- 1Symbiosis Institute of Technology, Symbiosis International Deemed University, Pune, India
- 2Department of Instrumentation Engineering, D Y Patil Institute of Technology, Savitribai Phule Pune University, Pune, India
- 3Department of Biosciences, Saveetha School of Engineering, Saveetha Institute of Medical and Technical Sciences, Gazi University Faculty of Engineering, Department of Mechanical Engineering, Maltepe, Türkiye
- 4Department of Machining, Assembly and Engineering Metrology, Faculty of Mechanical Engineering, VSB-Technical University of Ostrava, Ostrava, Czechia
- 5Department of Industrial Engineering, College of Engineering, King Saud University, Riyadh, Saudi Arabia
The preparation and tribological behavior of the titanium metal matrix (Ti-6Al-4V) composite reinforced with tungsten carbide (WCp) and graphite (Grp) particles were investigated in this study. The stir casting procedure was used to fabricate the titanium metal matrix composites (TMMCs), which had 8 weight percent of WCp and Grp. The tribological studies were designed using Taguchi’s L27 orthogonal array technique and were carried out as wear tests using a pin-on-disc device. According to Taguchi’s analysis and ANOVA, the most significant factors that affect wear rate are load and distance, followed by velocity. The wear process was ascertained by scanning electron microscopy investigation of the worn surfaces of the composite specimens. Pearson’s heatmap and Feature importance (F-test) were plotted for data analysis to study the significance of input parameters on wear. Machine learning classification algorithms such as k-nearest neighbors, support vector machine, and XGBoost algorithms accurately classified the wear rate data, giving an accuracy value of 71.25%, 65%, and 56.25%, respectively.
1 Introduction
Titanium alloys are widely used in many technical applications due to their excellent combination of high hardness, wear resistance, strength, corrosion resistance, stiffness, and low density (Jiao et al., 2018; Suresh et al., 2018; Cao and Liang, 2020). Titanium alloys are widely utilized for their exceptional strength-to-weight ratio to lower energy consumption, increase productivity, and extend product life inthe automotive, aerospace, sports, transportation, and medical equipment industries (Attar et al., 2018; Chao et al., 2019; Perundyurai Thangavel et al., 2020). Titanium metal matrix composites (TMMCs) can be broadly classified into two types based on the shape and distribution of reinforcements: continuously reinforced TMMCs and discontinuously reinforced TMMCs (Guo et al., 2012; Jiao et al., 2018; Hayat et al., 2019). WC, Al2O3, TiB, CNTs, SiC, FE3O4, B4C, TiC, Gr, and other ceramic particles and whiskers commonly reinforce TMMCs (Li et al., 2015; Zhang et al., 2016; Sun et al., 2020).
Frary et al. (Frary et al., 2003) reported that the mechanical and physical properties of pure titanium reinforced with 10 weight percent WP were comparable to those of the Ti-6Al-4V (Ti64) alloy. A study by Choe et al. (Choe et al., 2005a; Choe et al., 2005b) found that the size of the WP significantly influences the mechanical characteristics of WP/Ti composites. A range of microstructures with good tensile strength and elongation were produced as a consequence of Wang et al.’s investigations of the varied size distribution of TiC reinforcement to titanium by DED (Wang et al., 2018). Using Ti/B4C composite powder feedstocks, Xia et al. produced TiB + TiC reinforced titanium in situ. They also thoroughly examined the development of the in situ microstructure and the interaction zone between the titanium and ceramic reinforcement (Xia et al., 2017).
Furthermore, in situ B4C/BN reinforced Ti6Al4V composites were studied by Gupta et al. The main issue was wear performance, and strengthening reduced the sliding coefficient of friction (COF) by half compared to the Ti6Al4V matrix (Gupta et al., 2018). Using an in-situ approach, Choi et al. produced hybrid composites of Ti6Al4V alloy with reinforcements TiB and TiC that had a constant reinforcement allocation. The results showed a significant relationship between reinforcement content and more excellent TMC wear resistance, with more reinforcement content resulting in lower wear loss (Kim et al., 2011). After electroless plating to manufacture copper-coated CNTs, Wang et al. (2017) used spark plasma sintering procedures to create copper matrix composites. The results showed that electroless plating significantly improved the mechanical properties by enhancing the element link between copper and carbon nanotubes and enabling uniform dispersion of CNTs.
By using electroless nickel plating and SPS to create composites of graphite flakes and copper, Ren et al. dramatically increased the bonding at the graphite/copper base contact. According to the findings, the bending characteristics and coefficient of thermal expansion were significantly improved by installing the NieP transition layer (Chen et al., 2017). When 0.35wt% of multi-walled carbon nanotubes (MWCNTs) was added, Kondoh et al. discovered that the tensile parameters, such as strength and yield, increased by up to 27% and 42%, respectively, in contrast to those of pure titanium (Kondoh et al., 2008). Wang et al. used an 823 K sintering temperature in conjunction with a spark plasma sintering technique to create a TMC composite. The findings demonstrated that when the volume fraction of MWCNTs reached 0.4 weight percent, the material’s compressive strength and yield strength both attained their maximum values. Then, when the MWCNT content was raised even further, the compressive strength dropped (Wang et al., 2015). Jin et al. studied the production of pure titanium powder with TiB2 reinforcing particles using a selective laser melting method. All sorts of wear characteristics, including adhesion, abrasion, and oxidation, were improved due to the Ti/TiB2 composites (Jin et al., 2021). The titanium and ZrO2 nanoparticle composites that Abd-Elwahed et al. produced were made via powder metallurgy. The results demonstrated that raising the usual load improved the bonding, wear, and friction properties, increased the total amount of ZrO2 nanoparticles, and enhanced the sliding wear rate (Abd-Elwahed et al., 2020). An et al. assessed the tribological behaviors of TMC coatings at high temperatures. The findings demonstrate that delamination, plowing, and oxidation wear processes occur at 500 C together with increased wear rates for TMC coatings (An Qi et al., 2019).
Regarding hardness and wear resistance at room temperature, An et al.’s tests (An et al., 2018; An Q. et al., 2019) show that the hybrid TiBand TiC particles boosted with titanium coating and inter-growth ceramic structures outperform the TiB/Ti64 coating. Farias concentrated on how the tribological characteristics of the TiC reinforcing particles with open porosity in TMCs were affected by spark plasma sintering. The study’s findings revealed that adding TiC particles enhanced tribological characteristics like wear resistance, coefficient of friction, and nano hardness (Farías et al., 2019). Insufficient bonding between the reinforcement particles and matrix might cause ceramic particles to function as an abrasive material, as per the findings of Ram Prabh et al. The dimensions, mass, and morphology of the alternative phase reinforced particles, the equivalent material, the load, the microstructure, the environment, and the humidity can all impact the wear resistance of composite materials (Ram et al., 2014). Room temperature research was done by Huang Xie, who also carried out sliding wear trials on TMCs with mild steel grade 35. Iron oxide additives, independent of load, rarely improve the TMC’s wear performance because they require lubricating capabilities. The MLG/Fe2O3 nanocomposite and MLG with Fe2O3 mechanical combination may significantly improve the wear performance (Xie et al., 2021).
