- School of Accounting, Jiangsu University, Zhenjiang, China
Introduction: The digital era has brought about new vitality and innovation across various industries, introducing concepts such as AI, information centers, cloud data, the Internet of Things, and digital government. This article investigates the mechanisms of financial information disclosure within the context of digital transformation.
Methods: A four-party game model is developed using a game approach, involving digitally-intelligent enterprises, financial data analysts, new quality productivity-based government, and fintech users. Through Matlab simulations, the evolutionary relationships and equilibrium game strategies among the four parties are examined.
Conclusion: (1) There are four conditional stabilization points in the four-way evolutionary game. These four conditional stabilization points represent the strategic equilibriums that stakeholders may achieve under the principle of competitive neutrality. They mainly reflect the strategic choices of the government and enterprises under different scenarios. (2) The numerical simulation analysis reveals that when the new quality productivity-based government will strengthen the regulation of the firm, it will force the firm to disclose information. And when the enterprise chooses to disclose information when disclosing financial information, then the government will relax the state of its regulation. This means that the government plays a stronger supervisory role in the process of corporate disclosure, which makes enterprises tend to increase the degree of information disclosure under external pressure, thus reducing information asymmetry and enhancing information transparency, which helps financial data analysts and fintech users to collect and organize information, and improves the stability of the market. The overall strategy evolves in the direction of “information disclosure, intelligent decision-making, intensive regulation, and investment”. (3) From the sensitivity analysis, the government’s influence on the utility of digital intelligence transformation is more significant, compared with other social actors, government departments show more significant institutional advantages in the digital intelligence transformation process; and the stronger the incentive and punishment mechanism imposed by the new quality productivity-oriented government, the strongest the reaction of enterprises in the societies studied in this paper; furthermore, it is found that there exists a bidirectional feedback mechanism between analysts and investors, and that when the poor market information environment leads to low investment intention of investors, it will make analysts reluctant to spend time and cost. Further, it is found that there is a two-way feedback mechanism between analysts and investors, when the poor market information environment leads to low investors' willingness to invest, it makes analysts reluctant to spend time, energy and cost on information processing.
1 Introduction
The advent of the Internet era has ushered in an unprecedented wave of digitalization and informatization, fundamentally characterized by technological convergence [1], systemic reengineering [2], and collaborative value generation [3]. Digital transformation now represents an inexorable shift in modern economic paradigms, with scholars increasingly scrutinizing the critical function of digital transformation facilitators in organizational ecosystems [4]. This economic metamorphosis serves as both a catalyst for sustainable economic practices through green economy advancement [5] and a prerequisite condition for innovation output optimization phenomena intrinsically interwoven with contemporary accounting management frameworks [6, 7]. Digital transformation, although fostering innovation, presents notable risks and challenges to an organization’s strategic decision-making [8]. It complicates effective governance and management, thereby altering the approach to technology development and maintenance [9]. Consequently, internal governance and external regulation face heightened demands [10]. Yet, this does not imply stagnation. The deep integration of digitalization and finance represents an inevitable trend in modern society.
Corporate entities, governmental regulators, financial analysts, and institutional investors constitute the principal architects and accelerators of digital transformation. The process enhances organizational agility and market responsiveness [11], while simultaneously elevating financial disclosure capabilities and improving the quality of accounting information. This evolution has precipitated the emergence of specialized financial data analysts sophisticated information intermediaries who employ advanced analytical techniques to interpret corporate disclosures and generate authoritative investment intelligence [12, 13]. Distinguished from traditional analysts through superior information retrieval competencies and technological utilization capacities [14], these professionals represent a paradigm shift in financial intermediation. The process establishes firms as information providers and analysts as information intermediaries, whose processed outputs influence downstream users. Within this digital milieu, the concept of new quality productivity has emerged as a transformative framework emphasizing technological sophistication, operational excellence, and output superiority [15, 16]. Concurrently, governmental entities are undergoing their own digital metamorphosis to enhance public service delivery and market regulation effectiveness [17], transitioning toward governance models rooted in new quality productivity principles [18]. This dual transformation across public and private sectors has precipitated stakeholder dissatisfaction with conventional financial metrics and disclosure paradigms [19], resulting in intensified pressure for enhanced corporate transparency and growing demand for high-fidelity financial information characterized by superior quality and operational verifiability.
Emerging scholarship substantiates the transformative effects of digitalization on corporate disclosure practices. He et al. [20] empirically established that digital transformation induces structural enhancements in organizational disclosure behavior. Complementing this, Henide [21] demonstrated that corporate strategic evolution exhibits responsiveness to investor expectations, while De Villiers et al. [22] identified concurrent improvements in environmental performance metrics. Donkor et al. [23] further corroborated these findings, revealing a positive correlation between technological advancement and disclosure quality. From a methodological perspective, research on digitalization and disclosure has evolved through distinct phases. Initial studies relied on traditional empirical approaches using panel data. Subsequent advancements introduced computational tools like Python, enabling real-time sampling and fully automated data collection. The methodological framework was further enhanced through machine learning techniques, expanding the analytical dimensions. Currently, the field has progressed to incorporate AI technologies, demonstrating a clear trajectory toward interdisciplinary integration. Furthermore, game-theoretic analytical frameworks have been incorporated to model disclosure dynamics. Calford [24] posited that strategic uncertainty serves as a critical determinant of agent behavior within game-theoretic constructs, which fundamentally examine multi-agent interactions characterized by divergent utility functions [25] and optimize payoff maximization strategies [26]. This implies that the game evolution is a search for logistic lines among various uncertainties. Early applications in disclosure research include Guan et al. [27], who delineated regulatory pathway mechanisms within state-dominated market paradigms. Subsequent investigations by Zhu et al. [28] revealed industry-wide spillover effects of corporate carbon disclosure practices, whereas Zhao and Zhu [29] identified countervailing pressures from escalating disclosure costs, suggesting potential dampening effects on voluntary transparency incentives. The direction of evolution is described from different perspectives. All in all, these findings collectively underscore the inherent stochasticity in corporate disclosure decisions, mediated through cost-benefit calculus. Game-theoretic modeling predominantly centers on government-enterprise dyads as principal strategic actors. Illustrative applications include tripartite equilibria analysis for green production strategies involving government, firms, and civil society [30], and bilateral models of carbon disclosure governance [31]. While quadripartite game architectures have been deployed to examine patent valuation dynamics [32] and competitive neutrality evolution [33], significant lacunae persist in understanding disclosure pathway mechanisms under digital transformation regimes. Notably absent are sophisticated models that reconcile the dual imperatives of technological disruption and regulatory adaptation within accounting information ecosystems.
