- 1Research Group on Global Health, University of Balearic Islands, Palma, Spain
- 2Primary Care of Mallorca, Public Health Service of the Balearic Islands, Palma, Spain
- 3Research Group on Nursing, Community & Global Health, Health Research Institute of the Balearic Islands (IdISBa), Palma, Spain
- 4CIBER of Physiopathology of Obesity and Nutrition (CIBEROBN), Instituto de Salud Carlos III, Madrid, Spain
- 5Physical Activity and Sport Sciences Research Group (GICAFE), Institute for Educational Research and Innovation, University of the Balearic Islands, Palma, Spain
- 6Nursing and Physiotherapy Department, University of the Balearic Islands, Palma, Spain
- 7Hospital Sant Joan de Deu, Palma, Spain
- 8Network for Research on Chronicity, Primary Care, and Health Promotion (RICAPPS), Palma, Spain
- 9Centre for Biomedical Research Network (CIBER) in Epidemiology and Public Health (CIBERESP), Madrid, Spain
Aim: To assess the association between physical activity (PA), sedentary time (ST), and sleep with body composition, and to explore the effects of reallocating ST to PA or sleep on body composition in individuals with prediabetes and overweight/obesity.
Material, methods and results: Baseline data from the PREDIPHONE trial, including 159 participants (mean age 59.6 years) with prediabetes (Fasting Plasma Glucose 100–125 mg/dl) and overweight/obesity (Body Mass Index 27–40 kg/m²), were analyzed. Body composition was assessed via bioelectrical impedance, while PA, ST, and sleep were measured with accelerometry. Linear regression and isotemporal substitution models evaluated associations. Increased ST was positively associated with body fat mass (kg) (β = 0.016; CI 95%: 0.003–0.030), body fat mass (%) (β = 0.009; 0.001–0.018), and visceral adipose tissue (β = 0.005; 0.001–0.010). Moderate-to-vigorous PA (MVPA) was negatively associated with body fat mass (kg) [β = −0.031; 0.055- (−0.008)], body fat mass (%) [β = −0.017; −0.032-(−0.003)], and Visceral adipose tissue [β = −0.009; −0.02-(−0.002)]. Replacing ST with MVPA was linked to lower Visceral adipose tissue [β = −0.012; −0.024-(−0.001)] and body fat mass (kg) [β = −0.039; −0.074-(−0.006)], but not with lean mass. No significant associations were found when substituting ST with light PA or sleep.
Discussion: In individuals with prediabetes and overweight/obesity, replacing ST with MVPA could reduce body fat and VAT but not increases lean mass.
Introduction
Obesity is a multifactorial, chronic, and complex condition characterized by adipose tissue expansion, which significantly increases the risk of cardiometabolic diseases such as type 2 diabetes (T2D) and cardiovascular disease (CVD) (1–3). Obesity represents a serious public health concern due to its rising global prevalence (3). In 2020, 14% of the population worldwide suffered from obesity, and this prevalence is predicted to rise to 24% by 2035 (2). In Spain, the prevalence of obesity in 2022 was 22%, or rather well above the worldwide average (4). Importantly, this prevalence is also rising among middle-aged and older individuals, possibly accelerating the likelihood of new-onset cardiometabolic conditions in these populations (5).
The age-related development of obesity is likely associated with a state of positive energy balance, dependent on lifestyle changes characterized by reduced physical activity (PA), increased sedentary behavior and increased caloric intake (5). Moreover, ageing leads to a series of changes in body composition, including an increase of adipose tissue, redistribution of fat, increasing fat accumulation in the visceral area, and accumulation in ectopic areas such as skeletal muscle and liver (6–9). However, these changes can be followed by loss of muscle mass, reduced strength, and decreased function, frequently progressing to sarcopenic obesity (10). Such obesity often results in functional limitations in the short term, and lower capacity to perform PA in the long term (10). These physiological changes significantly affect glucose metabolism and insulin sensitivity, and stimulate inflammatory processes, thereby accelerating the development of chronic conditions such as T2D and CVD (11–13). Notably, with ageing, visceral adipose tissue promotes systemic insulin resistance and chronic inflammation even when Body Mass Index (BMI) is in the normal or overweight categories and is an independent predictor of diabetes risk and excess mortality (11, 12, 14).
In individuals with overweight or obesity, a 5%–10% weight loss is considered clinically significant in preventing or delaying the onset of chronic conditions such as T2D and CVD (15, 16). However, if weight loss strategies such as caloric restriction are not accompanied by PA, they may lead to loss of lean mass, further exacerbating the decline in muscle quality and strength seen in age-related sarcopenic obesity (7, 17). Reducing sedentary time (ST) and increasing regular PA over the long term are, in fact, key strategies that preserve muscle mass while addressing obesity, and should always be recommended (18, 19). It has been observed that a combination of aerobic and resistance training effectively improves body composition [preserves muscle mass and reduces Visceral adipose tissue (VAT) and ectopic fat], physical function, and cardiometabolic health (reduces skeletal inflammatory markers and ameliorates insulin resistance and pancreatic β-cell function, aiding glucose homeostasis) (20–24), besides improving mental health and overall well-being (19).
