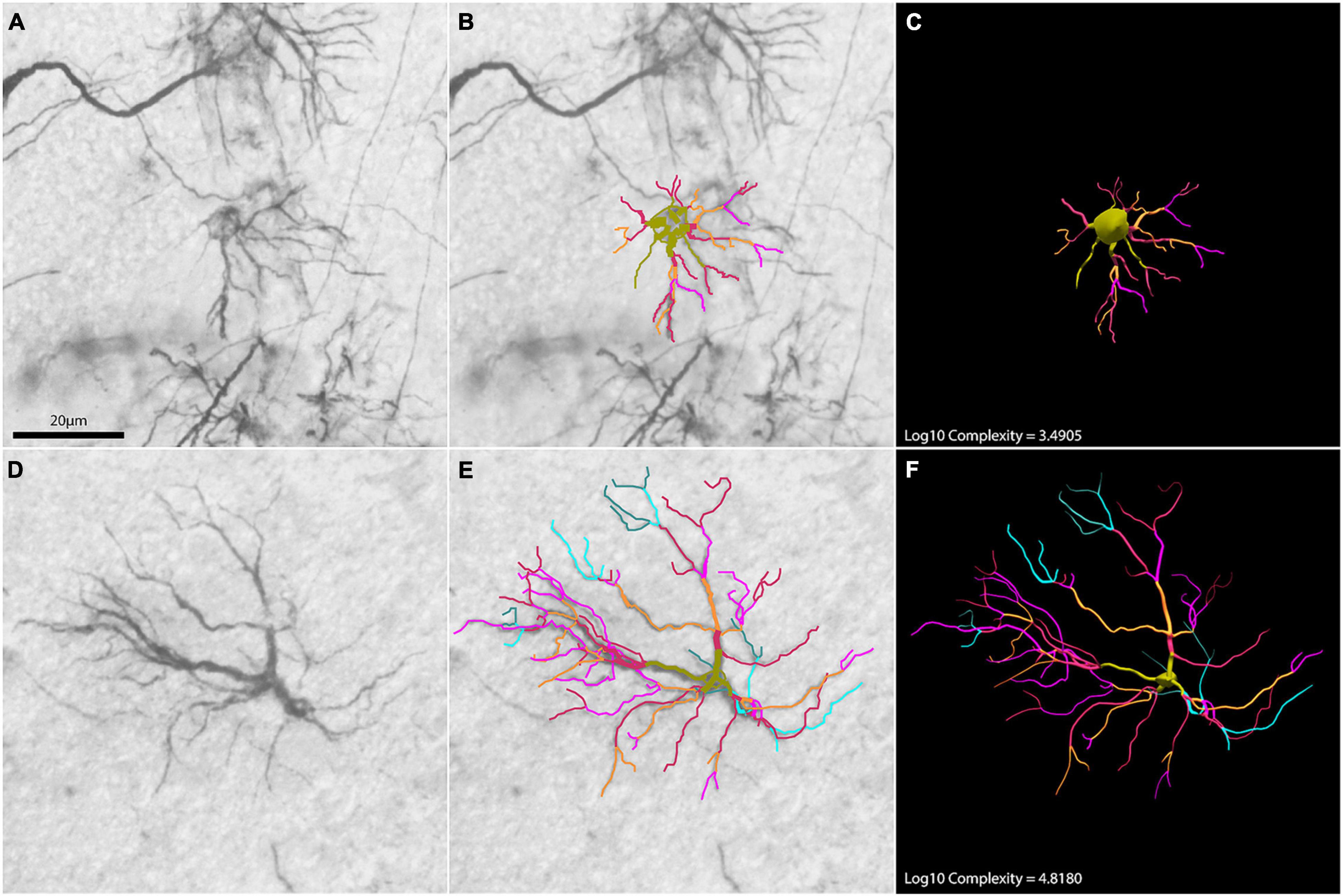
Figure 11. Two astrocyte photomicrographs (A,D) overlayed with correspondent branch reconstructions (B,E) are used to illustrate contrasting morphological complexity values. Panels (C,F) are correspondent 3D reconstructions of astrocytes illustrating low and high morphological complexities, respectively. Numbers indicate correspondent logarithmized values of morphological complexity.
Discussion
The systematic search for correlations between genes and behavioral phenotypes, looking at differential gene expression or the occurrence of allele polymorphisms associated with a specific phenotype, may be useful for understanding the mechanisms underlying the migration process and its molecular control (Bazzi et al., 2016b; Contina et al., 2018). Although the observed relationships between candidate genes CLOCK and ADCYAP1 and migratory behavior in birds appear to vary across species, previous studies revealed that both loci can be significantly correlated with a variety of distinct phenotypes in long distance migratory birds (Saino et al., 2015; Bazzi et al., 2016b). For example, in blackpoll warblers (Setophaga striata), a Neotropical-Nearctic migrant, minimum allele length was associated with later spring departure date for breeding grounds and earlier fall arrival date at wintering ground (Ralston et al., 2019).
The ADCYAP1 gene codes for PACAP which influences circadian rhythms directly by activating CLOCK and other genes in the circadian oscillator complex (Nagy and Csernus, 2007). PACAP also modulates a number of astrocyte activities such as proliferation, plasticity, glycogen production, and biosynthesis of neurotrophic factors and gliotransmitters (Masmoudi-Kouki et al., 2007). In previous studies we demonstrated that contrasting long-distance migratory flights of C. pusilla and C. semipalmatus differentially shape the morphological complexity of two morphotypes of hippocampal astrocytes (Henrique et al., 2020). Here we searched for associations between astrocyte morphological complexity, migratory distance, and size of the ADCYAP1 allele. Our findings showed significant differences in the size of the microsatellites of C. pusilla, C. semipalmatus, A. macularius, and C. collaris, in association with distinct mean values of astrocyte morphological complexity in the hippocampal formation of these species. These findings support previous suggestions for a role of ADCYAP1 in shaping the avian migratory phenotype (Bazzi et al., 2016b) and its relation with astroglial physiology. We propose that these associations may be part of the adaptive response to the migratory process.
The Increase in ADCYAP1 Microsatellite Repetitions Was Associated With Longer Distances Migration
Before and during migration, birds undergo significant changes in physiology and behavior that are adapted and motivated differently in spring and autumn: spring migration takes place in search of reproductive sites while autumn migration takes place in search of wintering sites with milder temperatures and greater food availability (Sharma et al., 2018a,b). As compared with autumn migration, buntings show greater body mass, higher levels of triglycerides and free fatty acids, accumulate more subcutaneous fat and liver lipids, and more intense Zugunruhe in spring migration (Samojłowicz et al., 2019). A relação entre o tamanho do microsatellite e diferentes funções fisiológicas foi previamente descrita (Ralston et al., 2019). In the present report all individuals were captured between August 15 and April 8, during the wintering period in the mangroves of Bragança (Brazil) in the Amazon River estuary, where they experienced temperatures between 20.4 and 32.8°C with the minimum values coincident with the increase in pluviometry mean values in March (Ribeiro, 2001).
In all comparisons of mean allele length between migrant species (A. macularius, C. pusilla, and C. semipalmatus), and the non-migratory species (C. collaris) we found significant differences, with the lower mean value for Euclidean distance found in the non-migratory species. In addition, the mean values of allele length in migratory birds increased significantly as a function of migratory distance.
Thus, based on earlier studies of ADCYAP1 variation (Mueller et al., 2011), our prediction that migratory species A. macularius, C. pusilla, and C. semipalmatus would possess longer microsatellite repeat-length alleles of ADCYAP1 compared with those of non-migratory C. collaris, was confirmed. In addition, we found proportional direct association between the size of microsatellite repeat-length alleles at the ADCYAP1 locus and autumnal migratory distances. These findings are in line with previous studies that demonstrated that ADCYAP1 polymorphism covaries with breeding latitude (Bazzi et al., 2016b).
The Morphological Complexity of Astrocytes Is Greater in Migrating Birds
Differences in migratory behavior affect not only the number of astrocytes in the hippocampal formation of migrating birds, but also their morphology (Henrique et al., 2020). Indeed, Henrique and collaborators compared the number and 3-D morphology of hippocampal astrocytes of C. semipalmatus before and after autumnal migration with those of C. pusilla to test the hypothesis that the contrasting migratory flights of these species could differentially shape hippocampal astrocyte number and morphology. C. pusilla migration to the southern hemisphere includes a 5-day non-stop flight over the Atlantic Ocean (Brown, 2014), whereas C. semipalmatus migration, to the same area, is largely over land with stopovers for feeding and rest. After hierarchical cluster analysis of astrocyte morphological features, two families of morphological phenotypes in C. pusilla and C. semipalmatus hippocampal formations named Type I and Type II were distinguished, which were differentially affected after autumnal migratory flights. Stereological counts of hippocampal astrocytes demonstrated that the number of astrocytes decreased significantly in C. pusilla but did not change in C. semipalmatus.