Critical analysis of extant literature reveals three substantive limitations in current digitalized financial disclosure research: First, methodological insularity persists, with predominant reliance on empirical methodologies confined within economic discipline paradigms, exhibiting insufficient engagement with cross-disciplinary integration and methodological pluralism. Second, game-theoretic examinations remain constrained by limited participant scope, disproportionately focused on enterprise-government dyads while neglecting broader stakeholder ecosystems essential in digital transformation contexts. Third, thematic orientation excessively prioritizes regulatory compliance dynamics, failing to establish comprehensive analytical frameworks that elucidate systemic pathway mechanisms governing financial information flows.
The research layout of this paper is as follows. Section 2 is the game model construction, which describes the research assumptions and the stability analysis of the subject’s strategies; Section 3 is the stability analysis of the strategy combinations; Section 4 is the simulation to understand the trend of the game evolution of each party; and Section 5 introduces the conclusion as well as the practical suggestions.
2 Construction of the four-party evolutionary game model
2.1 Problem description and basic model assumptions
The new quality productivity-based government implements incentives for companies and compensation mechanisms for analysts and investors in order to realize the transformation of government functions and the upgrading of services. And digital-intelligent companies enhance their services in management mode through digital and intelligent means, and disclose digital-intelligent information to the public. And further influence analysts decision-making strategy and investor investment strategy. Financial data analysts compared to traditional analysts are more favorable and use technology to collect, process and analyze data. Their intelligent decision-making will have a greater impact on investors’ strategic choices and corporate disclosure. Fintech users are able to make wise, objective and logical investment decisions based on the implementation of the basis of the user. They tend to obtain numerical intelligent information to satisfy their needs. This paper focuses on investors in this user group.
Corporate entities, driven by profit-maximization imperatives, strategically suppress adverse operational intelligence to distort analyst evaluations and investor valuations. Regulatory enforcement mechanisms mandate partial disclosure compliance, though their efficacy remains constrained by jurisdictional enforcement capacities. In order to better study the financial information disclosure mechanism of digital intelligence, this paper constructs a four-party game model with the participation of digital intelligence enterprises, financial data analysts, new quality productivity-based government and fintech users, and the logical relationship is shown in Figure 1.
Assumption 1. The digital intelligence enterprise applies digital and information technology in depth to disclose high-quality financial information to the outside world. In the case of information disclosure and information concealment, the value-added of the enterprise’s digital intelligence disclosure is E1 and E2, respectively. And the disclosure cost is CE; based on the incentive mechanism, it needs to pay the government’s penalty expenditure P, when the enterprise is found to conceal the information under government supervision. And the enterprise is given an incentive amount of R1 for the enterprise’s proactive disclosure of information.
Assumption 2. The value-added gains of financial data analysts’ intelligent and traditional decision-making are A1 and A2, respectively; the costs of extracting information from the complicated market environment versus making decisions are CA and CA’, respectively. In the case of digital information gain, analysts use fintech tools to collect and analyze data, which makes information acquisition more convenient. Therefore, the analyst’s benefit under fintech will be enhanced by the coefficient of a (a≥1), and the cost of data analysis will be optimized by the coefficient of b (0 ≤ b ≤ 1).
Assumption 3. The benefits of intensive and weak regulation by the new quality productivity government are G1 and G2, respectively; and their costs are CG and CG’ respectively, which are both affected by the gain of firms’ digital intelligence. The government has a compensation policy for analysts’ information decision-making and investors’ investment behavior under fintech, subsidizing them accordingly with R2 and R3.
Assumption 4. Fintech users include individuals or enterprises that carry out various financial activities through the Internet financial platforms. It mainly includes groups that use digital financial technology to carry out investment and other operations, and this paper chooses one of the representative groups, investors, as the main research subject. When they collect information, they pay attention to the decision-making opinions of professional analysts, so the investment returns in the case of intelligent decision-making and traditional decision-making by analysts under financial technology are I1 and I2, respectively; while the return of not investing is I3; the cost of pre-investment decision-making and analysis by investors based on facts and data are CI and CI’, respectively. Investor behavior will also be affected by firms’ digital intelligence disclosure, and the benefits and costs will be improved by the coefficients of a and b.
Assumption 5. Furthermore, when analysts make intelligent decisions under fintech, it affects the decisions of corporate management and investors. Digital-intelligent firms and institutional investors add additional revenues M and N, respectively; similarly, the latter can add value to the former in terms of revenue K; and when analysts make intelligent decisions under fintech, they will be favored by institutional investors and will bring additional revenues Q.
Based on the above assumptions, the payoff matrix of the four main subjects can be obtained as shown in Table 1.
2.2 Analysis of strategy portfolio and strategy stability of each game subject
2.2.1 Analysis of subject strategy stability for digital intelligence enterprise
Digital intelligence enterprise exhibit differentiated expected payoffs when disclosing that is UE1 versus concealing that is UE2 data-driven financial intelligence, with UE denoting mean equilibrium returns. And the strategic adaptation trajectories are governed by the replicator dynamics formalized in Equations 1, 2, with behavioral strategy evolution characterized through first-order derivative analysis.
According to Equation 1, let
Proposition 1 Digital intelligence enterprises exhibit phase-dependent disclosure equilibria: z < z0 induces evolutionarily stable strategies (ESS) favoring information obfuscation; z > z0 precipitates ESS convergence toward transparency adoption. Strategic indeterminacy emerges at z = z0, where the critical disclosure threshold is: z0 = [1/(P + R1)]*[CE + E2-E1+M(1-a)y].
Proof According to the differential variance stability theorem, if “Information Disclosure” is the evolutionary stable state of numerical intelligence enterprise strategy choice, then it needs to satisfy F(x) = 0 and F′(x) < 0.
