- 1School of Business, Suzhou University of Science and Technology, Suzhou, China
- 2Postdoctoral Station of Business Administration, Fudan University, Shanghai, China
Digital tourism has developed rapidly, especially in museums. However, as people become increasingly familiar with digital museums, their use intentions and behavior have changed. Taking the Digital Palace Museum in China as an example, applying the PLS-SEM method, this study uncovers visitors’ use intentions and actual use behavior for digital museums by integrating the new UTAUT model (UTAUT2) and TTF model (TTF under social distancing) and introduces the PATS (Pandemic Anxiety Travel Scale) model to reveal how pandemic anxiety promotes the transformation of use intentions into use behavior more easily. The results show that performance expectations, hedonic motivations, habits, and task-technology-fit positively affect use intentions for digital museums. However, the price-saving orientation negatively affects use intentions. Pandemic anxiety moderates the effect of use intentions on actual behavior. When travel anxiety is relatively high, use intentions have a greater effect on use behavior for digital museums. The results reveal the influencing factors on use intentions of digital museums and the moderating effect of pandemic anxiety on the relation between use intentions and actual behavior.
Introduction
Digital tourism is a typical manifestation of the tourism industry’s paradigm shifts (Joo et al., 2021). During the past few years, tourists’ risk perceptions (Karl et al., 2020; Rather, 2021) created great obstacles to people’s tourism behavior and the tourism industry was severely affected (Rasoolimanesh et al., 2021). Under this background, technology-based tourism activities have become one of the most critical alternatives to overcome these obstacles (Itani and Hollebeek, 2021; Lv et al., 2022) since Internet technologies facilitate safe distancing in tourism activities (García-Milon et al., 2021; Nanni and Ulqinaku, 2021). As a result, tourism destinations rapidly began promoting digital virtual tourism experiences online (Talafubieke et al., 2021; Zhang et al., 2022) to capture tourist revenue by applying technologies such as online applications and digital instruments (Bec et al., 2021).
As an important component of tourist destinations (Jin et al., 2020), the museums in China are facing a key problem of over-crowding (Chen and Ryan, 2020). To tackle this issue, the digital transformation with the application of virtual (VR) and augmented reality (AR) technologies (Zhang et al., 2022) has become an effective way out. Researchers have previously analyzed the effect of digital technologies on visitors’ purchase intentions (e.g., He et al., 2018). However, the new value of digital museums is in their role as an alternative experience to on-site presence. Museums are currently experiencing new challenges and adjustments in the transformation of their customer orientation from “high-touch” to “high-tech” (Wang et al., 2021).
What is more challenging is that consumers have frequently interacted with high-tech service (Yang et al., 2022) and become increasingly familiar with digital tourism; therefore, their digital product needs and requirements change constantly. However, online tourism is still in its infancy for many tourism destinations (Chen et al., 2021). Therefore, it is still difficult for researchers to understand the public’s attitude toward virtual tourism during a crisis (Zhang et al., 2022), such as the travel restrictions caused by the epidemic. Both practical and theoretical fields have raised questions for the new research field, such as what elements of online tourism products determine people’s use intentions and behavior?
Traditional studies have used the UTAUT model (unified theory of acceptance and use of technology) and the TTF model (task–technology fit) to analyze customers’ acceptance of science and technologies. However, most studies consider the use of these technologies as supporting tourists’ offline behavior, such as making their travel plans more convenient through digital payment or online reservation methods (Martín and Herrero, 2012; Escobar-Rodríguez and Carvajal-Trujillo, 2014; Wu et al., 2021; Erjavec and Manfreda, 2022). Nevertheless, the pandemic accelerates the wide application of Internet tools (García-Milon et al., 2021) and many tourism destinations have created digital platforms to replace their on-site experiences with digital experiences. In contrast with auxiliary functions such as online payments and reservations, digital experiences replace the original on-site experience, and studies of consumer responses to these new initiatives are still scant (Itani and Hollebeek, 2021). Therefore, the variables used in the UTAUT and TTF models should be reconsidered in this new situation. However, few studies have researched this aspect of online tourism. Although scholars have discussed the use intentions for digital platforms, it is still unclear how these use intentions could transform into use behavior.
Three research gaps were identified:
Gap 1: When people become increasingly familiar with digital platforms, their requirements for digital experiences increase. Therefore, it is necessary to update and integrate the original model variables to better explain use intentions and behavior for digital museums.
Gap 2: The combination of updated UTAUT (from the consumer perspective) and TTF (from the supplier perspective) models is required to explain the use of digital museums to substitute for on-site tourism activities, which differs from earlier studies that considered digital platforms as a subsidiary tool for on-site tourism.
Gap 3: Can the travel anxiety influence the transformation of digital museum use intentions into actual use behavior?
Therefore, researchers are encouraged to analyze digital tourism experiences. The UTAUT and TTF models have great explanatory power in traditional studies of digital behavior for online businesses because they can predict use intentions from the perspectives of supplier technologies and user experiences. However, the UTAUT and TTF models should also be considered in the context of a pandemic situation.
Based on the above, taking digital museums as an example, using data collected by the questionnaire survey, and applying the PLS-SEM method, this study integrates the new UTAUT model (UTAUT2) and TTF model (TTF under social distancing), introduces the scale of pandemic anxiety, explores how the different characteristics of online products and people’s personal needs affect their use intentions for digital museums and how the pandemic anxiety promotes or hinders the transformation of use intentions into use behavior.
Literature Review
The Original and Updated TTF Models
Goodhue (1998) developed the TTF model to explain the relationship between technology and consumers’ acceptance, performance (Hung et al., 2021), and use (Kim et al., 2010). The TTF model refers to the characteristics of technologies and tasks, and how they fit together. In addition, the TTF model can also be explained as matching technological capabilities to task demands (Kim et al., 2015) or as the extent to which technological tools can help individuals to complete their tasks (Hung et al., 2021). The TTF model is used widely in analyzing use intentions for digital libraries, health care, and decision-making, in addition to the effects on performance, attitudes, and beliefs (McGill and Klobas, 2009).