Zhou et al. examined the effect of graphene/Fe2O3 nanocomposites on the tribological performance of the TC11 alloy. They found that on the injured surface, a thin, stable double tribolayer consisting of layers primarily composed of MLG and Fe2O3 was created, significantly reducing wear and friction. This research shows that adding specific nanoparticles to materials can improve their tribological properties (Zhou et al., 2017). Titanium hybrid composites with single and multiple reinforcements were created by Lixia Xi et al. and fabricated using SLM. The hybrid composite result set between the reinforcements and matrix generated the interfacial structure. The wear resistance and CoF characteristics are enhanced when TiC and TiN reinforcement particles are introduced (Xi et al., 2021).
Prakash considered removing this property lag. Titanium alloy (Ti-6Al-4V) is used to fortify boron carbide (B4C) ceramic particles using powder metallurgy (PM). As a result of this research, a newer composite was created and tested, improving hardness, corrosion resistance, and reduced density. The wear performance of the composite specimens is more affected by the applied loads than by the amount of B4C added. Scanning electron microscopy results demonstrate that the B4C-reinforced Ti-6Al-4V composite has better wear resistance than the unreinforced Ti alloy and shows signs of mildly worn surfaces (Soorya Prakash et al., 2016). Hu investigated and analyzed the quality of the components, the processes by which microstructures form, and the efficacy of workpiece wear in TiB-reinforced Ti matrix composites, which were produced using the LENS approach. The results suggest that TiB-TMCs, with their innovative microstructures and TiB reinforcement, exhibited superior wear performance compared to bulk components composed of commercially pure titanium. Furthermore, by varying the laser power, the characteristics of the produced components were improved with fewer internal defects, leading to better wear performance (Hu et al., 2017). Using titanium alloy reinforcement to improve the grapheme’s ultimate compressive strength, tensile strength, wear resistance, thermal conductivity, and diffusivity, Gurbuz et al. investigated the properties of tribological, mechanical, and thermal aspects (Gürbüz et al., 2021).
Measuring the particular wear rate is crucial to the material selection and optimization process. Engineers can choose the best materials for a given application by comparing the wear rates of several magnesium or magnesium alloys with other materials. This is especially crucial in sectors like manufacturing, aerospace, and automotive, where wear resistance significantly impacts the longevity and dependability of components. Artificial Intelligence (AI) has yielded many benefits and made significant strides in several industries, including manufacturing and healthcare. Artificial intelligence (AI) has shown to be extremely useful in the medical industry for tasks including disease diagnosis, therapy planning, medication development, and patient monitoring (Mathews, 2019; Buccino et al., 2023a; Buccino et al., 2023b; Yang et al., 2023). A type of artificial intelligence called machine learning algorithms has been widely used to evaluate complex medical data, spot trends, and generate precise forecasts. As a result, there has been an improvement in the precision of diagnoses, the creation of customized treatment programs, and the general quality of patient care.
Similarly, AI has considerably changed operations in the manufacturing sector by facilitating predictive maintenance, increasing productivity, and streamlining procedures (Maleki et al., 2022; Maleki et al., 2023). Demand forecasting, supply chain optimization, automation, and quality control have all benefited from using machine learning algorithms. These applications have improved operational effectiveness, lowered production costs, and improved product quality. The integration of Evolutionary Computing with Machine Learning algorithms has received very little research attention despite the remarkable advancements in AI and Machine Learning. Evolutionary computing is a subfield of artificial intelligence that uses methods from natural evolution, including genetic algorithms, particle swarm optimization, and ant colony optimization, to tackle challenging optimization issues. Combining machine-learning techniques and evolutionary computing has excellent promise in several fields. Researchers can more effectively handle complex optimization and prediction tasks by combining the adaptive search capabilities of Evolutionary Computing with the learning and predictive powers of Machine Learning algorithms. This integration can be further implemented in various material science and manufacturing domains (Dhungana et al., 2019; Dhungana et al., 2021; Sadek et al., 2021; Dhungana et al., 2022; Mishra and Jatti, 2023a; Dhungana et al., 2023; Greco et al., 2023). This hybrid technique can help find the best solutions in complex problem spaces, increase the precision and effectiveness of optimization algorithms, and improve feature selection in machine-learning models.
Estimating the precise wear rate of Hybrid Metal Matrix Titanium alloy is essential in sectors where wear resistance is a vital component. While machine learning has demonstrated potential in predictive modeling, evaluating their performance and choosing which algorithm works best for this particular use case is necessary. Furthermore, there needs to be a more thorough analysis and comparison of various algorithms and a paucity of research in this area. Thus, comparing the effectiveness of machine learning algorithms, namely K-Nearest Neighbouring (KNN), Support Vector Machine (SVM), and XGBoost classification in forecasting the precise wear rate of the Hybrid Metal Matrix Titanium alloy is the topic this research study attempts to address.
This study used the stir casting method to create a titanium alloy, Ti-6Al-4V, with reinforcements made of tungsten carbide (WC) and graphite (Gr) hybrid metal matrix composite. Process variables like load, sliding velocity, sliding distance, and tribological experiments were performed based on the Taguchi L27 orthogonal array.
2 Materials and methods
The primary matrix material used was the titanium alloy Ti-6Al-4V; Table 1 shows the chemical composition of the matrix values for this alloy. The particles reinforced with WC and Gr have been selected. The average size of the graphite particles was 25 μm, whereas the WC particles were 45 μm. The required amount of Ti-6Al-4V titanium alloy was melted in a graphite crucible using an electrical furnace. The reinforcing particles were heated to 500°C to remove the moisture. A specific quantity of reinforcing particles was mixed with the titanium alloy. The hybrid composite material was regularly blended. After the hybrid composite was inserted into the prepared die at 800°C, it was left to solidify at room temperature.
The sample’s microstructures and worn surfaces were examined using optical and scanning electron microscopy. Microhardness tester of model-Microhardness tester from OMNI tech, MVH-1 automatic test load 10 gm to 1,000 gm employed for microhardness measurement. As indicated in Figure 1, the composite specimens’ dry sliding wear qualities were evaluated using the DUCOM pin-on-disc sliding wear testing apparatus (Manufacturer: DUCOM, Bangalore, India). The ASTM G99-95 rules were followed when conducting the dry sliding wear testing. The pin was cleaned with acetone, and its initial mass was measured with a digital electronic balance. After that, the pin was held up against a revolving EN-32 steel disk (counter face) with 65 HRC hardness throughout the test. Figure 2A,B depicts the casted and machined samples, respectively.
