- 1School of Education and Psychology, University of Navarra, Pamplona, Spain
- 2School of Psychology, University of Almería, Almería, Spain
- 3Department of Psychology, Fundación Universitaria Konrad Lorenz, Bogotá, Colombia
- 4Department of Psychology, Teesside University, Middlesbrough, United Kingdom
The self- vs. external-regulation behavior theory, SR-ER Theory (2021) model has postulated the Self-Regulation /Non or De-Regulation/Dys-regulation (SR-NR-DR) continuum in the person and in their context. The model also generates a behavioral heuristic that allows us to predict and explain the variability of other dependent behavioral variables in a range of scenarios (clinical, educational, health and technology contexts). Consequently, the objective of this study was to validate the different scales prepared on the basis of the theory presented. A total of 469 students voluntarily completed at different times the five questionnaires presented, to give a total of 1,385 completed questionnaires. Using an ex post facto design, descriptive, correlational, confirmatory factorial analysis (CFA), reliability, and concurrent validity analyses were carried out. The scales were analyzed individually and as a whole. The results showed the acceptable structure of scale and consistent levels of reliability. The five levels generated by the SR-NR-DR (personal and contextual) combinatory heuristic that arises from the theoretical model determined significant differences in the levels of the variables analyzed for each psychological context. We discuss the theoretical implications and the implications for the assessment and improvement of the behaviors analyzed in function of the personal and contextual regulation levels evaluated.
Introduction
Classical theoretical psychological models of human self-regulatory behavior (Self-Regulation, SR) have been fertile ground for work on defining, conceptualizing, evaluating, and creating strategies to improve self-regulation (Carver and Scheier, 1981; Mischel, 1981). From the seminal work of Bandura (1991) in his Social Cognitive Theory in which he described the construct of Self-Regulation until today there has been an avalanche of research. Searching for the term self-regulation in Google Scholar produced 1.95 million articles, an indication of the level of research interest in this area of study. Further, a search for self-regulation and health yielded 1.45 million articles and another for self-regulation and education gave 1.44 million articles.
There is copious support from both research findings and theoretical works for the importance of self-regulation as a psychological construct and the need to measure self-regulation (Pandey et al., 2018; Solé-Ferrer et al., 2019). Work in classical self-regulation theory has thus far focused on determining the contribution of self-regulation to the variability of studied behaviors. However, like other concepts in Psychology, the concept of self-regulation behavior is continuously developing as researchers endeavor to explain and better adapt to the reality studied. Our research group identified that this research approach left out of account psychological phenomena whose relationship with different levels or types of self-regulation has been insufficiently considered and did not adequately explore the extent to which context is predictive of self-regulatory behavior. That realization raised a number of questions that gave rise to this line of research (new theory of Self-Regulatory Behavior). Does self-regulatory behavior carry with it different meanings or levels that have not thus far been sufficiently examined? Can self-regulation be seen as a characteristic of the subject alone? Alternatively, should we also assume that context (depending on its nature) can promote or not promote self-regulation and may operate in the same way in terms of predicting such behavior? These open questions, raised by our research team, gave rise to the new theoretical model that supports this work (de la Fuente et al., 2022a). Finally, we concluded that it was necessary to create the new scales presented here. For this reason, the objectives of this manuscript are two: (1) to synthetically show the underlying theoretical construct; there are other recent works that do it more precisely (de la Fuente et al., 2022a), (2) present the structure and initial validation process of the Scales that allow it to be evaluated.
The classical theory of self-regulation
Self-Regulation (SR) is a construct of personality (Mithaug, 1993; Boekaerts et al., 1999; Hoyle, 2010) that describes the capacity of people to exercise planning, monitoring, and evaluation of their own behavior (Karoly, 1993; Brown, 1998; Vohs and Baumeister, 2016; Koopmann et al., 2019; Robson et al., 2020). The abundant prior research has shown SR’s positive association with factors such as personal adjustment (Mithaug, 1993; Wrosch et al., 2003) and its associations with aspects of personality: positive with conscientiousness and negative with neuroticism (Guido et al., 2015; de la Fuente et al., 2020b). An association has also been shown with behavioral adjustment in academic performance (Becker et al., 2014; Blair and Raver, 2015; Akfırat et al., 2016; Panadero, 2017; Bernardo et al., 2019; Alonso-Tapia et al., 2020). The classical understanding of the construct can be found in the work of Pervin (1988). Early notions of SR, based as they were on a molecular psychological analysis (de la Fuente et al., 2020b), had three common principles:
1. SR is a variable of the subject and is determined by other variables or factors particular to the subject, such as aspects of personality and metacognition (Hoyle, 2010; Malanchini et al., 2019; Jacqueline et al., 2020; Valikhani et al., 2020; Vega et al., 2020).
2. Contextual factors are of secondary importance and do not have a significant role in explaining the variability of behavioral regulation in the individual or its level, either in general or specifically in relation to education and health.
3. Individuals have higher or lower levels of SR; there are no defined categories of SR, merely degrees of SR.
The new vision of self-regulated vs. externally regulated behavior theory (SR–ER)
This Self- vs. External- Regulated Behavior Theory, or SR vs ER Theory model (de la Fuente, 2021b; de la Fuente et al., 2022a) has emerged to specify and expand the previous explanatory model, based exclusively on Self-Regulation (SR) variable (for a review, focused on the Educational Psychology context, please, see: de la Fuente, 2017). Through a molar analysis, this new model seeks to analyze the interaction between the regulatory characteristics of the person and the regulatory characteristics of their context (de la Fuente et al., 2020a). The SR-ER model is based on three principles and hypothesis:
Principle and Hypothesis 1: Types of Behavioral Regulation. Self-Regulation is a personal variable, which can be gradual, that is, levels or typologies can be established:
1. Self-Regulation Behavior Type (SR): It is the action of self-regulation (planning, self-control, and self-assessment) or internal regulation of the three levels of behavior: thoughts, emotions, and actions. It is considered an adaptative and positively proactive behavioral level (SR = +1).
2. Non-Regulation or De-regulation Behavior Type (NR): It can be considered as the action of ceasing to regulate or moving to a behavioral state of non-regulation of thoughts, emotions, and actions. It is considered a reactive or neutral behavioral level in positive and negative proactivity (SR = 0).
3. Dys-Regulation Behavior Type (DR): It refers to being unable to control behavior (thoughts, emotions, actions) in the way most people can. Before a situation. It supposes an excessive level of response (hyper-response or behavioral excesses) or negligible (under-response or behavioral deficits) that would characterize this type of behavior level. It is considered an adaptative and negative proactive behavioral level (SR = −1). See Figure 1.
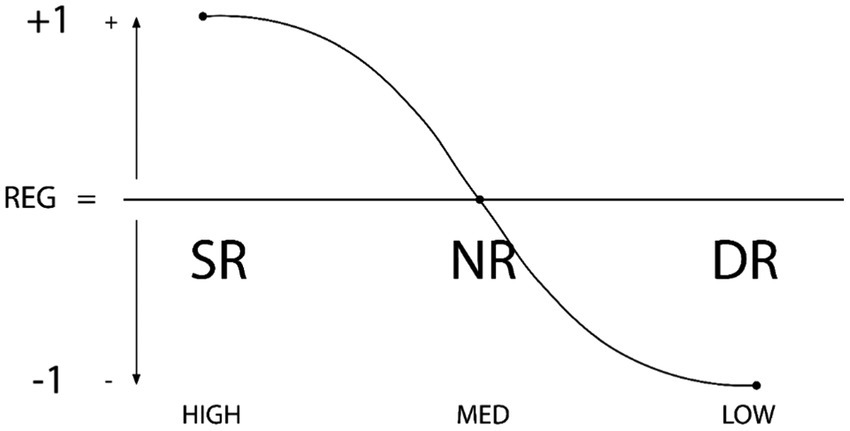
Figure 1. Graphic representation of the types of regulation: SR (Self-Regulation), NR (Non-Regulation or De-Regulation) and DR (Dys-Regulation). The degree of regulation (high-medium-low) is plotted on the x axis and the y axis shows positivity/negativity (+1, 0, −1). The curved line shows the possible types of regulatory stages of a person and also the possible directionality of behavioral change.
In this case, the concept of SR is assumed from Zimmerman’s previous model (Zimmerman, 2000; Zimmerman and Labuhn, 2012), but the types of non-regulatory behavior are incorporated, such as the absence of regulation and dysregulatory behavior such as malfunction of regulation. In the biological field, the concept of biological dysregulation has been coined to define the malfunction of a biological system (Goldman et al., 2006; Gouin, 2011; Carbone, 2020); consequently, it is possible to coin the term behavioral dysregulation this term in the psychological field. Previous behavioral research has also assumed it to define a maladjusted psychological or behavioral level (Beauchaine and Crowell, 2020; Forkus et al., 2020). The American Psychological Association (APA) defines dysregulation as “any excessive or otherwise poorly managed mechanism or response1”. In the field of psychology, a commonly discussed type of dysregulation is that of emotion dysregulation, which can negatively impact our well-being. Such is the human capacity for behavioral regulation that the individual can carry out SR, NR, and DR behaviors. These types of self-regulation are then associated with the three possible levels of SR (high-medium-low) whereby positive SR describes the presence of self-regulation whilst there are two levels for absence of regulation. SR and NR can therefore be expected to be negatively associated, whilst NR and DR are positively associated, such that NR is the intermediate or prior step toward DR.
Principle and Hypothesis 2: Types of External Regulation. Context factors are also considered proximal or influential when determining the variability of this behavior, with the External-Regulation Behavior (ER), External Non-Regulation or De-regulation behavior (ENR), and External Dys-Regulation behavior (ER) typologies:
4. External-Regulation Behavior Type (SR): It refers to the design and the characteristics of the context (such as antecedents and consequences of behavior), which probabilize and help exercise behavioral self-regulation (thoughts, emotions, and actions). It is considered an context adaptive and positively proactive behavioral level (ER = +1).
5. External Non-Regulation or External De-regulation Behavior Type (NR): It refers to the design and the characteristics of the context (such as antecedents and consequences of behavior), which do not externally probabilize or help self-regulation or dys-regulation; that is, the design of the context leaves the entire weight of regulation in the hands of the person. It is considered a context reactive or neutral contextual behavioral level in positive and negative proactivity (ER = 0).
6. External Dys-Regulation Behavior Type (DR): It refers to the design and the characteristics of the context (such as antecedents and consequences of behavior), which make possible and help exercise behavioral dys-regulation (in thoughts, emotions, and actions), making different kinds of behavioral excesses or deficits probable. It is considered a context adaptative and negative proactive behavioral level (ER = −1). See Figure 2.
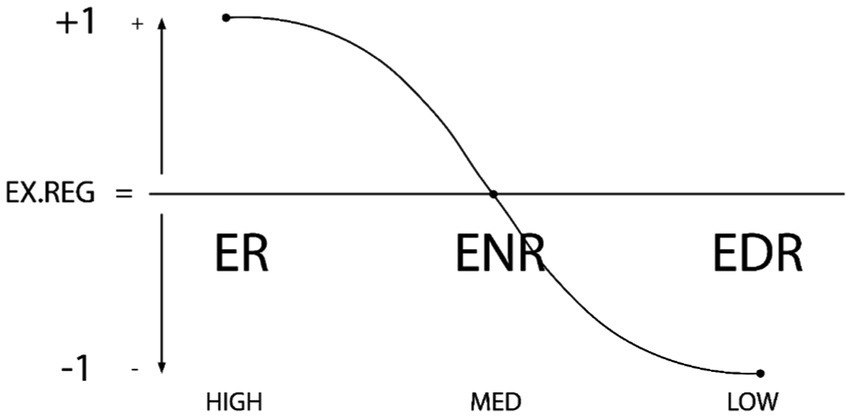
Figure 2. Graphic representation of the types of external regulation: ER (External Regulation), ENR (External Non-Regulation or De-regulation) and EDR (External Dys-Regulation). The degree of external regulation (high-medium-low) is plotted on the x axis and the y axis shows the positivity/negativity of external regulation (+1, 0, −1). The curved line shows the possible types of the context regulatory types and the possible directionality of the change in types of contexts.
From a behavioral perspective, if a context has a pro-regulatory value that means that it promotes self-regulation through specific behavioral mechanisms: adequate understanding of the precursors to and consequences of behavior, the degree of behavioral predictability that can be inferred from the context. Such is the susceptibility of human beings to the influence of their context that context can induce or externally promote SR, NR, and DR behaviors. Thus, context can be categorized into the same three levels of external regulation: ER (External Regulation), ENR (External Non-Regulation); and EDR (External Dys-Regulation). Here too, the absence of regulation has two levels rather than just one. ER and ENR can therefore be expected to be negatively associated, whilst ENR and EDR are positively associated such that ENR is the intermediate or prior step toward EDR.
