- 1Faculty of Psychology, Tianjin Normal University, Tianjin, China
- 2School of Psychology, Beijing Normal University, Beijing, China
- 3Key Research Base of Humanities and Social Sciences of the Ministry of Education, Academy of Psychology and Behavior, Tianjin Normal University, Tianjin, China
- 4Tianjin Social Science Laboratory of Students’ Mental Development and Learning, Tianjin, China
The great number of mobile phone users in the world has increased in recent years. More time spent on a phone, more negative effects such as problematic mobile phone use. Many researchers have devoted themselves to revise tools to measure problematic mobile phone use better and more precisely. Previous studies have shown that these tools have good reliability and validity, but that most of them have some shortcomings because they were traditional paper-and-pencil tests based on Classical Test Theory (CTT). This study, based on Item Response Theory (IRT) in order to solve these shortcomings, developed Computerized Adaptive Test for problematic mobile phone use (CAT-PMPU) and discussed the performance of CAT-PMPU. Then, we used real data to simulate CAT, and the measurement accuracy and reliability between a paper-and-pencil test and CAT-PMPU were compared under the same test length. The results showed that CAT-PMPU was better than the paper-and-pencil test in all aspects, and that it can reduce the number of items and improve measurement efficiency effectively. In conclusion, the CAT-PMPU was developed in this study has good reliability, and it provided novel technical support for the measurement of problematic mobile phone use. It had a good application prospect.
Introduction
There were 5.29 billion mobile phone users worldwide in 2021, an increase of 100 million compared with 2020 according to Digital Reports (2021). Peking University Post-95 Mobile Phone Use Psychological and Behavioral White Paper reported that individuals spend more than 8.33 h per day on their phone (Wang et al., 2019). Mobile phone use brought convenience to people’s social communication (Gul et al., 2018) and leisurely experience (Lee et al., 2014; Ra et al., 2018). However excessive mobile phone use also led to many negative consequences such as sleep disorders (Acharya et al., 2013), distress (Chóliz, 2010), and mental health problems (Gezgin and Çakir, 2016; Gezgin, 2017). At present, there are more and more research studies on excessive mobile phone use and mental health.
Different concepts about excessive mobile phone use were put forward in different studies. Toda et al. (2008) defined it as “mobile phone dependence,” a more common term was mobile phone addiction (Chóliz, 2010), and another definition was smartphone addiction (Su et al., 2014). Shotton (1989) and Griffiths (1998) believed that excessive use of technology should be characterized as problematic use rather than addiction. Later, researchers also argued whether mobile phone addiction should be fully considered as an addictive behavior (Bianchi and Phillips, 2005; Tossell et al., 2015). Recently, problematic mobile phone use (PMPU) was widely used in studies (Hao and Jin, 2020; Li et al., 2020). PMPU refers to an uncontrolled and excessive mobile phone use that adversely affects individual’s daily life (Billieux, 2012). PMPU was defined from both psychological and physiological aspects as inappropriate use of mobile phone that would affect personal life and study, and show withdrawal symptoms in the Chinese culture (Xiong et al., 2012).
A review on the prevalence of PMPU in China showed that the prevalence of PMPU ranged from 14 to 31.2% among children and from 27.6 to 29.8% among young people, indicating higher risk of PMPU among young people in China (Lai et al., 2020). Many risk factors of PMPU were revealed, such as residence, depressive symptoms, social anxiety, loneliness, academic pressure, professional interests, family conflicts, neuroticism, openness, and solitude behaviors (Liu et al., 2020; Lu et al., 2021, 2022; Wang and Shi, 2021). Cognitive and executive functions (e.g., attention function and response inhibition function) were proved to be affected if individuals had PMPU (Zhou et al., 2015).
A variety of instruments with good reliability and validity has been developed to measure PMPU. Xiong et al. (2012) developed the Mobile Phone Addiction Tendency Scale (MPATS) to measure improper use of mobile phones. Su et al. (2014) developed the Smartphone Addiction Scale for College Students (SAS-C) to measure the psychological and behavioral performance caused by smartphone abuse. Chen et al. (2017) developed the Smartphone Addiction Scale for Chinese Adults (SAS-CA), which was adapted from college students to adults for assessing PMPU. Basu et al. (2021) developed the Problematic Use of Smartphone Questionnaire (PSUQ) for adults in India. Billieux et al. (2008) developed the Problematic Mobile Phone Use Questionnaire (PMPUQ); subsequently, the short version of PMPUQ showed measurement invariance of various language versions (i.e., French, German, and Hungarian), and could be used in cross-culture research (Olatz et al., 2018).
Most of these PMPU instruments were developed based on symptomology. In other words, each dimension of these PMPU instruments described a typical symptom of PMPU in individuals. MPATS contained four dimensions, withdrawal symptoms, salience, social, comfort, and mood changes. The SAS-C was developed based on the diagnostic criteria of internet addiction proposed by Young (1998). It contained four symptoms, withdrawal behavior, salience behavior, social comfort, and negative effects. The Chinese version of SAS-CA also had four subscales, withdrawal, salience, social impairment, and somatic discomfort. The Smartphone Addiction Proneness Scale (SAPS) and Smartphone Addiction Inventory (SPAI) were developed based on the existing internet addiction literature (Kim et al., 2014; Lin et al., 2014). The SAPS had four subscales, including disturbance of adaptive functions, virtual life orientation, withdrawal, and tolerance. The SPAI had four subscales, compulsive behavior, functional impairment, withdrawal, and tolerance. The PSUQ had five subscales, dependence, impaired control, denial, decreased productivity, and emotional attachment (Basu et al., 2021). The PMPUQ had four subscales, prohibited use, dangerous use, dependence, and financial problems (Billieux et al., 2008).
Generally, the logic for all the PMPU scales above is that the more symptoms an individual has, the higher the PMPU level he is at. The level of PMPU was indicated by the sum of scores of a scale. Besides, there was some overlap in existing instruments. For example, there are two same dimensions in the MPATS, SAS-C, and SAS-CA, namely, withdrawal and prominent behaviors. Both the SAPS and the SPAI have withdrawal and tolerance dimensions. Moreover, no matter what dimensions a PMPU scale has, the key purpose is to accurately assess the level of PMPU.
In addition, all existing PMPU instruments are traditional paper-and-pencil (P and P) tests based on Classical Test Theory (CTT). In this case, a large number of items are necessary to ensure the accuracy of assessment, and participants should answer all items. Consequently, excessive items would increase the cognitive burden of participants. Also, a long test would lead to boredom and reduce the validity of a test (Lin et al., 2014). Besides, CTT has limitations, such as the estimation of ability being dependent on properties of items and the estimation of item parameters being dependent on participants. Accordingly, two sets of measurement results based on different participants using the same instrument were not comparable (Sun and Guan, 2009). In short, the traditional test is not the optimal way to assess individuals when the instrument is too long and participants are not homogeneous.