Given these considerations, weight loss recommendations for middle-aged and older individuals should not be based solely on body weight or BMI but rather on body composition and other anthropometric measurements such as waist circumference (WC) and take into account not only the absolute values of each component but also their distribution in the body (25, 26).
Isotemporal substitution models are recognized as one of the most suitable statistical approaches for examining the relationships between reallocating activity patterns—particularly time spent in sedentary behavior and physical activity—and health outcomes. This analytical method acknowledges the finite nature of time (27, 28), meaning that an increase in one behavior (e.g., sedentary time) inevitably reduces time allocated to another (e.g., moderate-to-vigorous physical activity). Furthermore, it considers the interdependence of daily behaviours, including sleep, sedentary time, and physical activity (28, 29). By applying this model, valuable insights can be gained into how changes in activity distribution affect cardiometabolic markers in adults. This is essential for designing tailored interventions aimed at enhancing body composition in this population.
For all of the above, the objectives of this cross-sectional analysis were to (1) assess whether PA, ST and sleeping time are associated with body composition, and (2) explore whether reallocating ST to PA at different intensities or sleeping time is associated with differences in body composition in individuals with overweight/obesity and at increased T2D risk.
Material and methods
Study design
The present cross-sectional analysis includes baseline data from the PREDIPHONE trial (clinicaltrial.gov identifier: NCT04735640 registered on February 3, 2021), which assesses the effectiveness of a nurse-led personalized telephone intervention on lifestyle changes in people with prediabetes. The design and methods of the PREDIPHONE trial have been previously described (30). The study was approved by the Institutional Review Board of the Research Ethics Committee of the Balearic Islands (CEI-IB Ref No: IB 3947/19 PI) and complies with the Declaration of Helsinki ethical standards. Informed consent was obtained from all subjects involved in the study.
Study sample
Between May 2021 and September 2022, a total of 206 participants were screened and recruited in 5 primary care centers of Palma, Mallorca, Spain. Inclusion criteria were age 25–75 years, BMI 27–40 kg/m2, Fasting plasma glucose 100–125 mg/dl (American Diabetes Association criteria) (31), none use of oral antidiabetic medication, and written informed consent. Further details of inclusion/exclusion criteria are reported in the published protocol (30).
Of the 206 participants, 159 had valid data available for accelerometry and bioimpedance, and were included in the analysis. Figure 1 shows the flow chart for sample selection (32).
Data collection and measurements
Data was collected at baseline visits by qualified healthcare professionals and included sociodemographic characteristics (age, sex, educational level, and occupation), medical history, use of medications, and smoking behavior.
Participants were categorized as white collar (executives, managers, university professionals, intermediate workers, and employees) or blue collar (manual laborers), according to the occupation they self-reported (33).
Smoking behavior was classified as never, former, or current smoker according to the World Health Organization (WHO) classification.
Anthropometric measurements were collected following the International Standards for Anthropometric Assessment (ISAK) (34). A body composition scale for body weight with bioelectrical impedance analysis (Tanita BC-418, Tanita Corp, Tokyo, Japan) was used to determine weight to the nearest 0.1 kg and body composition (35). Height was measured using a stadiometer [Seca 220 (CM) Telescopic Height Rod for Column Scales, Seca GmbH, Hamburg, Germany] with the participant standing straight and the head in the anatomical position. BMI was calculated using the standard formula [weight (kg)/height (m2)] that classifies obesity as BMI ≥ 30 kg/m2. Waist circumference (WC) was measured in double, halfway between the last rib and the top of the iliac crest, using an anthropometric tape, with participants standing upright, with arms folded across the chest and feet evenly spread apart. The mean of the two measurements was considered for statistical analyses. Waist circumference cut-off points for abdominal obesity were ≥88 cm for women and ≥102 cm for men (36).
Blood pressure (BP) was measured using an electric sphygmomanometer (OMRON M3, Healthcare Europe, Barcelona, Spain) with the participant sitting after 15 min of rest. The average of two measurements, one minute apart, was recorded (37). The arm used for BP reading was the one showing the highest BP.
Venous blood samples were collected from the antecubital vein in suitable vacutainers following an 8–12 h overnight fast.
Physical activity, sleeping time and ST were derived using an accelerometer (GENEActive, ActivInsights Ltd., Cambridgeshire, United Kingdom) on their non-dominant wrist, during 7 consecutive days after the baseline visit. The GENEActiv accelerometer collects triaxial (x, y, and z) accelerometer data to assess patterns of light, moderate, and vigorous PA, sedentary time and sleep (38). It has a dynamic range of ±8 g, which is equivalent to the Earth's gravitational force. It was programmed to collect and store accelerations with a sampling frequency of 40 Hz.
Data extracted from the GENEActiv (all in bouts of at least 1 min) were clustered as: inactive time (cut-off intensity level <40 mg) for those behaviors during waking hours equivalent to <1.5 Metabolic Equivalent Tasks (METs); light-intensity PA (LPA) (39) (cut-off intensity level between ≥40 mg and <100 mg) equivalent to 1.5–3 METs; moderate-to-vigorous PA (MVPA) (cut-off intensity level ≥100 mg) equivalent to >3 METs; and sleeping time (time between going to bed and getting up, calculated using a validated heuristic algorithm from accelerometer raw data unaided by a sleep diary) (40–42). MVPA was analyzed together, as this population practiced very (LPA. The University of the Balearic Islands maintained the raw data files on its servers. To process the data, the open-source R-package GGIR, version 4.2–1 (cran.rproject.org/web/packages/GGIR/index. html), was utilized. The package was run using R (R Core Team, Vienna, Austria). The open-source code in GGIR has been verified against functions that self-calibrate, ensuring its reliability (43).
