- 1Department of Health and Human Performance, University of Tennessee at Chattanooga, Chattanooga, TN, United States
- 2Oslo Sports Trauma Research Center, Norwegian School of Sport Science, Oslo, Norway
- 3Orthopedics and Sports Medicine, Emory Healthcare, Atlanta, GA, United States
Standard clinical assessments of mild traumatic brain injury are inadequate to detect subtle abnormalities that can be revealed by sophisticated diagnostic technology. An association has been observed between sport-related concussion (SRC) and subsequent musculoskeletal injury, but the underlying neurophysiological mechanism is not currently understood. A cohort of 16 elite athletes (10 male, 6 female), which included nine individuals who reported a history of SRC (5 male, 4 female) that occurred between 4 months and 8 years earlier, volunteered to participate in a 12-session program for assessment and training of perceptual-motor efficiency. Performance metrics derived from single- and dual-task whole-body lateral and diagonal reactive movements to virtual reality targets in left and right directions were analyzed separately and combined in various ways to create composite representations of global function. Intra-individual variability across performance domains demonstrated very good SRC history classification accuracy for the earliest 3-session phase of the program (Reaction Time Dispersion AUC = 0.841; Deceleration Dispersion AUC = 0.810; Reaction Time Discrepancy AUC = 0.825, Deceleration Discrepancy AUC = 0.794). Good earliest phase discrimination was also found for Composite Asymmetry between left and right movement directions (AUC = 0.778) and Excursion Average distance beyond the minimal body displacement necessary for virtual target deactivation (AUC = 0.730). Sensitivity derived from Youden's Index for the 6 global factors ranged from 67 to 89% and an identical specificity value of 86% for all of them. Median values demonstrated substantial improvement from the first 3-session phase to the last 3-session phase for Composite Asymmetry and Excursion Average. The results suggest that a Composite Asymmetry value ≥ 0.15 and an Excursion Average value ≥ 7 m, provide reasonable qualitative approximations for clinical identification of suboptimal perceptual-motor performance. Despite acknowledged study limitations, the findings support a hypothesized relationship between whole-body reactive agility performance and functional connectivity among brain networks subserving sensory perception, cognitive decision-making, and motor execution. A complex systems approach appears to perform better than traditional data analysis methods for detection of subtle perceptual-motor impairment, which has the potential to advance both clinical management of SRC and training for performance enhancement.
Introduction
At all ages and levels of competitive sports, athletes are exposed to the potential for injuries that can result in performance impairment (Bahr, 2014), progressive disability (Maffulli et al., 2010), and reduced health-related quality of life (Filbay et al., 2019). Sport-related concussion (SRC) specifically refers to a single head impact that produces symptoms of a mild traumatic brain injury (mTBI), but the cumulative effects of multiple head impacts that do not elicit symptoms may ultimately produce similar long-term effects (Montenigro et al., 2017; Hirad et al., 2019; Hunter et al., 2019). Numerous recent studies have documented a substantial increase in musculoskeletal injury incidence following SRC occurrence (McPherson et al., 2018), but the neurophysiological mechanism that elevates such risk is not understood (Brown et al., 2015; Lynall et al., 2017; Howell et al., 2018; Buckley et al., 2020). Additionally, the likelihood for a subsequent SRC is great (Brett et al., 2018, 2020), and a history of multiple SRCs appears to further elevate risk for musculoskeletal injury (Houston et al., 2018; Harada et al., 2019). Current clinical guidelines for return to sport following SRC rely on self-reported symptom resolution (Baker and Cinelli, 2014; Harmon et al., 2019), but an asymptomatic neuroinflammatory response may persist for months or years beyond the point of return to normal activity (Ezza and Khadrawyb, 2014; Tremblay et al., 2014; Churchill et al., 2017; Shahim et al., 2017; Yanuck, 2019; Brett et al., 2020). Improved clinical assessment methods are needed to identify subtle alterations in brain function that could elevate risk for musculoskeletal injury, occurrence of a subsequent SRC, or development of a neurodegenerative disorder.
Relatively recent technological advances in neuroimaging and neurophysiological testing have generated a dramatic increase in understanding of neural information processing by brain networks (Bressler and Menon, 2010; van den Heuvel and Pol, 2010). Regardless of measurement method, temporal variability derived from a combination of excitatory and inhibitory neural signals appears to be an inherent property of brain function (Garrett et al., 2013). The term heterostasis refers to the phenomenon of variability in one neural system producing consistency in the output of a related system (Williams et al., 2016). Conversely, behavioral performance variability appears to result from unstable flow of electrochemical signals across damaged white matter tracts in the superior longitudinal fasciculus and corpus callosum (Fjell et al., 2011). Brain executive function is an inclusive term that refers to the set of neural processes that support goal-directed behaviors, which includes the core processes of working memory, inhibitory control, and cognitive flexibility (Diamond, 2013). Rapid and flexible responses to uncertainty in a changing environment reflects an ability to switch between brain states, which appears to depend on variability in global synchronization of neural activity (Garrett et al., 2013; Hellyer et al., 2015; Deco et al., 2017; Grady and Garrett, 2018).
Assessment of Perceptual-Motor Efficiency
Impaired information processing efficiency following SRC may be a clinically measurable factor that is highly relevant to future injury risk (Fino et al., 2017), as well as long-term brain health. The term neuromechanics has been defined as “the interaction between the nervous system and the mechanical properties of the body” (Enoka, 2002), and the term neuromechanical responsiveness has been used to refer to the ability to optimally integrate neurocognitive and neuromuscular processes to generate forces that will meet the demands of rapidly changing environmental challenges (Wilkerson et al., 2017, 2018, 2020). Separate brain networks have been identified that process information specific to visual attention, cognition, and motor functions in a somewhat independent manner, but the respective processes are integrated within specific areas of the thalamus and basal ganglia (Greene et al., 2020). Although sensory perception, cognitive decision-making, and motor execution are often viewed as distinct processes, references to perceptual-cognitive function (Wang et al., 2017; Hadlow et al., 2018; Wilke et al., 2020; Cardoso Fd et al., 2021) and cognitive-motor function (Brown et al., 2015; Hurtubise et al., 2016; Leone et al., 2017) reflect the high degree of interrelated neural processing that is necessary for successful performance of goal-directed actions. Perception involves awareness of sensory inputs and their inferred causes (Adams et al., 2013), and motor activation occurs before a decision-making process has been completed (Selen et al., 2012; Gallivan, 2014). Brain metastability refers to the capacity for rapid and flexible integration of information across large-scale networks, which may be differentially affected by the specific locations of microstructural damage induced by a traumatic brain injury (Hellyer et al., 2015). We use the term perceptual-motor efficiency to refer to optimal processing of neural information that can be quantified by neuromechanical responsiveness to environmental stimuli (Wilkerson et al., 2021a).
Reaction time (RT) provides a unique behavioral measure that has been directly related to connectivity between key brain networks (Erickson et al., 2005; Niogi S. et al., 2008; Mennes et al., 2011; Jilka et al., 2014; Churchill et al., 2021; Urban et al., 2021), which validates the use of RT as an index of brain information processing efficiency (Jensen, 2006; Marmolejo-Ramos et al., 2015). The nature of the presented stimulus and the required response for a given testing procedure can produce an extremely wide range of RT values among different populations, which can range from ~150 ms for initiation of a horizontal eye saccade in response to a visual stimulus among healthy young adults (Danna-Dos-Santos et al., 2018) to more than 800 ms for a stimulus-response compatibility button-press task among older adults (McAuley et al., 2006). Simple RT refers to speed of response to appearance of a stimulus that does not change, whereas Choice RT requires a decision about whether or not to respond to binary stimuli (i.e., go vs. no-go decision). Discrimination RT involves cognitive interpretation of complex stimuli that determine a correct vs. incorrect response, which prolongs the process of completing the proper response (Jensen, 2006). The Eriksen “flanker test” imposes a visual-cognitive processing demand for interference control that has been recognized as “one of the most important experimental tasks in the history of cognitive psychology” (Ridderinkhof et al., 2021). The task involves determination of the direction indicated by a central arrow that is flanked by pairs of arrows that are either congruent (i.e., <<<<< or >>>>>) or incongruent (<<><< or >><>>). The ability to restrain incorrect responses to the distraction imposed by incongruent flanking arrows provides a measure of executive function (Themanson and Rosen, 2015) that has been related to various aspects of brain structure and function with functional magnetic resonance imaging (Erickson et al., 2005; Fan et al., 2005; Kelly et al., 2008; Mennes et al., 2010, 2011; Zhu et al., 2010), diffusion tensor imaging (Niogi S. et al., 2008; Niogi S. N. et al., 2008; FitzGerald and Crosson, 2011; Fjell et al., 2011), and electroencephalography (De Beaumont et al., 2009; Pontifex et al., 2009; Moore et al., 2014, 2015; Parks et al., 2015; Themanson and Rosen, 2015; Wang et al., 2017; Guth et al., 2018).
Testing of RT has often been done as a single-task assessment that requires a relatively simple motor response (e.g., keyboard tap, mouse click, or button press), which appears to have limited value for identification of a performance deficiency following SRC (Urban et al., 2021). Functional RT assessment involves a whole-body response to visual or auditory stimulus during dynamic activities, such as walking gait, jump landing, single-leg hopping, anticipated cutting, and unanticipated cutting (Lempke et al., 2020; Lynall et al., 2021). Lack of strong correlation between functional RT measures with RT measures derived from a simple motor response in a stationary position suggests that the latter may have lesser relevance to performance of sport-specific movement patterns (Lempke et al., 2020). Whole-body reactive agility (WBRA) involves rapid changes of movement velocity or direction in response to a stimulus (Sheppard et al., 2006). If the nature of a test stimulus does not require a substantial amount of cognitive effort to produce a rapid whole-body movement response, a measure of RT derived from such a test could be described as a representation of WBRA Simple RT. Other neuromechanical performance measures derived from initiation of a stimulus response to whole-body displacement over a specified distance could include speed, acceleration and deceleration (Wilkerson et al., 2018, 2020, 2021a).
Dual-task assessment that requires decision-making during performance of whole-body movements may provide an optimal means to quantify subtle neuromechanical performance deficiencies (Baker and Cinelli, 2014; Brown et al., 2015; Leone et al., 2017; Howell et al., 2018; Kung et al., 2020; Urban et al., 2021). The imposition of simultaneous cognitive and motor demands of sufficient complexity may challenge finite neural resources beyond a point that allows compensatory mechanisms to sustain a given level of single-task performance. Dual-task WBRA can be decomposed into two somewhat distinct tasks: (1) stimulus perception and cognitive interpretation, and (2) generation of a rapid motor response that changes the velocity or direction of body movement (McGinnis et al., 2017). Because finite neural resources require selective prioritization of visual inputs that are most relevant to a behavioral goal (Buschman and Kastner, 2015), identification of a subtle impairment of perceptual-cognitive function requires a task that will challenge detection of salient stimuli (Churchill et al., 2021). The incongruent stimuli of the flanker test impose such a demand for focused attention, as well as a requirement for resolution of stimulus-response conflict that prolongs neural processing time (Moore et al., 2015; Servant and Logan, 2019). In terms of dual-task motor response, bradykinesia (i.e., slowing of movement) has been documented among individuals with a history of SRC (Ozolins et al., 2016; Fueger and Huddleston, 2018). Thus, a dual-task WBRA assessment that incorporates the flanker test appears to provide a good method for quantification of perceptual-motor efficiency (Supplementary Figure).
Potential for Improved Risk Screening and Individualized Interventions
Considering the differing specialized functions of the two brain hemispheres (Serrien et al., 2006; Takeuchi et al., 2012), along with documented microstructural disruption within the connecting white matter tracts following SRC (Niogi S. et al., 2008; Fjell et al., 2011; Womack et al., 2017; Yin et al., 2019), asymmetrical bradykinesia could be an indirect indicator of a subtle impairment of brain function. The majority of studies included in a recent systematic review of literature provided some amount of evidence that lower extremity asymmetry contributes to injury risk, which could be due to a constraint on an athlete's repertoire of movement options (Helme et al., 2021). Previous research that has utilized a Simple WBRA testing protocol has documented greater movement direction performance asymmetries among elite athletes who self-reported a history of SRC compared to those who denied ever having sustained such an injury (Wilkerson et al., 2018, 2020, 2021a). A key factor that differentiates WBRA asymmetry from prior limb performance asymmetry research is the simultaneous engagement of both extremities in generation of body displacements in a given direction (Wilkerson et al., 2021a). The collective research results derived from multiple small-scale projects suggest that poor perceptual-motor performance prospectively associates with musculoskeletal injury occurrences among college football players (Wilkerson et al., 2017), which raises a question about the potential for injury prevention through reduction of performance asymmetries (e.g., RT, speed, acceleration, or deceleration).
