- College of General Education, Kookmin University, Seoul, South Korea
Nonprofit organizations (NPO) can utilize social networking sites (SNSs) for their activities. Like individual users, they can create SNS accounts, upload posts to show what they are doing, and communicate with other users. Thus, their accounts can be investigated from the same perspective of personality which has been one of the key lenses through which SNS posts of individual users was investigated. In the line of literature that analyzed the personality of non-human objects such as products, stores, brands, and websites, the present research analyzed the personality of NPOs’ Instagram accounts using an online AI service. Also, it investigated how their personality traits were related to the characteristics of the uploaded photos at content and pixel levels. The results of analysis of 223,446 photos on 177 Instagram accounts suggested that the personality of NPOs’ Instagram accounts can be summarized as being high in openness and agreeableness but low in extraversion and neuroticism. And it was found that openness and agreeableness were the personality traits that associated the most with the photo features. Also, the personality traits of NPOs’ Instagram accounts, except neuroticism, were predicted from the photo features with an acceptable level of accuracy. Implications of this research and suggestions for further research were presented.
Introduction
Personality has been utilized to explain human behavior in various domain (Uher, 2008). In the context of social networking sites (SNSs), the personality of their users has been reported to be related to the characteristics of the posts they upload. The relationship has been mainly investigated in one of two ways. The personality traits of SNS users were measured and the according differences in their posts were examined (Kim and Kim, 2018), or the personality traits were predicted from the features of the posts they uploaded (Settanni et al., 2018). These investigations have contributed to the understanding of individual users’ online behaviors on SNSs.
Not only to individual users, this approach can be applied to organizational accounts of SNSs. Attempts have been made to understand the appearances and behaviors of various nonhuman objects, such as products (Dumitrescu, 2019), stores (Willems and Brengman, 2019), and websites (Jain and Yadav, 2019), from the perspective of personality. In this regard, the online behaviors of organizational SNS accounts can be examined from the perspective of personality. The accounts managed by organizations are similar to those run by individual users in their functionalities; they can upload posts, follow or become friends with other users, and like or comment on other users’ posts. Thus, the relationship between the personality of accounts and the characteristics of their posts can be investigated in the same manner both in organizational and individual accounts.
Meanwhile, social media photos have not received sufficient attention in terms of their relationships with uploaders’ personality. Social media data in text form have been mainly analyzed in the literature because they remain the most dominant form in social media posts. However, the importance of photo data has been rapidly increasing. One photo can express what a large amount of text can, and a growing number of users are using photo-centric SNSs such as Instagram as well as photo-uploading functions of existing SNSs such as Twitter and Facebook. In this vein, studies have analyzed photo data and explored how they are related to uploaders’ personalities (Segalin et al., 2017; Kanchana and Zoraida, 2020). However, their research samples were limited to individual users’ accounts, and it remains understudied how SNS photos in organizational accounts are related to the personality of the accounts. Notably, unlike texts, photos can be analyzed at two levels: the content (high) and pixel (low) levels. Photos can convey what is shown in the photos and how the pixels of the photos show it, and viewers can interpret the meaning of the photos in these two aspects. Thus, relationships between SNS photos and uploaders’ personality should be investigated at these two levels, but this has not been actively done especially concerning organizational accounts.
This study focuses on nonprofit organizations (NPOs), among many types of organizations, because they use social media as a main communication channel with the public and stakeholders (Wang and Yang, 2020). They usually do not have sufficient monetary and human resources for large-scale campaigns or mass media advertisements, so they use social media functions that enable them to communicate with many individuals quickly and cheaply. Additionally, their social media messages are usually consistent with their purpose of establishment and less concerned about external factors. This is less the case in other types of organizations such as political parties and businesses, because they must consider surrounding conditions such as political or market situations and actions of competing organizations.
The purpose of this study is to investigate how the personality of NPOs’ Instagram accounts is related to the characteristics of their photos at the content and pixel levels. To achieve this aim, we employ an online artificial intelligence (AI) service to measure the personality of organizational accounts by using the caption text attached to each Instagram photo. The personality measured using online data has been reported to have the same or higher level of accuracy (Youyou et al., 2015; Hinds and Joinson, 2019), and the AI service has been used in the literature for various purposes (Dutta et al., 2017; Balakrishnan et al., 2019; Kern et al., 2019; Yun et al., 2019; Whittingham et al., 2020; Kop et al., 2021). Additionally, photo features used in the literature to analyze social media photos are extracted at the content and pixel levels, and we examine how these features are associated with and whether the features can predict the accounts’ personalities.
The remainder of this paper is organized as follows. Studies on personality and social media posts, the personality of nonhuman objects, and NPOs’ social media posts are reviewed. Additionally, we describe how the research sample was selected, how the personality of NPOs was measured, and which photo features were used for analysis. The analysis results are presented, the implications and limitations of this research are discussed, and topics for further research are suggested.
Literature review
Analysis of nonprofit organizations’ social media posts
The characteristics of SNS posts that NPOs uploaded to their accounts has been a research subject for many years. Many studies have focused on the posts’ aim, which was mainly analyzed based on the information–community–action framework (Lovejoy and Saxton, 2012). This theoretical framework considers messages from organizations as doing one of the followings: spreading information about the organizations and their activities, conducting dialogic communication that can create relationships and build communities, or calling for particular actions such as donation, buying products, or attending events (Lovejoy and Saxton, 2012). Results in literature have suggested that NPOs use SNSs mainly for spreading information (Gao, 2016; Zhou and Pan, 2016; Qu, 2020). While some studies have shown the difference in SNS messages by culture (Waters and Lo, 2012) and emergency situations (Olson et al., 2019), a larger body of literature had reached a similar conclusion that one-way delivery of information was the dominant reason that NPOs use SNSs (Chung et al., 2020).
Other studies have provided insights into the more diverse aspects of SNS posts on the accounts of NPOs. For example, Campbell and Lambright (2020) focused on human service organizations, such as United Ways and Community foundations, and analyzed how SNS messages differed in these organizations from those of other types of NPOs. They found that human service organizations delivered more messages on taking action. Vedel et al. (2020) analyzed SNS posts of nonprofit healthcare organizations to show how they use SNSs to achieve their organizational goals. Their results suggest that the organizations use SNSs to replicate their existing websites, improve their engagement with the public, or employ novel functions of SNSs. Wang and Yang (2020) compared Twitter messages of nonprofit and for-profit organizations in terms of their dialogic relationship with their publics. They found that the emphasis of these two types of organizations differed: NPOs focused on the usefulness of information, while for-profit organizations focused on dialogic loops. Dineva et al. (2020) examined how NPOs managed the conflicts among consumers on their SNS pages. Their results from content analysis identified five strategies for managing conflicts, namely, non-engaging, censoring, bolstering, educating, and mobilizing.
Many studies, including those that we briefly reviewed, have focused on the characteristics of SNS posts uploaded by NPOs, and most of them have analyzed text data such as tweets. By contrast, the SNS data in photo form uploaded to NPOs’ accounts have not drawn much attention in the literature. Some studies have analyzed visual data on NPOs’ accounts (Guidry et al., 2017; Boscarino, 2022), but their analysis was mainly performed by human coders. This limited method prevented researchers from analyzing a large amount of data and investigating photo data at the pixel level, which is distinct from the content level, in which information is delivered and meanings are created. This research attempts to fill this gap and conduct a computational analysis of the Instagram photos uploaded to NPOs’ accounts.