Thus, after hierarchical cluster analysis with non-normalized data, we previously found at the greater Euclidian distances two morphotypes of hippocampal astrocytes (Carvalho-Paulo et al., 2018; Henrique et al., 2020). Here, we performed hierarchical clustering on variance-shrunk logarithmized values of multimodal morphometrical features for reduction of the asymmetrical influence of non-standardized morphological features on cluster segregation. With logarithmized morphometric values, we compared the morphologies of astrocytes from the hippocampus of long-distance migratory birds with contrasting migratory flights (C. semipalmatus, C. pusilla, and A. macularius) with each other, and with a non-migratory species (C. collaris). Except for the hippocampal formation of A. macularius where we found four morphotypes, all other species showed three hippocampal astrocytes morphotypes. Thus, in this work, we expanded the studies of morphometry of astrocytes to C. collaris and A. macularius hippocampal formations indicating the occurrence of three main morphological types of astrocytes in C. pusilla, C. semipalmatus, and C. collaris and 4 morphotypes in A. macularius. Morphological complexity and convex hull volume were the variables that best distinguished the morphological families suggested by hierarchical cluster analysis. These morphological families of astrocytes were validated by comparing the mean values of morphological variables for type and species studied using PERMANOVA and canonical discriminant analysis. Type I was used to name the cell population with the highest average values of morphological complexity. Type II, Type III, and Type IV named astrocytes with decreased morphological complexity. We suggest that the differences found between migratory birds’ hippocampal astrocyte morphologies might be related to the adaptive response imposed by the contrasting long-distance migratory flights on learning and memory for recognition of olfactory, geomagnetic, and visual cues during migration or to recognize local cues for both migratory birds and non-migratory C. collaris (Mouritsen et al., 2016; Magalhaes et al., 2017; Carvalho-Paulo et al., 2018; da Costa et al., 2020; Henrique et al., 2020; Guerreiro et al., 2021).
Notably, a phylogenetically independent contrast (PIC) approach (Felsenstein, 1985) showed that most morphometric differences in astrocytes found in the different species were not influenced by phylogenetic differences (Henrique et al., 2020). The PIC approach used DNA sequences of intron 7 of beta-fibrinogen (fib 7) gene, recombination activating gene 1(RAG1), cytochrome oxidase c subunit 1 (COI) gene and cytochrome b (cyt b) gene obtained from GeneBank for C. pusilla, C. semipalmatus, A. macularius, and C. collaris (Henrique et al., 2020).
In the present report, however, we were expecting that A. macularius and C. semipalmatus, that rely more on remembering visual cues during overland migration than C. pusilla, which migrates via a long-distance non-stop flight over the Atlantic Ocean (Diniz et al., 2016) would show higher mean values of morphological complexity. We also hypothesized that C. collaris, a non-migrant species, as compared with the migrant species would show the lowest morphological complexity. We found that the non-migratory species C. collaris and C. semipalmatus showed similar mean values for type I (higher complexity) and III (lower complexity) and that type II (median complexity) mean values in C. collaris were greater than that of C. semipalmatus. Similar mean values were found for morphological complexity of type I and II in A. macularius and C. pusilla but these values were significantly higher than those of correspondently named astrocytes of C. collaris and C. semipalmatus. In addition, A. macularius showed greater type III mean values than those of C. pusilla correspondent morphotype.
From these findings, it is not possible to anticipate the mechanisms underneath the hippocampal astrocyte morphological differences between wintering migrating birds with contrasting migratory flights and non-migrating birds. However, in a previous study it clearly emerged that the glial morphologies of birds collected in August in the Bay of Fundy (Canada) and September to March on Isla Canela (Bragança, Brazil) are clearly different, both in C. pusilla and C. semipalmatus (Henrique et al., 2020). These findings were interpreted as due to contrasting migratory routes (non-stop transatlantic flight vs mostly overland migration). However, many other possibilities were pointed out as possible contributors to the morphological differences. For example, different capture dates (Carvalho-Paulo et al., 2018) with differential implications for hormones and receptors for stress and pre- and post-breeding conditions (Riou et al., 2010; Eikenaar et al., 2015; Deviche et al., 2016; Surbhi Rastogi et al., 2016). Here, we also raise the hypothesis that distinct morphotypes, may have differential physiological roles in different species and this may be at least part of the underlying hippocampal circuitry adaptive response for behavioral changes in those species (Hwang et al., 2021).
Migratory Behavior, ADCYAP1 Microsatellites and Hippocampal Astrocyte Morphology
Calidris pusilla, C. semipalmatus, and A. macularius migratory journeys between the northern breeding sites of United States and Canada and wintering grounds in the Amazon basin of northern South America are among the longest migratory routes of shorebirds. As previously indicated, significant association was observed between the size of the simple sequence repeats (SSR) and migration distance in these three migrant species. Consistent with this, differences in food intake and glucocorticoid effects during overland and transatlantic flights may differentially affect migrating and wintering birds’ metabolic pathways, with significant influences on astrocyte morphologies. For example, during the 5-day non-stop flight of C. pusilla a short supply of glucose and a high demand for lipids occurs, inducing the brain to increase ketone body metabolism to support the transoceanic flight (Achanta and Rae, 2017). This uninterrupted flight of C. pusilla compared with the multiple stopover flights of C. semipalmatus and A. macularius, may impose differential demand for PACAP, which is synthesized by ADCYAP1.
Because SSR in the regulatory region 3’UTR of ADCYAP1 may modify gene function and post-transcriptional processes (Riley and Krieger, 2009; Steinmeyer et al., 2009) it is reasonable to expect that any changes in SSR found in A. macularius, C. pusilla, and C. semipalmatus, may benefit migratory behavior of these species. In agreement with this expectation, it has been demonstrated that in the CNS, PACAP is involved in the rhythmicity of melatonin production and in the increase of cAMP in birds (Nakahara et al., 2002; Nowak and Zawilska, 2003), as well as acting as a co-transmitter with glutamate to shift the phase of the CNS circadian rhythm in a similar way to light (Michel et al., 2006; Vaudry et al., 2009). In addition, previous data show that PACAP plays important role in controlling astroglial functions by regulating cell proliferation and glycogen metabolism (Magistretti et al., 1998; Masmoudi-Kouki et al., 2007; Nakamachi et al., 2011).
Moreover, PACAP is affected by diet and fasting (Iwasa et al., 2016; Nakata et al., 2016) with important implications for regulation of food intake (Kataoka et al., 2013; Nguyen et al., 2020) and energy homeostasis (Chang et al., 2021) and this seems to include birds (Tachibana et al., 2015; Simon et al., 2017). PACAP is also affected by photoperiodic light changes (Saino et al., 2015; Bazzi et al., 2016a; Adamska et al., 2018; Haraguchi et al., 2019), reproductive conditions (Prisco et al., 2019; Winters and Moore, 2020) and stress (Agarwal et al., 2005; Norrholm et al., 2005; Hammack et al., 2010). Due to differential pre- and post-breeding physiological conditions, distinct metabolic demands and diets imposed by migration with multiple stopovers and fasting uninterrupted transoceanic flight, along with differential stress levels along the migratory journey of migrant species compared to the non-migrant C. collaris, it may be possible that at least part of the astrocyte morphological differences between species may be associated with interspecific differential expression of ADCYAP1. Indeed, PACAP mimicked effects of forskolin, a direct activator of adenylate cyclase, on the actin cytoskeleton of astrocytes with resultant astrocyte morphological changes (Perez et al., 2005). In addition, PACAP is essential for lactate production and secretion in astrocytes, a central step in the neuronal physiology of hippocampal learning and memory (Kambe et al., 2021) the center for integrative information for familiar landmarks and landscape features in homing pigeons (Bingman and Ewry, 2020; Gagliardo et al., 2021), and for avian long-distance migratory journeys (Frost and Mouritsen, 2006; Barkan et al., 2016; Bingman and MacDougall-Shackleton, 2017).