Many leisure and learning interactions were digitalized during the pandemic; thus, consumers have embraced a variety of digital forms (Wang et al., 2021). The TTF model is an important model for studies of the effect of online experiences, such as technology-facilitated student learning activities (Lçi and Abubakar, 2021). However, the characteristics of the two main factors in the TTF model, technologies and tasks, have changed during the past 2 years of the pandemic (Wu et al., 2021). On the one hand, consumers’ technology demands are increasing. On the other hand, the change in task characteristics is reflected in the influence of offline social distancing. Therefore, Wang et al. (2021) also upgraded the specifically related concepts: namely, the characteristics of tasks (TAS) and technologies (TEC) under social distancing. In addition, Mehraliyev et al. (2020) demonstrated the effect of TTF on use intentions. Thus, we propose H1a, H1b, and H1c:
H1a: Characteristics of tasks under social distancing (TAS) positively affect TTF.
H1b: Characteristics of technologies under social distancing (TEC) positively affect TTF.
H1c: The TTF model positively affects use intentions for digital museums.
The UTAUT and UTAUT2 Models
Venkatesh et al. (2003) constructed the UTAUT model to explain the initial and repeated adoption of technologies (Venkatesh et al., 2012; Fong et al., 2017). The UTAUT model was constructed from the factors that affect usage intentions (Escobar-Rodríguez and Carvajal-Trujillo, 2014). In their tourism study, Martín and Herrero (2012) analyzed the online purchase intention regarding rural tourism.
Four core factors anchor the traditional UTAUT model (Fong et al., 2017; Abou-Shouk and Soliman, 2021): performance expectancy (PE), effort expectancy (EE), social influence (SI), and facilitating conditions (FC). PE is an essential element in the adoption of new technologies because it refers to the degree that tourists consider that using a digital museum will be beneficial (Venkatesh et al., 2003; García-Milon et al., 2021). Tourism studies have demonstrated the positive influence of PE on acceptance and use intentions for new technologies (García-Milon et al., 2021). EE could be understood as the degree of ease (Venkatesh et al., 2003; García-Milon et al., 2021) in using digital museums. Studies have identified the positive effect of EE on behavior intentions (García-Milon et al., 2021). SI relates to the extent to which tourists believe that key individuals in their lives believe they should visit digital museums (Venkatesh et al., 2003; García-Milon et al., 2021). The positive effect of SI on behavioral intentions was demonstrated, especially in tourism activities (García-Milon et al., 2021). FC refers to the degree to which individuals consider that there is an organizational and technical structure to support them in their use intentions (Venkatesh et al., 2003; García-Milon et al., 2021). García-Milon et al. (2021) also demonstrated the positive effect of FC on use intentions for innovative technologies in tourism activities. Martín and Herrero (2012) identified the positive effects of PE, EE, SI, and FC on online purchase intentions. Based on these findings, we propose the following hypotheses:
H2a: Performance expectancy (PE) positively affects use intentions for digital museums.
H2b: Effort expectancy (EE) positively affects use intentions for digital museums.
H2c: Social influence (SI) positively affects use intentions for digital museums.
H2d: Facilitating conditions (FC) positively affects use intentions for digital museums.
Venkatesh et al. (2012) constructed the UTAUT2 model further by upgrading the earlier UTAUT model through introducing three new elements, namely hedonic motivations (HM), price value (PV) or price-saving orientation (PO), and habits (HT). The updated version of the UTAUT model is more appropriate for studying technology adoption from a consumer perspective (Escobar-Rodríguez and Carvajal-Trujillo, 2014). The positive effect of the new variable on use intentions was identified (Escobar-Rodríguez and Carvajal-Trujillo, 2014). Although PO was previously considered to be a positive factor affecting use intentions for online platforms, people’s normal consumption demands were suppressed during the pandemic, which resulted in abnormal consumption demand and consumption psychology. Scholars subsequently identified the new behavioral trends of “retaliation consumption” (Qiao et al., 2021) and “compensatory consumption” (He et al., 2021). People confined to their homes by the pandemic are in urgent need of psychological satisfaction (Cheung et al., 2021) through online consumption, such as the digital economy consumption demands of online content like games (Xu et al., 2016), reading, and watching videos (Zheng, 2020). Hence, consumers will participate in digital museum experiences to facilitate their consumption behavior instead of in attempt to save costs. Therefore, this study assumes a new effect of PO on use intentions for digital museums. Thus, we propose hypotheses of H3a, H3b, H3c, and H4:
H3a: Hedonic motivations (HM) positively affects use intentions for digital museums.
H3b: Price-saving orientation (PO) negatively affects use intentions for digital museums.
H3c: Habits (HT) positively affects use intentions for digital museums.
H4: Use intentions positively affect use behavior for digital museums.
Pandemic Anxiety and Digital Travel Behavior
Many concepts have been established to describe the effect of the pandemic on travel behavior, such as travel fears and health concerns (Shin et al., 2022), which lead to protection motivation and travel avoidance (Zheng et al., 2021). Zenker et al. (2021) constructed the PATS model, namely the pandemic anxiety travel scale to measure people’s travel anxiety and found that pandemic anxiety harmed their intentions to travel.
Travel fear evokes different coping strategies (Zheng et al., 2021; Zhang et al., 2022) and perceived risk is linked to engagement in protective behaviors (Villacé-Molinero et al., 2021). Therefore, digital experiences have become an important alternative. Studies have identified tourists’ positive sentiments about virtual tourism (Zhang et al., 2022) and their potential switch to technologically safe substitutes (Nanni and Ulqinaku, 2021). In addition, García-Milon et al. (2021) identified the moderating role of the pandemic anxiety on the willingness of using smartphones in tourism experiences. Considering digital behavior as a substitute for tourism behavior in visiting museums, will the pandemic anxiety affect the use behavior for digital museums? Thus, we propose the following hypothesis and show our research model in Figure 1.
H5a: Pandemic anxiety regarding travel positively moderates the effect of use intentions on use behavior for digital museums.
Methodology
Research Context
Located in the heart of Beijing, China, the Palace Museum is a World Cultural Heritage Site. The Palace Museum is based on the Ming and Qing dynasties’ imperial palaces, artworks and collections. In July 2020, the Palace Museum and Tencent jointly created a WeChat mini program called Digital Palace Museum (DPM), which is an applet based on the WeChat app. With more than 1.26 billion daily users (Tencent, 2022), WeChat has become the most widely used social media application in China. Within 1 year since its launch, the number of users of Digital Palace Museum applet has exceeded 5.5 million, and the number of visits has exceeded 23 million (Guangming Net, 2021). On 21 December 2021, the DPM applet was officially updated to version 2.0, which introduced multiple new functions, such as AR live navigation, location-based services (LBS), a built-in “customized tour route,” and a bookstore, in which souvenirs can be purchased and directly mailed home (CNBN, 2021). During the pandemic, the innovative DPM applet has become a representative form of the Palace Museum and follows digital trends by offering multiple digital experiences, such as VR experiences with guides (Figure 2), three-dimensional (3D) maps, VR tours, digital relics, and virtual tour games (Figure 3), and has changed Palace Museum visitors’ experiences and expectations.
