- 1Cork University Hospital (CUH), Cork, Ireland
- 2Five Lives SAS, Tours, France
- 3Unit of Psychological Medicine, Centre for Psychiatry and Mental Health, Wolfson Institute of Population Health, Queen Mary, University of London, London, United Kingdom
- 4Department of Psychiatry, University of Oxford, Oxford, England, United Kingdom
- 5Nuffield Department of Clinical Neurosciences, University of Oxford, Level 6, West Wing, John Radcliffe Hospital, Oxford, England, United Kingdom
- 6Department of Age Related Health Care, Tallaght University Hospital, Dublin, Ireland
- 7Trinity College Dublin, Dublin, Ireland
Modifiable risk factors for dementia remain prevalent in Ireland. A detailed examination of barriers to risk reduction behaviours in an Irish context has heretofore been lacking. Many existing studies examining barriers to brain health behaviours fail to examine how they might vary across different modifiable risk factors. This study undertook a detailed assessment of barriers to individual risk reduction behaviours. As existing research suggests that barriers may vary across sociodemographic factors, we sought to investigate the distribution of barriers across age, gender, educational status, and household income. The Five Lives Brain Health Ireland Survey is a cross-sectional survey that was distributed online amongst a non-patient population. The survey captured the following: (1) Sociodemographic factors; (2) Barriers to brain health behaviours; (3) Exposure to, and knowledge of, modifiable risk factors for dementia, namely diet, social interaction, exercise, hypertension, sleep, current low mood/depression, current smoking, alcohol consumption, cognitive stimulation, hearing impairment, diabetes, air pollution, and head injury; (4) Participants’ perceptions regarding potential for dementia prevention, and risk reduction. Lack of motivation was the most prevalent barrier to consuming a healthy diet (64%, n = 213), physical activity (77.7%, n = 167), smoking cessation (68%, n = 85), and moderation of alcohol intake (56.3%, n = 67). Practical factors were the most prevalent barriers to addressing low mood (56.5%, n = 87), air pollution (30.1%, n = 58), hearing impairment (63.8%, n = 44), diabetes (11.1%, n = 5), and head injury (80%, n = 8). Emotional factors were the most prevalent barriers to engaging in mentally stimulating activity (56.9%, n = 66), social activity (54.9%, n = 302), and good sleep (70.1%, n = 129). Lack of knowledge was the most prevalent barrier to hypertension control (14.4%, n = 29). Distribution of barriers varied across age, gender, educational status, and household income. This study investigated barriers to lifestyle change to improve brain health in an Irish sample of adults aged 50 and above. Detailed subtyping of barriers, as well as examination of differences according to age, gender, education, and income were undertaken. The heterogeneity of barriers to brain health behaviours revealed in this study highlights the necessity to tailor public health interventions to their target population, taking into account the gender, age, educational status, and income of recipients.
1. Introduction
The number of people living with dementia globally is increasing. Estimated dementia prevalence is projected to increase from 57·4 million cases globally in 2019 to 152·8 million cases in 2050 (GBD 2019 Dementia Forecasting Collaborators, 2022). Negative stereotypes of the condition predominate (Low and Purwaningrum, 2020), and dementia has been cited as one of the most feared conditions amongst members of the public (MetLife Foundation, 2011; Kessler et al., 2012). Dementia research funding is increasing, with spending doubling in many Western countries between 2011 and 2016 (Pickett and Brayne, 2019). The importance of research into dementia prevention, and risk reduction is emphasised in the WHO’s Global action plan on the public health response to dementia 2017–2025 (WHO, 2017).
Burgeoning evidence supports the potential for dementia prevention globally. The Lancet Dementia Commission identified 12 modifiable risk factors which account for 40% of dementias globally (Livingston et al., 2020). The population attributable fraction for modifiable dementia risk factors is higher in low-and middle-income countries where certain risk factors are more prevalent (Livingston et al., 2020). Less childhood education, smoking, hypertension, obesity, and diabetes are, for example, more prevalent in China, India, and certain Latin American countries as compared to high income countries (Mukadam et al., 2019). Whilst improvements in cardiovascular risk reduction in particular may account for falling dementia incidence in Western Society (Larson et al., 2013; Wolters et al., 2020), these trends have not been replicated in Low-and Middle-Income Countries (LMICs; Li et al., 2007; Wu et al., 2018).
It is recognised that cognition exists on a spectrum encompassing normal cognition, subjective cognitive decline, mild cognitive impairment (MCI), and dementia (Knopman et al., 2015). People with MCI have cognitive impairment beyond that expected for age and education but remain functionally unimpaired (Petersen et al., 1999, 2021). The entities of subjective cognitive decline and MCI have represented opportune stages at which to intervene from a risk reduction perspective. Specific risk reduction strategies for those with MCI, as well as normal cognition, are set out in the World Health Organisation Risk Reduction of Cognitive decline and dementia guidelines (World Health Organization, 2019). In clinical settings, such risk reduction is increasingly addressed in a personalised and protocolised fashion. Evolving brain health services are likely in time to fulfil a number of roles, including risk profiling, risk communication, and risk reduction (Altomare et al., 2021). Furthermore, large scale, multidomain interventions have demonstrated the potential to benefit cognition in those at risk of dementia (Kivipelto et al., 2018). Particular efforts are ongoing to harmonise lifestyle intervention studies based on the FINGER study globally (Kivipelto et al., 2020). Such ‘high risk’ prevention strategies may however have limited impact at population level (Rose, 2001). Whilst secondary prevention in those with, or indeed seeking, a diagnosis is important, shifting the focus to encompass primary prevention in the general population is vital if dividends are to be seen at a population level (Milne et al., 2021). Population based strategies are radical and may confer a large benefit (Rose, 2001). Increasing awareness of modifiable risk factors for dementia represents a core function of dementia awareness campaigns (WHO, 2017). Indeed, lack of knowledge has been cited as the main barrier to behavioural change in dementia risk reduction (Curran et al., 2021). Barriers to engaging in risk reduction behaviours are numerous and diverse. In addition to lack of knowledge, existing research suggests that barriers might include lack of motivation, lack of time, organisational issues, financial reasons, health problems, and other factors (Heger et al., 2019; Curran et al., 2021; Van Asbroeck et al., 2021). In this context, whether risk reduction strategies are implemented on an individual or population level, it is uncertain if increasing awareness of dementia risk factors alone will be sufficient to engender behavioural change. Addressing barriers to dementia risk reduction is thus essential.
As illustrated elsewhere in this issue, modifiable risk factors for dementia remain prevalent in Ireland (Dukelow et al., 2023). A detailed examination of barriers to risk reduction behaviours in an Irish context has heretofore been lacking. More broadly, many existing studies examining barriers to brain health behaviours consider barriers generically, failing to examine how they might vary across different modifiable risk factors for dementia. In this context, this study aimed to undertake a detailed assessment of barriers to individual risk reduction behaviours. As existing research suggests that barriers to brain health behaviours may vary across sociodemographic factors (Heger et al., 2019; Van Asbroeck et al., 2021), we sought to investigate the distribution of barriers across factors such as age, gender, educational status, and household income.
