- 1Sport Sciences School of Rio Maior, Polytechnic Institute of Santarém, Santarém, Portugal
- 2Interdisciplinary Centre for the Study of Human Performance, Faculty of Human Kinetics, Cruz-Quebrada, Portugal
- 3Centro de Investigação Interdisciplinar em Saúde, Universidade Católica Portuguesa, Lisbon, Portugal
- 4Nursing Research, Innovation and Development Centre of Lisbon (CIDNUR), Nursing School of Lisbon, Lisbon, Portugal
- 5ESECS – Polytechnic of Leiria, Leiria, Portugal
- 6Research Centre in Sport, Health and Human Development (CIDESD), Vila Real, Portugal
- 7Life Quality Research Centre (CIEQV), Leiria, Portugal
- 8Comprehensive Health Research Centre (CHRC), NOVA Medical School, Universidade Nova de Lisboa, Lisbon, Portugal
- 9Centro de Investigação Interdisciplinar Egas Moniz (CiiEM), Instituto Universitário Egas Moniz, Monte de Caparica, Portugal
Introduction: Anthropomorphic conversational agents (ACA) are a promising digital tool to support self-management of type 2 diabetes (T2D), albeit little explored. There is a dearth of literature on the detailed content of these interventions, which may limit effectiveness and replication. Our aim is to describe the development of an evidence and theory-based intervention to improve physical activity in older adults with T2D, subsumed in a multi-behavior intervention via a mobile application with an ACA.
Methods: Overall decisions on the multi-behavior intervention design, such as the use of standardized behavior change techniques (BCTTv1), guided the development of the physical activity component. Firstly, recommendations on ambulatory activity were used to select the target behavior (walking). Meta-research on effective behavior change techniques (BCTs) was then identified. One meta-analysis linked effective BCTs with the three basic psychological needs of the self-determination theory (SDT). This meta-analysis, taken together with additional evidence on SDT, led to the selection of this theory to inform the design. BCTs were extracted from meta-research; we selected the most appropriate to be operationalized via the conversational agent through multidisciplinary discussions. Rules governing the dialogue flow and BCTs tailoring, taking the form “if some conditions hold then execute some action,” were derived based on the Basic Psychological in Exercise Scale (competence, autonomy, and relatedness scores), in conjunction with published evidence and multidisciplinary discussions.
Results: Thirteen BCTs were implemented in the prototype via the ACA (e.g., goal setting behavior 1.1). Six if-then rules were derived and depicted in the dialogue steps through process flow diagrams, which map how the system functions. An example of a rule is “If competence score ≤ 10 then, apply BCT 1.1 with 500 steps increments as options for the daily walking goal; If competence score > 10 then, apply BCT 1.1 with 1,000 steps increments as options for the daily walking goal.”
Conclusion: Evidence and SDT were translated into a mobile application prototype using an ACA to promote physical activity in older adults with T2D. This approach, which includes 13 BCTs and six if-then rules for their tailoring, may leverage the efforts of others in developing similar interventions.
Introduction
The sustainability and quality of healthcare provision in many countries is threatened by a constellation of factors, such as aging, the rising burden of non-communicable diseases and shortage of health professionals. It is estimated that the number of older people in the European Union (EU) will increase significantly, from 90.5 million at the start of 2019 to 129.8 million by 2050 (European Union, 2020). Public expenditure on health and long-term care has been increasing over the last decades in all EU Member States (European Union, 2020). These factors have driven the reengineering of health care delivery and the role of digital health technology.
Diabetes is one of the fastest growing health issues. Globally it affects around 537 million adults; type 2 diabetes (T2D) accounts for over 90% of all diabetes cases and has an increasing prevalence by age (International Diabetes Federation, 2021). Cardiovascular diseases are the leading cause of morbidity and mortality for individuals with diabetes accounting for an estimated cardiovascular-related cost of $37.3 billion per year, associated with diabetes (American Diabetes Association, 2018).
Furthermore, additional common metabolic conditions often coexist with T2D (e.g., hypertension and dyslipidemia) confers an increased health risk in this specific group (American Diabetes Association, 2021a). Diabetes-related health expenditures, irrespective of being borne by people living with diabetes, their families, or the health system, grew globally from USD 232 billion in 2007 to USD 966 billion in 2021 for adults aged 20–79 years, representing a 316% increase over 15 years (International Diabetes Federation, 2021).
Sustained hyperglycemia in persons with T2D also increases the risk of other complications, such as renal failure and retinopathy (International Diabetes Federation, 2021). T2D may also engender distress, understood as “negative emotional or affective experience resulting from the challenge of living with the demands of diabetes” (Skinner et al., 2020), and impaired health-related quality of life (Cannon et al., 2018).
It has been estimated that persons with diabetes spend fewer than 6 h per year consulting with healthcare professionals (Holt and Speight, 2017), which illustrates the importance of empowering and supporting these persons to actively self-manage the condition. Physical activity and other lifestyle behaviors fall under the remit of self-management, as they are dependent on the daily role taken by persons living with diabetes (American Diabetes Association, 2021b). Such behaviors, as regular physical activity and healthy eating are the cornerstone of T2D management (American Diabetes Association, 2021b; Kanaley et al., 2022).
Physical activity and exercise have been endorsed as a treatment or adjunct therapy for T2D and, at least, 25 other health conditions, including some T2D cardiometabolic comorbidities (Pedersen and Saltin, 2015; Shah et al., 2021). Sound evidence-based recommendations for physical activity and exercise for persons with T2D have been released (American Diabetes Association, 2021b; Kanaley et al., 2022). Simple physical activity behaviors such as walking one mile per day, or more (≥1.6 km/day), may provide a two-fold reduction in adjusted risk of all-cause mortality and a five-fold reduction in adjusted risk of non-coronary cardiovascular disease (CVD) death in older adults with diabetes (Smith et al., 2007).
Digital Behavior Change Interventions are a coordinated sets of activities or products designed to change specified behavioral patterns (e.g., physical activity) of individuals through digital technology, such as mobile applications, wearable technology (e.g., activity trackers), or websites. Digital Behavior Change Interventions are a promising approach for empowering diabetes self-management, as they have the capability to deliver personalized solutions to influence complex and challenging health behaviors (Michie et al., 2017). These digital interventions can support persons with diabetes to engage in physical activity, healthy eating, and overall disease management behaviors, intended to improve health outcomes and reduce complications (Fleming et al., 2020). Although T2D has a growing prevalence in older people, it has been recognized that mobile applications have limited usability for this group, which may hinder their use (Arnhold et al., 2014; Berenguer et al., 2017).
A meta-analysis of mobile applications for T2D, included 6 randomized controlled trials, with a total of 1,022 participants, and found an overall efficacy in reducing glycated hemoglobin (HbA1c), with a mean 0.40% decrease (95% CI 0.11 to 0.69%; Cui et al., 2016). Typically, a change in glycated hemoglobin of 0.5% is considered clinically significant (Little and Rohlfing, 2013). As for the cost-effectiveness of T2D digital interventions, a systematic review, included seven full economic evaluations, three of which comprised self-management support, albeit non-automated. Of these, the two studies that reported cost per quality-adjusted life-year (QALY) gained were cost-effective (Rinaldi et al., 2020). Although economic evaluation of these interventions is still in a nascent stage, it shows encouraging results, favoring resource allocation to digital diabetes self-management interventions.
Conversational agents, defined as computer programmes designed to simulate two-way human conversation using language (speech and/or text), potentially supplemented with non-language modalities are regarded as a promising approach to support diabetes self-management (Guerreiro et al., 2021). They may, for example, be more user friendly for people with lower literacy. These agents can be integrated in multiple devices, including mobile phones. Virtual human is another term to describe anthropomorphic conversational agents. A meta-analysis demonstrated the effectiveness of virtual humans in patient-facing systems, based on 26 controlled studies. Future accumulation of research may help to overcome the moderate heterogeneity in study results (Chattopadhyay et al., 2020). Notably, no trial involving long-term self-management support in T2D was included in this meta-analysis.
More recently, Luo et al. reported a dearth of research on conversational agents targeting physical activity in persons with T2D. In particular, published interventions do not always rely on behavior change theory (Luo et al., 2021), which may curtail their effectiveness, nor explicitly present their active components, which limits replication and knowledge transfer.
One example of the use of conversational agents in T2D management is the VASelfCare project (2018/01-2020/03).1 This project developed a multi-behavior change digital intervention for older people with T2D, via an anthropomorphic conversational agent and a connected web-based dashboard for health professionals. Vitoria, a 3D female virtual human, was designed as a coach for three target behaviors: medication taking, physical activity, and healthy eating, resorting to design principles for older adults. The overall intervention has been described previously (Balsa et al., 2020; Guerreiro et al., 2020) and the development of the medication taking component detailed elsewhere (Félix et al., 2019). The current paper focuses on the development of the physical activity component, a key behavior in diabetes management in older adults (Bellary et al., 2021).
The Medical Research Council (MRC) framework (Skivington et al., 2021) for developing and evaluating complex intervention, which guided the VASelfCare project, recommends an accurate process of development drawing on existing theories, modeling of process and outcomes, followed by feasibility assessment.
The importance of theory-based interventions for promoting physical activity in persons with T2D has been recently underscored (Konerding and Szel, 2021). Theory-based interventions are recommended as it provides assumptions about why interventions differently affect health behavior (Gourlan et al., 2016). The effectiveness of the intervention depends on the theory selected and how design and implementation of the intervention fits to the theoretical constructs. Additionally, only a small proportion of interventions publish the link between theory and behavior change techniques (BCTs), despite the recommendations to improve the transparency of theory-based interventions (Michie and Abraham, 2004; Abraham et al., 2014; National Institute for Health and Care Excellence, 2014). To enable interventions to be evaluated and implemented, BCTs should be well specified, increasing their accurate replication.
Enhancing the importance of adequate description of the interventions, the MRC endorses the publication of the intervention development process as it allows others to establish links between this process and the subsequent success of interventions and learn about the endeavors of this approach, which may be useful for developers (O’Cathain et al., 2019). Currently, there are limited examples in the literature of detailed descriptions of how systematic processes of developing digital health behaviors change interventions (Encantado et al., 2021), including in physical activity.
This paper describes the development of an evidence and theory-based intervention to improve physical activity in older adults with T2D, subsumed in a multi-behavior intervention via a mobile application with an anthropomorphic conversational agent.