Personality of nonhuman objects
The attempt to understand the appearances and behaviors from the perspective of personality was expanded to various nonhuman objects. First, the personality of products was explored. Jordan (2002) pointed out that each product has a personality that differentiate it from other products, just as each individual can be differentiated by personality. This product personality influences designers’ selection concerning the product (Choi, 2017) and customers’ preference for the product (Prieto et al., 2020). Store personality has also been examined. For example, Hoa and Thao (2017) devised questionnaires for store personality, and showed that it comprised four dimensions, namely, sophistication, enthusiasm, economy, and reliability. Concerning online objects, the personality of websites was investigated in literature (Lal and Katole, 2021). Akrimi (2016) examined the personality of Internet service providers and showed that enthusiasm and genuineness were positively associated, while solidity and unpleasantness were negatively associated, with satisfaction with the websites. In their work regarding the personality of online tourism products stores, Rezaei et al. (2016) found that website personality had positive impacts on utilitarian web browsing, hedonic web browsing, and impulsive buying. Jain and Yadav (2019) reported that website personality positively influenced on visitors’ purchase intention.
However, organizational SNS accounts have not been actively investigated from the perspective of personality. According to the computers are social actors (CASA) paradigm (Nass et al., 1994; Nass and Moon, 2000), individuals perceive computers as having the same personality as humans because they apply human social rule when they interact with computers (Gambino et al., 2020; Lombard and Xu, 2021). In a similar vein, SNS users may perceive organizational accounts as being like a human, and this similarity enables this study to explore organizational SNS accounts from the perspective of personality. Based on this consideration, this study raises following research question:
RQ1. What are the characteristics of the personality of NPOs’ Instagram accounts?
Personality of social media users and the characteristics of their posts
The Big Five personality model (Digman, 1990; McCrae and John, 1992) has been widely used to examine SNS users. It considers human personality as comprising five factors—openness, conscientiousness, extraversion, agreeableness, and neuroticism—and represents human personality by relative strengths of these factors.
The literature has examined the characteristics of SNS posts in terms of the difference according to the uploaders’ personality. For example, Pentina and Zhang (2017) found that Facebook users high in extraversion, agreeableness, and conscientiousness disclosed more positive emotions on their posts. Agarwal and Toshniwal (2020) reported that Twitter users high in extraversion and agreeableness revealed stronger leadership in their online behavior during natural hazards. Wang and Chen (2020) investigated relationships between the personality of CEOs and organizational performance: their results suggests that extraversion, agreeableness, and emotional stability (the inverse of neuroticism) were positively related to cost efficiency and profitability and that conscientiousness was negatively related to them. Miller (2020) examined how the Big Five personality traits were related to inappropriate posting by college students on Facebook and Twitter. Their results indicated that users with a higher level of conscientiousness posted less inappropriate content.
In this study, we attempt to apply this approach to the SNS accounts of NPOs. Although the posts on organizational SNS accounts have been actively analyzed (Yan et al., 2018; Liao et al., 2020; Wang and Yang, 2020; Liu et al., 2021), what has been relatively understudied is their relation to the accounts’ personality. The following research questions are raised:
RQ2. How are the personality traits of NPOs’ Instagram accounts associated with the characteristics of the photos uploaded to the accounts?
Predicting personality from social media photos
The literature has utilized SNS data for predicting users’ psychological characteristics including personality. SNS data can be considered as digital traces of the users, and personality traits are reported to be linked more strongly with online behaviors than offline ones (Azucar et al., 2018). Thus, analyzing SNS data has much potential for an unobtrusive way of measuring personality (Settanni et al., 2018). Especially, predicting personality using photo data is known to generate better results than using text data (Ferwerda et al., 2016), and previous studies have adopted this approach.
A group of studies used a particular type of SNS photos for predicting personality: profile photos (Kanchana and Zoraida, 2020), selfies (Moreno-Armendariz et al., 2020), or the photos that users liked (Segalin et al., 2017). However, photos that users posted were reported to have more predictive power than those photos (Samani et al., 2018), and another group of studies used photos that users uploaded on their accounts. Ferwerda et al. (2016) predicted the Big Five personality traits of 113 Instagram users from 22,398 photos on their accounts. Similarly, Kim and Kim (2018) used 25,394 Instagram photos to predict the Big Five personality traits of 179 users. Samani et al. (2018) showed that the uploaders’ personality can be predicted more accurately when their photos on different platforms, Twitter and Flickr, are used together for prediction. Also, Mohammadiani and Sadeghi (2020) showed that the predictive performance can be improved using profile, posted, and liked images of Flickr users.
Based on these studies, this research predicts the personality of NPOs’ Instagram accounts. The photo features that were used to predict the personality of individual users’ accounts in the literature are extracted, and machine learning models are trained to show the features’ predictability on personality. The following research questions are pursued:
RQ3. How are the personality traits of NPOs’ Instagram accounts predicted from the characteristics of the photos uploaded to the accounts?
Materials and methods
Research sample
The list of NPOs was obtained from the Nonprofit Times’ Top 100 Nonprofits on the Web,1 The Global Journal’s Top 100 nongovernmental organizations (NGOs),2 and the NGOs affiliated with the United Nations.3 We visited the official webpage of each organization to obtain its Instagram account. If its Instagram account was not presented on the webpage, we searched for it on Google. Additionally, during this search, nonprofit organizations not on the list were found and added to the list. The organizations without Instagram accounts or whose uploaded posts were less than 30 were excluded from the research sample. As a result, 177 Instagram accounts of NPOs were selected as the research sample (Table 1), and all posts (photos and accompanying caption texts) were downloaded using Instagram scraper.4 For analysis, 223,446 posts were used.
Measuring personality of organizational accounts
The personality of each account was assessed using IBM Watson Personality Insights, which was selected due to of its ease of use.5 This service assesses the personality of the author of a given text (Hrazdil et al., 2021) based on the research about the relationship between language and personality (Fast and Funder, 2008; Hirsh and Peterson, 2009; Yarkoni, 2010), and it has been used in the literature to examine the personality of the author of SNS texts (Kern et al., 2019; Yun et al., 2019; Whittingham et al., 2020; Sakib et al., 2021; Gruda and Ojo, 2022). In this study, the caption texts of all Instagram photos uploaded to a given account were sent to the server via application programming interface (API), which returned the Big Five personality traits—openness, conscientiousness, extraversion, agreeableness, and neuroticism—of the account by providing a value between 0 and 1 for each trait.
Instagram photo features
Photo features that have been used in the literature (Ferwerda et al., 2016; Ferwerda and Tkalcic, 2018; Kim and Kim, 2020; Kim and Lee, 2021) to analyze Instagram photos were used for analysis. The features were extracted at the content and pixel levels: the content-level features were content category and facial features, and the pixel-level features were pixel color features and visual features. Because the unit of analysis in this study is an account, the following features were extracted from each photo and averaged across all photos on a given account (except features in the content category, which are account-level metrics).
Content category
The category to which the content of a photo belongs was determined using Computer Vision API in Microsoft Azure Cognitive Services.6 For a photo sent to the server via API, its content was categorized into one of the 15 predetermined classes by the pretrained AI: the classes are abstract, animal, building, dark, drink, food, indoor, others, outdoor, people, plant, object, sky, text, and transportation. Next, the share of each class out of all photos on a given account was calculated. Thus, for example, if people of an account is 0.5, half of the photos uploaded to the account were of people. Additionally, the Gini coefficient was measured. Since it is a metric of the degree of concentration (Gini, 1912), it shows nondiversity in terms of the content category of the photos in an account.