Let
Therefore, H(z) is an increasing function with respect to z. When z < z0, H(z) < 0, then F(x)|x=0 = 0, F′(x)|x=0 < 0, at this time x = 0 is the evolutionary stability point, which indicates that when the probability of the new productivity government choosing the “Intensive Regulation” strategy is less than z0, the digital intelligence enterprise will ultimately choose the “Information Concealment” strategy. When z > z0, H(z) > 0, then F(x)|x=1 = 0, F′(x)|x=1 < 0, at this time x = 1 is the evolutionary stability point, which indicates that when the probability of the new productivity government choosing the “Intensive Regulation” strategy is greater than z0, the number of intellectualized firms will ultimately choose the “Information Disclosure” strategy. Q.E.D.
2.2.2 Analysis of subject strategy stability for financial data analysts
The expected returns of financial data analysts making decisions using smart decisions versus traditional decisions are UA1 and UA2 respectively. The average expected return is UA. The replicated dynamic equations and first-order derivatives of their behavioral strategies are shown in Equations 3, 4.
According to Equation 3, let
Proposition 2 Financial analysts’ evolutionarily stable strategies (ESS) demonstrate phase-dependent strategic adaptation: w < w0 induces ESS convergence toward traditional information decision-making; w > w0 precipitates ESS shifts to intelligent information decision-making frameworks. The strategic indeterminacy emerges at w = w0, where the critical threshold is: w0=(-1/Q)*[A1-A2-CA + CA'+(A2-A1+CA-CA'+aA1-aA2+bCA'-bCA)x].
Proof According to the differential variance stability theorem, if “Intelligent Decision Making” is the evolutionary stable state chosen by financial data analysts, then F(y) = 0 and F′(y) < 0 should be satisfied.
Let
Therefore, H(w) is an increasing function with respect to w. When w < w0, H(w) < 0, then F(y)|y=0 = 0, F′(y)|y=0 < 0, at this time, y = 0 is the evolutionary stability point, which indicates that when the probability of the investor in the fintech user choosing “Invest” is less than w0, the financial data analyst will ultimately choose the “Traditional Decision Making” strategy. When w > w0, H(w) > 0, then F(y)|y=1 = 0, F′(y)|y=1 < 0, at this time y = 1 is the evolutionary stability point, which indicates that when the probability of investors choosing to “Invest” is greater than w0, financial data analysts will ultimately choose the “Intelligent Decision Making”. Q.E.D.
2.2.3 Analysis of subject strategy stability for new quality productivity-based government
The expected returns to intensive and weak implementation of regulation by the new quality productivity type of government are UG1 and UG2 respectively. The average expected return is UG. The replicated dynamic equations and the first order derivatives of their behavioral strategies are shown in Equations 5, 6.
According to Equation 5, let
Proposition 3 The equilibrium regulatory posture of new quality productivity-based government demonstrates phase-dependent adaptation: under x < x0, stringent oversight mechanisms constitute evolutionarily stable strategies (ESS); whereas x > x0 induces ESS convergence toward attenuated regulatory interventions. The strategic indeterminacy emerges at x = x0, where the critical threshold parameter x0 is mathematically defined: x0 = −[1/(P + R1)]*(G2-CG'-P + R2+bCG-aG1+R3w).
Proof According to the differential variance stability theorem, if “Intensive regulation” is the evolutionary stable state of the new productivity-oriented government strategy choice, then F(z) = 0 and F′(z) < 0 should be satisfied.
Let
Thus, H(x) is an increasing function with respect to x. When x < x0, H(x) < 0, then F(z)|z=1 = 0, F′(z)|z=1 < 0, at this time z = 1 is the evolutionary stability point, which indicates that when the probability of numerical intelligence enterprises choosing the “Information Disclosure” is less than x0, the new quality productivity government will ultimately choose the “Intensive Regulation” strategy. When x > x0, H(x) > 0, then F(z)|z=0 = 0, F′(z)|z=0 < 0, at this time z = 0 is the point of evolutionary stability, which indicates that when the probability of NIEs choosing to “Information Disclosure” is greater than x0, the new productivity-based government will eventually choose the “Weak Regulation” strategy. Q.E.D.
2.2.4 Analysis of subject strategy stability for fintech users
The expected returns of fintech users who make investment decisions to choose to invest and not to invest are UI1 and UI2, respectively. The average expected return is UI. The replicated dynamic equations and first-order derivatives of their behavioral strategies are shown in Equations 7, 8.
According to Equation 7, let
Proposition 4 Fintech users demonstrate phase-dependent investment equilibria: z < z0 induces evolutionarily stable strategies (ESS) favoring investment avoidance; z > z0 precipitates ESS convergence toward non-investment. Strategic indeterminacy emerges at z = z0, and the investment is: z0=(-1/R3)*{(I2-CI'-I3)+[(1-b)CI'+(a-1)I2]x+(CI'−CI + I1+I2+N)y+[(1-b)CI+(b-1)CI'+(a-1)I1+(1-a)I2]xy}.
Proof According to the differential variance stability theorem, if “Invest” is the evolutionary stable state chosen by fintech users, then F(w) = 0 and F′(w) < 0 should be satisfied.
Let
Therefore, H(z) is an increasing function with respect to z. When z < z0, H(z) < 0, then F(w)|w=0 = 0, F′(w)|w=0 < 0, at this time, w = 0 is the evolutionary stability point, which indicates that when the probability of the new productive government choosing the “Intensive Regulation” strategy is less than z0, the investors among the fintech users will eventually choose the “Don’t Invest” strategy. When z > z0, H(z) > 0, then F(w)|w=1 = 0, F′(w)|w=1 < 0, at this time, w = 1 is the evolutionary stability point, which suggests that when the probability of the new productive government choosing the “Intensive regulation” strategy is greater than z0, the investors among the fintech users will eventually choose the “Invest” strategy. Q.E.D.