Materials and Methods
The design of the VASelfCare intervention is described below, focusing firstly on the overall design, and then on the procedures to design the physical activity component, as part of the multi-behavior intervention (medication-taking, physical activity and healthy eating). The overall design of the intervention framed the development of the physical activity component and is therefore critical to its understanding. These procedures for the latter detail the use of theory and evidence for deriving the content of the physical activity component and rules to tailor them.
Overall Design of the Multi-Behavior Intervention
The anthropomorphic conversational agent, Vitoria, developed within the VASelfCare project, was designed to support behavior change through daily interactions with users. Based on the literature (e.g., Bickmore et al., 2010), users are offered the possibility of interacting with Vitoria once a day only. Vitória is capable of speaking European Portuguese and expressing emotions through facial and body animations; verbal content is supplemented with subtitles to help reduce potential communication barriers such as hearing deficits. User’s input consists of a set of options depicted in response buttons or through values recorded. For details on the IT development of the anthropomorphic conversational agent prototype refer to Balsa et al. (2020) and Guerreiro et al. (2020).
An overall design choice, regardless of the component, was to address each target behavior in two stages: in the evaluation stage Vitoria collects data to tailor the intervention content in the subsequent follow-up stage, which purports to promote or maintain the behaviors.
Tailoring is employed in this paper as “any combination of information and behavior change strategies intended to reach one specific person based on characteristics that are unique to that person related to the outcome of interest and derived from an individual assessment” (Kreuter et al., 2000). Across the three components of the VASelfCare intervention, tailoring relies not only on data from the evaluation stage, but also from previous interactions, and addresses both general information about diabetes, to improve health literacy, and the selection of BCTs, as explained later in this section.
Each daily interaction was structured in sequential steps based on the literature (Bickmore et al., 2005). In the evaluation stage, the sequential steps are depicted in Figure 1 (opening, social talk, assess, feedback, pre-closing and closing). The “opening” and “social talk” steps involve greeting the user and inquiries about the general emotional and physical state, respectively. Finally, the content of the next interaction is described in the “pre-closing” step and a farewell is delivered (“closing”). The dialogue in the follow-up stage has three additional steps, “review tasks,” “counseling,” and “assign tasks,” also described in Figure 1.
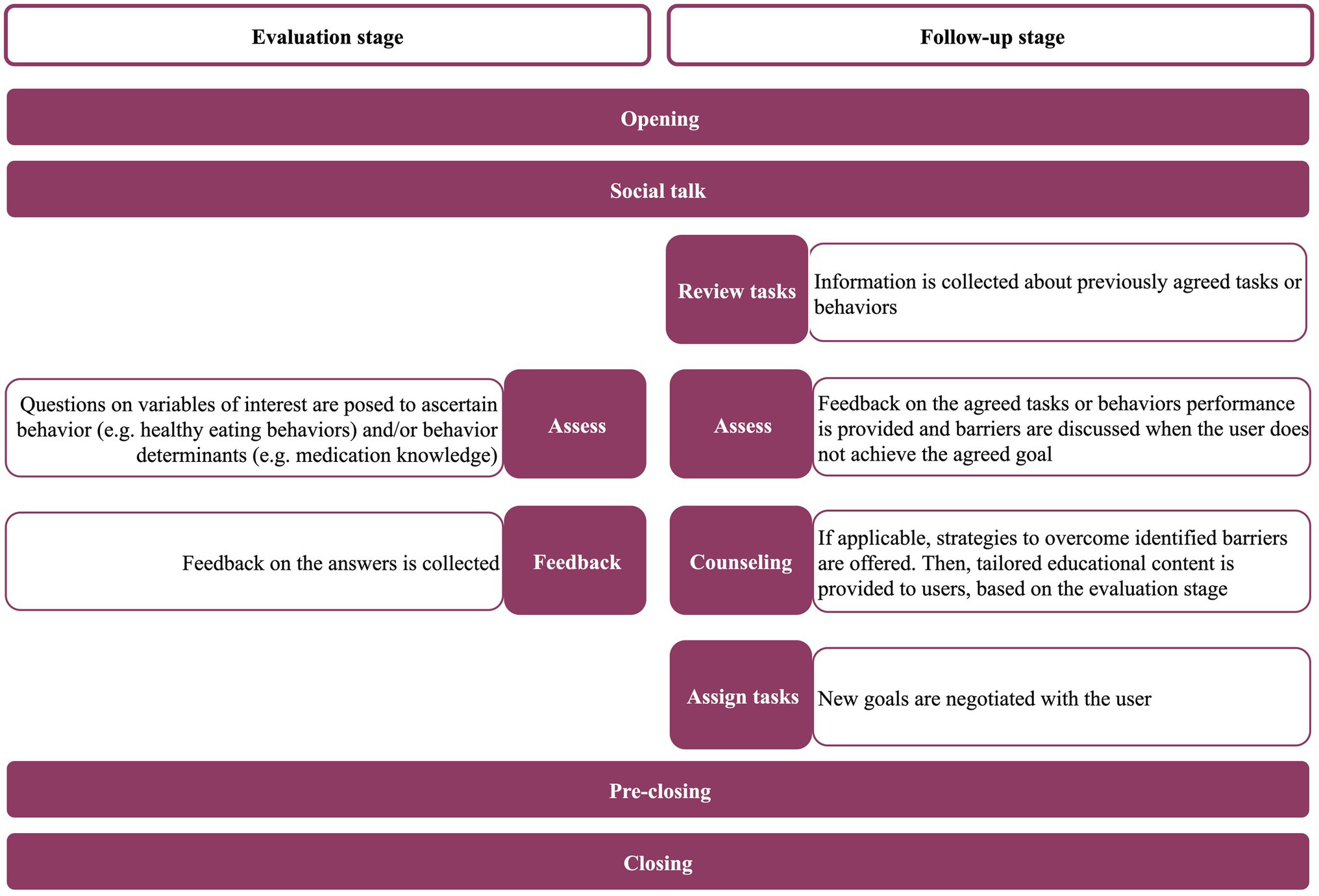
Figure 1. Steps of each daily interaction in the evaluation and follow-up stages across the three components (medication taking, physical activity and healthy eating).
A critical design decision was how to combine the physical activity component with the remaining components of the intervention: medication taking and healthy eating. There is little guidance on the best approach to design digital multi-behavior interventions; general recommendations indicate that interventions targeting behaviors requiring inaction (such as not eating high fat food) and those requiring action (such as increasing physical activity or taking medication) should not be pursued concomitantly (Albarracín et al., 2018). From a practical standpoint, pursuing three behaviors at the same time from the outset of the intervention would increase the length of each interaction to a point that was deemed detrimental for engagement. Taken together, these two aspects determined a stepwise approach, in which medication taking is firstly addressed, then the physical activity component added, while reducing the intensity of the medication taking component; as depicted in Figure 2, the same approach was employed for the healthy eating component. A less intensive intervention in each component, designated as “lite” (Figure 2), comes up after firstly, addressing each target behavior more intensively. “Lite” interventions have reduced number of dialogue steps and provide feedback to users based on weekly data (e.g., average medication taken in the last week). They encompass repeated collection of evaluation data, such as on medication knowledge and healthy eating behaviors, to enable intervention tailoring in the long term.
Design of the Physical Activity Component as Part of the Multi-Behavior Intervention
For the physical activity component, the VASelfCare intervention focused on ambulatory activity, mostly walking, as it has been endorsed for persons with T2D (Moghetti et al., 2020). This target group is regarded at high risk for exercise-related complications (Burr et al., 2012). Hence, we refrained from including recommendations of moderate to vigorous exercise, as the anthropomorphic conversational agent is unable to assess exercise tolerance and monitor exercise. Walking or walking-related activities are part of daily routine and considered feasible and low risk for older persons with T2D (Dasgupta et al., 2017; Barbosa et al., 2020). Correspondingly, daily step counting was chosen as a primary marker of this lower-level target behavior, focusing on helping users to achieve healthy levels of ambulatory physical activity (Tudor-Locke et al., 2011b).
An increase of 4,000 steps/day on a 5-day average step counts, from baseline, was found to be the threshold to elicit a clinically meaningful reduction on glycated hemoglobin in persons with type 2 diabetes, in a 24-week pedometer-based physical activity intervention (Van Dyck et al., 2013). More recently, a randomized controlled trial, resorting to a simple physician-delivered step count prescription strategy incorporated into routine clinical practice, showed that an average increase of 1,220 steps/day elicited a significant increase in insulin sensitivity and reduction of glycated hemoglobin (Dasgupta et al., 2017). A systematic review of studies in adults from non-clinical populations found that an increase of 1,000 steps/day elicited a reduction in the risk of all-cause mortality and cardiovascular morbidity and mortality (Hall et al., 2020). Data are currently lacking to identify an optimal health enhancing minimum threshold of daily step counts, still, health benefits can be found below 10.000 steps/day (Hall et al., 2020).
A walking cadence of 100 steps/min has been considered to be a reasonable empirical value for adults, indicative of walking at approximately the lower limit of the moderate-intensity spectrum (i.e., ≈ 3 METs; Tudor-Locke et al., 2011b; Tudor-Locke and Rowe, 2012). A target step counts of 7,000 steps/day, considering a step cadence of about 100 steps/min, is equivalent to approximately 70 min of walking per day. However, not all daily steps are expected to be performed at a cadence of 100 steps/min and, therefore, within the spectrum of moderate-intensity physical activity (Tudor-Locke et al., 2011a). Still, the goal of 7,000 steps/day has been shown to be consistent with physical activity guidelines for adults (Tudor-Locke et al., 2011c), particularly with the recommendation of 150 to 300 min/week of moderate–intensity, which has been recently endorsed also for persons with T2D (Kanaley et al., 2022), and has already been used and found beneficial in interventions in older and clinical populations (Gardner et al., 2021; Saad et al., 2021), including in persons with T2D (Rossen et al., 2021).
According to the rationale presented and the selected physical activity primary surrogate, a pedometer (New-Lifestyles NL-2000i Activity Monitor; New-Lifestyles Inc., Euless, TX, United States) was chosen to count daily steps. This device provides a reliable measure of this marker (Crouter et al., 2005; Grant et al., 2008; Tedesco et al., 2019); additionally, self-monitoring via a pedometer has been found useful on its own to increase daily step counts (Bravata et al., 2007), and beneficial for persons with T2D (Idowu et al., 2021). To foster scalability (future use across a range of devices), daily steps are inputted directly by users in the application interface.