Appropriate screening test design, along with proper interpretation of results, offers the potential to identify individuals who would derive greatest benefit from targeted interventions that can reduce risk for a future adverse outcome (Hirad et al., 2019; Stern et al., 2020). However, some experts consider the majority of available evidence inadequate to support the use of sport injury risk screening results in making clinical decisions (Hegedus and Cook, 2015; Bahr, 2016). Other experts believe reductionist study designs and data analyses should be replaced with a complex systems approach for development of injury prevention strategies (Quatman et al., 2009; Bittencourt et al., 2016; Kenzie et al., 2017; Fonseca et al., 2020). A reductionist approach involves a search for isolated risk factors that cause injury (Bittencourt et al., 2016), whereas a complex systems approach involves a search for high-order variables that represent the collective function of multiple interacting system elements that lead to the emergence of injury susceptibility (Fonseca et al., 2020). For example, a Composite Asymmetry value derived from multiple WBRA performance metrics has been shown to provide better SRC history discrimination than any single asymmetry metric (Wilkerson et al., 2020, 2021a). Excursion (i.e., body displacement beyond that required to reach a target) represents a possible cumulative indicator of suboptimal sensorimotor integration. Other composite performance variables, such as a Global Index derived from multiple standardized test scores, may provide a sufficiently sensitive means to identify a subtle deficiency in neural processing efficiency (Roalf et al., 2016).
An extensive body of literature pertaining to change in cognitive performance attributable to the aging process and neurodegenerative conditions has documented greater value of intra-individual (i.e., within-person) variability measures than between-group comparisons of mean values for early detection of impairment (Costa et al., 2019). Intra-individual variability (IIV) is believed to reflect endogenous and exogenous influences on the efficiency of executive control processes, rather than being due to random error or lack of measurement reliability (MacDonald et al., 2009). Different types of IIV include the following: (1) Inconsistency, which can refer to either trial-to-trial variability during a single measurement session or variability in performance across measurement sessions conducted on different days (Holtzer et al., 2008; MacDonald et al., 2009; Costa et al., 2019), (2) Dispersion, which refers to variability in an individual's standardized scores across multiple performance domains (Holtzer et al., 2008; MacDonald et al., 2009; Roalf et al., 2016; Costa et al., 2019), and (3) Discrepancy, which refers to the difference between the maximum and minimum standardized scores from a set of measures representing multiple performance domains (Schretlen et al., 2003). Because neural processing efficiency may be affected by multiple transient factors that are difficult to control (e.g., motivation, fatigue, stress, emotions, or pain), measurements acquired over multiple days are likely to provide a better performance index than measurements acquired on a single occasion Furthermore, transient exogenous factors may have differential effect magnitudes on the neural processing efficiency of individuals who experience persisting adverse effects from a prior SRC.
Multicomponent training for improved movement control has been shown to reduce the incidence of both musculoskeletal injury and SRC among rugby players (Hislop et al., 2017; Attwood et al., 2018), and we have documented improvement of WBRA performance following upper extremity perceptual-motor training among elite athletes who self-reported a history of SRC (Wilkerson et al., 2021a). Thus, the overall goal of this study was to develop a method for accurate identification of athletes who may possess a subtle and potentially modifiable impairment in perceptual-motor processing efficiency, which might be used to guide training for simultaneously enhancement of sport performance capabilities and reduction of risk for future injury. Specifically, the purposes of this exploratory study included: (1) Assessment of the reliability of various WBRA performance metrics across the entire training period, (2) Assessment of the discriminatory power of each WBRA performance metric for identification of athletes who self-reported a history of SRC, (3) Identification of any differences in WBRA performance metrics between early and late phases of training, including any effect attributable to self-reported history of SRC, and (4) Comparison of the classification accuracy provided by Composite Asymmetry and Excursion values to that of Dispersion and Discrepancy values derived from different WBRA performance metrics.
Materials and Methods
A cohort of 16 elite athletes at a residential training center volunteered to participate in a 12-session WBRA training program (Table 1). Any athlete receiving treatment for any acute injury or illness was excluded from participation. The Institutional Review Board of the University of Tennessee at Chattanooga approved all study procedures. Surveys administered prior to initiation of the first training session included the Sports Fitness Index (Wilkerson et al., 2016), the Overall Wellness Index (Wilkerson et al., 2021b), and the Depression, Anxiety, and Stress Scale (Edmed and Sullivan, 2012), which were used to confirm greater frequency of self-reported problems among the athletes who affirmed a history of at least one SRC (HxSRC).
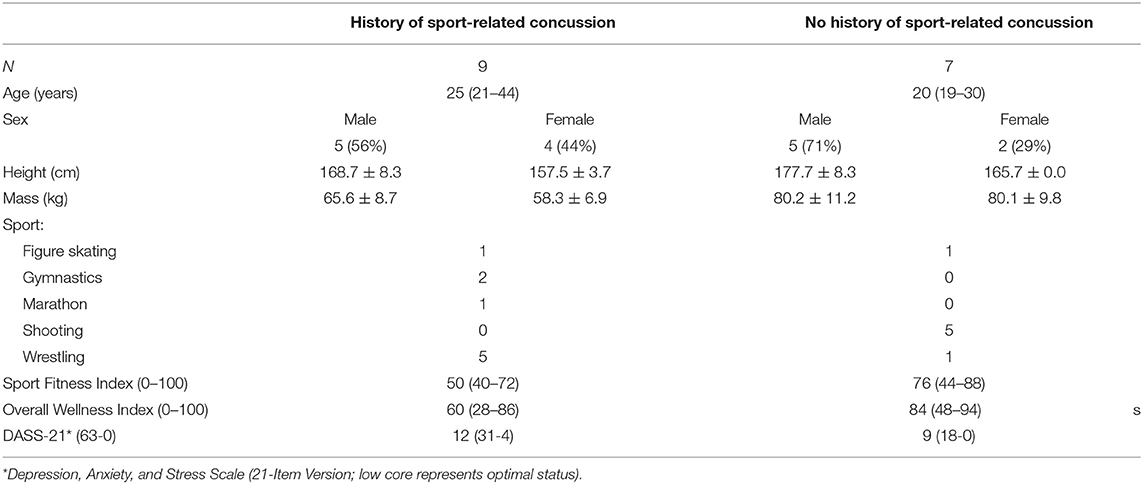
Table 1. Cohort characteristics; height and mass: mean ± standard deviation; age and survey scores: median (range).
Assessment and Training Procedures
Each athlete was provided with verbal instructions and the opportunity to perform each of 4 different WBRA task modes during a familiarization session that preceded the first training session. Each training session involved completion of 8 movement sequences (i.e., repetitions) for each of 4 WBRA task modes, which required a total of only 3–4 min per session. To accommodate differing schedules for sport-specific activities, the time intervals between sessions were not identical for each participant. Each athlete completed 2–4 WBRA training sessions per week for a total of 12 sessions that were each separated by a period of 24–72 h.
The WBRA task modes involved either Lateral or Diagonal whole-body movements in response to visual targets displayed on a 48 X 86 cm monitor located 1.83 m in front of the athlete. The system used to administer the task (TRAZER® Sports Stimulator, Traq Global Ltd; Westlake, OH) has been shown to provide good test-retest measurement reliability and a valid representation of movement precision in deactivation of virtual targets (Hogg et al., 2021). From a central starting position, each reactive movement in a left or right direction was performed with the goal to quickly deactivate a virtual target on the monitor by moving the body core to a corresponding 2-dimensional X-Y spatial coordinate within the performance space (Figure 1). Immediately after virtual target deactivation, the athlete reversed the movement direction to rapidly return to the original central position for the start of another trial. A single-task (ST) mode presented a single target on either the left side (4 repetitions) or right side (4 repetitions) of the monitor, which served as a simple visual cue for a reactive movement in the correct direction. Lateral movement, direction reversal, and return to the central position required a minimum body displacement of 1.83 m for each of 4 left and 4 right targets presented in a random order. Diagonal movement, direction reversal, and return to the central position required a minimum body displacement of 2.59 m for each of 4 back-left and 4 back-right targets presented in random order. A dual-task (DT) mode simultaneously presented targets on both the left and right sides of the monitor, with a central display of flanker test arrows in either a congruent configuration (4 repetitions) or incongruent configuration (4 repetitions) for 500 ms. The direction indicated by the center arrow of the flanker test display served as the cue for the movement direction in a randomly determined left or right direction, which added a cognitive challenge to the task (Supplementary Materials). This study used an early version of the DT mode, which required 25% greater lateral displacement and 13% greater diagonal displacement than the ST task mode.
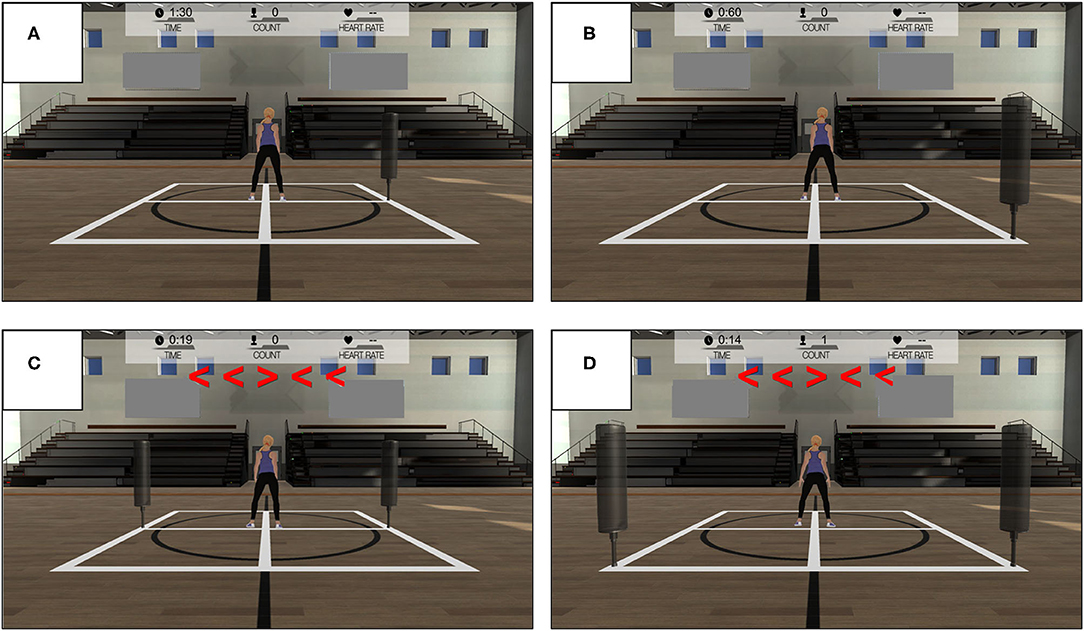
Figure 1. Whole-body reactive agility test modes: (A) Lateral Single-Task, (B) Diagonal Single-Task, (C) Lateral Dual-Task, and (D) Diagonal Dual-Task.
Performance Metrics
Reaction Time was defined as the average amount of time that elapsed between visual target appearance on the monitor and 20 cm of body core displacement in the correct movement direction for the 8 repetitions of the Lateral-ST and Diagonal-ST task modes. The Lateral-DT and Diagonal-DT task modes defined RT as the time that elapsed between the appearance of a flanker test arrow set on the monitor and 15 cm of body core displacement in the correct movement direction. Other WBRA performance metrics, including Speed, Acceleration, and Deceleration, were derived from the average of body core displacement values for each movement sequence (i.e., central starting position to target and back to central starting position). With the exception of Excursion (i.e., total distance covered in excess of the minimum distance required to deactivate 8 targets for a given task mode), the motion analysis system provided average performance values for left vs. right movement directions. Asymmetry for RT, Speed, Acceleration, and Deceleration was defined as the ratio of the absolute difference between performance values for the two directions to the better of the two performance values.
