- Department of Agricultural Sciences, University of Naples “Federico II”, Portici, Italy
The expanding consumption of plant proteins in the diet to overcome the environmental issues associated with animal proteins is increasing the incidence of food-induced allergic reactions. One of the 21st-century research drivers in agriculture sciences is the development and validation of concrete approaches for modulating the expression of allergenic proteins in crops before harvesting. The increasing incidence of plant food allergies is primarily induced by seed storage proteins that clinicians are experiencing recently because of the more predominant use of plant-derived proteins in the food industry. Increased availability of high-throughput technologies has generated an ever-growing number of omics data, allowing us to have better structural knowledge of SSPs and molecular properties that can inform the allergenicity assessment. The recent systems for targeted genome engineering, without double-strand DNA breaks, allow the introduction of precise modifications directly into commercial plant species. Artificial intelligence is significantly transforming scientific research across every stage, assisting scientists, processing large-scale data, making predictions, automating tasks. During this epochal change, marked by the encounter between artificial intelligence and synthetic biology, a next-generation research assistant (NGA) is coming alive. Here, we propose a new conceptual vision to facilitate and speed up the editing of cross-reactivity sites to obtain hypoallergenic cultivars and avoid pleiotropic effects. Finally, we discuss the potential applications of this new way to conceive the research. NGA may be undoubtedly capable of managing the evolution of SPP allergies through the prediction of novel epitopes, as well as the prediction of immunological response mechanisms.
Introduction
Food allergy (FA) is an IgE mediated response of the immune system of sensitive subjects to specific food proteins, as described by the National Institute of Allergy and Infectious Diseases (NIAID) guidelines. Food allergens are a tremendous concern for consumers and for the food industry around the globe, particularly because there is no reliable prophylaxis in the case of accidental ingestion, aside from the utilization of adrenaline injections to prevent severe anaphylactic reactions (Du Toit et al., 2015; Loh and Tang, 2018). Symptoms can range from mild to severe depending on environmental and genetic factors. The prevalence of food allergies is increasing because of the complexity and global nature of a modern diet (Liu et al., 2022), and the introduction in the market of novel food ingredients (Nitride et al., 2023; Quintieri et al., 2023). Antigen-presenting cells (APC) recognize and process allergens that first enter the body through the epithelial barrier, inducing Immunoglobulin E (IgE) production in B cells. IgE then binds to mast cells and basophils, sensitizing the body. Subsequent exposure to the same antigen activates mast cells and basophils and releases potent inflammatory mediators. The substances released by these cells (such as histamine, prostaglandins, and leukotrienes) are the major factors responsible for symptoms like skin rash or anaphylaxis (Sathe et al., 2016; Dong et al., 2021). According to the World Health Organization (WHO), the incidence of FA has been increasing in developed countries over the past 2 decades. The European Academy of Allergy and Clinical Immunology estimates the prevalence of allergies among European children to be between 1.7% and 5%. Researchers have observed similar trends in developing countries alongside their economic growth (Leung et al., 2018; Prescott SL et al., 2013). Indeed, the rapid increase in FA is evident in Asian countries and in Africa (Arakali et al., 2017). Gupta et al. (2013) calculated that food allergies cost the economy a total of $24.8 billion per year. Among the allergenic proteins, plant allergens affect 1 in 100 adults and 1 in 10 children (Shahali and Dadar, 2018). According to WHO/IUIS (International Union of the Immunological Societies) classification and curated databases like COMPARE (https://db.comparedatabase.org/) and FARRP AllergenOnline databases (http://www.allergenonline.org/), several plant-based foods have the potential to induce severe anaphylactic reactions in susceptible individuals (Shahali and Dadar, 2018). The prevalence of sensitization to a given allergenic ingredient significantly varies across geographical regions and subjects ages. The recent WHO/FAO expert consultation includes only gluten, peanut, and specific tree nuts (almond, cashew, hazelnut, pecan, pistachio, and walnut) in the list of global priority allergenic ingredients, restraining several others to individual countries (Datema et al., 2015; Datema et al., 2018). Most plant allergens are proteins associated with storage proteins in seeds (Breiteneder and Radauer, 2004). Seed storage proteins (SSPs) encompass prolamins and cupins, which are the most common groups of plant allergens (Table 1). Cupin and prolamin superfamilies exemplify class I food allergens (Moreno, 2007; Moreno and Clemente, 2008). These proteins are normally considered highly stable to thermal denaturation, acidic environment and gastrointestinal proteases and can cause moderate to severe allergic reactions (Harrer et al., 2010; Mendes et al., 2019). Members of the cupin superfamily are characterized by two short-conserved consensus sequences and a beta-barrel structural core domain, which is the source of its designation “cupin” (Breiteneder and Mills, 2005). Vicilins and legumins are 7S- and 11S-type globulins, respectively, with two core domains (bicupins), considered the prevalent SSPs in several nuts and pulses (Breiteneder and Mills, 2005). Scientists divided the Prolamin superfamily into various families. The nonspecific lipid transfer proteins (nsLTPs), is typically located in several parts of the plant, including seeds, leaves, roots and fruits. The 2S albumins, α-Amylases and cereal prolamins are generally found in the seeds (Breiteneder and Mills, 2005). These three protein superfamilies include almost 65% of the identified plant food allergens so far. The nsLTP and the 2S albumin are major triggers of allergic reactions in peanut and soybean allergy (Ara h 2, 6 and 7 and Gly m 8, respectively) (Taylor et al., 2021). In cashew nut, Ana o 1 and Ana o 2 (cupin superfamily) have been classified as major allergens, according to Mendes et al. (2019). Radauer et al. (2017) reported that in peanut (Arachis hypogaea), the 2S albumins, Ara h 2 and Ara h 6 are the most potent allergens followed by the cupins Ara h 1 and Ara h 3, 7S vicilin and 11S globulins, respectively. Gly m 5 and Gly m 6 have been identified in Soybean (Glycine max), the Cor a 11 and Cor a 9 in Hazelnut (Corylus avellana), the Jug r 2 in walnut, and several other SSPs belonging to the cupin superfamily, have been identified as important allergens in legumes, nuts, and other fruit seeds (Radauer et al., 2017; Barre et al., 2018). Storage proteins like Act d 12, an 11S globulin, or Fra a 3, an ns-LTP, are the major allergenic proteins in fruits such as kiwifruit and strawberry, respectively, whose seeds are consumed alongside the flesh (Sirvent et al., 2014; Sirvent et al., 2014). The WHO FAO Expert committee on Risk assessment of Food Allergens recently included Kiwi fruit in the watch list for CODEX as emerging allergen (Risk Assessment of Food Allergens, 2022). Recently, Barre et al. (2018) reported a detailed table of fruits containing cupin allergens (legumin, vicilin, germin and germin-like protein). High sequence and structural homology frequently result in the cross-allergenicity, which is often attributed to the similar epitopic sequences (Shahali and Dadar, 2018). Therefore, allergic individuals sensitive to specific SSPs of a particular plant may exhibit cross-reactive IgE to homologous proteins found in other plants.