To sum up, the existing PMPU measures were all traditional paper-and-pencil tests, which were not convenient to conduct as a large-scale investigation. These PMPU measures were developed in different contexts, although most PMPU measures illustrated a series of same or similar symptoms and shared a common purpose, which was to assess individuals’ PMPU levels.
With the development of Item Response Theory (IRT), computerized adaptive testing (CAT) could provide an optimal solution to psychological assessments with long-scale and heterogeneous samples. Embretson (1996) suggested that CAT was more suitable for measurement of various types of psychological assessment. CAT mainly emphasized that the test was performed by individuals, matched items perfectly with individuals’ trait levels, so it would get the most effective assessment results with a smaller number of items (Weiss, 1982). Many CATs for non-cognitive tests or typical performance tests (e.g., personality tests, mental health tests, emotion regulation tests, subjective well-being tests, self-esteem tests, and social responsibility tests) were already developed (Forbey and Ben-Porath, 2007; Fritts and Marszalek, 2010; Nieto et al., 2018; Wu et al., 2019; Zheng and Cai, 2019; Dai et al., 2020; Xu et al., 2020). Herwin et al. (2022) designed a mobile CAT assessment tool and found that students’ learning motivation could be significantly improved after using the mobile assessment tool, and Miyasawa and Ueno (2013), based on the CAT combined with TTP, designed a mobile testing tool for authentic assessment on the basis of saving time and effort to improve measurement accuracy.
At present, the number of mobile phone users is enormous around the world. Considering the adverse effects of PMPU on mental health, a large-scale instrument for PMPU assessment is necessarily needed (Fumero et al., 2018). Developing a CAT-PMPU (computerized adaptive testing for problematic mobile phone use) tool can provide great convenience for accurate and rapid assessment of PMPU.
The purpose of this study was to develop a CAT-PMPU tool with good psychometric properties. In the first step of this study, a total of 98 items of seven scales were used to develop a PMPU item bank based on IRT. Unidimensionality tests, item calibrations, and differential item functioning analyses were conducted. In the second step, empirical data were used to compare the traditional P and P PMPU tests and the new CAT-PMPU.
Materials and Methods
Participants
We recruited 980 participants to complete online or paper-pencil questionnaires. The participants were college students and graduate students from Tianjin, China. Convenience sampling was conducted. The measurement invariance was supported across the paper-pencil questionnaire and the online questionnaire by multiple-group confirmatory factor analysis, so two data sets were analyzed as one. Ninety-five participants were excluded because of not answering more than one item. Finally, a total of 885 valid data were retained, including 318 men and 567 women, and their age ranged from 19 to 25 (M = 20.67, SD = 2.64).
Instruments
All scales that are published on journals that were indexed in the Chinese Social Sciences Citation Index (CSSCI), Bei Da He Xin, and Chinese Science Citation Database (CSCD) from the CNKI database in recent 3 years were taken into consideration. The short versions of scales were excluded, since their items were from original versions. Translated versions of the scales that were used in applied studies were excluded, because the development or refinement procedures were not clear and the quality of scale could not be ensured. Finally, seven Chinese version scales of PMPU were included in this study.
Chinese Version of the Nomophobia Questionnaire
The Chinese version of the Nomophobia Questionnaire based on the original Nomophobia Questionnaire of Yildirim and Correia (2015) and Ren et al. (2020) revised the Chinese version of Nomophobia Questionnaire (NMP-C) using an exploratory structure equation model (ESEM) and a polytomous item response model. The NMP-C contained 16 items and four dimensions, fear of being unable to access information, losing convenience, losing contact, and losing Internet connection. The NMP-C used a 7-point Likert scale, ranging from 1 (“Not meet at all”) to 7 (“Completely in conformity with”) and had good reliability and validity. Cronbach’s α for the whole scale was 0.948, and for the four dimensions it ranged from 0.867 to 0.916 (Ren et al., 2020). In this study, Cronbach’s α for the whole scale was 0.954.
Smartphone Addiction Proneness Scale
The Smartphone Addiction Proneness Scale was a 4-point Likert scale, ranging from 1 (“Strongly disagree”) to 4 (“Strongly agree”), and contained 10 items and four dimensions, disturbance of adaptive functions, virtual life orientation, withdrawal, and tolerance. It had good reliability and validity in empirical studies (Kim et al., 2014). Cronbach’s α for the whole scale was 0.884, and construct validity for the whole scale was great (CFI = 0.962, TLI = 0.902, NFI = 0.943, RMSEA = 0.034). The correlation result between the SAPS and the Mental Health Problems Scale showed that the SAPS has good criterion validity. In this study, Cronbach’s α for the whole scale was 0.838.
Smartphone Addiction Inventory
The Smartphone Addiction Inventory was a 4-point Likert scale, ranging from 1 (“Strongly disagree”) to 4 (“Strongly agree”), and contained 20 items and four dimensions, compulsive behavior, functional impairment, withdrawal, and tolerance. Lin et al. (2014) demonstrated it had good reliability and validity. Cronbach’s α for the whole scale was 0.94, and for the four dimensions it ranged from 0.72 to 0.88. The 2-week test–retest reliability of the four subscales ranged from 0.8 to 0.91, and the correlation results among the four subscales of SPAI and the phantom vibration ranged from 0.56 to 0.78, which meant that there was a moderate/high inter-factor correlation (Lin et al., 2014). In this study, Cronbach’s α for the whole scale was 0.926.
Mobile Phone Addiction Scale
The Mobile Phone Addiction Scale was a 5-point Likert scale, ranging from 1 (“Never”) to 5 (“Always”), and contained 11 items and four dimensions, inability to control craving, feeling anxious and lost, withdrawal and escape, and productivity loss. It had good reliability and validity. Cronbach’s α for the whole scale was 0.9, and the MPAS was correlated mostly in a hypothesized manner with measures of psychologically meaningful constructs such as leisure, boredom, and sensation-seeking (Leung, 2008). In this study, Cronbach’s α for the whole scale was 0.877.
Mobile Phone Addiction Tendency Scale
The Mobile Phone Addiction Tendency Scale was developed by Xiong et al. (2012). It was a 5-point Likert scale, ranging from 1 (“Very inconsistent”) to 5 (“Very well suited to”). It was composed of 16 items and four factors, withdrawal symptoms, salience, social comfort, and mood changes. Previous studies found this scale reliable and valid. Cronbach’s α of the whole scale was 0.83, and for the four dimensions it ranged from 0.81 to 0.92. The result of the confirmatory factor analysis showed that the four-factor model had good fitting indices (CFI = 0.96, RMSEA = 0.07, NFI = 0.94, IFI = 0.96, RFI = 0.93). In addition, researchers have used the MPATS in other studies and proved its high construct validity (CFI = 0.92, TLI = 0.94, RMSEA = 0.07, IFI = 0.96) (Jiang and Bai, 2014). In this study, Cronbach’s α for the whole scale was 0.911.