3 Analysis of the stable strategy of the four-party evolutionary game
3.1 Analysis of the equilibrium points
The equation for the replication dynamics of the subjects of the associative game, let
According to Selten [34], the strict nash equilibrium is a stable solution of the evolutionary game, and this strict nash equilibrium is pure strategy. As a result, we can obtain a total of 16 strategy equilibria that meet the conditions, which are: (0,0,0,0), (0,0,0,1), (0,0,1,0), (0,0,1,1), (0,1,0,0), (1,0,0,0), (1,0,0,1), (0,1,0,1), (0,1,1,0), (1,0,1,0), (0,1,1,1), (1,0,1,1), (1,1,0,1), (1,1,0,0), (1,1,1,0) and (1,1,1,1).
Based on the evolutionary game analysis method of Friedman [35], the article constructs the Jacobi matrix of the replicated dynamical system: J.
The 16 equilibrium points are substituted into the Jacobi matrix J, and the corresponding Jacobi matrix eigenvalues of each equilibrium point are shown in Table 2.
In order to simplify and without loss of generality the stability analysis of replicated dynamic systems for the discovery and transformation of pathways for the role of digital intelligence transformation. The article takes into account the practical context and hypothesizes:
3.2 Stability analysis of the combination of intensive regulation by new quality productivity-based government
The asymptotic stability analysis of the equilibrium points of the complex dynamic system in the state of strong government regulation, satisfying the ∆G-∆CG > R condition. From Column 1 in Table 3, the strategy is eventually stabilized at the points E (1,1,1,1) and E (0,1,1,1). This suggests that the government is under strong regulation and firms will choose information disclosure when they fulfill the condition that the sum of the difference between the benefits of disclosure and concealment, plus the additional benefits from analysts, is greater than the cost of disclosure. This implies that strong government regulation will bring pressure for firms to disclose information, forcing firms to enhance information transparency. Thus, it brings more intellectualized information to analysts, which is more conducive to making intelligent decisions. This also provides more information for investors to choose to invest. When the sum of the difference between the benefits of disclosure and concealment, plus the additional benefits brought by analysts, is less than the cost of disclosure, companies will take the risk of choosing information concealment, even if they are still under strong government regulation.
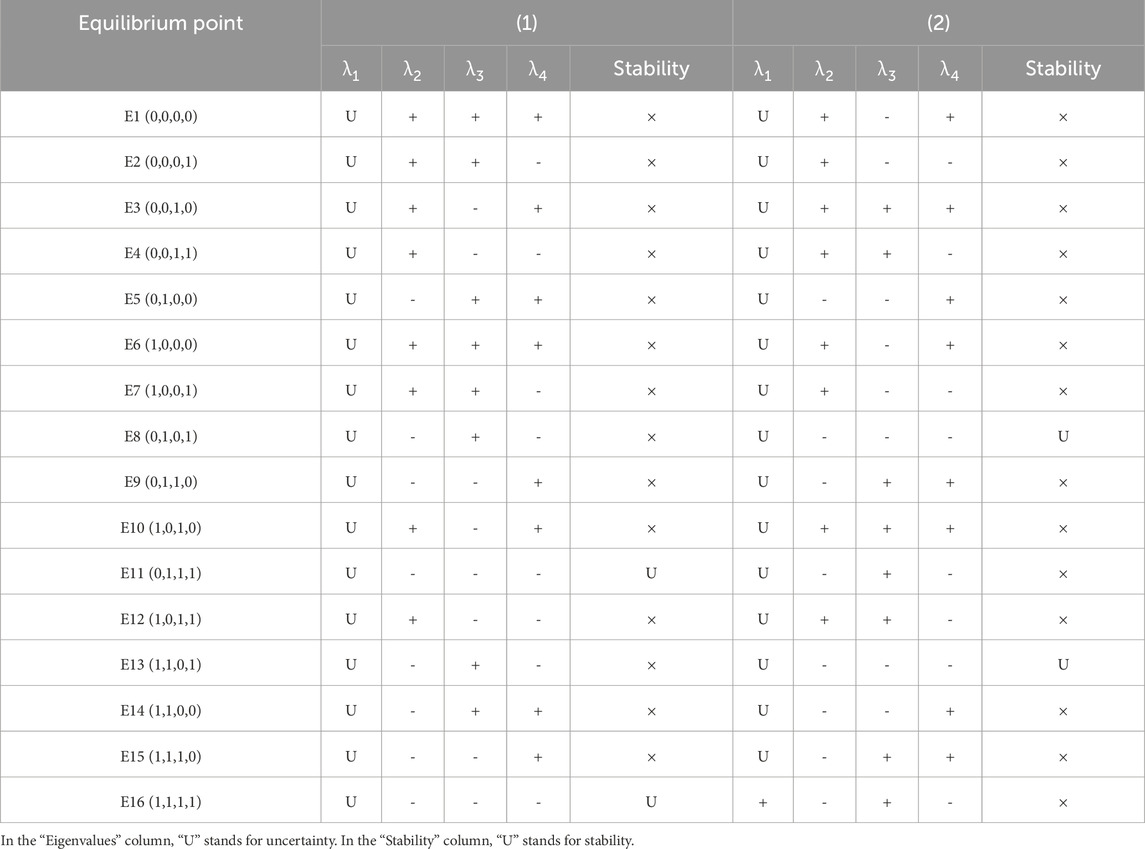
Table 3. Asymptotic stability analysis of equilibrium points of complex dynamic systems under intensive government regulation.
3.3 Stability analysis of the combination of weak regulation by new quality productivity-based government
The asymptotic stability analysis of the equilibrium points of the complex dynamic system in the state of strong government regulation, satisfying the conditions ∆G-∆CG < R, P < R1 or R2 or R3. From Column 2 in Table 3, it can be seen that the final stabilization is at the points E (1,1,0,1) and E (0,1,0,1). That is to say, when the government is under weak regulation, when the enterprise meets the condition that the difference between the benefits of disclosure and concealment, plus the sum of the additional benefits brought by analysts, is greater than the cost of disclosure, the enterprise prefers to choose the disclosure of information, and at this time, the analysts choose the wisdom of decision-making, and the investor chooses to invest. And when the enterprise satisfies the condition that the difference between the benefits of information disclosure and concealment, plus the sum of additional benefits brought by analysts, is smaller than the cost of information disclosure, the enterprise will choose information concealment.