Facial features
Features regarding human faces in a photo were extracted using Face API in Microsoft Azure Cognitive Services.7 First, the number of faces was the measure of how many faces appeared in a photo, closeup was the measure of the ratio of the size of the biggest face in a photo to the total size of the photo, and face ratio was the measure of the ratio of the sum of sizes of all faces in a photo to the total size of the photo. Next, age was the measure of the average age of the appearing faces, and gender was the measure of the number of female faces in a photo. Additionally, the emotions expressed on each appearing face were determined using Face API. The emotions include anger, contempt, disgust, fear, happiness, sadness, surprise, and neutral, and the sum of the eight emotions becomes 1 for a given face. The averages for each of the eight emotions on all faces in a photo were measured.
Pixel color features
Digital photos consist of pixels containing information on visual characteristics such as colors. It can be expressed by diverse color space models such as RGB (red, green, blue) and HSV (hue, saturation, value). Using this pixel-level information, we extracted the following features using the Python programming language and OpenCV library (codes are presented in Supplementary material).
First, the red, green, and blue in RGB were, respectively, averaged across all pixels in a photo, and their variances were also obtained. The same was performed for saturation and value (i.e., lightness) in HSV. As a result, the following features were measured: red mean, red variance, green mean, green variance, blue mean, blue variance, saturation mean, saturation variance, value mean, and value variance. Hue is a nominal feature unlike saturation and value. Thus, its total range (0 to 179 in OpenCV) was divided into intervals ([7, 23, 35, 90, 136, 169]) so that each interval corresponds to each key color (red, orange, yellow, green, blue, and violet), and the share of pixels whose hue falls into each color interval was calculated (Kim and Kim, 2018). As a result, the following features were measured: red share, orange share, yellow share, green share, blue share, and violet share. Also, the share of warm colors (red, orange, and yellow; warm share) and the share of cold colors (green, blue, and violet; cold share) were also measured. In addition, the number of peaks in a hue histogram (hue peaks) was measured: a hue histogram was generated, smoothed by kernel density estimation, and the number of local maximums was counted (Kim and Kim, 2018).
Visual features
The features concerning the visual attractiveness of a photo, suggested by San Pedro and Siersdorfer (2009) and used in the literature (Trattner et al., 2018; Messina et al., 2019; Yazdavar et al., 2020; Zhang et al., 2020), were extracted (see Table 2 for summary of visual features). First, brightness, which represents how bright a photo is, was measured by the average of luminance (Y values in the YUV color space) in the pixels of the photo. Next, colorfulness, which represents how colorful a photo is, was measured using the means and standard deviations of metrics composed of relative amounts of red, green, and blue values in the pixels. Naturalness, which represents how much a photo corresponds to the human perception of reality, was measured using the proportion of pixels whose saturation and luminance fall in a certain range. Contrast, which represents the relation of local luminance variations to the surrounding luminance, was measured by the standard deviation of luminance in pixels divided by the number of pixels. RGB contrast was also measured by extending contrast into the three-dimensional RGB color space. Sharpness, which represents a photo’s clarity and level of detail, was measured as a function of Laplacian of each pixel’s luminance, normalized by the local average luminance in the surroundings of each pixel. Two additional visual features concerning color were measured. Color diversity, which represents how diverse the colors used in a photo are, was measured by fractal dimension using the box-counting method (Feng et al., 1996); fractal dimension has been used as a metric of color diversity in the literature (Kim et al., 2014; Kim and Kim, 2019). Color harmony, which represents how harmonious the dominant colors in a photo are, was measured by the geometric formulations generated by the dominant colors on the color wheel; the highest and the second highest peaks in the smoothed hue histogram were identified as the top two dominant colors, and the internal angle made between the two colors on the color wheel is color harmony (Kim and Kim, 2019). Finally, pleasure, arousal, and dominance were measured by the affections from the PAD model using the formula8 in the literature (Valdez and Mehrabian, 1994).
Results and discussions
Mean personality traits of nonprofit organizations’ Instagram accounts (RQ1)
Figure 1 presents the mean personality traits of NPOs in the research sample for RQ1. It shows that openness and agreeableness were relatively high, while extraversion and neuroticism were relatively low. In other words, the personality of NPOs in the research sample can be summarized as being open and agreeable rather than extraverted and neurotic.
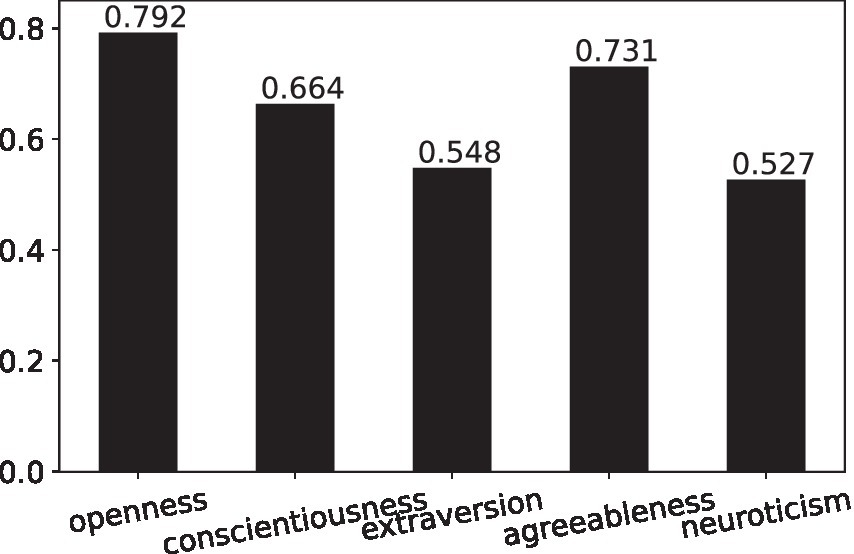
Figure 1. Mean personality traits of nonprofit organizations’ Instagram accounts in the research sample.
This pattern in Big Five personality traits of NPOs’ Instagram accounts was compared with the ones of other individuals and organizations in the literature which used IBM Watson Personality Insights. The personality of McDonald’s Twitter account was relatively high in extraversion and agreeableness while relatively low in openness and neuroticism, that of Harley-Davidson was relatively high in openness and conscientiousness while relatively low in neuroticism, and that of Tom’s Shoes was relatively high in extraversion and agreeableness while relatively low in neuroticism (Yun et al., 2019). Also, the personalities of Indian celebrities were measured from their tweets (Dutta et al., 2017): actors and sports figures were relatively high in extraversion, and politicians were relatively high in openness. Additionally, the personality of mass murderers measured from their writings was relatively high in openness while relatively low in extraversion and agreeableness (Kop et al., 2021).
These results are distinct from the pattern in the personality of NPOs’ Instagram accounts reported in this study. However, a similar pattern can be found in the personality of public health organizations’ Instagram accounts (Kim and Lee, 2021): this suggest that Instagram accounts of public health organizations and NPOs share the similar personality in common. This result may be explained by the characteristics of those organizations: public health organizations and NPOs usually aim to contribute to the public interest, and this might have made their online communication open and agreeable.