2. Methods
2.1 Study design and participants
The Five Lives Brain Health Ireland Survey (FLBHIS) is a cross-sectional survey that was distributed online amongst an Irish non-patient population. The FLBHIS was developed through an iterative process. Informed by current literature and expert opinion, a 100-question survey was devised (see Appendix 1). The survey was adapted from the Lifestyle Barriers for Cognitive Health Questionnaire (K. Muhammed 2021, personal communication, 2 September) to be suitable for an Irish population. Risk factors were derived from the 2020 report of the Lancet Commission on Dementia prevention, intervention, and care (Livingston et al., 2020). Additional items relating to other modifiable risk factors for cognitive decline such as sleep issues (Spira et al., 2014) and mental stimulation (Krell-Roesch et al., 2019) were also added to the survey. The barriers used in our study were derived and adapted from the behaviour change wheel, specifically from the COM-B model (Michie et al., 2011). At the heart of this model are three essential conditions, namely capability, opportunity, and motivation. From this model, five subcategory barriers were derived specifically to cover the risk factors for brain health. Conditions were developed and subcategories defined as follows: capability (emotional factors, practical factors, and lack of knowledge), opportunity (social factors), and motivation (lack of motivation). Subcategories related to capability differ from those set out by Michie who distinguished between physical and psychological capability (Michie et al., 2011). Questions were constructed in order to elicit information related to each subcategory. Examples of questions/statements related to smoking and its associated barriers, for example, include the following: ‘I want to stop smoking but I have not got the willpower to stop on my own’ (motivation-lack of motivation); ‘I do not think I could stop smoking due to the social life I have’ (opportunity-social); and ‘Stress and pressures from work or personal life mean I cannot quit smoking’ (capability-emotional). This approach allowed the main sources of behaviour to be captured at a higher level whilst allowing more granular subcategorisation where possible.
Inclusion criteria comprised people aged over 50. Participants were excluded if they had a diagnosis of dementia, or had worked in the healthcare sector. A pilot version of the survey was undertaken in January 2022 (n = 50). The survey pilot revealed a tendency for respondents to ‘straight-line’ answers, potentially indicating respondent satisficing (Reuning and Plutzer, 2020) or indeed respondent fatigue related to repetitive questions in the original survey design. Phraseology and number of questions were modified accordingly. The modified survey was subsequently administered to a further 555 volunteers in February 2022. A professional market research company, CDR Insights & Analytics Limited, was responsible for administration of the survey online to an existing market research panel. Choice of sample size was guided by logistical constraints and review of comparable literature (Heger et al., 2019). With regard to the sampling technique, a 1:1 male to female gender split was pre-specified in order to approximately reflect the Irish population. No upper age-limit was pre-determined.
Prior to commencement, this study was reviewed by The St. James’ Hospital/Tallaght University Hospital Joint Research Ethics Committee who advised that, in keeping with local institutional and legislative requirements, formal approval was not necessary.
2.2. Measures
The survey captured the following information: (1) Sociodemographic factors (gender, age, ethnicity, household income and level of education); (2) Barriers to brain health behaviours; (3) Exposure to, as well as knowledge of modifiable risk factors for dementia, namely diet, social interaction, exercise, hypertension, sleep, current low mood/depression, current smoking, alcohol consumption, cognitive stimulation, hearing impairment, diabetes, air pollution and head injury; (4) Participants’ perceptions regarding potential for dementia prevention and risk reduction.
Alcohol intake (>14 units per week), being overweight, smoking, low physical activity, sleep quality, depression/low mood, low social interaction, low mental stimulation and residing in an area with high air pollution were self-reported risk factors, whereas diabetes, hypertension and hearing impairment were defined as being diagnosed by a healthcare professional.
2.3. Statistical analysis
All personal data were removed from the dataset prior to receipt by the research team. Analyses were conducted using IBM SPSS Statistics software. Frequency counts and percentages were used to show rates of exposure to modifiable risk factors for dementia amongst the sample. For reporting and analysis purposes, barriers were broadly classified as relating to lack of knowledge, lack of motivation, emotional factors, social factors and practical factors. Frequency counts and percentages were used to illustrate prevalence of barriers relevant to individual risk factors. For all analyses, statistical significance was defined as p < 0.05.
Z-tests with a Bonferroni correction for multiple comparisons were utilised to examine distribution of barriers between groups. Significant differences were confirmed using chi-square tests. Age was categorised into two groups, defined as 50–64 years old, and 65 years and above for the purpose of analysis. Household income was categorised into two groups, defined as ≤€40,000 per year, and > €40,000 per year. Due to small numbers in this group (n = 3), those who responded that they would prefer not to disclose their gender were excluded from the dataset for analyses. Due to small numbers who had been educated to primary school level (n = 10), this group was combined with those who had been educated to secondary school level for the purpose of analysis. People who chose not to disclose their household income (n = 55) were excluded from analyses including the household income variable. Due to low numbers which precluded Z-tests, blood pressure, hearing impairment, diabetes and head injury were excluded from the analysis of sociodemographic factors as predictors of barrier prevalence.
A two-step cluster analysis was used to classify participants in distinct groups based on age, gender, education and household income. The distance measure was log-likelihood and the clustering criterion was Schwartz’s Bayesian Criterion. Initially, the automatic clustering algorithm of SPSS produced three clusters based on these settings but the cluster quality was classified as ‘Fair’ (average Silhouette measure of cohesion and separation = 0.4). To improve the cluster quality, the number of clusters was increased manually by a factor of 1 until reaching the lowest number of clusters for which a ‘Good’ cluster quality was achieved.
The percentages of people reporting barriers to alcohol use represent a fraction of those exposed to greater than 14 units of alcohol per week. For the barriers to preventing all other risk factors, the percentages refer to the fraction of people who reported exposure to the respective risk factor.
3. Results
3.1. Sample characteristics
The study population ultimately comprised 551 participants. Of the 555 volunteers to whom the survey was originally administered, three participants were excluded as they did not disclose their gender. One further participant was excluded due to an incomplete dataset. Table 1 outlines the sociodemographic characteristics of the sample. The study population comprised 551 participants with an almost equal proportion of male and female respondents (50.3% male; 49.6% female). Mean age was 59.7 years, with the majority of the sample ranging between 50 and 59 years of age (54.3%). 98.9% of respondents were of White ethnicity. Most participants were educated to secondary school level or higher (98.2%), were employed or self-employed (52.2%), and cohabited with one or more persons (74.4%).
3.2. Risk factor exposure and barrier prevalence for individual risk factors
Table 2 outlines exposure to modifiable risk factors, as well as the prevalence of different barriers across individual risk factors. Various practical barriers, and lack of motivation were the most prevalently cited barriers across multiple risk factors. Lack of motivation was the most prevalent barrier to consuming a healthy diet (64%, n = 213), physical activity (77.7%, n = 167), smoking cessation (68%, n = 85), and moderation of alcohol intake (56.3%, n = 67). Practical factors were the most prevalent barriers to addressing low mood (56.5%, n = 87), air pollution (30.1%, n = 58), hearing impairment (63.8%, n = 44), diabetes (11.1%, n = 5), and head injury (80%, n = 8). Emotional factors were the most prevalent barriers to engaging in mentally stimulating activity (56.9%, n = 66), social activity (54.9%, n = 302), and good sleep (70.1%, n = 129). Lack of knowledge was the most prevalent barrier to hypertension control (14.4%, n = 29).
3.3. Overall barrier prevalence
Figure 1 illustrates the proportion of participants reporting at least one barrier of each subtype. Practical barriers were most commonly reported, with at least one practical barrier reported by 84.2% of the study population (n = 464). One or more emotional barriers were reported by 83.5% of the population (n = 460), motivational barriers by 62.6% (n = 345), and social barriers by 48.8% (n = 269). Knowledge barriers were least commonly reported with 34.7% of participants reporting one or more knowledge barriers (n = 191).