Principle and Hypothesis 3: Internal vs. External Behavior Combination Regulation (combined regulation). Variability in human behavioral regulation depends on the combination of personal and contextual factors. That is, on the specific combination of the subject’s levels of personal self-regulation (high-medium-low) and the regulatory levels of the contextual regulation (high-medium-low). The heuristic used has five possible combinations of self-regulation and external regulation. This hypothesis has previously been tested and validated, with considerable consistency (de la Fuente et al., 2017, 2019b). The combination of both joint levels will be able to predict the level of this behavior, in different areas of behavior, for example, the clinical, educational, health, or technological field. The categories of high-medium-low behavioral combination of the subject and the context define 5 types of possible heuristic levels, already reported previously (de la Fuente, 2017; de la Fuente et al., 2022a). See Table 1.
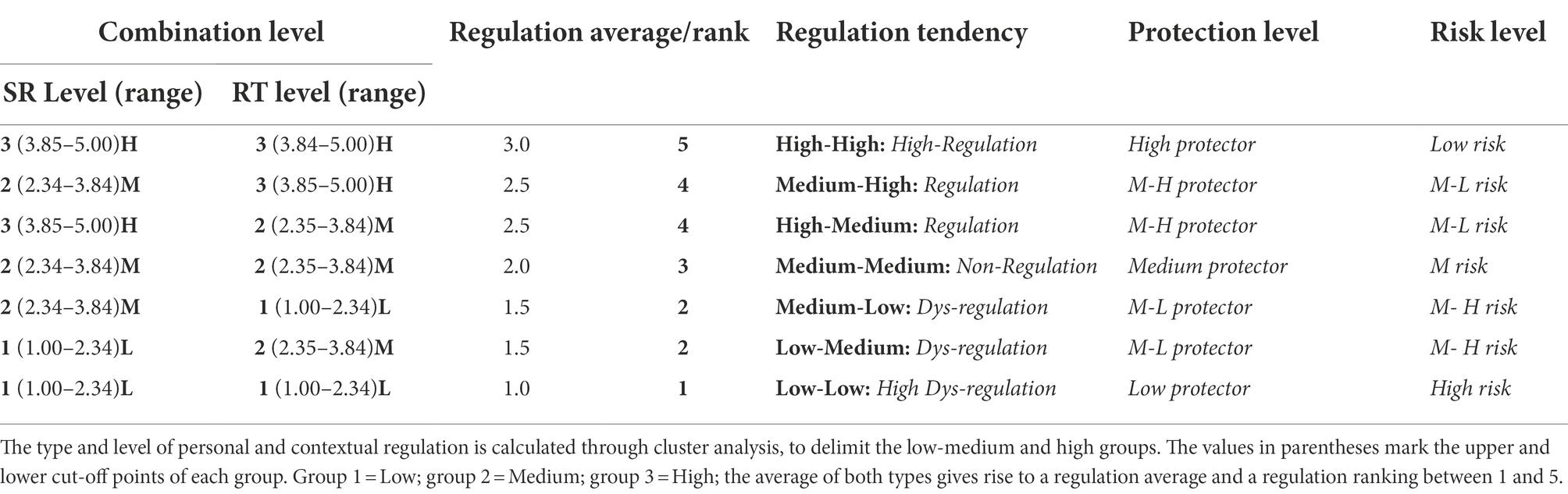
Table 1. Combinations of the model parameters hypothesized by SRL vs. ERL Theory (de la Fuente et al., 2019a).
A graphical presentation of the SR-ER combination can be seen in a number of published works which have repeatedly corroborated the same trend (de la Fuente et al., 2017). See Figure 3.
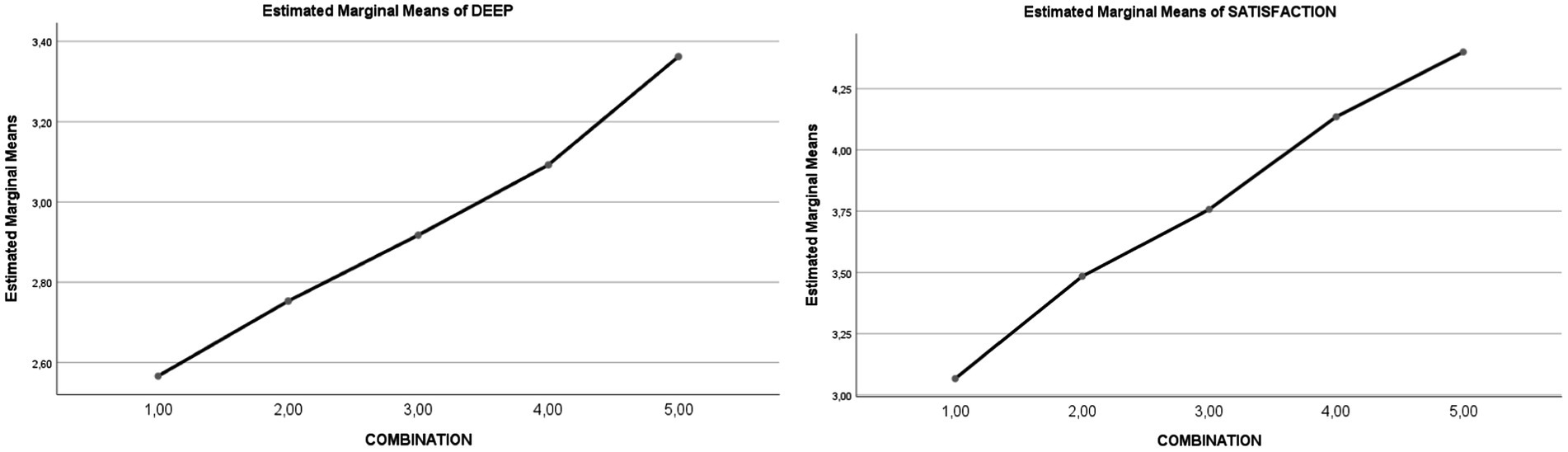
Figure 3. Effect of the SR-ER couple on the variables of deep learning and satisfaction in learning.
Self-regulation vs. external behavior regulation (SR-ER) in clinical psychology contexts
Self-regulation (SR) in clinical psychology contexts
In the field of clinical research, the self-regulation variable has appeared to be important for the explanation of other psychological constructs, such as personality (Inzlicht et al., 2021), resilience (de la Fuente et al., 2017), personal strengths (Lerner et al., 2021), coping strategies (Amate-Romera and de la Fuente, 2021), emotionality (Lajoie et al., 2021) and perfectionism (Thakre and Sebastian, 2021). Recently, studies have considered the dysfunctional level of self-regulation (dys-regulation) as a transdiagnostic variable (p factor) underlying numerous psychiatric psychopathologies (Duncan et al., 1996; Choi and Abbott, 2020; Huffhines et al., 2020; Smith et al., 2020; Levin-Aspenson et al., 2021; Romer et al., 2021) varying levels of which are relevant to criminal pathologies (Billen et al., 2021).
Internal vs. external self-regulation, non-regulation, dys-regulation (SR-ER) behavior in clinical psychology contexts
The SR-ER theoretical model (de la Fuente, 2017) proposes that the interaction of each person’s SR-NR-DR levels with their contextual ER-ENR-EDR levels is predictive and explanatory of adaptive vs. maladaptive behaviors for which explanation is sought. Thus, that interaction has been shown to determine the level of the variables of psychological reactance (Pachón-Basallo et al., 2021), procrastination (de la Fuente et al., 2021b), symptoms of stress and anxiety (de la Fuente et al., 2021b), positive–negative affects and psychological well-being and executive functioning and emotional dysregulation (Leerkes et al., 2020; de la Fuente et al., 2022b) with repeated consistent effects. In each case, the five-level SR-ER combinatory heuristic shows discriminatory power to determine the level of the dependent variables measured. Recent research has also shown the dysregulatory effect of traumatic experiences in childhood and adolescence, because they have produced regulatory imbalances, producing cognitive, emotional, and behavioral excesses or deficits (Claudine et al., 2021).
Self-regulated vs. externally regulated learning (SRL–ERL) in educational psychology contexts
SR in educational psychology contexts
In the field of education, SR research has focused on the Self-Regulated Learning (SRL) construct. Historically, different theoretical models of SRL have coexisted (Panadero, 2017). Of those competing models, one of the most successful in determining the specific behavioral levels of the learning process is the model put forward by Zimmerman (Zimmerman and Schunk, 2001).
There is extensive evidence available in relation to the role of SRL in education and educational processes. SRL has been shown to be associated with numerous aspects of the learning process: motivation, emotion, and performance (de la Fuente and Eissa, 2010; Peña-Lara, 2015; Dinsmore et al., 2020). Those associations have also been shown in different stages of education, in particular university education (de la Fuente et al., 2017).
Recent studies have also shown that SR is a personality variable that suggests or predicts SRL (de la Fuente et al., 2015), academic emotions (de la Fuente et al., 2020a), coping strategies (de la Fuente et al., 2020c), and levels of academic stress (de la Fuente et al., 2020c).
Internally vs. externally regulated, unregulated, and dysregulated learning (SRL-ERL) behavior in educational psychology contexts
A number of studies have considered SRL-ERL, some of which have gone as far as to propose that the environment has a greater regulatory value than the subject in computer contexts (Azevedo et al., 2005). Earlier work by our research team using the SRL-ERL model (de la Fuente, 2017) also showed how the combination of the low-medium-high SR level of students and the RT level of the teaching process produces an effect with a stable linear rising function for the five levels described. That linear function determines the level of other dependent variables, such as emotions associated with academic achievement (de la Fuente et al., 2020a), learning focus (de la Fuente et al., 2020c), academic confidence (de la Fuente et al., 2021c), and protective and risk factors for stress (de la Fuente, 2021a) in a recurrent manner.
Self-regulation vs. external regulation in psychology of health (SRH-ERH) contexts
SR and psychology of health contexts
Research into SR has also been significant in the field of health. SR has been integrated into models of a number of health problems and their prevention (Hull and Slone, 2004; Blood, 2012; Mann et al., 2013; Rathnayake and Chandradasa, 2020). The positive predictive value of SR in relation to health has been confirmed (Quinn and Fromme, 2010; Garzón-Umerenkova et al., 2017). Evidence in relation to the role of SR in chronic disease in the field of the Psychology of Health is extensive (Hennessy et al., 2020; Wilson et al., 2020). The SR model has also been used in specific pathologies (Clark and Zimmerman, 1990; Zimmerman et al., 1999).
Generally speaking, earlier evidence has in common the secondary or indirect value attributed to context in the explanation of the probability of different behaviors, although some recent work has considered context (Höhn et al., 2020). Hence the need to widen the focus of our vision, moving from the molecular to the molar, to pay closer attention to the interaction between the person and their environment (de la Fuente et al., 2020a).
Internal vs. external self-regulation, non-regulation, dys-regulation (SRH-ERH) behavior in psychology of health contexts
Earlier research has shown the harmful effects of dysregulatory contexts on psychological well-being. Other earlier studies have consistently shown that the SR-ER combination of the low-medium-high SR level of students and of the teaching process produces an effect with a linear function—that rises or fall depending on the variable—for the five levels described. That linear function determines in a recurrent format the level of other dependent variables such as the factors leading to, and the symptoms of, academic stress (de la Fuente et al., 2020c) and the coping strategies used (de la Fuente et al., 2020a).
Self-regulation vs. external regulation In psychology of technology (SRT-ERT) contexts
SR and psychology of technology contexts
SR has appeared to be an important variable in determining how appropriately technology is used that offers a degree of protection against addictive behaviors (Chen et al., 2021; Khan et al., 2021). Alongside that, there is considerable prior research that provides systematic evidence that an individual’s level of’ behavioral self-regulation (impulsiveness and lack of control) affects and may determine where on the appropriate use-abusive dependent use of technology continuum the individual falls (Azevedo and Feyzi-Behnagh, 2011). In fact, the term “behavioral addiction” was coined to refer to the problem and the maladjustment inherent to lack of self-regulation in the use of today’s technological devices (Kuss et al., 2014; Maya, 2020).
Internal vs. external self-regulation, non-regulation, dys-regulation (SRH-ERH) behavior in psychology of technology contexts
Contextual factors have also been associated with or predictive of technology-related addictive behaviors (Li, 2021). However, we know relatively little about the role of the interaction of the individual with their context in terms of the fostering of maladjusted behavior in the use and abuse of technology. Knowledge of the interaction between an individual’s level of self-regulation (SR-NR-DR) and their context (ER-ENR-ED) could materially advance our knowledge of the relative contribution of combinations of those factors to explaining the variability of addictive vs. non-addictive use of technology. The different levels predicted by this new theoretical model have yet to be shown.