Migratory phenotypes and ADCYAP1 and CLOCK genes have been tested in other species including migratory distance, wing morphology and spring migration arrival of Sylvia atricapilla (Mueller et al., 2011; Mettler et al., 2015); migratory restlessness in the songbird genus Junco and S. atricapilla (Mueller et al., 2011; Peterson et al., 2013); time of C. pusilla migration (Bazzi et al., 2016b); and activation of CLOCK and other circadian genes in Gallus gallus (Nagy and Csernus, 2007).
In line with these findings, we found significant differences in all comparisons of allele mean length between migrant species (A. macularius, C. pusilla, and C. semipalmatus), and the non-migratory species (C. collaris), with the lower mean value in the non-migratory C. collaris. This may suggest that different migratory behaviors are associated with size differences of ADCYAP1 microsatellites, and this may be acting through PACAP to induce morphological and functional changes in the astrocytes of the hippocampal formation (Vaudry et al., 2009). It is reasonable to assume as well, that in C. collaris, ADCYAP1 microsatellites are not under positive selection for migratory behavior.
Methodological Limitations and Potential Sources of Non-biological Variation
Because we did not track individuals during the migratory flights we estimated the distance between breeding sites and the wintering places of capture (see section “Materials and Methods”). We followed previous descriptions of suggested trajectories between stop overs for each species (Williams and Williams, 1978; Brown, 2014; Anderson et al., 2019), as indicated in Figure 2. Because C. collaris is not a migrant species we arbitrarily attributed 0 km as distance traveled for this species and because this species is not sedentary, this is a limitation of the present report. Migrant birds also move during the wintering periods and their local movements were ignored as well. Because local displacements of all individuals during the wintering period may not be similar in different species, their potential influence on astrocytes morphologies could not be assessed, and this is limitation to be explored in future studies.
To measure possible influence of capture dates on hippocampal astrocyte morphological complexities of wintering birds, in a previous report dedicated to C. pusilla, we compared astrocyte morphologies of birds captured at different time points of the wintering period using the same statistical analysis (Carvalho-Paulo et al., 2018). We found smaller changes in the mean values of hippocampal astrocytes morphological complexity which did not significantly affect the results (Carvalho-Paulo et al., 2018). However, recent findings from morphological analysis of hippocampal astrocytes of Arenaria interpres, differed significantly in the morphological complexity of hippocampal astrocytes of autumnal recently arrived migrant birds (captured in September/October) and spring premigratory individuals (captured in April/May) suggesting that as wintering period progresses, significant changes in hippocampal circuitry occur (da Costa et al., 2020). Thus, it is reasonable to expect that astrocytes have their morphology changed during the wintering period.
Because there is no information in the literature about potential influence of sex and age on hippocampal astrocyte morphology in long-distance migratory birds, and we did not measure the age of individuals in our sample due to technical limitations, it is difficult to discuss these potential influences in detail. However, experience and sex are important variables that have been previously demonstrated to influence hippocampal-dependent tasks in birds (Astié et al., 2015; Rensel et al., 2015; Guigueno et al., 2016; Bingman and MacDougall-Shackleton, 2017), and migratory behavior is accompanied by hippocampal morphological changes including volume, and neurogenesis (Barkan et al., 2016, 2017; de Morais Magalhaes et al., 2017) which should be considered in future studies of hippocampal astrocyte morphologies in long-distance migratory birds.
Although the correlational analysis between the length of ADCYAP1 microsatellites, distance of migration, and differences in the morphologies of astrocytes of the hippocampal formation seems to be coherent, the story could be different if another higher order brain area, less involved in migration, exhibited similar astrocytes differences and this is a potential limitation of the present study, that could be avoided if another area was explored (Carvalho-Paulo et al., 2018; Henrique et al., 2020).
It is not uncommon to find contradictory results in comparative studies due to ambiguities in the definition of the objects and areas of interest, variations in histological procedures, or in the case of 3D reconstructions, dissimilarities in the sampling approach to select cells for reconstruction (West, 2002). In this report all samples were obtained with the same tissue processing protocols (perfusion, antigen retrieval, immunoreaction, dehydration, counterstaining, and clearing) and the specificity of the immunohistochemical pattern was confirmed using a control reaction that omitted the primary antibody (Saper and Sawchenko, 2003; Schmitt et al., 2004; Fritschy, 2008). To obtain sufficient contrast between foreground and background we improved the signal/noise ratio with glucose-oxidase-DAB-Nickel peroxidase amplification method (Shu et al., 1988).
It has been also demonstrated that the z-axis (perpendicular to the cutting surface), shrinks by approximately 75% of the cut thickness after dehydration and clearing (Carlo and Stevens, 2011). Based on those findings, all astrocytes’ reconstructions were corrected for z-axis shrinkage. No corrections were applied to X/Y axes and used the same software and hardware approaches for sampling, reconstruction, and analysis. These procedures guarantee similar, systematic and random sampling selection of astrocytes across all regions of the areas of interest. Finally, to detect possible variations in the criteria for identifying and including only complete astrocyte arbors inside the area of interest, we undertook checking procedures of the results by having different investigators reconstruct astrocytes in the same regions using the same GFAP antibody as a marker for selective labeling. Thus, we expected to reduce possible sources of non-biological variation.
Conclusion
The largest microsatellite repeat-length alleles, the highest mean value of astrocyte morphological complexity and the longest migratory distance were found in A. macularius, followed by intermediate values in C. pusilla and C. semipalmatus, while the smallest microsatellite repeat-length alleles and the smallest morphological complexity mean values were found in the resident non-migratory C. collaris. Taking these findings together, we suggest that polymorphism in the gene ADCYAP1 may underlie variation in the migratory phenotype and both are strongly related to migratory distances.
Data Availability Statement
The original contributions presented in the study are included in the article/Supplementary Material, further inquiries can be directed to the corresponding author. Tabulated data used for microsatellite sizes and astrocyte morphological parameters are available from: https://github.com/patrick-douglas/Miranda_et_al_2021.
Ethics Statement
The animal study was reviewed and approved under license N° 44551-2 from the Chico Mendes Institute for conservation of Biodiversity (ICMBio) and Scientific Capture permit ST2783 from the Canadian Wildlife Service. All procedures were carried out in accordance with the Association for the Study of Animal Behavior / Animal Behavior Society Guidelines for the Use of Animals in Research and with approval of the Animal Users Subcommittee of the University of Western Ontario. All efforts were made to minimize the number of animals used, stress and discomfort.
Author Contributions
All authors contributed substantially to the conception or design of the work, acquisition, analysis, or interpretation of data for the work, drafting the work or revising it critically for important intellectual content, and/or final approval of the version to be published, and agreed to be accountable for all aspects of the work in ensuring that questions related to the accuracy or integrity of any part of the work are appropriately investigated and resolved.
Funding
This research was supported by: Coordenação de Aperfeiçoamento de Pessoal de Nível Superior (CAPES), Programa Ciências do Mar II; The Canadian Bureau for International Education (CBIE); The Brazilian Research Council (CNPq), Edital Universal Grant Number 440722/2014-4; Fundação Amazônia Paraense de Amparo à Pesquisa (FAPESPA), Programa de Apoio a Núcleos Emergentes, Convênio 03/2017, Centro de Pesquisa e Aplicação em Piscicultura da Amazônia Brasileira – CPAM; Financiadora de Estudos e Projetos (FINEP-PROINFRA 2012), Instituto Brasileiro de Neurociências (IBNnet); Pró-Reitoria de Pesquisa e Pós-Graduação da Universidade Federal do Pará (Edital PAPq, 2021); and the Natural Sciences and Engineering Research Council of Canada (NSERC).
Conflict of Interest
The authors declare that the research was conducted in the absence of any commercial or financial relationships that could be construed as a potential conflict of interest.
Publisher’s Note
All claims expressed in this article are solely those of the authors and do not necessarily represent those of their affiliated organizations, or those of the publisher, the editors and the reviewers. Any product that may be evaluated in this article, or claim that may be made by its manufacturer, is not guaranteed or endorsed by the publisher.