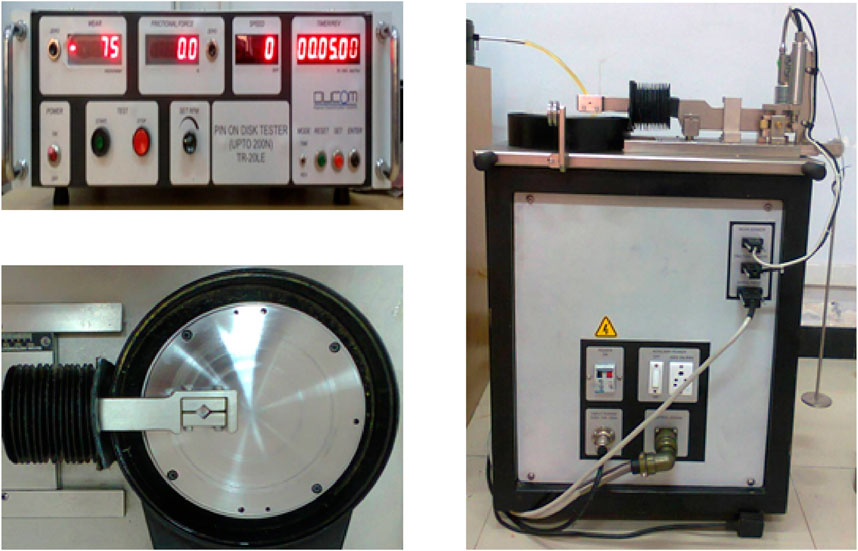
Figure 1. Wear test on disc (Mishra and Jatti, 2023b; Mishra et al., 2023)
Throughout the testing, adjustments were made to the distance, velocity, and average load. At the end of each test, the pin’s ultimate mass was measured after being cleaned with acetone. We calculated the mass loss of the pin due to sliding wear by taking the difference between its initial and final masses. The volume loss owing to wear was calculated using the density values linked with the pin. Next, the wear rate of the composite pins was ascertained.
The following is the process for carrying out the wear test:
First, the test sample is carefully weighed on a state-of-the-art digital balance, and its original mass is recorded. The specimen is then securely secured using the notch, and its surface is positioned so that it makes contact with the disk. The track radius is then modified to meet the unique requirements of the test. Following proper specimen positioning specified normal loads are supplied, and the sliding velocity is set in compliance with the test parameters. The test is then run to cover the specified distance over a computed time interval. For every test, the pin volume loss was determined using the pin-height loss method. Each test was conducted three times to guarantee repeatability, and the average of the three tests was used to determine the wear rate using Eq. (1). This process is repeated for other specimens with varying volume percentages and could be tested under various conditions. This method makes it possible to compare wear characteristics under various circumstances.
The wear parameters chosen for the testing based on machine capacity, literature analysis, and pilot trials are shown in Table 2. Pilot experiments were conducted to ascertain the practical limits of the previously indicated parameters necessary for the wear to occur in a steady state. Referring to ASTM G99-95, the pin used in the wear test is 30 mm long and has an 8 mm diameter.
The Taguchi technique aims to minimize variation in a process through robust experiment design. The main objective of the procedure is to provide the maker with high-quality output at a low cost. Dr. Genichi Taguchi of Japan developed the Taguchi method and has persisted in using that variation. Thus, both the producer and society are impacted by low process quality. He developed a system for designing experiments to investigate how different parameters affect the mean and variance of a process performance characteristic that shows how well the process is doing.
Taguchi’s experimental design gathers the necessary data to identify the variables significantly influencing product quality with minor experimentation, saving time and resources. This is accomplished using orthogonal arrays to organize the variables influencing the procedure and the magnitudes at which they should be shifted. Key process factors were identified using analysis of variance. An L27 orthogonal array was chosen for the current experiment, as indicated in Table 3.
This study classifies and predicts the wear rate of hybrid metal matrix composite using KNN, SVM, and XG Boost machine learning classification techniques. To build a confusion matrix and AUC-ROC curves for further in-depth analysis and to precisely assess the wear rate using classification, sample data was constructed using a synthetic data generation tool in MATLAB based on the experimental data. The classification method is a supervised learning technique that categorizes new observations using training data. Using the dataset or supplied observations, a program learns how to categorize fresh observations into various classes or groups in the classification process. The data in the current study is divided into two groups according to whether the value exceeds or falls short of the 0.084 average of all wear rate values. The confusion matrix was plotted using the Python metrics module from the sklearn package. In order to achieve precise outcomes for data prediction, the dataset was split into two sections: 80 percent of training data and 20 percent of random test data. The confusion matrix is composed of three types of data: False positives (FP) and False negatives (FN), which reflect wrongly forecasted data or suggest that there was an error in the prediction process; and True positives (TP) and True negatives (TN), which represent successfully anticipated data. K-fold (k = 1) was considered for the number of neighbors (k), and KNN, SVM, and XGBoost classification was performed to obtain a wide range of classification data. Pearson’s heatmap analysis and feature importance plot (F-test) were plotted further to understand the significance of features on wear loss. A flowchart explaining heatmap analysis is depicted in Figure 3.
The current study uses a k-nearest neighbor (kNN) classification method, which finds the closest Euclidean distance between the average value and each wear rate value (refer to Figure 4A). For the kNN classification method, there are various types of hyperparameter options, such as:
a) The number of neighbors decides the number of nearest neighbors to classify each value or point in the target dataset.
b) Distance metric, which is used to measure distance between 2 points.
c) Distance weight decides whether the distance is equal or inverse (1/distance).
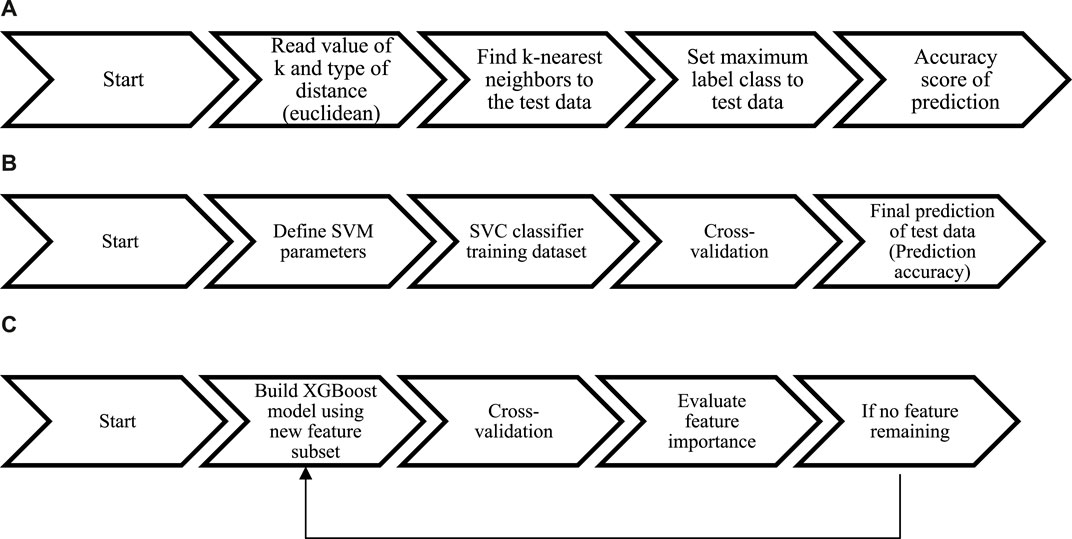
Figure 4. (A) kNN classification flowchart. (B) SVM classification flowchart. (C) XG-Boost classification flowchart.