Data Analyses
Assessment of any group differences in survey scores utilized non-parametric Mann-Whitney tests. Analysis of the WBRA data was initially focused on basic time and distance performance metrics derived from each of the four task modes. Procedures corresponding to the 4 purposes of the study were as follows:
Measurement Reliability
The intraclass correlation coefficient (ICC) was calculated using a 2-way mixed-effects and average of measures (3, K) method for consistency across the 12 training sessions. Reliability values were calculated separately for left and right movement directions, the Average of left and right movement directions, and Asymmetry in left and right movement directions. Qualitative interpretation of ICC3,K values were as follows: <0.50 poor; 0.50–0.74 moderate; 0.75–0.89 good; ≥0.90 excellent (Lynall et al., 2021).
Discriminatory Power
Classification accuracy for differentiation of HxSRC vs. no self-report of prior SRC (NoSRC) was represented by a receiver operating characteristic area under curve (AUC) value for each of 4 phases of the 12-session training program (i.e., Phase 1: sessions 1–3; Phase 2: sessions 4–6; Phase 3: sessions 7–9; Phase 4: sessions 10–12). Qualitative interpretation of the AUC value derived from the 3-session average for a given performance metric were as follows: <0.60 poor discrimination; 0.60–0.74 possibly helpful discrimination; ≥0.75 clearly useful discrimination (Hosmer and Lemeshow, 2000; Alba et al., 2017).
Pre- to Post-training Change
Parametric analysis of the WBRA data used repeated measures analysis of variance (ANOVA) to assess difference between Phase 1 and Phase 4, difference between Groups (i.e., HxSRC and NoSRC), and Phase X Group interaction for each performance metric, as well as Asymmetry for each one that provided direction-specific data. The distribution normality of each variable was assessed by the Shapiro-Wilk test, with P < 0.05 deemed non-normal. In such cases, logarithmic, square root, and reciprocal transformations of the data were done to identify the best means to achieve a more normal distribution. Statistical significance was defined as P < 0.05 for all test results. Because of the study's exploratory purpose, no adjustment was made for multiple comparisons (Rothman, 1990; Cao and Zhang, 2014).
Comparisons of Derived Metrics
To assess IIV, each bilateral performance metric was converted into a standardized t-score (mean = 50; standard deviation = 10) for each athlete. Because a high value for RT represents poor performance, it was multiplied by −1 in the process of mathematical conversion to a t-score. Thus, a t-score > 50 represented superior performance for RT, Speed, Acceleration, and Deceleration. Dispersion was calculated as the standard deviation of 8 t-scores for a given performance metric and training session (i.e., 2 movement directions X 4 task modes). Discrepancy was calculated as the difference between the maximum and minimum t-scores for a given performance metric and training session. Both representations of IIV were averaged over the 3 consecutive sessions that defined a Phase of the training program. Because the motion analysis system did not provide data for each repetition of a given task mode, within-session inconsistency for the performance metrics could not be quantified. However, the Asymmetry metric does capture inconsistency of performance in left vs. right movement directions, which is derived from an average of 4 repetitions in either direction. Thus, Asymmetry for the 4 task modes was averaged over the 3 sessions of a given training Phase for comparison to Dispersion and Discrepancy values. Excursion average for the four task modes was also evaluated as a possible indicator of imprecise visuospatial guidance of whole-body movements.
To assess the discriminatory power of Dispersion, Discrepancy, Asymmetry, and Excursion for identification of HxSRC, and the relative value of each as a potential Global Index of perceptual-motor efficiency, receiver operating characteristic analysis was performed for each of the 4 bilateral performance metrics, a Composite Dispersion value that represented the standard deviation of 16 t-scores (i.e., 2 movement directions × 4 task modes × 4 metrics), a Composite Discrepancy value that represented the difference between the maximum and minimum of the 16 t-scores, a Composite Asymmetry value derived from the average for the 4 bilateral performance metrics, and Excursion average for the 4 test modes. Results that yielded a Phase 1 AUC value > 0.75 were compared to Phase 4 results for the corresponding predictor to assess a possible differential influence of the training program on performance for HxSRC and NoSRC athletes. Logistic regression analysis was used to assess a possible effect of sex on the results for Phase 1 or Phase 4. Youden's Index was used to identify the Phase 1 cut point that provided maximum classification accuracy, which was compared to that for Phase 4. Global values that provided good discrimination were further assessed by non-parametric tests. The Wilcoxon Signed-Ranks test was used to assess Phase 1 to Phase 4 change and the Mann-Whitney test was used to identify any significant Group differences.
Results
Self-reported HxSRC at 3.0 ± 2.2 years prior to testing (range: 4 months−8 years) represented 56% of the cohort (9/16; 5 males, 4 females). The number of prior SRCs ranged from 1 to 3, with 44% (4/9) reporting a single SRC and 56% (5/9) reporting 2 or more SRCs. All 3 survey scores demonstrated poorer values for HxSRC athletes compared to NoSRC athletes, with the most significant difference found for the Sport Fitness Index (P = 0.031). Non-significant differences in survey scores were found for the Overall Wellness Index (P = 0.142) and the Depression, Anxiety, and Stress Survey (P = 0.408).
The majority of WBRA performance metrics demonstrated excellent reliability for each task mode, whereas Asymmetry ICC values were generally quite poor (Table 2; statistically significant results identified by bold font). Excursion for the Lateral-DT task mode was the only performance metric that demonstrated clearly useful Phase 1 discrimination, which was also potentially useful for the Diagonal-ST and Diagonal-DT task modes (Table 3; statistically significant results identified by bold font). Repeated measures ANOVA failed to identify any statistically significant effects for any performance metric derived from the Lateral-ST or Diagonal-ST task modes (Table 4). Conversely, use of the flanker test to specify the correct WBRA movement direction resulted in statistically significant change from Phase 1 to Phase 4 for 3 Lateral-DT performance metrics and all 5 of the performance metrics for the Diagonal-DT task mode (Table 5; statistically significant P-values identified by bold font). Excursion for the Lateral-DT task mode was the only performance metric that demonstrated a significant Phase X Group interaction effect.
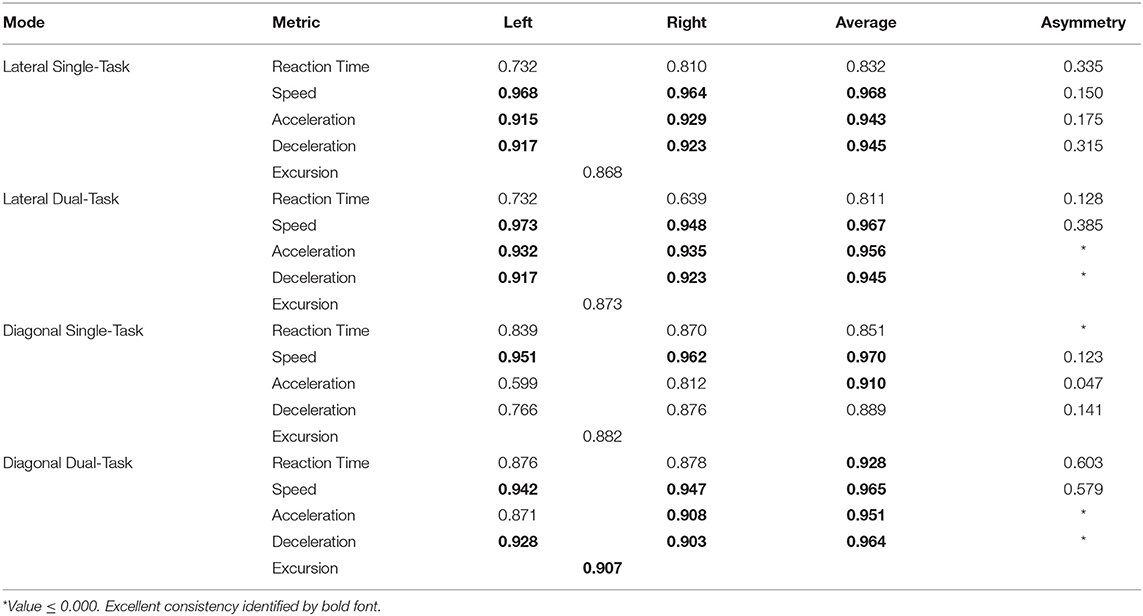
Table 2. Intraclass Correlation Coefficient (3, K) values for consistency of measurements across 12 training sessions.
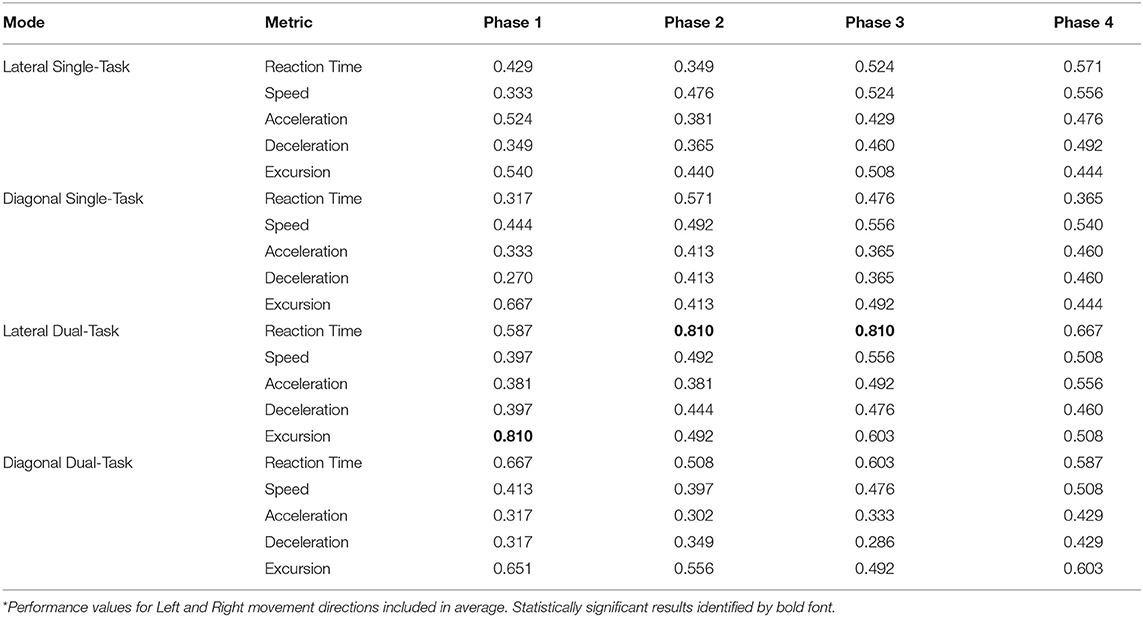
Table 3. Receiver operating characteristic area under curve values for Average* of performance metrics for each task mode.
Analyses of WBRA Asymmetry in left vs. right movement directions generally demonstrated better Phase 1 discrimination (Table 6; statistically significant P-values identified by bold font) and greater Phase 1 to Phase 4 change for the Composite metric than values specific to RT, Speed, Acceleration, and Deceleration (Tables 7, 8; statistically significant P-values identified by bold font). Asymmetry values were generally lower for both the HxSRC and NoSRC groups in Phase 4 compared to Phase 1. A Composite Asymmetry metric representing averaged values across the 4 task modes demonstrated clearly useful Phase 1 discrimination, but IIV measures (i.e., Dispersion and Discrepancy) demonstrated greater levels of Phase 1 discrimination for both RT and Deceleration (Table 9; statistically significant results identified by bold font). A graphic comparison of Phase 1 and Phase 4 discrimination for RT Dispersion and Deceleration Dispersion demonstrates lesser Phase 4 discrimination and poorer Phase 4 values at the same sensitivity level defining optimal Phase 1 discrimination (Figure 2). Similar results were found for RT Discrepancy and Deceleration Discrepancy (Figure 3), as well as Composite Asymmetry and Excursion 4-Mode Average (Figure 4). Despite Composite Asymmetry having demonstrated substantial Phase 4 discrimination, the Youden's index value providing an optimal balance of sensitivity and specificity was substantially smaller than the corresponding Phase 1 value. Sex did not demonstrate a significant effect on any Global Index association with HxSRC. Analysis of median values demonstrated greatest Phase 1 differences between the HxSRC and NoSRC groups for the RT and Deceleration IIV measures (Table 10; statistically significant P-values identified by bold font). Greatest Phase 1 to Phase 4 change in median values was evident for Composite Asymmetry and Excursion 4-Mode Average.