4 Simulation analysis
According to the system of replicated dynamic equations and constraints, the simulation experiment is carried out by Matlab software. It is assumed that the probability of choosing different strategies by digitalized enterprises, fintech analysts, new quality productivity-based government and financial user investors are in the middle of unbiased, i.e., x = y = z = w = 0.5. Referring to the actual situation, the values of the parameters in the evolutionary game are assigned as Table 4 below.
4.1 Evolutionary paths of the parties’ strategies under intensive and weak government regulation
As shown in Figure 2, under strong government regulation, when firms tend to disclose information, the strategy stabilizes at E (1,1,1,1); while under weak government regulation, when firms tend to conceal information, the strategy stabilizes at E (0,1,0,1). This implies that firms’ Numerical Intelligence disclosure is closely related to government regulation. Firms demonstrate greater adherence to market norms when a high-quality, proactive government effectively employs digital regulations. The new productivity-based government, in a state of strong regulation, will give full play to its own high-tech, high-efficiency and high-quality regulatory means to enhance the supervision and management of financial information disclosure of digitally-intelligent enterprises. In addition, due to the existence of reward and punishment mechanisms for enterprises, enterprises tend to be more inclined to disclose information in order to avoid bearing the cost of rectification and punishment by the government.
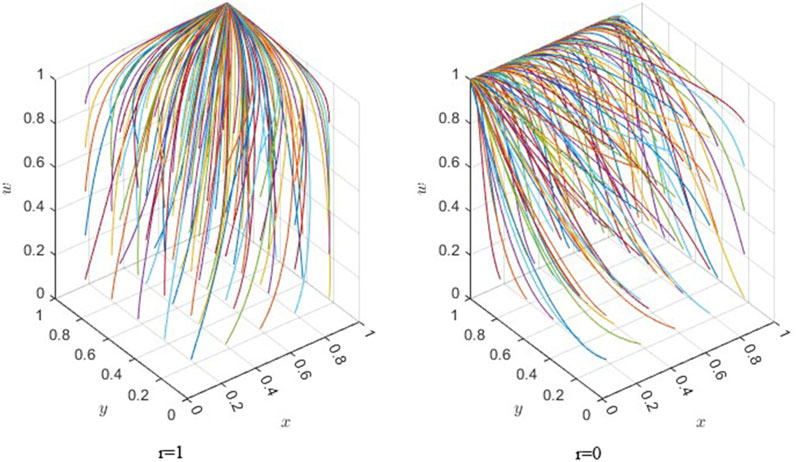
Figure 2. The impact of government regulatory probability on the evolutionary strategies of each party.
4.2 Effect of initial value changes on evolutionary paths
This article chooses, (x,y), (x,z), (x,w), (y,z), (y,w), (z,w) and (z,w), six groups for the simulation of initial value changes. As shown in Figures 3a–c, when x gradually increases, the higher the probability of firms choosing information disclosure, the more analysts tend to make the intelligent decisions, the weaker the government regulation, and investors choose to invest. Similarly, Figures 4a–c demonstrate that increasing y results in analysts making more intelligent decisions, the government leaning towards weaker regulation, and investors showing a greater inclination to invest. And z gradually increases, i.e., the more the government tends to strongly regulate, the more investors tend to invest. Relatively speaking, investors are more sensitive to the response of corporate disclosure, while firms are more sensitive to the strength of government regulation.
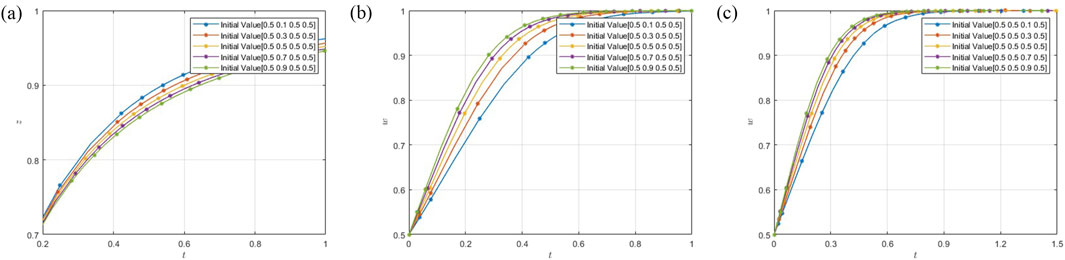
Figure 4. (a–c) Effect of initial value y change on z and w evolutionary paths, and the effect of initial value z change on w evolutionary paths.
The interdependent nature of the strategies chosen by the four parties suggests that each party’s decision significantly influences the interests of the others, leading each party to select strategies that align with its own interests. Specifically, in cases where firms disclose their Numerical Intelligence positively, this disclosure can benefit analysts and investors, prompting their proactive engagement. The government’s strategic choices are contingent upon the maintenance of market fairness by the other parties. When the collective strategies of the other parties ensure economic stability, the government tends to adopt a less stringent regulatory approach. Conversely, a strong regulatory stance by the government compels the other parties to align their strategies within a defined framework, establishing a dynamic of mutual influence and constraint.
4.3 Sensitivity analysis of parameters
4.3.1 Digital intelligence and informatization levels
Let a∈{1,1.3,1.5}, while b∈{0.9,0.6,0.4}.
From Figure 5, it can be seen that for enterprises, the higher the level of digital intelligence, the more enterprises tend to disclose information; the stronger the information processing ability of analysts, the more they tend to use intelligent decision-making; the stronger the government’s technological and informational changes, the more it tends to be weakly regulated; the stronger the ability of investors to use financial technology, the more they tend to invest in high-numerical intelligence enterprises under the influence of the enterprise’s digital intelligence disclosure and the analysts’ intelligent decision-making. At this time, investors show more rapid and stronger positive responses. The government wields a demonstrably more significant influence on the realization of utility derived from digital and intelligent transformation compared to other social entities. Government departments, in particular, exhibit salient institutional advantages during the digital and intelligent transformation process. The construction of intelligent regulatory systems and the establishment of data sharing platforms, driven by the pursuit of new quality productivity, have substantially improved the accuracy and responsiveness of government regulation.
4.3.2 Government incentives and subsidies
Let R1+R2+R3 be equal to R∈{1,2.5,3} while P∈{1,2.5,3}.
