- School of Kinesiology and Nutrition, The University of Southern Mississippi, Hattiesburg, MS, United States
Background: The association between poor gait and functional movement ability and metabolic syndrome (MetS) has been well established in older adults. A continuous cardiometabolic risk score, MetSindex, may more easily identify individuals at risk for cardiometabolic disease who do not yet meet the stringent criteria for a formal MetS diagnosis. Although the association between MetS and gait velocity is well established in older adults, no such relationship has been identified in younger adults; a group experiencing a rapid increase in the development of MetS.
Methods: MetSindex was determined for 21 young adults using standard procedures. Gait velocity was measured as participants completed a ten-meter walk test. Spatiotemporal parameters of gait were also derived using a motion capture system. Simple linear regression was used to determine the relationship between MetSindex and gait velocity, as well as MetSindex and spatiotemporal parameters of gait.
Results: There was a large inverse relationship between MetSindex and gait velocity. A large inverse relationship was also observed between MetSindex and cadence, and a large positive relationship was observed between stance time and double limb support time.
Conclusions: Gait velocity slows in young adults who do not necessarily meet the criterion for positive diagnosis of MetS—but demonstrate an increased risk for MetS and cardiovascular disease through higher MetSindex scores. The mechanism underlying reduced gait velocity may be fewer, but not shorter steps. Determining easy-to-use surrogates of MetS (e.g., gait velocity) may help combat the growing prevalence of MetS by increasing access to preventative approaches.
1 Introduction
The association between metabolic syndrome (MetS) and poor gait and functional movement ability has been well established in older adults (1–4). Gait velocity is a well-known indicator of functional decline and is often used as a simple and reliable measure of the functional capacity of older adults (5). Some have even suggested that gait velocity be considered “the functional” or “sixth vital sign” and research suggests that it is highly sensitive to change (with advanced age, training status, disease, cognition, etc.) similar to heart rate or blood pressure (6). Reduced gait velocity, for example, has been shown to be an important predictor of metabolic complications, with 0.1 m/s reductions in gait velocity associated with an 8% increase in cardiovascular disease (3, 4, 7). To that end, an increasing body of research has used gait analysis to predict metabolic complications including MetS, type II diabetes, and cardiovascular disease (CVD) (2, 7). A recent meta-analysis and systematic review has even recommended the inclusion of gait velocity as a predictor of CVD and mortality (7).
Currently, MetS is diagnosed based on the presence of at least three of the following cardiometabolic risk factors: (1) excess abdominal adiposity, (2) elevated blood pressure, (3) hyperglycemia, (4) low HDL cholesterol, and (5) elevated serum triglycerides (8). While the current MetS diagnosis employs a dichotomous classification system (i.e., “yes” or “no”), research from our group and others suggests this diagnostic approach does not adequately identify all individuals with an elevated risk of cardiometabolic disease, particularly in young adults (9). For instance, members of our research team recently collected data from 104 young adults, 55 (64.7%) of whom presented with at least one cardiometabolic risk factor and 19 (18.3%) met the criteria for MetS (10).
Recently, a continuous cardiometabolic risk score (MetSindex) has been developed and may be equipped to identify individuals at risk for cardiometabolic disease who do not yet meet the stringent criteria for a formal MetS diagnosis (9). This score accounts for the severity of each of the five MetS risk factors, while also adjusting for race and biological sex. The final outcome of this score is a single value (interpreted as a z-score) that compares an individual's cardiometabolic disease risk to that of the general US adult population, with a more negative score indicating less cardiometabolic disease risk and a positive score indicating increased cardiometabolic disease risk. This continuous MetSindex allows researchers and clinicians to evaluate cardiometabolic disease risk along a continuum, offering the potential to preemptively identify disease risk in individuals not yet meeting the defined criteria for MetS. Therefore, MetSindex is more relevant for populations that might be at risk of developing cardiometabolic disease, such as young adults.
Although the association between MetS and gait velocity is well established in older adults, no such relationship has been identified between gait velocity and MetSindex in younger adults. Understanding this association may be highly valuable in the early detection and prevention of MetS, allowing more time for targeted interventions. Thus, we sought to explore the relationship between gait velocity and MetSindex in younger adults. We hypothesized that a negative relationship would exist between gait velocity and MetSindex scores in younger adults. As such, our primary variables of interest were MetSindex and gait velocity. We also analyzed the spatiotemporal parameters of gait as explanatory variables defining the underlying mechanistic changes in gait velocity we hypothesized.