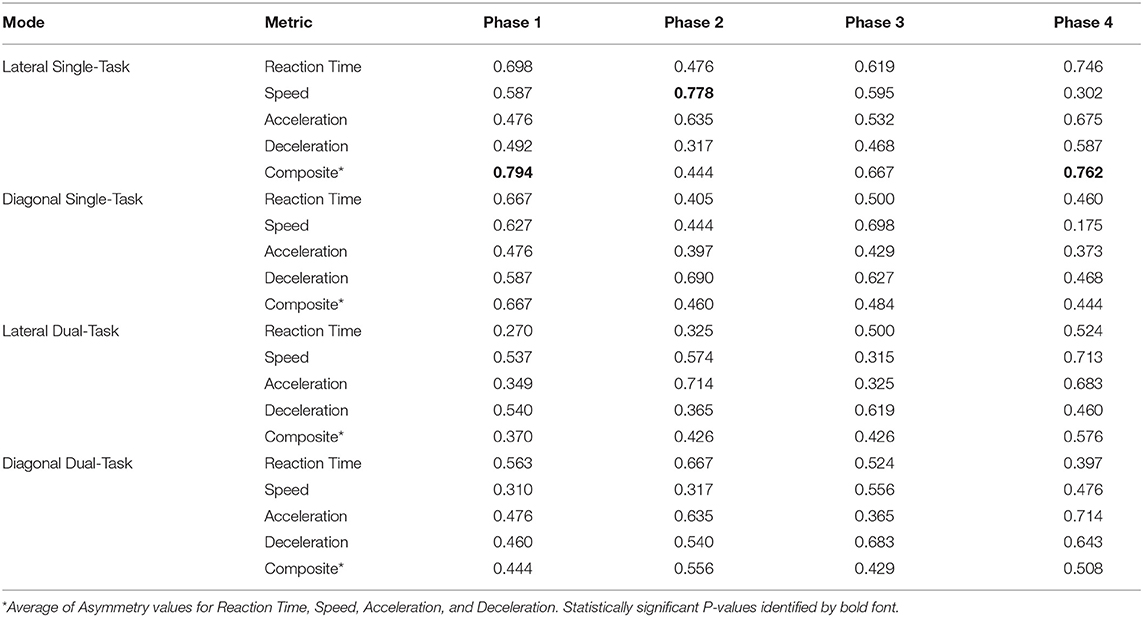
Table 6. Receiver operating characteristic area under curve values for Asymmetry of Left and Right performance metrics for each task mode.
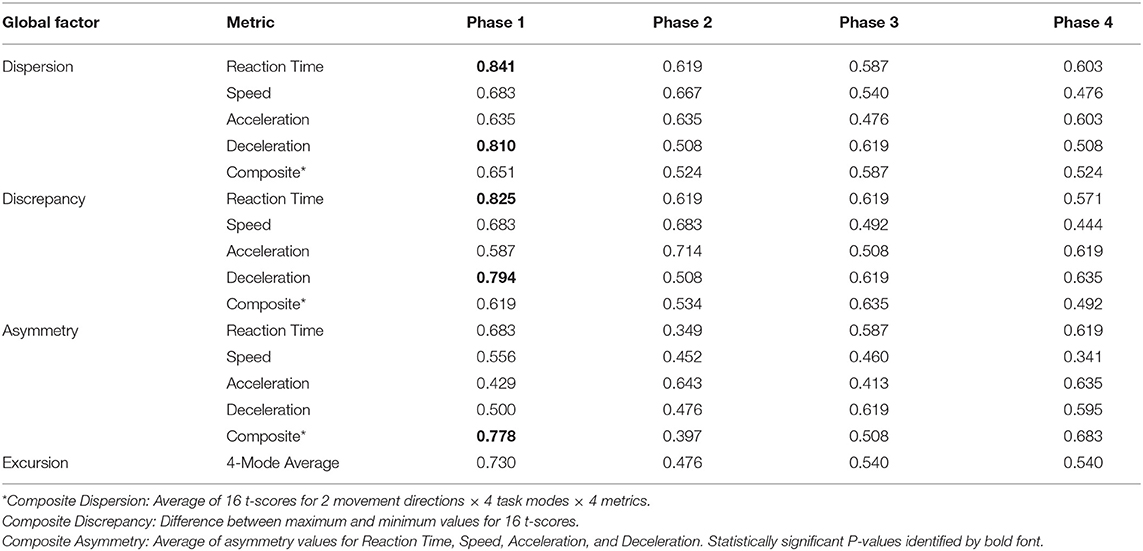
Table 9. Receiver operating characteristic area under curve values for intra-individual variability measures (Dispersion and Discrepancy of t-scores for 2 movement directions and 4 task modes), Asymmetry (differences between movement directions averaged for task modes), and Excursion (distances averaged for task modes).
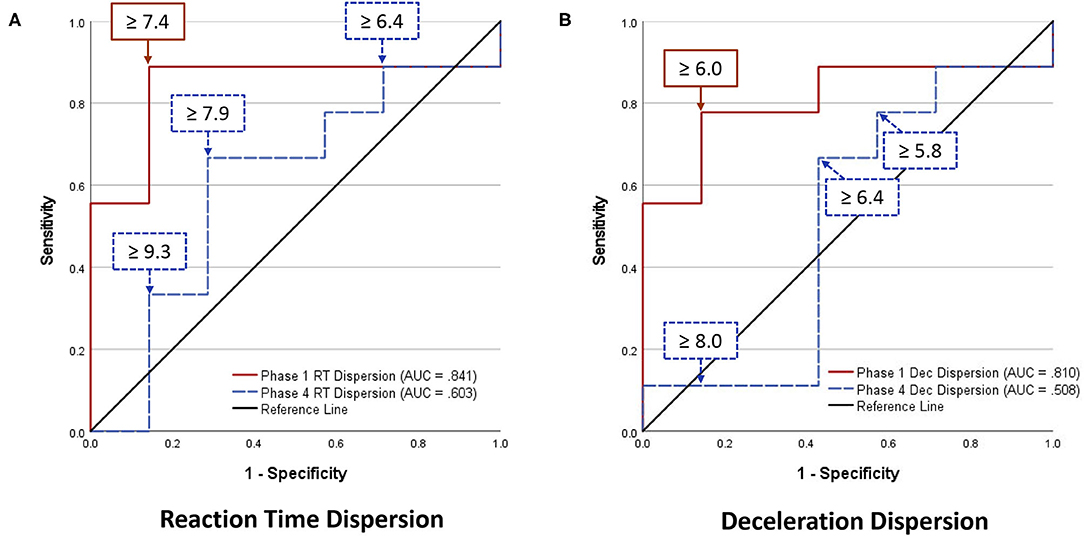
Figure 2. Receiver operating characteristic curves identifying History of Sport-Related Concussion cases in early phase (red solid line; sessions 1–3) and late phase (blue dashed line; sessions 10–12) of 12-session whole-body reactive agility training program on the basis of Dispersion (standard deviation of t-scores for 2 movement directions and 4 task modes): (A) Reaction Time Dispersion, (B) Deceleration Dispersion.
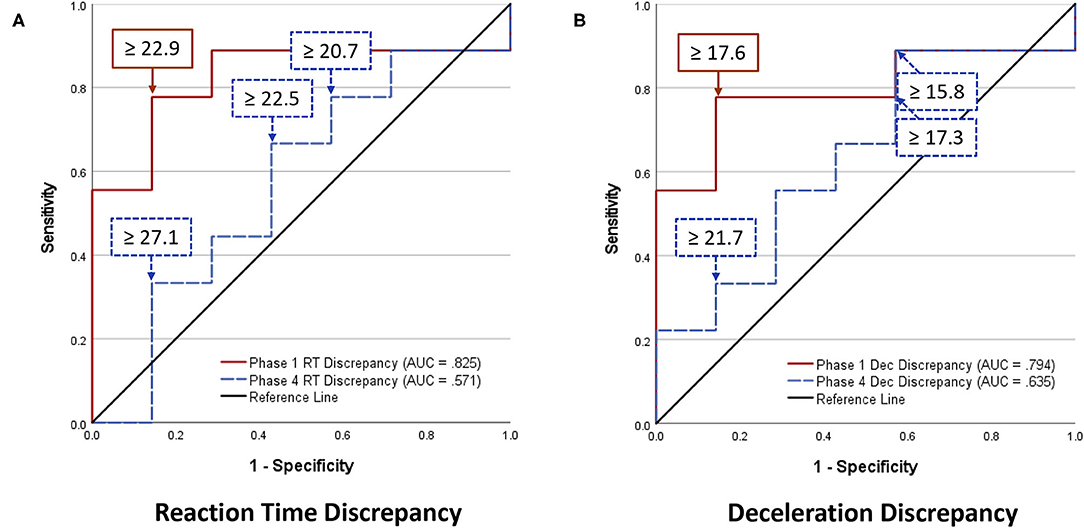
Figure 3. Receiver operating characteristic curves identifying History of Sport-Related Concussion cases in early phase (red solid line; sessions 1–3) and late phase (blue dashed line; sessions 10–12) of 12-session whole-body reactive agility training program on the basis of Discrepancy (maximum–minimum difference) among t-scores for 2 movement directions and 4 task modes: (A) Reaction Time Discrepancy, (B) Deceleration Discrepancy.
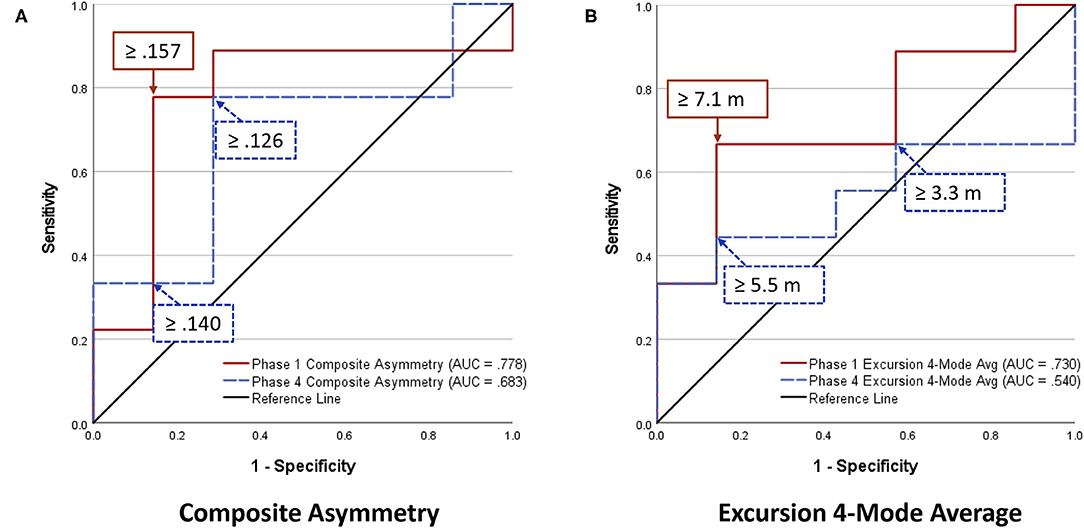
Figure 4. Receiver operating characteristic curves identifying History of Sport-Related Concussion cases in early phase (red solid line; sessions 1–3) and late phase (blue dashed line; sessions 10–12) of 12-session whole-body reactive agility training program: (A) Composite Asymmetry (average for 4 task modes and 4 performance metrics), (B) Excursion (average for 4 task modes).
Discussion
Intra-Individual Variability Association With History of Sport-Related Concussion
Dispersion of standardized scores representing different aspects of neurocognition has been used as a Global Index of executive function, which has identified subtle early-stage alteration of neural processing among older adults diagnosed with mild cognitive impairment, dementia, and Alzheimer's disease (Holtzer et al., 2008; MacDonald et al., 2009; Roalf et al., 2016; Costa et al., 2019). Discrepancy between the largest and smallest standardized scores for various measures of cognitive function has been shown to increase with age (Schretlen et al., 2003), which is clearly associated with a decline of mental capabilities that varies among individuals (Wahl et al., 2021). Although utilization of such IIV analysis methods has been advocated for identification of subtle impairment of neural processing in different clinical populations (Costa et al., 2019), our study is the first to demonstrate the potential value of Dispersion and Discrepancy measures for assessment of perceptual-motor efficiency among elite athletes. RT Dispersion demonstrated the greatest HxSRC discriminatory power, with 89% sensitivity and 86% specificity. In terms of clinical utility, a major limitation is the need for normative data from an appropriate reference population to calculate an individual's standardized scores, as well as the need to calculate the standard deviation among them or the difference between largest and smallest values.