A requirement for the content of the intervention was using behavior change techniques (BCTs) from an established taxonomy across the three target behaviors addressed by the intervention. A BCT is “an observable, replicable and irreducible component of an intervention designed to alter or redirect causal processes that regulate behavior (Michie et al., 2013). The Behaviour Change Taxonomy version 1 (BCTTv.1) was selected to specify these active ingredients of the intervention. BCTTv1 is an extensive hierarchically ordered and reliable taxonomy of 93 distinct BCTs that are categorized into 16 groups. For the physical activity component, the literature offered meta-research on effective BCTs (Cradock et al., 2017; Gillison et al., 2019), and therefore selecting BCTs using a systematic and structured process, as described for the medication adherence component (Félix et al., 2019), was deemed unnecessary.
In particular, one of the meta-analysis linked BCTs from the BCTTv.1 with the three basic psychological needs encompassed in the self-determination theory (Gillison et al., 2019).
Self-determination Theory (SDT) is a broad meta-theory of motivation. Central to the theory is the distinction between autonomous (self-determined) and controlled (non-self-determined) forms of motivation, which are further specified in a motivational continuum ranging from the most autonomous form of motivation (intrinsic motivation) to the most controlled form of motivation (external motivation; Ryan and Deci, 2017).
Autonomous motivation refers to a motivation that is based on self-endorsed reasons to choose and pursue a goal or action, when one has a full sense of willingness, volition, and choice, independently of the activity. One can feel autonomously motivated when doing an activity that is intrinsically enjoyable or fun (intrinsic motivation) or because that activity or goal genuinely fits their sense of self and values (integrated motivation). When acting with autonomy, a person is fully functioning, willingly engaged in activity with awareness and congruence, and able to harness vitality in the self-regulation of action (Ryan and Deci, 2017). In contrast, controlled motivation refers to reasons for acting that are not self-endorsed, that are subject to some form of pressure, either external by others or internally by the individual (e.g., feelings of guilt, for an external reward). Research in diverse life domains suggests that more autonomous, relative to controlled, motives are not only associated with, but essential to, a variety of positive outcomes (Ryan and Deci, 2017).
Additionally, the authors argue that all human beings have three basic psychological needs - Autonomy, Relatedness, Competence - that need to be satisfied for one to feel autonomously motivated and, consequently, to achieve optimal performance, psychological health and well-being (Ryan and Deci, 2017; Vansteenkiste et al., 2020). The need for competence reflects the need to feel effectance and mastery over tasks and behavior; the need of autonomy reflects the need to feel a sense of ownership and choice in acting; lastly, the need of unconditional support and connectedness with others is reflected by relatedness. It is posited that when the three basic psychological needs are satisfied, autonomous motivation and mental health are enhanced (Ryan and Deci, 2000).
There is strong evidence on the effectiveness of interventions based on the self-determination theory across a wide range of health domains, including physical activity (Teixeira et al., 2012). Primary research also supports the use of this theory as successful in increasing physical activity in older adults with type 2 diabetes (Koponen et al., 2018). Taken together, this evidence led to choosing the self-determination theory (SDT) to inform the design of the physical activity component. A more recent meta-analysis corroborates this choice; Ntoumanis et al. (2021) showed that SDT-based interventions (n = 73) positively affect health behaviors at the end of the intervention period and at the follow-up, in particular physical activity.
BCTs were extracted and listed from meta-research (Cradock et al., 2017; Gillison et al., 2019). As explained, Gillison et al. (2019) presented BCTs to promote psychological needs, satisfaction and motivation in health interventions based on the self-determination theory; Cradock et al. (2017) identified the BCTs associated with changes in glycated hemoglobin and body weight in persons with T2D.
From the listed BCTs, we selected the most appropriate to be operationalized via the conversational agent through multidisciplinary discussion, inspired by the practicality criterion, as defined by Michie et al. (2014) - extent to which the intervention can be delivered as designed through the means intended to the target population. In essence, foci of discussions were whether it was practicable to deliver the listed BCTs through the conversational agent. The team included expertise from the disciplines of sport sciences, psychology, nursing, pharmacy, and informatics.
Vitoria dialogues resort to an artificial intelligence rule-based engine. Rules derived to control the dialogue flow take the form “if some conditions hold then execute some action,” where the conditions may include context information regarding the interaction (e.g., user characteristics or the date when interaction takes place) and the action represents the subsequent act to be performed by the conversational agent. These rules were informed by the Basic Psychological in Exercise Scale (Moutão et al., 2012), published evidence (BCTs emanating from meta-research, as explained) and multidisciplinary discussions. The Basic Psychological in Exercise Scale is used to assess psychological needs for exercise underlying Self-Determination Theory (Ryan and Deci, 2017); it comprises a total of 12 items grouped into three factors: autonomy (four items), competence (four items) and relatedness (four items). Responses are provided on a 5-point Likert-type scale ranging from 1 (strongly disagree) to 5 (strongly agree). The maximum score for each construct is 20.
Results
Selection and Operationalization of BCTs in the Physical Activity Component
Thirteen BCTs were selected for implementation in the mobile application prototype via the conversational agent. Table 1 details these BCTs and their operationalization according to the interaction steps in the follow-up stage. In the evaluation stage, BCTs are not applicable as its purpose is not changing behavior.
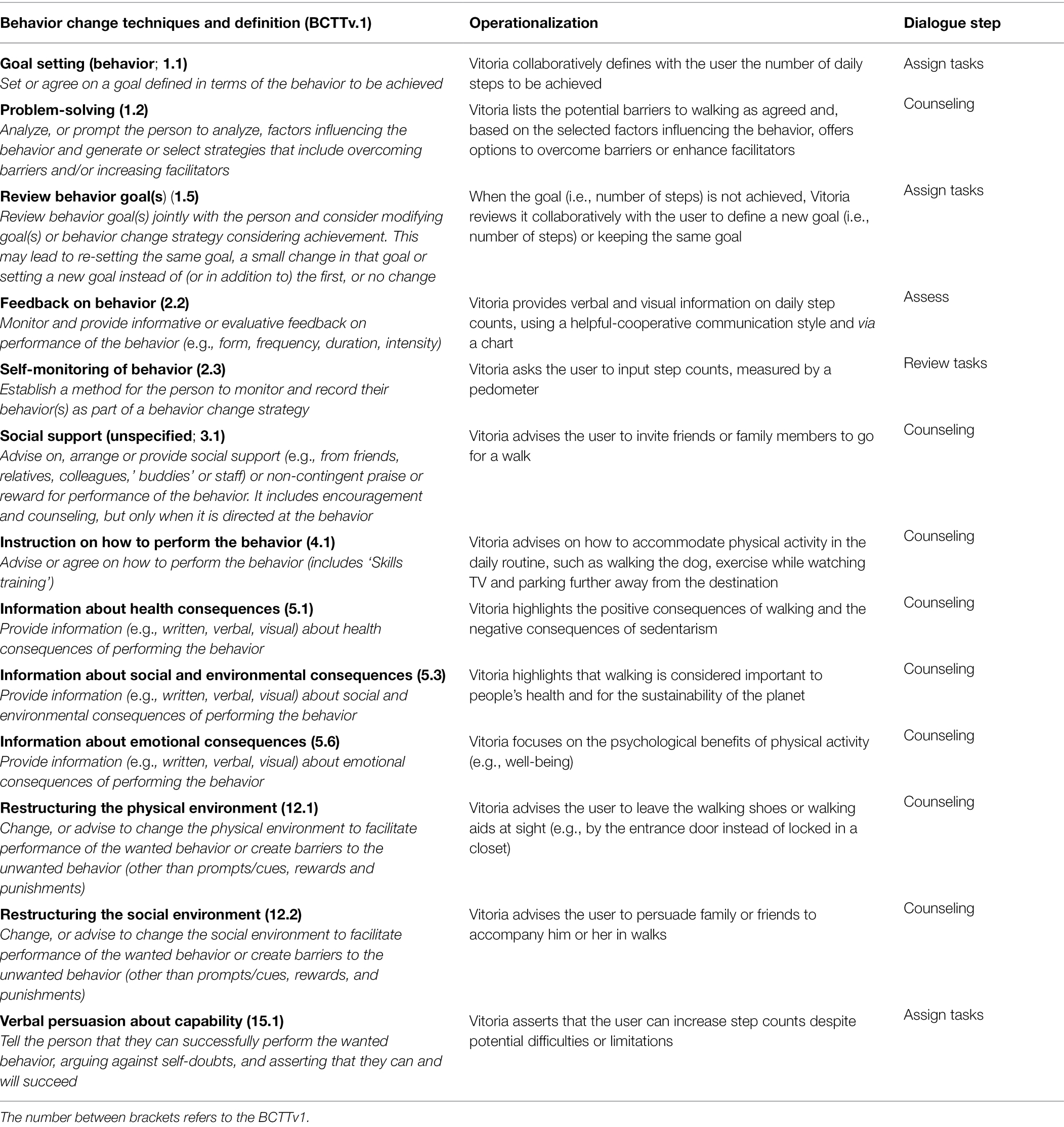
Table 1. Description of the BCTs and operationalization used by the anthropomorphic conversational agent.
Rules for Tailoring BCTs in the Physical Activity Component
This section presents rules for tailoring BCTs in the physical activity component. Firstly, the “if-then” rules are summarized in Table 2, then rules are put into context in the interaction steps through process flow diagrams, which map how the system functions.
BCTs were operationalized differently on the first day of follow-up and on the subsequent even and odd days. Underlying this approach was the fact that the walking goal defined with Vitoria on any given day (D) pertains to the day after the interaction (D + 1); in other words, the walking goal entails the full steps of D + 1. Since the user can interact with Vitoria at any time on D + 1, goal achievement could be unduly compromised. Therefore, the goal set on day 1 was designed to be assessed on day 3 (Feedback on behavior, 2.2), by reporting the step counts on day 2, corresponding to a full day, and so forth.
Day 1 of Physical Activity Component: Follow-Up Stage
Figure 3 details the dialogue flow on day 1 of the follow-up stage according to the rules described above.
The first and last two steps (“opening” and “social talk” plus “pre-closing” and “closing,” respectively) are common in terms of content across the interactions, regardless of the day, as explained in the Materials and Methods section.
On the first day of follow-up, information is collected about the average step counts of the last 7 days, in “review tasks” (self-monitoring of behavior 2.3). At this stage no behavior goal has been set up yet, and therefore the “assess” step, in which feedback would be given, is skipped.
In the “counseling” step, Vitoria gives general information about T2D and complications, such as details on hypoglycemia symptoms and how to manage them, to improve health literacy.