Smartphone Addiction Scale for College Students
The Smartphone Addiction Scale for College Students was a 5-point Likert scale, ranging from 1 (“Strongly unacceptable”) to 5 (“Strongly acceptable”), and contained 11 items and four dimensions, withdrawal behavior, salience behavior, social comfort, and negative effects. Cronbach’ s α for the whole scale was 0.93, and for each factor it ranged from 0.5 to 0.85; the test–rest coefficients were 0.93 for the whole scale and 0.72–0.82 for the four factors. It had great construct validity (CFI = 0.92, TLI = 0.9, RMSEA = 0.05, SRMR < 0.001) (Su et al., 2014). In this study, Cronbach’s α for the whole scale was 0.902.
Smartphone Addiction Scale for Chinese Adults
The Smartphone Addiction Scale for Chinese Adults was a 5-point Likert scale, ranging from 1 (“Strongly unacceptable”) to 5 (“Strongly acceptable”), and contained 14 items and four dimensions, withdrawal, salience, social impairment, and somatic discomfort. Cronbach’ s α for the whole scale was 0.909, and for each factor it ranged from 0.714 to 0.814; the test-rest coefficients were 0.931 for the whole scale and 0.743–0.85 for the four factors. It had satisfying structure validity (CFI = 0.94, RMSEA = 0.043, SRMR < 0.001) (Chen et al., 2017). In this study, Cronbach’s α for the whole scale was 0.929.
Item Response Theory Models
All the PMPU scales in this study were polytomous. Some frequently employed multi-index IRT models for polytomous data involve the graded response model (GRM; Samejima, 1969) and the generalized partial credit model (GPCM; Muraki, 1992). Their item response functions were described as follows.
where P(Xij≥t) referred to the probability that the subject i will score t or more on item j.
where αj was the discrimination parameter of the j-th item, bjt was the difficulty parameter of the subject getting t points on item j, and θi was the ability of the subject i.
where P(Xij = t) represented the probability of subject i to score t on item j, mj was the sum score of item j, t was the subject’s score on this item, δjv was intersections of two curves of P(Xij = t) and P(Xij = t−1), and it was the only intersection. It may fall anywhere in the θ scale. αj is the discrimination parameter of item j.
Indices
Item Information
Item information is a common concept in IRT. It refers to the information that an item could provide for evaluating participants. More information indicates higher reliability of measurement. In this study, the item selection strategy was the maximum information (MI) method, one of the most widely used in most research studies. The specific content was to select the item with largest information for subjects to answer.
Measurement standard error was inversely proportional to the square of test information, and the formula was as follows:
where θ was the level of psychological traits. In this study, subjects’ ability was estimated with the expected a posteriori (EAP) method, m was the total number of items, and Ii(θ) was the information provided by item i to subject with psychological trait level θ.
Some researchers have proposed marginal reliability (MR) based on the test information in IRT, which was easy to use and dynamically monitored the reliability of CAT (Green et al., 1984). The formula is as follows:
where N was the total number of subjects, i was the specific subjects, and SE(θi) was the measurement standard error of subject i at the final estimated θ.
The MR in IRT was the overall reliability of the test calculated by the average measurement error for all subjects rather than CTT’s reliability based on the original score of the test.
Stop Rules
The CAT had two stop rules: fixed length and variable length (fixed measurement accuracy) stop rules. In this study, the stop rule of fixed measurement accuracy was adopted, and a total of seven measurement accuracies (SE = 0.2/0.3/0.4/0.5/0.6/0.7/0.8) were set. We also designed a stop rule called “all”; that is, participants would terminate the CAT after completing all items in the item bank. Results under the “all” stop rule could be compared with the results under seven stop rules, and then we verified the measurement effectiveness of CAT. To prove the effectiveness and advantage of CAT-PMPU, we also calculated the measurement error of seven traditional P and P PMPU tests and then took them as the stop rule for CAT-PMPU (SE = 0.34/0.39/0.55/0.41/0.43/0.36/0.38).
Software
SPSS24.0 was used for data preprocessing, principal component analysis (PCA), and unidimensionality test in this study. The R mirt package was used to fit the IRT model and calibrate items, the lordif package was used to test differential item functioning, and the catR package was used to conduct simulation.
Results
Unidimensionality Test
We deleted some items with poor relevant to problematic mobile phone use in order to ensure the quality of the item bank. First, the loading of each item on the first principal component was obtained by PCA, and items whose loading was lower than 0.4 on first principal component was deleted. Therefore, five items were deleted at this step, three of them were from the SAPS, and the other items were from the SPAI and the MPAS. For example, the content of the MPAS_2 was “I received a mobile bill that I couldn’t afford.” This item was not related to problematic mobile phone use. The factor loading of all items is shown in Figure 1.
Item Response Theory models assumed that the measurement characteristics were unidimensional (Hambleton et al., 1991), so we conducted a unidimensionality test on 93 items. Previous studies indicated that the data could be considered to meet unidimensionality if the ratio of first and second eigenvalues was more than 4 and the variance ratio of the first factor was more than 20% in exploratory factor analysis (EFA) (Reckase, 1979; Andrich, 1996; Reeve et al., 2007). Therefore, we conducted an EFA on the 93 items, and the Kaiser–Meyer–Ollkin (KMO) test result was 0.977, which meant that the 93 items were suitable for factor analysis. Then, the results of the EFA showed that the factor loading of all items was greater than 0.4, the first eigenvalue was 35.631, the second eigenvalue was 6.107, the ratio of the first and second eigenvalues was 5.83, and variance of the first factor accounted for 38.312%, suggesting that the 93 items met unidimensionality.
Item Response Theory Model Selection
This step compared the model fitting according to fitting indicators such as AIC (Akaike, 1974), BIC (Schwarz, 1978), choosing a model that was relatively more fitting to the data between GPCM and GRM. The smaller the AIC and BIC, the better the model fit.
The specific results are shown in Table 1. Of the two models, the GRM fitted the remaining items best, as it had smaller AIC and BIC values. So GRM was employed to analyze the final CAT-PMPU item bank.
Differential Item Functioning Analysis
In order to ensure the quality of items in our item bank, item discrimination analysis and differential item functioning (DIF) analysis were carried out in our study.