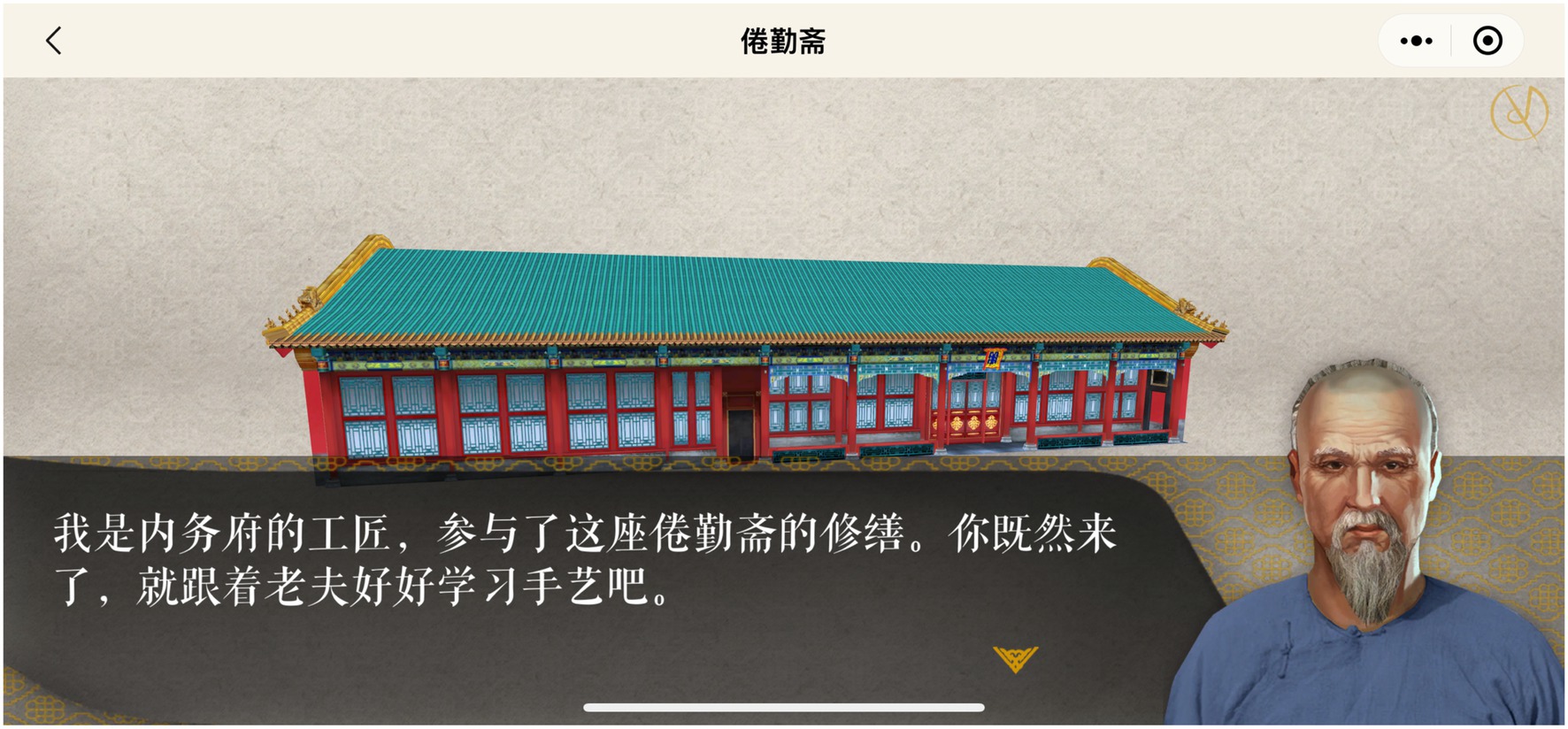
Figure 2. DPM: guided VR experience (the texts on the top of the figure show the name of the building “Qinjuanzhai”; the role in the figure says: I am a craftsman of the Imperial Household Department and participated in the repair of the House of Qinjuanzhai. Since you are here, you can learn the craft well with me).