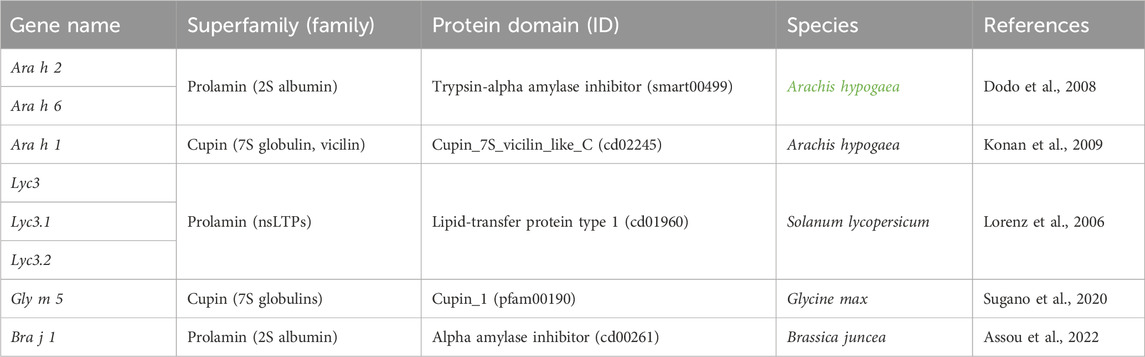
TABLE 1. Seed storage protein genes well-characterized using RNAi and CRISPR/Cas9 as biotechnological approaches.
The development of genetic approaches to obtain hypoallergenic crops dates to over 25 years ago. These approaches were based on classical breeding and mutagenesis (Takahashi et al., 1996; Hajika et al., 1998). To enhance comprehension of SSPs and mitigate food allergies, researchers have employed RNA interference (RNAi) to change the expression of SSPs. Notably, the downregulation of specific allergenic protein expression in plants has yielded promising outcomes, as evidenced in studies involving peanuts and tomato plants (Singh and Bhalla, 2008; Scheurer, 2009). On peanuts plants, researchers employed RNAi-mediated gene silencing to eliminate the immunodominant Ara h 1 and Ara h 2 proteins (Table 1). Chu et al. (2008) used biolistic methods that led to a stable transformation of the Ara h 2 gene in peanut plants. As Ara h 2 has sequence homology with Ara h 6, RNAi plasmid targeting Ara h 2 was reported to silence both the Ara h 2 and Ara h 6 proteins expression. Seed weight and germination of transgenic plants showed no significant differences. By utilizing patient sera as a source of IgE antibodies, they observed a decreased level of immunobinding, demonstrating a noteworthy reduction in the allergenicity level. Similarly, the Agrobacterium-mediated transformation method was used to silence Ara h 2 allergen (Dodo et al., 2008). However, several factors can interfere with the accumulation of RNAi molecules in the cell cytoplasm, which is why RNAi may not fully silence genes (Tseng et al., 2009; Ulrich et al., 2015). In this respect, it is worth mentioning that the dose of apple predicted to elicit a reaction in 50% of the allergic population is of 150 mg of protein. Therefore, it is still acceptable to partially silence genes, and researchers could define a suitable level of silencing for each allergenic commodity. Le et al., 2006 achieved substantial suppression of the Lyc e 3, an allergenic nsLTPs in transgenic tomato plants, with a decreased accumulation compared to wild-type plants (Table 1). Interestingly, one RNAi construct efficiently silenced both Lyc e 3 isoforms Lyc e 3.01 and Lyc e 3.02, which showed a 76.5% base pair identity. Using two patient sera with specific IgE-reactivity to Lyc e 3 it was possible to show that the ability to recognize the nsLTP Lyc e 3 in the deficient tomatoes was reduced by 10–100-fold. While in vitro testing using sera of allergic subjects is quite informative about the IgE binding capacity of protein exacts from transgenic plants, in vivo testing (skin testing and food challenges) are the golden standards for assessing the allergenic potential. Lorenz Y et al. (2006), tested genetically modified foods with reduced allergenic potential in humans with a remarkable reduction in skin reactivity. In addition to nsLTPs, researchers targeted tomato profilin Lyc e 1 for gene silencing through RNAi in transgenic tomato plants (Le et al., 2006). However, despite the utilization of RNAi as a tool to decrease the expression of allergenic proteins, silencing a single allergen will not automatically abolish the risk of food allergy in the population, however, it could contribute to its mitigation (Peters et al., 2011). Often, a tiny amount of allergenic food proteins can also trigger food allergic reactions and multiple gene products can confer these reactions (Knoll et al., 2011; Graham et al., 2020). Hence, researchers can achieve the simultaneous inhibition of gene family members by selecting nucleotide regions of high sequence homology, which are often found within coding regions (Chu et al., 2008). Therefore, scientists have the option to adopt other strategies to overcome problems derived from using RNAi.