Supplementary Material
The Supplementary Material for this article can be found online at: https://www.frontiersin.org/articles/10.3389/fpsyg.2021.784372/full#supplementary-material
Abbreviations
3-D, three dimensional reconstructions; ABC, avidin–biotin–peroxidase complex; ADCYAP1, adenylate cyclase activating polypeptide 1; ANOVA, analysis of variance; CE, coefficient of error; CNS, central nervous system; CV, coefficient of variation; CVB, coefficient of biological variation; DAB, diaminobenzidine; DNA, deoxyribonucleic acid; FH, hippocampal formation; GFAP, glial fibrillary acid protein; GLM, general linear model; ICMBio, Chico Mendes Institute for Biodiversity Conservation; M3, asymmetry; M4 = kurtosis; MMI, multimodality index; PACAP, pituitary adenylate cyclase-activating polypeptide; PBS, phosphate-buffered saline; PBST, Triton ™ phosphate-buffered saline; PCO, principal coordinate analysis; PCR, polymerase chain reaction; PERMANOVA, analysis of variance by multivariate permutation; PERMIDISP, dispersion homogeneity tests; SE, standard error; UTR, untranslated region.
References
Able, K. P. (1991). The development of migratory orientation mechanisms. EXS 60, 166–179. doi: 10.1007/978-3-0348-7208-9_8
Achanta, L. B., and Rae, C. D. (2017). β-hydroxybutyrate in the brain: one molecule. Multiple Mechanisms. Neurochem. Res. 42, 35–49. doi: 10.1007/s11064-016-2099-2
Adamska, I., Malz, M., Lewczuk, B., Blügental, N., Markowska, M. A., Meronka, R., et al. (2018). Daily profiles of neuropeptides, catecholamines, and neurotransmitter receptors in the chicken pineal gland. Front. Physiol. 9:1972. doi: 10.3389/fphys.2018.01972
Agarwal, A., Halvorson, L. M., and Legradi, G. (2005). Pituitary adenylate cyclase-activating polypeptide (PACAP) mimics neuroendocrine and behavioral manifestations of stress: Evidence for PKA-mediated expression of the corticotropin-releasing hormone (CRH) gene. Brain Res. Mol. Brain Res. 138, 45–57. doi: 10.1016/j.molbrainres.2005.03.016
Åkesson, S., Ilieva, M., Karagicheva, J., Rakhimberdiev, E., Tomotani, B., and Helm, B. (2017). Timing avian long-distance migration: from internal clock mechanisms to global flights. Philos. Trans R Soc. Lond. B Biol. Sci. 372:20160252. doi: 10.1098/rstb.2016.0252
Anderson, A., Duijins, S., Smith, P. A., Friis, C., and Nol, E. (2019). Migration distance and body condition influence shorebird migration sttrategies and stopover decisions during southband migration. Front. Ecol. Evol. 7:251.
Anderson, M., Ellingsen, K., and McArdle, B. (2006). Multivariate dispersion as a measure of beta diversity. Ecol. Lett. 9, 683–693. doi: 10.1111/j.1461-0248.2006.00926.x
Anderson, M., Gorley, R., and Clarke, K. (2008). For PRIMER: Guide to Software and Statistical Methods. Plymouth: Prim.Plymouth.
Astié, A. A., Scardamaglia, R. C., Muzio, R. N., and Reboreda, J. C. (2015). Sex differences in retention after a visual or a spatial discrimination learning task in brood parasitic shiny cowbirds. Behav. Proc. 119, 99–104. doi: 10.1016/j.beproc.2015.07.016
Atoji, Y., and Wild, J. M. (2004). Fiber connections of the hippocampal formation and septum and subdivisions of the hippocampal formation in the pigeon as revealed by tract tracing and kainic acid lesions. J. Comp. Neurol. 475, 426–461. doi: 10.1002/cne.20186
Atoji, Y., Sarkar, S., and Wild, J. M. (2016). Proposed homology of the dorsomedial subdivision and V-shaped layer of the avian hippocampus to Ammon’s horn and dentate gyrus, respectively. Hippocampus 26, 1608–1617. doi: 10.1002/hipo.22660
Bakalar, D., Sweat, S., Drossel, G., Jiang, S. Z., Samal, B. B., Stroth, N., et al. (2021). Relationships between constitutive and acute gene regulation, and physiological and behavioral responses, mediated by the neuropeptide PACAP. Psychoneuroendocrinology 135:105447.
Baliga, R. S., Macallister, R. J., and Hobbs, A. J. (2013). Vasoactive peptides and the pathogenesis of pulmonary hypertension: role and potential therapeutic application. Handb. Exp. Pharmacol. 218, 477–511. doi: 10.1007/978-3-642-38664-0_19
Balloux, F., and Lugon-Moulin, N. (2002). The estimation of population differentiation with microsatellite markers. Mol. Ecol. 11, 155–165. doi: 10.1046/j.0962-1083.2001.01436.x
Barkan, S., Roll, U., Yom-Tov, Y., Wassenaar, L. I., and Barnea, A. (2016). Possible linkage between neuronal recruitment and flight distance in migratory birds. Sci. Rep. 6:21983. doi: 10.1038/srep21983
Barkan, S., Yom-Tov, Y., and Barnea, A. (2017). Exploring the relationship between brain plasticity, migratory lifestyle, and social structure in birds. Front. Neurosci. 11:139. doi: 10.3389/fnins.2017.00139
Barros, J., Winkler, F. M., and Velasco, L. A. (2020). Assessing the genetic diversity in. Ecol. Evol. 10, 3919–3931.
Bazzi, G., Galimberti, A., Hays, Q. R., Bruni, I., Cecere, J. G., Gianfranceschi, L., et al. (2016b). Adcyap1 polymorphism covaries with breeding latitude in a nearctic migratory songbird, the Wilson’s warbler (Cardellina pusilla). Ecol. Evol. 6, 3226–3239. doi: 10.1002/ece3.2053
Bazzi, G., Cecere, J. G., Caprioli, M., Gatti, E., Gianfranceschi, L., Podofillini, S., et al. (2016a). Clock gene polymorphism, migratory behaviour and geographic distribution: a comparative study of trans-saharan migratory birds. Mol. Ecol. 25, 6077–6091. doi: 10.1111/mec.13913
Billerman, S., Bk, K., Pg, P., and Ts, S. (eds) (2020). Birds of the World. Ithaca, NY: Cornell Laboratory of Ornithology.
Bingman, V. P., and Ewry, E. M. (2020). On a search for a neurogenomics of cognitive processes supporting avian migration and navigation. Integr. Comp. Biol. 60, 967–975. doi: 10.1093/icb/icaa040
Bingman, V. P., and MacDougall-Shackleton, S. A. (2017). The avian hippocampus and the hypothetical maps used by navigating migratory birds (with some reflection on compasses and migratory restlessness). J. Comp. Physiol. A Neuroethol. Sens. Neural. Behav. Physiol. 203, 465–474. doi: 10.1007/s00359-017-1161-0
Bornstein, J. C., Costa, M., and Grider, J. R. (2004). Enteric motor and interneuronal circuits controlling motility. Neurogastroenterol Motil 16(Suppl. 1), 34–38. doi: 10.1111/j.1743-3150.2004.00472.x
Boutin, P., Hani, E. H., Vasseur, F., Roche, C., Bailleul, B., Hager, J., et al. (1997). Automated fluorescence-based screening for mutation by SSCP: use of universal M13 dye primers for labeling and detection. Biotechniques 23, 358–362. doi: 10.2144/97233bm01
Boutin-Ganache, I., Raposo, M., Raymond, M., and Deschepper, C. F. (2001). M13-tailed primers improve the readability and usability of microsatellite analyses performed with two different allele-sizing methods. Biotechniques 31:28.
Bozadjieva-Kramer, N., Ross, R. A., Johnson, D. Q., Fenselau, H., Haggerty, D. L., Atwood, B., et al. (2021). The role of mediobasal hypothalamic PACAP in the control of body weight and metabolism. Endocrinology 162:bqab012. doi: 10.1210/endocr/bqab012
Brown, S. (2014). The Remarkable Odyssey of a Semipalmated Sandpiper. In Shorebird Science. Canada: Manomet Soaring Solutions Grounded Science.
Campos, C., Naiff, R., and Araujo, A. (2008). Censo de Aves Migratórias (Charadriidae e Scolopacidae) da Porção Norte da Bacia Amazônica, Macapá, Amapá, Brasil. Ornithologia 3, 38–46.
Carlo, C. N., and Stevens, C. F. (2011). Analysis of differential shrinkage in frozen brain sections and its implications for the use of guard zones in stereology. J. Comp. Neurol. 519, 2803–2810. doi: 10.1002/cne.22652
Carvalho-Paulo, D., de Morais Magalhães, N. G., de Almeida Miranda, D., Diniz, D. G., Henrique, E. P. I, Moraes, A. M., et al. (2017). Hippocampal astrocytes in migrating and wintering semipalmated sandpiper. Front. Neuroanat 11:126.