Statistical analysis
Categorical variables were expressed as numbers and percentages. Chi-square test (χ2) was used to assess the associations between categorical variables, such the association between as low or high VAT and social class and smoking habits. Continuous variables were summarized as means and standard deviations (SDs) and associations were evaluated using analysis of variance.
Linear regression analyses were used to estimate the β-coefficients and 95% CI for the associations between PA and ST with body composition, using accelerometry data and bioimpedance, respectively.
Isotemporal substitution linear regression modelling was performed to examine the associations of replacing ST with sleep time, LPA, or MVPA on body composition. The resulting regression coefficient represents the effect of reallocating a unit of ST to a unit of sleep time, LPA, or MVPA. The model 1 was adjusted for age and sex, and the model 2 was further adjusted for social class, smoking status and adherence to mediterranean diet. The analyses followed published guidelines for isotemporal substitution (29). The isotemporal substitution model allows for the replacement of behaviours while accounting for the finite nature of time (24 h per day). This approach aligns with individuals' daily realities, enabling a more accurate and realistic interpretation of the data, with greater applicability to recommendations on healthy habits.
A P-values < 0.05 were considered statistically significant. All analyses were conducted using Stata v.16 software (StataCorp, Texas, USA).
Results
Of the 159 participants with prediabetes and overweight/obesity included in the present analysis, 52.8% were women. Mean age was 59.6 (10.1) years. Sociodemographic characteristics, smoking habits, anthropometric measures, body composition, clinical parameters, and 24 h movement behaviors of the sample as a whole and by VAT accumulation were shown in Table 1. Moreover, the same date has shown by sex in Supplementary Table S1. Most subjects (78%) were blue-collar and non-smokers (47.2%). The mean BMI was 32.1 [standard deviation (SD) 3.6]. Regarding body composition, the mean VAT was 13.1 (SD 3.9), and the mean body fat mass in percentage (%) was 35.8 (SD 7.0). The behavior that accumulated the most time in 24 h was ST, with an average of 751 (SD 93.4) minutes, or 12.5 h per day. Light PA was the main type of exercise performed, with a mean accumulation of181.1 min per day (SD 55.5), followed by MPA, with a mean of 108.4 min (SD 55.7). Participants only performed a mean of 2.7 (SD 3.6) minutes per day of VPA.
As compared to women, men presented a significantly lower fat body mass (%), but higher VAT (all p < 0.001), although women were more often abdominally obese than men (p = 0.02). Men also presented higher systolic and diastolic BP (p = 0.005 and p = 0.01, respectively) and lower HDL-cholesterol levels (p < 0.001) when compared to women. No differences between sexes were observed in 24 h movement behaviors (see Supplementary Table S1).
Table 1 provides an overview of the study sample categorized according to VAT accumulation. The population was classified into three groups: “low VAT accumulation” (0–9 units based on tetrapolar bioimpedance) with a mean 8.2 (SD 0.9), “moderate VAT accumulation” (10–14 units) with a mean 12.0 (SD1.3), and “high VAT accumulation” (≥15 units) with a mean 17.5 (SD 2.5). The population with high VAT accumulation was older, predominantly male, were former smokers (less than two years without smoke), and has higher BMI, greater WC, and has abdominal obesity as measured by WC. All p-values were significant. No significant differences were observed in relation to social class or adherence to the Mediterranean diet. Regarding body composition, participants with high VAT accumulation had greater total fat mass (kg) and muscle mass (kg) but lower total fat percentage. All p-values were significant. Concerning clinical parameters, the population with higher VAT presents higher SBP and DBP and lower HDL-c levels. In terms of 24 h behaviours, participants with higher VAT engaged in less LPA, MPA, and VPA while spending more time in sedentary activities. All p-values were significant.
The associations between ST, PA and sleeping time with BMI, WC and body composition variables are shown in Table 2. As expected, ST was positively and significantly associated with body fat mass (kg) (β = 0.016; CI 95%: 0.003–0.030), body fat mass (%) (β = 0.009; CI 95%: 0.001–0.018) and VAT (β = 0.005; CI 95%: 0.001–0.010). Moderate-to-vigorous PA was negatively and significantly associated with body fat mass (kg) [β = −0.031; CI 95%: 0.055- (−0.008)], body fat mass (%) [β = −0.017; CI 95%: −0.032-(−0.003)] and VAT [β = −0.009; CI 95%: −0.02-(−0.002)]. No significant associations were observed between LPA or sleeping time with any body composition variables. Results were consistent between models 1 and 2. When further adjusting for Mediterranean diet, social class and smoking, sleep and sedentary time were not significantly associated with VAT nor body fat. The remaining associations did not change with the additional adjustments.