During the Neolithic Revolution, starting approximately 10,000–13,000 years ago (Stitzer and Ross-Ibarra, 2018), gene knockout (or loss-of-function alleles) played a prominent role in crop evolution during domestication, diversification, and improvement (Monroe et al., 2020; Zhang, 2023). The modern biotech approaches, as an integral supplement to conventional plant breeding, have tried to emulate this complex natural process, proving to be assisted-evolution technologies for enhancing crop traits (Zsögön et al., 2018). CRISPR/Cas9 system often led to DNA double strand breaks, nucleotide deletion and frameshift with consequent gene loss-of-function and thus resulting as one of the most popular genome editing (GE) tool for plant breeding (Aksoy et al., 2022; Li et al., 2022). The development and implementation of this technology resulted in the obtainment of crops with enhanced resistance to biotic and abiotic stresses and reduced breeding cycles (Yıldırım et al., 2023). However, complete gene knockouts or knockdowns of fitness-relevant genes, and not only, may induce pleiotropic phenotypes (Bauer et al., 2015; Auge et al., 2019; Hanika et al., 2021), as one gene can directly or indirectly effects multiple traits (Burstin et al., 2007; Nguyen et al., 2015). Moreover, limited genomic information may lead to unintended off-target effects or result in new mutant variants due to repair post-cleavage (Yıldırım et al., 2023).
CRISPR/Cas9-mediated gene knockout has also been used to mitigate FA by targeting genes encoding for SSPs. For example, a recent study introduced large deletions and frameshifts in both Bra j 1 homologous, the major allergen in brown mustard plants (Brassica juncea) (Table 1). Mutations were inherited by the T1 generation, which showed reduced or absent quantities of Bra j 1 in seed extracts (Assou et al., 2022). Similarly, the CRISPR-Cas9 system has been used to induce mutations in genes encoding the 7S alpha and alpha’ subunits of beta-conglycinin, the Gly m 5 allergen in soybeans (Table 1). This approach resulted in the generation of cas9-free offspring devoid of these major allergenic proteins in the seeds, while preserving the processing properties (Sugano et al., 2020). However, to detect undesired effects that may have escaped visual evaluations, a deep phenotypic characterization is generally necessary. An innovative work was also carried out in the Italian durum wheat Svevo grains. Camerlengo et al. (2020) employed a multiplexing CRISPR-Cas9 marker gene-free approach, to induce mutations in the α-amylase/trypsin inhibitors (ATI) subunits WTAI-CM3 and WTAI-CM16, which are recognized as key proteins involved in the development of bakers’ asthma. Although they successfully achieved a complete gene knock-out, they noted the emergence of pleiotropic effects attributed to the activation of the ATI 0.28 pseudogene in the edited lines. By interfering with well-established gene networks upstream and downstream, classical gene knock-out can lead to unintended pleiotropic effects, consistent with these notions. Scientists have advanced our understanding by revealing that plant systems biology, including seed development and maturation, involves complex and intricate gene networks (Duan et al., 2023). Although the regulatory network controlling SSPs in developing seeds is being elucidated, loss-of-function mutations in master regulators of seed maturation give rise to unwanted seed phenotypes, including a significant reduction of SSPs (Tang et al., 2008). Selecting SSP targets for obtaining allergenic-free cultures requires a comprehensive understanding of gene regulation in plant growth and development (Ojolo et al., 2018). Modern and innovative approaches of precise genome editing (PGE) methods, well reviewed by Yıldırım et al. (2023), enable the modification of specific gene ortions by using catalytically impaired Cas9, allowing the direct writing of new genetic information into a specified DNA site (Andolfo et al., 2016). Recent advancements, including the identification of new Cas variants and the development of new editing strategies, demonstrated the evolving landscape of PGE techniques in plants (Yanagawa et al., 2023; Yıldırım et al., 2023). This would enable us to modify specifically the epitopic sequence instead of silencing the proteins, thus avoiding the risk of pleiotropic effects associated with traditional gene knock-out techniques. Additionally, it could be utilized to modify the cross-reactivity sites on genes encoding for SSPs. In particular, the huge amount of information obtained so far about the utilization of PGE techniques in plants through the CRISPR-Cas complex (Figure 1), including homology direct repair (HDR), base editing, prime editing, RNA guided transposases and the last CRISPR-tool called programmable addition via site-specific targeting element (PASTE) (Strecker et al., 2019; Anzalone et al., 2020; Anzalone et al., 2022) could be contextually evaluated by AI software to identify the best techniques for a specific purpose (e.g., changes of a specific nucleotide, introduction or substitution of specific nucleotide fragments).
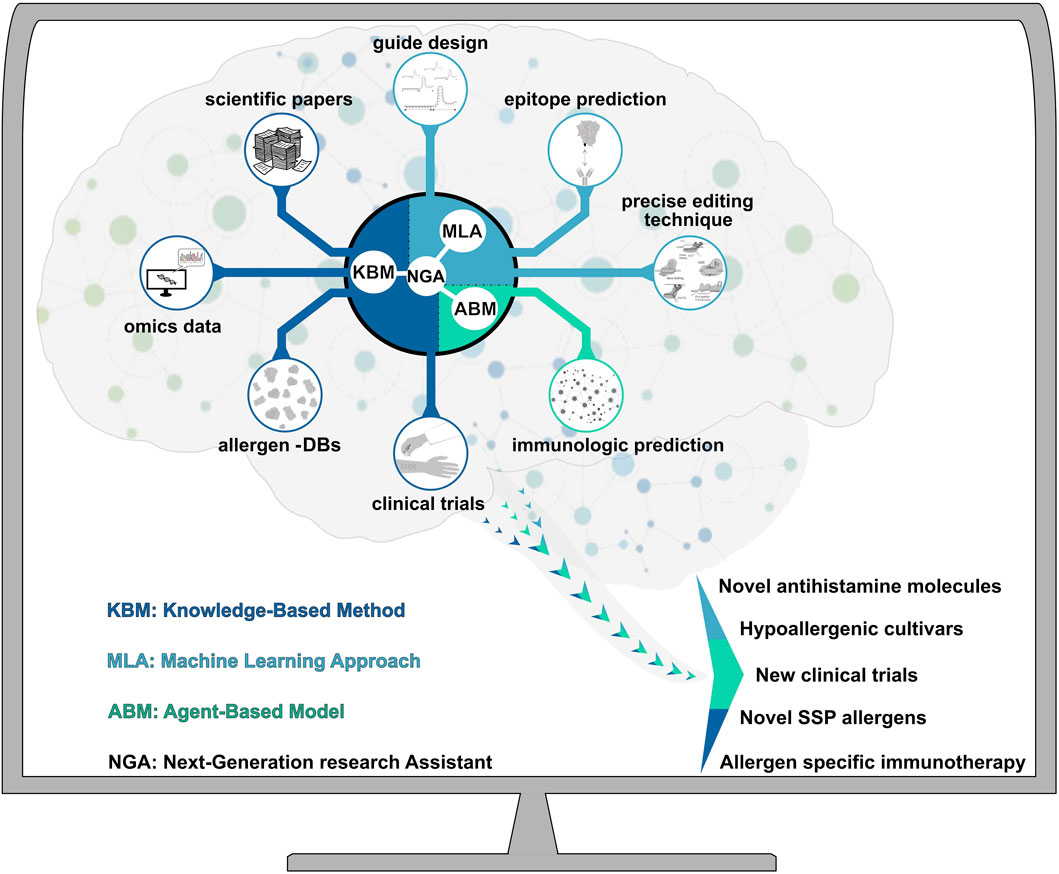