Correlations between personality traits and photo features (RQ2)
Correlational analyses were conducted for RQ2 and the results are presented in Table 3. It shows that certain personality traits were mainly associated with certain kinds of photo features. Openness was the personality traits that associated the most with all kinds of photo features, and agreeableness was associated with many of content category, facial, and visual features. Additionally, neuroticism was associated mainly with facial features, and conscientiousness was associated mainly with pixel color features. As shown in the previous subsection, openness and agreeableness were the highest traits in the NPOs’ mean personality traits, and this can be the possible reason why they had significant correlations with many of photo features.
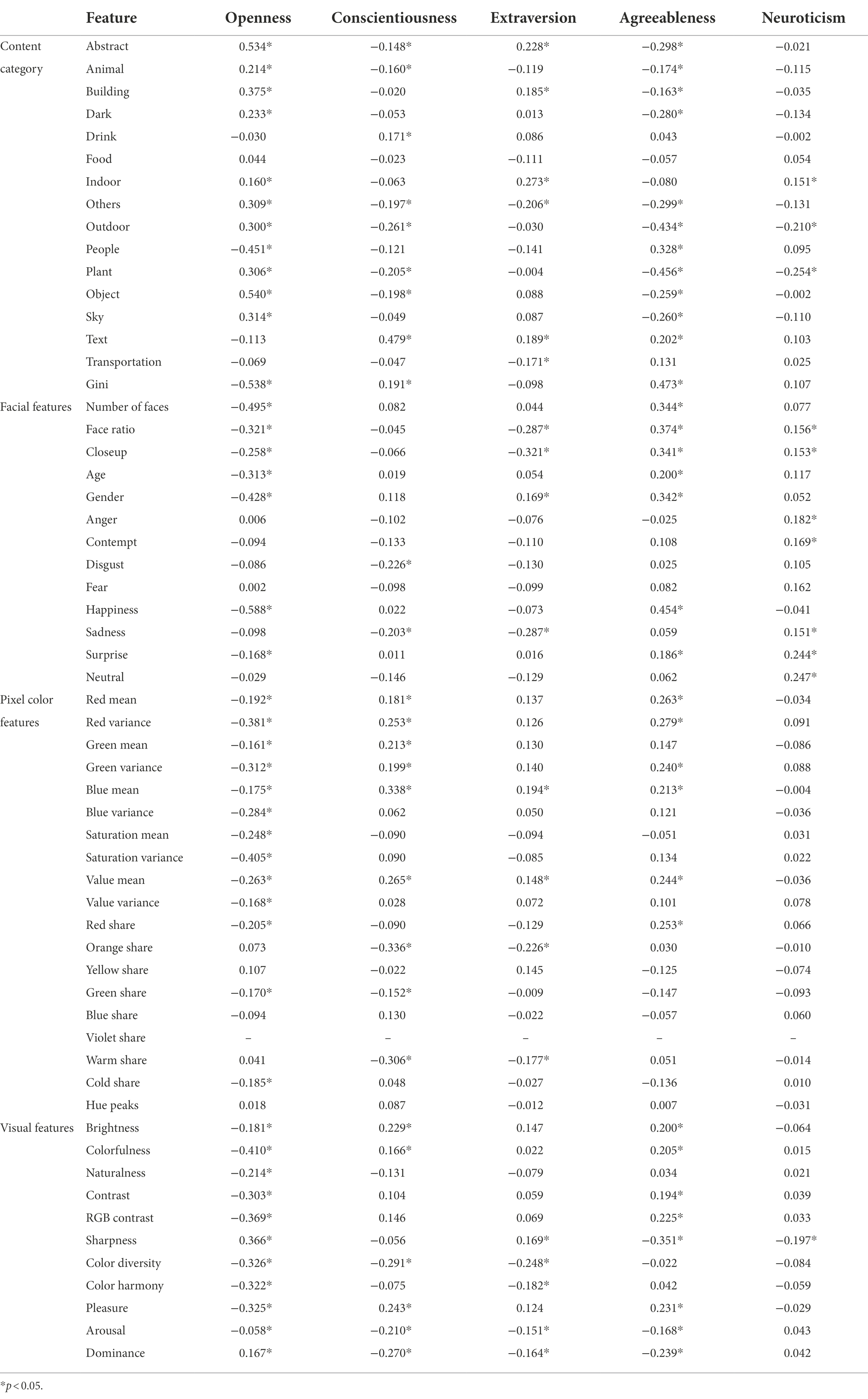
Table 3. Correlations between the personality traits of nonprofit organizations’ Instagram accounts and the features of their photos.
It was also found that openness and agreeableness showed correlations in opposite directions with content category features, facial features, and visual features. In other words, the features which were associated with openness positively (negatively) were associated with agreeableness negatively (positively). In terms of their meanings, openness and agreeableness are different but cannot be said to be opposite: the former is about curiosity and wide interests and the latter is being generous and sympathetic with others (McCrae and John, 1992). However, the findings suggest that these two traits were visually manifested in an opposite manner. Previous studies have reported that openness and agreeableness were associated in opposite directions with SNS photo features (Liu, 2016; Segalin et al., 2017; Matz et al., 2019; Kim and Lee, 2021), but that was not the case with SNS text features (Pentina and Zhang, 2017; Miller, 2020). Thus, it can be possibly presumed that the difference between the two traits is amplified to be opposite to each other when visually represented in SNS photos.
Openness and photo features
Table 3 shows that openness was significantly correlated with many of the content category features. Given that openness is related to curiosity and wide range of interests (McCrae and John, 1992), this diversity in content might be explained. The meaning of openness also corresponds with the negative association between openness and Gini, which indicates that the content of photos uploaded to the accounts of higher level of openness were more diverse. Notably, openness was negatively associated with the share of people photos. A possible explanation for this result may be the other aspect of openness which is related to artistic disposition (McCrae and John, 1992). Previous studies have reported that SNS users who are high in openness upload artistic photos (Kern et al., 2014; Liu, 2016), especially about abstract art (Feist and Brady, 2004), and these photos usually do not feature people (Matz et al., 2019). This may also hold for NPOs’ Instagram accounts. In a similar vein, less and smaller human faces appeared in the photos of accounts with higher openness (negative correlations with number of faces, race ratio, and closeup), and these results are consistent with previous studies (Liu, 2016; Matz et al., 2019).
Concerning pixel-level features, openness showed negative associations with means and standard deviations of RGB, saturation, and value. This was also the case with brightness, colorfulness, contrast, and color diversity. Those are the features whose high value might make the photos look fancy and splendid. These results suggest that the photos uploaded to the accounts with higher openness were generally darker, less strong in color, less bright, and less splendid. At first sight, it looks inconsistent with the artistic propensity in the definition of openness and the results of some previous studies (Fayn et al., 2015; Liu, 2016). However, other studies have suggested that the artistic propensity can be expressed in other ways including grayscale images (Guntuku et al., 2017), whose luminous and brightness were negatively associated with openness (Ferwerda et al., 2016), and this might be the possible explanation for the above results.
Conscientiousness and photo features
Conscientiousness is the propensity to leads one’s life in an efficient and well-organized manner, and this trait is related to being reliable and responsible (McCrae and John, 1992). The SNS photos of highly conscientious users were reported to be mainly of formal settings including office and classroom (Guntuku et al., 2017). These might be the reason why conscientiousness was positively associated with text but negatively with animal, outdoor, and plant in our results. Also, disgust and sadness were negatively associated with conscientiousness. This result may be explained by the above definition of conscientiousness, which can be linked to not expressing negative emotions in SNS photos (Liu, 2016; Bhatti et al., 2017).