2 Method
Twenty-one participants were prospectively recruited to attend 2 laboratory sessions. Inclusion criteria included adults between the ages of 18–29, and free from any lower extremity injury, surgery, or disorder that would affect gait (Male: 4; Female: 17; Height:165.5 ± 8.7 cm; Weight: 70.6 ± 18.2 kg; BMI: 22.1 ± 2.0 kg/m2), and all participants provided written informed consent approved by the local Institutional Review Board.
During the first visit, MetSindex was determined using previously established methodology (11, 12). Height was measured using a stadiometer and weight was collected using a calibrated digital scale (SECA, Hamburg, Germany).Waist circumference was collected by a trained investigator at the level of the iliac crest using a flexible aluminum tape measure per the guidelines for evaluating abdominal obesity put forth by the National Cholesterol Education Program (NCEP) Adult Treatment Panel III (ATP-III). Resting blood pressure was measured via an automated sphygmomanometer. Approximately 40 µl of capillary blood (serum) were collected into lithium heparin-lined capillary pipettes to measure fasting blood glucose (%CV: 4.5–6.2), HDL cholesterol (%CV: 3.3–4.9), and triglycerides (%CV: 1.6–3.6) using a capillary blood analyzer (Cholestech LDX; Abbott Laboratories, Chicago, IL). These variables served as the requisite inputs for the MetSindex algorithm (9). MetSindex was calculated from existing sex- and race-specific equations (9) using the five MetS risk described above with the exclusion of diastolic blood pressure. Specifically, MetSindex generates a continuous risk score that represents an estimate of MetS severity as opposed to the traditional binary approach that employs rigid cutoffs for MetS classification. The final estimate produced by the equation is reflective of a z-score, where negative scores indicate decreased MetS severity, and positive scores indicate increased MetS severity. Importantly, these equations have been cross validated in separate samples and have shown to be related to related cardiometabolic abnormalities (9).
During the second visit, participants completed three trials of the ten-meter walk test (10MWT) along a level walkway. Gait velocity was measured during the middle 6 meters of the walkway using 2 photocell timing gates (Blue, Dashr, Lincoln, NE, USA). The mean velocity from all three trials was computed and used for analysis. Spatiotemporal parameters of gait were determined using a standard bilateral lower extremity marker set to record lower extremity kinematics during each 10MWT with a 10-camera motion capture system (240 Hz, Qualisys, Göteborg, Sweden). Spatiotemporal parameters were determined during the stance phase of each step, defined between heel-strike and toe-off of the right leg, specifically when the ground reaction force measured by the force plate exceeded a threshold of 10 Newtons (heel-strike) or fell below a threshold of 10 Newtons (toe-off) (1,200 Hz, American Mechanical Technology Inc. Watertown, MA, USA). All Spatiotemporal parameters of gait were calculated using Visual 3D (version 6.0, C-Motion, Germantown, MD, USA).
The relationship between MetSindex and variables of interest was assessed using simple linear regression techniques. An alpha level of.05 was established a priori and the strength of regression was determined using cutoffs of 0.2 = small, 0.5 = moderate, and 0.8 = strong (13). All statistical analyses were performed using SPSS (version 28, IBM, Chicago, IL).
3 Results
Participant demographic, anthropometric, and metabolic information are presented in Table 1. There was a large, inverse relationship between MetSindex and gait velocity in younger adults (Figure 1, Table 2). Furthermore, a large association was observed between MetSindex and cadence, indicating participants walked with reduced cadence (steps per minute) as MetSindex increased. Stance time and double limb support time demonstrated large positive associations with MetSindex, indicating participants increased contact time during stance as MetSindex increased (Table 2).