FIGURE 1. Next-generation research assistant (NGA) for artificial intelligence–assisted synthetic biology. By integrating knowledge-based methods (KBMs), machine learning approaches (MLAs), and agent-based models (ABMs), NGA can largely advance our insights on SSP gene precise editing and promote the development of therapeutic strategies for seed storage allergens. KBM can identify feature goals from public and private allergenic multi-omic (genomics, transcriptomics, proteomics, metabolomics and phenomics) and digital (allergens DBs) data, clinical trials, and scientific knowledge. MLAs can identify novel epitopes (antigen-binding site), off- and on-targets and the most suitable precise editing technique. ABMs can predict innate immunity responses related to a specific SSP allergen. Arrow shows the different outputs (novel antihistamine molecules, hypoallergenic cultivars, new clinical trials, novel food allergens and allergen specific immunotherapy) generated by artificial research activity.
Discussion
In this scenario, synthetic biology (SynBio) approaches that meet artificial intelligence (AI) techniques became a genuine opportunity to tackle SSP-related food allergies (MacMath et al., 2023). A next-generation research assistant (NGA) based on AI-SynBio combination can be conceived as laboratory equipment supporting research activity (Figure 1). AI includes knowledge-based methods (KBMs), machine learning approaches (MLAs), and agent-based models (ABMs) (Maserat, 2022). KBMs can determine the goals of feature selection of allergenic omics data (genomics, transcriptomics, proteomics, metabolomics, and phenomics), biological, and hypersensitive reaction entities. Researchers have access to a vast amount of information obtained through different platforms, such as omics data obtained by single cells or multicellular tissue analysis (Haque et al., 2019). The innovation of high throughput biotechnologies has allowed the accumulation of omics data at an alarming rate, thus introducing the era of ‘big data’. Discovering and elucidating complex interactions among different data can be a complex and time-consuming process (Amoroso et al., 2023). Published and private data generated by different omic platforms and instruments (e.g., omics data, scientific evidence, datasets about clinical tests) can be simultaneously analyzed by NGA. Interestingly, KBMs have been utilized to facilitate the interpretation and understanding of complex omics data. For instance, Türei et al. (2021) conducted a fascinating study by analyzing several omics data to investigate cell–cell interactions and downstream intracellular signaling processes in humans, mice, and rats. In recent years, computational methods have also been used to create accessible allergen databases containing a large amount of information on allergen epitopes or antibodies (Kadam et al., 2017). Hence, to handle biological big data, modern prediction models have largely advanced our insights into the treatment of allergies also by discovering plant genetic patterns that could be potentially modified by precise editing techniques (Haque et al., 2019; Li et al., 2022). The development and application of MLAs and ABMs can perform analyses for epitope and immunological predictions (Jumper et al., 2021). Indeed, machine-learning approaches have been used to predict the potential allergenicity of novel proteins (Jumper et al., 2021; Nedyalkova et al., 2023). ML methods have contributed to the understanding of allergens and their potential epitopes in plants through epitope prediction and immunological analysis (Wang et al., 2013). Therefore, we assume that in the future, NGA will be able to drive scientists toward more precise and efficient research in the field of synthetic biology, for the development of new hypoallergenic crops, also via the identification of novel food allergens, antihistamine molecules and development of clinical trials (Figure 1). (Garcia-Moreno and Gutiérrez- Naranjo, 2022). Deep learning neural networks have been utilized to predict the activity and specificity of guide RNA sequences, used by PGEs (Luo et al., 2019). AI has already helped in predicting the best in vitro conditions for plant growth (Malabadi et al., 2023). Similarly, Akin et al. (2020) employed a data mining technique on tissue culture studies, which turned out to be a promising tool to analyze non-parametric data. Plant biological datasets are frequently distorted, high dimensional, dynamic, and heterogeneous (Ben Ayed et al., 2022). NGA could also support the choice of parametric or nonparametric machine learning algorithms, analyzing the data proprieties to be processed. Indeed, the most crucial stages for the efficient use of MLAs are data transformation and preprocessing pipeline design (Booij et al., 2022). NGA, by integrating different AI-systems, can serve as a scientific instrument for the analysis of information obtained through different methods, facilitating the identification of the best PGE technique, transformation, and regeneration procedure for specific purpose (Figure 1). Hence, in this global vision, PGE became an instrument, in which synthesis of DNA/RNA fragments, vector assembly, and choice of the transformation method became directly available for the researcher, depending on the final research goal. So far, ML has already proven to be a revolutionary tool in supporting clinical studies, medical and precise diagnosis (Mansour et al., 2021; Fang et al., 2022) with application in medical diagnostics and for detecting, forecasting, and predicting of immunity responses (Nawaz et al., 2021; Buendgens et al., 2022). In this context, the utilization of an NGA for the comprehensive analysis of allergens could be completely revolutionary in human health. NGA could work as a “fully fledged researcher” (Figure 1), providing new insights for developing hypoallergenic cultures. In the near future researchers should consider NGA as a helpful laboratory equipment to speed up and improve their research work. However, evaluating the outputs will still remain a fundamental part of the process.