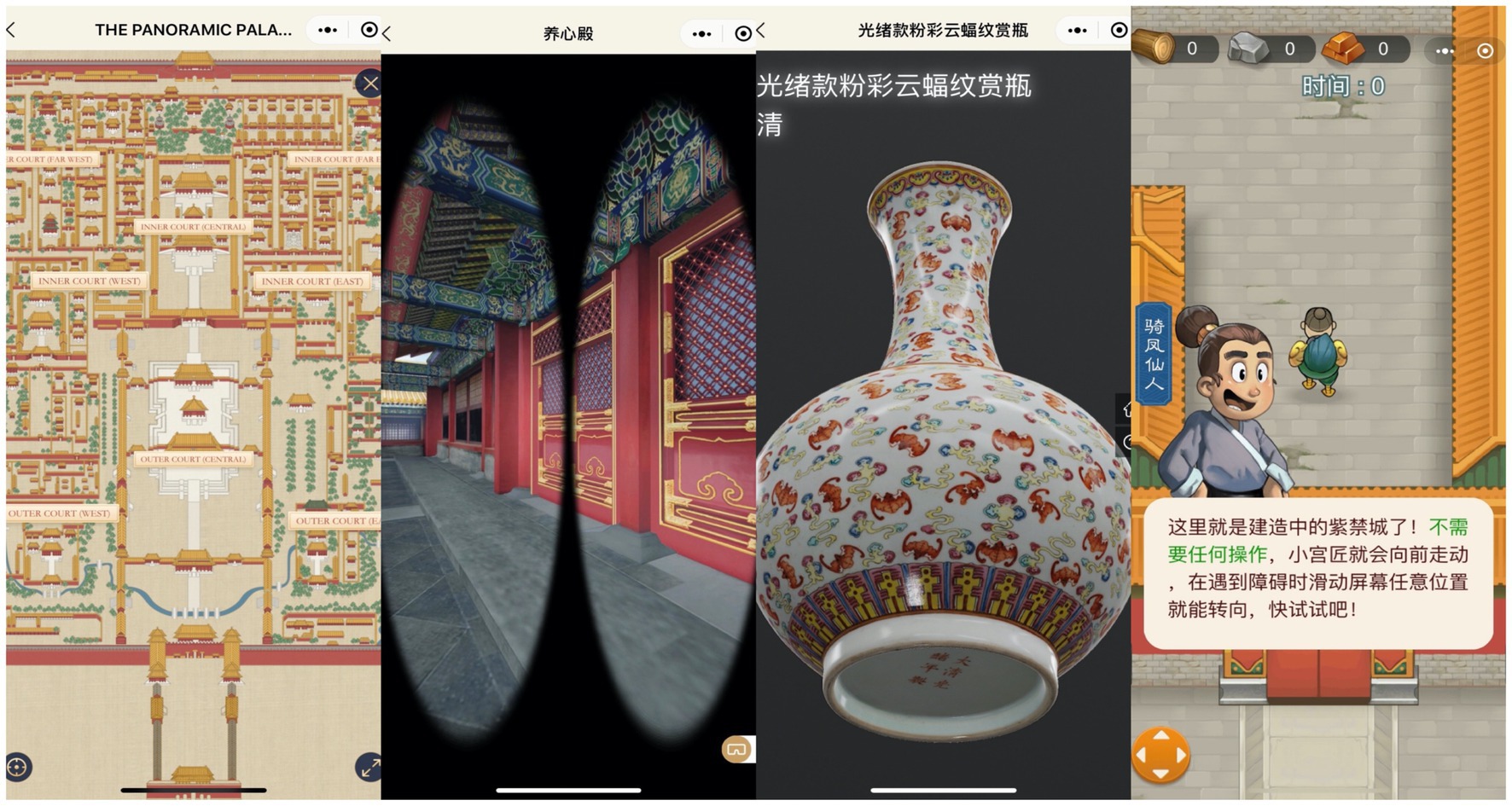
Figure 3. DPM: 3D map, VR tours, digital relics, and virtual tour games (the texts above the vase show the name of the vase and the dynasty of Qing, the cartoon character says: This is the Forbidden City under construction! Without any operation, the craftsman will move forward. When encountering obstacles, you can swipe the screen to make him turn. Try it!).
Data Collection and Measures
Using a questionnaire and interviews, this study collected data from people who had used the “digital Palace Museum” app. The five-part questionnaire adopted a Likert seven-point scale (1 = “strongly disagree” and 7 = “strongly agree”) and the respondents chose the corresponding scores based on their own experience. The first part of the questionnaire collects personal data, including gender and age, the second part uses the UTAUT2 model scale, the third part uses the scale of the TTF model under social distancing, the fourth part captures use intentions and use behavior for digital museums, and the fifth part is the PATS (Table 1). The sampling process consists of two steps. First, by conducting an open survey through the dominant communication apps in China, including Tencent QQ, WeChat, and Weibo, in addition to face-to-face in person, the researchers identified the potential participants who had used the “Digital Palace Museum” applet. Second, after determining the pool of potential participants, the researchers established and distributed paper and digital versions of the questionnaires from 2 January to 28 January 2022. In total, 236 questionnaires were collected and 15 were rejected, leaving 221 complete questionnaires with an effective rate of 93.44%. Table 2 shows the demographic information of the respondents.
Results
Measurement Model Analysis
The analysis and estimation procedure for partial least squares–structural equation modeling (PLS-SEM) can be divided into two steps. The reliability and validity of the measurement model were analyzed and the path coefficient test and model prediction ability estimation were performed for the structural model. To evaluate the reliability and validity of PLS-SEM, we must first calculate each variables’ composite reliability (CR) and average variance extracted (AVE). The CR of potential variables and Cronbach’s α all indicate the consistency of items within the variable. When Cronbach’s α is greater than 0.7, it can be considered to have good internal consistency (Fornell and Larcker, 1981). AVE is a measure of the ability of a potential variable to explain all its index items and its value must be greater than 0.5 to prove that it is feasible. In this study, the minimum value of Cronbach’s α is 0.762 and the minimum value of CR is 0.845, both of which are greater than 0.7, indicating that the potential variables in this study have good internal consistency. The minimum AVE value of potential variables is 0.579, which is greater than 0.5, indicating that the potential variables in this study have good convergence validity (Fornell and Larcker, 1981; Table 3).
The discriminant validity test can be achieved through three pathways. The first pathway is cross-loading, where the factor loadings of the items in each variable are higher than that of items in other variables (Table 4). The second pathway is Fornell and Larcker’s (1981) criterion. When the AVE value of the variable is greater than the square of the correlation coefficient between the variable and other variables, the result indicates discriminant validity. Because the AVE value is the square value of the standardized factor loading of the horizontal item under the same potential variable, the square roots are required during comparison. In this study, the AVE meets the standard (Table 5). The third pathway is the heterotrait–monotrait (HTMT) ratio test. Henseler et al. (2015) suggested that the HTMT ratio should be added to the discriminant validity analysis. When the HTMT value between two different latent variables is greater than 0.85, the result indicates that it can pass the discriminant validity test. The correlation coefficients between latent variables are 0.115 to 0.843, which meets this standard (Table 6). In summary, the validity of our findings was tested in three ways, indicating that the data passed the discriminant validity test.
Structural Model Analysis
After the reliability and validity of the measurement model were tested by confirmatory factor analysis, the structure of the model was analyzed further. The PLS algorithm was used to test the fitness of explanatory variables to the prediction of outcome variables, and 5,000 samples were selected by the bootstrap resampling method to calculate the parameters and evaluate the significance of model coefficients (Hair et al., 2011). In the PLS analysis, R2 is the primary index used to evaluate the explanatory effectiveness of the model. Chin et al. (2008) divided endogenous variables into three levels according to R2, namely, substantial, moderate, and weak, with index values of 0.67, 0.33, and 0.19, respectively. Therefore, the three indicators of 0.556, 0.578, and 0.344 identified in this study are moderate. On this basis, to further determine the stability and fitness of the model, the predictive sample reuse technique (Q2) was used, where Q2 greater than 0 indicates that the model has predictive relevance (Chin et al., 2008). The Q2 value obtained in this study was between 0.331 and 0.437, indicating that the model has cross validity.