Because our Dispersion and Discrepancy measures incorporated neuromechanical performance metrics that were calculated separately for left and right movement directions, some amount of the discriminatory power of these IIV measures probably overlap substantially with that of the Composite Asymmetry metric. Although Excursion did not incorporate separate measures for left and right movement directions, the 4-Mode Average can be viewed as another Global Index of neuromechanical function. In fact, the Phase 1 Youden's Index identified the identical 86% specificity value for RT Dispersion, Deceleration Dispersion, RT Discrepancy, Deceleration Discrepancy, Composite Asymmetry, and Excursion 4-Mode Average. Conversion of Excursion distances (m) to standardized T-scores resulted in a Youden's Index cut point corresponding to an Excursion T-Score 4-Mode Average of 50, with the median for the entire cohort closely approximating a value of 50. The Excursion metric demonstrated good to excellent test-retest reliability for each of the task modes, whereas Asymmetry was highly inconsistent for almost all of the other WBRA performance metrics (Table 2). Despite poor test-retest reliability for Composite Asymmetry, Youden's Index corresponded to 78% sensitivity, which was substantially greater than the 67% sensitivity for Excursion 4-Mode Average. Furthermore, the Composite Asymmetry cut point of ≥0.159 identified by Youden's Index and the median value of 0.157 for the entire cohort strongly support the clinical relevance of a prior finding of Composite Asymmetry ≥0.15 as a potentially good standard for identification of persisting SRC effects on neuromechanical function (Wilkerson et al., 2020). Although clinical utilization of classification cut points for risk factors has been criticized for having poor generalizability (Bahr, 2016), composite values are believed to have greater stability than the individual measurements from which they were derived (Costa et al., 2019; Churchill et al., 2021).
Neural Basis for Inconsistency of Perceptual-Motor Performance
Inconsistency among successive RT measurements acquired within a relatively short time period represents the most commonly investigated type of IIV. A substantial body of literature identifies RT inconsistency (RTI) as a good indicator of inefficient neural processing, which is believed to primarily depend on attentional control (Jackson et al., 2012; Garrett et al., 2013; Johnson et al., 2015; Wawrzyniak et al., 2016; Grady and Garrett, 2018; Stawski et al., 2019a,b; Shen et al., 2020). The default mode network (DMN) is defined by spontaneous neural activity among widespread areas of the brain mid-line in the absence of external task engagement (Mennes et al., 2010, 2011). Detection of goal-relevant external stimuli by the salience network (SN) simultaneously suppresses DMN activity and activates the ventral attention network (VAN) and the dorsal attention network (DAN), both of which have bidirectional connections with areas in the thalamus, basal ganglia and brainstem that control the rate and locations of saccadic eye movements (Corbetta et al., 2008; Parr and Friston, 2017; Taghdiri et al., 2018). Concurrent SN activation of the executive control network (ECN), which overlaps dorsolateral prefrontal and superior parietal areas of the DAN, exhibits an inverse relationship to the level of DMN activation when attention is externally focused (Seeley et al., 2007; Kelly et al., 2008; Jilka et al., 2014). Key nodes of the SN include the anterior cingulate cortex and the anterior insula, which together play a central role in response inhibition, conflict resolution, and motor planning (Seeley et al., 2007; Kelly et al., 2008; Sridharan et al., 2008; Jilka et al., 2014; Johnson et al., 2015). Numerous studies of RTI have utilized the flanker test to provide a cognitive challenge that requires more extensive brain activation and network integration than simpler visual stimuli (Kelly et al., 2008; Mennes et al., 2010, 2011; Fjell et al., 2011; Parks et al., 2015; Williams et al., 2016; Wang et al., 2017; Olson et al., 2018; McGowan et al., 2019). Disrupted neural communication within and between the DMN, SN, VAN, DAN, and ECN has been documented among individuals who have sustained brain injury (He et al., 2007; Jilka et al., 2014; Churchill et al., 2021), which may slow information processing and cause lapses in attention (i.e., inattentional blindness) that perturb perception (Kenzie et al., 2017). Thus, RTI appears to offer a better index of top-down control of attention than an individual's average RT (Johnson et al., 2015).
Studies of RTI have almost exclusively defined the end of each measurement interval by a simple motor response from a seated position (e.g., keyboard tap, mouse click, button press), which requires minimal integration of visual attention and cognitive decision-making with sensorimotor processes. Although RTI may provide the best representation of inefficiency in the performance of extremely fast perceptual processes, Dispersion and Discrepancy values may nonetheless provide unique information about neural processes. Performance metrics like Speed, Acceleration, and Deceleration only have relevance in the context of goal-directed whole-body movements that closely replicate sport-related maneuvers. Combining a cognitive challenge with execution of a sufficiently complex motor activity can clearly reveal performance deficiencies that are not apparent when the respective tasks are done separately, which is believed to be due to finite neural processing resources (Leone et al., 2017). The cerebellum appears to be particularly important for efficient performance of simultaneous cognitive-motor activity, which broadens the volume of information processing that needs to be integrated among spatially separated brain areas (Wu et al., 2013). Additionally, WBRA movements presumably require complex interhemispheric interactions for coordination of bilateral muscle activation patterns (Serrien et al., 2006; Takeuchi et al., 2012; Davidson and Tremblay, 2016). Individuals differ in terms of the locations of neural circuit integration zones (Greene et al., 2020), and probably many other aspects of connectivity among brain networks as well, which makes some amount of IIV across differing assessments of interrelated performance capabilities highly likely (Schretlen et al., 2003; Holtzer et al., 2008; MacDonald et al., 2009; Roalf et al., 2016). Thus, small Dispersion and Discrepancy values may index optimal neural efficiency, whereas excessively large values may be useful clinical indicators of impaired network connectivity (Costa et al., 2019).
Traditional reductionistic study designs, combined with parametric data analyses that treat IIV as measurement error, have probably limited the ability of many prior investigations to detect subtle and heterogenous impairments that could explain the association of SRC with elevated musculoskeletal injury incidence (Quatman et al., 2009; Kenzie et al., 2017). Reductionism refers to an attempt to reduce a complex problem into components that can purportedly be studied separately for attainment of an understanding of the whole phenomenon (Quatman et al., 2009; Bittencourt et al., 2016), whereas a complex systems approach involves a search for high-order composite variables that represent the collective function of multiple interacting elements (Kenzie et al., 2017; Fonseca et al., 2020). Rather than seeking to identify isolated risk factors that may be a primary cause of subsequent injury, a complex systems approach involves identification of patterns among interacting factors that lead to the emergence of elevated injury susceptibility (Bittencourt et al., 2016). A single assessment (e.g., pre-participation physical examination) is incompatible with a complex systems approach, which requires data from multiple time points to obtain a better representation of global behavior (Fonseca et al., 2020). With regard to assessment of within-session IIV, the data derived from a single measurement session could be affected by factors that vary over time, such as motivation, fatigue, stress, emotions, or pain. Thus, a single measurement may reflect a temporary state, whereas an average of measurements obtained over multiple assessment sessions is more likely to represent a stable trait (Stawski et al., 2019a).
Limitations and Future Directions
A major limitation of this study was the small cohort size, which was based on the available number of elite athletes who were willing to voluntarily commit to completion of the training program. A related limitation was the small number of female participants (6/16), which may have influenced our finding of no sex influence on the discriminatory power of the composite predictor variables. The lack of a sex effect could have been due to use of relative, rather than absolute, performance metrics to create Global Index values. Although WBRA Asymmetry metrics exhibited poor test-retest reliability, the corresponding performance metrics for the left and right movements from which they were derived demonstrated good to excellent reliability. The inconsistency of Asymmetry values across training sessions might be an indicator of inefficient perceptual-motor processes, which might explain the strong association of the Composite Asymmetry metric with HxSRC. Our use of the same motion analysis system for assessment and training of perceptual-motor efficiency precluded investigation of a transfer of training effect to some other ecologically valid measurement of dynamic sport performance capability, and the limited availability of the participants precluded investigation of the extent to which benefits were retained beyond the end of the training period. Future research with a motion analysis system that will provide data for each successive task repetition should compare the discriminatory power of IIV Inconsistency to that provided by Dispersion and Discrepancy calculated from the same dataset. Because a minimum of 7 measurements are recommended for assessment of IIV (MacDonald et al., 2009), lack of separate body displacement measurements for left and right movement directions limited our analysis of averaged WBRA Excursion values for the 4 task modes. The possible relevance of Excursion to visuospatial calibration between egocentric and allocentric coordinates makes it another neuromechanical performance metric that may contribute to a high-order representation of perceptual-motor efficiency (van der Ham et al., 2014; van Andel et al., 2017). A future means to acquire separate measurements for congruent and incongruent trials of the flanker test used for the DT mode might provide further insights relevant to inhibitory control.
Despite a lack of neuroimaging or electrophysiological data that directly relate our WBRA results to neural correlates within the same individuals, a strong theoretical basis exists for the premise that the Dispersion, Discrepancy, Composite Asymmetry, and Excursion Average differences we demonstrated between HxSRC and NoSRC athletes may represent high-order evidence of impaired connectivity among brain networks. Reduced SN deactivation of the DMN among individuals who have sustained traumatic brain injury has been shown to adversely affect detection of salient stimuli and integrated neural processing of visuospatial information (Jilka et al., 2014; Antonakakis et al., 2016; Churchill et al., 2021). Our interpretations of the study results are based on 3 interrelated assumptions: (1) Self-reported HxSRC greatly increases the likelihood that microstructural damage to white matter tracts has had long-term adverse effects on perceptual-motor efficiency, (2) A Global Index derived from multiple WBRA task modes, performance metrics, and measurement sessions provides a better representation of perceptual-motor efficiency than traditional group-level central tendency statistics or any single performance metric derived from a single measurement session, and (3) Change in Global Index cut points and median values between early and late phases of a training program provide evidence of improvements in perceptual-motor efficiency that are probably attributable to improved functional connectivity within and between brain networks. To the extent that these assumptions are valid, our findings may have profound implications for clinical management of SRC and training for both injury risk reduction and enhancement of sport performance capabilities. More research is clearly needed to validate our findings in larger cohorts representing different populations, and to prospectively assess a possible effect of WBRA training on the incidence of sport-related injury.
The potential long-term consequences of SRC and repetitive “subconcussive” head impacts make early detection of any impairment of brain function an important consideration for protection of an athlete's performance capabilities and health-related quality of life (Hirad et al., 2019). However, the clinically silent nature of the underlying pathophysiological process, combined with the reluctance of some athletes to report persistent SRC symptoms, may result in an unrecognized vulnerability to repetitive injury and progressive dysfunction (Brett et al., 2020; Ledreux et al., 2020). The ability to rapidly generate appropriate responses to visual cues may facilitate collision avoidance and impact preparation, thereby preventing injury (Kung et al., 2020). Multi-modal neuromuscular training has been shown to reduce the incidence of both musculoskeletal injury and SRC among rugby players (Hislop et al., 2017; Attwood et al., 2018), and there is evidence from prior studies indicating that WBRA performance can be improved through training (Serpell et al., 2011; Wilkerson et al., 2021a). Furthermore, the findings of studies that have utilized the flanker test to assess the perceptual-cognitive skills of athletes suggest that long-term training for enhancement of sport-specific skills requiring both inhibitory control and rapid responses may induce beneficial neuroplastic adaptations (Wang et al., 2017; Wylie et al., 2018). Lacking any known adverse effects, our methods for assessment and training of perceptual-motor efficiency appear to offer a novel and potentially beneficial approach to injury risk reduction and performance enhancement.
Conclusions
Intra-individual variability represented as Dispersion among standardized neuromechanical performance metrics, as well as Discrepancy between maximum and minimum standardized scores, provided exceptionally good discrimination between elite athletes who either affirmed or denied a history of sport-related concussion. Composite Asymmetry and Excursion 4-Mode Average also provided good discrimination, both of which offer the advantage of easy calculation from performance metrics provided by the system used for assessment and training of perceptual-motor efficiency. Reaction time, deceleration, and precise control of whole-body movements directed to rapid deactivation of virtual reality targets appear to collectively differentiate elite athletes on the basis of whether or not a sport-related concussion has been sustained at any point in the past. Composite Asymmetry ≥0.15 and Excursion 4-Mode Average ≥ 7 m (or T-score Average <50) appear to be reasonable qualitative approximations for clinical identification of suboptimal perceptual-motor performance. Although more research is needed to document the potential value of whole-body perceptual-motor training for injury risk reduction, athlete participation in a relatively small number of brief training sessions resulted in substantial performance improvements. Our findings clearly support the potential for improved prevention and management of sport-related injuries through a complex systems approach that identifies high-order composite variables representing the collective function of multiple interacting perceptual and motor processes.