Item discrimination referred to the extent in which the item differentiates the actual level of subjects. In our study, items with discrimination less than 0.8 were deleted (Fliege et al., 2005). Four items were removed from item bank, namely, MPATS_1, MPATS_3, SPAI_6, and MPAS_1. The detailed results are shown in Table 2.
Differential item functioning was conducted to determine systematic differences caused by demographic variables such as gender and age (Gaynes et al., 2002). Logistic regression was applied to test DIF. Change in McFadden’s pseudo R2 was employed to test the effect size, the hypothesis of no DIF was rejected when R2> 0.02, and such items were excluded (Choi et al., 2011). The results showed that none of items’ R2 values were higher than 0.02. Therefore, there was no DIF according to gender for 89 items. Finally, the 89 items were retained and reanalyzed. The results showed that these items satisfied unidimensionality with no DIF and great discrimination.
Quality of Item Bank
The discrimination parameters of the remaining 89 items were all > 0.8 with mean of 1.26 (SD = 0.31) and from 0.87 to 2.36 with a difference of 1.49, indicating a high-quality item bank (Figure 2). Besides, the difficulty parameters of the items in the item bank were moderate. The specific results of some items are shown in Table 3 below.
This study also calculated the information and marginal reliability of the item bank. The specific results are shown in Figures 3, 4.
It could be seen that the average marginal reliability of the item bank was as high as 0.97, and that the information provided by the item bank was relatively sufficient. It provided high information to subjects whose problematic mobile phone use was at a moderate or upper level and low information to subjects whose problematic mobile phone use was at a low-down level. It showed that the item bank had very high measurement accuracy and reliability for most of the participants. In summary, the quality of the item bank was high and ideal.
Evaluating Computerized Adaptive Test for Problematic Mobile Phone Use Under Different Stop Rule Conditions
In this study, the specific steps of CAT simulation were as follows: we used the real data obtained by the participants in the P and P test to simulate the CAT; we used the maximum information (MI) as item selection strategy and expected a posteriori (EAP) as participants’ ability estimation method, and seven stop rules (SE = 0.2/0.3/0.4/0.5/0.6/0.7/0.8) were set.
Table 4 shows the simulation results of real data under several stop rules. It could be seen that under the stop rule of SE = 0.5, the correlation coefficient between the ability estimated using 4.07 items and the ability estimated using the entire item bank was as high as 0.93 (n = 885, p < 0.01), but its marginal reliability was only 0.77, which was not ideal. Under the stop rule of SE = 0.4, the correlation coefficient between the ability estimated using 6.93 items and the ability estimated using the entire item bank was as high as 0.95 (n = 885, p < 0.001), and the measurement reliability was as high as 0.85, so it was recommended to use this stop rule for real CAT. If higher marginal reliability of CAT-PMPU is wanted, the stop rule of SE = 0.3 can be used. The correlation coefficient between the ability estimated using 14.27 items and the ability estimated using the entire item bank was as high as 0.97 (n = 885, p < 0.001), and the marginal reliability reached 0.91, but the number of items used would slightly increase.
Comparison of Computerized Adaptive Test for Problematic Mobile Phone Use and Paper-and-Pencil Problematic Mobile Phone Use Tests
This part mainly explored how the accuracy and reliability of CAT-PMPU measurements were improved compared with P and P tests. Measurement accuracy and reliability were compared when the test length of CAT-PMPU was fixed to the same length as the traditional P and P PMPU test. For example, NMP contained 16 items; the measurement error and marginal reliability could be calculated when NMP was used alone to estimate subject’s ability. At the same time, a fixed-length stop rule (16 items) could be used in CAT-PMPU, and the corresponding measurement error and marginal reliability could be calculated. Then, the measurement accuracy and reliability of traditional P and P PMPU test improved by CAT-PMPU could be compared under the same test length.
Measurement Error of Two Test Methods Under Same Test Length
The measurement error results of the traditional P and P PMPU test and CAT-PMPU with the same test length are shown in Table 5. It could be seen that compared with the P and P PMPU test, CAT-PMPU significantly reduced the measurement error by 16.7–34.1% (M = 24.4%) (p < 0.001, Cohen’s d > 1). The measurement error of the traditional P and P PMPU test was high when test length was short. Measurement accuracy could be greatly improved from the unacceptable value in the original P and P test to an acceptable value by CAT-PMPU. For example, the measurement error of the traditional P and P PMPU test was 0.55 in 7-item SAPS, which was poor and unacceptable, while the measurement error of CAT-PMPU was 0.39.
Measurement Reliability of Two Test Methods Under Same Test Length
The measurement reliability results of the traditional P and P PMPU test and CAT-PMPU with the same test length are shown in Table 6. It could be seen that compared with the P and P PMPU test, CAT-PMPU significantly improved the measurement reliability by 4.5–23.2% (M = 11%) (p < 0.001, Cohen’s d > 1). The measurement reliability of the P and P PMPU test was low when test length was short. Measurement reliability could be greatly improved from the unacceptable value in the original P and P PMPU test to an acceptable value by CAT-PMPU. For example, the measurement reliability of the P and P PMPU test was 0.69 in seven-item SAPS, which was poor and unacceptable, while the measurement reliability of CAT-PMPU was 0.85.
The results showed that CAT-PMPU could greatly reduce measurement error and improve measurement accuracy under the same test length; that was to say, CAT-PMPU could achieve higher measurement accuracy than the P and P test if we use the scale with same length.
Comparison of the Accuracy of Computerized Adaptive Test for Problematic Mobile Phone Use and Each Paper-and-Pencil Problematic Mobile Phone Use Test
The ability estimation values were used to evaluate the accuracy of CAT-PMPU and P and P tests. First, the estimation values were calculated from original PMPU tests. Second, the length of the original PMPU tests was used as stop rule for CAT-PMPU. Then, seven sets of ability estimation values of seven CAT-PMPU tests could be gained. Eventually, the means and standard deviations from the seven P and P tests and seven CAT-PMPU could be compared. The correlations between each P and P test and CAT-PMPU were calculated. Besides, the ability estimation results based on all the 89 items were presented as a baseline. The results from Table 7 show that the correlations between CAP-PMPU and the P and P tests ranged from 0.78 to 0.92. The means of ability estimation from all the tests were approximate, around −0.2. It was noteworthy that the ability estimation results from seven CAT-PMPU were even better than those of the seven original P and P tests when compared with the 89-item test.