SVMs were created to solve binary classification issues. However, when computationally demanding multiclass problems become more common, several binary classifiers are built and coupled to create SVMs that can carry out these multiclass classifications using binary methods. The SVM classifier function (SVC) is defined using input parameters like the type of kernel used, the margin, and the hyperplane. Once the data is trained using the SVM classifier function, the test data is predicted based on the trained and validated data. The workflow of the SVM classification algorithm is depicted in Figure 4B. XGBoost is a scalable and accurate gradient-boosting solution that pushes the computational boundaries of boosted tree algorithms, primarily accelerating computational speed and machine learning model performance. As part of an ensemble approach meant to produce superior predictions with imbalanced-class data, Extreme Gradient Boosting, or XGBoost, has become increasingly popular as a prediction algorithm in recent years. XGBoost classification is similar to the F-test as it selects the best features to predict the data after it trains and validates the training dataset. It continues until the best feature, which has the most significance on the data, is selected, and no features remain in the dataset to evaluate. The workflow of the XGBoost classification algorithm is depicted in Figure 4C. The values are recorded after the dataset is collected using wear loss experiments. Heatmap analysis and F-test are done to identify the most significant features of the dataset. Then, supervised machine learning algorithms, in this case, classification algorithms like kNN, SVM, and XGBoost, are used to classify the data based on the training and validation dataset, and the data is predicted using the trained model. The results are then analyzed for the best wear loss rate based on the best features.
3 Results and discussions
In order to estimate the precise wear rate of hybrid composites, this section presents the wear rate results together with statistical analysis and a machine learning technique. The experimental parameters used for the studies are shown in Table 3, together with the corresponding observed wear rate values.
3.1 Statistical analysis
The dry sliding wear test was performed using pin-on-disc equipment. The analysis of variance and signal-to-noise (S/N) ratio techniques were used to determine the significant parameters. The S/N ratios are used to evaluate how noise factors affect performance metrics. Three S/N ratios are typical and frequently utilized; they evaluate the degree of variation in the answer data and the degree to which the average response resembles the target. Theoretically, higher, smaller, and better are the better of them. Smaller is better. The guideline was applied in this study to reduce wear rate. The signal-to-noise ratio, or S/N ratio, gauges the susceptibility of the quality attribute under study to experimentally induced uncontrollable events.
An analysis of variance (ANOVA) was performed to investigate the effects of load, velocity, and distance on the wear rate; the results are shown in Table 4. The load and the distance substantially impacted the wear rate, according to the F-test and p-value results. Table 5 and Table 6 depict the response table for signal-to-noise ratios and means, respectively. As per the results, the load followed by distance and velocity affects the wear rate.
Figure 5A displays the plot of the wear rate’s primary influence. It is evident that the wear rate tends to increase with higher velocities and normal loads. Furthermore, Figure 5B illustrates the wear rate interaction plot. The lines intersect to reveal a strong interaction effect between the wear rate and the load and velocity, load and distance, and velocity and distance.
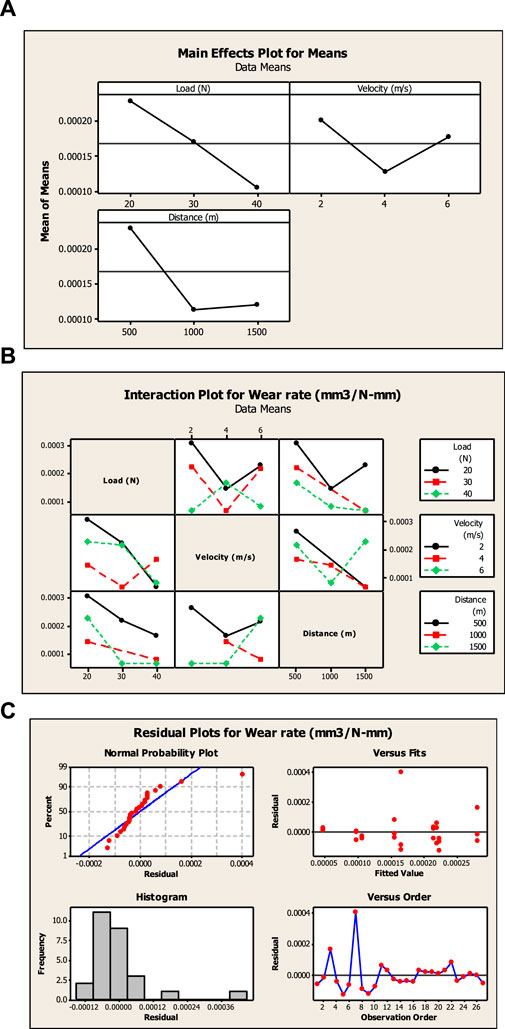
Figure 5. (A) Main effects plot for wear rate. (B) Interaction plot for wear rate. (C) Residual plots for wear rate.
Figure 5C displays a residual plot of the particular wear rate. A standard probability plot denotes a normal distribution for the residuals. There was no evidence of data skewness or outliers in the histogram plot. There were no apparent patterns in the residual versus ordered plot or the residual versus fitted plot. These findings imply that differences in time or environmental conditions did not cause any inaccuracies in the data gathering.
The wear rate indicates the amount of material loss or wear volume per unit of sliding distance and unit load. Several wear regimes were found in the wear map by analyzing the wear rate values. At different combinations of sliding velocities and normal loads, these wear regimes offer insights into the predominant wear mechanisms, including adhesive wear, abrasive wear, delamination, plastic deformation, oxidation, and melting. Changes in wear rate over relatively modest differences in parameters like average load, sliding velocity, temperature, and time are referred to as wear transitions. Wear transition charts usually identify and characterize various wear regimes or mechanisms. Low wear rates and mild contact conditions with a mix of adhesive and oxidative wear predominate are characteristics of the mild wear regime. On the other hand, the severe wear regime is characterized by elevated wear rates and usually arises from more rigorous working circumstances. Numerous mechanisms, including abrasive wear, plowing, and delamination, can contribute to severe wear.
Plowing removes material due to the interaction of microscopic asperities on one surface with another, which causes abrasive wear. Rough surfaces or the presence of hard particles are linked to this mechanism. Oxidative wear is caused by the interaction of the alloy with ambient oxygen, producing oxide layers on the alloy surface. Increased wear rate and surface deterioration may result from this mechanism. The term “delamination” describes how layers come away from the surface of a substance. It frequently happens in areas with a concentration of localized tension, which causes wear debris and surface roughness to accumulate. This phenomenon is known as plastic deformation, when a material deforms and flows due to an applied average load. A two-dimensional graph of the wear rate values is displayed in Figures 6A–C. The color represented the wear rate values. This image made the analysis of wear rate variations under various test settings possible. These wear maps help determine prevailing wear regimes, comprehend the wear mechanisms, and choose the best materials and operating settings for specific applications. This investigation found that the wear rate decreased at lower velocity and load values and increased at higher velocity and load values. The wear map’s varied colored zones correspond to distinct wear rates at varying velocities and loads. The transition lines were used to define the zones by the experimental setup.