Author contributions
AE: Investigation, Writing–original draft, Data curation. CA: Data curation, Investigation, Writing–original draft. CN: Writing–review and editing. GA: Conceptualization, Funding acquisition, Investigation, Supervision, Visualization, Writing–original draft, Writing–review and editing.
Funding
The author(s) declare that no financial support was received for the research, authorship, and/or publication of this article. This work was supported by the Ph.D. fund in Food Science of the Department of Agricultural Sciences of the University of Naples “Federico II.”
Conflict of interest
The authors declare that the research was conducted in the absence of any commercial or financial relationships that could be construed as a potential conflict of interest.
Publisher’s note
All claims expressed in this article are solely those of the authors and do not necessarily represent those of their affiliated organizations, or those of the publisher, the editors and the reviewers. Any product that may be evaluated in this article, or claim that may be made by its manufacturer, is not guaranteed or endorsed by the publisher.
References
Akin, M., Eyduran, S. P., Eyduran, E., and Reed, B. M. (2020). Analysis of macro nutrient related growth responses using multivariate adaptive regression splines. Plant Cell, Tissue Organ Cult. (PCTOC) 140 (3), 661–670. doi:10.1007/s11240-019-01763-8
Aksoy, E., Yildirim, K., Kavas, M., Kayihan, C., Yerlikaya, B. A., Çalik, I., et al. (2022). General guidelines for CRISPR/Cas-based genome editing in plants. Mol. Biol. Rep. 49 (12), 12151–12164. doi:10.1007/s11033-022-07773-8
Amoroso, C. G., D’Esposito, D., Aiese Cigliano, R., and Ercolano, M. R. (2023). Comparison of tomato transcriptomic profiles reveals overlapping patterns in abiotic and biotic stress responses. Int. J. Mol. Sci. 24 (4), 4061. doi:10.3390/ijms24044061
Andolfo, G., Iovieno, P., Frusciante, L., and Ercolano, M. R. (2016). Genome-editing technologies for enhancing plant disease resistance. Front. Plant Sci. 7, 1813. doi:10.3389/fpls.2016.01813
Anzalone, A. V., Gao, X. D., Podracky, C. J., Nelson, A. T., Koblan, L. W., Raguram, A., et al. (2022). Programmable deletion, replacement, integration and inversion of large DNA sequences with twin prime editing. Nat. Biotechnol. 40 (5), 731–740. doi:10.1038/s41587-021-01133-w
Anzalone, A. V., Koblan, L. W., and Liu, D. R. (2020). Genome editing with CRISPR–Cas nucleases, base editors, transposases and prime editors. Nat. Biotechnol. 38 (7), 824–844. doi:10.1038/s41587-020-0561-9
Arakali, S. R., Green, T. D., and Dinakar, C. (2017). Prevalence of food allergies in south asia. Ann. Allergy, Asthma & Immunol. 118 (1), 16–20. doi:10.1016/j.anai.2016.09.441
Assou, J., Zhang, D., Roth, K. D. R., Steinke, S., Hust, M., Reinard, T., et al. (2022). Removing the major allergen Bra j I from brown mustard (Brassica juncea) by CRISPR/Cas9. Plant J. 109 (3), 649–663. doi:10.1111/tpj.15584
Auge, G. A., Penfield, S., and Donohue, K. (2019). Pleiotropy in developmental regulation by flowering- pathway genes: is it an evolutionary constraint? New Phytol. 224 (1), 55–70. doi:10.1111/nph.15901
Barre, A., Simplicien, M., Benoist, H., and Rougé, P. (2018). Fruit allergies: beware of the seed allergens. Rev. Française d’Allergologie 58 (4), 308–317. doi:10.1016/j.reval.2018.01.009
Bauer, C. R., Li, S., and Siegal, M. L. (2015). Essential gene disruptions reveal complex relationships between phenotypic robustness, pleiotropy, and fitness. Mol. Syst. Biol. 11 (1), 773. doi:10.15252/msb.20145264
Ben Ayed, R., Hanana, M., Ercisli, S., Karunakaran, R., Rebai, A., and Moreau, F. (2022). Integration of innovative technologies in the agri-food sector: the fundamentals and practical case of DNA-based traceability of olives from fruit to oil. Plants 2022, 11, 1230. doi:10.3390/plants11091230
Booij, T. M., Chiscop, I., Meeuwissen, E., Moustafa, N., and Hartog, F. T. H. d. (2022). ToN_IoT: the role of heterogeneity and the need for standardization of features and attack types in IoT network intrusion data sets. IEEE Internet Things J. 9 (1), 485–496. doi:10.1109/jiot.2021.3085194
Breiteneder, H., and Mills, E. N. C. (2005). Plant food allergens—structural and functional aspects of allergenicity. Biotechnol. Adv. 23 (6), 395–399. doi:10.1016/j.biotechadv.2005.05.004
Breiteneder, H., and Radauer, C. (2004) A classification of plant food allergens☆. J. Allergy Clin. Immunol. 113(5): 821–830. doi:10.1016/j.jaci.2004.01.779
Buendgens, L., Cifci, D., Ghaffari Laleh, N., van Treeck, M., Koenen, M. T., Zimmermann, H. W., et al. (2022). Weakly supervised end-to-end artificial intelligence in gastrointestinal endoscopy. Sci. Rep. 12 (1), 4829. doi:10.1038/s41598-022-08773-1
Burstin, J., Marget, P., Huart, M., Moessner, A., Mangin, B., Duchene, C., et al. (2007). Developmental genes have pleiotropic effects on plant morphology and source capacity, eventually impacting on seed protein content and productivity in pea. Plant Physiol. 144 (2), 768–781. doi:10.1104/pp.107.096966