3.4. Effect of gender on exposure to barriers
Table 3 illustrates the effect of gender on exposure to barriers. Females were significantly more likely to report stress from their work and personal life [𝝌2(1) = 5.72, p = 0.017], and people they live with [𝝌2(1) = 9.03, p = 0.003] as barriers to consuming a healthy diet. Males were more likely to report not having many friends [χ2(1) = 6.46, p = 0.011], and living too far away [χ2(1) = 5.18, p = 0.023] as barriers to engaing in social activity. Females were more likely to report the gym being too expensive as a barrier to engaging in physical activity [χ2(1) = 3.87, p = 0.049] Male participants were more likely to report caffeine intake [𝝌2(1) = 4.77, p = 0.029], work commitments [𝝌2(1) = 9.97, p = 0.002] and residing in a noisy area [𝝌2(1) = 12.12, p < 0.001] as barriers to sleep. Males were also more likely to report work related stress as a barrier to treating low mood and depression [𝝌2(1) = 3.89, p = 0.049]. Female participants were more likely to report that alcohol helped them to cope with stress [χ2(1) = 4.51, p = 0.034]. Male participants were more likely to report a lack of close friends as being a barrier to engaging in mentally stimulating activity [χ2(1) = 5.56, p = 0.018].
3.5. Effect of age on exposure to barriers
Table 4 illustrates the effect of age on exposure to barriers. Younger participants were more likely to report stress from their work or personal life as being a barrier to consuming a healthy diet [𝝌2(1) = 17.08, p < 0.001]. Younger participants were more likely to report multiple barriers to engaging in social activity, namely not having many friends [𝝌2(1) = 4.09, p = 0.04], being unable to afford the cost [𝝌2(1) = 15.92, p < 0.001], being stressed by meeting others [𝝌2(1) = 4.79, p = 0.03], and not having enough time [𝝌2(1) = 4.16, p = 0.04]. With regard to physical activity, younger participants were more likely to report home commitments as being a barrier [𝝌2(1) = 7.71, p = 0.006]. Younger participants were more likely to report stress as being a barrier to sleep [𝝌2(1) = 8.29, p = 0.004], whereas older participants were more likely to be unable to identify a specific barrier [𝝌2(1) = 3.88, p = 0.049]. Younger participants were more likely to identify work related stress as being a potential cause of low mood and depression [𝝌2(1) = 5.91, p = 0.015]. Younger participants were more likely to report not having the willpower to stop [𝝌2(1) = 13.46, p < 0.001], and stress from work or personal life [𝝌2(1) = 4.15, p = 0.04] as barriers to smoking cessation. No statistically significant differences between age groups were noted for barriers pertaining to alcohol consumption, mentally stimulating activity and air pollution.
3.6. Effect of household income on exposure to barriers
Table 5 illustrates the effect of household income on exposure to barriers. No statistically significant differences between income groups were noted for barriers pertaining to smoking, alcohol consumption, low mental stimulaton, or air pollution. Participants with lower household income were more likely to report lack of motivation as a barrier to consuming a healthy diet [𝝌2(1) = 3.98, p = 0.046]. With regard to engaging in social activity, participants with lower household income were more likely to report liking being by oneself [χ2(1) = 6.69, p = 0.01] whereas those with higher household income were more likely to report not having enough time [χ2(1) = 4.24, p = 0.04]. Participants with greater household income were more likely to report home commitments [χ2(1) = 10.25, p = 0.001] and work commitments [𝝌2(1) = 13.59, p < 0.001] as barriers to undertaking physical activity. Work commitments were also more commonly reported as a barrier to sleep by participants with greater household income [𝝌2(1) = 13.36, p < 0.001]. With regard to low mood and depression, life at home [𝝌2(1) = 9.12, p = 0.002], and financial worries [𝝌2(1) = 4.32, p = 0.04] were more commonly reported as barriers by those with lower household income. Work-related stress was more commonly reported by those with greater household income as a potential cause of low mood and depression [𝝌2(1) = 16.53, p < 0.001].
3.7. Effect of education on exposure to barriers
Table 6 illustrates the effect of education on exposure to barriers. No statistically significant differences between education groups were noted for barriers pertaining to healthy diet, sleep, smoking, alcohol consumption, low social interaction, air pollution, or engaging in mentally stimulating activity. Participants with higher levels of educational attainment were more likely to report home [𝝌2(1) = 11.19, p < 0.001] and work [𝝌2(1) = 7.31, p = 0.007] commitments as barriers to engaging in physical activity whereas physical disability was more likely to impact those educated to secondary school level [𝝌2(1) = 6.10, p = 0.013]. With regard to low mood and depression, those educated to secondary school level were less likely to know how to access services [χ2(1) = 4.75, p = 0.03]. Distrust of healthcare professionals or previous bad experiences were, on the other hand, more likely to impact those of higher educational attainment [𝝌2(1) = 7.48, p = 0.006].
3.8. Cluster analysis
Cluster analysis revealed six clusters (average Silhouette measure of cohesion and separation = 0.6). Figure 2 shows the cluster size and the demographic variable distribution within each cluster. The colour grading reflects the overall predictor importance (age: 1.00; education = 0.79; gender = 0.68; household income = 0.40). The cluster label is based on the top two most important predictors within each cluster. The cluster descriptions mention all demographic characteristics of the cluster that are significantly more prevalent (i.e., characterise >50% of participants in the cluster). Appendix 2 shows the z-tests assessing the significance of this prevalence. Cluster 1 comprised younger females (aged 50–64), who had been educated to secondary school level and had a household income of less than €40,000 (16.4%, n = 81); cluster 2 comprised older adults (aged 65–91) who were more likely to be male, educated to high school level, and to have a household income of < €40,000 (20.6%, n = 102); cluster 3 comprised secondary school educated, younger adults with high household income, who were more likely to be male (12.9%, n = 64); cluster 4 comprised university educated younger females, who were more likely to have low household income (18.6%, n = 92); cluster 5 comprised secondary school educated, younger males with low household income (12.5%, n = 62); cluster 6 comprised university educated younger males, who were more likely have high household income (19%, n = 94).
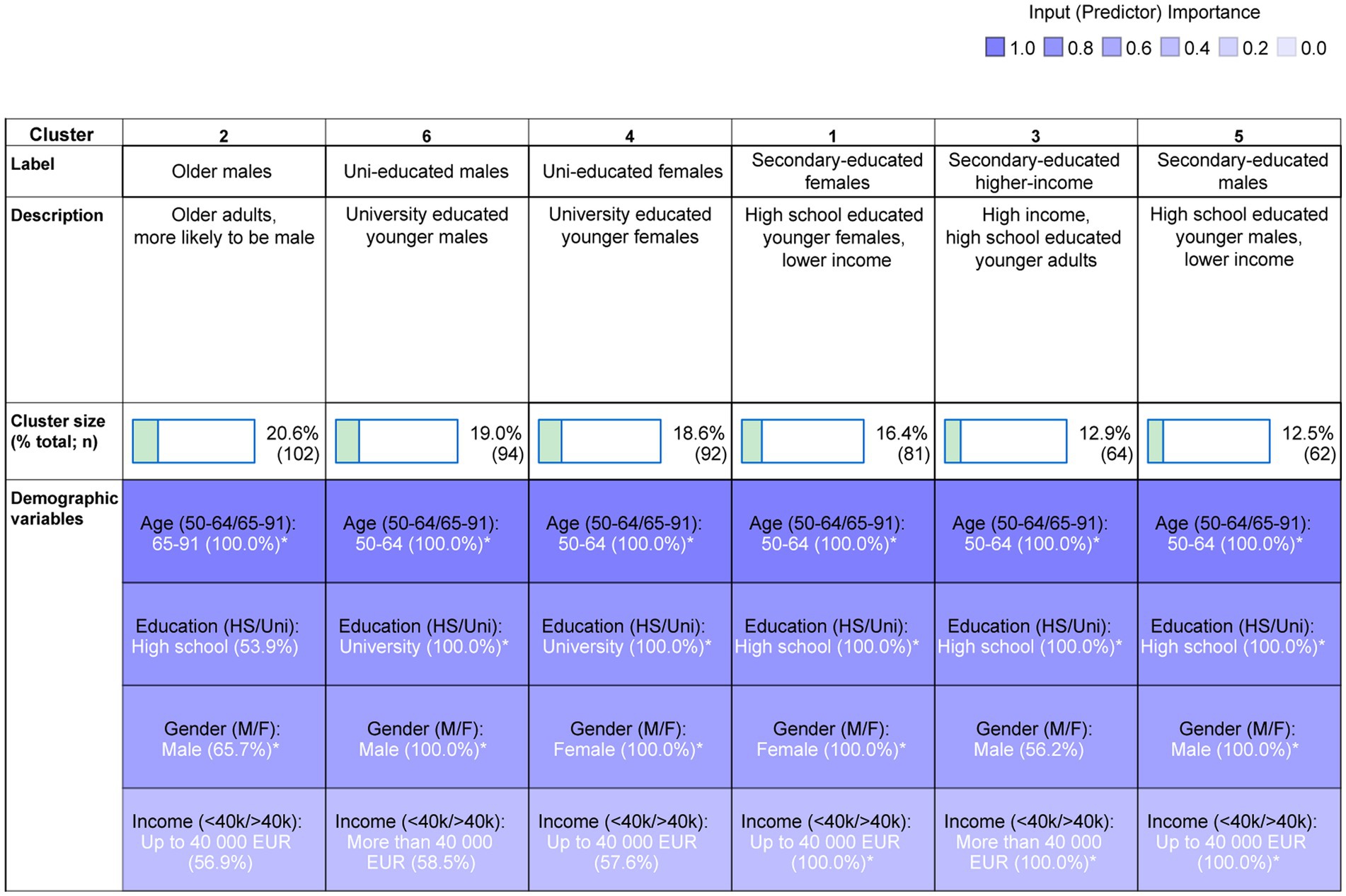
Figure 2. Two-step cluster analysis output and cluster composition. The cluster labels and descriptions are based on the relative contribution of variables within each cluster. *indicates a significant difference from 50/50 distribution.