As can be seen from Figure 6, the stronger the reward and punishment mechanism imposed by the government, the more enterprises tend to disclose information, while the influence of the later stage is gradually declining; the stronger the government subsidy mechanism, the more investors tend to invest, the overall enthusiasm of investors to invest weakened over time, meaning that in the early implementation of the policy to react more strongly; analysts react to the extent of a weaker, although it will be with the subsidy growth, but the effect of the implementation of the implementation is not obvious. Analysts compared to investors more professional financial background, for strategy selection will tend to be more cautious.
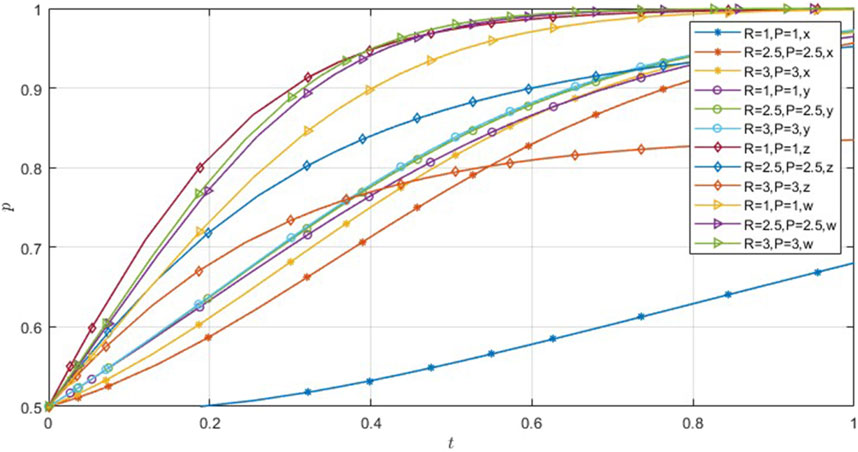
Figure 6. Sensitivity analysis of the coefficient of correlation between government incentives and subsidies.
4.3.3 Strategy cost impact
Let CE∈{1,6,14}, CA∈{1,5,11}, and CI∈{1,6,14}, respectively.
From Figure 7a, it can be seen that digital intelligence disclosure cost changes have a significant impact on firms and governments, with analyst and investor responses only becoming significant as time passes. The higher the CE at this time, the firms quickly choose information concealment, and more responsive in the early stages; analysts tend to make traditional decisions, the government takes strong regulation, and investors choose not to invest. In this process, the government, as a regulatory role, will strengthen regulation in order to maintain market stability. Analysts and investors are in an information-weak position and they will be more cautious to protect their own interests.
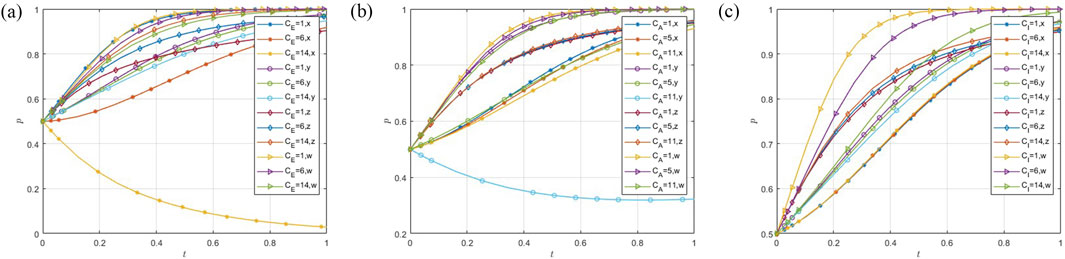
Figure 7. (a–c) Analysis of the correlation coefficients of the evolution of strategy costs after the respective changes of enterprises, analysts and investors.
As can be seen from Figure 7b, the intelligent decision-making cost changes have a large impact on firms, analysts, and investors, and a weak impact on governments. When CA is higher, firms choose information concealment, analysts quickly choose traditional decision-making, and investors tend not to invest. In this process, the increase of analysts’ decision-making cost means that analysts’ attention is reduced, and firms will choose information concealment out of speculation. The change itself does not cause excessive market turbulence, so the government’s reaction to it is weak. Overall, none of the four subjects had a significant response in the early period and a significant response in the middle period.
Next, from Figure 7c, it can be found that changes in investors’ upfront input costs have a significant effect on governments, investors and analysts, and a very weak effect on firms. When the CI is higher, the government chooses to strongly regulate and investors quickly choose not to invest. In this process, the government’s concern about investor tendency also implies concern about capital flow, and regulation will be strengthened to avoid market disorder. And there is a two-way feedback mechanism between analysts and investors, when the market information environment is poor resulting in low investor willingness to invest, it will make the analysts do not want to spend time and energy and cost of information processing.
5 Conclusion and recommendations
5.1 Main conclusions
The study presents a four-party evolutionary game model involving digital intelligence enterprises, financial data analysts, governments emphasizing new quality productivity, and fintech users. It explores stabilization strategies across various scenarios and utilizes simulations to assess how initial values and parameter adjustments influence the system. The analysis reveals four conditional stabilization points within the four-way evolutionary game, indicating strategic equilibriums attainable by stakeholders adhering to competitive neutrality. It fosters sustainable economic development by enhancing its economic efficiency and facilitating data sharing through the establishment of a symbiotic evolutionary framework termed the “regulation-market” model. This model encourages market competition and stimulates innovation.
Numerical simulations reveal that a proactive government, focused on enhancing the quality and productivity of enterprises, intensifies regulatory measures, compelling firms to disclose information. Conversely, when numerical Intelligence-driven enterprises opt for information disclosure, governmental oversight is relaxed. The dynamic underscores the government’s pivotal role in supervising corporate disclosure processes, prompting firms to elevate their transparency levels in response to external pressures. Consequently, reduced information asymmetry and heightened transparency empower financial analysts and fintech users in information aggregation and structuring, thereby bolstering market stability. This strategic evolution aligns with the paradigm of “information disclosure, intelligent decision-making, stringent regulation, and investment.