Camerlengo, F., Frittelli, A., Sparks, C., Doherty, A., Martignago, D., Larré, C., et al. (2020). CRISPR-Cas9 multiplex editing of the α- amylase/trypsin inhibitor genes to reduce allergen proteins in durum wheat. Front. Sustain. Food Syst. 4, 104. doi:10.3389/fsufs.2020.00104
Chu, Y., Faustinelli, P., Ramos, M. L., Hajduch, M., Stevenson, S., Thelen, J. J., et al. (2008). Reduction of IgE binding and nonpromotion of Aspergillus flavus fungal growth by simultaneously silencing Ara h 2 and Ara h 6 in peanut. J. Agric. Food Chem. 56 (23), 11225–11233. doi:10.1021/jf802600r
Datema, M. R., Van Ree, R., Asero, R., Barreales, L., Belohlavkova, S., de Blay, F., et al. (2018). Component- resolved diagnosis and beyond: multivariable regression models to predict severity of hazelnut allergy. Allergy 73 (3), 549–559. doi:10.1111/all.13328
Datema, M. R., Zuidmeer-Jongejan, L., Asero, R., Barreales, L., Belohlavkova, S., de Blay, F., et al. (2015). Hazelnut allergy across Europe dissected molecularly: a EuroPrevall outpatient clinic survey. J. Allergy Clin. Immunol. 136 (2), 382–391. doi:10.1016/j.jaci.2014.12.1949
Dodo, H. W., Konan, K. N., Chen, F. C., Egnin, M., and Viquez, O. M. (2008). Alleviating peanut allergy using genetic engineering: the silencing of the immunodominant allergen Ara h 2 leads to its significant reduction and a decrease in peanut allergenicity. Plant Biotechnol. J. 6 (2), 135–145. doi:10.1111/j.1467-7652.2007.00292.x
Dong, X., Wang, J., and Raghavan, V. (2021). Critical reviews and recent advances of novel non-thermal processing techniques on the modification of food allergens. Crit. Rev. Food Sci. Nutr. 61 (2), 196–210. doi:10.1080/10408398.2020.1722942
Duan, Z., Li, Q., Wang, H., He, X., and Zhang, M. (2023). Genetic regulatory networks of soybean seed size, oil and protein contents. Front. Plant Sci. 14, 1160418. doi:10.3389/fpls.2023.1160418
Du Toit, G., Roberts, G., Sayre, P. H., Bahnson, H. T., Radulovic, S., Santos, A. F., et al. (2015). Randomized trial of peanut consumption in infants at risk for peanut allergy. N. Engl. J. Med. 372 (9), 803–813. doi:10.1056/NEJMoa1414850
Fang, Y., Idnay, B., Sun, Y., Liu, H., Chen, Z., Marder, K., et al. (2022). Combining human and machine intelligence for clinical trial eligibility querying. J. Am. Med. Inf. Assoc. 29 (7), 1161–1171. doi:10.1093/jamia/ocac051
Garcia-Moreno, F. M., and Gutiérrez-Naranjo, M. A. (2022). ALLERDET: a novel web app for prediction of protein allergenicity. J. Biomed. Inf. 135, 104217. doi:10.1016/j.jbi.2022.104217
Graham, F., Caubet, J., and Eigenmann, P. A. (2020). Can my child with IgE- mediated peanut allergy introduce foods labeled with “may contain traces”? Pediatr. Allergy Immunol. Santos A 31 (6), 601–607. doi:10.1111/pai.13244
Gupta, R., Holdford, D., Bilaver, L., Dyer, A., Holl, J. L., and Meltzer, D. (2013). The economic impact of childhood food allergy in the United States. JAMA Pediatr. 167 (11), 1026–1031. doi:10.1001/jamapediatrics.2013.2376
Hajika, M., Takahashi, M., Sakai, S., and Matsunaga, R. (1998). Dominant inheritance of a trait lacking.BETA.- conglycinin detected in a wild soybean line. Jpn. J. Breed. 48 (4), 383–386. doi:10.1270/jsbbs1951.48.383
Hanika, K., Schipper, D., Chinnappa, S., Oortwijn, M., Schouten, H. J., Thomma, B. P. H. J., et al. (2021). Impairment of tomato WAT1 enhances resistance to vascular wilt fungi despite severe growth defects. Front. Plant Sci. 12, 721674. doi:10.3389/fpls.2021.721674
Haque, S., Ahmad, J. S., Clark, N. M., Williams, C. M., and Sozzani, R. (2019). Computational prediction of gene regulatory networks in plant growth and development. Curr. Opin. Plant Biol. 47, 96–105. doi:10.1016/j.pbi.2018.10.005
Harrer, A., Egger, M., Gadermaier, G., Erler, A., Hauser, M., Ferreira, F., et al. (2010). Characterization of plant food allergens: an overview on physicochemical and immunological techniques. Mol. Nutr. Food Res. 54 (1), 93–112. doi:10.1002/mnfr.200900096
Jumper, J., Evans, R., Pritzel, A., Green, T., Figurnov, M., Ronneberger, O., et al. (2021). Highly accurate protein structure prediction with AlphaFold. Nature 596 (7873), 583–589. doi:10.1038/s41586-021-03819-2
Kadam, K., Karbhal, R., Jayaraman, V. K., Sawant, S., and Kulkarni-Kale, U. (2017). AllerBase: a comprehensive allergen knowledgebase. Database 2017, bax066. doi:10.1093/database/bax066
Knoll, J. E., Ramos, M. L., Zeng, Y., Holbrook, C. C., Chow, M., Chen, S., et al. (2011). TILLING for allergen reduction and improvement of quality traits in peanut (Arachis hypogaea L.). BMC Plant Biol. 11 (1), 81. doi:10.1186/1471-2229-11-81
Konan, K. N., Ananga, A. O., and Dodo, H. (2009). “Reducing peanut allergy risks by means of genetic modification,” in Modification of seed composition to promote health and nutrition. Editor Krishnan, H. (Madison, WI: Agronomy Monograph) 51, 289–302.