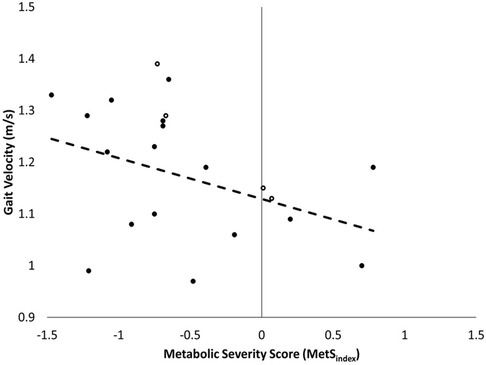
Figure 1. Correlation between metSindex and gait velocity in younger adults (n = 21), with females represented with solid markers and males with outlined markers.
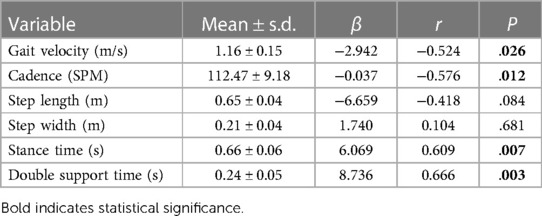
Table 2. Spatiotemporal parameters of gait, beta coefficients (β), correlation coefficients (r), and P-values (P) between each spatiotemporal parameter of gait and metSindex.
4 Discussion
These results indicate a strong, inverse relationship exists between young adults who do not necessarily meet the criterion for positive MetS diagnosis—but demonstrate an increased risk for MetS and CVD through a positive MetSindex score—and self-selected gait velocity (Figure 1). Because MetS is likely under classified in many young adults using the current diagnostic standards, using the MetSindex may aid in the early detection and prevention of modifiable risk factors in young adults, ultimately reducing their long-term risk of developing cardiometabolic diseases and improving their overall longevity. Despite the higher incidence of positive MetS risk factors, primarily driven by a prevalence of obesity, adults are less likely to receive the routine health evaluations necessary for the early detection of MetS (14, 15). Given that obesity is highly associated with the early development of MetS (which may be more effectively determined using MetSindex), discovering easy-to-use surrogates that can leverage this relationship (e.g., gait velocity) may help combat the growing prevalence of MetS by increasing access to preventative approaches.
In this current study, dyslipidaemia, hypertension, and abdominal obesity were the most prevalent metabolic variables—all of which have been associated with reduced gait velocity in older adult populations. Ashan et al. (2023) reported reduced gait velocity in hypertensive older adults compared to normotensive older adults (16), and a recent longitudinal study reported a greater rate of yearly decline in gait velocity with hypertensive patients compared to normotensive patients (17). In addition, the negative effects of obesity have been clearly demonstrated in adults. Hulens et al., for example, reported a significant reduction of 18% in gait velocity during a 6-minute walk test in obese and morbidly obese adult women (18). The results of this current study support the existing body of literature and demonstrate the negative impact of common metabolic dysfunction variables on gait velocity in older age are also manifest in younger adults. As such, monitoring gait velocity beginning earlier in adulthood may serve as an accessible and inexpensive indicator of underlying metabolic dysfunction.
Participants demonstrated reduced gait cadence as MetSindex increased yet demonstrated large positive relationships between stance time and double limb support time MetSindex. This hints that the mechanism underlying reduced gait velocity may be that participants took fewer steps—and not shorter steps—and thus were in contact with the ground for a longer duration during stance. Thus, future interventions may seek to focus on improving gait cadence as a means of increasing gait velocity in young adults with higher MetSindex. Improved gait function by means of improved cadence may address exacerbating conditions that interfere with walking, such as reduced aerobic capacity and perceived discomfort and/or pain, improving participation and compliance with life-style modifications (18).
The results of this investigation should be considered in the context of notable limitations. The participants of this study were predominantly female. In our sample of 21 individuals, gait velocity was 1.16 m/s (females: 1.15 m/s, males: 1.17 m/s). Though the unequal distribution of sex between males and females could bias these results, there is a substantial body of literature which has established normative gait velocity data across different deciles of age (19, 20). Tolea et al., for example, demonstrated that preferred gait velocity between large samples of males (N = 401) and females (N = 595) under the age of 35 was comparable, at 1.2 m/s for both sexes (20). Given the established similarities between males and females in gait velocities of younger adults, we feel any bias due to unequal distribution of males and females is likely of minimal consequence on these results.