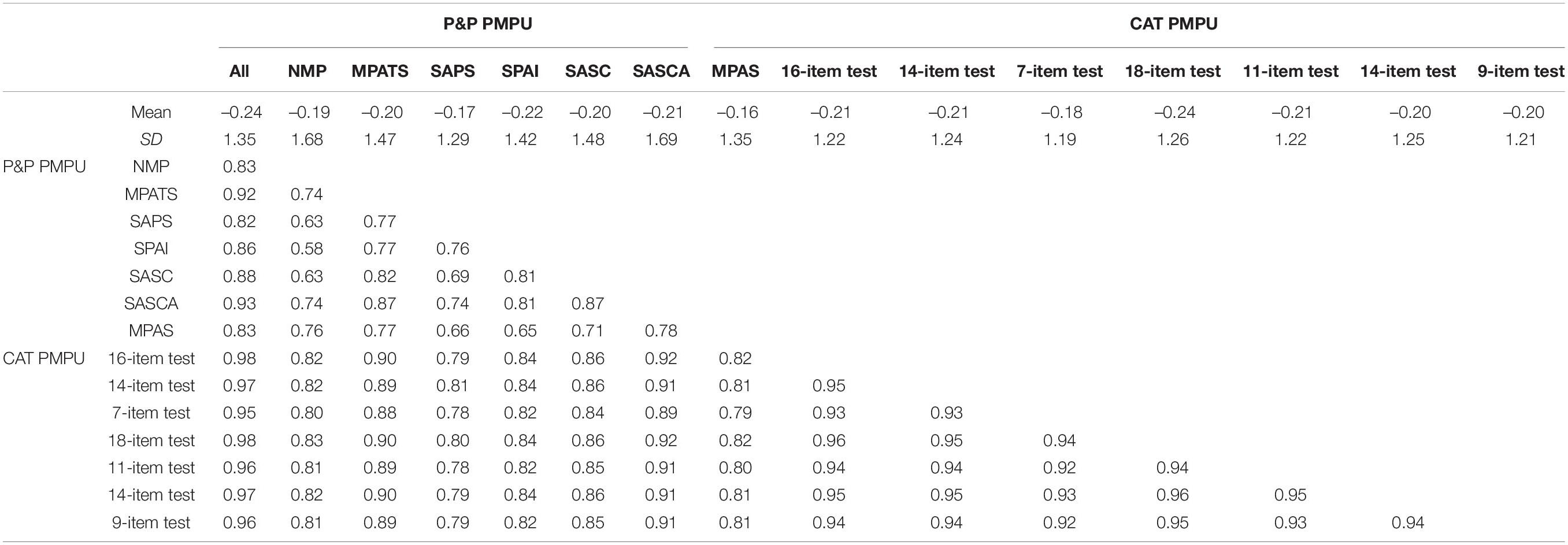
Table 7. Ability means, SDs, and correlation coefficients of computerized adaptive testing (CAT) and paper-and-pencil (P and P) tests under the same test length.
Comparison of the Effectiveness of Computerized Adaptive Test for Problematic Mobile Phone Use and Each Paper-and-Pencil Problematic Mobile Phone Use Test
In order to compare the effectiveness between CAT-PMPU and each P and P test, the item usage of two test methods was calculated. Specifically, measurement errors of the seven P and P tests were calculated as stop rules for CAT-PMPU. The average item usage for CAT-PMPU under the seven stop rules was calculated. The specific results were shown in Table 8, take P and P SPAI for instance, when individuals finished all 18 items, the accuracy of PMPU was 0.41 (SE = 0.41). By comparison, individuals only needed to complete 6.54 items to reach the same accuracy as P and P SPAI.
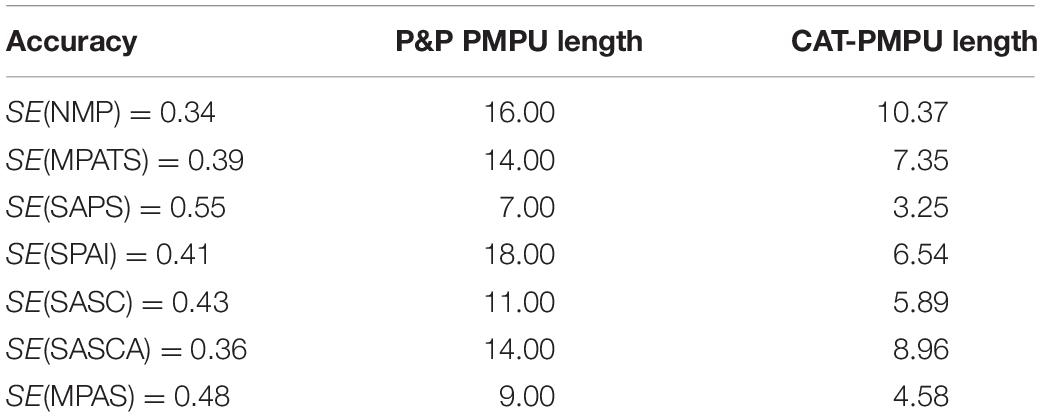
Table 8. Simulation results under seven stop rules based on P and P problematic mobile phone use (PMPU) tests.
Discussion
This study developed a computerized adaptive testing tool for problematic mobile phone use based on IRT. A simulation study based on an empirical dataset showed that the length of CAT-PMPU was much shorter than that of a P and P test, and that measurement errors were also much lower. Compared with the length of the seven P and P PMPU scales, CAT-PMPU reduced the test length, which further proved the advantages and effectiveness of CAT-PMPU. The CAT-PMPU developed in this study could reduce the cognitive burden of participants by reducing item consumption without decreasing the accuracy of PMPU assessment. That is an advantage of CAT (Pilkonis et al., 2014).
In this study, the participants only needed to complete four items in CAT-PMPU, and the estimated ability level was as high as 0.93 compared with the estimated ability level of completing all 89 items in the traditional P and P test. These results were similar to the results of CAT in other fields. For example, in computerized adaptive testing for college student social responsibility, participants only needed to answer 5.84 items to be highly correlated with the estimated ability of all items (Dai et al., 2020). It can be seen that CAT-PMPU will be easily used for large-scale investigations.
Compared with traditional P and P tests, CAT-PMPU offered different items for participants when measurement standard error was fixed at an acceptable level (for example, 0.3 and 0.4). Specifically, the individuals’ PMPU estimated from the new CAT-PMPU was significantly correlated with those estimated from a traditional full PMPU test (SE < 0.5, r = 0.93). It indicated that in CAT-PMPU, individuals’ PMPU levels could be accurately assessed with much less items with higher discrimination. In fact, if a little higher measurement error was permitted, less items would be used (92.2% of items were reduced when SE < 0.4, and 95.4% of items were reduced when SE < 0.5). This result was similar to some previous studies, i.e., in the computerized adaptive testing for self-esteem, the individuals’ ability estimated based on 9 items was significantly correlated with the ability estimated based on a full test (SE < 0.45, r = 0.91; SE < 0.32, r = 0.94) (Zheng and Cai, 2019).