Next, in the “assign tasks” step, Vitoria collaboratively agrees with the user on a goal for step counts for the next day (goal setting behavior 1.1). The three options presented are informed by the competence score, yielded in the evaluation phase, and the average step counts of the last 7 days, as shown in example A of Table 2. For example, if the user reports an average of 7,000 steps in the last 7 days and the competence score is >10, Vitoria suggests three goals for the next day: 8000, 7,000 and 6,000 steps.
Day 2 and Subsequent Even Days of the Physical Activity Component: Follow-Up Stage
On the second day and even days of the follow-up stage, Vitoria dialogue follows the steps detailed in Figure 4.
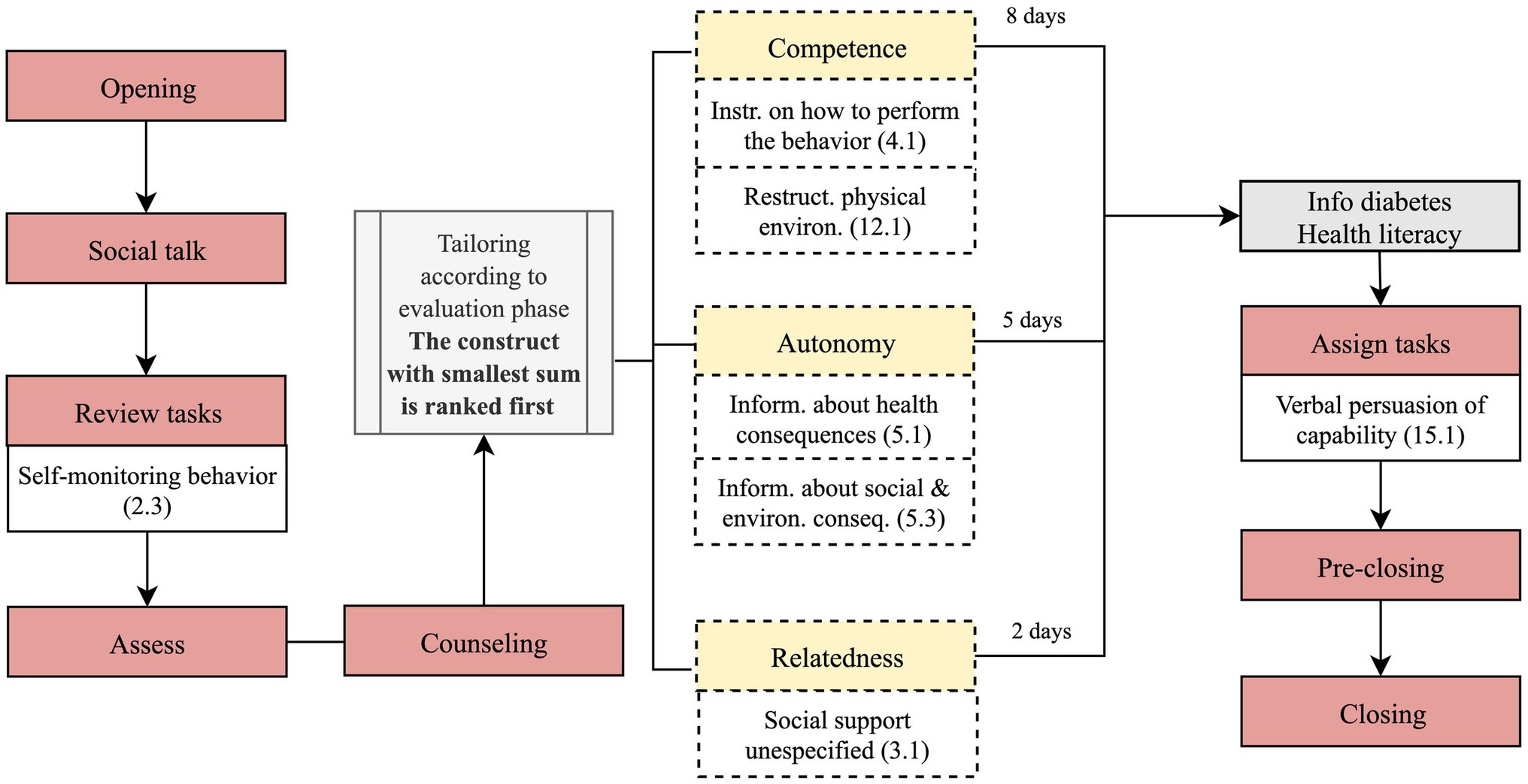
Figure 4. Dialogue flow on day 2 and even days of physical activity component of the follow-up stage.
In the “review tasks” step, Vitoria asks the user to input the step counts recorded by the pedometer on the previous day (self-monitoring of behavior 2.3). This step is followed by the “assess” step, in which Vitoria reminds the user of the agreed goal (first day and odd days).
Then, information to improve competence, autonomy and relatedness is delivered stepwise throughout the intervention (“counseling” step), starting with the construct with the lowest score. For instance, in a user with a competence, autonomy and relatedness scores of 4, 12, 18, respectively, Vitoria will firstly address competence, encompassing a set of BCTs, as illustrated by example B, Table 2. The number of days allocated to each construct varies between two for relatedness and eight for competence, assuming one interaction per day, as already explained in section 2.1. Each construct is targeted in consecutive days, or interspersed with eliciting behavioral barriers, if the walking goal is not met, as assessed on odd days. When competence is addressed, Vitoria explains, for instance, how to use resources to walk, such as a walker, a cane, or a trekking pole (instruction on how to perform the behavior 4.1). Addressing autonomy entails, for example, information about the positive consequences of walking (information about health consequences 5.1). To promote relatedness, Vitoria suggests inviting a friend or a family member to walk or joining group classes (social support unspecified 3.1).
Day 3 and Subsequent Odd Days of the Physical Activity Component: Follow-Up Stage
Figure 5 presents the dialogue flow on the third day and subsequent odd days; the key features in relation to even days is that feedback on behavior is provided, and the walking goal is reviewed. As illustrated in Figure 5, the step counts collected in “review tasks” is compared with the behavior goal, through verbal and visual feedback (2.2), via Vitoria’s speech and a chart (“assess” step). If the agreed goal has not been reached, Vitoria addresses barriers and proposes strategies to overcome them in the “counseling” step (problem-solving 1.2), as already explained in example C (Table 2). In Figure 6, Vitoria is portrayed implementing problem-solving (1.2). For instance, if the user chooses time constraints as a barrier, Vitoria recommends simple ways to improve step counts by integrating walking in the user’s routine, such as parking the car further away from the destination, selecting different routes to walk longer distances or using the stairs.
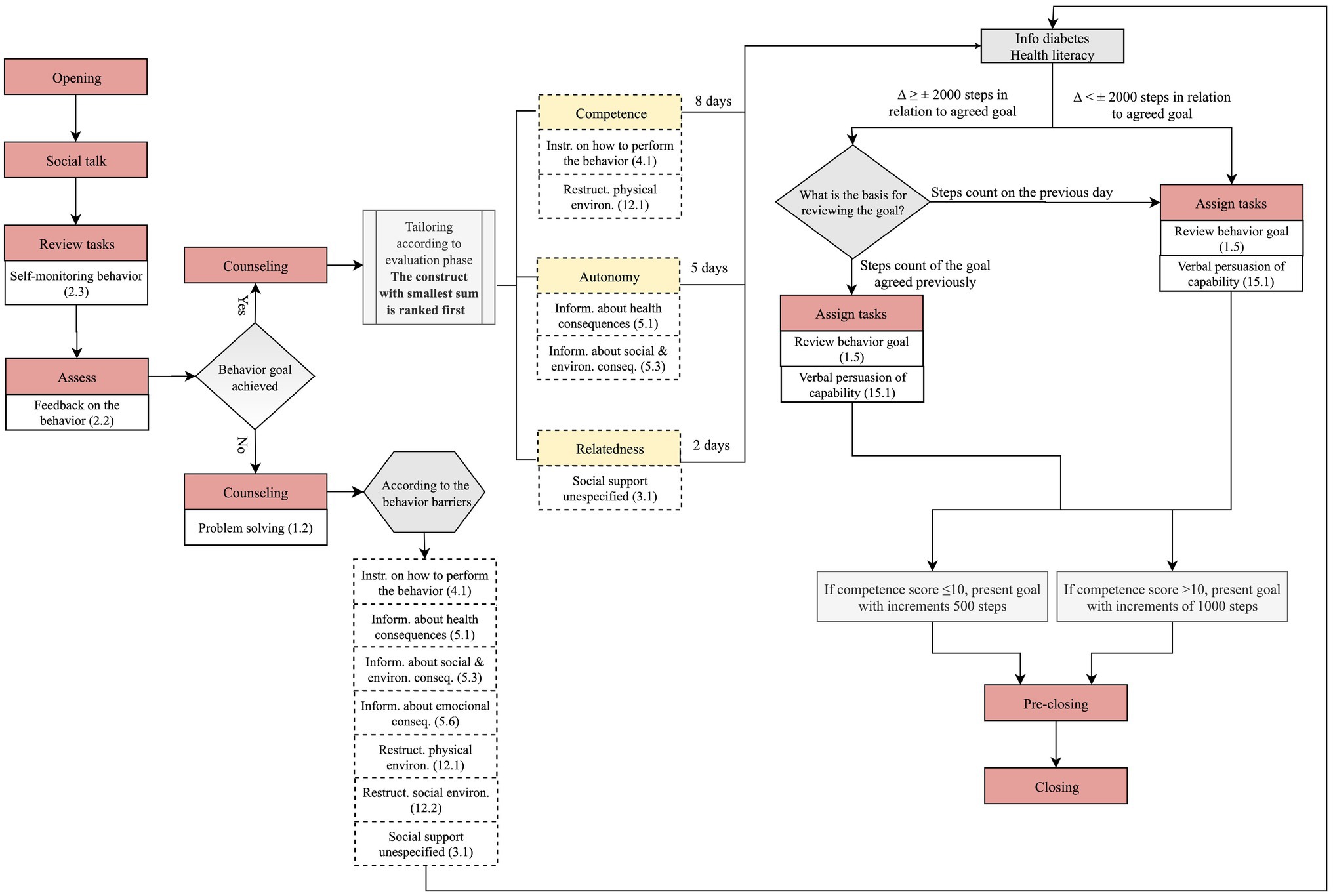
Figure 5. Dialogue flow in day 3 and odd days of physical activity component of the follow-up stage.