It is possible that the strength of the relationship between gait velocity and MetSindex may be moderated by additional anthropometric and functional components that impact both MetSindex and gait velocity but were not evaluated here. Thus, future endeavours may investigate these relationships on a larger sample, and consider additional anthropometric (e.g., weight distribution) and functional tests (e.g., balance, strength) that will provide additional data that can be used in further correlations and regressions to strengthen the relationship between function and MetSindex. Likewise, determining functional limitations may also identify barriers impeding an individual's participation in physical activity interventions intended to minimize cardiometabolic disease risk.
Data availability statement
The raw data supporting the conclusions of this article will be made available by the authors, without undue reservation.
Ethics statement
The studies involving humans were approved by Institutional Review Board, The University of Southern Mississippi. The studies were conducted in accordance with the local legislation and institutional requirements. The participants provided their written informed consent to participate in this study.
Author contributions
TT: Conceptualization, Formal Analysis, Investigation, Methodology, Visualization, Writing – original draft, Writing – review & editing. NO: Writing – original draft, Writing – review & editing. AG: Conceptualization, Formal Analysis, Investigation, Methodology, Visualization, Writing – original draft, Writing – review & editing. JS: Writing – original draft, Writing – review & editing.
Funding
The author(s) declare that no financial support was received for the research, authorship, and/or publication of this article.
Conflict of interest
The authors declare that the research was conducted in the absence of any commercial or financial relationships that could be construed as a potential conflict of interest.
Publisher's note
All claims expressed in this article are solely those of the authors and do not necessarily represent those of their affiliated organizations, or those of the publisher, the editors and the reviewers. Any product that may be evaluated in this article, or claim that may be made by its manufacturer, is not guaranteed or endorsed by the publisher.
References
1. Botoseneanu A, Chen H, Ambrosius WT, Allore HG, Anton S, Folta SC, et al. Metabolic syndrome and the benefit of a physical activity intervention on lower-extremity function: results from a randomized clinical trial. Exp Gerontol. (2021) 150:111343. doi: 10.1016/j.exger.2021.111343
2. Efthymiou D, Zekakos DX, Papatriantafyllou E, Ziagkas E, Petrelis AN, Vassilopoulou E. Gait alterations in the prediction of metabolic syndrome in patients with schizophrenia: a pilot study with PODOSmart (R) insoles. Front Psychiatry. (2022) 13:756600. doi: 10.3389/fpsyt.2022.756600
3. Okoro CA, Zhong Y, Ford ES, Balluz LS, Strine TW, Mokdad AH. Association between the metabolic syndrome and its components and gait speed among U.S. adults aged 50 years and older: a cross-sectional analysis. BMC Public Health. (2006) 6(1):282. doi: 10.1186/1471-2458-6-282
4. Slater L, Gilbertson NM, Hyngstrom AS. Improving gait efficiency to increase movement and physical activity - the impact of abnormal gait patterns and strategies to correct. Prog Cardiovasc Dis. (2021) 64:83–7. doi: 10.1016/j.pcad.2020.12.003
5. Studenski S, Perera S, Patel K, Rosano C, Faulkner K, Inzitari M, et al. Gait speed and survival in older adults. Jama. (2011) 305(1):50–8. doi: 10.1001/jama.2010.1923
6. Middleton A, Fritz SL, Lusardi M. Walking speed: the functional vital sign. J Aging Phys Act. (2015) 23(2):314–22. doi: 10.1123/japa.2013-0236
7. Veronese N, Stubbs B, Volpato S, Zuliani G, Maggi S, Cesari M, et al. Association between gait speed with mortality, cardiovascular disease and cancer: a systematic review and meta-analysis of prospective cohort studies. J Am Med Dir Assoc. (2018) 19(11):981–988.e7. doi: 10.1016/j.jamda.2018.06.007