Data Availability Statement
The raw data supporting the conclusions of this article will be made available by the authors, without undue reservation.
Ethics Statement
The studies involving human participants were reviewed and approved by Institutional Review Board of the University of Tennessee at Chattanooga. The patients/participants provided their written informed consent to participate in this study.
Author Contributions
All authors made significant contributions to the conceptualization, design, and implementation of the study. The manuscript draft was written by GW, with the content of the final draft revised in response by the co-authors (DN and TP) and reviewers. All components of the data analysis were done by GW and reviewed by the co-authors to ensure that the results were derived from accurate data collected by TP under the direct supervision of DN.
Conflict of Interest
Whole-body reactive agility data were acquired from equipment loaned to the United States Olympic and Paralympic Committee by Traq Global Ltd (Westlake, OH).
The authors declare that the research was conducted in the absence of any commercial or financial relationships that could be construed as a potential conflict of interest.
Publisher's Note
All claims expressed in this article are solely those of the authors and do not necessarily represent those of their affiliated organizations, or those of the publisher, the editors and the reviewers. Any product that may be evaluated in this article, or claim that may be made by its manufacturer, is not guaranteed or endorsed by the publisher.
Supplementary Material
The Supplementary Material for this article can be found online at: https://www.frontiersin.org/articles/10.3389/fspor.2021.729729/full#supplementary-material
Supplementary Video can be downloaded through this link: https://youtu.be/RtHrslYOxtQ
References
Adams, R. A., Shipp, S., and Friston, K. J. (2013). Predictions not commands: active inference in the motor system. Brain Struct. Funct. 218, 611–643. doi: 10.1007/s00429-012-0475-5
Alba, A. C., Agoritsas, T., Walsh, M., Hanna, S., Iorio, A., Devereaux, P., et al. (2017). Discrimination and calibration of clinical prediction models: users' guides to the medical literature. JAMA 318, 1377–1384. doi: 10.1001/jama.2017.12126
Antonakakis, M., Dimitriadis, S. I., Zervakis, M., Micheloyannis, S., Rezaie, R., Babajani-Feremi, A., et al. (2016). Altered cross-frequency coupling in resting-state MEG after mild traumatic brain injury. Int. J. Psychophysiol. 102, 1–11. doi: 10.1016/j.ijpsycho.2016.02.002
Attwood, M. J., Roberts, S. P., Trewartha, G., England, M. E., and Stokes, K. A. (2018). Efficacy of a movement control injury prevention programme in adult men's community rugby union: a cluster randomised controlled trial. Br. J. Sports Med. 52, 368–374. doi: 10.1136/bjsports-2017-098005
Bahr, R. (2014). Demise of the fittest: are we destroying our biggest talents? 48, 1265–1267. doi: 10.1136/bjsports-2014-093832
Bahr, R. (2016). Why screening tests to predict injury do not work—and probably never will…: a critical review. Br. J. Sports Med. 50, 776–780. doi: 10.1136/bjsports-2016-096256
Baker, C. S., and Cinelli, M. E. (2014). Visuomotor deficits during locomotion in previously concussed athletes 30 or more days following return to play. Physiol. Rep. 2:e12252. doi: 10.14814/phy2.12252
Bittencourt, N., Meeuwisse, W., Mendonça, L., Nettel-Aguirre, A., Ocarino, J., and Fonseca, S. (2016). Complex systems approach for sports injuries: moving from risk factor identification to injury pattern recognition—narrative review and new concept. Br. J. Sports Med. 50, 1309–1314. doi: 10.1136/bjsports-2015-095850
Bressler, S. L., and Menon, V. (2010). Large-scale brain networks in cognition: emerging methods and principles. Trends Cogn. Sci. 14, 277–290. doi: 10.1016/j.tics.2010.04.004
Brett, B. L., Kuhn, A. W., Yengo-Kahn, A. M., Solomon, G. S., and Zuckerman, S. L. (2018). Risk factors associated with sustaining a sport-related concussion: an initial synthesis study of 12,320 student-athletes. Arch. Clin. Neuropsychol. 33, 984–992. doi: 10.1093/arclin/acy006
Brett, B. L., Wu, Y.-C., Mustafi, S. M., Saykin, A. J., Koch, K. M., Nencka, A. S., et al. (2020). The association between persistent white-matter abnormalities and repeat injury after sport-related concussion. Front. Neurol. 10:1345. doi: 10.3389/fneur.2019.01345
Brown, J. A., Dalecki, M., Hughes, C., Macpherson, A. K., and Sergio, L. E. (2015). Cognitive-motor integration deficits in young adult athletes following concussion. BMC Sports Sci. Med. Rehabil. 7:25. doi: 10.1186/s13102-015-0019-4
Buckley, T. A., Howard, C. M., Oldham, J. R., Lynall, R. C., Swanik, C. B., and Getchell, N. (2020). No clinical predictors of postconcussion musculoskeletal injury in college athletes. Med. Sci. Sports Exerc. 52, 1256–1262. doi: 10.1249/MSS.0000000000002269
Buschman, T. J., and Kastner, S. (2015). From behavior to neural dynamics: an integrated theory of attention. Neuron 88, 127–144. doi: 10.1016/j.neuron.2015.09.017
Cao, J., and Zhang, S. (2014). Multiple comparison procedures. JAMA 312, 543–544. doi: 10.1001/jama.2014.9440
Cardoso Fd, SL, Neves, J. A., Roca, A., and Teoldo, I. (2021). The association between perceptual-cognitive processes and response time in decision making in young soccer players. J. Sports Sci. 39, 926–935. doi: 10.1080/02640414.2020.1851901
Churchill, N. W., Hutchison, M. G., Graham, S. J., and Schweizer, T. A. (2021). Brain function associated with reaction time after sport-related concussion. Brain Imaging Behav. 15, 1508–1517. doi: 10.1007/s11682-020-00349-9
Churchill, N. W., Hutchison, M. G., Richards, D., Leung, G., Graham, S. J., and Schweizer, T. A. (2017). Neuroimaging of sport concussion: persistent alterations in brain structure and function at medical clearance. Sci. Rep. 7, 1–9. doi: 10.1038/s41598-017-07742-3
Corbetta, M., Patel, G., and Shulman, G. L. (2008). The reorienting system of the human brain: from environment to theory of mind. Neuron 58, 306–324. doi: 10.1016/j.neuron.2008.04.017
Costa, A. S., Dogan, I., Schulz, J. B., and Reetz, K. (2019). Going beyond the mean: intraindividual variability of cognitive performance in prodromal and early neurodegenerative disorders. Clin. Neuropsychol. 33, 369–389. doi: 10.1080/13854046.2018.1533587
Danna-Dos-Santos, A., Mohapatra, S., Santos, M., and Degani, A. M. (2018). Long-term effects of mild traumatic brain injuries to oculomotor tracking performances and reaction times to simple environmental stimuli. Sci. Rep. 8, 1–11. doi: 10.1038/s41598-018-22825-5
Davidson, T. W., and Tremblay, F. (2016). Evidence of alterations in transcallosal motor inhibition as a possible long-term consequence of concussions in sports: a transcranial magnetic stimulation study. Clin. Neurophysiol. 127, 3364–3375. doi: 10.1016/j.clinph.2016.07.012
De Beaumont, L., Theoret, H., Mongeon, D., Messier, J., Leclerc, S., Tremblay, S., et al. (2009). Brain function decline in healthy retired athletes who sustained their last sports concussion in early adulthood. Brain 132, 695–708. doi: 10.1093/brain/awn347
Deco, G., Kringelbach, M. L., Jirsa, V. K., and Ritter, P. (2017). The dynamics of resting fluctuations in the brain: metastability and its dynamical cortical core. Sci. Rep. 7, 1–14. doi: 10.1038/s41598-017-03073-5
Diamond, A. (2013). Executive functions. Ann. Rev. Psychol. 64, 135–168. doi: 10.1146/annurev-psych-113011-143750
Edmed, S., and Sullivan, K. (2012). Depression, anxiety, and stress as predictors of postconcussion-like symptoms in a non-clinical sample. Psychiatry Res. 200, 41–45. doi: 10.1016/j.psychres.2012.05.022
Erickson, K. I., Ho, M.-H. R., Colcombe, S. J., and Kramer, A. F. (2005). A structural equation modeling analysis of attentional control: an event-related fMRI study. Brain Res. Cogn. Brain Res. 22, 349–357. doi: 10.1016/j.cogbrainres.2004.09.004
Ezza, H., and Khadrawyb, Y. (2014). Glutamate excitotoxicity and neurodegeneration. J. Mol. Genet. Med. 8:141. doi: 10.4172/1747-0862.1000141
Fan, J., McCandliss, B. D., Fossella, J., Flombaum, J. I., and Posner, M. I. (2005). The activation of attentional networks. Neuroimage 26, 471–479. doi: 10.1016/j.neuroimage.2005.02.004
Filbay, S., Pandya, T., Thomas, B., McKay, C., Adams, J., and Arden, N. (2019). Quality of life and life satisfaction in former athletes: a systematic review and meta-analysis. Sports Med. 49, 1723–1738. doi: 10.1007/s40279-019-01163-0
Fino, P. C., Becker, L. N., Fino, N. F., Griesemer, B., Goforth, M., and Brolinson, P. G. (2017). Effects of recent concussion and injury history on instantaneous relative risk of lower extremity injury in Division I collegiate athletes. Clin. J. Sport Med. 29, 218–223. doi: 10.1097/JSM.0000000000000502
FitzGerald, D. B., and Crosson, B. A. (2011). Diffusion weighted imaging and neuropsychological correlates in adults with mild traumatic brain injury. Int. J. Psychophysiol. 82, 79–85. doi: 10.1016/j.ijpsycho.2011.02.011
Fjell, A. M., Westlye, L. T., Amlien, I. K., and Walhovd, K. B. (2011). Reduced white matter integrity is related to cognitive instability. J. Neurosci. 31, 18060–18072. doi: 10.1523/JNEUROSCI.4735-11.2011
Fonseca, S. T., Souza, T. R., Verhagen, E., Van Emmerik, R., Bittencourt, N. F., Mendonça, L. D., et al. (2020). Sports injury forecasting and complexity: a synergetic approach. Sports Med. 50, 1757–1770. doi: 10.1007/s40279-020-01326-4
Fueger, C., and Huddleston, W. E. (2018). Effects of concussions on visually guided motor actions: a literature review. J. Clin. Exp. Neuropsychol. 40, 1074–1080. doi: 10.1080/13803395.2018.1458823
Gallivan, J. P. (2014). A motor-oriented organization of human ventral visual cortex? J. Neurosci. 34, 3119–3121. doi: 10.1523/JNEUROSCI.0060-14.2014
Garrett, D. D., Samanez-Larkin, G. R., MacDonald, S. W., Lindenberger, U., McIntosh, A. R., and Grady, C. L. (2013). Moment-to-moment brain signal variability: a next frontier in human brain mapping? Neurosci. Biobehav. Rev. 37, 610–624. doi: 10.1016/j.neubiorev.2013.02.015
Grady, C. L., and Garrett, D. D. (2018). Brain signal variability is modulated as a function of internal and external demand in younger and older adults. Neuroimage 169, 510–523. doi: 10.1016/j.neuroimage.2017.12.031
Greene, D. J., Marek, S., Gordon, E. M., Siegel, J. S., Gratton, C., Laumann, T. O., et al. (2020). Integrative and network-specific connectivity of the basal ganglia and thalamus defined in individuals. Neuron 105, 742–758. doi: 10.1016/j.neuron.2019.11.012
Guth, T., Ketcham, C. J., and Hall, E. E. (2018). Influence of concussion history and genetics on event-related potentials in athletes: potential use in concussion management. Sports 6:5. doi: 10.3390/sports6010005
Hadlow, S. M. P. D., Mann, D. L., Portus, M. R., and Abernethy, B. (2018). Modified perceptual training in sport: a new classification framework. J. Sci. Med. Sport. 21, 950–958. doi: 10.1016/j.jsams.2018.01.011
Harada, G. K., Rugg, C. M., Arshi, A., Vail, J., and Hame, S. L. (2019). Multiple concussions increase odds and rate of lower extremity injury in National Collegiate Athletic Association athletes after return to play. Am. J. Sports Med. 47, 3256–3262. doi: 10.1177/0363546519872502
Harmon, K. G., Clugston, J. R., Dec, K., Hainline, B., Herring, S., Kane, S. F., et al. (2019). American Medical Society for Sports Medicine position statement on concussion in sport. Br. J. Sports Med. 53, 213–225. doi: 10.1136/bjsports-2018-100338
He, B. J., Snyder, A. Z., Vincent, J. L., Epstein, A., Shulman, G. L., and Corbetta, M. (2007). Breakdown of functional connectivity in frontoparietal networks underlies behavioral deficits in spatial neglect. Neuron 53, 905–918. doi: 10.1016/j.neuron.2007.02.013
Hegedus, E. J., and Cook, C. E. (2015). Return to play and physical performance tests: evidence-based, rough guess or charade? Br. J. Sports Med. 49, 1288–1289. doi: 10.1136/bjsports-2015-094796
Hellyer, P. J., Scott, G., Shanahan, M., Sharp, D. J., and Leech, R. (2015). Cognitive flexibility through metastable neural dynamics is disrupted by damage to the structural connectome. J. Neurosci. 35, 9050–9063. doi: 10.1523/JNEUROSCI.4648-14.2015
Helme, M., Tee, J., Emmonds, S., and Low, C. (2021). Does lower-limb asymmetry increase injury risk in sport? A systematic review. Phys. Ther. Sport. 49, 204–213. doi: 10.1016/j.ptsp.2021.03.001
Hirad, A. A., Bazarian, J. J., Merchant-Borna, K., Garcea, F. E., Heilbronner, S., Paul, D., et al. (2019). A common neural signature of brain injury in concussion and subconcussion. Sci. Adv. 5:eaau3460. doi: 10.1126/sciadv.aau3460
Hislop, M. D., Stokes, K. A., Williams, S., McKay, C. D., England, M. E., Kemp, S. P., et al. (2017). Reducing musculoskeletal injury and concussion risk in schoolboy rugby players with a pre-activity movement control exercise programme: a cluster randomised controlled trial. Br. J. Sports Med. 51, 1140–1146. doi: 10.1136/bjsports-2016-097434
Hogg, J. A., Carlson, L. M., Rogers, A., Briles, M. W., Acocello, S. N., and Wilkerson, G. B. (2021). Reliability and concurrent validity of TRAZER compared to three-dimensional motion capture. J. Clin. Transl. Res. 7, 100–107. doi: 10.18053/jctres.07.202101.013
Holtzer, R., Verghese, J., Wang, C., Hall, C. B., and Lipton, R. B. (2008). Within-person across-neuropsychological test variability and incident dementia. JAMA 300, 823–830. doi: 10.1001/jama.300.7.823
Hosmer, D. W., and Lemeshow, S. (2000). Applied Logistic Regression, 2nd Edn. New York, NY: John Wiley & Sons.