The results in Table 3 also suggest that conscientiousness is related to the photos’ being strong in colors and luminous (positive correlations with RGB mean, value mean, colorfulness, and brightness). It is possibly because conscientious SNS users may prefer uploading posts in a normative and conventional manner, and they usually do not upload artistic or experimental photos as highly open users do (Guntuku et al., 2017). And these results correspond with the literature which has reported positive correlations of conscientiousness with RGB mean and value mean (Liu, 2016; Guntuku et al., 2017), colorfulness (Bhatti et al., 2017), and brightness (Liu, 2016).
Extraversion and photo features
Extraversion is the trait of being outgoing, talkative, and active and energetic in social interaction (McCrae and John, 1992), and the SNS posts of highly extrovert users have been reported to include social words and phrases like “party” and “cannot wait” (Kern et al., 2014). Thus, photos about people, social interaction (drink and food), and outdoor activities (outdoor, sky, plant, and transportation) can be expected to take large share in the accounts of high level of extraversion. On the contrary to this expectation, however, none of those content category showed significant correlations with extraversion. Rather, indoor, building, and text were positively associated with extraversion. This may be due to the characteristics of NPOs: they are organizations for social activities, so they may show their activities and opinions rather than leisure and pastime. Also, this might be why faces were small (negative associations with face ratio and closeup) and the color of photos were less diverse and less harmonious (negative associations with color diversity and color harmony) in the photos of highly extravert accounts.
Agreeableness and photo features
Agreeableness refers to the propensity of being generous, kind, and sympathetic with others (McCrae and John, 1992). This may be why photos of people-related content had large share in the highly agreeable accounts (positive association with people), as in previous studies (Guntuku et al., 2017). The negative associations with animal, outdoor, plant, and sky could also be attributed to this propensity because high agreeableness is known to prefer social interaction over nature and sustainable lifestyle (Saitov et al., 2021). And the photos on the highly agreeable accounts contained more and larger human faces (positive correlations with number of faces, face ratio, and closeup): these results are thought to be the reflections of the generous and sympathetic social interactions and correspond with the results of previous studies (Liu, 2016; Matz et al., 2019).
Concerning pixel-level characteristics, the photos of highly agreeable accounts were bright (positive associations with value mean and brightness), colorful (positive associations with colorfulness), and contrasting (positive associations with contrast). The generous, kind, and sympathetic propensity is consistent with these appealing characteristics of photos (Celli et al., 2014; Liu, 2016; Bhatti et al., 2017). Also, this propensity would make the affection of photos less aroused (negative association with arousal).
Neuroticism and photo features
Neuroticism, or emotional instability, is concerned with being anxious, worrying, touchy, and self-pitying (McCrae and John, 1992). This propensity can be the possible explanation for the positive associations with negative emotions such as anger, contempt, fear, sadness, and surprise, and neutral emotion. The associations between neuroticism and negative/neutral emotions on SNS posts have been reported in many previous studies (Golbeck et al., 2011; Schwartz et al., 2013; Kern et al., 2014; Liu, 2016). Also, the literature has found that neuroticism is closely linked to not presenting faces on SNS photos (Liu, 2016) and was associated negatively with the number and size of human faces on the photos (Celli et al., 2014; Matz et al., 2019). However, the results in this study contradict the literature: neuroticism was positively associated with face ratio and closeup. It suggests that the bigger (although not more) human faces appeared in the photos of highly neurotic accounts. This can be the characteristics of NPOs’ Instagram accounts because similar results are hardly found in the literature.
Predicting personality traits using photo features (RQ3)
For RQ3, in addition to the correlational analyses, predictive models were built and analyzed to examine the predictability of the photo features on the personality traits of NPOs’ Instagram accounts. A random forest regressor with 10-fold cross-validation was trained for each personality trait, and a root mean square error (RMSE) was calculated to observe the predictability of each model (Table 4). These RMSEs were compared with those in the literature in which SNS user personalities were predicted from their photo features and the predictive power of the models were reported to be adequate. The RMSEs were 0.7–0.9 (Skowron, 2016), 0.66–0.78 (Ferwerda et al., 2016), or 0.561–0.737 (Kim and Kim, 2018), in which the personality traits were measured using 5-point Likert scale. For comparison, these RMSEs were divided by 4, the range of 5-point scale, to convert into the [0, 1] scale used in this study. As a result, the RMSEs of 0.5–0.7 in the 5-point scale were converted into 0.125–0.175 in the [0, 1] scale.

Table 4. Root mean square errors in 10-fold cross validation of random forest regression on personality traits.
We compared our RMSEs and those in the literature. Table 4 presents the results, suggesting that the predictive power of Instagram photo features on the Big Five personality traits, except neuroticism, of NPOs’ accounts was acceptable. This result is consistent with the literature suggesting that the personality of SNS users can be predicted from what they have written or uploaded to their accounts (Ferwerda et al., 2016; Azucar et al., 2018; Kim and Kim, 2018; Samani et al., 2018; Settanni et al., 2018). In addition, the result that neuroticism was not predicted from the features is also consistent with the literature which has suggested that neuroticism is the most difficult trait to predict (Ferwerda et al., 2016). These consistencies indicate that organizational SNS accounts can be investigated from the perspective of personality as has been performed for individual users’ accounts.
Conclusion
The present study investigated the personality of NPOs’ Instagram accounts and according differences in their uploaded photos at content and pixel levels. It was found that the personality of NPOs was relatively high in openness and agreeableness but relatively low in extraversion and neuroticism. It was also found that openness and agreeableness were the personality traits which were associated the most the features of Instagram photos on NPOs’ accounts. And this study found that the personality traits of NPOs’ Instagram accounts, except neuroticism, can be predicted from the features of photos uploaded to the accounts.
Implications of this study
The findings of this study imply that online behaviors of NPOs can be understood from the perspective of personality. We demonstrated that, similar to those of individual users, NPOs’ Instagram accounts differed in what they upload to their accounts on the basis of their personality. Thus, a SNS campaign by NPOs can be considered by the public to be a communication with an individual with a particular type of personality that is open and agreeable and not extraverted and neurotic. This can provide practical, expedient methods for designing SNS messages because messages that correspond more with organizations’ personalities are expected to have a stronger influence on their communication with the public. For example, NPOs would emphasize features that associated with openness and agreeableness but not those associated with extraversion and neuroticism, and this can increase the appeal of their Instagram photos.
Limitations and suggestions for further research
The main limitation of this study is that its research sample comprised a limited number of NPOs. Further research which employs larger and diverse research sample can reveal the difference in the personality of NPOs by their activity area, countries, and culture. This study showed that the personality of NPOs’ Instagram accounts is similar with that of public health organizations’ Instagram accounts. Further research is expected to examine whether this similarity is general across organizational Instagram accounts or peculiar to those of particular types. Concerning the relationships between personality traits and photo features, future research may examine how the relationships differ by types of organizations. It is expected that more correlations and predictions are obtained and the relationships between personality traits and photo features in organizational SNS accounts are theorized.
In addition, it can be questioned that the personality of NPOs’ Instagram accounts can be attributed to the personality of account manager and/or of who are allowed to upload photos to the account. Future research can address this question by investigating whether the personality of account manager is directly represented on the accounts or other factors in organizations influence the accounts’ personality.
Data availability statement
The original contributions presented in the study are included in the article/Supplementary material; further inquiries can be directed to the corresponding author.
Author contributions
The author confirms being the sole contributor of this work and has approved it for publication.