The β-coefficients (95% CI) of the isotemporal substitution models are shown in Table 3. Isotemporal substitution of 1 min per day of ST with the equivalent time of MVPA (i.e., decreasing ST at the expenses of increasing MVPA time, without changing the time spent on other behaviors) was associated with less VAT [β = −0.012; CI 95%: −0.024-(−0.001)] and less body fat mass (kg) [β = −0.039; CI 95%: −0.074-(−0.006)]. Reallocating 1 min of ST for LPA or sleeping time was not associated with any of the body composition variables, nor WC or BMI. There were trends indicating associations between increased levels of any PA and greater lean mass, as well as replacing ST with sleeping time and lower lean mass but did not reach statistical significance. The results obtained for Model 1 and Model 2 were consistent and exhibited minimal variation.
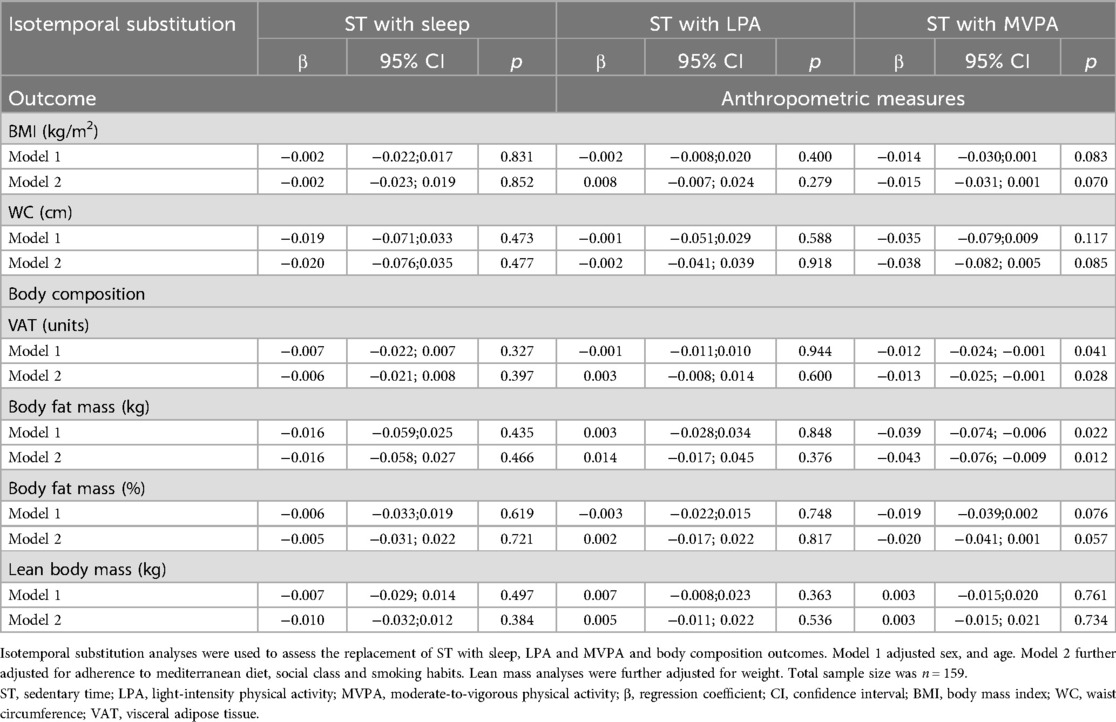
Table 3. Isotemporal substitution of sitting time with physical activity and sleep time on body composition and anthropometric measures.
Discussion
The main results from the present cross-sectional analysis including middle-aged individuals with prediabetes and overweight or obesity show that an increase in ST was associated with increased VAT and body fat mass, while an increase in MVPA was associated with reduced VAT and body fat mass. Moreover, replacing ST with MVPA was associated with lower VAT and body fat mass accumulation, but not with changes in lean mass. Of note, only a small proportion of individuals regularly engaged in MVPA, with the vast majority engaging in none or LPA, which is characteristic of the obese population (44).
Our findings are in line with previous evidence showing that when ST is replaced with MVPA it is associated with improved body composition and specifically less total body fat and VAT (45, 46).
We did not find associations between replacing ST with LPA and changes in body composition. This is in contrast with other studies that have shown the potential beneficial effect of substituting ST with LPA on health outcomes in high-risk populations with prediabetes and T2D (47). Previous research has in fact showed a significant decrease in fat mass (48) and VAT associated to LPA increase in older population with overweight or obesity (45). However, these effects were less pronounced compared to substituting ST with MVPA, possibly indicating that the degree of body and visceral fat loss is dependent on the intensity of the PA.
Replacing ST with sleeping time did not improve weight or body composition in our sample. This is also in contrast with other studies that found this substitution associated with lower BMI and WC (45). Sleep loss is linked to increased inflammation and hormonal disruptions, including insulin, leptin and ghrelin, which can affect energy balance and body weight regulation (49). However, it has also been observed that disturbed sleep rather than sleep loss can increase cardiometabolic risk factors, including weight gain, in the long term (50, 51). The lack of association in our study may be due to the fact that we considered sleep duration rather than sleep quality, possibly masking the potential benefits of replacing ST with sleeping time if the quality of sleep was poor.