5.2 Strategy recommendations
Based on the above research results, the article carries out the following recommendations:
Digitally intelligent enterprises must prioritize enhanced social responsibility and information transparency to align with the evolving landscape of enterprise digital transformation. By leveraging this transformation, enterprises can gain a competitive edge in accessing information. It is imperative for enterprises to uphold their social responsibility by promptly disclosing precise and simplified financial information. Moreover, they should adapt their policies in accordance with legal requirements to ensure both compliance and strategic alignment. Engaging in ongoing dialogue with regulatory bodies is essential to address analysts’ inquiries and investors’ preferences effectively. Ultimately, enterprises should strive to enhance their economic performance while fostering sustainable economic growth.
Financial data analysts should enhance data sensitivity and utilization efficiency to better influence business operations and investors. To achieve this, analysts must elevate their proficiency in financial technology, enhance decision-making through digitalization, and information transformation. By consistently monitoring and conducting multi-dimensional analyses of digital intelligence data, analysts can generate more precise secondary information.
New quality productivity-based government should enhance the efficacy, and it is imperative to bolster its digitalization and oversight capacities. Given the inherent regulatory nature of governments, the integration of the new quality productivity paradigm necessitates staying abreast of contemporary trends. The government ought to proactively adopt cutting-edge tools to align with evolving market dynamics. By leveraging advanced information gathering and processing capabilities, the government can more effectively oversee the digital reporting practices of enterprises. Concurrently, it should refine legal and institutional frameworks to augment the transparency and expediency of information dissemination. Subsequently, sustained enhancements to the feedback mechanisms are essential to fortify monitoring and service capabilities.
Fintech users must enhance their professionalism and discernment across various sectors. Investors, in particular, often find themselves at a disadvantage due to their position in the information disclosure chain. Consequently, they need to improve their capacity to recognize, gather, and analyze information. Embracing digitalization trends and leveraging technological tools can help investors mitigate blind conformity, leading to enhanced investment yields.
Data availability statement
The raw data supporting the conclusions of this article will be made available by the authors, without undue reservation.
Author contributions
JY: Conceptualization, Funding acquisition, Resources, Supervision, Validation, Writing – review and editing. YS: Conceptualization, Data curation, Investigation, Methodology, Software, Writing – original draft.
Funding
The author(s) declare that financial support was received for the research and/or publication of this article. This work was supported by the National Social Science Fund Youth Project of China (Grant 21CJY040).
Conflict of interest
The authors declare that the research was conducted in the absence of any commercial or financial relationships that could be construed as a potential conflict of interest.
Generative AI statement
The author(s) declare that no Generative AI was used in the creation of this manuscript.
Publisher’s note
All claims expressed in this article are solely those of the authors and do not necessarily represent those of their affiliated organizations, or those of the publisher, the editors and the reviewers. Any product that may be evaluated in this article, or claim that may be made by its manufacturer, is not guaranteed or endorsed by the publisher.
References
1. Martínez-Gutiérrez A, Díez-González J, Verde P, Perez H. Convergence of virtual reality and digital twin technologies to enhance digital operators’ training in industry 4.0. INTERNATIONAL JOURNAL HUMAN-COMPUTER STUDIES (2023) 180:103136. doi:10.1016/j.ijhcs.2023.103136
2. Hilbert M. The end justifies the definition: the manifold outlooks on the digital divide and their practical usefulness for policy-making. TELECOMMUNICATIONS POLICY (2011) 35(8):715–36. doi:10.1016/j.telpol.2011.06.012
3. Wang XD, Deng YF, Mao XM. The impact of bank digital transformation on enterprises digital technology innovation in China. Int Rev Financial Anal (2025) 102:104068. doi:10.1016/j.irfa.2025.104068
4. Petzolt S, Seckler C. The human side of digital transformation: a tool for identifying innovation promotors. TECHNOVATION (2025) 140:103161. doi:10.1016/j.technovation.2024.103161
5. Singh K, Chaudhuri R, Chatterjee S. Assessing the impact of digital transformation on green supply chain for achieving carbon neutrality and accelerating circular economy initiatives. COMPUTERS and INDUSTRIAL ENGINEERING (2025) 201:110943. doi:10.1016/j.cie.2025.110943
6. Rehman SU, Ness S, Kucinskas G. WITHDRAWN: digital transformation and schumpeterian growth: inter-rationality in open innovation dynamics and finance. J Open Innovation: Technol Market, Complexity (2025):100506. doi:10.1016/j.joitmc.2025.100506
7. Chotia V, Khoualdi K, Broccardo L, Yaqub MZ. The role of cyber security and digital transformation in gaining competitive advantage through strategic management accounting. Technology Soc (2025) 81:102851. doi:10.1016/j.techsoc.2025.102851
8. Jiang L, Li B, Zhang M. The impact of digital transformation on the efficiency of corporate resource allocation: internal mechanisms and external environment. TECHNOLOGICAL FORECASTING AND SOCIAL CHANGE (2025) 215:124107. doi:10.1016/j.techfore.2025.124107
9. Ajoudanian S, Aboutalebi HR. A capability maturity model for smart city process-aware digital transformation. J Urban Management (2025). doi:10.1016/j.jum.2025.03.001
10. Seidel S, Berente N, Baiyere A. Stability and change in digital transformation: a repertoire model of institutionally embedded technology affordances. Inf Organ (2025) 35(2):100564. doi:10.1016/j.infoandorg.2025.100564
11. Moreira LL, Pinto SS, Costa L, Araújo N. Evaluating digital transformation in small and medium enterprises using the alkire-foster method. Heliyon (2025) 11(2):e41838. doi:10.1016/j.heliyon.2025.e41838
12. Liu H, Song YX, Zhang L, Wang YH. Crowding-out or calling-out? The influence of mandatory industry-related firm-specific information disclosure on analyst reports. JOURNAL ACCOUNTING AND PUBLIC POLICY (2025) 49:107266. doi:10.1016/j.jaccpubpol.2024.107266
13. Stolowy H, Paugam L, Gendron Y. Competing for narrative authority in capital markets: activist short sellers vs. Financial analysts. Account Organizations Soc (2022) 100:101334. doi:10.1016/j.aos.2022.101334
14. Cronqvist H, Ladika T, Pazaj E, Sautner Z. Limited attention to detail in financial markets: evidence from reduced-form and structural estimation. JOURNAL FINANCIAL ECONOMICS (2024) 154:103811. doi:10.1016/j.jfineco.2024.103811
15. Zhang ZH, Li PX, Wang XY, Ran R, Wu WS. New energy policy and new quality productive forces: a quasi-natural experiment based on demonstration cities. Econ Anal Policy (2024) 84:1670–88. doi:10.1016/j.eap.2024.10.039
16. Xue RH, Chen JB. ESG performance and stability of new quality productivity forces: from perspective of China's modernization construction. Int Rev Econ and Finance (2025) 98:103911. doi:10.1016/j.iref.2025.103911
17. Shirish A, Srivastava SC, Panteli N, Shanahan JO. A knowledge-centric model for government-orchestrated digital transformation among the microbusiness sector. The J Strateg Inf Syst (2025) 34(1):101870. doi:10.1016/j.jsis.2024.101870
18. Xu K, Zhou M Changes in the role of government in the development of new quality productivity: logic and approach-an analytical framework of bipartite interaction. The truth (2024) (05):14–27+109.