Carvalho-Paulo, D., Magalhaes, N. G. D., Miranda, D. D., Diniz, D. G., Henrique, E. P. I, Moraes, A. M., et al. (2018). Hippocampal astrocytes in migrating and wintering semipalmated sandpiper calidris pusilla. Front. Neuroanatomy 11:126. doi: 10.3389/fnana.2017.00126
Chang, R., Hernandez, J., Gastelum, C., Guadagno, K., Perez, L., and Wagner, E. J. (2021). Pituitary adenylate cyclase-activating polypeptide excites proopiomelanocortin neurons: implications for the regulation of energy homeostasis. Neuroendocrinology 111, 45–69. doi: 10.1159/000506367
Ciranna, L., and Costa, L. (2019). Pituitary adenylate cyclase-activating polypeptide modulates hippocampal synaptic transmission and plasticity: new therapeutic suggestions for fragile X syndrome. Front. Cell Neurosci. 13:524. doi: 10.3389/fncel.2019.00524
Clarke, K., and Warwick, R. (2001). Change in Marine Communities: An Approach to Statistical Analysis and Interpretation. 2nd Edn. Plymouth: PRIMER-E, 172.
Contina, A., Bridge, E. S., Ross, J. D., Shipley, J. R., and Kelly, J. F. (2018). Examination of clock and adcyap1 gene variation in a neotropical migratory passerine. PLoS One 13:e0190859. doi: 10.1371/journal.pone.0190859
da Costa, E. R., Henrique, E. P., da Silva, J. B., Pereira, P. D. C., de Abreu, C. C., Fernandes, T. N., et al. (2020). Changes in hippocampal astrocyte morphology of ruddy turnstone (arenaria interpres) during the wintering period at the mangroves of Amazon river estuary. J. Chem. Neuroanat 108:101805. doi: 10.1016/j.jchemneu.2020.101805
de Morais Magalhaes, N. G., Guerreiro Diniz, C., Guerreiro Diniz, D., Pereira Henrique, E., Correa Pereira, P. D., Matos Moraes, I. A., et al. (2017). Hippocampal neurogenesis and volume in migrating and wintering semipalmated sandpipers (Calidris pusilla). PLoS One 12:e0179134. doi: 10.1371/journal.pone.0179134
Del Hoyo, J., Elliot, A., and Sargatal, J. (1992). Handbook of the Birds of the World. Barcelona: Lynx Editions.
Dennis, T. E., Rayner, M. J., and Walker, M. M. (2007). Evidence that pigeons orient to geomagnetic intensity during homing. Proc. Biol. Sci. 274, 1153–1158. doi: 10.1098/rspb.2007.3768
Deviche, P., Valle, S., Gao, S., Davies, S., Bittner, S., and Carpentier, E. (2016). The seasonal glucocorticoid response of male rufous-winged sparrows to acute stress correlates with changes in plasma uric acid, but neither glucose nor testosterone. Gen Comp. Endocrinol. 235, 78–88. doi: 10.1016/j.ygcen.2016.06.011
Diniz, C. G., Magalhães, N. G., Sousa, A. A., Santos Filho, C., Diniz, D. G., Lima, C. M., et al. (2016). Microglia and neurons in the hippocampus of migratory sandpipers. Braz J. Med. Biol. Res. 49:e5005. doi: 10.1590/1414-431X20155005
Eikenaar, C., Müller, F., Klinner, T., and Bairlein, F. (2015). Baseline corticosterone levels are higher in migrating than sedentary common blackbirds in autumn, but not in spring. Gen Comp. Endocrinol. 224, 121–125. doi: 10.1016/j.ygcen.2015.07.003
Ellegren, H. (2004). Microsatellites: simple sequences with complex evolution. Nat. Rev. Genet. 5, 435–445. doi: 10.1038/nrg1348
Emlen, S. (1975). The stellar-orientation system of a migratory bird. Sci. Am. 233, 102–111. doi: 10.1038/scientificamerican0875-102
Excoffier, L., Laval, G., and Schneider, S. (2007). Arlequin (version 3.0): an integrated software package for population genetics data analysis. Evol. Bioinform 1, 47–50.
Felsenstein, J. (1985). Phylogenies and the comparative method. Am. Nat. 125, 1–15. doi: 10.1086/284325
Fritschy, J. M. (2008). Is my antibody-staining specific? How to deal with pitfalls of immunohistochemistry. Eur. J. Neurosci. 28, 2365–2370. doi: 10.1111/j.1460-9568.2008.06552.x
Frost, B. J., and Mouritsen, H. (2006). The neural mechanisms of long distance animal navigation. Curr. Opin. Neurobiol. 16, 481–488. doi: 10.1016/j.conb.2006.06.005
Gagliardo, A., Bried, J., Lambardi, P., Luschi, P., Wikelski, M., and Bonadonna, F. (2013). Oceanic navigation in Cory’s shearwaters: evidence for a crucial role of olfactory cues for homing after displacement. J. Exp. Biol. 216, 2798–2805. doi: 10.1242/jeb.085738
Gagliardo, A., Colombo, S., Pollonara, E., Casini, G., Rossino, M. G., Wikelski, M., et al. (2021). GPS-profiling of retrograde navigational impairments associated with hippocampal lesion in homing pigeons. Behav. Brain Res. 412:113408. doi: 10.1016/j.bbr.2021.113408
Gahete, M. D., Durán-Prado, M., Luque, R. M., Martínez-Fuentes, A. J., Quintero, A., Gutiérrez-Pascual, E., et al. (2009). Understanding the multifactorial control of growth hormone release by somatotropes: lessons from comparative endocrinology. Ann. N.Y. Acad. Sci. 1163, 137–153. doi: 10.1111/j.1749-6632.2008.03660.x
Ganea, D., and Delgado, M. (2002). Vasoactive intestinal peptide (VIP) and pituitary adenylate cyclase-activating polypeptide (PACAP) as modulators of both innate and adaptive immunity. Crit. Rev. Oral. Biol. Med. 13, 229–237. doi: 10.1177/154411130201300303
Gastelum, C., Perez, L., Hernandez, J., Le, N., Vahrson, I., Sayers, S., et al. (2021). Adaptive changes in the central control of energy homeostasis occur in response to variations in energy status. Int. J. Mol. Sci. 22:2728. doi: 10.3390/ijms22052728
Gilmartin, M. R., and Ferrara, N. C. (2021). Pituitary adenylate cyclase-activating polypeptide in learning and memory. Front. Cell Neurosci. 15:663418.