Table 7 shows the number and proportion of participants within each cluster that have reported being exposed to any of the 13 dementia risk factors. Table 8 shows the significant differences between clusters in the prevalence of each risk factor (with value of ps not corrected for multiple comparisons). Several statistically significant differences were detected. Multiple risk factors disproportionately impact those of lower educational status (clusters A, C, and E). Secondary educated males (cluster E) were more likely to report exposure to excess alcohol when compared to other clusters, with the exception of university educated males (cluster F). They were also more likely to report smoking when compared to other clusters, with the exception of secondary educated females (cluster A). Secondary educated females (cluster A) were more likely to report poor sleep when compared to older males (cluster B), Secondary-educated, higher income (cluster C), and Uni-educated males (cluster F).
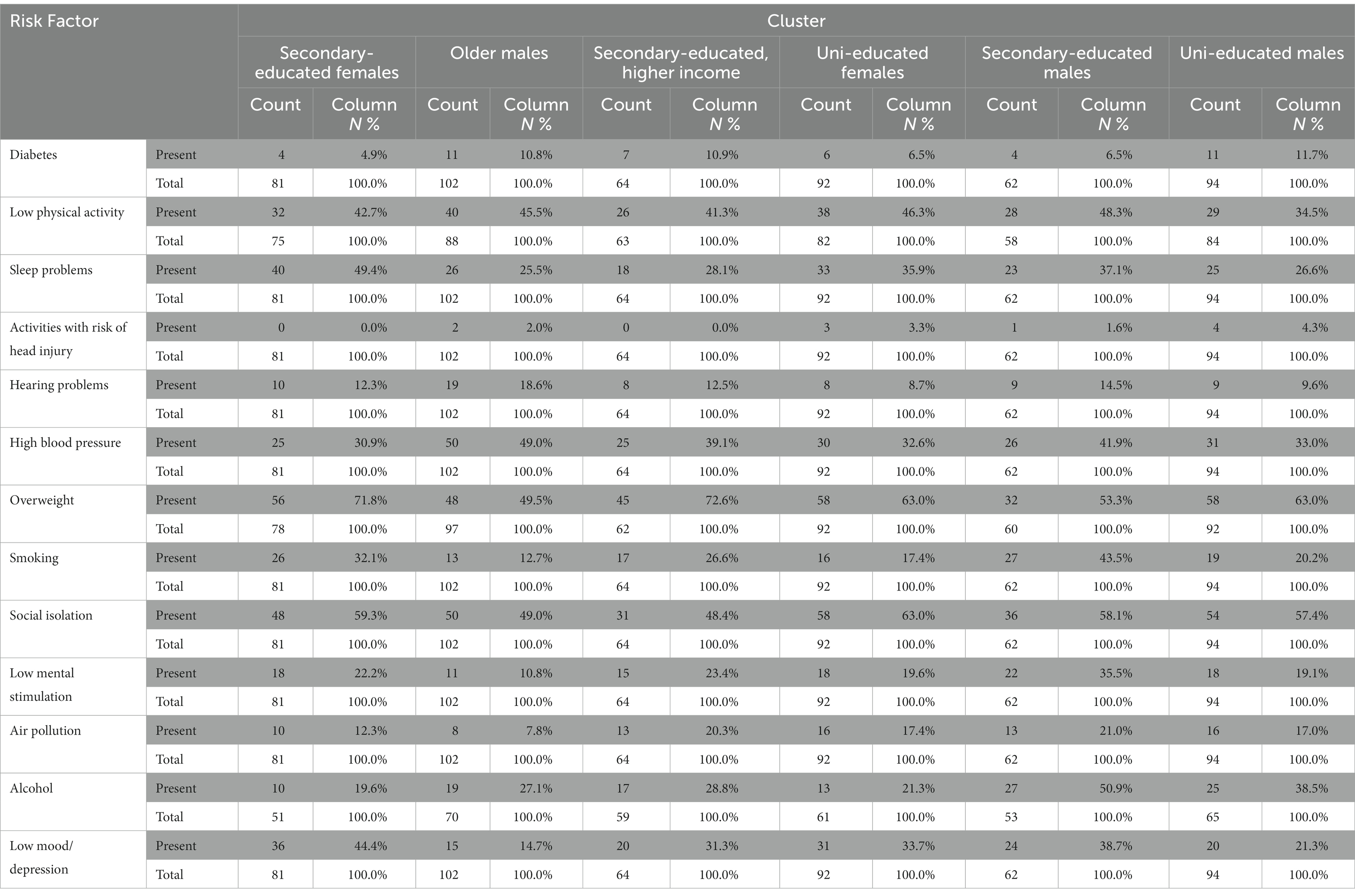
Table 7. Number and proportion of participants within each cluster that have reported being exposed to any of the 13 dementia risk factors.
4. Discussion
In this study, we investigated barriers to lifestyle change to improve brain health in an Irish sample of adults aged 50 and above. Detailed subtyping of barriers, as well as examination of differences according to age, gender, education and income were undertaken, highlighting the diverse nature of barriers to brain health behaviours. Awareness of, and exposure to, modifiable risk factors for dementia, in addition to their associations with gender, age, and education were examined elsewhere and demonstrate a lack of awareness amongst our study population that dementia could be prevented through lifestyle modifications (Dukelow et al., 2023). Increasing knowledge in this regard has been identified as a potential priority in Ireland’s National Dementia public awareness campaign (Hickey, 2019). Effective dementia risk reduction approaches are likely to encompass individual and population focused approaches. In clinical contexts, it is anticipated that evolving memory clinics and dedicated brain health services will fulfil a significant role in dementia risk assessment, communication and delivery of personalised prevention (Frisoni et al., 2023). Our study highlights the individualised nature of risk factor profiles, and barriers to brain health behaviours, thereby underlining the utility of devising personalised risk reduction plans. Beyond secondary prevention, shifting the focus to encompass primary prevention in the general population is vital if dividends are to be seen at a population level (Milne et al., 2021). As well as increasing awareness of modifiable risk factors, we posit the argument that, in order to optimise their impact, public health interventions, rooted in the sociocultural contexts of their intended recipients, should target relevant barriers to risk reduction behaviours. It is recognised that homogeneous public health messages might not be persuasive to heterogeneous audiences (Wakefield et al., 2010). The heterogeneity of barriers to brain health behaviours revealed in this study further highlights that messaging may be irrelevant if it fails to take into account the gender, age, educational status, and income of its target audience. These points are further developed below.