19. Borrero-Domínguez C, Cortijo-Gallego V, Escobar-Rodríguez T. Digital transformation voluntary disclosure: insights from leading European companies. Int J Account Inf Syst (2024) 55:100711. doi:10.1016/j.accinf.2024.100711
20. He Y, Li JZ, Ren YH. Digital transformation and corporate ESG information disclosure herd effect. Finance Res Lett (2024) 65:105557. doi:10.1016/j.frl.2024.105557
21. Henide K. Voluntary disclosure and adverse selection: bayesian game theoretical inference for green bond labelling regimes. Int Rev Financial Anal (2022) 83:102248. doi:10.1016/j.irfa.2022.102248
22. De Villiers C, Dumay J, Farneti F, Jia J, Li ZT. Reprint of: does mandating corporate social and environmental disclosure improve social and environmental performance?: broad-based evidence regarding the effectiveness of directive 2014/95/Eu. The Br Account Rev (2025) 57:101558. doi:10.1016/j.bar.2025.101558
23. Donkor A, Appiagyei K, Tenakwah ES, Tenakwah EJ, Kwakye TO. Corporate governance and ESG disclosure in fintech firms: does culture matter? Sustainable Futures (2025) 9:100528. doi:10.1016/j.sftr.2025.100528
24. Calford EM. Uncertainty aversion in game theory: experimental evidence. JOURNAL ECONOMIC BEHAVIOR and ORGANIZATION (2020) 176:720–34. doi:10.1016/j.jebo.2020.06.011
25. Aumann R. Game theory-more than just games. Front Young Minds (2024) 12:1215124. doi:10.3389/frym.2023.1215124
26. Amiri-Pebdani S, Alinaghian M, Safarzadeh S. Time-of-Use pricing in an energy sustainable supply chain with government interventions: a game theory approach. ENERGY (2022) 255:124380. doi:10.1016/j.energy.2022.124380
27. Guan KL, Fu MT, Zhu HN. Opportunistic behaviour behind corporate digitalization disclosure: the moderating role of economic policy uncertainty. Finance Res Lett (2024) 66:105704. doi:10.1016/j.frl.2024.105704
28. Zhu CP, Su YX, Fan RG, Xu RH, Li B. Research on the optimal incentive and constraint mechanisms for corporate carbon information disclosure considering different market contexts: a network-based evolutionary game analysis. ENERGY ECONOMICS (2025) 142:108207. doi:10.1016/j.eneco.2025.108207
29. Zhao CH, Zhu WD. Strategic IPO timing of technology innovation-driven enterprises: a differential game analysis of market returns, disclosure costs, and industry dynamics. Finance Res Lett (2025) 72:106588. doi:10.1016/j.frl.2024.106588
30. Chang YC. The tripartite evolutionary game of enterprises' green production strategy with government supervision and people participation. JOURNAL ENVIRONMENTAL MANAGEMENT (2024) 370:122627. doi:10.1016/j.jenvman.2024.122627
31. Zhou WW, Shi Y, Zhao T, Cao XM, Li JL. Government regulation, horizontal coopetition, and low-carbon technology innovation: a tripartite evolutionary game analysis of government and homogeneous energy enterprises. ENERGY POLICY (2024) 184:113844. doi:10.1016/j.enpol.2023.113844
32. Ren SC, Cao YG, Du H, Rui SW. Transformation of enterprise patent market value base on the perspective of the four-party evolutionary game. J Syst Management (2024) 33(01):104–23. Available online at: https://link.cnki.net/urlid/31.1977.N.20230421.1100.006 (Accessed April 22, 2025).
33. Zhu YQ, Wu MY, Lu J. “Zero-sum game” or “win-win cooperation”: an analysis of the evolution effect of competition neutrality based on the participation of four parties. Front Phys (2024) 12:1429728. doi:10.3389/fphy.2024.1429728
34. Selten R. A note on evolutionarily stable strategies in asymmetric animal conflicts. Models Strateg Rationality (1988) 67–75. doi:10.1007/978-94-015-7774-8_3
Keywords: digital-intelligent transformation, information disclosure, financial data analyst, new quality productivity, government regulation, four-party evolutionary game
Citation: Yao J and Sun Y (2025) A study on the financial information disclosure mechanism of digital intelligence based on the participation of four parties. Front. Phys. 13:1603371. doi: 10.3389/fphy.2025.1603371
Received: 31 March 2025; Accepted: 28 April 2025;
Published: 16 May 2025.
Edited by:
Dun Han, Jiangsu University, ChinaReviewed by:
Ru Liang, Nanjing University, ChinaQi Suo, Qingdao University of Science and Technology, China
Copyright © 2025 Yao and Sun. This is an open-access article distributed under the terms of the Creative Commons Attribution License (CC BY). The use, distribution or reproduction in other forums is permitted, provided the original author(s) and the copyright owner(s) are credited and that the original publication in this journal is cited, in accordance with accepted academic practice. No use, distribution or reproduction is permitted which does not comply with these terms.
*Correspondence: Yinan Sun, l8335798326@163.com