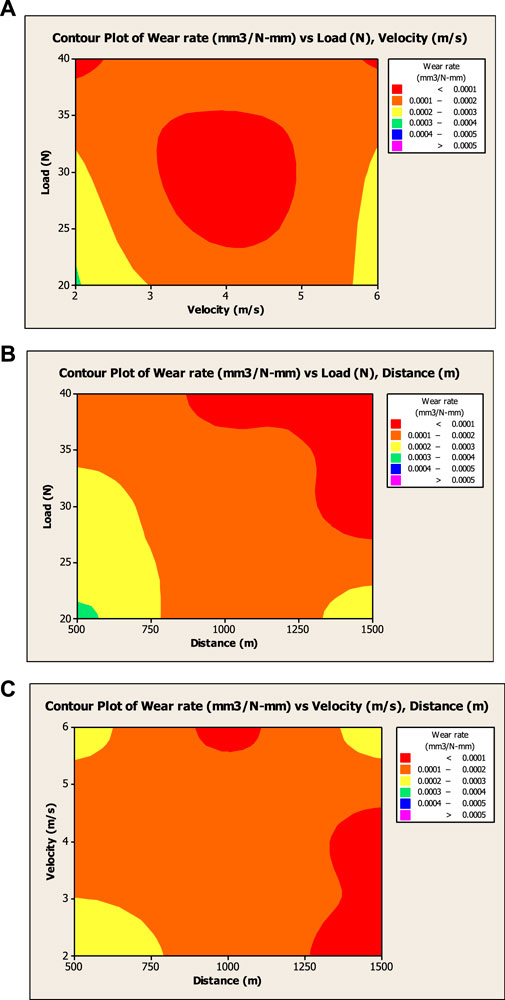
Figure 6. (A) Contour plot of wear rate versus load, velocity. (B) Contour plot of wear rate versus load, distance. (C) Contour plot of wear rate versus velocity, distance.
The ideal velocity and load combination that reduces wear rate can be found through wear map analysis, making it possible to identify operating situations where the material exhibits excellent wear performance. This knowledge is a reference for technical applications using the hybrid metal matrix composite alloy. This is especially important in the automotive, aerospace, and biomedical industries, where wear resistance is critical. For the present study, the wear rate is lower between 3.5 and 4.5 m/s velocity and 25 N–35 N load; see Figures 6A,B shows that the wear rate is lower between 27.5 N and 40 N load and 1,000 m–1,500 m distance. Figure 6C shows that the wear rate is lower between 2 and 4.5 m/s velocity and 1,250 m–1,500 m distance.
3.2 Optical and SEM analysis
The optical micrograph of the composite is shown in Figure 7A. The sample has excellent compactness and is free of micro-fissures and pores. The WCp-Grp reinforcement was evenly distributed throughout the composites to permit its presence in the Ti-6Al-4V matrix. The Ti-6Al-4V matrix has uniformly distributed tiny WCp-Grp reinforcements, even though Titanium Composites Interaction does not observe any reactions. It is important to note that WCp and Grp do not combine to form a straightforward binary combination but produce a new interface structure. The average value of Vickers Microhardness for the hybrid composite obtained using a microhardness tester is 892 VHN.
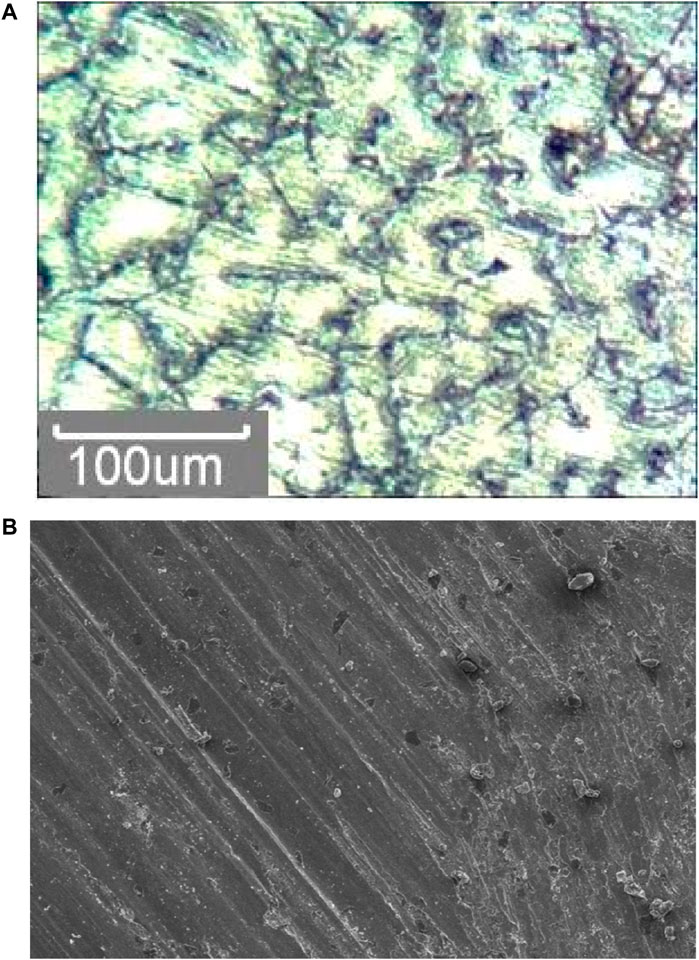
Figure 7. (A) Optical micrograph for hybrid composite. (B) Wear track on pin surface for experiment no.7.
SEM analysis of the wear surfaces developed into dry sliding wear in the steady state regime provides a crucial tool for accurately characterizing the wear behavior of the composites. Figure 7B shows the composites’ worn-out surface following wear. The extraordinary hardness of the composite means that the worn-out surfaces are barely perceptible. This example has a very smooth surface because the WCp and GRp reinforcement particles are securely bonded to the matrix phase at that level. It is also clear that reinforcements have not worn down only a little. The self-lubricating action of the tribo surface reinforcements causes this. The worn surface of the composite makes the presence of laminated layers quite evident. In this picture, the layer has changed the easily observable sliding surface. The surfaces also appear smooth because of the reinforcing component.
3.3 Wear rate prediction using machine learning classifier
Data was categorized using machine learning based on the taper angle of the square slots in the stainless steel plate. Using Python libraries, Pearson’s heatmap and F-test plots were created to determine the feature importance of input parameters, including load, velocity, and distance. Pearson’s heatmap analysis is plotted as shown in Figure 8A, which depicts the minimal significance of load and velocity on the wear rate.
As shown in Figure 8B, a feature is deemed insignificant if its F-test value is below the F-distribution value. However, any F-test value of an input parameter over the critical F-distribution will be regarded as a significant feature or input parameter. The k-nearest Neighbors (kNN) method predicts the label or value of a new data point by considering the labels or values of its k-nearest neighbors in the training dataset. In the current analysis of wear loss, the values below the average value of wear loss were considered as 1, and the values above the average value were considered as 0, refer to Figure 9A. Wear loss should be minimal as it will give better results. The prediction accuracy of the kNN classification of wear loss was 71.25%, refer to Table 7.