In this study, clusters defined by lower socio-economic status (SES; as indicated by lower education and household income, namely clusters A and E) were disproportionately impacted by multiple risk factors, and several barriers were unequally distributed across socioeconomic groups. SES is a latent construct, comprising measures of income, education and occupation, or some combination of these factors (Baker, 2014). In a global context, income inequality has increased in most high-income countries, and in some middle-income countries since 1990 (United Nations Department of Economic and Social Affairs, 2020). Lower SES is associated with a 2.1-year reduction in life expectancy (Stringhini et al., 2017), and in its own right has been recognised as a barrier to healthy behaviour at mid-life (Kelly et al., 2016). Those of lower SES have a higher risk of dementia, a difference which is explained in part by modifiable risk factors (Deckers et al., 2019). From a dementia perspective, it is known that individuals with higher household income may benefit from earlier diagnosis (Petersen et al., 2021). In our study, those of lower household income were more likely to report lack of motivation as a barrier to consuming a healthy diet. Liking being by oneself was more likely to represent a barrier to social activity in those with lower household income. Home and work commitments, on the other hand, were more likely to represent a barrier to physical activity in those with higher household income. It has been suggested that effective public health programmes must take the needs of those with lower SES into account when designing interventions for dementia prevention at individual and societal level (Deckers et al., 2019). We further postulate that large scale interventions targeted at those of lower SES should be tailored to account for the barriers to risk reduction behaviours which disproportionately impact this cohort. Public health interventions, if they do not account for sociocultural contexts, may paradoxically serve to worsen health inequalities (Stephens et al., 2012). Behaviour change interventions for low-income groups have, however, demonstrated positive effects on physical activity, smoking, and healthy eating (Bull et al., 2014), all of which represent modifiable risk factors for dementia. Specific behavioural change techniques and delivery/context components may significantly increase the effectiveness of interventions targeting healthy eating and physical activity in low-income groups (Bull et al., 2018). The investigation of interventions targeting the broader spectrum of modifiable risk factors for dementia in lower SES cohorts, and clarification of individual components conferring effectiveness, represent priorities in the field of brain health research.
Educational status is significantly associated with awareness of multiple modifiable risk factors for dementia (Dukelow et al., 2023). In the context of dementia, education has long been thought to impact cognitive reserve, reducing susceptibility to age-related changes and Alzheimer’s Disease (AD) pathology (Stern, 2012). Higher educational status appears to impact dementia risk indirectly through its relationship with higher wealth and better lifestyle (Deckers et al., 2019). Lower educational attainment in women, however, is independently associated with an increased risk of dementia-related death (Russ et al., 2013). In our study, individuals of lower educational status were more likely to report a physical disability as a barrier to engaging in physical activity. This cohort were also more likely to report lack of knowledge with regard to accessing services as a barrier to treatment of low mood and depression. Individuals educated to undergraduate level or higher were more likely to report a range of practical barriers. Education is thought to impact health via multiple potential pathways (Cohen and Syme, 2013). Whilst the relationship between education and health behaviours such as smoking, alcohol consumption, and exercise is explained in part by differences in health knowledge, most effects remain after differences in knowledge are controlled for (Kenkel, 1991). Amongst other factors, attending university education is associated with a range of preventative health behaviours (Folta et al., 2009). More broadly speaking, as opposed to relating to quantity, health benefits may relate to the delivery of quality education (Cohen and Syme, 2013). The relationship between educational status, exposure to modifiable risk factors, and broader health outcomes has profound policy implications. Educational inequality has long been a theme in Irish educational discourse (Jeffers and Lillis, 2021). A policy central to tackling educational disadvantage in an Irish context is ‘Delivering Equality of Opportunity in Schools’ (DEIS). Whilst DEIS has demonstrated consistent success in improving academic outcomes across all grade levels (Kavanagh et al., 2017), considerable disparities persist. The consistent prioritisation of educational equity in national policy may ultimately have unintended benefits on health outcomes, potentially impacting dementia risk.
Our study highlighted multiple gender differences across barriers to brain health behaviours. Gender based inequalities related to dementia are widespread (Andrew and Tierney, 2018; Bartlett et al., 2018). Globally, females are disproportionately impacted by dementia. Dementia incidence rates are higher amongst women, with a particular divergence in incidence after the age of 80 (Beam et al., 2018). Disability adjusted life years (DALYs) due to dementia are approximately 60% higher amongst women than men (WHO, 2022). In our study, certain barriers disproportionately impacted female participants. Females were more likely to report particular emotional factors as being barriers to consuming a healthy diet and reducing alcohol intake. The disproportionate impact of stress in these contexts is broadly in keeping with previous work highlighting a tendency for women to relate stress to family and health-related events more frequently than men (Matud, 2004). Females were less likely to report practical factors as barriers to getting a good night’s sleep. At a population health level, designing risk reduction strategies to target gender-specific differences in reported barriers may confer additional impact. Previously, gender has been neglected in many National Dementia Strategies (Bartlett et al., 2018). Despite dementia’s disproportionate impact on those of female gender, it has been suggested that women’s focused dementia research is underfunded. Only 12% of the $2.398 billion 2019 NIH Alzheimer’s disease budget went to women-focused research (Baird et al., 2021). Gender-sensitive public health practise is a necessity, and recent years have seen delineation of implementation strategies at European level (Oertelt-Prigione et al., 2017). Successful examples of gender-sensitive public health interventions can be identified (Gaston et al., 2007; Folta et al., 2009), and may provide a basis for addressing gender-specific differences in brain health behaviours and associated barriers. The StrongWomen—Healthy Hearts programme for example has demonstrated efficacy in reducing cardiovascular disease risk in sedentary midlife and older women who were overweight or obese (Folta et al., 2009), and may provide a template for gender specific dementia risk reduction interventions.
Lack of motivation was the most often cited barrier across multiple cardiovascular risk factors (healthy diet, physical activity, smoking and alcohol excess). Cardiovascular risk factors were prevalent amongst the study population (Table 2). Whilst mortality related to stroke (Soto et al., 2021), and coronary heart disease (Marasigan et al., 2020) in Ireland are falling, the prevalence of some cardiovascular risk factors is increasing (Marasigan et al., 2020) and globally the burden of cardiovascular disease attributable to modifiable risk factors continues to increase (Roth et al., 2020). Burgeoning evidence supports a link between individual cardiovascular risk factors and Alzheimer’s disease dementia (Takeda et al., 2020). Whilst improvements in cardiovascular risk factor control may account for falling dementia prevalence in high-income countries, we demonstrate that cardiovascular risk factors remain prevalent in Ireland. Engaging and motivating those disinclined to participate may be hard to achieve via a public health campaign. In this context, the high prevalence of lack of motivation as a barrier to cardiovascular risk factor control in our study is concerning. Many social psychological models applied in a health behaviour context assume a degree of intrinsic motivation (Hardcastle et al., 2015). Whilst campaigns with mass media components aimed at increasing physical activity have yielded short-term increases, such increases are mainly in highly motivated individuals (Wakefield et al., 2010).