Aims
Against that theoretical background, the objectives of this research were: (1) to provide empirical validation of the (internal and external) SR-NR-DR continuum proposed by using the instrument put forward; (2) to validate the different versions of the tool, as applied to different psychological contexts: clinical, educational, health and technology. The assumed hypotheses were: (1) The total scores for the different versions of the instrument would share a construct structure and acceptable levels of reliability in the continuum proposed and would have sufficient discriminant validity to categorize the different types of combination proposed in the SR-ER combination: 1. Low; 2. Medium-Low; 3. Medium; 4. Medium-High; 5. High. (2) The different versions of the instrument would have adequate construct validity and reliability with sufficient discriminant power or external validity with respect to different constructs of relevance in each field: clinical, educational, health, and technology.
Materials and methods
Participants
A total sample of 1,358 (770 women and 558 men) carry out was obtained through convenience sampling, from Spanish university students attending public universities. The students were studying different academic subjects at different levels. The age range was 18–25 (mean = 22.50; dt = 1.90). Each scale was completed by an average of 489 students. The sample was randomly divided into two subsamples (50 and 50%) in order to carry out parallel studies that would allow corroborating and verifying the results obtained (cross validation). The first half (subsample 1) was made up of 680 students: 390 women and 294 men. The second half (subsample 2) was made up of 678 students: 380 women and 264 men.
Instruments
Self-regulation vs. external regulation behavior (de la Fuente, 2022; See Supplementary Material).
1. Self-Regulation vs. External Regulation in Clinical Psychology Contexts (ER vs. ER). This variable was measured using the Self-Regulation vs. External Regulation Scale (de la Fuente, 2022). The scale consists of a total of 36 items self-reported against a Likert scale (1 = does not apply to me, 5 = very much applies to me). It has six components each formed of six items, through which both the behavioral types, Self-Regulation Behavior (SR), Non-regulation Behavior (NR), and Dys-Regulation Behavior (DR), and the contextual types, external regulation behavior (ER), External Non-regulation behavior (ENR), and External dys-regulation Behavior (EDR) are measured.
2. Self-Regulated vs. Externally Regulated Learning Behavior in Educational Psychology Contexts (SRL vs. ERL). This variable was measured using the Self-Regulated vs. Externally Regulated Learning Scale in Educational Psychology (de la Fuente, 2022). This scale consists of a total of 36 items self-reported against a Likert scale (1 = does not apply to me, 5 = very much applies to me). It contains six factors each formed of six items through which both the behavioral types SRL (Self-Regulated Learning), NRL (Non-Regulated Learning) and DRL (Dys-Regulated Learning), and the contextual types ERL (Externally Regulated Learning), ENRL (Externally Non-Regulated Learning) and EDRL (Externally Dys-Regulated Learning) are measured.
3. Self-Regulation vs. External Regulation Behavior in Health Psychology Contexts (SRH vs. ERH). This variable was measured using the Self-Regulation vs. External Regulation Scale in Health Psychology Contexts (de la Fuente, 2022). This scale consists of a total of 36 items self-reported on a Likert scale (1 = does not apply to me, 5 = very much applies to me). It has six components each formed of six items through which both the behavioral types SRH (Self-Regulation in Health), NRH (Non-Regulation in Health) and DRH (Dys-Regulation in Health), and the contextual types ERH (External Regulation in Health), ENH (External Non-Regulation in Health) and EDH (External Dys-Regulation in Health) are measured.
4. Self-Regulation vs. External Regulation Behavior in Technology Contexts. This variable was measured using the Self-Regulation vs. External Regulation Scale in Technology Contexts (de la Fuente, 2022). This scale consists of a total of 36 items self-reported on a Likert scale (1 = does not apply to me, 5 = very much applies to me). It has six components each formed of six items through which both the behavioral types SRT (Self-Regulation in Technology), NRT (Non-Regulation in Technology), and DRT (Dys-Regulation in Technology), and the contextual types ERT (External Regulation in Technology), ENT (External Non-Regulation in Technology), and EDT (External Dys-Regulation in Technology) are evaluated. See Table 2.
Self-Regulation Behavior. This variable was measured using the Short Self-Regulation Questionnaire (SSRQ), based on the original Self-Regulation Questionnaire. It has previously been validated in Spanish samples (Pichardo et al., 2014), and has acceptable validity and reliability values comparable to those of the English version. The SSRQ is composed of four factors (goal setting-planning, perseverance, decision-making, and learning from mistakes) and 17 items (all of them with saturations greater than 0.40), with a consistent confirmatory factor structure (Chi-square = 845,593, df = 113, CH/DF = 7.48; p < 0.001; RMR = 0.0299; NFI = 0.959, RFI = 0.951, IFI = 0.964, TLI = 0.957; CFI = 0.964; RMSEA =0.06). Internal consistency was acceptable for all questionnaire items collectively (α = 0.811) and for the factors of goal setting-planning (α = 0.709), perseverance (α = 0.735), and decision making (α = 0.757), and learning from mistakes (α = 0.703).
Negative Emotional Reactivity. The Perth Emotional Reactivity Scale, PERS (Becerra et al., 2017). This scale measures domains such as positive and negative emotional reactivity, it comprises 30 items and has a consistent confirmatory factor structure (Chi-square = 26.054, df = 5, CH/DF = 5.211; p < 0.001; RMR = 0.039; NFI = 0.954, RFI = 0.916, IFI = 0.962, TLI = 0.958; CFI = 0.961; RMSEA = 0.08). Reliability coefficients are Alpha total = 0.878, Omega = 0.846; Alpha 1 = 0.775, Alpha 2 = 0.797; Spearman–Brown = 0.867; Guttman = 0.867.
Psychological Well-Being. We used the Scales of Psychological Well-Being (Ryff, 1989) in Spanish (Díaz et al., 2006) in the 29-item version which has a consistent confirmatory factor structure (Chi-square = 845,593, df = 113, CH/DF = 7.48; p < 0.001; RMR = 0.029; NFI = 0.937, RFI = 0.942, IFI = 0.961, TLI = 0.956; CFI = 0.964; RMSEA = 0.05). The scale has six sub-scales: self-acceptance, positive relationships, autonomy, environmental mastery, personal growth, and purpose in life. We used a six-point Likert scale from “Does not apply to my life at all” to “Totally applicable.” Reliability coefficients are appropriate: Alpha total = 0.905, Omega = 0.886; Alpha 1 = 0.823, Alpha 2 = 0.832; Spearman–Brown = 0.867; Guttman = 0.867.
Achievement Emotion (Studying). Learning-Related Emotions (de la Fuente et al., 2015). The psychometric properties of LRE were satisfactory in students from Spain. In this sample, the model obtained good fit indices. Unidimensionality of the scale and metric invariance were confirmed in the samples evaluated (Chi-square = 10,885.597, Degrees of freedom = 3,052, p < 0.001; CFI = 0.959, TLI = 0.942, IFI = 0.969, TLI = 0.955, and CFI = 0.958; RMSEA = 0.038; HOELTER = 501, p < 0.05; 511 p < 0.01). Reliability coefficients are appropriate [Cronbach Alpha = 0.930, omega = 0.897; part 1 = 0.880 (38 items), and part 2 = 0.846 (37 items), respectively, for each part (75 items)].
TABP: Impatience-Hostility. Action-emotion style. The Jenkins Activity Survey for Students – Form H (JASE-H) was used. This scale for measurement of TABP was adapted (Bermúdez et al., 1990, 1991) from the form T Jenkins Activity Survey (Krantz et al., 1974). It measures four components: Impatience, Hostility, Competitiveness, and Overwork. In total, the questionnaire contains 32 items, each with a six-point Likert-type response format. The subject has to choose the degree to which an item applies to them, where 1 means that the item does not apply at all to the subject and 6 means that it is fully applicable. The JASE-H offers both a global TABP score, obtained by adding the scores for all the items, and specific measurements for each component of the TABP. The JASE-H shows high internal consistency (alpha coefficient of 0.85 for the total scale, 0.81 for Impatience-Hostility, 0.82 for Competitiveness, and 0.70 for Overwork) and high stability over time, both for the complete scale (0.68) and for each subscale (0.61, 0.76 and 0.70, respectively). Reliability and validity measurements reported by the authors are consistent. The statistics are Alpha = 0.832, Omega = 8.031; and Guttman Split-Half = 0.803.
Procedure
In five different studies, students completed their questionnaires (see Complementary Material) on an online platform: www.inetas.net (de la Fuente et al., 2015), after signing an informed consent form. Different students completed five specific questionaries during a two-year academic period. Inventory 1 was assessed in September–October of 2019 and 2020; Inventory 2, in November–December of 2019 and 2020; Inventory 3, in February–March of 2019 and 2020; Inventory 4, in April–May of 2019 and 2020; and Inventory 5 variables in May–June of 2019 and 2020. The Self-Regulation Questionnaire was completed with the other questionnaires in April–May 2019–2020. Questionnaire completion was voluntary. The respective Ethics Committees of the two universities approved the procedure as part of an R&D Project (2018–2021): http://www.estres.investigacion-psicopedagogica.org/lib/pdf/CERTIFICADO_COMITE_DE_ETICA_UNAV.pdf.
Data analysis
Sample design. A random sample was designed to estimate the proportion of interest if measured at a level that is greater than 200 people (n > 200); that is, the maximum permissible error in the estimation of the proportions of 7% and equivalently for the estimation of the average score of the scale.
Content validity: through expert validity. The methodological reference for the process of content validity by expert judgment was considered as “an informed opinion of people with experience in the subject, who are recognized by others as qualified experts in it, and who can provide information, evidence, judgments and assessments” (Escobar and Cuervo, 2008; p. 29). A template was used, developed by these authors, with four categories, and a licker-type response range from 1 (not at all) to 5 (a lot):
1. Clarity: the items are understood correctly, with adequate syntax and semantics.
2. Coherence: the items have an adequate relationship with the dimension and scale.
3. Relevance: the items are completely related to the dimension and scale under analysis.
4. Sufficiency: the items of each dimension are sufficient to measure it adequately.
This template was sent to seven experts on the topic (self-regulation), from each area and type of Scale. They were considered so if they were accredited by their research experience with more than 10 recently published articles on the topic. Upon receipt, a content validity coefficient analysis was applied by degree of interjudge agreement per item. A degree of agreement of 80% was obtained in the items of each scale, which was considered acceptable, es decir un IFV de 0.80 (Rubio et al., 2003).
Preliminary analysis. Adequacy of parametric analyses was first confirmed by determination of normal distribution (Kolmogorov–Smirnov test), skewness, and kurtosis (+/−0.05). In this case, the majority of values were below or near 0.50.
Criterion or concurrent validity: Correlation. For purposes of evaluation of the associations posited by the study hypotheses, positivity was correlated with resilience, coping strategies, and engagement-burnout (Pearson bivariate correlation) using SPSS (v.26). The assumptions for the bivariate correlation were: (1) The data have a linear relationship as established by scatter plot; (2) The variables are normally distributed; (3) The observations used for the bivariate correlation are a random sample from the reference population. Correlation bands were set according to customary criteria: low (0.10–0.30), medium (0.40–0.70), and high (0.80–0.90).
Construct validity. The sample was randomly divided into two subsamples (50 and 50%) using the Statistical Package for the Social Sciences (SPSS, version 26) in order to carry out parallel studies that would allow corroborating and verifying the results obtained (cross validation):
1. Exploratory Factorial Analysis (EFA). This analysis was performed with 50% of the sample. The Kaiser–Meyer–Olkin indices, Bartlett’s Sphericity Test, and factor communality values were used. Varimax rotation was used, with maximum likelihood and percentage of variance explained by each factor and the total of the scale. KMO was taken to be 0.80 and the Bartlett significance level was p < 0.001.
2. Confirmatory Factorial Analysis (CFA). With the remaining 50% of the sample, the previous factorial structure was calculated. Model fit was assessed by the Chi-square: degrees of freedom ratio, Comparative Fit Index (CFI), Normed Fit Index (NFI), Incremental Fit Index (IFI), and Relative Fit Index (RFI). Target values were greater than 0.90. We used the Hoelter Index to confirm that the sample was of adequate size (Tabachnick and Fidell, 2001). AMOS (v.26) was used.
Reliability. Cronbach’s Alpha index and the Omega Index (McDonald, 1999) were used. Cut-off values were set at 0.80.
Variance Analysis. ANOVA and MANOVA were performed to analyze external and concurrent validity. First, each subject’s score for regulation in each questionnaire was calculated as: Total Internal and External Regulation = [(SR + ER)/2 − (SNR + ENR)/2 − (SDR + EDR)/2)]/3. This continuous heuristic is adjusted to a linear format (see Figures 1, 2) with respect to the previous scalar heuristic (see Table 1). Subsequent cluster analysis determined the central values and the intersection points between them for each questionnaire and for the questionnaires as a whole. As can be seen, the distribution of the inventories follows the curve of the proposed theoretical relationship, albeit in a wider range of approximately −2.00–1.00. This comes about because levels of regulation are totaled; thus, whilst self-regulation is positive (+1.00), non-regulation and dys-regulation are negative (up to −2.00 at most). It should be noted that the scores in the table are similar for the different scales and the General Scale. See Table 3.