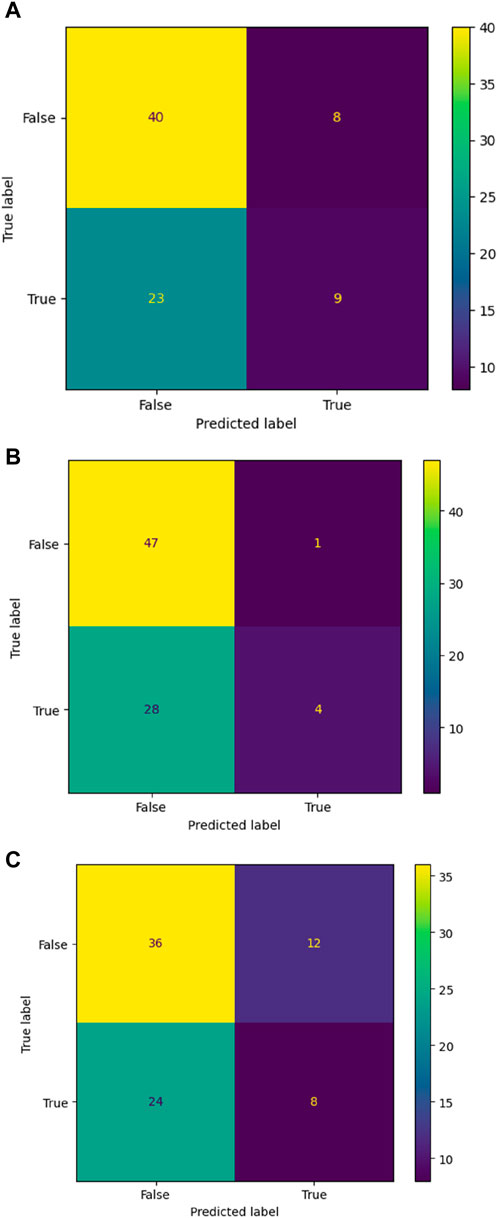
Figure 9. (A) Confusion matrix for kNN classifier. (B) Confusion matrix for SVM classifier. (C) Confusion matrix for XGBoost classifier.
Support vector machine classification or support vector classification segregates or separates the data by a 2-D hyperplane across the data points. The data used in SVM classification may be either linear or non-linear. An SVM classifier has various kernels that can be configured. We can designate the kernel as “linear” for a linear dataset. It classifies the values above the average wear loss (0) and those below the average wear loss (1); refer to Figure 9B. The prediction accuracy of SVM was found to be 65%; refer to Table 8.
A distributed gradient boosting library optimized for maximum efficiency, versatility, and portability is called XGBoost. It uses the Gradient Boosting framework to implement machine learning algorithms. Figure 9C depicts the confusion matrix for XGBoost. The prediction accuracy of the XGBoost classifier was found to be 56.25%, refer to Table 5c.
4 Conclusion
Using the stir casting method, a novel hybrid composite Ti-6Al-4V/WCp/Grp was created, and its tribological behavior was examined. The following are the key findings of the study:
i. The load and the distance significantly impacted the wear rate, with velocity following suit, as indicated by the F-test, p-value findings, and ANOVA.
ii. Based on the wear maps, the wear rate for the current investigation is lower between 3.5 and 4.5 m/s velocity and 25 N–35 N load.
iii. The composite’s optical micrograph demonstrates the sample’s outstanding compactness and lack of pores and micro-fissures. It is evident that for the WCp-Grp reinforcement to exist in the Ti-6Al-4V matrix, it was dispersed equally throughout the composites.
iv. At the steady state regime, the WCp and GRp reinforcement particles are firmly attached to the matrix phase, resulting in a smooth surface, as revealed by SEM characterization of the wear surfaces developed into dry sliding wear. Furthermore, reinforcements have remained relatively high. This is brought about by the tribo surface reinforcements’ self-lubricating properties.
v. The results showed that the XGBoost classifier had a prediction accuracy of 56.25%, and the SVM had a prediction accuracy of 65%. It was discovered that the kNN classification has a prediction accuracy of 71.25%.
Future scope of the work includes synthesis of WCp-Grp reinforced Ti6Al4V matrix composite with higher percentage of reinforcement. Further enhancing the accuracy of the machine learning classifiers by hyper-parameters tuning.
Data availability statement
The original contributions presented in the study are included in the article/Supplementary Material, further inquiries can be directed to the corresponding author.
Author contributions
SS: Data curation, Funding acquisition, Investigation, Methodology, Project administration, Resources, Validation, Writing–review and editing. VJ: Conceptualization, Data curation, Funding acquisition, Investigation, Project administration, Supervision, Visualization, Writing–original draft, Writing–review and editing. DS: Investigation, Project administration, Resources, Writing–original draft, Writing–review and editing. RD: Investigation, Methodology, Software, Validation, Visualization, Writing–review and editing. RC: Conceptualization, Data curation, Funding acquisition, Investigation, Validation, Writing–review and editing. EN: Conceptualization, Software, Validation, Writing–review and editing. HM: Data curation, Formal Analysis, Funding acquisition, Software, Writing–review and editing.
Funding
The author(s) declare that financial support was received for the research, authorship, and/or publication of this article. The authors present their appreciation to King Saud University for funding this research through Researchers Supporting Program number (RSPD2024R1006), King Saud University, Riyadh, Saudi Arabia.
Acknowledgments
Authors thank the college administration for supporting this work.
Conflict of interest
The authors declare that the research was conducted in the absence of any commercial or financial relationships that could be construed as a potential conflict of interest.
Publisher’s note
All claims expressed in this article are solely those of the authors and do not necessarily represent those of their affiliated organizations, or those of the publisher, the editors and the reviewers. Any product that may be evaluated in this article, or claim that may be made by its manufacturer, is not guaranteed or endorsed by the publisher.