Conflict of interest
The author declares that the research was conducted in the absence of any commercial or financial relationships that could be construed as a potential conflict of interest.
Publisher’s note
All claims expressed in this article are solely those of the authors and do not necessarily represent those of their affiliated organizations, or those of the publisher, the editors and the reviewers. Any product that may be evaluated in this article, or claim that may be made by its manufacturer, is not guaranteed or endorsed by the publisher.
Supplementary material
The Supplementary material for this article can be found online at: https://www.frontiersin.org/articles/10.3389/fpsyg.2022.923305/full#supplementary-material
Footnotes
1. ^https://topnonprofits.com/lists/best-nonprofits-on-the-web/
2. ^http://theglobaljournal.net/top100ngos/
3. ^http://www.ngo.org/links/list.htm
4. ^https://github.com/arc298/instagram-scraper
5. ^This service was deprecated as of 1 December 2021. Interested readers can consider other alternatives including:• https://github.com/senticnet/personality-detection (Majumder et al., 2017)• http://farm2.user.srcf.net/research/personality/recognizer.html (Mairesse et al., 2007)• Apply Magic sauce. https://applymagicsauce.com/
6. ^https://azure.microsoft.com/services/cognitive-services/computer-vision/
7. ^https://azure.microsoft.com/services/cognitive-services/face/
8. ^Pleasure = 0.69 × Value + 0.22 × Saturation; Arousal = −0.31 × Value + 0.60 × Saturation; Dominance = −0.76 × Value +0.32 × Saturation.
References
Agarwal, A., and Toshniwal, D. (2020). Identifying leadership characteristics from social media data during natural hazards using personality traits. Sci. Rep. 10:2624. doi: 10.1038/s41598-020-59086-0
Akrimi, Y. (2016). Usability, interactivity, website personality and consumers’ responses: a case of internet service provider. Int. J. Electron. Mark. Retail. 7, 204–228. doi: 10.1504/IJEMR.2016.078950
Azucar, D., Marengo, D., and Settanni, M. (2018). Predicting the big 5 personality traits from digital footprints on social media: a meta-analysis. Pers. Individ. Differ. 124, 150–159. doi: 10.1016/j.paid.2017.12.018
Balakrishnan, V., Khan, S., Fernandez, T., and Arabnia, H. R. (2019). Cyberbullying detection on twitter using big five and dark triad features. Pers. Individ. Differ. 141, 252–257. doi: 10.1016/j.paid.2019.01.024
Bhatti, S. K., Muneer, A., Lali, M. I., Gull, M., and Din, S. M. U. (2017). Personality analysis of the USA public using twitter profile pictures. The 2017 international conference on information and communication technologies, Karachi, Pakistan.
Boscarino, J. E. (2022). Constructing visual policy narratives in new media: the case of the Dakota Access pipeline. Inf. Commun. Soc. 25, 278–294. doi: 10.1080/1369118x.2020.1787483
Campbell, D. A., and Lambright, K. T. (2020). Terms of engagement: Facebook and twitter use among nonprofit human service organizations. Nonprofit Manag. & Leadersh. 30, 545–568. doi: 10.1002/nml.21403
Celli, F., Bruni, E., and Lepri, B. (2014). Automatic personality and interaction style recognition from Facebook profile pictures. The ACM international conference on multimedia, Orlando, FL.
Choi, J. (2017). Material selection by the evaluation of diffuse interface of material perception and product personality. IJIDeM. 11, 967–977. doi: 10.1007/s12008-016-0320-9
Chung, A., Woo, H., and Lee, K. (2020). Understanding the information diffusion of tweets of a non-profit organization that targets female audiences: an examination of women who Code’s tweets. J. Commun. Manag. 25, 68–84. doi: 10.1108/JCOM-05-2020-0036
Digman, J. M. (1990). Personality structure: emergence of the five-factor model. Annu. Rev. Psychol. 41, 417–440. doi: 10.1146/annurev.ps.41.020190.002221
Dineva, D., Breitsohl, J., Garrod, B., and Megicks, P. (2020). Consumer responses to conflict-management strategies on non-profit social media fan pages. J. Interact. Mark. 52, 118–136. doi: 10.1016/j.intmar.2020.05.002
Dumitrescu, A. (2019). Product personality: testing a new approach. U.P.B. Sci. Bull Series D. 81, 327–340.
Dutta, K., Singh, V. K., Chakraborty, P., Sidhardhan, S. K., Krishna, B. S., and Dash, C. (2017). Analyzing big-five personality traits of Indian celebrities using online social media. Psychol. Stud. 62, 113–124. doi: 10.1007/s12646-017-0408-8
Fast, L. A., and Funder, D. C. (2008). Personality as manifest in word use: correlations with self-report, acquaintance report, and behavior. J. Pers. Soc. Psychol. 94, 334–346. doi: 10.1037/0022-3514.94.2.334
Fayn, K., Mac Cann, C., Tiliopoulos, N., and Silvia, P. J. (2015). Aesthetic emotions and aesthetic people: openness predicts sensitivity to novelty in the experiences of interest and pleasure. Front. Psychol. 6:1877. doi: 10.3389/fpsyg.2015.01877
Feist, G. J., and Brady, T. R. (2004). Openness to experience, non-conformity, and the preference for abstract art. Empir. Stud. Arts 22, 77–89. doi: 10.2190/Y7CA-TBY6-V7LR-76GK
Feng, J., Lin, W.-C., and Chen, C.-T. (1996). Fractional box-counting approach to fractal dimension estimation. The 13th International Conference on Pattern Recognition, Vienna, Austria.
Ferwerda, B., Schedl, M., and Tkalcic, M. (2016). “Using Instagram picture features to predict users’ personality,” in Multimedia modeling. ed. Q. Tian (Cham: Springer International Publishing), 850–861.
Ferwerda, B., and Tkalcic, M. (2018). You are what you post: what the content of Instagram pictures tells about users’ personality. The second workshop on theory-informed user modeling for tailoring and personalizing interfaces (HUMANIZE), Tokyo, Japan.
Gambino, A., Fox, J., and Ratan, R. (2020). Building a stronger CASA: extending the computers are social actors paradigm. Hum. mach. commun. 1, 71–86. doi: 10.30658/hmc.1.5
Gao, F. (2016). Social media as a communication strategy: content analysis of top nonprofit foundations’ micro-blogs in China. Int. J. Strateg. Commun. 10, 255–271. doi: 10.1080/1553118X.2016.1196693
Gini, C. (1912). Variabilità e Mutuabilità: Contributo allo Studio delle Distribuzioni e delle Relazioni Statistiche. Bologna: Tipogr. di P. Cuppini.
Golbeck, J., Robles, C., and Turner, K. (2011). Predicting personality with social media. The ACM CHI Conference on Human Factors in Computing Systems, Vancouver, BC, Canada.
Gruda, D., and Ojo, A. (2022). All about that trait: examining extraversion and state anxiety during the SARS-CoV-2 pandemic using a machine learning approach. Pers Individ Dif. 188:111461. doi: 10.1016/j.paid.2021.111461
Guidry, J. P. D., Jin, Y., Orr, C. A., Messner, M., and Meganck, S. (2017). Ebola on Instagram and twitter: how health organizations address the health crisis in their social media engagement. Public Relat. Rev. 43, 477–486. doi: 10.1016/j.pubrev.2017.04.009
Guntuku, S.C., Lin, W., Carpenter, J., Ng, W. K., Ungar, L. H., and Preoţiuc-Pietro, D. (2017). Studying personality through the content of posted and liked images on twitter. The 2017 ACM on Web Science Conference, Troy, NY.