Le, L. Q., Lorenz, Y., Scheurer, S., Fötisch, K., Enrique, E., Bartra, J., et al. (2006). Design of tomato fruits with reduced allergenicity by dsRNAi- mediated inhibition of ns- LTP (Lyc e 3) expression. Plant Biotechnol. J. 4 (2), 231–242. doi:10.1111/j.1467-7652.2005.00175.x
Leung, A. S. Y., Wong, G. W. K., and Tang, M. L. K. (2018). Food allergy in the developing world. J. Allergy Clin. Immunol. 141 (1), 76–78. doi:10.1016/j.jaci.2017.11.008
Li, R., Li, L., Xu, Y., and Yang, J. (2022). Machine learning meets omics: applications and perspectives. Briefings Bioinforma. 23 (1), bbab460. doi:10.1093/bib/bbab460
Liu, Q., Lin, S., and Sun, N. (2022). How does food matrix components affect food allergies, food allergens and the detection of food allergens? A systematic review. Trends Food Sci. Technol. 127, 280–290. doi:10.1016/j.tifs.2022.07.009
Loh, W., and Tang, M. (2018). Adjuvant therapies in food immunotherapy. Immunol. Allergy Clin. N. Am. 38 (1), 89–101. doi:10.1016/j.iac.2017.09.008
Lorenz, Y., Enrique, E., Lequynh, L., Fötisch, K., Retzek, M., Biemelt, S., et al. (2006). Skin prick tests reveal stable and heritable reduction of allergenic potency of gene-silenced tomato fruits. J. Allergy Clin. Immunol. 118 (3), 711–718. doi:10.1016/j.jaci.2006.05.014
Luo, J., Chen, W., Xue, L., and Tang, B. (2019). Prediction of activity and specificity of CRISPR-Cpf1 using convolutional deep learning neural networks. BMC Bioinforma. 20 (1), 332. doi:10.1186/s12859-019-2939-6
MacMath, D., Chen, M., and Khoury, P. (2023). Artificial intelligence: exploring the future of innovation in allergy Immunology. Curr. Allergy Asthma Rep. 23 (6), 351–362. doi:10.1007/s11882-023-01084-z
Malabadi, R. B., Tl, N., Kolkar, K. P., Chalannavar, R. K., Mudigoudra, B., Lakshminarayana, L., et al. (2023). Cannabis sativa: applications of artificial intelligence AI and plant tissue culture for micropropagation. Int. J. Res. Innovation Appl. Sci. 8, 117–142. doi:10.51584/ijrias.2023.8614
Mansour, R. F., Escorcia-Gutierrez, J., Gamarra, M., Gupta, D., Castillo, O., and Kumar, S. (2021). Unsupervised deep learning based variational autoencoder model for COVID-19 diagnosis and classification. Pattern Recognit. Lett. 151, 267–274. doi:10.1016/j.patrec.2021.08.018
Maserat, E. (2022). Integration of artificial intelligence and CRISPR/Cas9 system for vaccine design. Cancer Inf. 21, 11769351221140102. doi:10.1177/11769351221140102
Mendes, C., Costa, J., Vicente, A. A., Oliveira, M. B. P. P., and Mafra, I. (2019). Cashew nut allergy: clinical relevance and allergen characterisation. Clin. Rev. Allergy & Immunol. 57 (1), 1–22. doi:10.1007/s12016-016-8580-5
Monroe, J. G., Arciniegas, J. P., Moreno, J. L., Sánchez, F., Sierra, S., Valdes, S., et al. (2020). The lowest hanging fruit: beneficial gene knockouts in past, present, and future crop evolution. Curr. Plant Biol. 24, 100185. doi:10.1016/j.cpb.2020.100185
Moreno, F. J. (2007). Gastrointestinal digestion of food allergens: effect on their allergenicity. Biomed. Pharmacother. 61 (1), 50–60. doi:10.1016/j.biopha.2006.10.005
Moreno, F. J., and Clemente, A. (2008). 2S albumin storage proteins: what makes them food allergens? Open Biochem. J. 2, 16–28. doi:10.2174/1874091X00802010016
Nawaz, M. S., Fournier-Viger, P., Shojaee, A., and Fujita, H. (2021). Using artificial intelligence techniques for COVID-19 genome analysis. Appl. Intell. 51 (5), 3086–3103. doi:10.1007/s10489-021-02193-w
Nedyalkova, M., Vasighi, M., Azmoon, A., Naneva, L., and Simeonov, V. (2023). Sequence-based prediction of plant allergenic proteins: machine learning classification approach. ACS Omega 8 (4), 3698–3704. doi:10.1021/acsomega.2c02842
Nguyen, T.-P., Cueff, G., Hegedus, D. D., Rajjou, L., and Bentsink, L. (2015). A role for seed storage proteins in Arabidopsis seed longevity. J. Exp. Bot. 66 (20), 6399–6413. doi:10.1093/jxb/erv348
Nitride, C., D’Auria, G., and Ferrara, A. (2023). “Environmental, nutritional, and cultural sustainability of novel food protein sources,” in Sustainable food science - a comprehensive approach (Elsevier), 199–215. Available at: https://linkinghub.elsevier.com/retrieve/pii/B9780128239605000561 (Accessed January 13, 2024).
Ojolo, S. P., Cao, S., Priyadarshani, SVGN, Li, W., Yan, M., Aslam, M., et al. (2018). Regulation of plant growth and development: a review from a chromatin remodeling perspective. Front. Plant Sci. 9, 1232. doi:10.3389/fpls.2018.01232
Peters, S., Imani, J., Mahler, V., Foetisch, K., Kaul, S., Paulus, K. E., et al. (2011). Dau c 1.01 and Dau c 1.02-silenced transgenic carrot plants show reduced allergenicity to patients with carrot allergy. Transgenic Res. 20 (3), 547–556. doi:10.1007/s11248-010-9435-0
Prescott, S. L., Pawankar, R., Allen, K. J., Campbell, D. E., Sinn, J. K., Fiocchi, A., et al. (2013). A global survey of changing patterns of food allergy burden in children. World Allergy Organ. J. 6, 21. doi:10.1186/1939-4551-6-21
Quintieri, L., Nitride, C., De Angelis, E., Lamonaca, A., Pilolli, R., Russo, F., et al. (2023). Alternative protein sources and novel foods: benefits, food applications and safety issues. Nutrients 15 (6), 1509. doi:10.3390/nu15061509
Radauer, C., Kleine-Tebbe, J., and Beyer, K. (2017). “Stable plant food allergens II: storage proteins,” in Molecular allergy diagnostics. Editors J. Kleine-Tebbe, and T. Jakob (Cham: Springer International Publishing), 77–90. Available at: http://link.springer.com/10.1007/978-3-319-42499-6_5 (Accessed January 18, 2024).
Risk Assessment of Food Allergens (2022). Part 1: Review and Validation of codex alimentarius priority allergen List through risk assessment (2022) FAO. Available at: http://www.fao.org/documents/card/en/c/cb9070en (Accessed January 16, 2024).