References
Abd-Elwahed, M. S., Ibrahim, A. F., and Reda, M. M. (2020). Effects of ZrO2 nanoparticle content on microstructure and wear behavior of titanium matrix composite. J. Mater. Res. Technol. 9 (4), 8528–8534. doi:10.1016/j.jmrt.2020.05.021
An, Q., Huang, L., Jiang, S., Wang, S., Zhang, R., Sun, F., et al. (2019a). The wear rate of titanium matrix composite coating at high temperature is further increased by non-stoichiometric TixC oxidation. Ceram. Int., S0272884219335096. doi:10.1016/j.ceramint.2019.12.032
An, Q., Huang, L., Jiao, Y., Bao, Y., Zhong, B., and Geng, L. (2019b). Intergrowth microstructure and superior wear resistance of (TiB + TiC)/Ti64 hybrid coatings by gas tungsten arc cladding. Mat. Des. 162, 34–44. doi:10.1016/j.matdes.2018.11.039
An, Q., Huang, L. J., Jiang, S., Bao, Y., Ji, M., Zhang, R., et al. (2018). Two-scale TiB/Ti64 composite coating fabricated by two-step process. J. Alloy. Comp. 755, 29–40. doi:10.1016/j.jallcom.2018.05.002
Attar, H., Ehtemam-Haghighi, S., Kent, D., and Dargusch, M. S. (2018). Recent developments and opportunities in additive manufacturing of titanium-based matrix composites: a review. Int. J. Mach. Tools Manuf. 133, 85–102. doi:10.1016/j.ijmachtools.2018.06.003
Buccino, F., Aiazzi, I., Casto, A., Liu, B., Sbarra, M. C., Ziarelli, G., et al. (2023b). The synergy of synchrotron imaging and convolutional neural networks towards the detection of human micro-scale bone architecture and damage. J. Mech. Behav. Biomed. Mater. 137, 105576. doi:10.1016/j.jmbbm.2022.105576
Buccino, F., Zagra, L., Longo, E., D’Amico, L., Banfi, G., Berto, F., et al. (2023a). Osteoporosis and Covid-19: detected similarities in bone lacunar-level alterations via combined AI and advanced synchrotron testing. Mater. Des. 231, 112087. doi:10.1016/j.matdes.2023.112087
Cao, H. C., and Liang, Y. L. (2020). The microstructures and mechanical properties of graphene reinforced titanium matrix composites. J. Alloys Compd. 812, 152057. doi:10.1016/j.jallcom.2019.152057
Chao, C. A. I., Shan, H. E., Li-fan, L. I., Qing, TENG, Bo, SONG, Chun-ze, Y. A. N., et al. (2019). In-situ TiB/Ti−6Al−4V composites with a tailored architecture produced by hot isostatic pressing: microstructure evolution, enhanced tensile properties and strengthening mechanisms. Compos. Part. B Eng. 164, 546–558. doi:10.1016/j.compositesb.2019.01.080
Chen, J., Ren, S., He, X., and Qu, X. (2017). Properties and microstructure of nickel-coated graphite flakes/copper composites fabricated by spark plasma sintering. Carbon 121, 25–34. doi:10.1016/j.carbon.2017.05.082
Choe, H., Abkowitz, S. M., Abkowitz, S., and Dunand, D. C. (2005a). Effect of tungsten dissolution on the mechanical properties of Ti W composites. J. Alloys Compd. 390, 62–66. doi:10.1016/j.jallcom.2004.08.021
Choe, H., Abkowitz, S. M., Abkowitz, S., and Dunand, D. C. (2005b). Effect of tungsten additions on the mechanical properties of Ti-6Al- 4V. Mater Sci. Eng. A 396, 99–106. doi:10.1016/j.msea.2005.01.051
Dhungana, D. S., Bonaventura, E., Martella, C., Grazianetti, C., and Molle, A. (2023). Solid phase crystallization of amorphous silicon at the two-dimensional limit. Nanoscale Adv. 5, 668–674. doi:10.1039/d2na00546h
Dhungana, D. S., Grazianetti, C., Martella, C., Achilli, S., Fratesi, G., and Molle, A. (2021). Two-dimensional silicene–stanene heterostructures by epitaxy. Adv. Funct. Mater. 31 (30), 2102797. doi:10.1002/adfm.202102797
Dhungana, D. S., Hemeryck, A., Sartori, N., Fazzini, P. F., Cristiano, F., and Plissard, S. R. (2019). Insight of surface treatments for CMOS compatibility of InAs nanowires. Nano Res. 12, 581–586. doi:10.1007/s12274-018-2257-8
Dhungana, D. S., Mallet, N., Fazzini, P. F., Larrieu, G., Cristiano, F., and Plissard, S. R. (2022). Self-catalyzed InAs nanowires grown on Si: the key role of kinetics on their morphology. Nanotechnology 33 (48), 485601. doi:10.1088/1361-6528/ac8bdb
Farías, I., Olmos, L., Jiménez, O., Flores, M., Braem, A., and Vleugels, J. (2019). Wear modes in open porosity titanium matrix composites with TiC addition processed by spark plasma sintering. Trans. Nonferrous Metals Soc. China 29 (8), 1653–1664. doi:10.1016/S1003-6326(19)65072-7
Frary, M., Abkowitz, S., Abkowitz, S. M., and Dunand, D. C. (2003). Microstructure and mechanical properties of Ti/W and Ti-6Al-4V/W composites fabricated by powder-metallurgy. Mater Sci. Eng. A 344, 103–112. doi:10.1016/S0921-5093(02)00426-4
Greco, L., Buccino, F., Xu, Z., Vergani, L., Berto, F., Guagliano, M., et al. (2023). Design and analysis of energy-absorbent bioinspired lattice structures. J. Bionic Eng. 20, 1670–1686. doi:10.1007/s42235-023-00358-6
Guo, X., Wang, L., Wang, M., Qin, J., Zhang, D., and Lu, W. (2012). Effects of degree of deformation on the microstructure, mechanical properties and texture of hybrid-reinforced titanium matrix composites. Acta Mater 60, 2656–2667. doi:10.1016/j.actamat.2012.01.032
Gupta, A., Hussain, M., Misra, S., Das, A. K., and Mandal, A. (2018). Processing and characterization of laser sintered hybrid B 4 C/cBN reinforced Ti-based metal matrix composite. Opt. Lasers Eng. 105, 159–172. doi:10.1016/j.optlaseng.2018.01.015
Gürbüz, M., Mutuk, T., and Uyan, P. (2021). Mechanical, wear and thermal behaviors of graphene reinforced titanium composites. Met. Mat. Int. 27, 744–752. doi:10.1007/s12540-020-00673-1
Hayat, M. D., Singh, H., He, Z., and Cao, P. (2019). Titanium metal matrix composites: an overview. Compos. Part A 121, 418–438. doi:10.1016/j.compositesa.2019.04.005
Hu, Y., Ning, F., Wang, H., Cong, W., and Zhao, B. (2017). Laser engineered net shaping of quasi-continuous network microstructural TiB reinforced titanium matrix bulk composites: microstructure and wear performance. Opt. Laser Technol. 99, 174–183. doi:10.1016/j.optlastec.2017.08.032
Jiao, Y., Huang, L., and Geng, L. (2018). Progress on discontinuously reinforced titanium matrix composites. J. Alloys Compd. 767, 1196–1215. doi:10.1016/j.jallcom.2018.07.100
Jin, J., Zhou, S., Zhao, Y., Zhang, Q., Wang, X., Li, W., et al. (2021). Refined microstructure and enhanced wear resistance of titanium matrix composites produced by selective laser melting. Opt. Laser Technol. 134, 106644. doi:10.1016/j.optlastec.2020.106644
Kim, I. Y., Choi, B. J., Kim, Y. J., and Lee, Y. Z. (2011). Friction and wear behavior of titanium matrix (TiBþTiC) composites. Wear 271, 1962e5. doi:10.1016/j.wear.2010.12.072
Kondoh, K., Threrujirapapong, T., Imai, H., Umeda, J., and Fugetsu, B. (2008). CNTs/TiC reinforced titanium matrix Nanocomposites via powder metallurgy and its microstructural and mechanical properties. J. Nanomater. 2008, 1–4. doi:10.1155/2008/127538
Li, S., Kondoh, K., Imai, H., Chen, B., Jia, L., and Umeda, J. (2015). Microstructure and mechanical properties of P/M titanium matrix composites reinforced by in-situ synthesized TiC-TiB. Mater Sci. Eng. A 628, 75–83. doi:10.1016/j.msea.2015.01.033
Maleki, E., Bagherifard, S., and Guagliano, M. (2023). Correlation of residual stress, hardness and surface roughness with crack initiation and fatigue strength of surface treated additive manufactured AlSi10Mg: experimental and machine learning approaches. J. Mater. Res. Technol. 24, 3265–3283. doi:10.1016/j.jmrt.2023.03.193
Maleki, E., Unal, O., Guagliano, M., and Bagherifard, S. (2022). Analysing the fatigue behaviour and residual stress relaxation of gradient nano-structured 316L steel subjected to the shot peening via deep learning approach. Metals Mater. Int. 28, 112–131. doi:10.1007/s12540-021-00995-8
Mathews, S. M. (2019). “Explainable artificial intelligence applications in NLP, biomedical, and malware classification: a literature review,” in Intelligent computing: proceedings of the 2019 computing conference, volume 2 (Springer International Publishing), 1269–1292.