Additionally, our results did not show associations between any PA level and lean mass, and a non-significant trend indicating associations between increased levels of any PA and greater lean mass. Previous studies show that engaging in MVPA and reducing ST were associated with a higher lean mass (52). Even among older people, increased time dedicated to MVPA, and reduced ST were linked to improved body composition and a decreased likelihood of loss of muscle mass (53). The discrepancies with our findings may be explained by the fact that lean mass, like fat mass, is dependent of PA intensity. In our sample, the main type of PA performed for MVPA was moderate rather than vigorous (98.1% of women and 96.7% of men performed MPA within the MVPA category), and thus not enough intensive to significantly impact lean mass. Previous studies, in fact, observe that achieving a significant change in lean mass may require more intensive PA (45, 53, 54). Since skeletal muscle accounts for 80% of insulin-stimulated glucose disposal, and that obesity is associated with increasing insulin resistance in muscle tissue (55, 56), maintaining muscle mass while reducing total fat and VAT should always be advised when loss of weight is recommended.
Importantly, the dose-dependent effect of PA is not limited to body composition. Although it has been observed that any PA intensity is beneficial for overall health and chronic disease prevention (54), including CVD and T2D (47, 57, 58), VPA shows the highest risk reduction (47), making it one of the main strategies to maintain and improve individual's health.
A marked strength of this study was the objective and validated measurements used for both exposure and outcome variables, increasing the opportunities for comparison across the literature and reducing any potential bias or measurement error. Both the tetra-polar bioimpedance and accelerometry are valid, quick, and easy-to-use techniques (35, 38). The accelerometry electronic devices record the acceleration linked to body movements, offering an objective estimate of the movement's duration and intensity (45, 54). Additionally, the isotemporal substitution analysis represents a robust and novel research methodology.
In terms of limitations, the cross-sectional design limits drawing conclusions on causality or evaluating changes in body composition over the time. Our findings suggest associations between the ST, PA, and body composition, but the nature of these relationships remains observational. Previous longitudinal and intervention studies indicate that decreasing sedentary time (ST) and increasing physical activity (PA) may contribute to enhancements in body composition (59–62), but our results should be interpreted as correlational rather than causal. Future prospective and experimental studies are needed to confirm these associations and better understand the direction of effect. Secondly, the modest sample size may limit the generalizability of the results to individuals with prediabetes and overweight or obese.
In conclusion, this study showed that among middle-aged individuals with prediabetes and overweight or obesity, increased ST is associated with higher VAT and body fat mass, while MVPA is associated with reductions in both. Substituting ST with MVPA could lower VAT and body fat mass but does not increase lean mass. Rather than solely focusing on body weight, weight loss recommendations should be aimed at reducing adipose tissue while preserving or improving muscle mass.
Data availability statement
The raw data supporting the conclusions of this article will be made available by the authors, without undue reservation.
Ethics statement
The studies involving humans were approved by The study was approved by the Institutional Review Board of the Research Ethics Committee of the Balearic Islands (CEI-IB Ref No: IB 3947/19 PI) and complies with the Declaration of Helsinki ethical standards. Informed consent was obtained from all subjects involved in the study. The studies were conducted in accordance with the local legislation and institutional requirements. The participants provided their written informed consent to participate in this study. Written informed consent was obtained from the individual(s) for the publication of any potentially identifiable images or data included in this article.
Author contributions
MT-C: Conceptualization, Data curation, Formal analysis, Writing – original draft. AG-P: Conceptualization, Formal analysis, Supervision, Writing – review & editing. MA-F: Data curation, Investigation, Writing – review & editing. AH-T: Data curation, Investigation, Writing – review & editing. MA: Data curation, Investigation, Writing – review & editing. SF: Data curation, Investigation, Writing – review & editing. CS-R: Investigation, Writing – review & editing. AY: Conceptualization, Formal analysis, Methodology, Project administration, Supervision, Writing – review & editing. MB-V: Conceptualization, Formal analysis, Funding acquisition, Methodology, Project administration, Supervision, Writing – review & editing.
Funding
The author(s) declare that financial support was received for the research and/or publication of this article. This work was supported by the Ministry of Economy and Competitiveness, Carlos III Health Institute (grant number PI18/012019). Primary Care and Health Promotion Network (RICAPPS) RD21/0016/0009, co-financed by Next Generation (EU) funds from the Recovery and Resilience Mechanism. Funders were not involved in the study design, data collection, data analysis, interpretation analyses, decision to publish, preparation, writing review, or approval of the manuscript.
Acknowledgments
The authors sincerely appreciate all the participants for their valuable contributions.
Conflict of interest
The authors declare that the research was conducted in the absence of any commercial or financial relationships that could be construed as a potential conflict of interest.
Generative AI statement
The author(s) declare that no Generative AI was used in the creation of this manuscript.
Publisher's note
All claims expressed in this article are solely those of the authors and do not necessarily represent those of their affiliated organizations, or those of the publisher, the editors and the reviewers. Any product that may be evaluated in this article, or claim that may be made by its manufacturer, is not guaranteed or endorsed by the publisher.