The test results for the structural equation model are shown in Table 7. TAS had a significant positive effect on TTF; therefore, H1a was supported. TEC had a significant positive effect on TTF; therefore, H1b was supported. TTF had a significant positive effect on use intentions for digital museums; therefore, H1c was supported. PE had a significant positive effect on use intentions for digital museums; therefore, H2a was supported. HM had a significant positive effect on use intentions for digital museums; therefore, H3a was supported. PO had a significant negative effect on use intentions for digital museums; therefore, H3b was supported. HT had a significant positive effect on use intentions for digital museums; therefore, H3c was supported. Use intentions for digital museums had a significant positive effect on use behavior for digital museums; therefore, H4 was supported. However, EE, SI, and FC had no significant effect on use intentions for digital museums; therefore, H2b, H2c, and H2d were not supported.
The moderating effect of body distance on H4 was analyzed (Table 8). Using the goodness-of-fit (GoF) index proposed by Tenenhaus et al. (2005), the GoF value in this study is 0.432, which is higher than the standard GoF value of 0.36 proposed by Wetzels et al. (2009), indicating good fit. The p values are used as the criterion to evaluate whether the moderating effect is significant. The results show that H5 (p < 0.05) is significant. That is, the effect of use intentions on use behavior is moderated by travel anxiety. When travel anxiety is relatively high, use intentions have a greater effect on use behavior for digital museums (Figure 4).
Conclusion, Limitations, and Future Research
Theoretical Conclusions
This study examines visitors’ use intentions and use behavior for digital museums by integrating the new UTAUT (UTAUT2) and new TTF (TTF under social distancing) models to explore how the supply side and user factors affect the willingness of potential museum visitors to use the digital platform, and introduces the PATS model to reveal how pandemic anxiety can limit travel behavior and promote the transformation of use intentions into use behavior more effectively for digital museums.
The previous studies analyzed digital tools in tourism as an auxiliary tool in simplifying the payment or enhancing tourism experience. Such as, Skard et al. (2021) revealed the effect of digital experience on tourism intentions; Shin and Jeong (2022) explored the virtual trip as a driver evoking future intentions to visit. However, this study does not take digital tool as the antecedent or auxiliary function before or during the on-site experiences as in previous studies, but as a substitute for on-site visits during the pandemic. In addition, we discussed the production process of use intentions and its transformation into use behavior for digital museums.
In our analysis of use intentions, we found that except for PE, three of the four influential factors (i.e., EE, SI, and FC) in the traditional UTAUT model had no significant effect on use intentions for digital museums in this study. This finding differs from the conclusions of earlier studies (Martín and Herrero, 2012; García-Milon et al., 2021). Whether the task is simple or the equipment is easy to use will not affect use intentions. This result shows that as people become increasingly familiar with digital products, they take for granted their simple and good operation. At the same time, social influence will not significantly affect use intentions and behavior for digital museums, which is different from earlier studies (e.g., Šumak and Šorgo, 2016), however, consistent with the recent study (Erjavec and Manfreda, 2022), that is, SI does not have a significant role in behavioral intentions to adopt online shopping. It shows that, as a product based on personal smartphones, willingness to use digital museums is gaining more and more privacy characteristics and the choices are more personalized.
The UTAUT2 model shows that two influential factors, HM and HT, had significant positive effects on use intentions, which shows that use intentions for digital museums can be based on personal factors to a greater extent, including personal experiences, behavior, and interests. From the perspective of the UTAUT2 model, the satisfaction of personal needs, rather than social expectations, has become the main variable affecting use intentions for digital museums. Studies found that VR will lead to isolation in the future (Merkx and Nawijn, 2021) and may reduce actual travel intention (Li and Chen, 2019). Furthermore, this study found that during the pandemic, people were excluded from the social environment for longer and must endure social and physiological isolation, which means that the production of their use intentions or decisions is less influenced by social peers. These states of isolation and digitization are constantly promoting each other, which will make people become increasingly accustomed to physical and psychological isolation. Because the pandemic hinders offline consumption, people have the need for psychological compensation. Wang and Xia (2021) pointed out the “revenge travel phenomenon,” this study revealed the “revenge consumption” before people could go on a real travel. The more people want to consume, the more willing they are to participate in consuming digital museum products. Therefore, there are also opportunities for the promotion of cultural products during the pandemic.
From the perspective of the TTF model, the characteristics of tasks and technologies have changed in the context of the pandemic, namely, TAS and TEC promote people’s use intentions for digital museums. The need to avoid the risk generated by the crowding (Yin et al., 2020) makes people more willing to use digital museum products.
Furthermore, this study introduced PATS as a moderating variable to analyze the transformation from use intentions to use behavior. Gössling et al. (2020) analyzed the negative psychological results of tourists during the pandemic and restrictions on travel behavior. This study goes further and analyzes how this fear of travel promotes the transformation of use intentions into actual use behavior.
We found that when people have higher travel anxiety, their willingness to use digital museums can be transformed into use behavior more easily. When people have lower travel anxiety, they have more confidence in travel. Even if they have the willingness to use digital museums, their use intentions may not be transformed into use behavior for digital museums.
Managerial Implications
Based on our theoretical analysis, destinations must pay attention to the satisfaction of users’ personal needs from digital museums rather than the satisfaction of their social expectations. Hence, we produce the following management recommendations.
First, the positive effect of the TTF under social distance model and the performance expectations of the UTAUT2 model on use intentions show that people hope that the digital museum experience can not only meet their basic needs, but also obtain the experience faster and more efficiently. The loss of digital product users often depends on a few seconds of page loading. Therefore, waiting for pages to be converted for smartphones will make these users quickly lose patience. Hence, digital museums should improve their perceived efficiency for users with timely updates of content and improve their technological fluency.
Second, based on the positive effect of HM on use intentions, digital products must create a fun, enjoyable, and entertaining environment instead of delivering a serious history lesson. Especially for museums like the Palace Museum in particular, which is based on the Forbidden City’s long history and royal culture, innovation is particularly important to attract consumers in the digital age. It is necessary to ensure high-quality aesthetic design, construct participatory game activities, introduces virtual communication, and even introduce role-playing among other modes, so that the participants are not only holding their smartphone to “see” museum exhibits and learn about history but can engage in lively role-playing activities in an interesting digital historical world.