Mishra, A., and Jatti, V. S. (2023a). Novel coupled genetic algorithm–machine learning approach for predicting surface roughness in fused deposition modeling of polylactic acid specimens. J. Mater. Eng. Perform., 1–10. doi:10.1007/s11665-023-08379-2
Mishra, A., and Jatti, V. S. (2023b). Prediction of wear rate in Al/SiC metal matrix composites using a neurosymbolic artificial intelligence (NSAI)-Based algorithm. Lubricants 11, 261. doi:10.3390/lubricants11060261
Mishra, A., Jatti, V. S., and Messele Sefene, E. (2023). Exploratory analysis and evolutionary computing coupled machine learning algorithms for modelling the wear characteristics of AZ31 alloy. Mater. Today Commun. 37, 107507. doi:10.1016/j.mtcomm.2023.107507
Perundyurai Thangavel, S., Vellingiri, S., Rajendrian, S., Munusamy, S., and Chinnaiyan, S. (2020). K-nearest neighbour technique for the effective prediction of refrigeration parameter compatible for automobile. Therm. Sci. Link. is Disabl. 24 (1), 565–569. doi:10.2298/tsci190623436p
Ram, P. T., Varma, V. K., and Vedantam, S. (2014). Tribological and mechanical behavior of multilayer Cu/SiC + Gr hybrid composites for brake friction material applications. Wear 317, 201–212. doi:10.1016/j.wear.2014.06.006
Sadek, D., Dhungana, D. S., Coratger, R., Durand, C., Proietti, A., Gravelier, Q., et al. (2021). Integration of the rhombohedral BiSb (0001) topological insulator on a cubic GaAs (001) substrate. ACS Appl. Mater. Interfaces 13 (30), 36492–36498. doi:10.1021/acsami.1c08477
Soorya Prakash, K., Gopal, P. M., Anburose, D., and Kavimani, V. (2016). Mechanical, corrosion and wear characteristics of powder metallurgy processed Ti-6Al-4V/B4C metal matrix composites. Ain Shams Eng. J. 9, 1489–1496. doi:10.1016/j.asej.2016.11.003
Sun, S., Zhao, E., Hu, C., Tian, Y., Chen, W., Cui, H., et al. (2020). Deformation behavior and softening mechanism of TiB reinforced near-a titanium matrix composite during hot compression. J. Mater Res. Technol. 9 (6), 13250–13263. doi:10.1016/j.jmrt.2020.09.030
Suresh, V., Hariharan, N., and Vellingiri, S. (2018). An investigation on the tensile properties and micro-structure of hybrid metal matrix composites. Int. J. Mater. Prod. Technol. 56 (1/2), 84–94. doi:10.1504/IJMPT.2018.089119
Wang, F. C., Zhang, Z. H., Sun, Y. J., Liu, Y., Hu, Z. Y., Wang, H., et al. (2015). Rapid and lowtemperature spark plasma sintering synthesis of novel carbon nanotube reinforced titanium matrix composites. Carbon 95, 396–407. doi:10.1016/j.carbon.2015.08.061
Wang, H., Zhang, Z., Zhang, H., Hu, Z., Li, S., and Cheng, X. (2017). Novel synthesizing and characterization of copper matrix composites reinforced with carbon nanotubes. Mater Sci. Eng. A 696, 80–89. doi:10.1016/j.msea.2017.04.055
Wang, J., Li, L., Lin, P., and Wang, J. (2018). Effect of TiC particle size on the microstructure and tensile properties of TiCp/Ti6Al4V composites fabricated by laser melting deposition. Opt. Laser Technol. 105, 195–206. doi:10.1016/j.optlastec.2018.03.009
Xi, L., Ding, K., Gu, D., Guo, S., Cao, M., Zhuang, J., et al. (2021). Interfacial structure and wear properties of selective laser melted Ti/(TiC+TiN) composites with high content of reinforcements. J. Alloys Compd. 870, 159436. doi:10.1016/j.jallcom.2021.159436
Xia, M., Liu, A., Hou, Z., Li, N., Chen, Z., and Ding, H. (2017). Microstructure growth behavior and its evolution mechanism during laser additive manufacture of in-situ reinforced (TiB+TiC)/Ti composite. J. Alloy. Compd. 728, 436–444. doi:10.1016/j.jallcom.2017.09.033
Xie, H., Jin, Y., Niu, M., Liu, N., and Wang, J. (2021). Effect of tribofilm induced by nanoparticle addition on wear behavior of titanium-matrix composite. Tribol. Lett. 69, 18. doi:10.1007/s11249-020-01387-6
Yang, Q., Wang, Z., Guo, K., Cai, C., and Qu, X. (2023). Physics-driven synthetic data learning for biomedical magnetic resonance: the imaging physics-based data synthesis paradigm for artificial intelligence. IEEE Signal Process. Mag. 40 (2), 129–140. doi:10.1109/msp.2022.3183809
Zhang, C., Zhang, S., Lin, P., Hou, Z., Kong, F., and Chen, Y. (2016). Thermomechanical processing of (TiB þ TiC)/Ti matrix composites and effects on microstructure and tensile properties. J. Mater Res. 31, 1244e53. doi:10.1557/jmr.2016.143
Keywords: titanium metal matrix composite, K-nearest neighboring, support vector machine, XGBoost, wear rate, tribology
Citation: Jatti VS, Sawant DA, Deshpande R, Saluankhe S, Cep R, Nasr EA and Mahmoud HA (2024) Tribological analysis of titanium alloy (Ti-6Al-4V) hybrid metal matrix composite through the use of Taguchi’s method and machine learning classifiers. Front. Mater. 11:1375200. doi: 10.3389/fmats.2024.1375200
Received: 23 January 2024; Accepted: 21 March 2024;
Published: 12 April 2024.
Edited by:
Natrayan L., Saveetha University, IndiaReviewed by:
Mahesh Shewale, ASML, United StatesNaveen Venkatesh S., Vellore Institute of Technology (VIT), India
Subash Thanappan, KAAF University College, Ghana
Copyright © 2024 Jatti, Sawant, Deshpande, Saluankhe, Cep, Nasr and Mahmoud. This is an open-access article distributed under the terms of the Creative Commons Attribution License (CC BY). The use, distribution or reproduction in other forums is permitted, provided the original author(s) and the copyright owner(s) are credited and that the original publication in this journal is cited, in accordance with accepted academic practice. No use, distribution or reproduction is permitted which does not comply with these terms.
*Correspondence: Sachin Saluankhe, ZHJzYWx1bmtoZXNhY2hpbkB2ZWx0ZWNoLmVkdS5pbg==