Supplementary material
The Supplementary Material for this article can be found online at: https://www.frontiersin.org/articles/10.3389/fspor.2025.1579962/full#supplementary-material
References
1. Ortega MA, Fraile-Martinez O, Naya I, Garcia-Honduvilla N, Alvarez-Mon M, Bujan J, et al. Type 2 diabetes mellitus associated with obesity (diabesity). the central role of gut microbiota and its translational applications. Nutrients. (2020) 12(9):2749. doi: 10.3390/nu12092749
4. Perez-Rodrigo C, Hervas Barbara G, Gianzo Citores M, Aranceta-Bartrina J. Prevalence of obesity and associated cardiovascular risk factors in the Spanish population: the ENPE study. Rev Esp Cardiol (Engl Ed). (2022) 75(3):232–41. doi: 10.1016/j.rec.2020.12.020
5. Jura M, Kozak LP. Obesity and related consequences to ageing. Age (Dordr). (2016) 38(1):23. doi: 10.1007/s11357-016-9884-3
6. Correa-de-Araujo R, Addison O, Miljkovic I, Goodpaster BH, Bergman BC, Clark RV, et al. Myosteatosis in the context of skeletal muscle function deficit: an interdisciplinary workshop at the national institute on aging. Front Physiol. (2020) 11:963. doi: 10.3389/fphys.2020.00963
7. Raynor HA, Davidson PG, Burns H, Nadelson MDH, Mesznik S, Uhley V, et al. Medical nutrition therapy and weight loss questions for the evidence analysis library prevention of type 2 diabetes project: systematic reviews. J Acad Nutr Diet. (2017) 117(10):1578–611. doi: 10.1016/j.jand.2017.06.361
8. Vatcheva KP, Fisher-Hoch SP, Reininger BM, McCormick JB. Sex and age differences in prevalence and risk factors for prediabetes in Mexican-Americans. Diabetes Res Clin Pract. (2020) 159:107950. doi: 10.1016/j.diabres.2019.107950
9. Frank AP, de Souza Santos R, Palmer BF, Clegg DJ. Determinants of body fat distribution in humans may provide insight about obesity-related health risks. J Lipid Res. (2019) 60(10):1710–9. doi: 10.1194/jlr.R086975
10. Bosello O, Vanzo A. Obesity paradox and aging. Eat Weight Disord. (2021) 26(1):27–35. doi: 10.1007/s40519-019-00815-4
11. Neeland IJ, Ross R, Despres JP, Matsuzawa Y, Yamashita S, Shai I, et al. Visceral and ectopic fat, atherosclerosis, and cardiometabolic disease: a position statement. Lancet Diabetes Endocrinol. (2019) 7(9):715–25. doi: 10.1016/S2213-8587(19)30084-1
12. Deng R, Chen W, Zhang Z, Zhang J, Wang Y, Sun B, et al. Association between visceral obesity index and diabetes: a systematic review and meta-analysis. J Clin Endocrinol Metab. (2024) 109(10):2692–707. doi: 10.1210/clinem/dgae303
13. Kuk JL, Saunders TJ, Davidson LE, Ross R. Age-related changes in total and regional fat distribution. Ageing Res Rev. (2009) 8(4):339–48. doi: 10.1016/j.arr.2009.06.001
14. Bowman K, Atkins JL, Delgado J, Kos K, Kuchel GA, Ble A, et al. Central adiposity and the overweight risk paradox in aging: follow-up of 130,473 UK biobank participants. Am J Clin Nutr. (2017) 106(1):130–5. doi: 10.3945/ajcn.116.147157
15. American Diabetes Association Professional Practice C. 3. Prevention or delay of diabetes and associated comorbidities: standards of care in diabetes-2024. Diabetes Care. (2024) 47(Suppl 1):S43–51. doi: 10.2337/dc24-S003
16. Hamman RF, Wing RR, Edelstein SL, Lachin JM, Bray GA, Delahanty L, et al. Effect of weight loss with lifestyle intervention on risk of diabetes. Diabetes Care. (2006) 29(9):2102–7. doi: 10.2337/dc06-0560
17. Colleluori G, Villareal DT. Aging, obesity, sarcopenia and the effect of diet and exercise intervention. Exp Gerontol. (2021) 155:111561. doi: 10.1016/j.exger.2021.111561
18. Boutari C, Mantzoros CS. A 2022 update on the epidemiology of obesity and a call to action: as its twin COVID-19 pandemic appears to be receding, the obesity and dysmetabolism pandemic continues to rage on. Metab Clin Exp. (2022) 133:155217. doi: 10.1016/j.metabol.2022.155217