Third, a “personal relationship” with users could be beneficial for both digital museums and their users. The digital platform not only designs virtual museums but is also included in a handheld companion that is integrated with the users’ daily life. For example, digital museums could launch regular participation activities and communications, establish a “personal platform” relationship or a communication network between platform users, establish long-term relationships between themselves and users, and provide people with a digital community and co-creation experience (Lam et al., 2020).
Fourth, digital museums can meet their users’ need to maintain social distance during the pandemic (Im et al., 2021) and facilitate museum experiences while safely staying at home. In this way, the pandemic becomes an intensive driver for the development of digital museums. To utilize the driving force, museums should build digital experiences that differ from on-site experiences. In this way, they can give full play to the new functions that can be realized by the digital platform, such as providing 3D videos so that people can watch cultural relics at a close distance and allow them to experience protected buildings that cannot otherwise be accessed on-site through virtual “access.” These activities would improve the vividness of users’ knowledge and participation through their pleasant experiences, and emotional connections. Digital museums could then improve their brand image by including personality and character in their digital experiences.
Finally, in the context of the pandemic, digitization enables people in our modern society to meet their daily needs without leaving home, which makes them more physically isolated. Digital museums have the responsibility and ability to improve the social condition of their users by increasing their interactive and communicative functions. This would promote their users’ emotional connections on digitalized platforms and enhance their pleasure while providing historical knowledge. Therefore, digital museums not only explore and develop new markets, but also have great social and ethical significance.
Limitations and Future Research
Digital museums bring convenience to people with limited travel abilities or people who are afraid of the increasing risks in the travel environment (Yen et al., 2021). However, for those who are not good at using electronic products, large-scale digital constructions, especially in the development of digital museums that replace on-site facilities with digital facilities, may cause more inequality in accessibility, such as for the seniors or people with visual impairments. These groups of people often have high levels of travel anxiety and great potential demand for user-friendly digital products. Museums must confront this contradiction and explore how to provide useful and valuable digital products for these groups, which is also a very valuable research direction for the future.
Data Availability Statement
The raw data supporting the conclusions of this article will be made available by the authors, without undue reservation.
Author Contributions
JS: methodology, data curation, formal analysis, writing, and funding acquisition. YG: conceptualization and supervision. All authors contributed to the article and approved the submitted version.
Funding
This research was supported by the National Natural Science Foundation of China (42101225 and 72074053), Humanities and Social Sciences Youth Foundation, Ministry of Education of the People’s Republic of China (21YJCZH140), and China Postdoctoral Science Foundation (2021 M690652).
Conflict of Interest
The authors declare that the research was conducted in the absence of any commercial or financial relationships that could be construed as a potential conflict of interest.
Publisher’s Note
All claims expressed in this article are solely those of the authors and do not necessarily represent those of their affiliated organizations, or those of the publisher, the editors and the reviewers. Any product that may be evaluated in this article, or claim that may be made by its manufacturer, is not guaranteed or endorsed by the publisher.
References
Abou-Shouk, M., and Soliman, M. (2021). The impact of gamification adoption intention on brand awareness and loyalty in tourism: the mediating effect of customer engagement. J. Destin. Mark. Manag. 20:100559. doi: 10.1016/j.jdmm.2021.100559
Bec, A., Moyle, B., Schaffer, V., and Timms, K. (2021). Virtual reality and mixed reality for second chance tourism. Tour. Manag. 83:104256. doi: 10.1016/j.tourman.2020.104256
Chen, H., and Ryan, C. (2020). Transforming the museum and meeting visitor requirements: the case of the Shaanxi history museum. J. Destin. Mark. Manag. 18:100483. doi: 10.1016/j.jdmm.2020.100483
Chen, S. X., Wang, X. K., Zhang, H. Y., Wang, J. Q., and Peng, J. J. (2021). Customer purchase forecasting for online tourism: a data-driven method with multiplex behavior data. Tour. Manag. 87:104357. doi: 10.1016/j.tourman.2021.104357
Cheung, C., Takashima, M., Choi, H., Yang, H., and Tung, V. (2021). The impact of COVID-19 pandemic on the psychological needs of tourists: implications for the travel and tourism industry. J. Travel Tour. Mark. 38, 155–166. doi: 10.1080/10548408.2021.1887055
Chin, W. W., Peterson, R. A., and Brown, P. S. (2008). Structural equation modelling in marketing: Some practical reminders. J. Mark. Theory Pract. 16, 287–298. doi: 10.2753/MTP1069-6679160402
CNBN (2021). “Digital palace museum” applet 2.0 was officially released: smarter, friendlier, simpler and more open. Available at: https://baijiahao.baidu.com/s?id=1719743948050773321&wfr=spider&for=pc (Accessed January 5,2022).
Erjavec, J., and Manfreda, A. (2022). Online shopping adoption during COVID-19 and social isolation: extending the UTAUT model with herd behavior. J. Retail. Consum. Serv. 65:102867. doi: 10.1016/j.jretconser.2021.102867
Escobar-Rodríguez, T., and Carvajal-Trujillo, E. (2014). Online purchasing tickets for low cost carriers: an application of the unified theory of acceptance and use of technology (UTAUT) model. Tour. Manag. 43, 70–88. doi: 10.1016/j.tourman.2014.01.017
Fong, L. H. N., Lam, L. W., and Law, R. (2017). How locus of control shapes intention to reuse mobile apps for making hotel reservations: evidence from Chinese consumers. Tour. Manag. 61, 331–342. doi: 10.1016/j.tourman.2017.03.002
Fornell, C., and Larcker, D. F. (1981). Evaluating structural equation models with unobservable variables and measurement error. J. Market. Res. 18, 39–50. doi: 10.1177/002224378101800104
García-Milon, A., Olarte-Pascual, C., and Juaneda-Ayensa, E. (2021). Assessing the moderating effect of COVID-19 on intention to use smartphones on the tourist shopping journey. Tour. Manag. 87:104361. doi: 10.1016/j.tourman.2021.104361
Goodhue, D. L. (1998). Development and measurement validity of a task-technology fit instrument for user evaluations of information systems. Decis. Sci. 29, 105–138. doi: 10.1111/j.1540-5915.1998.tb01346.x
Gössling, S., Scott, D., and Hall, C. (2020). Pandemics, tourism and global change: A rapid assessment of COVID-19. J. Sustain. Tour. 29, 1–20. doi: 10.1080/09669582.2020.1758708
Guangming Net. (2021). Palace tickets are hard to get! “digital palace museum” helps online in-depth tour. Available at: https://m.gmw.cn/baijia/2021-07/22/35014198.html (Accessed January 25, 2022).