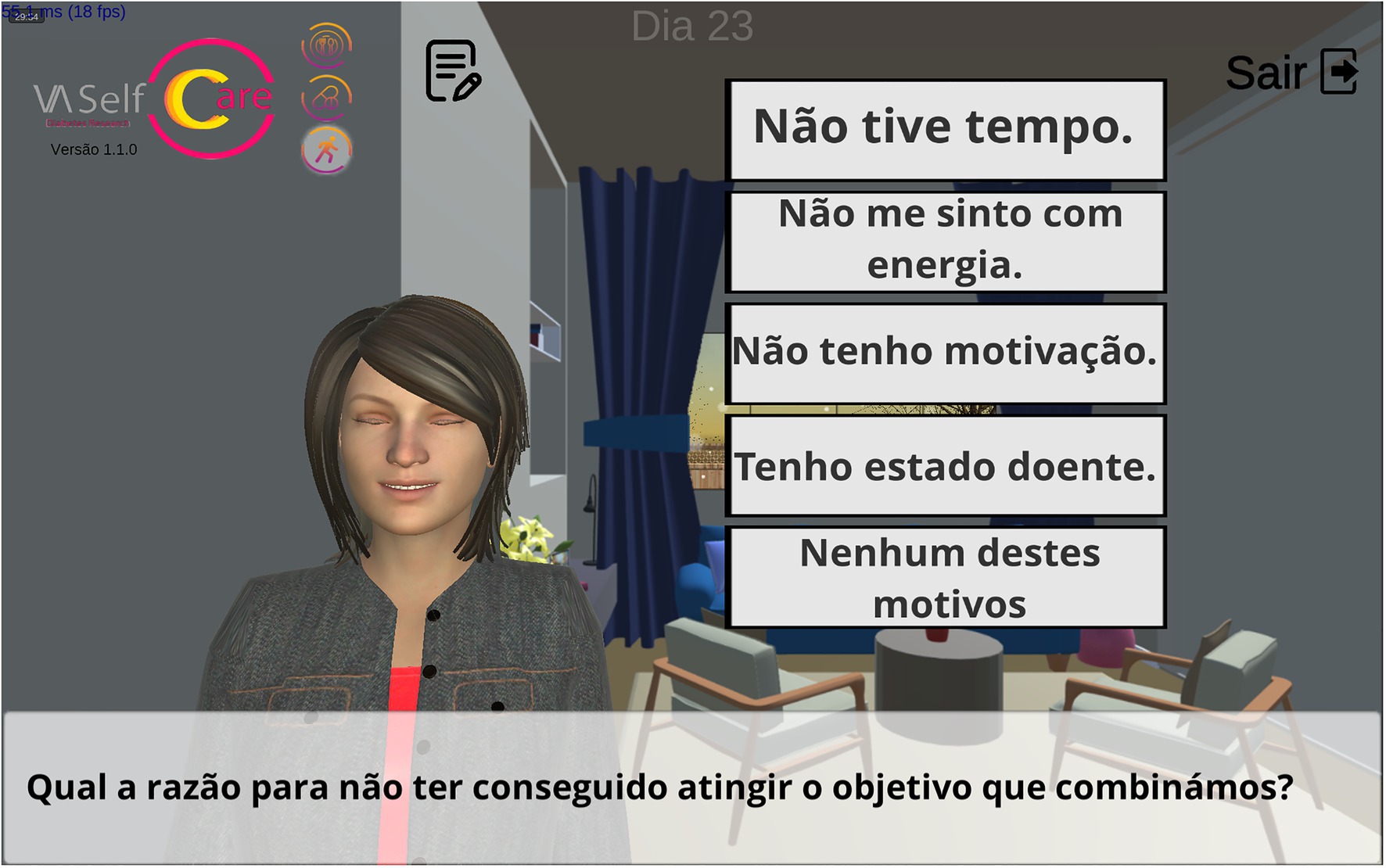
Figure 6. Interface of the mobile application: Vitoria addressing barriers to walking (problem-solving 1.2). Translation to English: What is the reason for not having achieved the goal we agreed upon? (subtitle); (A) I did not have time; (B) I do not feel energetic; (C) I am not motivated; (D) I have been sick and (E) None of these reasons (response options).
If the agreed goal is achieved, the intervention turns to the three basic psychological needs (competence, autonomy, and relatedness) in “counseling,” according to the ranking set up on day 2 for these constructs, as already explained. BCTs operationalized in this step are detailed in Table 2 (see example B).
Next, in the “assign tasks” the variation of step counts in relation to the agreed goal determines two paths (example D, Table 2). For the sake of illustration, if a user reports a count of 14,000 steps and the agreed goal was 6,000 steps, the variation is greater than 2000 (Δ ≥ ± 2000 steps). Vitoria asks whether the user wants to review the goal using the reported step counts or the agreed goal as the basis. Assuming the user selects the latter, the goal is reviewed using 6,000 steps as the basis, with increments above and below determined by the competence score (e.g., 5,500, 6,000, 6,500 steps). Figure 7 depicts another example.
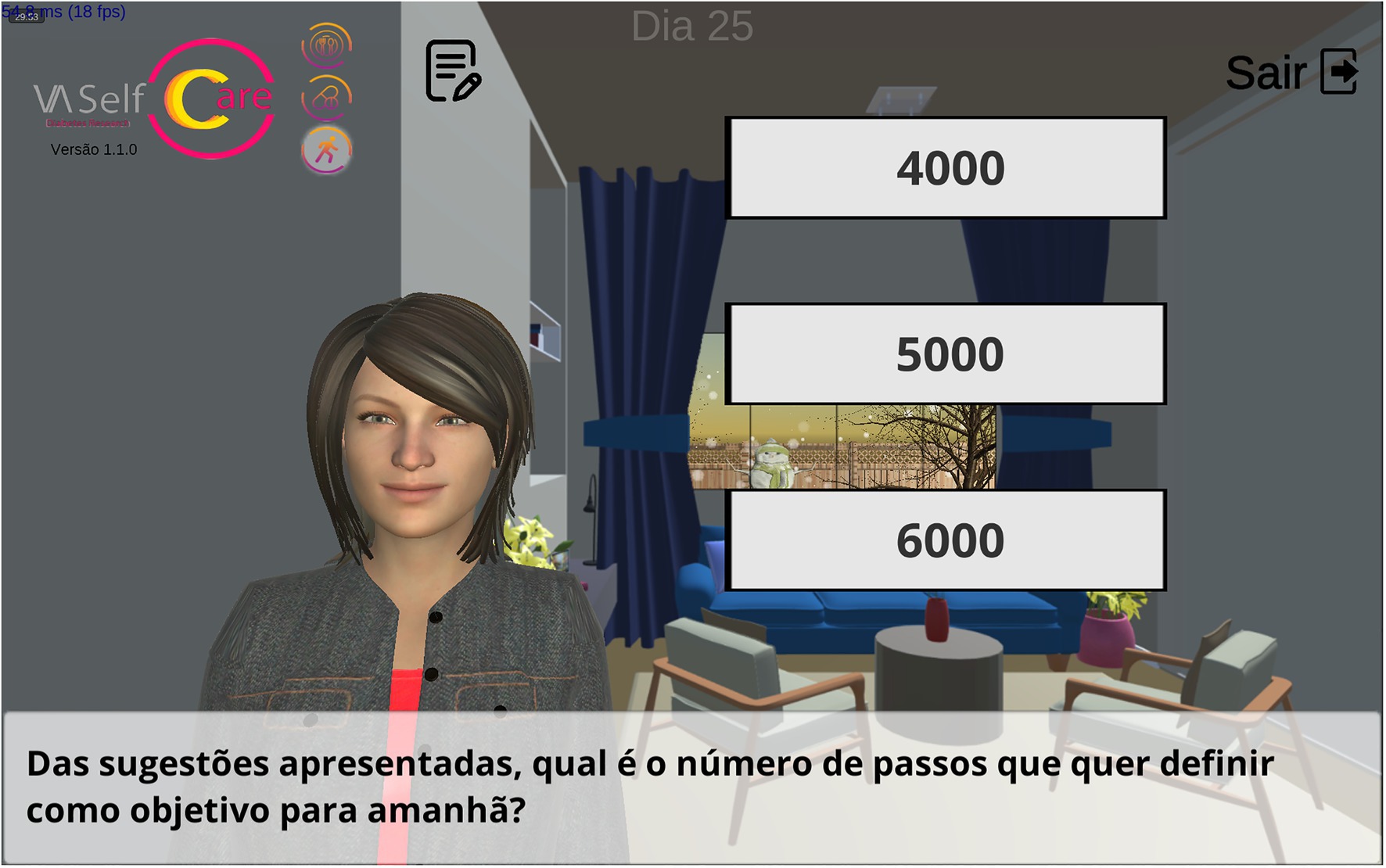
Figure 7. Interface in the mobile application: Vitoria reviewing the number of steps goal (review behavior goal 1.5). Translation to English: Of the suggestions presented, what is the number of steps you want to set as a goal for tomorrow? (subtitle).
“Lite” Physical Activity Component
The “lite” physical activity component, depicted in Figure 2, starts on the first day of the healthy eating component, when both the medication taking, and physical activity have been addressed more thoroughly. In essence, in the “lite” component, Vitoria collects information on step counts in each daily interaction but only assesses and counsels every 8 days; moreover, “assign tasks” set goals for the next week, and not for the next day.
Figure 8 presents only the dialogue steps regarding the “lite” version of the physical activity component. Vitoria starts by asking the user to input the step counts (“review tasks”). Then, the dialogue guides the user to the healthy eating component. This flow is repeated for 7 days. On the eighth day, Vitoria gives feedback on the average step counts of the last 7 days, which corresponds to the “assess” step (feedback on behavior 2.2).
Next, in the “assign tasks” the variation of step counts in relation to the average weekly goal determines two paths (example F, Table 2). For the sake of illustration, if a user has an average weekly count of 6,600 steps and the agreed goal for the week was 6,000 steps, the variation is smaller than 2000 (Δ < ± 2000 steps), the goal is reviewed using 6,600 steps as the basis, with increments above and below determined by the competence score (e.g., 5,600, 6,600, 7,600 steps).
Discussion
This article illustrates an evidence and theory-based approach to specify the BCTs and their tailoring of an intervention to improve physical activity in older adults with T2D, subsumed in a multi-behavior intervention, via a mobile application with an anthropomorphic conversational agent.