Sathe, S. K., Liu, C., and Zaffran, V. D. (2016). Food allergy. Annu. Rev. Food Sci. Technol. 7 (1), 191–220. doi:10.1146/annurev-food-041715-033308
Scheurer, S., and Sonnewald, S. (2009). Genetic engineering of plant food with reduced allergenicity. Front. Biosci. Volume (14), 59–71. doi:10.2741/3231
Shahali, Y., and Dadar, M. (2018). Plant food allergy: influence of chemicals on plant allergens. Food Chem. Toxicol. 115, 365–374. doi:10.1016/j.fct.2018.03.032
Singh, M. B., and Bhalla, P. L. (2008). Genetic engineering for removing food allergens from plants. Trends Plant Sci. 13 (6), 257–260. doi:10.1016/j.tplants.2008.04.004
Sirvent, S., Cantó, B., Gómez, F., Blanca, N., Cuesta-Herranz, J., Canto, G., et al. (2014). Detailed characterization of Act d 12 and Act d 13 from kiwi seeds: implication in IgE cross-reactivity with peanut and tree nuts. Allergy 69 (11), 1481–1488. doi:10.1111/all.12486
Stitzer, M. C., and Ross- Ibarra, J. (2018). Maize domestication and gene interaction. New Phytol. 220 (2), 395–408. doi:10.1111/nph.15350
Strecker, J., Ladha, A., Gardner, Z., Schmid-Burgk, J. L., Makarova, K. S., Koonin, E. V., et al. (2019). RNA-guided DNA insertion with CRISPR-associated transposases. Science 365 (6448), 48–53. doi:10.1126/science.aax9181
Sugano, S., Hirose, A., Kanazashi, Y., Adachi, K., Hibara, M., Itoh, T., et al. (2020). Simultaneous induction of mutant alleles of two allergenic genes in soybean by using site-directed mutagenesis. BMC Plant Biol. 20 (1), 513. doi:10.1186/s12870-020-02708-6
Takahashi, K., Mizuno, Y., Yumoto, S., Kitamura, K., and Nakamura, S. (1996). Inheritance of the.ALPHA.-subunit deficiency of.BETA.-conglycinin in soybean(Glycine max L. MERRILL) line induced by.GAMMA.-ray irradiation. Jpn. J. Breed. 46 (3), 251–255. doi:10.1270/jsbbs1951.46.251
Tang, X., Hou, A., Babu, M., Nguyen, V., Hurtado, L., Lu, Q., et al. (2008). The arabidopsis BRAHMA chromatin-remodeling ATPase is involved in repression of seed maturation genes in leaves. Plant Physiol. 147 (3), 1143–1157. doi:10.1104/pp.108.121996
Taylor, S. L., Marsh, J. T., Koppelman, S. J., Kabourek, J. L., Johnson, P. E., and Baumert, J. L. (2021). A perspective on pea allergy and pea allergens. Trends Food Sci. Technol. 116, 186–198. doi:10.1016/j.tifs.2021.07.017
Tseng, Y.-C., Mozumdar, S., and Huang, L. (2009). Lipid-based systemic delivery of siRNA. Adv. Drug Deliv. Rev. 61 (9), 721–731. doi:10.1016/j.addr.2009.03.003
Türei, D., Valdeolivas, A., Gul, L., Palacio-Escat, N., Klein, M., Ivanova, O., et al. (2021). Integrated intra- and intercellular signaling knowledge for multicellular omics analysis. Mol. Syst. Biol. 17 (3), e9923. doi:10.15252/msb.20209923
Ulrich, J., Dao, V. A., Majumdar, U., Schmitt-Engel, C., Schwirz, J., Schultheis, D., et al. (2015). Large scale RNAi screen in Tribolium reveals novel target genes for pest control and the proteasome as prime target. BMC Genomics 16 (1), 674. doi:10.1186/s12864-015-1880-y
Wang, J., Yu, Y., Zhao, Y., Zhang, D., and Li, J. (2013). Evaluation and integration of existing methods for computational prediction of allergens. BMC Bioinforma. 14 (S4), S1. doi:10.1186/1471-2105-14-S4-S1
Yanagawa, Y., Suenaga, Y., Iijima, Y., Endo, M., Sanada, N., Katoh, E., et al. (2023). Genome editing by introduction of Cas9/sgRNA into plant cells using temperature-controlled atmospheric pressure plasma. PLOS ONE Ezura H. 18 (2), e0281767. doi:10.1371/journal.pone.0281767
Yıldırım, K., Miladinović, D., Sweet, J., Akin, M., Galović, V., Kavas, M., et al. (2023). Genome editing for healthy crops: traits, tools and impacts. Front. Plant Sci. 14, 1231013. doi:10.3389/fpls.2023.1231013
Zhang, J. (2023). Patterns and evolutionary consequences of pleiotropy. Annu. Rev. Ecol. Evol. Syst. 54 (1), 1–19. doi:10.1146/annurev-ecolsys-022323-083451
Keywords: seed storage proteins, plant allergens, gene editing, artificial intelligence, IgE-mediated reaction
Citation: Evangelista AR, Amoroso CG, Nitride C and Andolfo G (2024) Seed storage allergens tackled via next-generation research assistant. Front. Food. Sci. Technol. 4:1372770. doi: 10.3389/frfst.2024.1372770
Received: 18 January 2024; Accepted: 14 February 2024;
Published: 12 March 2024.
Edited by:
Jiangtao Zhou, ETH Zürich, SwitzerlandReviewed by:
Meleksen Akin, Iğdır Üniversitesi, TürkiyeCopyright © 2024 Evangelista, Amoroso, Nitride and Andolfo. This is an open-access article distributed under the terms of the Creative Commons Attribution License (CC BY). The use, distribution or reproduction in other forums is permitted, provided the original author(s) and the copyright owner(s) are credited and that the original publication in this journal is cited, in accordance with accepted academic practice. No use, distribution or reproduction is permitted which does not comply with these terms.
*Correspondence: Giuseppe Andolfo, giuseppe.andolfo@unina.it
†These authors share first authorship