Results
Study 1. Self-regulation vs. external regulation behavior psychology total inventory (SRT-ERT)
Descriptive results
The descriptive values found met the normality requirements to be expected of this type of sample and subsequent analysis. See Table 4.
Construct validity
Correlation
SRTOT was negatively correlated with NRTOT and DRTOT; NRTOT and DRTOT had a significant negative correlation. Across this context, the correlations are the same in terms of direction: negative between ERTOT and ENTOT and positive between ENTOT and EDTOT. Note also the consistent negative and positive correlation of components of the scale with the aggregate score for the SR-ER.TOT construct. See Table 5.
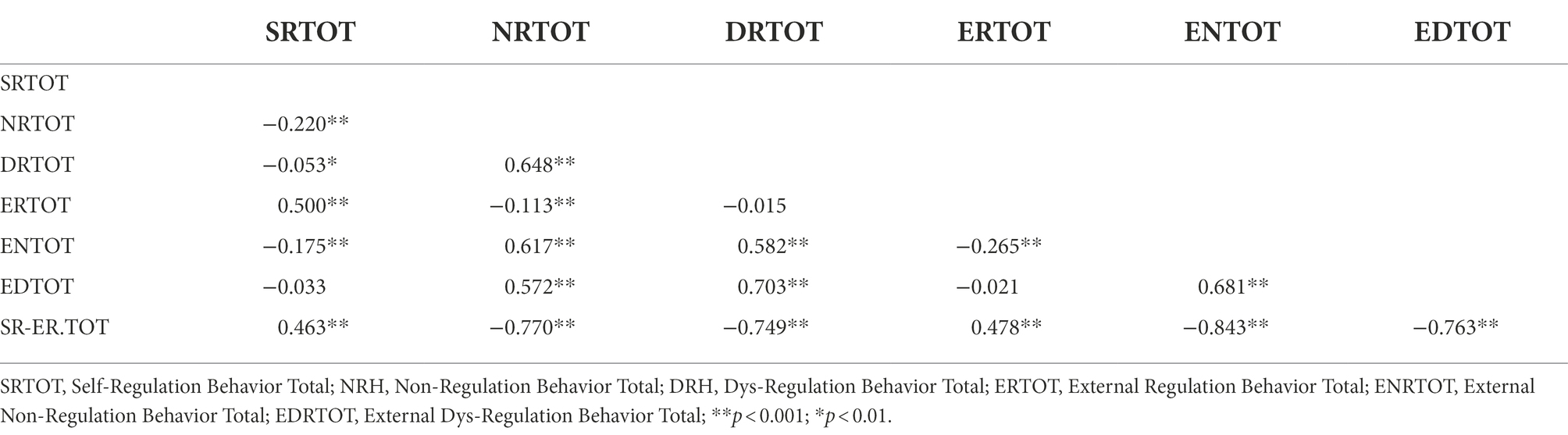
Table 5. Correlation between internal and external regulation and the total score for the scale (n = 1,358).
Exploratory Factorial Analysis (EFA). This analysis was carried out with 50% of the sample, obtaining adjusted values: Kaiser–Meyer–Olkin = 0.936; Bartlett’s Sphericity Test (630) = 15,703, 146, p < 0.001; factor communality was between 0.426 (item 8) and 0.785 (item 34). In the varimax rotation, six factors appeared that explained 65% of the variance: Factor 1, EDRT (24.5% variance) = items 34, 33, 35, 36, 32, 31; Factor 2, ERT (13.14% variance) = 21,23,20, 24, 22, 19; Factor 3, SRT (14.05%) = 3,4,5,6,2,1; Factor 4, DRT (11.54% variance) = 16,15,13, 17,18, 14; Factor 5, NRT (10.64%) = 29, 28, 27,25,26,30; Factor 6, NRT (5.71%) = 10,7,9,8,11,12.
Confirmatory Factorial Structure. The structural values for this construct appeared to be acceptable [Chi-square = 3,527.914, p < 0.001; df (702–118) = 584; CH/DF = 6.041; CFI = 0.912; GFI = 0.900; IFI = 0.926; TLI = 0.915; CFI = 0.926, RMSEA = 0.019; RSMR = 0.045; Hoelter = 2,417 (p < 0.05), 2,512 (p < 0.01)]. See Table 6.
Criterion-related validity: SR-ER general
Formation of groups. The ANOVA carried out to form the groups showed a significant principal Group Factor effect for SR-ER TOTAL relative to the total score for SR-ER.TOT [F(4.1353) = 5430.739, p < 0.001; eta2 = 0.941, power = 1.00; post-hoc = 5 > 4 > 3 > 2 > 1, p < 0.001]. Levene’s test of error variance based on the mean showed the adequacy of the groups [L(4.1353) = 1.949, p < 0.127]. See Table 7 for the descriptive statistics.
Effect of the SR-ER General group on each type of regulation. The ANOVA carried out showed a significant principal effect of the SR-ER General Group relative to each FACTOR IN TOTAL REGULATION [F(24.5404) = 77.493 (Pillai), p < 0.001; eta2 = 0.256, power = 1.00], and to the individual components: SRT [F(4,1,353) = 93.301, p < 0.001; eta2 = 0.216, power = 1.00]; NRG [F(4.1353) = 93.301, p < 0.001; eta2 = 0.561, power = 1.00]; DRT [F(4.1353) = 387.232, p < 0.001; eta2 = 0.534, power = 1.00]; ERT [F(4,1,353) = 93.301, p < 0.001; eta2 = 0.261, power = 1.00]; ENRT [F(4.1353) = 93.301, p < 0.001; eta2 = 0.676, power = 1.00]; EDRT [F(4.1353) = 93.301, p < 0.001; eta2 = 0.556, power = 1.00]. Note the greater explanatory weight of the indices in both the internal and external non-regulation and dys-regulation components. Levene’s test of error variance based on the mean showed the adequacy of the groups [L(4.1353) = 2.788, p < 0.099]. See Table 8 for the descriptive statistics.
Reliability
The total reliability of the scale showed adequate ratios (Cronbach’s Alpha = 0.900; Omega Index = 0.897). Split-half analysis showed adequate values (Alpha 1 = 0.802; Alpha 2 = 0.858; Spearman–Brown Coefficient = 0.828; Guttman Split-half Coefficient = 0.828). The ratios for each scale also: SR (Alpha = 0.888), NR (Alpha = 0.738), DR (Alpha = 0.857), ER (Alpha = 0.943) ENR (Alpha = 0.880); EDR (Alpha = 0.918).
Study 2. Self-regulation vs. regulatory behavior inventory regulation in clinical psychology contexts (SR-ER)
Descriptive results
The descriptive values found met the normality requirements to be expected of this type of sample and subsequent analysis. See Table 9.
Construct validity
Correlation
There was a significant negative correlation of SR with NR and DR and a significant positive correlation of NR with DR. Across this context, the correlations are consistent in direction: negative between ER and EN and positive between ENR and EDR. Finally, the trend seen with general Self-Regulation was confirmed. The correlations between the components of the scale and the scores for the total construct have the same directions. See Table 10.
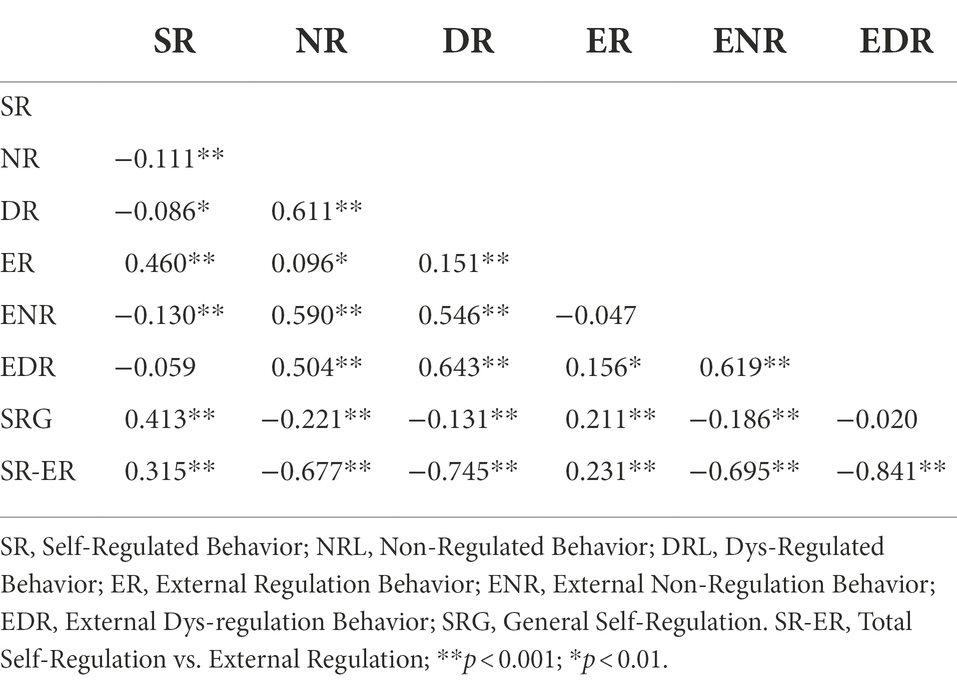
Table 10. Correlations between the Self-Regulation General (SRG) construct and the types of self-regulation and external regulation (SR-ER; n = 422).
Exploratory Factorial Analysis (EFA). This analysis was carried out with 50% of the sample, obtaining adjusted values: Kaiser–Meyer–Olkin = 0.876; Bartlett’s Sphericity Test (630) = 4, 154, 307, p < 0.001; factor communality was between 0.362 (item 8) and 0.827 (item 22). In the varimax rotation, six factors appeared that explained 65.50% of the variance: Factor 1, EDR (15.38% variance) = items 34, 36, 35, 32, 31, 33; Factor 2, ER (13.30% variance) = 23, 22, 21, 19, 24, 20; Factor 3, SR (11.21%) = 5, 3, 4, 6, 1, 2; Factor 4, ENR (10.17% variance) = 28, 29, 26, 30, 27, 25; Factor 5, NR (7, 78% variance) = 10, 7, 9, 11, 8, 12; Factor 6, DR (7.63%) = 14, 16, 17, 15, 13, 18.
Confirmatory Factorial Analysis (CFA). The structural values for this construct appeared to be adequate [Chi-square = 1,575.861, df (702–118) = 584, p < 0.001; Chi/df = 2.689; RMR = 0.0351; NFI = 0.910, RFI = 917; IFI: 938; TLI = 0.903; CFI = 0.928; RMSEA = 0.0231; HOELTER = 1,353 (p < 0.05) and 406 (p < 0.01)], showing six factors with six items each: SRL, NRL, SDL, ERL, ENRL, EDRL, with acceptable standardized effects, factorial weights adjusted. See Table 11.
Reliability
The reliability of the total Scale showed adequate ratios (Cronbach Alpha = 0.902; Omega Index = 0.896). Split-half analysis (Alpha 1 = 0.805; Spearman–Brown Coefficient = 0.828; Guttman Split-half Coefficient = 0.828). Additionally, the values for the subscales were consistent: SR (Alpha = 0.864; Omega = 0.843); SNR (Alpha = 0.717; Omega = 0.701); SDR (Alpha = 0.818; Omega = 0.802); ER (Alpha = 0.845; Omega = 0.846); ENR (Alpha = 0.877; Omega = 0.853); EDR (Alpha = 0.900; Omega = 0.878).
External validity: Negative emotional reactivity
Formation of groups. The ANOVA carried out to form the groups showed a significant principal Group Factor effect for SR-ER relative to the total score for SR-ER [F(4.335) = 1185.439, p < 0.001; eta2 = 0.930, power = 1.00; post-hoc = 5 > 4 > 3 > 2 > 1, p < 0.001]. Levene’s test of error variance based on the mean showed the adequacy of the groups [L(4.355) = 2.430, p < 0.100]. See Table 6 for the descriptive statistics.
Effect of the SR-ER group on the level of Negative Emotional Reactivity. The ANOVA carried out showed a significant principal Group Factor effect for SR-ER relative to reactance [F(4.307) = 6.887, p < 0.001; eta2 = 0.08, power = 0.999; post-hoc = 5.4 > 4 > 3.2 > 2 > 1, p < 0.001]. Levene’s test of error variance based on the mean showed the adequacy of the groups [L(4.307) = 1.099, p < 0.357]. See Table 12 for the descriptive statistics.