Guerreiro, L. C. F., Henrique, E. P., da Silva Rosa, J. B., Pereira, P. D. C., de Abreu, C. C., Fernandes, T. N., et al. (2021). Plasticity in the hippocampal formation of shorebirds during the wintering period: stereological analysis of parvalbumin neurons in actitis macularius. Learn. Behav. [Epub ahead of print], doi: 10.3758/s13420-021-00473-6
Guigueno, M. F., MacDougall-Shackleton, S. A., and Sherry, D. F. (2016). Sex and seasonal differences in hippocampal volume and neurogenesis in brood-parasitic brown-headed cowbirds (molothrus ater). Dev. Neurobiol. 76, 1275–1290. doi: 10.1002/dneu.22421
Hammack, S. E., Roman, C. W., Lezak, K. R., Kocho-Shellenberg, M., Grimmig, B., Falls, W. A., et al. (2010). Roles for pituitary adenylate cyclase-activating peptide (PACAP) expression and signaling in the bed nucleus of the stria terminalis (BNST) in mediating the behavioral consequences of chronic stress. J. Mol. Neurosci. 42, 327–340. doi: 10.1007/s12031-010-9364-7
Hansson, E., Westerlund, A., Björklund, U., and Rönnbäck, L. (2009). PACAP attenuates 5-HT, histamine, and ATP-evoked Ca2+ transients in astrocytes. Neuroreport 20, 957–962. doi: 10.1097/WNR.0b013e32832ca201
Haraguchi, S., Kamata, M., Tokita, T., Tashiro, K. I., Sato, M., Nozaki, M., et al. (2019). Light-at-night exposure affects brain development through pineal allopregnanolone-dependent mechanisms. Elife 8:e45306. doi: 10.7554/eLife.45306
Hardy, O. J., Charbonnel, N., Fréville, H., and Heuertz, M. (2003). Microsatellite allele sizes: a simple test to assess their significance on genetic differentiation. Genetics 163, 1467–1482. doi: 10.1093/genetics/163.4.1467
Henrique, E. P., de Oliveira, M. A., Paulo, D. C., Pereira, P. D. C., Dias, C., de Siqueira, L. S., et al. (2020). Contrasting migratory journeys and changes in hippocampal astrocyte morphology in shorebirds. Eur. J. Neurosci. 54, 5687–5704. doi: 10.1111/ejn.14781
Hicklin, P. Gratto-Trevor, C. L. (2020). “Semipalmated sandpiper (Calidris pusilla) version 1.0,” in Birds of the World, ed. A. F. Poole (Ithaca, NY: Cornell Lab of Ornithology). doi: 10.2173/bow.semsan.01
Hwang, S. N., Lee, J. S., Seo, K., and Lee, H. (2021). Astrocytic regulation of neural circuits underlying behaviors. Cells 10:296. doi: 10.3390/cells10020296
Iwasa, T., Matsuzaki, T., Tungalagsuvd, A., Munkhzaya, M., Yiliyasi, M., Kato, T., et al. (2016). Developmental changes in the hypothalamic mRNA expression levels of PACAP and its receptor PAC1 and their sensitivity to fasting in male and female rats. Int. J. Dev. Neurosci. 52, 33–37. doi: 10.1016/j.ijdevneu.2016.05.003
Johnson, G. C., Parsons, R., May, V., and Hammack, S. E. (2020). The role of pituitary adenylate cyclase-activating polypeptide (PACAP) signaling in the hippocampal dentate gyrus. Front. Cell Neurosci. 14:111. doi: 10.3389/fncel.2020.00111
Kambe, Y., Yamauchi, Y., Thanh Nguyen, T., Thi Nguyen, T., Ago, Y., Shintani, N., et al. (2021). The pivotal role of pituitary adenylate cyclase-activating polypeptide for lactate production and secretion in astrocytes during fear memory. Pharmacol. Rep. 73, 1109–1121. doi: 10.1007/s43440-021-00222-6
Karpiesiuk, A., and Palus, K. (2021). Pituitary adenylate cyclase-activating polypeptide (PACAP) in physiological and pathological processes within the gastrointestinal tract: a review. Int. J. Mol. Sci. 22:8682. doi: 10.3390/ijms22168682
Kataoka, S., Takuma, K., Hara, Y., Maeda, Y., Ago, Y., and Matsuda, T. (2013). Autism-like behaviours with transient histone hyperacetylation in mice treated prenatally with valproic acid. Int. J. Neuropsychopharmacol. 16, 91–103. doi: 10.1017/S1461145711001714
Kinhult, J., Andersson, J. A., Uddman, R., Stjärne, P., and Cardell, L. O. (2000). Pituitary adenylate cyclase-activating peptide 38 a potent endogenously produced dilator of human airways. Eur. Respir J. 15, 243–247. doi: 10.1034/j.1399-3003.2000.15b04.x
Kirry, A. J., Herbst, M. R., Poirier, S. E., Maskeri, M. M., Rothwell, A. C., Twining, R. C., et al. (2018). Pituitary adenylate cyclase-activating polypeptide (PACAP) signaling in the prefrontal cortex modulates cued fear learning, but not spatial working memory, in female rats. Neuropharmacology 133, 145–154. doi: 10.1016/j.neuropharm.2018.01.010
Kong, L., Albano, R., Madayag, A., Raddatz, N., Mantsch, J. R., Choi, S., et al. (2016). Pituitary adenylate cyclase-activating polypeptide orchestrates neuronal regulation of the astrocytic glutamate-releasing mechanism system xc (.). J. Neurochem. 137, 384–393. doi: 10.1111/jnc.13566
Levene, H. (1960). Contributionsto Probability and Statistics. Redwood City, CA: Stanford University Press, 278–292.
Lindén, A., Cardell, L. O., Yoshihara, S., and Nadel, J. A. (1999). Bronchodilation by pituitary adenylate cyclase-activating peptide and related peptides. Eur. Respir J. 14, 443–451. doi: 10.1034/j.1399-3003.1999.14b34.x
Magalhaes, N. G. D., Diniz, C. G., Diniz, D. G., Henrique, E. P., Pereira, P. D. C. I, Moraes, A. M., et al. (2017). Hippocampal neurogenesis and volume in migrating and wintering semipalmated sandpipers (Calidris pusilla). PLos One 12:e0179134. doi: 10.1371/journal.pone.0179134
Magistretti, P. J., Cardinaux, J. R., and Martin, J. L. (1998). VIP and PACAP in the CNS: regulators of glial energy metabolism and modulators of glutamatergic signaling. Ann. N.Y. Acad. Sci. 865, 213–225. doi: 10.1111/j.1749-6632.1998.tb11181.x
Mann, R. P., Armstrong, C., Meade, J., Freeman, R., Biro, D., and Guilford, T. (2014). Landscape complexity influences route-memory formation in navigating pigeons. Biol. Lett. 10:20130885. doi: 10.1098/rsbl.2013.0885
Masmoudi-Kouki, O., Gandolfo, P., Castel, H., Leprince, J., Fournier, A., Dejda, A., et al. (2007). Role of PACAP and VIP in astroglial functions. Peptides 28, 1753–1760. doi: 10.1016/j.peptides.2007.05.015
Mayo, O. (2008). A century of hardy-weinberg equilibrium. Twin Res. Hum. Genet. 11, 249–256. doi: 10.1375/twin.11.3.249
McArdle, B., and Anderson, M. (2001). Fitting multivariate models to community data: a comment on distance−based redundancy analysis. Ecology 82, 290–297. doi: 10.1890/0012-9658(2001)082[0290:fmmtcd]2.0.co;2
Meirmans, P. G. (2020). genodive version 3.0: easy-to-use software for the analysis of genetic data of diploids and polyploids. Mol. Ecol. Resour. 20, 1126–1131. doi: 10.1111/1755-0998.13145
Meirmans, P. G., Liu, S., and van Tienderen, P. H. (2018). The analysis of polyploid genetic data. J. Hered 109, 283–296. doi: 10.1093/jhered/esy006
Mendes de Lima, C. P., Douglas Corrêa Pereira, P., Pereira Henrique, E., Augusto de Oliveira, M., Carvalho Paulo, D., and Silva de Siqueira, L. (2019). Differential change in hippocampal radial astrocytes and neurogenesis in shorebirds with contrasting migratory routes. Front. Neuroanat 13:82. doi: 10.3389/fnana.2019.00082
Mettler, R., Segelbacher, G., and Schaefer, H. M. (2015). Interactions between a candidate gene for migration (ADCYAP1), morphology and sex predict spring arrival in blackcap populations. PLoS One 10:e0144587. doi: 10.1371/journal.pone.0144587
Michel, S., Itri, J., Han, J. H., Gniotczynski, K., and Colwell, C. S. (2006). Regulation of glutamatergic signalling by PACAP in the mammalian suprachiasmatic nucleus. BMC Neurosci. 7:15. doi: 10.1186/1471-2202-7-15
Montero, M., Yon, L., Kikuyama, S., Dufour, S., and Vaudry, H. (2000). Molecular evolution of the growth hormone-releasing hormone/pituitary adenylate cyclase-activating polypeptide gene family. functional implication in the regulation of growth hormone secretion. J. Mol. Endocrinol. 25, 157–168. doi: 10.1677/jme.0.0250157
Moura, R. F., Dawson, D. A., and Nogueira, D. M. (2017). The use of microsatellite markers in neotropical studies of wild birds: a literature review. An. Acad. Bras Cienc 89, 145–154. doi: 10.1590/0001-3765201620160378
Mouritsen, H., Heyers, D., and Güntürkün, O. (2016). The neural basis of long-distance navigation in birds. Annu Rev. Physiol. 78, 133–154. doi: 10.1146/annurev-physiol-021115-105054