While the design of the physical activity component drew on evidence and theory, there was a strong concern to keep a good fit with daily routine, by resorting to step counts as a measure of physical activity and by drawing on the multidisciplinary experience of the team to list facilitators and barriers.
Thirteen standardized BCTs from the BCTTv1 were chosen, based on meta-research and their practicality to the intervention. These BCTs were tailored in predefined steps of each interaction, using six if-then rules, which determine how the anthropomorphic conversational agent, Vitoria, interacts with users.
A limitation of our work is that users have to choose from a limited set of options when talking to Vitoria; moreover, BCTs are embedded in the dialogues in a rigid way, which limits the possibilities of tailoring. More recently, we attempted to overcome these issues by using an advanced natural language platform (e.g., Google Dialogflow) and an ontology-based knowledge representation, indicating how BCTs can be operationalized (Bastos et al., 2022).
Our work can also be criticized by the fact that the effectiveness of the digital intervention has not yet been evaluated. Usability testing with older adults living with T2D showed encouraging results (Balsa et al., 2020), but ultimately the merit of the intervention will be judged based on its ability to produce positive health outcomes. While a clinically significant decrease in glycated hemoglobin is typically warranted, humanistic health outcomes should not be demeaned. A paper provocatively entitled “If it does not significantly change HbA1c levels why should we waste time on it?,” reminds us of the perils of providing care to persons with diabetes contingent only upon achieving clinical outcomes (Jones et al., 2015). The only example that we are aware of a virtual human coach intervention for self-managing chronic disease resulted in statistically significant improvement in health-related quality of life, but not in glycated hemoglobin (Gong et al., 2020). This should be regarded as equally beneficial as improvements in glycated hemoglobin. The mean age of participants in the intervention group (n = 93) of this Australian randomized effectiveness-implementation trial was 55.4 years (SD 9.7; Gong et al., 2020), which reinforces the need for trialing our intervention in older adults, in a different context, leading to the accumulation of evidence on fully automated virtual human coaches. To determine trends in outcomes of interest, a protocol was drafted for a non-randomized non-controlled 3-month feasibility trial in nursing consultations in primary care. Endpoints, selected according to the International Consortium for Health Outcomes Measurement (ICHOM, 2019), included steps count as part of lifestyle factors, glycated hemoglobin for diabetes control, and psychological well-being as a patient-reported outcome. Self-monitoring of step counts resorting to pedometers is, on its own, beneficial to promote physical activity and related health outcomes (Bravata et al., 2007; Idowu et al., 2021). However, the need to input step counts manually in the mobile application prototype may be burdensome over time and can impact on intervention engagement and intervention fatigue, two important mechanisms influencing the use of mobile applications and retention (Nahum-Shani et al., 2018).
Just-in-time adaptive interventions (JITAIs) provide the right type (or amount) of support, at the right time, while eliminating support that is not beneficial, through continuous monitoring of the person’s state and context (Nahum-Shani et al., 2018). In what concerns physical activity, JITAIs are exemplified by prompting users for exercise at a particular time of the day if a certain accumulated steps count collected via passive assessment (e.g., via a smartphone accelerometer) has not been reached (Nahum-Shani et al., 2018). While self-report of step counts may undermine engagement over time and contribute to intervention fatigue, it has the advantage of improving the scalability of the intervention, by keeping it functional on a range of devices and simple to use. The latter is also important when attempting not to aggravate health inequalities. It has been suggested that the digital divide is shifting from access and connectivity to a knowledge gap on how to use information and communication technology (McAuley, 2014). This is particularly important for older adults, who are our target group. While global data show a consistent upward trend in smartphone penetration in those 65+, this does not necessarily translate in the use of mobile applications (Berenguer et al., 2017). Therefore, currently it appears sensible to keep applications for older adults as simple as possible. Usage data from trials in conjunction with qualitative explorations will shed light on older adults’ engagement with the VASelfCare digital intervention and their preferences.
An analysis of 16 mobile applications marketed for the prevention and management of T2D pleaded for more transparency in reporting the app features and employed BCTs (Keller et al., 2022). We believe the same plea should be made to researchers. We found little guidance from the scientific literature on granular aspects of intervention design and the operationalization of BCTs for digital behavior change interventions in T2D. For instance, the work of Gong et al. (2020), while providing much needed evidence on the effectiveness and implementation of a virtual human coach to support T2D self-management, offers little detail on the BCTs employed, hindering not only replication but also improvements in the intervention design and content.
The way in which the behavior change techniques are delivered is considered an element of behavior change interventions that warrants consideration as it can explain the (in-)efficacy of a given technique (Marques et al., 2020). Vitoria’s dialogues were created resorting to a helpful-cooperative communication style (Niess and Diefenbach, 2016), aiming to build rapport and trust. This non-judgmental approach is considered to increase autonomy and relatedness which are core basic psychological needs according to the Self-Determination Theory. Further, this communication style is in line with recommendations on the use of language to communicate with and about persons with diabetes, grouped under the umbrella of the Language Matters Diabetes global movement.2 These recommendations were developed based on evidence and expert opinion in countries such as Australia, United States and the United Kingdom (Dickinson et al., 2017; Cooper et al., 2018; Speight et al., 2021) and adapted for other countries, for guiding health professionals and other stakeholders (Batata et al., 2022). We believe there is room for applying the preferred language and principles entailed in these recommendations to digital behavior change interventions in T2D, to harness higher rapport and trust, aiming at higher engagement and effectiveness.
A final point meriting discussion is whether the VASelfCare prototype is appropriate to other cultures. Walking is a commonly accepted form of physical activity for both men and women in western societies, but maybe less common in other cultures. For example, research in India suggested that women associate physical activity mostly with household chores and do not contemplate walking as an exercise option, albeit finding it feasible (Mathews et al., 2016). This raises the point of cultural adaptation of digital behavior change interventions.
Conclusion
Evidence and theory have been translated into an m-health prototype using an anthropomorphic conversational agent to promote physical activity in older adults with type 2 diabetes, as part of a multi-behavioral intervention. This approach, which includes 13 BCTs and six if-then rules that determine their tailoring and dialogue flow, is expected to maximize effectiveness and to facilitate replication. Ultimately, the present work may leverage the efforts of others in developing self-management interventions targeting lifestyle behaviors.
Data Availability Statement
The original contributions presented in the study are included in the article/supplementary material, further inquiries can be directed to the corresponding author.
Author Contributions
IF, NP, and MG conceived the work reported in this paper. IF and NP led the design of the physical activity component, supported by MG, DM, and MM. IF, NP, and MG wrote the first draft of the manuscript. MM performed a first critical review. Subsequent iterations of the manuscript were commented on by all authors. All authors contributed to the article and approved the submitted version.
Funding
This project was supported by FCT and Compete 2020 (grant number LISBOA-01-0145-FEDER-024250, 02/SAICT/2016).
Conflict of Interest
The authors declare that the research was conducted in the absence of any commercial or financial relationships that could be construed as a potential conflict of interest.
Publisher’s Note
All claims expressed in this article are solely those of the authors and do not necessarily represent those of their affiliated organizations, or those of the publisher, the editors and the reviewers. Any product that may be evaluated in this article, or claim that may be made by its manufacturer, is not guaranteed or endorsed by the publisher.
Acknowledgments
We are indebted to the VASelfCare team for their contribution to the software development (http://vaselfcare.esel.pt). In particular, a warm thank you goes to Ana Paula Cláudio, Beatriz Carmo, João Balsa, Susana Buinhas, Pedro Alves, Pedro Neves and João Anastácio, for the IT development of the mobile application prototype. Building on knowledge generated by the VASelfCare project, Maria Inês Bastos, João Balsa and Ana Paula Cláudio led more recent work on the new architecture for the design of behavior change conversational agents.
Footnotes
References
Abraham, C., Johnson, B. T., De Bruin, M., and Luszczynska, A. (2014). Enhancing reporting of behavior change intervention evaluations. J. Acquir. Immune Defic. Syndr. 66, Suppl. 3, S293–S299. doi: 10.1097/QAI.0000000000000231
Albarracín, D., Wilson, K., Chan, M. P. S., Durantini, M., and Sanchez, F. (2018). Action and inaction in multi-behaviour recommendations: a meta-analysis of lifestyle interventions. Health Psychol. Rev. 12, 1–24. doi: 10.1080/17437199.2017.1369140
American Diabetes Association (2018). Economic costs of diabetes in the U.S. in 2017. Diabetes Care 41, 917–928. doi: 10.2337/dci18-0007
American Diabetes Association (2021a). Cardiovascular disease and risk management: Standards of medical care in diabetes - 2021. Diabetes Care 44, S125–S150. doi: 10.2337/dc21-S010
American Diabetes Association (2021b). Facilitating behavior change and well-being to improve health outcomes: Standards of medical care in diabetes−2021. Diabetes Care 44, S53–S72. doi: 10.2337/dc21-S005
Arnhold, M., Quade, M., and Kirch, W. (2014). Mobile applications for diabetics: a systematic review and expert-based usability evaluation considering the special requirements of diabetes patients age 50 years or older. J. Med. Int. Res. 16, 1–18. doi: 10.2196/jmir.2968
Balsa, J., Félix, I., Cláudio, A. P., Carmo, M. B., Silva, I. C., Guerreiro, A., et al. (2020). Usability of an intelligent virtual assistant for promoting behavior change and self-care in older people with type 2 diabetes. J. Med. Syst. 44:130. doi: 10.1007/s10916-020-01583-w
Barbosa, A., Brito, J., Costa, J., Figueiredo, P., Seabra, A., and Mendes, R. (2020). Feasibility and safety of a walking football program in middle-aged and older men with type 2 diabetes. Prog. Cardiovasc. Dis. 63, 786–791. doi: 10.1016/j.pcad.2020.06.014
Bastos, M. I., Cláudio, A. P., Félix, I. B., Guerreiro, M. P., and Beatriz, M. (2022). “Operationalizing Behavior Change Techniques in Conversational Agents.” In 14th International Conference on Agents and Artificial Intelligence (ICAART 2022), 216–224.
Batata, M., Braz, A., Camolas, J., Costa, A. F., Ferreira, H., Martins, H., et al. (2022). “Language Matters,” Portugal: Recomendações sobre a Linguagem Preferencial na Comunicação Com e Sobre as Pessoas com Diabetes. Revista Portuguesa de Diabetes 17, 26–33.