Study 3. Self-regulatory vs. external regulatory learning behavior inventory in educational psychology contexts (SRL-ERL)
Descriptive results
The descriptive values found met the normality requirements to be expected of this type of sample. See Table 13.
Construct validity
Correlation
There was a significant negative correlation of SRL with NRL and DRL and a significant positive correlation of NRL and DRL. Across this context, the correlations are all in the same directions: negative for ERL with ENL and positive for ENL with EDL. Note also the positive and negative correlations between the components of SRL-ERL and the general SR construct. See Table 14.
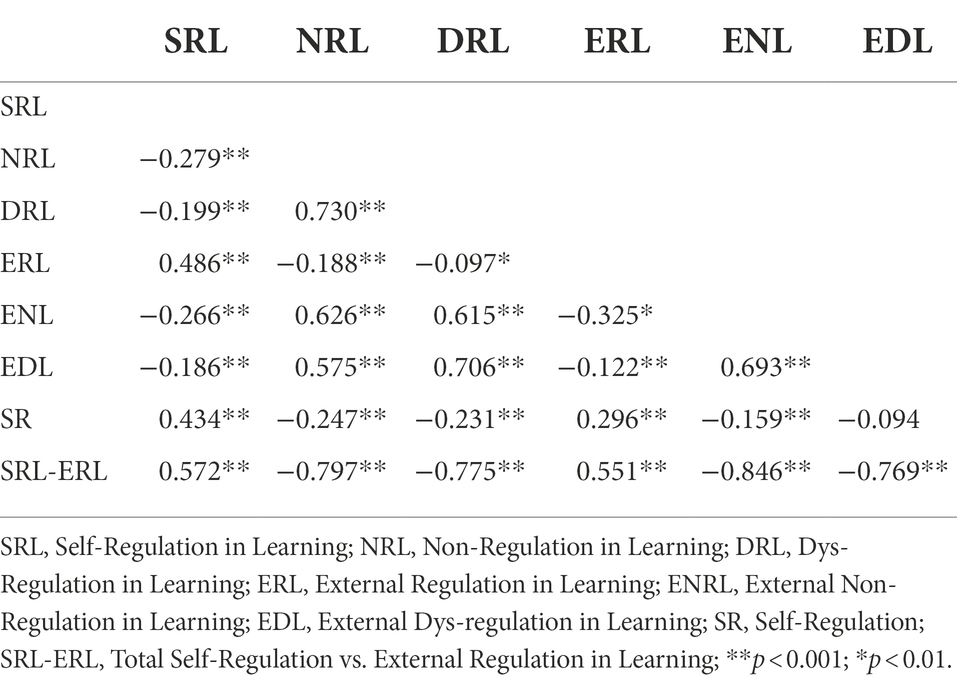
Table 14. Correlation between the types of internal and external regulation and the total score for the scale (n = 320).
Exploratory Factorial Analysis (EFA). This analysis was carried out with 50% of the sample, obtaining adjusted values: Kaiser–Meyer–Olkin = 0.888; Bartlett’s Sphericity Test (630) = 4,782,893 p < 0.001; factor communality was between 0.546 (item 6) and 0.831 (item 21). In the varimax rotation, six factors appeared that explained 67.00% of the variance: Factor 1, EDRL (15.92% variance) = items 34, 32, 33, 35, 31, 36; Factor 2, DRL (13.81% variance) = 15,13,16, 14, 17, 18; Factor 3, ERL (13.54%) = 20, 21, 24, 23, 22 19; Factor 4, SRL (12.27% variance) = 4,5,2, 3, 1, 6; Factor 5, ENRL (7, 41% variance) = 30, 29,28, 25, 26, 27; Factor 6, SNRL (4.19%) = 8,10,7, 9,11,12.
Confirmatory factor analysis
The structural values for this construct are acceptable [Chi-square = 1,598.384, df = (702–118) 584; Chi/df = 2,737; RMR = 0.0321; NFI = 0.967, RFI = 958; IFI: 918; TLI = 0.906; CFI = 0.917; RMSEA = 0.023; 1,334; 1,386], showing six components each containing six items (SRL, NRL, SDL, ERL, ENRL, EDRL), with consistent weights. See Table 7.
Reliability
The reliability of the total Scale showed adequate ratios (Cronbach Alpha = 0.881; Omega Index = 0.876). Split-half analysis showed adequate values (Alpha 1 = 0.781; Alpha 2 = 0.831; Spearman–Brown Coefficient = 0.787; Guttman Split-half Coefficient = 0.780). The reliability of the subscales also appeared to be acceptable: SRL (Alpha = 0.897; Omega = 0.886); SNL (Alpha = 0.753; Omega = 0.732); SDL (Alpha = 0.880; Omega = 0.821); ERL (Alpha = 0.940; Omega = 0.902); ENL (Alpha = 0.877; Omega = 0.851); EDL (Alpha = 0.922; Omega = 0.901).
External validity: Study achievement emotions
Formation of groups. The ANOVA carried out to form the groups showed a significant principal Group Factor effect for SRL-ERL relative to the total score for SRL-ERL [F(4.385) = 1,798.369, p < 0.001; eta2 = 0.949, power = 1.00; post-hoc = 5 > 4 > 3 > 2 > 1, p < 0.001]. Levene’s test of error variance based on the mean showed the adequacy of the groups [L(4.385) = 1.825, p < 0.100]. See Table 11 for the descriptive statistics.
Effect of the SRL-ERL group on the type and level of achievement emotion (during the study). The ANOVA carried out showed a significant principal effect of the SR-ER Group relative to academic achievement emotions during the study [F(32.1072) = 4.538, p < 0.001; eta2 = 0.119, power = 1.00]. Levene’s test of error variance based on the mean showed the adequacy of the groups [L(4.385) = 1.825, p < 0.157]. See Table 11 for the descriptive statistics. See Table 15.
Study 4. Self-regulatory vs. external regulatory behavior inventory in health psychology context (SRH-ERH)
Descriptive results
The descriptive values found met the normality requirements to be expected of this type of sample. See Table 16.
Construct validity
Correlations
There was significant negative correlation of SRH with NRH and DRH and significant positive correlation of NRH and DRH. Across this context, the correlations are consistent in direction: negative for ERH with ENH and positive for ENH with EDH. Note also the consistent negative and positive correlation of components of the scale with the SR and SR- ER constructs. See Table 17.
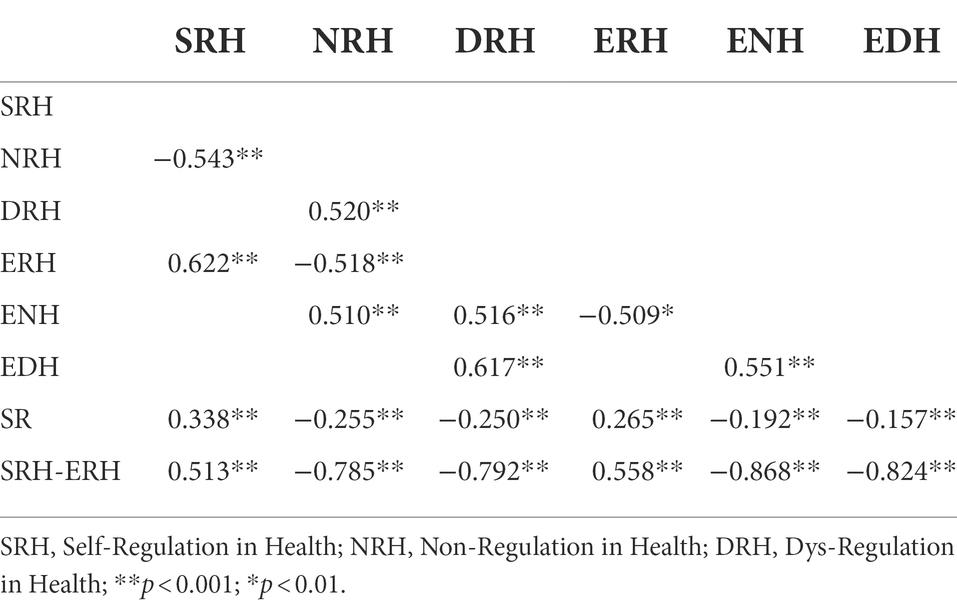
Table 17. Correlation between the types of internal and external regulation Health Psychology (n = 400).
Exploratory Factorial Analysis (EFA). This analysis was carried out with 50% of the sample, obtaining adjusted values: Kaiser–Meyer–Olkin = 0.892; Bartlett’s Sphericity Test (630) = 4, 459, 189 p < 0.001; factor communality was between 0.513 (item 6) and 0.842 (item 23). In the varimax rotation, six factors appeared that explained 70.04% of the variance: Factor 1, EDRH (21.60% variance) = items 34, 36, 35, 33, 31, and 30; Factor 2, ERH (14.97% variance) = 23, 20, 21, 22, 24, 19; Factor 3, SRH (11,24%) = 1, 3, 2, 4, 5, 6; Factor 4, DRH (10,81% variance) = 16, 15, 13, 17, 14, 18; Factor 5, NRH (7, 56% variance) = 9, 11, 7, 10, 12, 8; Factor 6, ENRH (4,19%) = 28, 26, 29, 25, 30, 31.
Factorial Confirmatory Structure. The structural values for this construction appeared to be acceptable [Chi-square = 1647.619, p < 0.001; df(702–118) = 584; CH/DF = 2,821; CFI = 0.958; GFI = 0.938; IFI = 0.926; TLI = 0.928; CFI = 0.926, RMSEA = 0.023; RSMR = 0.052; Hoelter = 1,294 (p < 0.05), 1,345 (p < 0.01)]. See Table 18.
Reliability
The total reliability of the scale showed adequate ratios (Cronbach Alpha = 0.897; Omega Index = 0.868). Split-half analysis showed adequate values (Alpha 1 = 0.790; Alpha 2 = 0.855; Spearman–Brown Coefficient = 0.837; Guttman Split-half Coefficient = 0.829). The reliability of the subscales also appeared to be acceptable: SRL (Alpha = 0.901; Omega = 0.888); SNL (Alpha = 0.785; Omega = 0.743); SDL (Alpha = 0.873; Omega = 0.852); ERL (Alpha = 0.950; Omega = 0.934); ENL (Alpha = 0.805; Omega = 0.794); EDL (Alpha = 0.939; Omega = 0.914).
External validity: Psychological well-being
Formation of groups. The ANOVA carried out to form the groups showed a significant principal Group Factor effect for SRH-ERH relative to the total score for SRH-ERH [F(4.315) = 1426.336, p < 0.001; eta2 = 0.948, power = 1.00; post-hoc = 5 > 4 > 3 > 2 > 1, p < 0.001]. Levene’s test of error variance based on the mean showed the adequacy of the groups [L(4.315) = 1.848, p < 0.119]. See Table 18 for the descriptive statistics.
Effect of the SRH-ERH group on the level of psychological well-being. The ANOVA carried out showed a significant principal Group Factor effect for SRH-ERH relative to the total score for psychological well-being. [F(4) = 22.295, p < 0.001; eta2 = 0.241, power = 1.00; post-hoc = 4.3 > 4 > 2.1 > 2 > 1, p < 0.001]. Levene’s test of error variance based on the mean showed the adequacy of the groups [L(4.281) = 1.788, p < 0.131]. See Table 19 for the descriptive statistics.
Study 5. Self-regulatory vs. external regulatory inventory in technology psychology contexts
Descriptive results
The descriptive values found met the normality requirements to be expected of this type of sample. See Table 20.
Construct validity
Correlations
There was a significant negative correlation of SRT with NRT and DRT and a significant positive correlation of NRT with DRT. Across this context, correlations were consistent in direction: negative between ERT and ENRT and positive between ENT and EDRT. Note also the consistent negative and positive correlation of components of the scale with the SR and SR-ERT constructs. See Table 21.
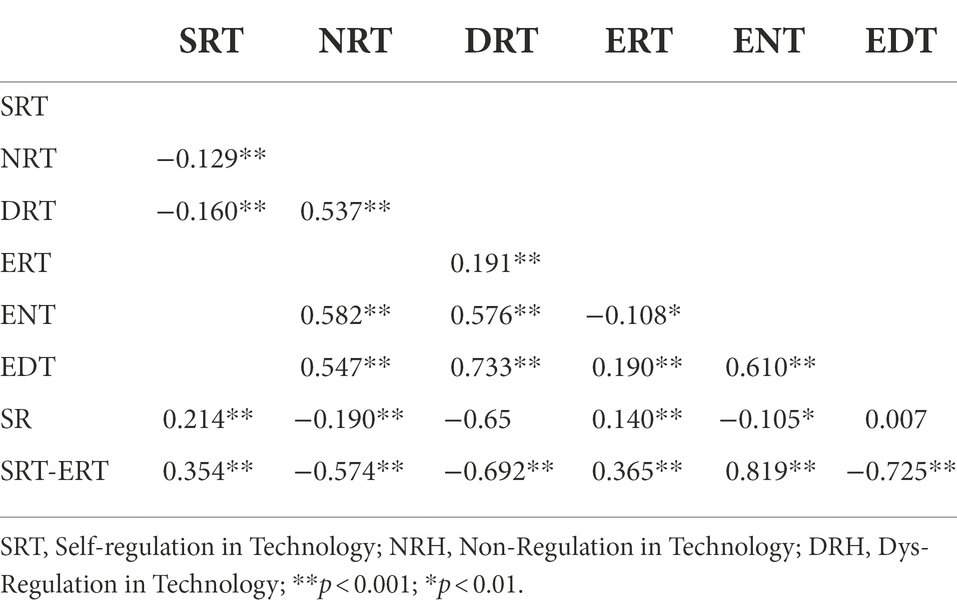
Table 21. Correlation between the types of internal and external regulation in Technology Psychology (n = 760).