We have stressed the importance of tailored health communication in dementia risk reduction interventions. Tailored health communication may demonstrate increased personal relevance, thereby increasing intent to engage in behavioural change (Bol et al., 2020). Tailored health messaging aside, the authors advocate for the design, implementation, and evaluation of preventive, personalised medicine interventions targeting modifiable risk factors for dementia, and their associated barriers. Ideally, such studies would incorporate measures of genetic, and pathological risk which confer a far higher relative risk than the lifestyle factors discussed herein (Frisoni et al., 2023). The optimal means of evaluating such complex interventions is unclear and may no longer comprise traditional gold standard study designs such as randomised, controlled trials (Garton et al., 2022). Garton et al. (2022) highlight that complex interventions require complex evaluations, underlining the potential role of a non-standard research methodology, namely transdisciplinary research (TDR), in this context. Whilst definitions of TDR vary, common characteristics include, amongst others, transcending disciplinary boundaries through a focus on theoretical unity of knowledge; the inclusion of societal actors as process participants; a focus on specific, complex, societally relevant issues; and working in a transformative manner (Lawrence et al., 2022). TDR can influence scientific productivity and capacity, increasing academic output (Grigorovich et al., 2019). As well as impacting prespecified ‘traditional’ patient outcomes, TDR may have broader social impacts which are difficult to capture through traditional techniques such as assessing policy outcomes (Grigorovich et al., 2019). TDR may represent a promising means to tackle the ‘wicked problem’ (Rittel and Webber, 1973) of increasing dementia incidence.
This study is not without its limitations. The study design introduces the potential for selection bias as participants were members of an existing market research panel. Our study population was highly educated, perhaps limiting the degree to which our results are generalisable in a global context. Our exploration of barriers is systematic, and comparatively exhaustive, based as it is on the Behaviour Change Wheel and under-pinning COM-B model (Michie et al., 2011). It is, however, limited by the use of explicit questions and absence of free text responses which might have revealed novel barriers unanticipated by the research team. We thus cannot be sure that certain barriers for each risk factor were not missed. Patient and public involvement in survey design would have further strengthened this study.
Our study’s principal strength comprises its robust and comprehensive design. Existing literature highlights a multitude of barriers to dementia risk reduction behaviours. Lack of knowledge has been highlighted as the main barrier to behavioural change (Curran et al., 2021). Two studies utilising an identical survey instrument in different populations (Heger et al., 2019; Van Asbroeck et al., 2021) highlight lack of knowledge, and lack of motivation as the two most prevalent barriers. Other factors cited included lack of time, difficulty organising, financial reasons, health problems, and ‘other reasons’. Our work is differentiated by the survey construction, and risk factor-specific nature of the barriers contained therein. The results are likely to be useful in guiding public health messaging and intervention design. A range of barriers were explored across multiple risk reduction behaviours. The heterogeneity of barriers to brain health behaviours revealed in this study highlights the necessity to tailor public health interventions to their target population, taking into account the gender, age, educational status, and income of recipients. Our study serves to add weight to one of the stated goals of the WHO Global action plan on the public health response to dementia 2017–2025, namely the need to organise national and local public health and awareness campaigns that are community and culture specific.
Data availability statement
The raw data supporting the conclusions of this article will be made available by the authors, without undue reservation.
Ethics statement
Ethical review and approval was not required for the study on human participants in accordance with the local legislation and institutional requirements. Written informed consent for participation was not required for this study in accordance with the national legislation and the institutional requirements.
Author contributions
TD and SPK were responsible for the concept and study design and were responsible for final review and editorial decisions. KM was responsible for design of the questionnaire on which the survey was based. TD, SPK, and EGL were responsible for amending and expanding the questionnaire. PV assisted with statistical analysis. Study writeup was undertaken by TD and SPK with input from LJ, EGL, PV, and IK. All authors contributed to the article and approved the submitted version.
Funding
This study received funding from Five Lives SAS/SharpTx Ltd., who sponsored performance of the survey.
Acknowledgments
We would like to thank Charles du Reau, Managing Director of market research company CDR Insights & Analytics Limited, for the survey administration and professional advice on the questionnaire design.
Conflict of interest
This study received funding from Five Lives SAS/SharpTx Ltd., who sponsored performance of the survey. EGL and KM were previously employed by Five Lives SAS/SharpTx Ltd. LJ and PV are currently employed by Five Lives. IK is a paid medical advisor of Five Lives SAS/SharpTx Ltd.
The remaining authors declare that the research was conducted in the absence of any commercial or financial relationships that could be construed as a potential conflict of interest.
Publisher’s note
All claims expressed in this article are solely those of the authors and do not necessarily represent those of their affiliated organizations, or those of the publisher, the editors and the reviewers. Any product that may be evaluated in this article, or claim that may be made by its manufacturer, is not guaranteed or endorsed by the publisher.
Supplementary material
The Supplementary material for this article can be found online at: https://www.frontiersin.org/articles/10.3389/fpsyg.2023.1101514/full#supplementary-material
References
Altomare, D., Molinuevo, J. L., Ritchie, C., Ribaldi, F., Carrera, E., Dubois, B., et al. (2021). European task force for brain health services. Brain health services: organization, structure, and challenges for implementation. A user manual for brain health services-part 1 of 6. Alzheimers Res. Ther. 13:168. doi: 10.1186/s13195-021-00827-2
Andrew, M. K., and Tierney, M. C. (2018). The puzzle of sex, gender and Alzheimer’s disease: why are women more often affected than men? Womens Health 14:174550651881799. doi: 10.1177/1745506518817995
Baird, MD, Zaber, MA, Dick, AW, Bird, CE, Chen, A, Waymouth, M, et al. (2021) The WHAM report: Societal impact of research funding for women's health in Alzheimer's disease and Alzheimer’s disease–related dementias. Santa Monica, CA: Rand Corporation.
Baker, E. H. (2014). “Socioeconomic status, definition” in The Wiley Blackwell Encyclopedia of Health, Illness, Behavior, And Society. eds. W. C. Cockerham, R. Dingwall, and S. R. Quah (Hoboken, NJ: Wiley-Blackwell), 2210–2214.