Houston, M. N., Hoch, J. M., Cameron, K. L., Abt, J. P., Peck, K. Y., and Hoch, M. C. (2018). Sex and number of concussions influence the association between concussion and musculoskeletal injury history in collegiate athletes. Brain Inj. 32, 1353–1358. doi: 10.1080/02699052.2018.1512718
Howell, D. R., Lynall, R. C., Buckley, T. A., and Herman, D. C. (2018). Neuromuscular control deficits and the risk of subsequent injury after a concussion: a scoping review. Sports Med. 48, 1097–1115. doi: 10.1007/s40279-018-0871-y
Hunter, L. E., Branch, C. A., and Lipton, M. L. (2019). The neurobiological effects of repetitive head impacts in collision sports. Neurobiol. Dis. 123, 122–126. doi: 10.1016/j.nbd.2018.06.016
Hurtubise, J., Gorbet, D., Hamandi, Y., Macpherson, A., and Sergio, L. (2016). The effect of concussion history on cognitive-motor integration in elite hockey players. Concussion 1:CNC17. doi: 10.2217/cnc-2016-0006
Jackson, J. D., Balota, D. A., Duchek, J. M., and Head, D. (2012). White matter integrity and reaction time intraindividual variability in healthy aging and early-stage Alzheimer disease. Neuropsychologia 50, 357–366. doi: 10.1016/j.neuropsychologia.2011.11.024
Jensen, A. R., (ed.). (2006). “Reaction time as a function of experimental conditions,” in Clocking the Mind: Mental Chronometry and Individual Differences (Amsterdam: Elsevier), 43–54.
Jilka, S. R., Scott, G., Ham, T., Pickering, A., Bonnelle, V., Braga, R. M., et al. (2014). Damage to the salience network and interactions with the default mode network. J. Neurosci. 34, 10798–10807. doi: 10.1523/JNEUROSCI.0518-14.2014
Johnson, B. P., Pinar, A., Fornito, A., Nandam, L. S., Hester, R., and Bellgrove, M. A. (2015). Left anterior cingulate activity predicts intra-individual reaction time variability in healthy adults. Neuropsychologia 72, 22–26. doi: 10.1016/j.neuropsychologia.2015.03.015
Kelly, A. C., Uddin, L. Q., Biswal, B. B., Castellanos, F. X., and Milham, M. P. (2008). Competition between functional brain networks mediates behavioral variability. Neuroimage 39, 527–537. doi: 10.1016/j.neuroimage.2007.08.008
Kenzie, E. S., Parks, E. L., Bigler, E. D., Lim, M. M., Chesnutt, J. C., and Wakeland, W. (2017). Concussion as a multi-scale complex system: an interdisciplinary synthesis of current knowledge. Front. Neurol. 8. doi: 10.3389/fneur.2017.00513
Kung, S. M., Suksreephaisan, T. K., Perry, B. G., Palmer, B. R., and Page, R. A. (2020). The effects of anticipation and visual and sensory performance on concussion risk in sport: a review. Sports Med Open 6:54. doi: 10.1186/s40798-020-00283-6
Ledreux, A., Pryhoda, M. K., Gorgens, K., Shelburne, K., Gilmore, A., Linseman, D. A., et al. (2020). Assessment of long-term effects of sports-related concussions: biological mechanisms and exosomal biomarkers. Front. Neurosci. 14:761. doi: 10.3389/fnins.2020.00761
Lempke, L. B., Johnson, R. S., Schmidt, J. D., and Lynall, R. C. (2020). Clinical versus functional reaction time: implications for postconcussion management. Med. Sci. Sports Exerc. 52, 1650–1657. doi: 10.1249/MSS.0000000000002300
Leone, C., Feys, P., Moumdjian, L., D'Amico, E., Zappia, M., and Patti, F. (2017). Cognitive-motor dual-task interference: a systematic review of neural correlates. Neurosci. Biobehav. Rev. 75, 348–360. doi: 10.1016/j.neubiorev.2017.01.010
Lynall, R. C., Johnson, R. S., Lempke, L. B., and Schmidt, J. D. (2021). Test–retest reliability of a functional reaction time assessment battery [published online ahead of print May 5, 2021]. J. Sport Rehabil. 30, 1–15. doi: 10.1123/jsr.2021-0021
Lynall, R. C., Mauntel, T. C., Pohlig, R. T., Kerr, Z. Y., Dompier, T. P., Hall, E. E., et al. (2017). Lower extremity musculoskeletal injury risk after concussion recovery in high school athletes. J. Athl. Train. 52, 1028–1034. doi: 10.4085/1062-6050-52.11.22
MacDonald, S. W., Li, S.-C., and Bäckman, L. (2009). Neural underpinnings of within-person variability in cognitive functioning. Psychol. Aging. 24, 792–808. doi: 10.1037/a0017798
Maffulli, N., Longo, U. G., Gougoulias, N., Loppini, M., and Denaro, V. (2010). Long-term health outcomes of youth sports injuries. Br. J. Sports Med. 44, 21–25. doi: 10.1136/bjsm.2009.069526
Marmolejo-Ramos, F., Cousineau, D., Benites, L., and Maehara, R. (2015). On the efficacy of procedures to normalize Ex-Gaussian distributions. Front. Psychol. 5:1548. doi: 10.3389/fpsyg.2014.01548
McAuley, T., Yap, M., Christ, S. E., and White, D. A. (2006). Revisiting inhibitory control across the life span: insights from the ex-Gaussian distribution. Dev. Neuropsychol. 29, 447–458. doi: 10.1207/s15326942dn2903_4
McGinnis, R. S., Cain, S. M., Davidson, S. P., Vitali, R. V., McLean, S. G., and Perkins, N. C. (2017). Inertial sensor and cluster analysis for discriminating agility run technique and quantifying changes across load. Biomed. Signal Process. Control. 32, 150–156. doi: 10.1016/j.bspc.2016.10.013
McGowan, A. L., Bretzin, A. C., Savage, J. L., Petit, K. M., Covassin, T., and Pontifex, M. B. (2019). Acute and protracted disruptions to inhibitory control following sports-related concussion. Neuropsychologia 131, 223–232. doi: 10.1016/j.neuropsychologia.2019.05.026
McPherson, A. L., Nagai, T., Webster, K. E., and Hewett, T. E. (2018). Musculoskeletal injury risk after sport-related concussion: a systematic review and meta-analysis. Am. J. Sports Med. 47, 1754–1762. doi: 10.1177/0363546518785901
Mennes, M., Kelly, C., Zuo, X.-N., Di Martino, A., Biswal, B. B., Castellanos, F. X., et al. (2010). Inter-individual differences in resting-state functional connectivity predict task-induced BOLD activity. Neuroimage 50, 1690–1701. doi: 10.1016/j.neuroimage.2010.01.002
Mennes, M., Zuo, X.-N., Kelly, C., Di Martino, A., Zang, Y.-F., Biswal, B., et al. (2011). Linking inter-individual differences in neural activation and behavior to intrinsic brain dynamics. Neuroimage 54, 2950–2959. doi: 10.1016/j.neuroimage.2010.10.046
Montenigro, P. H., Alosco, M. L., Martin, B. M., Daneshvar, D. H., Mez, J., Chaisson, C. E., et al. (2017). Cumulative head impact exposure predicts later-life depression, apathy, executive dysfunction, and cognitive impairment in former high school and college football players. J. Neurotrauma 34, 328–340. doi: 10.1089/neu.2016.4413
Moore, R. D., Hillman, C. H., and Broglio, S. P. (2014). The persistent influence of concussive injuries on cognitive control and neuroelectric function. J. Athl. Train. 49, 24–35. doi: 10.4085/1062-6050-49.1.01
Moore, R. D., Pindus, D. M., Drolette, E. S., Scudder, M. R., Raine, L. B., and Hillman, C. H. (2015). The persistent influence of pediatric concussion on attention and cognitive control during flanker performance. Biol. Psychol. 109, 93–102. doi: 10.1016/j.biopsycho.2015.04.008
Niogi, S., Mukherjee, P., Ghajar, J., Johnson, C., Kolster, R., Sarkar, R., et al. (2008). Extent of microstructural white matter injury in postconcussive syndrome correlates with impaired cognitive reaction time: a 3T diffusion tensor imaging study of mild traumatic brain injury. AJNR Am. J. Neuroradiol. 29, 967–973. doi: 10.3174/ajnr.A0970
Niogi, S. N., Mukherjee, P., Ghajar, J., Johnson, C. E., Kolster, R., Lee, H., et al. (2008). Structural dissociation of attentional control and memory in adults with and without mild traumatic brain injury. Brain 131, 3209–3221. doi: 10.1093/brain/awn247
Olson, R. L., Brush, C. J., Ehmann, P. J., Buckman, J. F., and Alderman, B. L. (2018). A history of sport-related concussion is associated with sustained deficits in conflict and error monitoring. Int. J. Psychophysiol. 132, 145–154. doi: 10.1016/j.ijpsycho.2018.01.006
Ozolins, B., Aimers, N., Parrington, L., and Pearce, A. J. (2016). Movement disorders and motor impairments following repeated head trauma: a systematic review of the literature 1990–2015. Brain Inj. 30, 937–947. doi: 10.3109/02699052.2016.1147080
Parks, A. C., Moore, R. D., Wu, C.-T., Broglio, S. P., Covassin, T., Hillman, C. H., et al. (2015). The association between a history of concussion and variability in behavioral and neuroelectric indices of cognition. Intl. J. Psychophysiol. 98, 426–434. doi: 10.1016/j.ijpsycho.2015.08.006
Parr, T., and Friston, K. J. (2017). The active construction of the visual world. Neuropsychologia 104, 92–101. doi: 10.1016/j.neuropsychologia.2017.08.003
Pontifex, M. B., O'Connor, P. M., Broglio, S. P., and Hillman, C. H. (2009). The association between mild traumatic brain injury history and cognitive control. Neuropsychologia 47, 3210–3216. doi: 10.1016/j.neuropsychologia.2009.07.021
Quatman, C. E., Quatman, C. C., and Hewett, T. E. (2009). Prediction and prevention of musculoskeletal injury: a paradigm shift in methodology. Br. J. Sports Med. 43, 1100–1107. doi: 10.1136/bjsm.2009.065482
Ridderinkhof, K. R., Wylie, S. A., van den Wildenberg, W. P., Bashore, T. R., and van der Molen, M. W. (2021). The arrow of time: advancing insights into action control from the arrow version of the Eriksen flanker task. Atten. Percept. Psychophys. 83, 700–721. doi: 10.3758/s13414-020-02167-z
Roalf, D. R., Quarmley, M., Mechanic-Hamilton, D., Wolk, D. A., Arnold, S. E., and Moberg, P. J. (2016). Within-individual variability: an index for subtle change in neurocognition in mild cognitive impairment. J. Alzheimers. Dis. 54, 325–335. doi: 10.3233/JAD-160259
Rothman, K. J. (1990). No adjustments are needed for multiple comparisons. Epidemiology 1, 43–46. doi: 10.1097/00001648-199001000-00010
Schretlen, D. J., Munro, C. A., Anthony, J. C., and Pearlson, G. D. (2003). Examining the range of normal intraindividual variability in neuropsychological test performance. J. Int. Neuropsychol. Soc. 9, 864–870. doi: 10.1017/S1355617703960061