Hair, J. F., Ringle, C. M., and Sarstedt, M. (2011). PLS-SEM: Indeed a silver bullet. J. Mark. Theory Pract. 19, 139–152. doi: 10.2753/MTP1069-6679190202
He, W., Ma, S. S., Ma, S. Y., Wu, M. J., and Cao, L. (2021). Study on the factors affecting consumers’ compensatory consumption under the normalization of epidemic prevention and control. Times Econ. Trade 18, 16–23.
He, Z., Wu, L., and Li, X. (2018). When art meets tech: the role of augmented reality in enhancing museum experiences and purchase intentions. Tour. Manag. 68, 127–139. doi: 10.1016/j.tourman.2018.03.003
Henseler, J., Ringle, C. M., and Sarstedt, M. (2015). A new criterion for assessing discriminant validity in variance-based structural equation modeling. J. Acad. Market Sci. 43, 115–135. doi: 10.1007/s11747-014-0403-8
Hung, M.-C., Talley, P. C., Kuo, K. M., and Chiu, M. L. (2021). Exploring cloud-based bookstore continuance from a deconstructed task–technology fit perspective. J. Theor. Appl. Electron. Commer. Res. 16, 356–376. doi: 10.3390/jtaer16030023
Im, J., Kim, J., and Choeh, J. Y. (2021). COVID-19, Social distancing, and risk-averse actions of hospitality and tourism consumers: a case of South Korea. J. Destin. Mark. Manag. 20:100566. doi: 10.1016/j.jdmm.2021.100566
Itani, O. S., and Hollebeek, L. D. (2021). Light at the end of the tunnel: Visitors’ virtual reality (versus in-person) attraction site tour-related behavioral intentions during and post-COVID-19. Tour. Manag. 84:104290. doi: 10.1016/j.tourman.2021.104290
Jin, L., Xiao, H., and Shen, H. (2020). Experiential authenticity in heritage museums. J. Destin. Mark. Manag. 18:100493. doi: 10.1016/j.jdmm.2020.100493
Joo, D., Xu, W., Lee, J., Lee, C. K., and Woosnam, K. M. (2021). Residents’ perceived risk, emotional solidarity, and support for tourism amidst the COVID-19 pandemic. J. Destin. Mark. Manag. 19:100553. doi: 10.1016/j.jdmm.2021.100553
Karl, M., Muskat, B., and Ritchie, B. W. (2020). Which travel risks are more salient for destination choice? An examination of the tourist’s decision-making process. J. Destin. Mark. Manag. 18:100487. doi: 10.1016/j.jdmm.2020.100487
Kim, M. J., Chung, N., Lee, C. K., and Preis, M. W. (2015). Motivations and use context in mobile tourism shopping: applying contingency and task–technology fit theories. Int. J. Tour. Res. 17, 13–24. doi: 10.1002/jtr.1957
Kim, T., Suh, Y. K., Lee, G., and Choi, B. G. (2010). Modelling roles of task-technology fit and self-efficacy in hotel employees’ usage behaviours of hotel information systems. Int. J. Tour. Res. 12, 709–725. doi: 10.1002/jtr.787
Lam, J. M. S., Ismail, H., and Lee, S. (2020). From desktop to destination: user-generated content platforms, co-created online experiences, destination image and satisfaction. J. Destin. Mark. Manag. 18:100490. doi: 10.1016/j.jdmm.2020.100490
Lçi, A., and Abubakar, A. M. (2021). The configurational effects of task-technology fit, technology-induced engagement and motivation on learning performance during Covid-19 pandemic: An fsQCA approach. Educ. Inf. Technol. 26, 7259–7277. doi: 10.1007/s10639-021-10580-6
Li, C., and Chen, Y. (2019). Will virtual reality be a double-edged sword? Exploring the moderation effects of the expected enjoyment of a destination on travel intention. J. Destin. Mark. Manag. 12, 15–26. doi: 10.1016/j.jdmm.2019.02.003
Lv, X., Luo, J., Liang, Y., Liu, Y., and Li, C. (2022). Is cuteness irresistible? The impact of cuteness on customers’ intentions to use AI applications. Tour. Manag. 90:104472. doi: 10.1016/j.tourman.2021.104472
Martín, H. S., and Herrero, A. (2012). Influence of the user’s psychological factors on the online purchase intention in rural tourism: integrating innovativeness to the UTAUT framework. Tour. Manag. 33, 341–350. doi: 10.1016/j.tourman.2011.04.003
McGill, T. J., and Klobas, J. E. (2009). A task–technology fit view of learning management system impact. Comput. Educ. 52, 496–508. doi: 10.1016/j.compedu.2008.10.002
Mehraliyev, F., Choi, Y., and King, B. (2020). Expert online review platforms: interactions between specialization, experience, and user power. J. Travel Res. 60, 384–400. doi: 10.1177/0047287520901595
Merkx, C., and Nawijn, J. (2021). Virtual reality tourism experiences: addiction and isolation. Tour. Manag. 87:104394. doi: 10.1016/j.tourman.2021.104394
Nanni, A., and Ulqinaku, A. (2021). Mortality threats and technology effects on tourism. Ann. Tour. Res. 86:102942. doi: 10.1016/j.annals.2020.102942
Qiao, L., Zhang, Y., and Li, D. (2021). The logic of retaliation consumption and the coping strategies of enterprises. Future Dev. 45, 35–43 (in Chinese).