19. Bull FC, Al-Ansari SS, Biddle S, Borodulin K, Buman MP, Cardon G, et al. World health organization 2020 guidelines on physical activity and sedentary behaviour. Br J Sports Med. (2020) 54(24):1451–62. doi: 10.1136/bjsports-2020-102955
20. Asano RY, Sales MM, Browne RA, Moraes JF, Coelho Junior HJ, Moraes MR, et al. Acute effects of physical exercise in type 2 diabetes: a review. World J Diabetes. (2014) 5(5):659–65. doi: 10.4239/wjd.v5.i5.659
21. Bacchi E, Negri C, Zanolin ME, Milanese C, Faccioli N, Trombetta M, et al. Metabolic effects of aerobic training and resistance training in type 2 diabetic subjects: a randomized controlled trial (the RAED2 study). Diabetes Care. (2012) 35(4):676–82. doi: 10.2337/dc11-1655
22. Jiang Y, Tan S, Wang Z, Guo Z, Li Q, Wang J. Aerobic exercise training at maximal fat oxidation intensity improves body composition, glycemic control, and physical capacity in older people with type 2 diabetes. J Exerc Sci Fit. (2020) 18(1):7–13. doi: 10.1016/j.jesf.2019.08.003
23. Strasser B, Pesta D. Resistance training for diabetes prevention and therapy: experimental findings and molecular mechanisms. Biomed Res Int. (2013) 2013:805217. doi: 10.1155/2013/805217
24. Consitt LA, Dudley C, Saxena G. Impact of endurance and resistance training on skeletal muscle glucose metabolism in older adults. Nutrients. (2019) 11(11):2636. doi: 10.3390/nu11112636
26. Wang L, Yi Z. Obesity paradox and aging: visceral adiposity index and all-cause mortality in older individuals: a prospective cohort study. Front Endocrinol (Lausanne). (2022) 13:975209. doi: 10.3389/fendo.2022.975209
27. Lerma NL, Cho CC, Swartz AM, Miller NE, Keenan KG, Strath SJ. Isotemporal substitution of sedentary behavior and physical activity on function. Med Sci Sports Exerc. (2018) 50(4):792–800. doi: 10.1249/MSS.0000000000001491
28. Edwardson CL, Henson J, Bodicoat DH, Bakrania K, Khunti K, Davies MJ, et al. Associations of reallocating sitting time into standing or stepping with glucose, insulin and insulin sensitivity: a cross-sectional analysis of adults at risk of type 2 diabetes. BMJ Open. (2017) 7(1):e014267. doi: 10.1136/bmjopen-2016-014267
29. Dumuid D, Pedisic Z, Stanford TE, Martin-Fernandez JA, Hron K, Maher CA, et al. The compositional isotemporal substitution model: a method for estimating changes in a health outcome for reallocation of time between sleep, physical activity and sedentary behaviour. Stat Methods Med Res. (2019) 28(3):846–57. doi: 10.1177/0962280217737805
30. Abbate M, Fresneda S, Yanez A, Ricci-Cabello I, Galmes-Panades AM, Aguilo A, et al. Nurse-led telephone intervention for lifestyle changes on glycaemic control in people with prediabetes: study protocol for a randomized controlled trial. J Adv Nurs. (2021) 77(7):3204–17. doi: 10.1111/jan.14842
31. American Diabetes Association Professional Practice C. 2. Classification and diagnosis of diabetes: standards of medical care in diabetes-2022. Diabetes Care. (2022) 45(Suppl 1):S17–38. doi: 10.2337/dc22-S002
32. Arias-Fernandez M, Fresneda S, Abbate M, Torres-Carballo M, Huguet-Torres A, Sanchez-Rodriguez C, et al. Fatty liver disease in patients with prediabetes and overweight or obesity. Metabolites. (2023) 13(4):531. doi: 10.3390/metabo13040531
33. Domingo-Salvany A, Bacigalupe A, Carrasco JM, Espelt A, Ferrando J, Borrell C, et al. Proposals for social class classification based on the Spanish national classification of occupations 2011 using neo-Weberian and neo-Marxist approaches. Gac Sanit. (2013) 27(3):263–72. doi: 10.1016/j.gaceta.2012.12.009
34. Esparza-Ros F, Vaquero-Cristóbal R, Marfell-Jones M. International Society for Advancement of K. International Standards for Anthropometric Assessment: International Society for the Advancement of Kinanthropometry (ISAK) (2019).
35. Astorino TA, Baker JS, Brock S, Dalleck LC, Goulet EDB, Gotshall RW, et al. Validity and reliability of body composition analysis using the tanita BC 418-MA. J Exerc Physiol. (2012) 15:74–83.