8. Grundy SM, Cleeman JI, Daniels SR, Donato KA, Eckel RH, Franklin BA, et al. Diagnosis and management of the metabolic syndrome: an American heart association/national heart, lung, and blood institute scientific statement. Circulation. (2005) 112(17):2735–52. doi: 10.1161/CIRCULATIONAHA.105.169404
9. Gurka MJ, Lilly CL, Oliver MN, DeBoer MD. An examination of sex and racial/ethnic differences in the metabolic syndrome among adults: a confirmatory factor analysis and a resulting continuous severity score. Metab Clin Exp. (2014) 63(2):218–25. doi: 10.1016/j.metabol.2013.10.006
10. Graybeal AJ, Brandner CF, Henderson A, Aultman RA, Vallecillo-Bustos A, Ta'Quoris AN, et al. Associations between eating behaviors and metabolic syndrome severity in young adults. Eat Behav. (2023) 51:101821. doi: 10.1016/j.eatbeh.2023.101821
11. Stavres J, Aultman RA, Brandner CF, Newsome TQA, Vallecillo-Bustos A, Wise HL, et al. Hemodynamic responses to handgrip and metaboreflex activation are exaggerated in individuals with metabolic syndrome independent of resting blood pressure, waist circumference, and fasting blood glucose. Front Physiol. (2023) 14:1212775. doi: 10.3389/fphys.2023.1212775
12. Graybeal AJ, Brandner CF, Tinsley GM. Validity and reliability of a mobile digital imaging analysis trained by a four-compartment model. J Hum Nutr Diet. (2023) 36(3):905–11. doi: 10.1111/jhn.13113
13. Zou KH, Tuncali K, Silverman SG. Correlation and simple linear regression. Radiology. (2003) 227(3):617–28. doi: 10.1148/radiol.2273011499
14. Smith J, Lensing S, Horton JA, Lovejoy J, Zaghloul S, Forrester I, et al. Prevalence of self-reported nutrition-related health problems in the lower Mississippi Delta. Am J Public Health. (1999) 89(9):1418–21. doi: 10.2105/AJPH.89.9.1418
15. Clark CR, Chandler PD, Zhou G, Noel N, Achilike C, Mendez L, et al. Peer reviewed: geographic variation in obesity at the state level in the all of US research program. Prev Chronic Dis. (2021) 18:1–11. doi: 10.5888/pcd18.210094
16. Ahsan M, Turki A, Mohammed AS, Wafa AM, Maitha A, Shibili N, Abdullah A. Determining the characteristics of gait variability with a preferred walking speed in hypertensive and normotensive participants. Clin Epidemiol Glob Health. (2023) 23:101344. doi: 10.1016/j.cegh.2023.101344
17. Dumurgier J, Alexis E, Carole D, Béatrice T, Christophe T. Hypertension and lower walking speed in the elderly: the three-city study. J Hypertens. (2010) 28(7):1506–14. doi: 10.1097/HJH.0b013e328338bbec
18. Hulens M, Vansant G, Claessens AL, Roeland L, Erik M. Predictors of 6-minute walk test results in lean, obese and morbidly obese women. Scand J Med Sci Sports. (2003) 13(2):98–105. doi: 10.1034/j.1600-0838.2003.10273.x
19. Bohannon RW, Andrews AW. Normal walking speed: a descriptive meta-analysis. Physiotherapy. (2011) 97(3):182–9. doi: 10.1016/j.physio.2010.12.004
Keywords: walking, walking speed, gait analysis, metabolic syndrome, young adults
Citation: Thorsen T, Oliveira N, Graybeal A and Stavres J (2024) Exploring gait velocity as a predictor of cardiometabolic disease risk in young adults. Front. Sports Act. Living 6:1365717. doi: 10.3389/fspor.2024.1365717
Received: 4 January 2024; Accepted: 13 February 2024;
Published: 7 March 2024.
Edited by:
Carlos A. Celis-Morales, University of Glasgow, United KingdomReviewed by:
Jaime Vásquez-Gómez, Universidad Católica de Maule, ChileJared M. Gollie, Washington DC VA Medical Center, United States
© 2024 Thorsen, Oliveira, Graybeal and Stavres. This is an open-access article distributed under the terms of the Creative Commons Attribution License (CC BY). The use, distribution or reproduction in other forums is permitted, provided the original author(s) and the copyright owner(s) are credited and that the original publication in this journal is cited, in accordance with accepted academic practice. No use, distribution or reproduction is permitted which does not comply with these terms.
*Correspondence: Tanner Thorsen dGFubmVyLnRob3JzZW5AdXNtLmVkdQ==