Hinds, J., and Joinson, A. (2019). Human and computer personality prediction from digital footprints. Curr. Dir. Psychol. Sci. 28, 204–211. doi: 10.1177/0963721419827849
Hirsh, J. B., and Peterson, J. B. (2009). Personality and language use in self-narratives. J. Res. Pers. 43, 524–527. doi: 10.1016/j.jrp.2009.01.006
Hoa, N. L. T., and Thao, H. T. P. (2017). The antecedents and consequences of store personality: a study of specialty store chains in Vietnam. Int. Rev. Bus. Res. Pap. 13, 88–103. doi: 10.21102/irbrp.2017.03.131.07
Hrazdil, K., Mahmoudian, F., and Nazari, J. A. (2021). Executive personality and sustainability: do extraverted chief executive officers improve corporate social responsibility? Corp. Soc. Responsib. Environ. Manag. 28, 1564–1578. doi: 10.1002/csr.2116
Jain, K., and Yadav, D. (2019). “The role of website personality and website user engagement on individual’s purchase intention,” in System performance and management analytics: Asset analytics. ed. P. K. Kapur (Singapore: Springer Singapore), 347–360.
Jordan, P. W. (2002). “The personality of products,” in Pleasure with products: Beyond usability. eds. W. S. Green and P. W. Jordan (London: Taylor & Francis), 17–46.
Kanchana, T. S., and Zoraida, B. S. E. (2020). Analysis of social media images to predict user personality assessment. IJEET. 11, 1–9. doi: 10.34218/IJEET.11.7.2020.001
Kern, M. L., Eichstaedt, J. C., Schwartz, H. A., Dziurzynski, L., Ungar, L. H., Stillwell, D. J., et al. (2014). The online social self: an open vocabulary approach to personality. Assessment 21, 158–169. doi: 10.1177/1073191113514104
Kern, M. L., McCarthy, P. X., Chakrabarty, D., and Rizoiu, M. A. (2019). Social media-predicted personality traits and values can help match people to their ideal jobs. Proc. Natl. Acad. Sci. U. S. A. 116, 26459–26464. doi: 10.1073/pnas.1917942116
Kim, Y., and Kim, J. H. (2018). Using computer vision techniques on Instagram to link users’ personalities and genders to the features of their photos: an exploratory study. Inf. Process. Manag. 54, 1101–1114. doi: 10.1016/j.ipm.2018.07.005
Kim, J. H., and Kim, Y. (2019). Instagram user characteristics and the color of their photos: colorfulness, color diversity, and color harmony. Inf. Process. Manag. 56, 1494–1505. doi: 10.1016/j.ipm.2018.10.018
Kim, Y., and Kim, J. H. (2020). Using photos for public health communication: a computational analysis of the Centers for Disease Control and Prevention Instagram photos and public responses. J. Health Inform. 26, 2159–2180. doi: 10.1177/1460458219896673
Kim, Y., and Lee, S. (2021). Personality of public health organizations’ Instagram accounts and according differences in photos at content and pixel levels. Int. J. Environ. Res. Public Health 18:3903. doi: 10.3390/ijerph18083903
Kim, D., Son, S.-W., and Jeong, H. (2014). Large-scale quantitative analysis of painting arts. Sci. Rep. 4:7370. doi: 10.1038/srep07370
Kop, M., Read, P., and Walker, B. R. (2021). Pseudocommando mass murderers: a big five personality profile using psycholinguistics. Curr. Psychol. 40, 3015–3023. doi: 10.1007/s12144-019-00230-z
Lal, M., and Katole, H. (2021). Website personality: a theoretical study. Psychol. Edu. 58, 4041–4049. doi: 10.17762/pae.v58i2.2676
Liao, Q., Yuan, J., Dong, M., Yang, L., Fielding, R., and Lam, W. W. T. (2020). Public engagement and government responsiveness in the communications about COVID-19 during the early epidemic stage in China: Infodemiology study on social media data. J. Med. Internet Res. 22:e18796. doi: 10.2196/18796
Liu, L. (2016). Analyzing personality through social media profile picture choice. The 10th International AAAI Conference on Web and Social Media (ICWSM-16), Cologne, Germany.
Liu, W., Xu, W. W., and John, B. (2021). Organizational disaster communication ecology: examining interagency coordination on social media during the onset of the COVID-19 pandemic. Am. Behav. Sci. 65, 914–933. doi: 10.1177/0002764221992823
Lombard, M., and Xu, K. (2021). Social responses to media technologies in the 21st century: the media are social actors paradigm. Hum. mach. commun. 2, 29–55. doi: 10.30658/hmc.2.2
Lovejoy, K., and Saxton, G. D. (2012). Information, community, and action: how nonprofit organizations use social media. J. Comput.-Mediat. Commun. 17, 337–353. doi: 10.1111/j.1083-6101.2012.01576.x
Mairesse, F., Walker, M. A., Mehl, M. R., and Moore, R. K. (2007). Using linguistic cues for the automatic recognition of personality in conversation and text. J. Artif. Intell. Res. 30, 457–500. doi: 10.1613/jair.2349
Majumder, N., Poria, S., Gelbukh, A., and Cambria, E. (2017). Deep learning-based document modeling for personality detection from text. IEEE Intell. Syst. 32, 74–79. doi: 10.1109/MIS.2017.23
Matz, S. C., Segalin, C., Stillwell, D., Müller, S. R., and Bos, M. W. (2019). Predicting the personal appeal of marketing images using computational methods. J. Consum. Psychol. 29, 370–390. doi: 10.1002/jcpy.1092
McCrae, R. R., and John, O. P. (1992). An introduction to the five-factor model and its applications. J. Pers. 60, 175–215. doi: 10.1111/j.1467-6494.1992.tb00970.x
Messina, P., Dominguez, V., Parra, D., Trattner, C., and Soto, A. (2019). Content-based artwork recommendation: integrating painting metadata with neural and manually-engineered visual features. UMUAI. 29, 251–290. doi: 10.1007/s11257-018-9206-9
Miller, R. E. (2020). College students and inappropriate social media posting: is it a question of personality or the influence of friends. Pers. Individ. Differ. 158:109857. doi: 10.1016/j.paid.2020.109857
Mohammadiani, R. P., and Sadeghi, H. (2020). Inferring the relationship between users’ personality and their image on flicker using deep learning and clustering. The 6th Iranian Conference on Signal Processing and Intelligent Systems (ICSPIS), Mashhad, Iran.
Moreno-Armendariz, M. A., Duchanoy Martinez, C. A., Calvo, H., and Moreno-Sotelo, M. (2020). Estimation of personality traits from portrait pictures using the five-factor model. IEEE Access. 8, 201649–201665. doi: 10.1109/ACCESS.2020.3034639
Nass, C., and Moon, Y. (2000). Machines and mindlessness: social responses to computers. J. Soc. Issues 56, 81–103. doi: 10.1111/0022-4537.00153
Nass, C., Steuer, J., and Tauber, E. R. (1994). Computers are social actors. The SIGCHI Conference on Human Factors in Computing Systems, Boston, MA.