Mueller, J. C., Pulido, F., and Kempenaers, B. (2011). Identification of a gene associated with avian migratory behaviour. Proc. Biol. Sci. 278, 2848–2856. doi: 10.1098/rspb.2010.2567
Murat, C. B., and García-Cáceres, C. (2021). Astrocyte gliotransmission in the regulation of systemic metabolism. Metabolites 11:732. doi: 10.3390/metabo11110732
Nagy, A. D., and Csernus, V. J. (2007). Cry1 expression in the chicken pineal gland: effects of changes in the light/dark conditions. Gen Comp. Endocrinol. 152, 144–147. doi: 10.1016/j.ygcen.2007.01.019
Nakahara, K., Abe, Y., Murakami, T., Shiota, K., and Murakami, N. (2002). Pituitary adenylate cyclase-activating polypeptide (PACAP) is involved in melatonin release via the specific receptor PACAP-r1, but not in the circadian oscillator, in chick pineal cells. Brain Res. 939, 19–25. doi: 10.1016/s0006-8993(02)02538-6
Nakamachi, T., Farkas, J., Watanabe, J., Ohtaki, H., Dohi, K., Arata, S., et al. (2011). Role of PACAP in neural stem/progenitor cell and astrocyte–from neural development to neural repair. Curr. Pharm Des. 17, 973–984. doi: 10.2174/138161211795589346
Nakata, M., Zhang, B., Yang, Y., Okada, T., Shintani, N., Hashimoto, H., et al. (2016). High-fat diet augments VPAC1 receptor-mediated PACAP action on the liver, inducing LAR expression and insulin resistance. J. Diabetes Res. 2016:9321395. doi: 10.1155/2016/9321395
Nei, M. (1978). Estimation of average heterozygosity and genetic distance from a small number of individuals. Genetics 89, 583–590. doi: 10.1093/genetics/89.3.583
Nguyen, T. T., Kambe, Y., Kurihara, T., Nakamachi, T., Shintani, N., Hashimoto, H., et al. (2020). Pituitary adenylate cyclase-activating polypeptide in the ventromedial hypothalamus is responsible for food intake behavior by modulating the expression of agouti-related peptide in mice. Mol. Neurobiol. 57, 2101–2114. doi: 10.1007/s12035-019-01864-7
Nóbrega, P. F., Aguiar, J. A., and Figueira, J. E. (2015). First records of charadrius semipalmatus, bonaparte 1825 (charadriidae) and gelochelidon nilotica gmelin 1789 (sternidae) in the state of minas gerais, brazil. Braz J. Biol. 75, 451–454. doi: 10.1590/1519-6984.17013
Norrholm, S. D., Das, M., and Légrádi, G. (2005). Behavioral effects of local microinfusion of pituitary adenylate cyclase activating polypeptide (PACAP) into the paraventricular nucleus of the hypothalamus (PVN). Regul Pept. 128, 33–41. doi: 10.1016/j.regpep.2004.12.023
Nowak, J. Z., and Zawilska, J. B. (2003). PACAP in avians: origin, occurrence, and receptors–pharmacological and functional considerations. Curr. Pharm Des. 9, 467–481. doi: 10.2174/1381612033391586
Olkowski, A. A., and Classen, H. L. (1998). Safety of isoflurane anaesthesia in high risk avian patients. Vet. Rec. 143, 82–83. doi: 10.1136/vr.143.3.82
Perez, V., Bouschet, T., Fernandez, C., Bockaert, J., and Journot, L. (2005). Dynamic reorganization of the astrocyte actin cytoskeleton elicited by cAMP and PACAP: a role for phosphatidylInositol 3-kinase inhibition. Eur. J. Neurosci. 21, 26–32. doi: 10.1111/j.1460-9568.2004.03845.x
Peterson, M. P., Abolins-Abols, M., Atwell, J. W., Rice, R. J., Milá, B., and Ketterson, E. D. (2013). Variation in candidate genes CLOCK and ADCYAP1 does not consistently predict differences in migratory behavior in the songbird genus junco. F1000Res 2:115. doi: 10.12688/f1000research.2-115.v1
Piersma, T., and Wiersma, P. (1996). “Order charadriiformes. Family charadriidae (Plovers),” in Handbook of the Birds of the World, Vol. 3, eds J. del Hoyo, A. Elliot and J. Sargatal (Spain: Hoatzin to Auks. Lynx Edicions, Barcelona), 384–443.
Pillai, A. G., de Jong, D., Kanatsou, S., Krugers, H., Knapman, A., Heinzmann, J. M., et al. (2012). Dendritic morphology of hippocampal and amygdalar neurons in adolescent mice is resilient to genetic differences in stress reactivity. PLoS One 7:e38971. doi: 10.1371/journal.pone.0038971
Primmer, C. R., Møller, A. P., and Ellegren, H. (1996). A wide-range survey of cross-species microsatellite amplification in birds. Mol. Ecol. 5, 365–378. doi: 10.1111/j.1365-294x.1996.tb00327.x
Prisco, M., Rosati, L., Agnese, M., Aceto, S., Andreuccetti, P., and Valiante, S. (2019). Pituitary adenylate cyclase-activating polypeptide in the testis of the quail coturnix coturnix: expression, localization, and phylogenetic analysis. Evol. Dev. 21, 145–156. doi: 10.1111/ede.12285
Ralston, J., Lorenc, L., Montes, M., DeLuca, W. V., Kirchman, J. J., Woodworth, B. K., et al. (2019). Length polymorphisms at two candidate genes explain variation of migratory behaviors in blackpoll warblers. Ecol. Evol. 9, 8840–8855. doi: 10.1002/ece3.5436
Reed, J. M., and Oring, L. W. (1993). Philopatry, site fidelity, dispersal, and survival of spotted sandpipers. AUK 110, 541–551. doi: 10.2307/4088418
Reed, J., Oring, L., and Gray, E. (2013). “Spotted sandpiper (actitis macularius), version 2.0,” in The Birds of North America. In The Birds of North America, ed. A. Poole (Ithaca, NY: Cornell Lab of Ornithology).
Rensel, M. A., Ellis, J. M., Harvey, B., and Schlinger, B. A. (2015). Sex, estradiol, and spatial memory in a food-caching corvid. Horm Behav. 75, 45–54. doi: 10.1016/j.yhbeh.2015.07.022
Ribeiro, J. B. M. (2001). Micrometeorologia do manguezal e o impacto do desmatamento em Bragança-PA [tese]. São Carlos: Escola de Engenharia de São Carlos; Universidade de São Paulo (USP). doi: 10.11606/T.18.2001.tde-11112015-122408
Riley, D. E., and Krieger, J. N. (2009). UTR dinucleotide simple sequence repeat evolution exhibits recurring patterns including regulatory sequence motif replacements. Gene 429, 80–86. doi: 10.1016/j.gene.2008.09.030
Riou, S., Chastel, O., Lacroix, A., and Hamer, K. C. (2010). Stress and parental care: prolactin responses to acute stress throughout the breeding cycle in a long-lived bird. Gen Comp. Endocrinol. 168, 8–13. doi: 10.1016/j.ygcen.2010.03.011
Rodrigues, A. A. F. (2000). Seasonal abundance of neartic shorebirds in the gulf of maranhão, brazil. J. Field Ornithol. 71, 665–675. doi: 10.1648/0273-8570-71.4.665
Rodrigues, A. A. F. (2006). Aves da Reserva Biológica do Lago Piratuba e Entorno, Amapá, Brasil. Inventário Biológico das Áreas do Sucuriju e Região do lagos, Amapá: Relatório Final PROBIO. Macapá: Instituto de Pesquisas Científicas e Tecnológicas do Estado do Amapá, 188–195.
Rudecki, A. P., and Gray, S. L. (2016). PACAP in the defense of energy homeostasis. Trends Endocrinol. Metab. 27, 620–632. doi: 10.1016/j.tem.2016.04.008
Sadanandan, N., Cozene, B., Park, Y. J., Farooq, J., Kingsbury, C., Wang, Z. J., et al. (2021). Pituitary adenylate cyclase-activating polypeptide: a potent therapeutic agent in oxidative stress. Antioxidants (Basel) 10:354. doi: 10.3390/antiox10030354
Saino, N., Bazzi, G., Gatti, E., Caprioli, M., Cecere, J. G., Possenti, C. D., et al. (2015). Polymorphism at the clock gene predicts phenology of long-distance migration in birds. Mol. Ecol. 24, 1758–1773. doi: 10.1111/mec.13159
Samojłowicz, D., Twarowska-Małczyńska, J., Borowska-Solonynko, A., Poniatowski, Ł, Sharma, N., and Olczak, M. (2019). Presence of Toxoplasma gondii infection in brain as a potential cause of risky behavior: a report of 102 autopsy cases. Eur. J. Clin. Microbiol. Infect. Dis. 38, 305–317. doi: 10.1007/s10096-018-3427-z
Saper, C. B., and Sawchenko, P. E. (2003). Magic peptides, magic antibodies: guidelines for appropriate controls for immunohistochemistry. J. Comp. Neurol. 465, 161–163. doi: 10.1002/cne.10858
Schmitt, O., Preusse, S., and Haas, S. J. (2004). Comparison of contrast, sensitivity and efficiency of signal amplified and nonamplified immunohistochemical reactions suitable for videomicroscopy-based quantification and neuroimaging. Brain Res. Protoc. 12, 157–171. doi: 10.1016/j.brainresprot.2003.10.003
Schuelke, M. (2000). An economic method for the fluorescent labeling of PCR fragments. Nat. Biotechnol. 18, 233–234. doi: 10.1038/72708
Schweitzer, L., and Renehan, W. E. (1997). The use of cluster analysis for cell typing. Brain Res. Brain Res. Protoc. 1, 100–108.
