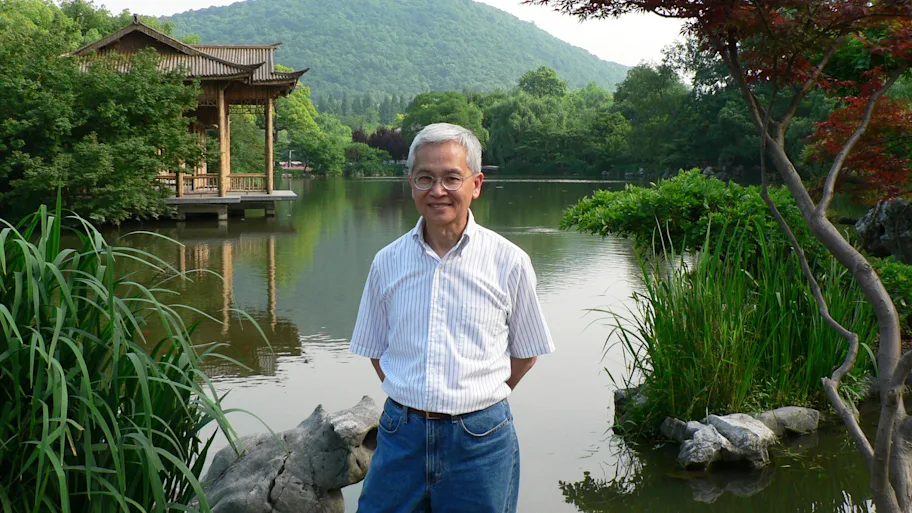
- Science News
- Frontiers news
- “Mathematics of Computation and Data Science” section now open for submissions
“Mathematics of Computation and Data Science” section now open for submissions
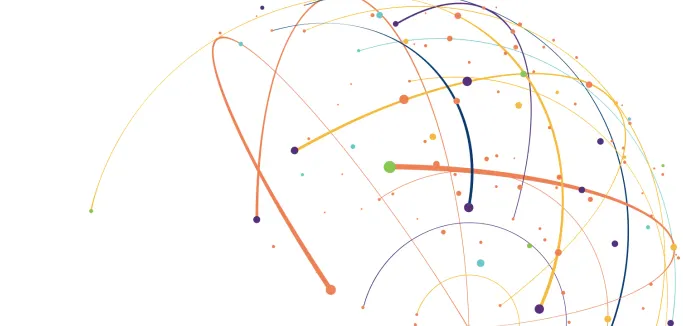
Frontiers in Applied Mathematics and Statistics is proud to announce the launch of its newest section – “Mathematics of Computation and Data Science” led by Specialty Chief Editor, Prof Charles Chui, of Stanford University and Hong Kong Baptist University. We welcome manuscript submissions and Research Topics to this brand new interdisciplinary section.
Message from Specialty Chief Editor, Charles Chui

“Mathematics of Computation and Data Science” is an interdisciplinary section that publishes original research papers, survey articles, tutorials, and innovative numerical modeling, as well as simulation and exciting experimentations, with emphasis on computational methods and algorithms, particularly for data processing, understanding and visualization. The mission of this section is to provide a common platform for communication among mathematicians, statisticians, computer scientists, physicists, biomedical scientists, engineers, and other researchers for sharing their innovative approaches, effective methods, and interesting experimental results, that are instrumental to the advancement of Data Science.
“Mathematics of Computation” is a popular field of research in Mathematics, with original papers published by several well established journals, including: Applied and Computational Harmonic Analysis, SIAM Journal of Scientific Computing, SIAM Journal of Numerical Analysis, Journal of Numerical Mathematics, IMA Journal of Numerical Analysis, Numerische Mathematik, Mathematics of Computation, Foundations of Computational Mathematics, Advances in Computational Mathematics, Applied Numerical Mathematics, BIT Numerical Mathematics, Journal of Computational and Applied Mathematics, Applied Mathematics and Computation, Computational Methods in Applied Mathematics, Journal of Symbolic Computation, and Journal of Computational Mathematics, as well as several other well established journals on mathematical computations in various specialized disciplines, such as Bioinformatics, Computational Mechanics, Computational Biology, Computational Geosciences, Computational Chemistry, Computational Statistics and Data Analysis, Computational Material Science, Computational Geometry, and many others that do not specify computation or numerical analysis in the journal titles.