There were some shortcomings needed to be further discussed. In this study, participants’ PMPU levels were estimated with fixed item parameters. Ignorance of estimation errors may lead to lower measurement accuracy. Niu and Choi (2022) proposed that performing the fully Bayesian adaptive testing with a revised proposal distribution would greatly reduce measurement errors and attain better estimation accuracy at the lower end of the ability scale. To get better measurement accuracy, this method will be employed in the future study.
The item selection strategy used in this study was MI, which was used by a large body of researchers (Luo et al., 2019; Zhang et al., 2020). However, this strategy prefer high discrimination items, which might result in overexposure of some items. The shadow-test approach or freezing shadow test approach that added random item-ineligibility constraints to the model would be a good solution to item exposure (Choi et al., 2021; van der Linden, 2021). Although PMPU is not a high-risk test, item exposure control techniques will be necessary when sample size keeps increasing.
Besides, PMPU tests are symptom-based tests. An individual always presents several symptoms at the same time. That means, items in PMPU tests are not thoroughly stand-alone. How to deal with this situation is a challenge for psychometricians (Choi and Lim, 2021). With augmentation of the item bank in the future, CAT-PMPU with adaptive test assembly methods will be taken into consideration.
Conclusion
The CAT-PMPU developed in this research meets the requirements of IRT measurement characteristics, and its measurement accuracy and efficiency are significantly better than those of P and P tests for problematic mobile phone use. In addition, CAT-PMPU can significantly improve measurement accuracy, which provides a new technical support for actual measurement of problematic mobile phone use.
Data Availability Statement
The original contributions presented in the study are included in the article/Supplementary Material, further inquiries can be directed to the corresponding author.
Ethics Statement
The studies involving human participants were reviewed and approved by Ethics Committee of Tianjin University (XL2020-12). The patients/participants provided their written informed consent to participate in this study.
Author Contributions
XL and TL contributed to conception and design of the study. ZZ organized the database. XL performed the statistical analysis and wrote the first draft of the manuscript. TL, HL, and MC revised the first draft of the manuscript. All authors contributed to manuscript read, and approved the submitted version.
Funding
This study was funded by the National Natural Science Foundation of China (Grant No. 31800945), which provided help in our data collection and data analysis, and also funded by Tianjin Undergraduate Training Program for Innovation and Entrepreneurship (Grant No. 202010065090), which provided help in our data collection.
Conflict of Interest
The authors declare that the research was conducted in the absence of any commercial or financial relationships that could be construed as a potential conflict of interest.
Publisher’s Note
All claims expressed in this article are solely those of the authors and do not necessarily represent those of their affiliated organizations, or those of the publisher, the editors and the reviewers. Any product that may be evaluated in this article, or claim that may be made by its manufacturer, is not guaranteed or endorsed by the publisher.
Supplementary Material
The Supplementary Material for this article can be found online at: https://www.frontiersin.org/articles/10.3389/fpsyg.2022.892387/full#supplementary-material
References
Acharya, J. P., Acharya, I., and Waghrey, D. (2013). A study on some of the common health effects of cell-phones amongst college students. J. Commun. Health 3:214. doi: 10.4172/2161-0711.1000214
Akaike, H. (1974). Stochastic theory of minimal realization. IEEE Trans. Automat. Control 19, 667–674. doi: 10.1109/cdc.1976.267680
Andrich, D. (1996). A general hyperbolic cosine latent trait model for unfolding polytomous responses: reconciling thurst-one and likert methodologies. Br. J. Math. Stat. Psychol. 49, 347–365. doi: 10.1177/014662169301700307
Basu, R., Pattanayak, S. K., De, R., Sarkar, A., Bhattacharya, A., and Das, M. (2021). Validation of a problematic use of smartphones among a rural population of West Bengal. Indian J. Public Health 64, 340–344. doi: 10.4103/ijph.ijph_2026_21
Bianchi, A., and Phillips, J. G. (2005). Psychological predictors of problem mobile phone use. Cyberpsychol. Behav. 8, 39–51. doi: 10.1089/cpb.2005.8.39
Billieux, J. (2012). Problematic use of the mobile phone: a literature review and a pathways model. Curr. Psychiatry Rev. 8, 299–307. doi: 10.2174/157340012803520522
Billieux, J., Linden, M., and Rochat, L. (2008). The role of impulsivity in actual and problematic use of the mobile phone. Appl. Cogn. Psychol. 22, 1195–1210. doi: 10.1002/acp.1429
Chen, H., Wang, L., Qiao, N. N., Cao, Y. P., and Zhang, Y. L. (2017). Development of the smartphone addiction scale for Chinese adults. Chin. J. Clin. Psychol. 25, 645–649. doi: 10.16128/j.cnki.1005-3611.2017.04.012
Choi, S. W., Gibbons, L. E., and Crane, P. K. (2011). Lordif: an R package for detecting differential item functioning using iterative hybrid ordinal logistic regression/item response theory and Monte Carlo simulations. J. Stat. Softw. 39, 1–30. doi: 10.18637/jss.v039.i08
Choi, S. W., and Lim, S. (2021). Adaptive test assembly with a mix of set-based and discrete items. Behaviormetrika 48, 1–24. doi: 10.1007/s41237-021-00148-6
Choi, S. W., Lim, S., and Van, D. (2021). Testdesign: an optimal test design approach to constructing fixed and adaptive tests in R. Behaviormetrika 48, 1–39. doi: 10.1007/s41237-021-00145-9
Chóliz, M. (2010). Mobile phone addiction: a point of issue. Addiction 105, 373–374. doi: 10.1111/j.1360-0443.2009.02854.x
Dai, B. Y., Xie, Y. X., and Jian, X. Z. (2020). The college students’ social responsibility testing based on computerized adaptation. J. Jiangxi Norm. Univ. 44, 142–147. doi: 10.16357/j.cnki.issn1000-5862.2020.02.05
Digital Reports (2021). A Decade in Digital. Available online at: https://datareportal.com/reports/a-decade-in-digital (accessed November 29, 2021).