Seo, H., and Lee, K. (2016). Epac2 contributes to PACAP-induced astrocytic differentiation through calcium ion influx in neural precursor cells. BMB Rep. 49, 128–133. doi: 10.5483/bmbrep.2016.49.2.202
Serrano, I. (2010). Distribuição e Conservação de Aves Migratórias Neárticas da Ordem Charadriiformes (Famílias Charadriidae e Scolopacidae) No Brasil. Brazil: department of zoology. universidade federal do pará museu paraense emílio goeldi programa de pós-graduação em zoologia curso de doutorado em zoologia, belém (PA), 174.
Shao, S., Yang, Y., Yuan, G., Zhang, M., and Yu, X. (2013). Signaling molecules involved in lipid-induced pancreatic beta-cell dysfunction. DNA Cell Biol. 32, 41–49. doi: 10.1089/dna.2012.1874
Shapiro, S., and Wilk, M. (1965). An analysis of variance test for normality (complete samples). Biometrika 52:591.
Sharma, A., Singh, D., Das, S., and Kumar, V. (2018a). Hypothalamic and liver transcriptome from two crucial life-history stages in a migratory songbird. Exp. Physiol. 103, 559–569. doi: 10.1113/EP086831
Sharma, A., Singh, D., Malik, S., Gupta, N. J., Rani, S., and Kumar, V. (2018b). Difference in control between spring and autumn migration in birds: insight from seasonal changes in hypothalamic gene expression in captive buntings. Proc. Biol. Sci. 285:20181531. doi: 10.1098/rspb.2018.1531
Shu, S., Ju, G., and Fan, L. (1988). The glucose oxidase-DAB-nickel method in peroxidase histochemistry of the nervous system. Neurosci. Lett. 85, 169–171. doi: 10.1016/0304-3940(88)90346-1
Simon, Á, Oláh, J., Komlósi, I., Jávor, A., Németh, J., Szilvássy, Z., et al. (2017). Changes in expression of neuropeptides and their receptors in the hypothalamus and gastrointestinal tract of calorie restricted hens. Acta Biol. Hung 68, 237–247. doi: 10.1556/018.68.2017.3.1
Skagen, S., Sharpe, P., Waltermire, R., and Dillon, M. (1999). Biogeographical Profiles of Shorebird Migration in Midcontinental North America. Springfield, VA: U.S. Dept. of the Interior, U.S. Geological Survey, 178.
Song, S., Dey, D. K., and Holsinger, K. E. (2011). Genetic diversity of microsatellite loci in hierarchically structured populations. Theor. Popul. Biol. 80, 29–37. doi: 10.1016/j.tpb.2011.04.004
Steinmeyer, C., Mueller, J. C., and Kempenaers, B. (2009). Search for informative polymorphisms in candidate genes: clock genes and circadian behaviour in blue tits. Genetica 136, 109–117. doi: 10.1007/s10709-008-9318-y
Surbhi Rastogi, A., Malik, S., Rani, S., and Kumar, V. (2016). Changes in brain peptides associated with reproduction and energy homeostasis in photosensitive and photorefractory migratory redheaded buntings. Gen Comp. Endocrinol. 23, 67–75. doi: 10.1016/j.ygcen.2016.03.031
Tachibana, T., Sugimoto, I., Ogino, M., Khan, M. S., Masuda, K., Ukena, K., et al. (2015). Central administration of chicken growth hormone-releasing hormone decreases food intake in chicks. Physiol. Behav. 139, 195–201. doi: 10.1016/j.physbeh.2014.11.043
Thalheimer, W., and Cook, S. (2002). How to calculate effect sizes from published research: a simplified methodology. Work Res. 1, 1–9.
Toth, D., Szabo, E., Tamas, A., Juhasz, T., Horvath, G., Fabian, E., et al. (2020). Protective effects of PACAP in peripheral organs. Front. Endocrinol. (Lausanne) 11:377. doi: 10.3389/fendo.2020.00377
van Toor, M. L., Hedenström, A., Waldenström, J., Fiedler, W., Holland, R. A., Thorup, K., et al. (2013). Flexibility of continental navigation and migration in European mallards. PLoS One 8:e72629. doi: 10.1371/journal.pone.0072629
Vaudry, D., Falluel-Morel, A., Bourgault, S., Basille, M., Burel, D., Wurtz, O., et al. (2009). Pituitary adenylate cyclase-activating polypeptide and its receptors: 20 years after the discovery. Pharmacol. Rev. 61, 283–357. doi: 10.1124/pr.109.001370
Vélez, E. J., and Unniappan, S. (2020). A comparative update on the neuroendocrine regulation of growth hormone in vertebrates. Front. Endocrinol. (Lausanne) 11:614981. doi: 10.3389/fendo.2020.614981
Ward, J. (1963). Hierarchical grouping to optimize an objective function. J. Am. Stat. Assoc. 58, 236–244.
West, M. J. (2002). Design-based stereological methods for counting neurons. Prog. Brain Res. 135, 43–51. doi: 10.1016/S0079-6123(02)35006-4
Williams, T., and Williams, W. (1978). An oceanic mass migration of land birds. Sci. Am. 239, 173–176. doi: 10.1038/scientificamerican1078-166
Wiltschko, R., and Wiltschko, W. (2019). Magnetoreception in birds. J. R. Soc. Interface 16:20190295. doi: 10.1098/rsif.2019.0295
Wiltschko, W., and Wiltschko, R. (2012). Global navigation in migratory birds: tracks, strategies, and interactions between mechanisms. Curr. Opin. Neurobiol. 22, 328–335. doi: 10.1016/j.conb.2011.12.012
Winters, S. J., and Moore, J. P. (2020). PACAP: a regulator of mammalian reproductive function. Mol. Cell. Endocrinol. 518:110912. doi: 10.1016/j.mce.2020.110912
Keywords: migratory birds, ADCYAP1 microsatellites, GFAP astrocytes morphological complexity, migratory distance, migration
Citation: de Almeida Miranda D, Araripe J, de Morais Magalhães NG, de Siqueira LS, de Abreu CC, Pereira PDC, Henrique EP, da Silva Chira PAC, de Melo MAD, do Rêgo PS, Diniz DG, Sherry DF, Diniz CWP and Guerreiro-Diniz C (2022) Shorebirds’ Longer Migratory Distances Are Associated With Larger ADCYAP1 Microsatellites and Greater Morphological Complexity of Hippocampal Astrocytes. Front. Psychol. 12:784372. doi: 10.3389/fpsyg.2021.784372
Received: 27 September 2021; Accepted: 29 December 2021;
Published: 04 February 2022.
Edited by:
Tom V. Smulders, Newcastle University, United KingdomReviewed by:
Alexey Brazhe, Lomonosov Moscow State University, RussiaLeslie Phillmore, Dalhousie University, Canada
Copyright © 2022 de Almeida Miranda, Araripe, de Morais Magalhães, de Siqueira, de Abreu, Pereira, Henrique, da Silva Chira, de Melo, do Rêgo, Diniz, Sherry, Diniz and Guerreiro-Diniz. This is an open-access article distributed under the terms of the Creative Commons Attribution License (CC BY). The use, distribution or reproduction in other forums is permitted, provided the original author(s) and the copyright owner(s) are credited and that the original publication in this journal is cited, in accordance with accepted academic practice. No use, distribution or reproduction is permitted which does not comply with these terms.
*Correspondence: Cristovam W. P. Diniz, Y3dwZGluaXpAZ21haWwuY29t
†Deceased