Exploratory Factorial Analysis (EFA). This analysis was carried out with 50% of the sample, obtaining adjusted values: Kaiser–Meyer–Olkin = 0.852; Bartlett’s Sphericity Test (630) = 3,672,012 p < 0.001; factor communality was between 0.476 (item 9) and 0.843 (item 3). In the varimax rotation, six factors appeared that explained 68.75% of the variance: Factor 1, ERT (14.30% variance) = items 23, 21, 20, 24, 22, 19; Factor 2, EDRT (12.58% variance) = 34, 33, 35, 31, 36, 32; Factor 3, SRT (10.52%) = 3, 1, 2 4,5,6; Factor 4, DR (12.27% variance) = 16, 17,14, 15, 13, 18; Factor 5, ENRT (10.20% variance) = 28, 29, 25, 27, 26, 30; Factor 6, SNRT (7.16%) = 7, 11, 12, 9, 8, 10.
Confirmatory Factorial Structure. The structural values for this construct appeared to be acceptable [Chi-square = 1628.730, p < 0.001; df(702–118) = 584; CH/DF = 2.789; CFI = 0.927; GFI = 0.903; IFI = 0.926; TLI = 0.946; CFI = 0.926, RMSEA = 0.023; RSMR = 0.042; Hoelter = 1,309 (p < 0.05), 1,360 (p < 0.01)]. See Table 22.
Reliability
The total reliability of this scale showed adequate values (Cronbach Alpha 0.916; Omega = 0.885). Split-half analysis showed adequate values (Alpha 1 = 0.824; Alpha 2 = 0.882; Spearman–Brown Coefficient = 0.858; Guttman Split-half Coefficient = 0.850). The reliability of the subscales also appeared to be acceptable: SRT (Alpha = 0.881; Omega = 0.876); NRT (Alpha = 0.701; Omega = 0.683); DRT (Alpha = 0.858; Omega = 0.834); ERT (Alpha = 0.943; Omega = 0.925); ENT (Alpha = 0.865; Omega = 0.850); EDT (Alpha = 0.915; Omega = 0.901).
External validity: Impatience-hostility (TABP)
Formation of groups. The ANOVA carried out to form the groups showed a significant principal Group Factor effect for SRT-ERT relative to the total score for SRT-ERT [F(4.294) = 1008.857, p < 0.001; eta2 = 0.932, power = 1.00; post-hoc = 5 > 4 > 3 > 2 > 1, p < 0.001]. Levene’s test of error variance based on the mean showed the adequacy of the groups [L(4.296) = 1.749, p < 0.128]. See Table 7 for the descriptive statistics.
Effect of the SRT-ERT group on the level of Type A Behavior Pattern (TABP). The ANOVA carried out showed a significant principal effect of the SRT-ERT group relative to the total TABP score [F(4.252) = 1.527, p < 0.05; eta2 = 0.035, power = 0.660;], its dimensions [F(8.504) = 3.103, p < 0.001; eta2 = 0.064, power = 0.964; IH, F(4.252) = 4.702, p < 0.001; eta2 = 0.069, power = 1.00; post-hoc, 5.4 < 1, 2, p < 0.05] and its components [F(16,1,008) =2,121, p < 0.01; eta2 = 0.033, power = 0.973; IMP, F(4,252) = 4.211, p < 0.001; eta2 = 0.063, power = 1.00; post-hoc, 5.4 < 1, 2, 3, p < 0.05]. Levene’s test of error variance based on the mean showed the adequacy of the groups [L(4.225) = 1.788, p < 0.199]. See Table 23 for the descriptive statistics.
Discussion
The results obtained provide support for these instruments, and the hypotheses proposed in relation to the instrument presented based on the SR-ER Theory model (de la Fuente, 2017, 2021). Hypothesis 1, relating to the demonstration of a stable, valid structure with six components inherent to the theoretical model and common to the different versions of the questionnaire has demonstrated empirical adequacy. It has also been empirically shown that the SR-ER construction allows scores to be ordered in a continuum of the combined scores for the SR-NR-DR (self-regulation) and ER-ENR-EDR (external regulation) components that make up the Scale in its different versions. The reliability and validity results are similar to those found previously with other samples (de la Fuente et al., 2021b; Pachón-Basallo et al., 2021). However, the design of Item 8 of the De-Regulation Scale should be reviewed since it appears to have a lower level of reliability. Future research will allow us to better adapt to the situations of different users in different contexts.
The total score, as an aggregate averaged continuum of Self-Regulation and External Regulation, has allowed the level of regulation in the behavior of a given person to be placed on a conceptual continuum from +1 to −1, as envisaged by the model whereby moving toward +1 represents increasing average regulation and moving toward −1 represents increasing dysregulation. Those scores could be used in practice to assess the degree of personal and contextual regulation of each person in a given environment. Future research should determine the connection between this construct and other more classical constructs in the area of the regulatory difficulties and problems inherent to different pathologies. Some recent studies have suggested that the dysregulatory level of subjects is an essential and predictive element in psychiatric pathologies (Billen et al., 2021; Levin-Aspenson et al., 2021); however, those studies have not explicitly addressed the dysregulatory effect of context, which remains to be determined.
Empirical support has also been established for Hypothesis 2, that the different versions of the instrument would have adequate construct validity and reliability with sufficient discriminant power or external validity with respect to different constructs of relevance in each field (clinical, educational, health and technology). The same consistent factorial structure with six factors appeared in all versions of the instrument, which can be interpreted as demonstrating factorial invariance (Meredith, 1993).
The relationship between the Self-Regulation, Non-Regulation, and Dys-Regulation constructs was also consistent across the different contexts, giving a stable relationship between Self-Regulation, Non-Regulation, and Dys-Regulation behaviors, both personal (self-regulated) and contextual (externally regulated). We believe that the ability to distinguish these three types or levels of behavioral regulation is of interest in itself given the behavioral continuum in which they are situated. In addition, we have established that it is possible to externally validate each version of the instrument through a continuous regulation heuristic of person-context combinations (with five levels), that has sufficient explanatory power to determine the variability of the different dependent variables analyzed in each context: clinical (negative emotional reactivity), educational (study achievement emotions), health (psychological well-being) and technology psychology (impatience-hostility). The consistency found allows us to infer the external convergence validity of the different scales.
A limitation of this work relates to the inconsistency described in the measurement of Item 8, which has now been amended. However, a strength of this work is that the instruments have been translated into other languages. Subsequent research should focus on validation of the instruments with samples from different countries and cultures as a form of transfer of the instrument and the inherent theoretical model and demonstration of factorial invariance, required as part of that process of validation.
Conclusion
These results support the hypothesis of the types of behavioral regulation—internal and external—proposed by SR vs. ER Behavior Theory (de la Fuente, 2021b). As such, they contribute to advance the operational definition of such behavior in the three behavioral level types (SR-NR-DR) and contexts (ER-ENR-EDR). These new constructs and the possibility of measuring them will allow us to detect new behavioral realities and to advance the understanding of the role and effect of personal behavior in its interaction with the environment.
Implications
The development of these versions of the SR-ER evaluation instrument (de la Fuente, 2020d,e) provides a tool to validate associations between the different levels or types of regulatory behavior, personal and contextual, in different psychological contexts. In addition, it is a step forward in the conceptualization of the typologies of self-regulatory behavior (which can be measured) in relation to other dependent variables measured.
This new model and these new scales have many academic and professional implications. In the academic sphere, the model will allow the determination of new theoretical and empirical relationships in a continuum of human behavior by confirming the connection between the three levels of self-regulation factors (internal and external). The model will allow the transdiagnostic transition between the three levels of self-regulation proposed from the positive or protective (self-regulation) to the negative or risk level (dysregulation). As such, this analytical framework will help to behaviorally operationalize the p factor in a transdiagnostic way as recently proposed in the field of psychiatry (Kaminski et al., 2022; Smith, 2022). The research agenda for those lines of investigation has recently been laid out as it applies to different fields of Psychology (de la Fuente et al., 2022a). It is also important in the professional arena because the model and the scales allow assessment of the levels of personal and contextual regulation of an individual in a given psychological context. It represents significant progress because it allows contextualization of personal and contextual regulatory factors in interaction (to give a general regulation score). This transcends a purely clinical perspective focused on personality-based factors to explain a given psychopathological behavior. The model also allows assessment and then intervention with knowledge of an individual’s specific behavioral momentum and its development in a particular regulatory direction: SR→NR→DR; SR←NR←DR; ER→ENR→EDR; ER←ENR←EDR.
This development is of theoretical and applied interest, because it supports the use of the concepts of regulation (R), non-regulation (NR), and dys-regulation (DR) which thus far have not been brought together in a coherent theoretical and applied continuum. As such, it opens the door to the exploration of assessment and intervention in different fields:
1. In the professional and academic field of Clinical Psychology, the categorization derived from this instrument (SR-NR-DR; ER-ENR-EDR) allows different types of potentially pathological behavior and contexts to be accurately determined. It is assumed that the different levels of self-regulation and external regulation may imply different types of behavioral dysfunction associated with levels of regulation through the p factor, as shown by psychiatric research (Billen et al., 2021; Levin-Aspenson et al., 2021).
2. In the professional and academic field of Educational Psychology, the existence of these new constructs (SR-NR-DR; ER-ENR-EDR) can help us to understand the factors that regulate the learning processes and the teaching context. Thus, psycho-educational intervention strategies can be based on assessment, evaluation, and intervention in both components of the teaching-learning process.
3. In the professional and academic field of Health Psychology, measurement along this continuum (SR-NR-DR; ER-ENR-EDR) will allow us to determine the profiles of individuals who require support and the contexts that promote or do not promote healthful behaviors.
4. In the professional and academic field of the Psychology of Use of Technology, measurement along this continuum (SR-NR-DR; ER-ENR-EDR) will allow us to more accurately identify maladjusted behaviors and maladjustive contexts associated with the use of technology at university.
However, there are as-yet unexplored fields to which the theoretical model can be applied, and for which tailored measurement instruments can be developed. Areas of intervention such as Organizational Psychology, Forensic Psychology, Sports Psychology, Psychology of Risk and Catastrophe, Traffic Psychology, and Aviation Psychology could be enriched by these contributions.
Data availability statement
The raw data supporting the conclusions of this article will be made available by the authors, without undue reservation.
Ethics statement
This study was reviewed and approved by Comité de Ética de la Investigación, University of Navarra (ref. 2018.170). The patients/participants provided their written informed consent to participate in this study.
Author contributions
JF and MP-B: R&D Project, idea, design, analysis, and initial writing. JM-V and FP-S: R&D Project, data collection, and revision of the draft. AG-U and PS: review of the final version in English. All authors contributed to the article and approved the submitted version.
Funding
This research was supported by R&D Project PGC2018-094672-B-I00, University of Navarra, Ministry of Education and Science (Spain), and the European Social Fund (EU); R&D Project UAL18- SEJ-DO31-A-FEDER. University of Almería (Spain), and the European Social Fund (EU) (www.inetas.net).
Conflict of interest
The authors declare that the research was conducted in the absence of any commercial or financial relationships that could be construed as a potential conflict of interest.
Publisher’s note
All claims expressed in this article are solely those of the authors and do not necessarily represent those of their affiliated organizations, or those of the publisher, the editors and the reviewers. Any product that may be evaluated in this article, or claim that may be made by its manufacturer, is not guaranteed or endorsed by the publisher.
Supplementary material
The Supplementary material for this article can be found online at: https://www.frontiersin.org/articles/10.3389/fpsyg.2022.922633/full#supplementary-material
Footnotes
References
Akfırat, S., Gül, A., and Yetim, Ü. (2016). Interrelations among regulation focus, authenticity and emotional responses to frustration of self-related experiences among university students in Turkey. Curr. Psychol. 35, 99–107. doi: 10.1007/s12144-015-9370-6
Alonso-Tapia, J., Abello, D. M., and Panadero, E. (2020). Regulating emotions and learning motivation in higher education students. Int. J. Emot. Educat. 12, 73–89.