Embretson, S. E. (1996). The new rules of measurement. Psychol. Assess. 8, 341–349. doi: 10.1037/1040-3590.8.4.341
Fliege, H., Becker, J., Walter, O. B., Bjorner, J. B., and Rose, K. M. (2005). Development of a computer-adaptive test for depression (D-CAT). Qual. Life Res. 14, 2277–2291. doi: 10.1007/s11136-005-6651-9
Forbey, J. D., and Ben-Porath, Y. S. (2007). Computerized adaptive personality testing: a review and illustration with the MMPI-2 computerized adaptive version. Psychol. Assess. 19, 14–24. doi: 10.1037/1040-3590.19.1.14
Fritts, B. E., and Marszalek, J. N. (2010). Computerized adaptive testing, anxiety levels, and gender differences. Soc. Psychol. Educ. 13, 441–458. doi: 10.1007/s11218-010-9113-3
Fumero, A., Marrero, R. J., Voltes, D., and Penate, W. (2018). Personal and social factors involved in internet addiction among adolescents: a meta-analysis. Comput. Hum. Behav. 86, 387–400. doi: 10.1016/j.chb.2018.05.005
Gaynes, B. N., Burns, B. J., Tweed, D. L., and Erickson, P. (2002). Depression and health-related quality of life. J. Nerv. Ment. Dis. 190, 799–806. doi: 10.1097/00005053-200212000-00001
Gezgin, D. (2017). Exploring the influence of the patterns of mobile internet use on university students’ nomophobia levels. Eur. J. Educ. Stud. 3, 29–53. doi: 10.5281/zenodo.572344
Gezgin, M., and Çakir, O. (2016). Analysis of nomofobic behaviours of adolescents regarding various factors. J. Hum. Sci. 13, 2504–2519. doi: 10.14687/jhs.v13i2.3797
Green, B. F., Bock, R. D., Humphreys, L. G., Linn, R. L., and Reckase, M. D. (1984). Technical guidelines for assessing computerized adaptive tests. J. Educ. Meas. 21, 347–360. doi: 10.2307/1434586
Griffiths, M. D. (1998). Psychology and the Internet: Intrapersonal, Interpersonal and Transpersonal Applications. New York, NY: Academic Press, 61–75.
Gul, H., Yurumez Solmaz, E., Gul, A., and Oner, O. (2018). Facebook overuse and addiction among Turkish adolescents: are ADHD and ADHD-related problems risk factors? Psychiatry Clin. Psychopharmacol. 28, 80–90. doi: 10.1080/24750573.2017.1383706
Hambleton, R. K., Swaminathan, H., and Rogers, H. J. (1991). Fundamentals of Item Response Theory. Newbury Park, CA: Sage Publications Inc. doi: 10.2307/2075521
Hao, Z., and Jin, L. (2020). Alexithymia and problematic mobile phone use: a moderated mediation model. Front. Psychol. 11:541507. doi: 10.3389/fpsyg.2020.541507
Herwin, H., Nurhayati, R., and Che, S. (2022). Mobile assessment to improve learning motivation of elementary school students in online learning. Int. J. Inf. Educ. Technol. 12, 436–442. doi: 10.18178/ijiet.2022.12.5.1638
Jiang, Y. Z., and Bai, X. L. (2014). College students rely on mobile internet making impact on alienation: the role of society supporting systems. Psychol. Dev. Educ. 30, 540–549. doi: 10.16187/j.cnki.issn1001-4918.2014.05.025
Kim, D., Lee, Y., Lee, J., Nam, J. E. K., and Chung, Y. (2014). Development of Korean smartphone addiction proneness scale for youth. PLoS One 9:e97920. doi: 10.1371/journal.pone.0097920
Lai, X. X., Huang, S. S., Zhang, C., Tang, B., Zhang, M. X., Zhu, C. W., et al. (2020). Association between mobile phone addiction and subjective well-being of interpersonal relationships and school identity among primary and secondary school students. Chin. J. Sch. Health 41, 613–616. doi: 10.16835/j.cnki.1000-9817.2020.04.036
Lee, Y.-K., Chang, C.-T., Lin, Y., and Cheng, Z.-H. (2014). The dark side of smartphone usage: psychological traits, compulsive behavior and technostress. Comput. Hum. Behav. 31, 373–383. doi: 10.1016/j.chb.2013.10.047
Leung, L. (2008). Linking psychological attributes to addiction and improper use of the mobile phone among adolescents in Hong Kong. J. Child. Media 2, 93–113. doi: 10.1080/17482790802078565
Li, Y. M., Liu, R., Hong, W., Gu, D., and Jin, F. K. (2020). The impact of conscientiousness on problematic mobile phone use: time management and self-control as chain mediator. J. Psychol. Sci. 43, 666–672. doi: 10.16719/j.cnki.1671-6981.20200322
Lin, Y. H., Chang, L. R., Lee, Y. H., Tseng, H. W., Kuo, T. B. J., and Chen, S. H. (2014). Development and validation of the smartphone addiction inventory (SPAI). PLoS One 9:e98312. doi: 10.1371/journal.pone.0098312
Liu, T., Guli, G. N., Yang, Y., Ren, S. X., and Chao, M. (2020). The relationship between personality and nomophobia: a mediating role of solitude behavior. Stud. Psychol. Behav. 18, 268–274.
Lu, X., Liu, T., Liu, X., Yang, H., and Elhai, J. D. (2022). Nomophobia and relationships with latent classes of solitude. Bull. Menninger Clin. 86, 1–19. doi: 10.1521/bumc.2022.86.1.1
Lu, X. R., Liu, T., and Lian, Y. X. (2021). Solitude behavior and relationships with problematic mobile phone use: based on the analysis of meta. Chin. J. Clin. Psychol. 29, 725–733. doi: 10.16128/j.cnki.1005-3611.2021.04.013
Luo, L. F., Tu, D. B., Wu, X. Y., and Cai, Y. (2019). The development of computerized adaptive social anxiety test for university students. J. Psychol. Sci. 42, 1485–1492. doi: 10.16719/j.cnki.1671-6981.20190630
Miyasawa, Y., and Ueno, M. (2013). “Mobile testing for authentic assessment in the field: evaluation from actual performances,” in Proceedings of the Humanitarian Technology Conference, Sendai, August 26-29, 2013 (Piscataway, NJ: IEEE). doi: 10.1007/978-3-642-39112-5_73
Muraki, E. (1992). A generalized partial credit model: application of an EM algorithm. ETS Res. Rep. 16, i–30. doi: 10.1177/014662169201600206
Nieto, M. D., Abad, F. J., and Olea, J. (2018). Assessing the Big Five with bifactor computerized adaptive testing. Psychol. Assess. 30, 1678–1690. doi: 10.1037/pas0000631
Niu, L., and Choi, S. W. (2022). More efficient fully Bayesian adaptive testing with a revised proposal distribution. Behaviormetrika. 49, 1–19. doi: 10.1007/s41237-021-00156-6
Olatz, L. F., Kuss, D. J., Pontes, H. M., Griffiths, M. D., Christopher, D., Justice, L. V., et al. (2018). Measurement invariance of the short version of the problematic mobile phone use questionnaire (PMPUQ-SV) across eight languages. Int. J. Environ. Res. Public Health 15:1213. doi: 10.3390/ijerph15061213
Pilkonis, P. A., Yu, L., Dodds, N. E., Johnston, K. L., Maihoefer, C. C., and Lawrence, S. M. (2014). Psychometric evaluation and calibration of health-related quality of life item banks: plans for the patient-reported outcomes measurement information system (PROMIS). Med. Care 45, S22–S31. doi: 10.1097/01.mlr.0000250483.85507.04
Ra, C. K., Cho, J., Stone, M. D., De La Cerda, J., Goldenson, N. I., Moroney, E., et al. (2018). Association of digital media use with subsequent symptoms of attention-deficit hyperactivity disorder among adolescents. JAMA 320, 255–263. doi: 10.1001/jama.2018.8931
Reckase, M. D. (1979). Unifactor latent trait models applied to multifactor tests: results and implications. J. Educ. Stat. 4, 207–230. doi: 10.3102/10769986004003207
Reeve, B. B., Hays, R. D., Bjorner, J. B., Cook, K. F., Crane, P. K., Teresi, J. A., et al. (2007). Psychometric evaluation and calibration of health-related quality of life item banks: plans for the patient-reported outcomes measurement information system (PROMIS). Med. Care 45, S22–S31. doi: 10.1097/01.mlr.0000250483.85507.04
Ren, S. X., Gu, L. G. N., and Liu, T. (2020). Revisement of nomophobia scale for Chinese. Psychol. Explor. 40, 247–253.