Bellary, S., Kyrou, I., Brown, J. E., and Bailey, C. J. (2021). Type 2 diabetes mellitus in older adults: clinical considerations and management. Nat. Rev. Endocrinol. 17, 534–548. doi: 10.1038/s41574-021-00512-2
Berenguer, A., Goncalves, J., Hosio, S., Ferreira, D., Anagnostopoulos, T., and Kostakos, V. (2017). Are Smartphones Ubiquitous? IEEE Consum. Electron. Mag. 6, 104–110.
Bickmore, T. W., Caruso, L., Clough-Gorr, K., and Heeren, T. (2005). ‘It’s just like you talk to a friend’ relational agents for older adults. Interact. Comput. 17, 711–735. doi: 10.1016/j.intcom.2005.09.002
Bickmore, T. W., Puskar, K., Schlenk, E. A., Pfeifer, L. M., and Sereika, S. M. (2010). Maintaining reality: relational agents for antipsychotic medication adherence. Interact. Comput. 22, 276–288. doi: 10.1016/j.intcom.2010.02.001
Bravata, D. M., Smith-Spangler, C., Sundaram, V., Gienger, A. L., Lin, N., Lewis, R., et al. (2007). Using pedometers to increase physical activity and improve health: A systematic review. J. Am. Med. Assoc. 298, 2296–2304. doi: 10.1001/jama.298.19.2296
Burr, J. F., Shephard, R. J., and Riddell, M. C. (2012). Prediabetes and type 2 diabetes mellitus: assessing risks for physical activity clearance and prescription. Can. Fam. Physician 58, 280–284.
Cannon, A., Handelsman, Y., Heile, M., and Shannon, M. (2018). Burden of Illness in Type 2 Diabetes Mellitus. J. Manag. Care Spec. Pharm. S5 24, S5–S13. doi: 10.18553/jmcp.2018.24.9-a.s5
Chattopadhyay, D., Ma, T., Sharifi, H., and Martyn-Nemeth, P. (2020). Computer-controlled virtual humans in patient-facing systems: Systematic review and meta-analysis. J. Med. Internet Res. 22:8839. doi: 10.2196/18839
Cooper, A., Kanumilli, N., Hill, J., Holt, R. I. G., Howarth, D., Lloyd, C. E., et al. (2018). Language matters. Addressing the use of language in the care of people with diabetes: position statement of the English Advisory Group. Diabet. Med. 35, 1630–1634. doi: 10.1111/dme.13705
Cradock, K. A., ÓLaighin, G., Finucane, F. M., Gainforth, H. L., Quinlan, L. R., and Ginis, K. A. M. (2017). Behaviour change techniques targeting both diet and physical activity in type 2 diabetes: a systematic review and meta-analysis. Int. J. Behav. Nutr. Phys. Act. 14, 18–17. doi: 10.1186/s12966-016-0436-0
Crouter, S. E., Schneider, P. L., and Bassett, D. R. (2005). Spring-levered versus piezo-electric pedometer accuracy in overweight and obese adults. Med. Sci. Sports Exerc. 37, 1673–1679. doi: 10.1249/01.mss.0000181677.36658.a8
Cui, M., Wu, X., Mao, J., Wang, X., and Nie, M. (2016). T2DM self-management via smartphone applications: A systematic review and meta-analysis. PLoS One 11, 1–15. doi: 10.1371/journal.pone.0166718
Dasgupta, K., Rosenberg, E., Joseph, L., Cooke, A. B., Trudeau, L., Bacon, S. L., et al. (2017). Physician step prescription and monitoring to improve ARTERial health (SMARTER): A randomized controlled trial in patients with type 2 diabetes and hypertension. Diabetes. Obes. Metab. 19, 695–704. doi: 10.1111/dom.12874
Dickinson, J. K., Guzman, S. J., Maryniuk, M. D., O’Brian, C. A., Kadohiro, J. K., Jackson, R. A., et al. (2017). The use of language in diabetes care and education. Diab. Care 40, 1790–1799. doi: 10.2337/dci17-0041
Encantado, J., Palmeira, A. L., Silva, C. C., Sniehotta, F., Stubbs, J., Gouveia, M. J., et al. (2021). What goes on in digital behaviour change interventions for weight loss maintenance targeting physical activity: a scoping review. PsyArXive. doi: 10.31234/osf.io/4rjpa
European Union (2020). Ageing Europe: looking at the lives of older people in the EU. Luxembourg: European Commission.
Félix, I. B., Guerreiro, M. P., Cavaco, A., Cláudio, A. P., Mendes, A., Balsa, J., et al. (2019). Development of a Complex Intervention to Improve Adherence to Antidiabetic Medication in Older People Using an Anthropomorphic Virtual Assistant Software. Front. Pharmacol. 10:680. doi: 10.3389/fphar.2019.00680
Fleming, G. A., Petrie, J. R., Bergenstal, R. M., Holl, R. W., Peters, A. L., and Heinemann, L. (2020). Diabetes digital app technology: benefits, challenges, and recommendations. A consensus report by the European Association for the Study of Diabetes (EASD) and the American Diabetes Association (ADA) Diabetes Technology Working Group. Diabetologia 63, 229–241. doi: 10.1007/s00125-019-05034-1
Gardner, A. W., Montgomery, P. S., Wang, M., Shen, B., Casanegra, A. I., Silva-Palacios, F., et al. (2021). Daily step counts in participants with and without peripheral artery disease. J. Cardiopulm. Rehabil. Prev. 41, 182–187. doi: 10.1097/HCR.0000000000000554
Gillison, F. B., Rouse, P., Standage, M., Sebire, S. J., and Ryan, R. M. (2019). A meta-analysis of techniques to promote motivation for health behaviour change from a self-determination theory perspective. Health Psychol. Rev. 13, 110–130. doi: 10.1080/17437199.2018.1534071
Gong, E., Baptista, S., Russell, A., Scuffham, P., Riddell, M., Speight, J., et al. (2020). My diabetes coach, a mobile app⇓based interactive conversational agent to support type 2 diabetes self-management: randomized effectiveness-implementation trial. J. Med. Internet Res. 22, 1–14. doi: 10.2196/20322
Gourlan, M., Bernard, P., Bortolon, C., Romain, A. J., Lareyre, O., Carayol, M., et al. (2016). Efficacy of theory-based interventions to promote physical activity. A meta-analysis of randomised controlled trials. Health Psychol. Rev. 10, 50–66. doi: 10.1080/17437199.2014.981777
Grant, P. M., Dall, P. M., Mitchell, S. L., and Granat, M. H. (2008). Activity-monitor accuracy in measuring step number and cadence in community-dwelling older adults. J. Aging Phys. Act. 16, 201–214. doi: 10.1123/japa.16.2.201
Guerreiro, M. P., Angelini, L., Henriques, H. R., Kamali, M.El, Baixinho, C., Balsa, J., et al. (2021). Conversational agents for health and well-being across the life course: Protocol for an evidence map. JMIR Res. Protoc. 10, 1–11. doi: 10.2196/26680
Guerreiro, M. P., Félix, I. B., Balsa, J., Carmo, M. B., Henriques, M. A., Cavaco, A., et al. (2020). “Contribution of an Intelligent Virtual Assistant to Healthy Ageing in Adults With Type 2 Diabetes,” in Exploring the Role of ICTs in Healthy Aging. eds. D. Mendes, C. Fonseca, M. J. Lopes, J. García-Alonso, and J. M. Murillo (Hershey, Pennsylvania: IGI Global), 194–230.
Hall, K. S., Hyde, E. T., Bassett, D. R., Carlson, S. A., Carnethon, M. R., Ekelund, U., et al. (2020). Systematic review of the prospective association of daily step counts with risk of mortality, cardiovascular disease, and dysglycemia. Int. J. Behav. Nutr. Phys. Act. 17, 1–14. doi: 10.1186/s12966-020-00978-9
Holt, R. I. G., and Speight, J. (2017). The language of diabetes: the good, the bad and the ugly. Diabet. Med. 34, 1495–1497. doi: 10.1111/dme.13520
ICHOM (2019). Type 1 Diabetes and Type 2 Diabetes in adults. Data collection reference guide. Available at: www.ichom.org/medical-conditions/diabetes (Accessed September 29, 2021).
Idowu, O. A., Adeniyi, A. F., Edo, A., and Fasanmade, A. (2021). Graded activity with and without daily-monitored-walking in patients with type 2 diabetes with low back pain: secondary analysis of a randomized-clinical trial. Arch. Physiother. 11, 1–11. doi: 10.1186/s40945-021-00104-3
International Diabetes Federation (2021). IDF Diabetes Atlas. 10th Edn. Available at: www.diabetesatlas.org (Accessed January 20, 2022).
Jones, A., Vallis, M., and Pouwer, F. (2015). If it does not significantly change HbA1c levels why should we waste time on it? A plea for the prioritization of psychological well-being in people with diabetes. Diabet. Med. 32, 155–163. doi: 10.1111/dme.12620
Kanaley, J. A., Colberg, S. R., Corcoran, M. H., Malin, S. K., Rodriguez, N. R., Crespo, C. J., et al. (2022). Exercise/Physical activity in individuals with type 2 diabetes: a consensus statement from the American College of Sports Medicine. Med. Sci. Sport. Exerc. 54, 353–368. doi: 10.1249/MSS.0000000000002800
Keller, R., Hartmann, S., Teepe, G. W., Lohse, K.-M., Alattas, A., Tudor Car, L., et al. (2022). Digital Behavior Change Interventions for the Prevention and Management of Type 2 Diabetes: Systematic Market Analysis. J. Med. Internet Res. 24:e33348. doi: 10.2196/33348
Konerding, U., and Szel, C. (2021). Promoting physical activity in persons with type 2 diabetes mellitus: A systematic review of systematic reviews. Patient Educ. Couns. 104, 1600–1607. doi: 10.1016/j.pec.2020.12.011
Koponen, A. M., Simonsen, N., and Suominen, S. (2018). Success in increasing physical activity (PA) among patients with type 2 diabetes: A self-determination theory perspective. Heal. Psychol. Behav. Med. 6, 104–119. doi: 10.1080/21642850.2018.1462707
Kreuter, M., Farrell, D., Olevitch, L., and Brennan, L. (2000). Tailoring Health Messages: Customizing Communication with Computer Technology. Mahwah New Jersey: Lawrence Erlbaum Associates.