Amate-Romera, J. A., and de la Fuente, J. (2021). Relationships between test anxiety, self-regulation and strategies for coping with stress, in professional examination candidates. Anales de Psicología/Annals of Psychology 37, 276–286. doi: 10.6018/analesps.411131
Azevedo, R., and Feyzi-Behnagh, R. (2011). Dysregulated learning with advanced learning technologies. J. e-Learn. Knowled. Soc. 7, 9–18.
Azevedo, R., Moos, D. C., Winters, F. I., Greene, J. A., Cromley, J., Olson, E., et al. (2005). “Why is externally-regulated learning more effective than self-regulated learning with hypermedia?” in Artificial Intelligence in Education: Supporting Learning through Intelligent and Socially Informed Technology. eds. C.-K. Looi, G. McCalla, B. Bredeweg, and J. Breuker (Amsterdam, The Netherlands: IOS Press), 41–48.
Bandura, A. (1991). Social cognitive theory of self-regulation. Organ. Behav. Hum. Decis. Process. 50, 248–287. doi: 10.1016/0749-5978(91)90022-L
Beauchaine, T. P., and Crowell, S. E. (Eds.). (2020). The Oxford Handbook of Emotion Dysregulation. New York, NY: Oxford University Press.
Becerra, R., Preece, D., Campitelli, G., and Scott-Pillow, G. (2017). The assessment of emotional reactivity across negative and positive emotions: development and validation of the Perth emotional reactivity scale (PERS). Assessment 26, 867–879. doi: 10.1177/1073191117694455
Becker, D. R., Miao, A., Duncan, R., and McClelland, M. M. (2014). Behavioral self-regulation and executive function both predict visuomotor skills and early academic achievement. Early Child. Res. Q. 29, 411–424. doi: 10.1016/j.ecresq.2014.04.014
Bermúdez, J., Pérez-García, A. M., and Sánchez-Elvira, A. (1990). Type-a behavior pattern and attentional performance. Personal. Individ. Differ. 11, 13–18. doi: 10.1016/0191-8869(90)90163-L
Bermúdez, J., Sánchez-Elvira, A., and Pérez-García, A. M. (1991). Medida del patrón de conducta type-a en muestras españolas: Datos psicométricos del JAS Para estudiantes. [Measuring the type-a behavior pattern in Spanish samples. Psychometric data from the JAS for students]. Boletín de Psicología 31, 41–77.
Bernardo, A., Esteban, M., Cervero, A., Cerezo, R., and Herrero, F. J. (2019). The influence of self-regulation behaviors on university students’ intentions of persistence. Front. Psychol. 10:2284. doi: 10.3389/fpsyg.2019.02284
Billen, E., Garofalo, C., and Bogaerts, S. (2021). Self-regulation all bass-Ackwards: similarities and differences in component structure in community and forensic psychiatric populations. Psychol. Assessm. 34, 247–260. doi: 10.1037/pas0001089
Blair, C., and Raver, C. C. (2015). School readiness and self-regulation: a developmental psychobiological approach. Annu. Rev. Psychol. 66, 711–731. doi: 10.1146/annurev-psych-010814-015221
Blood, R. A. (2012). The relationships among self-regulation, executive functioning, coping resources, and symptomatology resulting from a traumatic event doctoral dissertation. Atlanta, GA: Georgia State University).
Boekaerts, M., Zeidner, M., and Pintrich, P. R. (Eds.). (1999). Handbook of Self-Regulation. San Diego, CA: Elsevier.
Brown, J. M. (1998). “Self-regulation and the addictive behaviors” in Applied Clinical Psychology. Treating addictive behaviors. eds. W. R. Miller and N. Heather (New York: Plenum Press), 61–73.
Carbone, J. T. (2020). Allostatic load and mental health: a latent class analysis of physiological dysregulation. Stress 24, 394–403. doi: 10.1080/10253890.2020.1813711
Carver, C. S., and Scheier, M. F. (1981). Self-consciousness and reactance. J. Res. Pers. 15, 16–29. doi: 10.1016/0092-6566(81)90003-9
Chen, C., Zhang, K. Z., Gong, X., Lee, M. K., and Wang, Y. V. (2021). Preventing relapse to information technology addiction through weakening reinforcement: a self-regulation perspective. Inf. Manag. 58:103485. doi: 10.1016/j.im.2021.103485
Choi, J. H., and Abbott, S. M. (2020). “The impact of shift work and circadian dysregulation on Women’s health,” in Sleep Disorders in Women. ed. T. H. Mukundan (Cham: Humana), 159–176.
Clark, N. M., and Zimmerman, B. J. (1990). A social cognitive view of self-regulated learning about health. Health Educ. Res. 5, 371–379. doi: 10.1093/her/5.3.371
Claudine, C. M., Zhang, Y., and Wang, Z. (2021). Early parental loss in childhood and depression in adults: a systematic review and meta-analysis of case-controlled studies. J. Affect. Disord. 260, 272–280. doi: 10.1016/j.jad.2019.07.087
de la Fuente, J. (2017). Theory of self- vs. externally- regulated learning™: fundamentals, evidence, and applicability. Front. Psychol. 8:1675. doi: 10.3389/fpsyg.2017.01675
de la Fuente, J. (2021a). A path analysis model of protection and risk factors for university academic stress: analysis and psychoeducational implications for the COVID-19 emergency. Front. Psychol. 12:562372. doi: 10.3389/fpsyg.2021.562372
de la Fuente, J. (2021b). Self- vs. Externally- Regulated Behavior Theory in Psychological Contexts. Pamplona: University of Navarra Manuscript Awaiting Publication.
de la Fuente, J. (2022). Self- vs. externally-regulation behavior scales. Madrid: Industrial Property Registration (RPI) n° 765-688472 (2022/02/07) (see COMPLEMENTARY MATERIAL).
de la Fuente, J., Amate, J., González-Torres, M. C., Artuch, R., García-Torrecillas, J. M., and Fadda, S. (2020a). Effects of levels of self-regulation and regulatory teaching on strategies for coping with academic stress in undergraduate students. Front. Psychol. 11:22. doi: 10.3389/fpsyg.2020.00022
de la Fuente, J., and Eissa, M. A. (2010). International Handbook on Applying Self-Regulated Learning in Different Settings. Almería (Spain): Education & Psychology I+ D+ i, e-Publishing Series.
de la Fuente, J., González-Torres, M. C., Aznárez-Sanado, M., Martínez-Vicente, J. M., Peralta-Sánchez, F. J., and Vera, M. M. (2019a). Implications of unconnected micro, molecular, and molar level research in psychology: the case of executive functions, self-regulation, and external regulation. Front. Psychol. 10:1919. doi: 10.3389/fpsyg.2019.01919
de la Fuente, J., Martínez-Vicente, J. M., Pachón-Basallo, M., Peralta-Sánchez, F. J., Vera-Martínez, M. M., and Andrés-Romero, M. (2022b). Differential predictive effect of self-regulation and the combination self- vs. external-regulation regarding executive functions and emotional regulation difficulties, in university students. Front. Psychol. 13:876292. doi: 10.3389/fpsyg.2022.876292
de la Fuente, J., Martínez-Vicente, J. M., Peralta-Sánchez, F. J., Garzón-Umerenkova, A., Vera, M. M., and Paoloni, P. (2019b). Applying the SRL vs. ERL theory to the knowledge of achievement emotions in undergraduate university students. Front. Psychol. 10:2070. doi: 10.3389/fpsyg.2019.02070
de la Fuente, J., Martínez-Vicente, J. M., Santos, F., Sander, P., Fadda, S., Karagiannopoulou, A., et al. (2022a). Contributions of the SR vs. ER behavior theory™ to different contexts of psychology: implications for a new research agenda (a tribute to Albert E. Bandura). Front. Psychol. 13.
de la Fuente, J., Paoloni, P., Kauffman, D., Yilmaz Soylu, M., Sander, P., and Zapata, L. (2020b). Big five, self-regulation, and coping strategies as predictors of achievement emotions in undergraduate students. Int. J. Environ. Res. Public Health 17:3602. doi: 10.3390/ijerph17103602
de la Fuente, J., Paoloni, P. V., Vera-Martínez, M. M., and Garzón-Umerenkova, A. (2020c). Effect of levels of self-regulation and situational stress on achievement emotions in undergraduate students: class, study and testing. Int. J. Environ. Res. Public Health 17, 1–16. doi: 10.3390/ijerph17124293
de la Fuente, J., Peralta-Sánchez, F. J., Martínez-Vicente, J. M. V., Sander, P., Garzón-Umerenkova, A., and Zapata, L. (2020d). Effects of self- vs. external regulation on the factors and symptoms of academic stress in undergraduate students. Front. Psychol. 17. doi: 10.3390/ijerph17124293
de la Fuente, J., Sander, P., Garzón-Umerenkova, A., Vera-Martínez, M. M., Fadda, S., and Gaetha, M. L. (2021c). Self-regulation and regulatory teaching as determinants of academic behavioral confidence and procrastination in undergraduate students. Front. Psychol. 12:602904. doi: 10.3389/fpsyg.2021.602904
de la Fuente, J., Sander, P., Kauffman, D., and Yilmaz Soylu, M. (2020e). Differential effects of self- vs. external-regulation on learning approaches, academic achievement, and satisfaction in undergraduate students. Front. Psychol. 11:543884. doi: 10.3389/fpsyg.2020.543884
de la Fuente, J., Sander, P., Martínez-Vicente, J. M., Vera, M. M., Garzón, A., and Fadda, S. (2017). Combined effect of levels in personal self-regulation and regulatory teaching on meta-cognitive, on meta-motivational, and on academic achievement variables in undergraduate students. Front. Psychol. 8:232. doi: 10.3389/fpsyg.2017.00232
de la Fuente, J., Santos, F. H., Garzón-Umerenkova, A., Fadda, S., Solinas, G., and Pignata, S. (2021b). Cross-sectional study of resilience, positivity and coping strategies as predictors of engagement-burnout in undergraduate students: implications for prevention and treatment in mental well-being. Front. Psychiatry. 12:596453. doi: 10.3389/fpsyt.2021.596453
de la Fuente, J., Zapata, L., Martínez-Vicente, J. M., Sander, P., and Putwain, D. (2015). “Personal self-regulation, self-regulated learning and coping strategies, in university context with stress” in Metacognition: Fundaments, Applications, and Trends a Profile of the Current State-of-the-Art. ed. E. Peña-Ayala (Cham: Springer International Publishing Switzerland), 223–250.
Díaz, D., Rodríguez-Carvajal, R., Blanco, A., Moreno-Jiménez, B., Gallardo, I., Valle, C., et al. (2006). Adaptación española de las escalas de bienestar psicológico de Ryff. Psicothema 18, 572–577.
Dinsmore, D., Fryer, L., and Parkinson, M. (eds.). (2020). Handbook of Strategies and Strategic Processing. New York: Routledge
Duncan, J., Emslie, H., Williams, P., Johnson, R., and Freer, C. (1996). Intelligence and the frontal lobe: the organization of goal-directed behavior. Cogn. Psychol. 30, 257–303. doi: 10.1006/cogp.1996.0008
Escobar-Pérez, J., and Cuervo-Martínez, A. (2008). Validez de contenido y juicio de expertos: una aproximación a su utilización [Content validity and expert judgment: an approach to its use]. Avances en medición. 6, 27–36.
Forkus, S. R., Rosellini, A. J., Monteith, L. L., Contractor, A. A., and Weiss, N. H. (2020). Military sexual trauma and alcohol misuse among military veterans: the roles of negative and positive emotion dysregulation. Psychol. Trauma Theory Res. Pract. Policy 12, 716–724. doi: 10.1037/tra0000604
Garzón-Umerenkova, A., de la Fuente, J., Martínez-Vicente, J. M., Zapata, L., Pichardo, M. C., and García-Berbén, A. B. (2017). Validation of the Spanish Short Self-Regulation Questionnaire (SSSRQ) through Rasch Analysis. Front. Psychol. 8:276. doi: 10.3389/fpsyg.2017.00276
Goldman, N., Turra, C. M., Glei, D. A., Lin, Y. H., and Weinstein, M. (2006). Physiological dysregulation and changes in health in an older population. Exp. Gerontol. 41, 862–870. doi: 10.1016/j.exger.2006.06.050
Gouin, J. P. (2011). Chronic stress, immune dysregulation, and health. Am. J. Lifestyle Med. 5, 476–485. doi: 10.1177/1559827610395467.M
Guido, H. E., Tops, M., and Koole, S. L. (Eds.) (2015). Handbook of Biobehavioral Approaches to Self-Regulation. New York, NY: Springer
Hennessy, E. A., Johnson, B. T., Acabchuk, R. L., McCloskey, K., and Stewart-James, J. (2020). Self-regulation mechanisms in health behavior change: a systematic meta-review of meta-analyses, 2006–2017. Health Psychol. Rev. 14, 6–42. doi: 10.1080/17437199.2019.1679654
Höhn, C., Metzner, G., Waldeck, E., and Glattacker, M. (2020). Contextual factors of self-regulation in adolescent medical rehabilitation patients—a qualitative study. Mapping Intimacies. doi: 10.21203/rs.3.Rs-15587/v1
Hoyle, R. H. (2010). “Personality and self-regulation” in Handbook of Personality and Self-Regulation. ed. R. H. Hoyle, vol. 1 (Hoboken, NJ: Wiley-Blackwell), 18.