Bartlett, R., Gjernes, T., Lotherington, A. T., and Obstefelder, A. (2018). Gender, citizenship and dementia care: a scoping review of studies to inform policy and future research. Health Soc. Care Commun. 26, 14–26. doi: 10.1111/hsc.12340
Beam, C. R., Kaneshiro, C., Jang, J. Y., Reynolds, C. A., Pedersen, N. L., and Gatz, M. (2018). Differences between women and men in incidence rates of dementia and Alzheimer's disease. J. Alzheimers Dis. 64, 1077–1083. doi: 10.3233/JAD-180141
Bol, N., Smit, E. S., and Lustria, M. L. A. (2020). Tailored health communication: opportunities and challenges in the digital era. Digit. Health 6:205520762095891. doi: 10.1177/2055207620958913
Bull, E. R., Dombrowski, S. U., McCleary, N., and Johnston, M. (2014). Are interventions for low-income groups effective in changing healthy eating, physical activity and smoking behaviours? A systematic review and meta-analysis. BMJ Open 4:e006046. doi: 10.1136/bmjopen-2014-006046
Bull, E. R., McCleary, N., Li, X., Dombrowski, S. U., Dusseldorp, E., and Johnston, M. (2018). Interventions to promote healthy eating, physical activity and smoking in low-income groups: a systematic review with Meta-analysis of behavior change techniques and delivery/context. Int. J. Behav. Med. 25, 605–616. doi: 10.1007/s12529-018-9734-z
Cohen, A. K., and Syme, S. L. (2013). Education: a missed opportunity for public health intervention. Am. J. Public Health 103, 997–1001. doi: 10.2105/AJPH.2012.300993
Curran, E., Chong, T. W. H., Godbee, K., Abraham, C., Lautenschlager, N. T., and Palmer, V. J. (2021). General population perspectives of dementia risk reduction and the implications for intervention: a systematic review and thematic synthesis of qualitative evidence. PLoS One 16:e0257540. doi: 10.1371/journal.pone.0257540
Deckers, K., Cadar, D., van Boxtel, M. P. J., Verhey, F. R. J., Steptoe, A., and Köhler, S. (2019). Modifiable risk factors explain socioeconomic inequalities in dementia risk: evidence from a population-based prospective cohort study. J. Alzheimers Dis. 71, 549–557. doi: 10.3233/JAD-190541
Dukelow, T., Lawrence, E. G., Jacobson, L., Vassilev, P., Koychev, I., Muhammed, K., et al. (2023). Modifiable risk factors for dementia, and awareness of brain health behaviors: results from the five lives brain health Ireland survey (FLBHIS). Front. Psychol. 13:1070259. doi: 10.3389/fpsyg.2022.1070259
Folta, S. C., Lichtenstein, A. H., Seguin, R. A., Goldberg, J. P., Kuder, J. F., and Nelson, M. E. (2009). The strong women-healthy hearts program: reducing cardiovascular disease risk factors in rural sedentary, overweight, and obese midlife and older women. Am. J. Public Health 99, 1271–1277. doi: 10.2105/AJPH.2008.145581
Frisoni, G. B., Altomare, D., Ribaldi, F., Villain, N., Brayne, C., Mukadam, N., et al. (2023). Dementia prevention in memory clinics: recommendations from the European task force for brain health services. Lancet Reg. Health Eur. 26:100576. doi: 10.1016/j.lanepe.2022.100576
Garton, E. M., Savaş, S., Pell, C., Syurina, E. V., Stronks, K., and Cesuroglu, T. (2022). Complex interventions deserve complex evaluations: a transdisciplinary approach to evaluation of a preventive personalized medicine intervention. Front. Public Health 10:793137. doi: 10.3389/fpubh.2022.793137
Gaston, M. H., Porter, G. K., and Thomas, V. G. (2007). Prime time sister circles: evaluating a gender-specific, culturally relevant health intervention to decrease major risk factors in mid-life African-American women. J. Natl. Med. Assoc. 99, 428–438.
GBD 2019 Dementia Forecasting Collaborators (2022). Estimation of the global prevalence of dementia in 2019 and forecasted prevalence in 2050: an analysis for the global burden of disease study 2019. Lancet Public Health 7, e105–e125. doi: 10.1016/S2468-2667(21)00249-8
Grigorovich, A., Fang, M. L., Sixsmith, J., and Kontos, P. (2019). Defining and evaluating transdisciplinary research: implications for aging and technology. Disabil Rehabil Assist Technol. 14, 533–542. doi: 10.1080/17483107.2018.1496361
Hardcastle, S. J., Hancox, J., Hattar, A., Maxwell-Smith, C., Thøgersen-Ntoumani, C., and Hagger, M. S. (2015). Motivating the unmotivated: how can health behavior be changed in those unwilling to change? Front. Psychol. 6:835. doi: 10.3389/fpsyg.2015.00835
Heger, I., Deckers, K., van Boxtel, M., de Vugt, M., Hajema, K., Verhey, F., et al. (2019). Dementia awareness and risk perception in middle-aged and older individuals: baseline results of the Mijn Breincoach survey on the association between lifestyle and brain health. BMC Public Health 19:678. doi: 10.1186/s12889-019-7010-z
Hickey, D. (2019). The impact of a national public awareness campaign on dementia knowledge and help-seeking 373 intention in Ireland. 29, Dublin: Health Service Executive.
Jeffers, G., and Lillis, C. (2021). Responding to educational inequality in Ireland; harnessing teachers’ perspectives to develop a framework for professional conversations. Educ. Stud. 47, 1–27. doi: 10.1080/03055698.2021.1931043
Kavanagh, L., Weir, S., and Moran, E. (2017). The evaluation of DEIS: Achievements and attitudes of students in urban primary schools from 2007 to 2016. Report to the Department of Education and Skills.
Kelly, S., Martin, S., Kuhn, I., Cowan, A., Brayne, C., and Lafortune, L. (2016). Barriers and facilitators to the uptake and maintenance of healthy Behaviours by people at mid-life: a rapid systematic review. PLoS One 11:e0145074. doi: 10.1371/journal.pone.0145074
Kenkel, D. S. (1991). Health behavior, health knowledge, and schooling. J. Polit. Econ. 99, 287–305. doi: 10.1086/261751
Kessler, E. M., Bowen, C. E., Baer, M., Froelich, L., and Wahl, H. W. (2012). Dementia worry: a psychological examination of an unexplored phenomenon. Eur. J. Ageing 9, 275–284. doi: 10.1007/s10433-012-0242-8
Kivipelto, M., Mangialasche, F., and Ngandu, T. (2018). Lifestyle interventions to prevent cognitive impairment, dementia and Alzheimer disease. Nat. Rev. Neurol. 14, 653–666. doi: 10.1038/s41582-018-0070-3
Kivipelto, M., Mangialasche, F., Snyder, H. M., Allegri, R., Andrieu, S., Arai, H., et al. (2020). World-Wide FINGERS Network: A global approach to risk reduction and prevention of dementia. Alzheimers Dement. 16, 1078–1094. doi: 10.1002/alz.12123
Knopman, D. S., Beiser, A., Machulda, M. M., Fields, J., Roberts, R. O., Pankratz, V. S., et al. (2015). Spectrum of cognition short of dementia: Framingham heart study and Mayo Clinic study of aging. Neurology 85, 1712–1721. doi: 10.1212/WNL.0000000000002100
Krell-Roesch, J., Syrjanen, J. A., Vassilaki, M., Machulda, M. M., Mielke, M. M., Knopman, D. S., et al. (2019). Quantity and quality of mental activities and the risk of incident mild cognitive impairment. Neurology. 93, e548–e558. doi: 10.1212/WNL.0000000000007897
Larson, E. B., Yaffe, K., and Langa, K. M. (2013). New insights into the dementia epidemic. N. Engl. J. Med. 369, 2275–2277. doi: 10.1056/NEJMp1311405
Lawrence, M. G., Williams, S., Nanz, P., and Renn, O. (2022). Characteristics, potentials, and challenges of transdisciplinary research. One Earth 5, 44–61. doi: 10.1016/j.oneear.2021.12.010
Li, S., Yan, F., Li, G., Chen, C., Zhang, W., Liu, J., et al. (2007). Is the dementia rate increasing in Beijing? Prevalence and incidence of dementia 10 years later in an urban elderly population. Acta Psychiatr. Scand. 115, 73–79. doi: 10.1111/j.1600-0447.2006.00859.x
Livingston, G., Huntley, J., Sommerlad, A., Ames, D., Ballard, C., Banerjee, S., et al. (2020). Dementia prevention, intervention, and care: 2020 report of the lancet commission. Lancet 396, 413–446. doi: 10.1016/S0140-6736(20)30367-6
Low, L. F., and Purwaningrum, F. (2020). Negative stereotypes, fear and social distance: a systematic review of depictions of dementia in popular culture in the context of stigma. BMC Geriatr. 20:477. doi: 10.1186/s12877-020-01754-x
Marasigan, V., Perry, I., Bennett, K., Balanda, K., Capewell, S., O'Flaherty, M., et al. (2020). Explaining the fall in coronary heart disease mortality in the Republic of Ireland between 2000 and 2015- IMPACT modelling study. Int. J. Cardiol. 310, 159–161. doi: 10.1016/j.ijcard.2020.03.067
Matud, M. P. (2004). Gender differences in stress and coping styles. Personal. Individ. Differ. 37, 1401–1415. doi: 10.1016/j.paid.2004.01.010
Met Life Foundation (2011). What America thinks. Met Life Foundation Alzheimers Survey Report. Met Life Foundation.