Little, R. R., and Rohlfing, C. L. (2013). The long and winding road to optimal HbA1c measurement. Clin. Chim. Acta 418, 63–71. doi: 10.1016/j.cca.2012.12.026
Luo, T. C., Aguilera, A., Lyles, C. R., and Figueroa, C. A. (2021). Promoting physical activity through conversational agents: Mixed methods systematic review. J. Med. Internet Res. 23, 1–17. doi: 10.2196/25486
Marques, M. M., Carey, R. N., Norris, E., Evans, F., Finnerty, A. N., Hastings, J., et al. (2020). Delivering Behaviour Change Interventions: Development of a Mode of Delivery Ontology. Wellcome Open Res. 5:125. doi: 10.12688/wellcomeopenres.15906.1
Mathews, E., Lakshmi, J. K., Ravindran, T. K., Pratt, M., and Thankappan, K. R. (2016). Perceptions of barriers and facilitators in physical activity participation among women in Thiruvananthapuram City, India. Global health promotion 23, 27–36. doi: 10.1177/1757975915573878
McAuley, A. (2014). Digital health interventions: Widening access or widening inequalities? Public Health 128, 1118–1120. doi: 10.1016/j.puhe.2014.10.008
Michie, S., and Abraham, C. (2004). Intervention to change health beahviour: evidence-based or evidence-inspired? Psychol. Heal. 19:29. doi: 10.1080/0887044031000141199
Michie, S., Atkins, L., and West, R. (2014). The Behavior Change Wheel: A Guide To Designing Interventions. First edit. London: Silverback Publishing.
Michie, S., Richardson, M., Johnston, M., Abraham, C., Francis, J., Hardeman, W., et al. (2013). The behavior change technique taxonomy (v1) of 93 hierarchically clustered techniques: Building an international consensus for the reporting of behavior change interventions. Ann. Behav. Med. 46, 81–95. doi: 10.1007/s12160-013-9486-6
Michie, S., Yardley, L., West, R., Patrick, K., and Greaves, F. (2017). Developing and evaluating digital interventions to promote behavior change in health and health care: Recommendations resulting from an international workshop. J. Med. Internet Res. 19:7126. doi: 10.2196/jmir.7126
Moghetti, P., Balducci, S., Guidetti, L., Mazzuca, P., Rossi, E., and Schena, F. (2020). Walking for subjects with type 2 diabetes: a systematic review and joint AMD/SID/SISMES evidence-based practical guideline. Nutr. Metab. Cardiovasc. Dis. 30, 1882–1898. doi: 10.1016/j.numecd.2020.08.021
Moutão, J. M. R. P., Serra, L. F. C., Alves, J. A. M., Leitão, J. C., and Vlachopoulos, S. P. (2012). Validation of the Basic Psychological Needs in Exercise Scale in a Portuguese Sample. Span. J. Psychol. 15, 399–409. doi: 10.5209/rev_SJOP.2012.v15.n1.37346Copyright
Nahum-Shani, I., Smith, S. N., Spring, B. J., Collins, L. M., Witkiewitz, K., Tewari, A., et al. (2018). Just-in-time adaptive interventions (JITAIs) in mobile health: Key components and design principles for ongoing health behavior support. Ann. Behav. Med. 52, 446–462. doi: 10.1007/s12160-016-9830-8
National Institute for Health and Care Excellence (2014). NICE Guidance: Behaviour change: individual approaches. Available at: https://www.nice.org.uk/guidance/ph49 (Accessed January 4, 2022).
Niess, J., and Diefenbach, S. (2016). Communication Styles of Interactive Tools for Self-Improvement. Psychol. Well. Being. 6:3. doi: 10.1186/s13612-016-0040-8
Ntoumanis, N., Ng, J. Y. Y., Prestwich, A., Quested, E., Hancox, J. E., Thøgersen-Ntoumani, C., et al. (2021). A meta-analysis of self-determination theory-informed intervention studies in the health domain: effects on motivation, health behavior, physical, and psychological health. Health Psychol. Rev. 15, 214–244. doi: 10.1080/17437199.2020.1718529
O’Cathain, A., Croot, L., Duncan, E., Rousseau, N., Sworn, K., Turner, K. M., et al. (2019). Guidance on how to develop complex interventions to improve health and healthcare. BMJ Open 9:e029954. doi: 10.1136/bmjopen-2019-029954
Pedersen, B. K., and Saltin, B. (2015). Exercise as medicine - Evidence for prescribing exercise as therapy in 26 different chronic diseases. Scand. J. Med. Sci. Sport. 25, 1–72. doi: 10.1111/sms.12581
Rinaldi, G., Hijazi, A., and Haghparast-Bidgoli, H. (2020). Cost and cost-effectiveness of mHealth interventions for the prevention and control of type 2 diabetes mellitus: A systematic review. Diabetes Res. Clin. Pract. 162:108084. doi: 10.1016/j.diabres.2020.108084
Rossen, J., Hagströmer, M., Yngve, A., Brismar, K., Ainsworth, B., and Johansson, U. B. (2021). Process evaluation of the Sophia Step Study- a primary care based three-armed randomized controlled trial using self- monitoring of steps with and without counseling in prediabetes and type 2 diabetes. BMC Public Health 21, 1–11. doi: 10.1186/s12889-021-11222-9
Ryan, R. M., and Deci, E. L. (2000). Self-determination theory and the facilitation of intrinsic motivation, social development, and well-being. Am. Psychol. 55, 68–78. doi: 10.1037/0003-066X.55.1.68
Ryan, R., and Deci, E. L. (2017). Self-Determination Theory: Basic Psychological Needs in Motivation, Development, and Wellness. New York: Guilford publications.
Saad, M. F., Cheah, W. L., and Hazmi, H. (2021). The effects of a 7000-step goal and weekly group walking program for overweight and obese elderly people in sarawak, malaysia: A quasi-experimental study. J. Prev. Med. Public Heal. 54, 199–207. doi: 10.3961/jpmph.20.584
Shah, S. Z. A., Karam, J. A., Zeb, A., Ullah, R., Shah, A., Haq, I. U., et al. (2021). Movement is Improvement: The Therapeutic Effects of Exercise and General Physical Activity on Glycemic Control in Patients with Type 2 Diabetes Mellitus: A Systematic Review and Meta-Analysis of Randomized Controlled Trials. Diabetes Ther. 12, 707–732. doi: 10.1007/s13300-021-01005-1
Skinner, T. C., Joensen, L., and Parkin, T. (2020). Twenty-five years of diabetes distress research. Diabet. Med. 37, 393–400. doi: 10.1111/dme.14157
Skivington, K., Matthews, L., Simpson, S. A., Craig, P., Baird, J., Blazeby, J. M., et al. (2021). A new framework for developing and evaluating complex interventions: update of medical research council guidance. The BMJ 374:n2061. doi: 10.1136/bmj.n2061
Smith, T. C., Wingard, D. L., Smith, B., Kritz-Silverstein, D., and Barrett-Connor, E. (2007). Walking Provides Strong Protection from Cardiovascular Disease Mortality in Older Adults with Diabetes. J Clin Epidemiol 60, 309–317. doi: 10.1016/j.jclinepi.2006.06.013
Speight, J., Skinner, T. C., Dunning, T., Black, T., Kilov, G., Lee, C., et al. (2021). Our language matters: Improving communication with and about people with diabetes. A position statement by Diabetes Australia. Diabetes Res. Clin. Pract. 173:108655. doi: 10.1016/j.diabres.2021.108655
Tedesco, S., Sica, M., Ancillao, A., Timmons, S., Barton, J., and O’Flynn, B. (2019). Validity evaluation of the fitbit charge2 and the garmin vivosmart HR+ in free-living environments in an older adult cohort. JMIR 7, 1–15. doi: 10.2196/13084
Teixeira, P. J., Carraça, E. V., Markland, D., Silva, M. N., and Ryan, R. M. (2012). Exercise, physical activity, and self-determination theory: A systematic review. Int. J. Behav. Nutr. Phys. Act. 9:78. doi: 10.1186/1479-5868-9-78
Tudor-Locke, C., Camhi, S. M., Leonardi, C., Johnson, W. D., Katzmarzyk, P. T., Earnest, C. P., et al. (2011a). Patterns of adult stepping cadence in the 2005–2006 NHANES. Prev. Med. 53, 178–181. doi: 10.1016/j.ypmed.2011.06.004
Tudor-Locke, C., Craig, C. L., Aoyagi, Y., Bell, R. C., Croteau, K. A., De Bourdeaudhuij, I., et al. (2011b). How many steps/day are enough? For older adults and special populations. Int. J. Behav. Nutr. Phys. Act. 8, 1–19. doi: 10.1186/1479-5868-8-80
Tudor-Locke, C., Leonardi, C., Johnson, W. D., Katzmarzyk, P. T., and Church, T. S. (2011c). Accelerometer steps/day translation of moderate-to-vigorous activity. Prev. Med. 53, 31–33. doi: 10.1016/j.ypmed.2011.01.014
Tudor-Locke, C., and Rowe, D. A. (2012). Using cadence to study free-living ambulatory behaviour. Sport. Med 42, 381–398. doi: 10.2165/11599170-000000000-00000
Van Dyck, D., De Greef, K., Deforche, B., Ruige, J., Bouckaert, J., Tudor-Locke, C. E., et al. (2013). The relationship between changes in steps/day and health outcomes after a pedometer-based physical activity intervention with telephone support in type 2 diabetes patients. Health Educ. Res. 28, 539–545. doi: 10.1093/her/cyt038
Keywords: conversational agent, older adults, type 2 diabetes, physical activity, intervention development, behavior change techniques, self-determination theory
Citation: Pimenta N, Félix IB, Monteiro D, Marques MM and Guerreiro MP (2022) Promoting Physical Activity in Older Adults With Type 2 Diabetes via an Anthropomorphic Conversational Agent: Development of an Evidence and Theory-Based Multi-Behavior Intervention. Front. Psychol. 13:883354. doi: 10.3389/fpsyg.2022.883354
Edited by:
Pedro Sousa, Coimbra Nursing School, PortugalReviewed by:
Shuichi Nishio, Osaka University, JapanSathish Thirunavukkarasu, Emory University, United States
Copyright © 2022 Pimenta, Félix, Monteiro, Marques and Guerreiro. This is an open-access article distributed under the terms of the Creative Commons Attribution License (CC BY). The use, distribution or reproduction in other forums is permitted, provided the original author(s) and the copyright owner(s) are credited and that the original publication in this journal is cited, in accordance with accepted academic practice. No use, distribution or reproduction is permitted which does not comply with these terms.
*Correspondence: Mara Pereira Guerreiro, bWd1ZXJyZWlyb0BlZ2FzbW9uaXouZWR1LnB0
†These authors have contributed equally to this work and share first authorship