Huffhines, L., Gusler, S., and Jackson, Y. (2020). Adversity exposure and obesogenic food consumption in young children: the transgenerational role of emotion dysregulation. Pediatric. Obesity 15:e12658. doi: 10.1111/ijpo.12658
Hull, J. G., and Slone, L. B. (2004). “Alcohol and self-regulation” in Handbook of Self-Regulation: Research, Theory, and Applications. eds. R. F. Baumeister and K. D. Vohs (New York, NY: The Guilford Press), 466–491.
Inzlicht, M., Werner, K. M., Briskin, J. L., and Roberts, B. W. (2021). Integrating models of self-regulation. Annu. Rev. Psychol. 72, 319–345. doi: 10.1146/annurev-psych-061020-105721
Jacqueline, B., Stefaniak, J., and Bol, L. (2020). An examination of personality traits as a predictor of the use of self-regulated learning strategies and considerations for online instruction. Educ. Technol. Res. Dev. 68, 2659–2683. doi: 10.1007/s11423-020-09797-y
Kaminski, A., You, X., Flaharty, K., Jeppsen, C., Li, S., Merchant, J. S., et al. (2022). Cingulate-prefrontal connectivity during dynamic cognitive control mediates association between P-factor and adaptive functioning in a transdiagnostic pediatric sample. Biol. Psychiat, in press. Pre-prof.
Karoly, P. (1993). Mechanisms of self-regulation: a systems view. Annu. Rev. Psychol. 44, 23–52. doi: 10.1146/annurev.ps.44.020193.000323
Khan, N. A., Khan, A. N., and Moin, M. F. (2021). Self-regulation and social media addiction: a multi-wave data analysis in China. Technol. Soc. 64:101527. doi: 10.1016/j.techsoc.2021.101527
Koopmann, J., Johnson, R., Wang, M., Lanaj, K., and Wang, G. (2019). A self-regulation perspective on how and when regulatory focus differentially relates to citizenship behaviors. J. Appl. Psychol. 104, 629–641. doi: 10.1037/apl0000366
Krantz, D. S., Glass, D. C., and Snyder, M. L. (1974). Helplessness, stress level, and the coronary-prone behavior pattern. J. Exp. Soc. Psychol. 10, 284–300.
Kuss, D. J., Griffiths, D., Karila, L. M., and Billieux, J. (2014). Internet addiction: a systematic review of epidemiological research for the last decade. Curr. Pharm. Des. 20, 4026–4052. doi: 10.2174/13816128113199990617
Lajoie, S. P., Zheng, J., Li, S., Jarrell, A., and Gube, M. (2021). Examining the interplay of affect and self regulation in the context of clinical reasoning. Learn. Instr. 72:101219. doi: 10.1016/j.learninstruc.2019.101219
Leerkes, E. M., Su, J., and Sommers, S. A. (2020). Mothers’ self-reported emotion dysregulation: a potentially valid method in the field of infant mental health. Infant Ment. Health J. 41, 642–650. doi: 10.1002/imhj.21873
Lerner, J. V., Wong, C. A., Weiner, M. B., and Johnson, S. K. (2021). Profiles of adolescent character attributes: associations with intentional self-regulation and character role model relationships. J. Moral Educ. 50, 293–316. doi: 10.1080/03057240.2020.1755242
Levin-Aspenson, H. F., Watson, D., Clark, L. A., and Zimmerman, M. (2021). What is the general factor of psychopathology? Consistency of the p factor across samples. Assessment 28, 1035–1049. doi: 10.1177/1073191120954921
Li, H. (2021). Working memory depletion affects intertemporal choice among internet addicts and healthy controls. Front. Psychol. 12:675059. doi: 10.3389/fpsyg.2021.675059
Malanchini, M., Engelhardt, L. E., Grotzinger, A. D., Harden, K. P., and Tucker-Drob, E. (2019). “Same but different”: associations between multiple aspects of self-regulation, cognition, and academic abilities. J. Pers. Soc. Psychol. 117, 1164–1188. doi: 10.1037/pspp0000224
Mann, T., De Ridder, D., and Fujita, K. (2013). Self-regulation of health behavior: social psychological approaches to goal setting and goal striving. Health Psychol. 32, 487–498. doi: 10.1037/a0028533
Maya, T. (2020). Effortful emotion regulation as a unique form of cybernetic control. Perspect. Psychol. Sci. 16, 94–117. doi: 10.1177/1745691620922199
Meredith, W. (1993). Measurement invariance, factor analysis and factorial invariance. Psychometrika 58, 525–543. doi: 10.1007/BF02294825
Mithaug, D. E. (1993). Self-Regulation Theory: How Optimal Adjustment Maximizes Gain. Westport, CT: Praeger Publishers/Greenwood Publishing Group.
Pachón-Basallo, M., de la Fuente, J., and Gonzáles-Torres, M. C. (2021). Regulation/non-regulation/dys-regulation of health behavior, psychological reactance, and health of university undergraduate students. Int. J. Environ. Res. Public Health 18:3793. doi: 10.3390/ijerph18073793
Panadero, E. (2017). A review of self-regulated learning: six models and four directions for research. Technol. Soc. 8:422. doi: 10.3389/fpsyg.2017.00422
Pandey, A., Hale, D., Das, S., Goddings, A. L., Blakemore, S. J., and Viner, R. M. (2018). Effectiveness of universal self-regulation–based interventions in children and adolescents: a systematic review and meta-analysis. JAMA Pediatr. 172, 566–575. doi: 10.1001/jamapediatrics.2018.0232
Peña-Lara, A. (ed.) (2015). Metacognition: Fundaments, Applications, and Trends a Profile of the Current State-of-the-Art. Cham: Springer International Publishing Switzerland.
Pervin, L. A. (1988). Personalidad: Controversias, problemas e tendencias actuales. Psiquiatria y Psicología Humanista 19, 73–99.
Pichardo, C., Justicia, F., de la Fuente, J., Martínez-Vicente, J. M., and Berbén, A. B. (2014). Factor structure of the self-regulation questionnaire (SRQ) at Spanish Universities. Span. J. Psychol. 17:e62. doi: 10.1017/sjp.2014.63
Quinn, P. D., and Fromme, K. (2010). Self-regulation as a protective factor against risky drinking and sexual behavior. Psychol. Addict. Behav. 24, 376–385. doi: 10.1037/a0018547
Rathnayake, L. C., and Chandradasa, M. (2020). Emotional and behavioural dysregulation in children of health care workers in the frontline of COVID-19 response in Sri Lanka. Sri Lanka J. Psychiat. 11, 58–60. doi: 10.4038/sljpsyc.v11i1.8236
Robson, D. A., Allen, M. S., and Howard, S. J. (2020). Self-regulation in childhood as a predictor of future outcomes: a meta-analytic review. Psychol. Bull. 146, 324–354. doi: 10.1037/bul0000227
Romer, A. L., Hariri, A. R., and Strauman, T. J. (2021). Regulatory focus and the p factor: evidence for self-regulatory dysfunction as a transdiagnostic feature of general psychopathology. J. Psychiatr. Res. 137, 178–185. doi: 10.1016/j.jpsychires.2021.02.051
Rubio, D. M., Berg-Weber, M., Tebb, S. S., Lee, E. S., and Rauch, S. (2003). Objectifying content validity: conducting a content validity study in social work research. Soc. Work. Res. 27, 94–104. doi: 10.1093/swr/27.2.94
Ryff, C. (1989). Happiness is everything, or is it? Explorations on the meaning of psychological well-being. J. Pers. Soc. Psychol. 57, 1069–1081. doi: 10.1037/0022-3514.57.6.1069
Smith, G. T., Atkinson, E. A., Davis, H. A., Riley, E. N., and Oltmanns, J. R. (2020). The general factor of psychopathology. Annu. Rev. Clin. Psychol. 16, 75–98. doi: 10.1146/annurev-clinpsy-071119-115848
Solé-Ferrer, N., Mumbardó-Adam, C., Company-Romero, R., Balmaña-Gelpíl, N., and Sergi Corbella-Santomà, S. (2019). Instrumentos de evaluación de la autorregulación en población infanto-juvenil: una revisión sistemática [Instruments for evaluating self-regulation in children and adolescents: a systematic review]. Revista de Psicología Clínica con Niños y Adolescentes 6, 36–43. doi: 10.21134/rpcna.2019.06.2.5
Tabachnick, B. G., and Fidell, L. S. (2001). Using multivariate statistics. 4th Edn. Boston: Allyn & Bacon.
Thakre, N., and Sebastian, S. (2021). The role of perfectionism on self regulation and defensive pessimism at workplace. J. Psychosoc. Res. 16, 75–84. doi: 10.32381/JPR.2021.16.01.8
Valikhani, A., Mokaberian, M., Rahmati, L., and Moustafa, A. A. (2020). Dimensional investigation of individual differences in personality disorder traits based on the three-dimensional model of personality self-regulation. Curr. Psychol. 41, 5163–5175. doi: 10.1007/s12144-020-01031-5
Vega, D., Torrubia, R., Marco-Pallarés, J., Soto, A., and Rodriguez-Fornells, A. (2020). Metacognition of daily self-regulation processes and personality traits in borderline personality disorder. J. Affect. Disord. 267, 243–250. doi: 10.1016/j.jad.2020.02.033
Vohs, K. D., and Baumeister, R. F. (Eds.). (2016). Handbook of Self-Regulation. Research, Theory, and Applications. New York, NY: Guilford Publications.
Wilson, T. E., Hennessy, E. A., Falzon, L., Boyd, R., Kronish, I. M., and Birk, J. L. (2020). Effectiveness of interventions targeting self-regulation to improve adherence to chronic disease medications: a meta-review of meta-analyses. Health Psychol. Rev. 14, 66–85. doi: 10.1080/17437199.2019.1706615
Wrosch, C., Scheier, M. F., Miller, G. E., Schulz, R., and Carver, C. S. (2003). Adaptive self-regulation of unattainable goals: goal disengagement, goal reengagement, and subjective well-being. J. Pers. Soc. Psychol. 29, 1494–1508. doi: 10.1177/0146167203256921
Zimmerman, B. J. (2000). “Attaining self-regulation: a social cognitive perspective,” in Handbook of Self-Regulation. eds. M. Boekaerts, P. R. Pintrich, and M. Zeidner (London: Academic Press), 13–39.
Zimmerman, B. J., Bonner, S., Evans, D., and Mellins, R. B. (1999). Self-regulating childhood asthma: a developmental model of family change. Health Educ. Behav. 26, 55–71. doi: 10.1177/109019819902600106
Zimmerman, B. J., and Labuhn, A. S. (2012). “Self-regulation of learning: process approaches to personal development,” in APA Educational Psychology Handbook. eds. K. R. Harris, S. Graham, T. Urdan, C. B. McCormick, G. M. Sinatra, and J. Sweller (American Psychological Association), Vol 1, 399–425.
Keywords: self-regulated behavior/context, non-regulated behavior/context, dys-regulated behavior/context, validation, self- vs. external-regulation theory
Citation: de la Fuente J, Pachón-Basallo M, Martínez-Vicente JM, Peralta-Sánchez FJ, Garzón-Umerenkova A and Sander P (2022) Self- vs. External-Regulation Behavior ScaleTM in different psychological contexts: A validation study. Front. Psychol. 13:922633. doi: 10.3389/fpsyg.2022.922633
Edited by:
Rosario Cabello, University of Malaga, SpainReviewed by:
Salvatore Fadda, University of Sassari, ItalyAnita Habók, University of Szeged, Hungary
Copyright © 2022 de la Fuente, Pachón-Basallo, Martínez-Vicente, Peralta-Sánchez, Garzón-Umerenkova and Sander. This is an open-access article distributed under the terms of the Creative Commons Attribution License (CC BY). The use, distribution or reproduction in other forums is permitted, provided the original author(s) and the copyright owner(s) are credited and that the original publication in this journal is cited, in accordance with accepted academic practice. No use, distribution or reproduction is permitted which does not comply with these terms.
*Correspondence: Jesús de la Fuente Arias, amRsZnVlbnRlQHVuYXYuZXM=