Samejima, F. (1969). Estimation of latent ability using a pattern of graded responses. Psychometrika 34:100. doi: 10.1007/BF03372160
Schwarz, G. (1978). Estimating the dimension of a model. Ann. Stat. 6, 461–464. doi: 10.1214/aos/1176344136
Shotton, M. A. (1989). Computer Addiction: a Study of Computer Dependency. London: Taylor & Francis.
Su, S., Pan, T. T., Liu, Q. X., Chen, X. W., Wang, Y. J., and Li, M. Y. (2014). Development of the smartphone addiction scale for college students. Chin. Ment. Health J. 28, 392–397. doi: 10.3969/j.issn.1000-6729.2014.05.013
Sun, X. G., and Guan, D. D. (2009). A comparative study on classical test theory and item response theory. Assess. Meas. 9, 10–17. doi: 10.19360/j.cnki.11-3303/g4.2009.09.003
Toda, M., Ezoe, S., Nishi, A., Goto, M., and Morimoto, K. (2008). Mobile phone dependence of female students and perceived parental rearing attitudes. Soc. Behav. Pers. 36, 765–770. doi: 10.2224/sbp.2008.36.6.765
Tossell, C., Kortum, P., Shepard, C., Rahmati, A., and Zhong, L. (2015). Exploring smartphone addiction: insights from long-term telemetric behavioral measures. Int. J. Interact. Mob. Technol. 9, 37–43. doi: 10.3991/ijim.v9i2.4300
van der Linden, W. J. (2021). Review of the shadow-test approach to adaptive testing. Behaviormetrika. 48, 1–22. doi: 10.1007/s41237-021-00150-y
Wang, A. Q., and Shi, X. L. (2021). “Risk factors of smartphone addiction among Chinese college students: a prospective longitudinal study based on GEE model,” in Proceedings of the 23rd National Academic Conference of Psychology, Hohhot. doi: 10.26914/c.cnkihy.2021.040243
Wang, L., Li, B. C., Tang, N. Q., Feng, S., and Zheng, Q. (2019). Peking University Post-95 Mobile Phone Use Psychological and Behavioral White Paper. Available online at: https://max.book118.com/html/2019/0805/8017047136002040.shtm
Weiss, D. J. (1982). Improving measurement quality and efficiency with adaptive testing. Appl. Psychol. Meas. 6, 473–492. doi: 10.1177/014662168200600408
Wu, Y. F., Cai, Y., and Tu, D. B. (2019). A computerized adaptive testing advancing the measurement of subjective well-being. J. Pac. Rim Psychol. 13:e6. doi: 10.1017/prp.2019.6
Xiong, J., Zhou, Z. K., Chen, W., You, Z. Q., and Zhai, Z. Y. (2012). Development of the mobile phone addiction tendency scale for college students. Chin. Ment. Health J. 26, 222–225. doi: 10.3969/j.issn.1000-6729.2012.03.013
Xu, L. L., Jin, R. Y., Huang, F. F., Zhou, Y. H., Li, Z. L., and Zhang, M. Q. (2020). Development of computerized adaptive testing for emotion regulation. Front. Psychol. 11:561358. doi: 10.3389/fpsyg.2020.561358
Yildirim, C., and Correia, A. P. (2015). Exploring the dimensions of nomophobia: development and validation of a self-reported questionnaire. Comput. Hum. Behav. 49, 130–137. doi: 10.1016/j.chb.2015.02.059
Young, K. S. (1998). Internet addiction: the emergence of a new clinical disorder. Cyberpsychol. Behav. 1, 237–244. doi: 10.1089/cpb.1998.1.237
Zhang, L. F., Liu, K., Song, G., and Tu, D. B. (2020). The application of cat on emotional intelligence with item response theory. J. Jiangxi Norm. Univ. 44, 454–461. doi: 10.16357/j.cnki.issn1000-5862.2020.05.02
Zheng, Z. N., and Cai, Y. (2019). The development of a computer-adaptive test for self-esteem. J. Jiangxi Norm. Univ. 43, 448–453. doi: 10.16357/j.cnki.issn1000-5862.2019.05.02
Keywords: problematic mobile phone use, computerized adaptive testing, item banking, item response theory, paper-and-pencil test
Citation: Liu X, Lu H, Zhou Z, Chao M and Liu T (2022) Development of a Computerized Adaptive Test for Problematic Mobile Phone Use. Front. Psychol. 13:892387. doi: 10.3389/fpsyg.2022.892387
Received: 09 March 2022; Accepted: 26 April 2022;
Published: 31 May 2022.
Edited by:
Alessandro Giuliani, National Institute of Health (ISS), ItalyReviewed by:
Maomi Ueno, The University of Electro-Communications, JapanMaria Eugenia Ferrao, University of Beira Interior, Portugal
Copyright © 2022 Liu, Lu, Zhou, Chao and Liu. This is an open-access article distributed under the terms of the Creative Commons Attribution License (CC BY). The use, distribution or reproduction in other forums is permitted, provided the original author(s) and the copyright owner(s) are credited and that the original publication in this journal is cited, in accordance with accepted academic practice. No use, distribution or reproduction is permitted which does not comply with these terms.
*Correspondence: Tour Liu, bWlrZWJvbml0YUBob3RtYWlsLmNvbQ==