Seeley, W. W., Menon, V., Schatzberg, A. F., Keller, J., Glover, G. H., Kenna, H., et al. (2007). Dissociable intrinsic connectivity networks for salience processing and executive control. J. Neurosci. 27, 2349–2356. doi: 10.1523/JNEUROSCI.5587-06.2007
Selen, L. P., Shadlen, M. N., and Wolpert, D. M. (2012). Deliberation in the motor system: reflex gains track evolving evidence leading to a decision. J. Neurosci. 32, 2276–2286. doi: 10.1523/JNEUROSCI.5273-11.2012
Serpell, B. G., Young, W. B., and Ford, M. (2011). Are the perceptual and decision-making components of agility trainable? A preliminary investigation. J. Strength Cond. Res. 25, 1240–1248. doi: 10.1519/JSC.0b013e3181d682e6
Serrien, D. J., Ivry, R. B., and Swinnen, S. P. (2006). Dynamics of hemispheric specialization and integration in the context of motor control. Nat. Rev. Neurosci. 7, 160–166. doi: 10.1038/nrn1849
Servant, M., and Logan, G. D. (2019). Dynamics of attentional focusing in the Eriksen flanker task. Atten. Percept. Psychophys. 81, 2710–2721. doi: 10.3758/s13414-019-01796-3
Shahim, P., Tegner, Y., Marklund, N., Höglund, K., Portelius, E., Brody, D. L., et al. (2017). Astroglial activation and altered amyloid metabolism in human repetitive concussion. Neurology 88, 1400–1407. doi: 10.1212/WNL.0000000000003816
Shen, I.-H., Lin, Y.-J., Chen, C.-L., and Liao, C.-C. (2020). Neural correlates of response inhibition and error processing in individuals with mild traumatic brain injury: an event-related potential study. J. Neurotrauma 37, 115–124. doi: 10.1089/neu.2018.6122
Sheppard, J., Young, W. B., Doyle, T., Sheppard, T., and Newton, R. U. (2006). An evaluation of a new test of reactive agility and its relationship to sprint speed and change of direction speed. J. Sci. Med. Sport. 9, 342–349. doi: 10.1016/j.jsams.2006.05.019
Sridharan, D., Levitin, D. J., and Menon, V. (2008). A critical role for the right fronto-insular cortex in switching between central-executive and default-mode networks. Proc. Natl. Acad. Sci. U.S.A. 105, 12569–12574. doi: 10.1073/pnas.0800005105
Stawski, R. S., Cerino, E. S., Witzel, D. D., and MacDonald, S. W. (2019a). Daily stress processes as contributors to and targets for promoting cognitive health in later life. Psychosom. Med. 81, 81–89. doi: 10.1097/PSY.0000000000000643
Stawski, R. S., MacDonald, S. W., Brewster, P. W., Munoz, E., Cerino, E. S., and Halliday, D. W. (2019b). A comprehensive comparison of quantifications of intraindividual variability in response times: a measurement burst approach. J. Gerontol. B Psychol. Sci. Soc. Sci. 74, 397–408. doi: 10.1093/geronb/gbx115
Stern, B. D., Hegedus, E. J., and Lai, Y.-C. (2020). Injury prediction as a non-linear system. Phys. Ther. Sport. 41, 43–48. doi: 10.1016/j.ptsp.2019.10.010
Taghdiri, F., Chung, J., Irwin, S., Multani, N., Tarazi, A., Ebraheem, A., et al. (2018). Decreased number of self-paced saccades in post-concussion syndrome associated with higher symptom burden and reduced white matter integrity. J. Neurotrauma 35, 719–729. doi: 10.1089/neu.2017.5274
Takeuchi, N., Oouchida, Y., and Izumi, S.-I. (2012). Motor control and neural plasticity through interhemispheric interactions. Neural Plast. 2012:823285. doi: 10.1155/2012/823285
Themanson, J. R., and Rosen, P. J. (2015). Examining the relationships between self-efficacy, task-relevant attentional control, and task performance: evidence from event-related brain potentials. Br. J. Psychol. 106, 253–271. doi: 10.1111/bjop.12091
Tremblay, S., Henry, L. C., Bedetti, C., Larson-Dupuis, C., Gagnon, J.-F., Evans, A. C., et al. (2014). Diffuse white matter tract abnormalities in clinically normal ageing retired athletes with a history of sports-related concussions. Brain 137, 2997–3011. doi: 10.1093/brain/awu236
Urban, K., Schudlo, L., Keightley, M., Alain, S., Reed, N., and Chau, T. (2021). Altered brain activation in youth following concussion: using a dual-task paradigm. Dev. Neurorehabil. 24, 187–198. doi: 10.1080/17518423.2020.1825539
van Andel, S., Cole, M. H., and Pepping, G.-J. (2017). A systematic review on perceptual-motor calibration to changes in action capabilities. Hum. Mov. Sci. 51, 59–71. doi: 10.1016/j.humov.2016.11.004
van den Heuvel, M. P., and Pol, H. E. H. (2010). Exploring the brain network: a review on resting-state fMRI functional connectivity. Eur. Neuropsychopharmacol. 20, 519–534. doi: 10.1016/j.euroneuro.2010.03.008
van der Ham, I. J., Postma, A., and Laeng, B. (2014). Lateralized perception: the role of attention in spatial relation processing. Neurosci. Biobehav. Rev. 45, 142–148. doi: 10.1016/j.neubiorev.2014.05.006
Wahl, D., Cavalier, A. N., and LaRocca, T. J. (2021). Novel strategies for healthy brain aging. Exerc. Sport Sci. Rev. 49, 115–125. doi: 10.1249/JES.0000000000000242
Wang, C.-H., Yang, C.-T., Moreau, D., and Muggleton, N. G. (2017). Motor expertise modulates neural oscillations and temporal dynamics of cognitive control. Neuroimage 158, 260–270. doi: 10.1016/j.neuroimage.2017.07.009
Wawrzyniak, A. J., Hamer, M., Steptoe, A., and Endrighi, R. (2016). Decreased reaction time variability is associated with greater cardiovascular responses to acute stress. Psychophysiology 53, 739–748. doi: 10.1111/psyp.12617
Wilke, J., Groneberg, D., Banzer, W., and Giesche, F. (2020). Perceptual–cognitive function and unplanned athletic movement task performance: a systematic review. Int. J. Environ. Res. Public Health. 17:7481. doi: 10.3390/ijerph17207481
Wilkerson, G. B., Acocello, S. N., Davis, M. B., Ramos, J. M., Rucker, A. J., and Hogg, J. A. (2021b). Wellness survey responses and smartphone app response efficiency: associations with remote history of sport-related concussion. Percept. Mot. Skills 128, 714–730. doi: 10.1177/0031512520983680
Wilkerson, G. B., Colston, M. A., and Baker, C. S. (2016). A sport fitness index for assessment of sport-related injury risk. Clin. J. Sport Med. 26, 423–428. doi: 10.1097/JSM.0000000000000280
Wilkerson, G. B., Grooms, D. R., and Acocello, S. N. (2017). Neuromechanical considerations for postconcussion musculoskeletal injury risk management. Curr. Sports Med. Rep. 16, 419–427. doi: 10.1249/JSR.0000000000000430
Wilkerson, G. B., Nabhan, D. C., and Crane, R. T. (2020). Concussion history and neuromechanical responsiveness asymmetry. J. Athl. Train. 55, 594–600. doi: 10.4085/1062-6050-0401.19
Wilkerson, G. B., Nabhan, D. C., and Crane, R. T. (2021a). Upper-extremity perceptual-motor training improves whole-body reactive agility among elite athletes with history of sport-related concussion. J. Sport Rehabil. 30, 844–849. doi: 10.1123/jsr.2020-0337
Wilkerson, G. B., Nabhan, D. C., Prusmack, C. J., and Moreau, W. J. (2018). Detection of persisting concussion effects on neuromechanical responsiveness. Med. Sci. Sports Exerc. 50, 1750–1756. doi: 10.1249/MSS.0000000000001647
Williams, D. P., Thayer, J. F., and Koenig, J. (2016). Resting cardiac vagal tone predicts intraindividual reaction time variability during an attention task in a sample of young and healthy adults. Psychophysiology 53, 1843–1851. doi: 10.1111/psyp.12739
Womack, K. B., Paliotta, C., Strain, J. F., Ho, J. S., Skolnick, Y., Lytton, W. W., et al. (2017). Measurement of peripheral vision reaction time identifies white matter disruption in patients with mild traumatic brain injury. J. Neurotrauma 34, 1539–1545. doi: 10.1089/neu.2016.4670
Wu, T., Liu, J., Hallett, M., Zheng, Z., and Chan, P. (2013). Cerebellum and integration of neural networks in dual-task processing. Neuroimage 65, 466–475. doi: 10.1016/j.neuroimage.2012.10.004
Wylie, S. A., Bashore, T. R., Van Wouwe, N. C., Mason, E. J., John, K. D., Neimat, J. S., et al. (2018). Exposing an “intangible” cognitive skill among collegiate football players: enhanced interference control. Front. Psychol. 9:49. doi: 10.3389/fpsyg.2018.00049
Yanuck, S. F. (2019). Microglial phagocytosis of neurons: diminishing neuronal loss in traumatic, infectious, inflammatory and autoimmune CNS disorders. Front. Psychiatry 10:712. doi: 10.3389/fpsyt.2019.00712
Yin, B., Li, D.-D., Huang, H., Gu, C.-H., Bai, G.-H., Hu, L.-X., et al. (2019). Longitudinal changes in diffusion tensor imaging following mild traumatic brain injury and correlation with outcome. Front. Neural Circuits 13:28. doi: 10.3389/fncir.2019.00028
Keywords: sport-related concussion, mild traumatic brain injury, reactive agility, musculoskeletal injury, intra-individual variability, asymmetry, neuromechanics
Citation: Wilkerson GB, Nabhan DC and Perry TS (2021) A Novel Approach to Assessment of Perceptual-Motor Efficiency and Training-Induced Improvement in the Performance Capabilities of Elite Athletes. Front. Sports Act. Living 3:729729. doi: 10.3389/fspor.2021.729729
Received: 23 June 2021; Accepted: 31 August 2021;
Published: 01 October 2021.
Edited by:
Abbey Thomas, University of North Carolina at Charlotte, United StatesReviewed by:
Lisa Custer, Towson University, United StatesKornelius Kraus, Independent researcher, Leimen, Germany
Copyright © 2021 Wilkerson, Nabhan and Perry. This is an open-access article distributed under the terms of the Creative Commons Attribution License (CC BY). The use, distribution or reproduction in other forums is permitted, provided the original author(s) and the copyright owner(s) are credited and that the original publication in this journal is cited, in accordance with accepted academic practice. No use, distribution or reproduction is permitted which does not comply with these terms.
*Correspondence: Gary B. Wilkerson, R2FyeS1XaWxrZXJzb25AdXRjLmVkdQ==
†These authors have contributed equally to this work and share last authorship