Olson, M. K., Sutton, J., Vos, S. C., Prestley, R., Renshaw, S. L., and Butts, C. T. (2019). Build community before the storm: the National Weather Service’s social media engagement. Contingencies Crisis Manag. 27, 359–373. doi: 10.1111/1468-5973.12267
Pentina, I., and Zhang, L. (2017). Effects of social support and personality on emotional disclosure on Facebook and in real life. Behav. Inform. Technol. 36, 484–492. doi: 10.1080/0144929X.2016.1258086
Prieto, P., Briede, J. C., Beghelli, A., Canessa, E., and Barra, C. (2020). I like it elegant: imprinting personalities into product shapes. Int. J. Des. Creat. Innov. 8, 5–20. doi: 10.1080/21650349.2019.1673825
Qu, Y. (2020). Engaging publics in the mobile era: a study of Chinese charitable foundations’ use of WeChat. Public Relat. Rev. 46:101815. doi: 10.1016/j.pubrev.2019.101815
Rezaei, S., Ali, F., Amin, M., and Jayashree, S. (2016). Online impulse buying of tourism products: the role of web site personality, utilitarian and hedonic web browsing. J. Hosp. Tour. Technol. 7, 60–83. doi: 10.1108/JHTT-03-2015-0018
Saitov, I., Surikov, A., and Gorokhovatsky, L. (2021). Analysis of the relationship between the users personality traits and the images they post on social media. Procedia Comput. Sci. 193, 155–162. doi: 10.1016/j.procs.2021.10.015
Sakib, A. S., Mukta, M. S. H., Huda, F. R., Islam, A. K. M. N., Islam, T., and Ali, M. E. (2021). Identifying insomnia from social media posts: psycholinguistic analyses of user tweets. J. Med. Internet Res. 23:e27613. doi: 10.2196/27613
Samani, Z. R., Guntuku, S. C., Moghaddam, M. E., Preoţiuc-Pietro, D., and Ungar, L. H. (2018). Cross-platform and cross-interaction study of user personality based on images on twitter and Flickr. PLoS One 13:e0198660. doi: 10.1371/journal.pone.0198660
San Pedro, J., and Siersdorfer, S. (2009). Ranking and classifying attractiveness of photos in folksonomies. The 18th International Conference on World Wide Web, Madrid, Spain.
Schwartz, H. A., Eichstaedt, J. C., Kern, M. L., Dziurzynski, L., Ramones, S. M., Agrawal, M., et al. (2013). Personality, gender, and age in the language of social media: the open-vocabulary approach. PLoS One 8:e73791. doi: 10.1371/journal.pone.0073791
Segalin, C., Perina, A., Cristani, M., and Vinciarelli, A. (2017). The pictures we like are our image: continuous mapping of favorite pictures into self-assessed and attributed personality traits. IEEE Trans. Affect. Comput. 8, 268–285. doi: 10.1109/TAFFC.2016.2516994
Settanni, M., Azucar, D., and Marengo, D. (2018). Predicting individual characteristics from digital traces on social media: a meta-analysis. Cyberpsychol. Behav. Soc. Netw. 21, 217–228. doi: 10.1089/cyber.2017.0384
Skowron, M. (2016). Fusing social media cues: Personality prediction from twitter and Instagram. The 25th International Conference Companion on World Wide Web (WWW ‘16 Companion), Montréal, Québec, Canada.
Trattner, C., Moesslang, D., and Elsweiler, D. (2018). On the predictability of the popularity of online recipes. EPJ Data Sci. 7:20. doi: 10.1140/epjds/s13688-018-0149-5
Uher, J. (2008). Comparative personality research: methodological approaches. Eur. J. Personal. 22, 427–455. doi: 10.1002/per.680
Valdez, P., and Mehrabian, A. (1994). Effects of color on emotions. J. Exp. Psychol. Gen. 123, 394–409. doi: 10.1037/0096-3445.123.4.394
Vedel, I., Ramaprasad, J., and Lapointe, L. (2020). Social media strategies for health promotion by nonprofit organizations: multiple case study design. J. Med. Internet Res. 22:e15586. doi: 10.2196/15586
Wang, S., and Chen, X. (2020). Recognizing CEO personality and its impact on business performance: mining linguistic cues from social media. Inf. Manag. 57:103173. doi: 10.1016/j.im.2019.103173
Wang, Y., and Yang, Y. (2020). Dialogic communication on social media: how organizations use twitter to build dialogic relationships with their publics. Comput Human Behav. 104:106183. doi: 10.1016/j.chb.2019.106183
Waters, R. D., and Lo, K. D. (2012). Exploring the impact of culture in the social media sphere: a content analysis of nonprofit organizations’ use of Facebook. J. Intercult. Commun. Res. 41, 297–319. doi: 10.1080/17475759.2012.728772
Whittingham, N., Boecker, A., and Grygorczyk, A. (2020). Personality traits, basic individual values and GMO risk perception of twitter users. J. Risk Res. 23, 522–540. doi: 10.1080/13669877.2019.1591491
Willems, K., and Brengman, M. (2019). Fashion store personality: scale development and relation to self-congruity theory, a retrospective commentary. J. Glob. Fash. Mark. 10, 286–303. doi: 10.1080/20932685.2019.1611465
Yan, G., Pegoraro, A., and Watanabe, N. M. (2018). Student-athletes’ organization of activism at the University of Missouri: resource mobilization on twitter. J Sport Manage. 32, 24–37. doi: 10.1123/jsm.2017-0031
Yarkoni, T. (2010). Personality in 100,000 words: a large-scale analysis of personality and word use among bloggers. J. Res. Pers. 44, 363–373. doi: 10.1016/j.jrp.2010.04.001
Yazdavar, A. H., Mahdavinejad, M. S., Bajaj, G., Romine, W., Sheth, A., Monadjemi, A. H., et al. (2020). Multimodal mental health analysis in social media. PLoS One 15:e0226248. doi: 10.1371/journal.pone.0226248
Youyou, W., Kosinski, M., and Stillwell, D. (2015). Computer-based personality judgments are more accurate than those made by humans. Proc. Natl. Acad. Sci. U. S. A. 112, 1036–1040. doi: 10.1073/pnas.1418680112
Yun, J. T., Pamuksuz, U., and Duff, B. R. L. (2019). Are we who we follow? Computationally analyzing human personality and brand following on twitter. Int. J. Advert. 38, 776–795. doi: 10.1080/02650487.2019.1575106
Zhang, Q., Elsweiler, D., and Trattner, C. (2020). Visual cultural biases in food classification. Foods 9:823. doi: 10.3390/foods9060823
Keywords: Instagram, nonprofit, organization, personality, Big-Five, photos
Citation: Kim Y (2022) Personality of nonprofit organizations’ Instagram accounts and its relationship with their photos’ characteristics at content and pixel levels. Front. Psychol. 13:923305. doi: 10.3389/fpsyg.2022.923305
Edited by:
Sandra Maria Correia Loureiro, University Institute of Lisbon (ISCTE), PortugalReviewed by:
Eduardo Moraes Sarmento, University of Lisbon, PortugalJoão Ferreira Do Rosário, Instituto Politécnico de Lisboa, Portugal
Copyright © 2022 Kim. This is an open-access article distributed under the terms of the Creative Commons Attribution License (CC BY). The use, distribution or reproduction in other forums is permitted, provided the original author(s) and the copyright owner(s) are credited and that the original publication in this journal is cited, in accordance with accepted academic practice. No use, distribution or reproduction is permitted which does not comply with these terms.
*Correspondence: Yunhwan Kim, eXVuaHdhbmtpbTJAa29va21pbi5hYy5rcg==