Rasoolimanesh, S. M., Seyfi, S., Rastegar, R., and Hall, C. M. (2021). Destination image during the COVID-19 pandemic and future travel behavior: The moderating role of past experience. J. Destin. Mark. Manag. 21:100620. doi: 10.1016/j.jdmm.2021.100620
Rather, R. A. (2021). Demystifying the effects of perceived risk and fear on customer engagement, co-creation and revisit intention during COVID-19: a protection motivation theory approach. J. Destin. Mark. Manag. 20:100564. doi: 10.1016/j.jdmm.2021.100564
Shin, H. H., and Jeong, M. (2022). Does a virtual trip evoke travelers’ nostalgia and derive intentions to visit the destination, a similar destination, and share?: nostalgia-motivated tourism. J. Travel Tour. Mark. 39, 1–17. doi: 10.1080/10548408.2022.2044972
Shin, H., Nicolau, J. L., Kang, J., Sharma, A., and Lee, H. (2022). Travel decision determinants during and after COVID-19: the role of tourist trust, travel constraints, and attitudinal factors. Tour. Manag. 88:104428. doi: 10.1016/j.tourman.2021.104428
Skard, S., Knudsen, E. S., Sjåstad, H., and Thorbjørnsen, H. (2021). How virtual reality influences travel intentions: the role of mental imagery and happiness forecasting. Tour. Manag. 87:104360. doi: 10.1016/j.tourman.2021.104360
Šumak, B., and Šorgo, A. (2016). The acceptance and use of interactive whiteboards among teachers: differences in UTAUT determinants between pre- and post-adopters. Comput. Hum. Behav. 64, 602–620. doi: 10.1016/j.chb.2016.07.037
Talafubieke, M., Mai, S., and Xialifuhan, N. (2021). Evaluation of the virtual economic effect of tourism product emotional marketing based on virtual reality. Front. Psychol. 12:759268. doi: 10.3389/fpsyg.2021.759268
Tencent (2022). Wechat users exceeds 2billion. Available at: https://new.qq.com/rain/a/20220208A03M3R00 (Accessed February 25, 2022).
Tenenhaus, M., Vinzi, V. E., Chatelin, Y. M., and Lauro, C. (2005). PLS path modeling. Comput. Stat. Data Analy. 48, 159–205. doi: 10.1016/j.csda.2004.03.005
Venkatesh, V., Morris, M. G., Davis, G. B., and Davis, F. D. (2003). User acceptance of information technology: toward a unified view. MIS Q. 27, 425–478. doi: 10.2307/30036540
Venkatesh, V., Thong, J. Y. L., and Xu, X. (2012). Consumer acceptance and use of in-formation technology: extending the unified theory of acceptance and use of technology. MIS Q. 36, 157–178. doi: 10.2307/41410412
Villacé-Molinero, T., Fernández-Muñoz, J. J., Orea-Giner, A., and Fuentes-Moraleda, L. (2021). Understanding the new post-COVID-19 risk scenario: outlooks and challenges for a new era of tourism. Tour. Manag. 86:104324. doi: 10.1016/j.tourman.2021.104324
Wang, X., Wong, Y. D., Chen, T., and Yuen, K. F. (2021). Adoption of shopper-facing technologies under social distancing: a conceptualisation and an interplay between task-technology fit and technology trust. Comput. Hum. Behav. 124:106900. doi: 10.1016/j.chb.2021.106900
Wang, J., and Xia, L. (2021). Revenge travel: nostalgia and desire for leisure travel post COVID-19. J. Travel Tour. Mark. 38, 935–955. doi: 10.1080/10548408.2021.2006858
Wetzels, M., Odekerken, S. G., and Van, O. C. (2009). Using PLS path modeling for assessing hierarchical construct models: guidelines and empirical illustration. MIS Quarterly 33, 177–195. doi: 10.2307/20650284
Wu, R. Z., Lee, H. H., and Tian, X. F. (2021). Determinants of the intention to use cross-border mobile payments in Korea among Chinese tourists: an integrated perspective of UTAUT2 with TTF and ITM. J. Theor. Appl. Electron. Commer. Res. 16, 1537–1556. doi: 10.3390/jtaer16050086
Xu, F., Tian, F., Buhalis, D., Weber, J., and Zhang, H. (2016). Tourists as Mobile gamers: Gamification for tourism marketing. J. Travel Tour. Mark. 33, 1124–1142. doi: 10.1080/10548408.2015.1093999
Yang, Y., Liu, Y., Lv, X., Ai, J., and Li, Y. (2022). Anthropomorphism and customers’ willingness to use artificial intelligence service agents. J. Hosp. Market. Manag. 31, 1–23. doi: 10.1080/19368623.2021.1926037
Yen, C. H., Tsaur, S. H., and Tsai, C. H. (2021). Destination safety climate: scale development and validation. J. Travel Tour. Mark. 38, 303–315. doi: 10.1080/10548408.2021.1906385
Yin, J., Cheng, Y., Bi, Y., and Ni, Y. (2020). Tourists perceived crowding and destination attractiveness: the moderating effects of perceived risk and experience quality. J. Destin. Mark. Manag. 18:100489. doi: 10.1016/j.jdmm.2020.100489
Zenker, S., Braun, E., and Gyimóthy, S. (2021). Too afraid to travel? Development of a pandemic (COVID-19) anxiety travel scale (PATS). Tour. Manag. 84:104286. doi: 10.1016/j.tourman.2021.104286
Zhang, S. N., Li, Y. Q., Ruan, W. Q., and Liu, C. H. (2022). Would you enjoy virtual travel? The characteristics and causes of virtual tourists’ sentiment under the influence of the COVID-19 pandemic. Tour. Manag. 88:104429. doi: 10.1016/j.tourman.2021.104429
Zheng, A. Q. (2020). Implications of novel coronavirus pneumonia on digital economy consumption and countermeasures. Inform. Commun. Technol. Policy 2020, 78–82 (in Chinese).
Zheng, D., Luo, Q., and Ritchie, B. W. (2021). Afraid to travel after COVID-19? Self-protection, coping and resilience against pandemic ‘travel fear’. Tour. Manag. 83:104261. doi: 10.1016/j.tourman.2020.104261
Keywords: digital tourism, UTAUT2 model, TTF model under social distancing, pandemic anxiety travel scale, use intentions, use behavior
Citation: Sun J and Guo Y (2022) A New Destination on the Palm? The Moderating Effect of Travel Anxiety on Digital Tourism Behavior in Extended UTAUT2 and TTF Models. Front. Psychol. 13:965655. doi: 10.3389/fpsyg.2022.965655
Edited by:
Ying Qu, Zhejiang Gongshang University, ChinaReviewed by:
Zengxian Liang, Sun Yat-sen University, ChinaYubei Hu, University of Warwick, United Kingdom
Copyright © 2022 Sun and Guo. This is an open-access article distributed under the terms of the Creative Commons Attribution License (CC BY). The use, distribution or reproduction in other forums is permitted, provided the original author(s) and the copyright owner(s) are credited and that the original publication in this journal is cited, in accordance with accepted academic practice. No use, distribution or reproduction is permitted which does not comply with these terms.
*Correspondence: Yingzhi Guo, dG91Z2FveW91eGlhbmcxMDI4QDE2My5jb20=