On the other hand, “Data Science” is an interdisciplinary field of research, originated from Computer Science over three decades ago, and quickly became a major subject of studies in Statistics, particularly in the areas of data analysis and data mining. However, with the rapid exponential growth of available data during this current era of information explosion, it has been clear, even over 10 years ago, that innovative approaches and new analytical theory and methods are needed to meet the need of processing and understanding massive and unstructured data. October 2010 is often considered the month when the term “Big Data” was launched. According to Google Trends results for “Big Data”, there was a spike in this month, followed by another big spike a year later, and a series of spikes in 2012. Perhaps one of the contributions to this profound interest is that October 2010 was also the month when both IBM and Oracle held their biggest Information Management conferences, in Las Vegas and San Francisco, respectively. In addition, although the Facebook social networking service was already launched on February 4, 2004, the company only began to invite users to become beta testers in 2010. By October 2011, over 350 million users accessed Facebook through their mobile phones, accounting for 33% of all Facebook traffic.
So, what is “big data” anyway? In short, it is a broad term for data sets so large or complex, or both, that traditional data processing applications are inadequate. Challenges include: analysis, capture, data curation, search, sharing, storage, transfer, visualization, querying, information capture, and information privacy. In addition, the term “big data” does not limit to static data. Online or near real-time processing and understanding of complex dynamic data, such as multi-directional signals and multi-scale time series, are among the other challenges. In the following, we summarize some of the specific big data challenges:
Data Volume. Data originate from a variety of sources, including business transactions, social media, sensor-to-device data, machine-to-machine data, and biomedical data for individuals. The volumes of such data are massive.
Data transmission. Streaming data at an unprecedented speed must be dealt with in a timely manner. This problem is even more challenging for two-way or multi-party data communication.
Data variety. Data come in all types of formats: from structured numeric data in traditional data-bases to unstructured text documents, from non-numeric data to massive social network and e-mails communications, as well as from compressed video and audio to stock ticker data and financial transaction. All these have to be managed and processed accordingly.
Data Variability. In addition to the increasing transmission velocities and data varieties, data-flows can be highly inconsistent with periodic or non-periodic peaks. Hence, periodic (such as daily or seasonal) and non-periodic (such as event-triggered) peak loads can be challenging to manage. This is even more so for unstructured data. In addition, data trend extraction and forecasting pose an additional challenge.
Data Complexity. Data usually come from multiple sources, making it difficult to link, match, cleanse, and transfer across systems. However, it is necessary to connect and correlate data relationships, hierarchies, and multiple data linkages, before they quickly spiral out of control.
Dynamic data. Signals are everywhere, but usually arrive from some unknown source, and mixed with several signals and perhaps their echoes. Separation of the clean signals from a blind source is a challenge; and more so, if real-time performance is desired.
The above list is definitely far from being complete, but at least helps in providing some guideline for us to formulate relevant mathematical models and come up with corresponding effective computational schemes. As an example, a popular approach is to consider the data set as a point-cloud that lies on some (unknown) manifold in a high dimensional Euclidean space. Then the mathematical problems of interest include: estimation of the manifold dimension, determination of an approximate geodesic distance measurement on the manifold, association of the data set with some graph in terms of the distances among the data, and application of an effective clustering scheme to facilitate the study of the data geometry and topological structure. Manifold dimensionality reduction is also among the topics of investigation. To process or manipulate the data, an objective function defined on the data set could be extended to the graph or a submanifold, and local basis functions on the graph or submanifold could be introduced for approximation and representation of the extended objective function. However, for a massive data set, a significantly much smaller training data set is considered, and supervised or unsupervised learning is applied. Observe that for this particular approach, the theory, methods, and computational schemes considered in the above-mentioned the theory, methods, and computational schemes considered in the above-mentioned mathematical problems involve extension to manifolds or graphs, of certain relevant mathematical analysis from Approximation Theory, Harmonic Analysis, Differential Geometry, Topology, Statistics, and Learning Theory. In addition, if dynamic data are considered, some knowledge of nonlinear PDE is required.
Therefore, “Mathematics of Computation and Data Science” is an interdisciplinary section that provides an opportunity for the interaction among those applied mathematicians, including computer scientists and statisticians; other scientists, including physicists, chemists, biologists and biomedical researchers; as well as those engineers, who are interested in the computational aspects of Data Science. We welcome submission of original research papers, survey articles, and tutorials. The success of this section depends on the authors and the quality of the papers they submit. On behalf the Editorial Board of Associate Editors, we ask for your enthusiastic support.
Interested in getting involved?
If you are interested in becoming part of our international editorial board, submitting a manuscript, or organizing a Research Topic, please contact mathematics@frontiersin.org. We look forward to hearing from you!
Daisy Hessenberger, PhD
Journal Development Manager