Michie, S., van Stralen, M. M., and West, R. (2011). The behaviour change wheel: a new method for characterising and designing behaviour change interventions. Implement. Sci. 6:42. doi: 10.1186/1748-5908-6-42
Milne, R., Altomare, D., Ribaldi, F., Molinuevo, J. L., Frisoni, G. B., and Brayne, C. (2021). European task force for brain health services. Societal and equity challenges for brain health services. A user manual for brain health services-part 6 of 6. Alzheimers Res. Ther. 13:173. doi: 10.1186/s13195-021-00885-6
Mukadam, N., Sommerlad, A., Huntley, J., and Livingston, G. (2019). Population attributable fractions for risk factors for dementia in low-income and middle-income countries: an analysis using cross-sectional survey data. Lancet Glob. Health 7, e596–e603. doi: 10.1016/S2214-109X(19)30074-9
Oertelt-Prigione, S., Dalibert, L., Verdonk, P., Stutz, E. Z., and Klinge, I. (2017). Implementation strategies for gender-sensitive public health practice: a European workshop. J. Women's Health (Larchmt) 26, 1255–1261. doi: 10.1089/jwh.2017.6592
Petersen, J. D., Wehberg, S., Packness, A., Svensson, N. H., Hyldig, N., Raunsgaard, S., et al. (2021). Association of Socioeconomic Status with Dementia Diagnosis among Older Adults in Denmark. JAMA Netw. Open 4:e2110432. doi: 10.1001/jamanetworkopen.2021.10432
Petersen, R. C., Smith, G. E., Waring, S. C., Ivnik, R. J., Tangalos, E. G., Kokmen, E., et al. (1999). Mild cognitive impairment: clinical characterization and outcome. Arch Neurol. 56, 303–308. doi: 10.1001/archneur.56.3.303
Pickett, J., and Brayne, C. (2019). The scale and profile of global dementia research funding. Lancet 394, 1888–1889. doi: 10.1016/S0140-6736(19)32599-1
Reuning, K., and Plutzer, E. (2020). Valid vs. invalid straightlining: the complex relationship between straightlining and data quality. Survey Res. Methods 14, 439–459. doi: 10.18148/SRM/2020.V14I5.7641
Rittel, H. W. J., and Webber, M. M. (1973). Dilemmas in a general theory of planning. Policy. Sci. 4, 155–169. doi: 10.1007/BF01405730
Rose, G. (2001). Sick individuals and sick populations. Int. J. Epidemiol. 30, 427–432. doi: 10.1093/ije/30.3.427
Roth, G. A., Mensah, G. A., Johnson, C. O., Addolorato, G., Ammirati, E., Baddour, L. M., et al. (2020). GBD-NHLBI-JACC global burden of cardiovascular diseases writing group. Global burden of cardiovascular diseases and risk factors, 1990-2019: update from the GBD 2019 study. J. Am. Coll. Cardiol. 76, 2982–3021. doi: 10.1016/j.jacc.2020.11.010
Russ, T. C., Stamatakis, E., Hamer, M., Starr, J. M., Kivimäki, M., Batty, G. D., et al. (2013). Socioeconomic status as a risk factor for dementia death: individual participant meta-analysis of 86 508 men and women from the UK. Br J Psychiatry. 203, 10–17. doi: 10.1192/bjp.bp.112.119479
Stephens, N. M., Markus, H. R., and Fryberg, S. A. (2012). Social class disparities in health and education: reducing inequality by applying a sociocultural self model of behavior. Psychol. Rev. 119, 723–744. doi: 10.1037/a0029028
Stern, Y. (2012). Cognitive reserve in ageing and Alzheimer's disease. Lancet Neurol. 11, 1006–1012. doi: 10.1016/S1474-4422(12)70191-6
Stringhini, S., Carmeli, C., Jokela, M., Avendaño, M., Muennig, P., Guida, F., et al. (2017). LIFEPATH consortium. Socioeconomic status and the 25 × 25 risk factors as determinants of premature mortality: a multicohort study and meta-analysis of 1·7 million men and women. Lancet. 25, 1229–1237. doi: 10.1016/S0140-6736(16)32380-7
Soto, Á., Guillén-Grima, F., Morales, G., Muñoz, S., and Aguinaga-Ontoso, I. (2021). Trends in mortality from stroke in the European Union, 1996-2015. Eur J Neurol. 28, 182–191. doi: 10.1111/ene.14517
Spira, A. P., Chen-Edinboro, L. P., Wu, M. N., and Yaffe, K. (2014). Impact of sleep on the risk of cognitive decline and dementia. Curr Opin Psychiatry. 27, 478–483. doi: 10.1097/YCO.0000000000000106
Takeda, S., Rakugi, H., and Morishita, R. (2020). Roles of vascular risk factors in the pathogenesis of dementia. Hypertens Res. 43, 162–167. doi: 10.1038/s41440-019-0357-9
United Nations Department of Economic and Social Affairs (2020). World social report 2020, United Nations.
Van Asbroeck, S., van Boxtel, M. P. J., Steyaert, J., Köhler, S., Heger, I., de Vugt, M., et al. (2021). Increasing knowledge on dementia risk reduction in the general population: results of a public awareness campaign. Prev. Med. 147:106522. doi: 10.1016/j.ypmed.2021.106522
Wakefield, M. A., Loken, B., and Hornik, R. C. (2010). Use of mass media campaigns to change health behaviour. Lancet 376, 1261–1271. doi: 10.1016/S0140-6736(10)60809-4
WHO (2017). Global action plan on the public health response to dementia 2017–2025. Geneva: World Health Organization; Licence: CC BY-NC-SA 3.0 IGO.
WHO (2022). Dementia Fact Sheet. Available at: https://www.who.int/news-room/fact-sheets/detail/dementia (Accessed November 16, 2022).
Wolters, F. J., Chibnik, L. B., Waziry, R., Anderson, R., Berr, C., Beiser, A., et al. (2020). Twenty-seven-year time trends in dementia incidence in Europe and the United States: the Alzheimer cohorts consortium. Neurology 95, e519–e531. doi: 10.1212/WNL.0000000000010022
World Health Organization (2019) Risk reduction of cognitive decline and dementia: WHO guidelines. Geneva. Licence: CC BY-NC-SA 3.0 IGO.
Keywords: brain health, risk reduction, behavioural change, dementia prevention, lifestyle modification
Citation: Dukelow T, Vassilev P, Lawrence EG, Jacobson L, Koychev I, Muhammed K and Kennelly SP (2023) Barriers to brain health behaviours: results from the Five Lives Brain Health Ireland Survey. Front. Psychol. 14:1101514. doi: 10.3389/fpsyg.2023.1101514
Edited by:
Michelle Ellen Kelly, National College of Ireland, IrelandReviewed by:
Rachael Frost, University College London, United KingdomLouise Hopper, Dublin City University, Ireland
Copyright © 2023 Dukelow, Vassilev, Lawrence, Jacobson, Koychev, Muhammed and Kennelly. This is an open-access article distributed under the terms of the Creative Commons Attribution License (CC BY). The use, distribution or reproduction in other forums is permitted, provided the original author(s) and the copyright owner(s) are credited and that the original publication in this journal is cited, in accordance with accepted academic practice. No use, distribution or reproduction is permitted which does not comply with these terms.
*Correspondence: Tim Dukelow, dGltLmR1a2Vsb3dAaHNlLmll