36. Salas-Salvado J, Rubio MA, Barbany M, Moreno B, Grupo Colaborativo de la S. SEEDO 2007 consensus for the evaluation of overweight and obesity and the establishment of therapeutic intervention criteria. Med Clin (Barc). (2007) 128(5):184–96. doi: 10.1016/S0025-7753(07)72531-9
37. Gorostidi M, Gijon-Conde T, de la Sierra A, Rodilla E, Rubio E, Vinyoles E, et al. 2022 practice guidelines for the management of arterial hypertension of the Spanish society of hypertension. Hipertens Riesgo Vasc. (2022) 39(4):174–94. doi: 10.1016/j.hipert.2022.09.002
38. Rowlands AV, Mirkes EM, Yates T, Clemes S, Davies M, Khunti K, et al. Accelerometer-assessed physical activity in epidemiology: are monitors equivalent? Med Sci Sports Exerc. (2018) 50(2):257–65. doi: 10.1249/MSS.0000000000001435
39. Bhupathiraju SN, Hu FB. Epidemiology of obesity and diabetes and their cardiovascular complications. Circ Res. (2016) 118(11):1723–35. doi: 10.1161/CIRCRESAHA.115.306825
40. Bankoski A, Harris TB, McClain JJ, Brychta RJ, Caserotti P, Chen KY, et al. Sedentary activity associated with metabolic syndrome independent of physical activity. Diabetes Care. (2011) 34(2):497–503. doi: 10.2337/dc10-0987
41. Kazaz I, Angin E, Kabaran S, Iyigun G, Kirmizigil B, Malkoc M. Evaluation of the physical activity level, nutrition quality, and depression in patients with metabolic syndrome: comparative study. Medicine (Baltimore). (2018) 97(18):e0485. doi: 10.1097/MD.0000000000010485
42. Strasser B. Physical activity in obesity and metabolic syndrome. Ann N Y Acad Sci. (2013) 1281(1):141–59. doi: 10.1111/j.1749-6632.2012.06785.x
43. Gallardo-Alfaro L, Bibiloni MDM, Mascaro CM, Montemayor S, Ruiz-Canela M, Salas-Salvado J, et al. Leisure-time physical activity, sedentary behaviour and diet quality are associated with metabolic syndrome severity: the PREDIMED-plus study. Nutrients. (2020) 12(4):1013. doi: 10.3390/nu12041013
44. Silveira EA, Mendonca CR, Delpino FM, Elias Souza GV, Pereira de Souza Rosa L, de Oliveira C, et al. Sedentary behavior, physical inactivity, abdominal obesity and obesity in adults and older adults: a systematic review and meta-analysis. Clin Nutr ESPEN. (2022) 50:63–73. doi: 10.1016/j.clnesp.2022.06.001
45. Galmes-Panades AM, Varela-Mato V, Konieczna J, Warnberg J, Martinez-Gonzalez MA, Salas-Salvado J, et al. Isotemporal substitution of inactive time with physical activity and time in bed: cross-sectional associations with cardiometabolic health in the PREDIMED-plus study. Int J Behav Nutr Phys Act. (2019) 16(1):137. doi: 10.1186/s12966-019-0892-4
46. Winters-van Eekelen E, Van der Velde JH, Boone SC, Westgate K, Brage S, Lamb HJ, et al. Objectively measured physical activity and body fatness: associations with total body fat, visceral fat, and liver fat. Med Sci Sports Exerc. (2021) 53(11):2309–17. doi: 10.1249/MSS.0000000000002712
47. Cao Z, Xu C, Zhang P, Wang Y. Associations of sedentary time and physical activity with adverse health conditions: outcome-wide analyses using isotemporal substitution model. EClinicalMedicine. (2022) 48:101424. doi: 10.1016/j.eclinm.2022.101424
48. Gaba A, Pelclova J, Stefelova N, Pridalova M, Zajac-Gawlak I, Tlucakova L, et al. Prospective study on sedentary behaviour patterns and changes in body composition parameters in older women: a compositional and isotemporal substitution analysis. Clin Nutr. (2021) 40(4):2301–7. doi: 10.1016/j.clnu.2020.10.020
49. Knutson KL, Spiegel K, Penev P, Van Cauter E. The metabolic consequences of sleep deprivation. Sleep Med Rev. (2007) 11(3):163–78. doi: 10.1016/j.smrv.2007.01.002
50. Clark AJ, Salo P, Lange T, Jennum P, Virtanen M, Pentti J, et al. Onset of impaired sleep and cardiovascular disease risk factors: a longitudinal study. Sleep. (2016) 39(9):1709–18. doi: 10.5665/sleep.6098
51. Chaput JP, Dutil C, Featherstone R, Ross R, Giangregorio L, Saunders TJ, et al. Sleep timing, sleep consistency, and health in adults: a systematic review. Appl Physiol Nutr Metab. (2020) 45(10):S232–S47. doi: 10.1139/apnm-2020-0032
52. Rosique-Esteban N, Babio N, Diaz-Lopez A, Romaguera D, Alfredo Martinez J, Sanchez VM, et al. Leisure-time physical activity at moderate and high intensity is associated with parameters of body composition, muscle strength and sarcopenia in aged adults with obesity and metabolic syndrome from the PREDIMED-plus study. Clin Nutr. (2019) 38(3):1324–31. doi: 10.1016/j.clnu.2018.05.023
53. Scott D, Johansson J, Gandham A, Ebeling PR, Nordstrom P, Nordstrom A. Associations of accelerometer-determined physical activity and sedentary behavior with sarcopenia and incident falls over 12 months in community-dwelling Swedish older adults. J Sport Health Sci. (2021) 10(5):577–84. doi: 10.1016/j.jshs.2020.01.006
54. Yerrakalva D, Hajna S, Khaw KT, Griffin SJ, Brage S. Prospective associations between changes in physical activity and sedentary time and subsequent lean muscle mass in older english adults: the EPIC-Norfolk cohort study. Int J Behav Nutr Phys Act. (2024) 21(1):10. doi: 10.1186/s12966-023-01547-6
55. Merz KE, Thurmond DC. Role of skeletal muscle in insulin resistance and glucose uptake. Compr Physiol. (2020) 10(3):785–809. doi: 10.1002/cphy.c190029
56. Wilmot EG, Edwardson CL, Achana FA, Davies MJ, Gorely T, Gray LJ, et al. Sedentary time in adults and the association with diabetes, cardiovascular disease and death: systematic review and meta-analysis. Diabetologia. (2012) 55(11):2895–905. doi: 10.1007/s00125-012-2677-z
57. Llavero-Valero M, Escalada-San Martin J, Martinez-Gonzalez MA, Basterra-Gortari FJ, Gea A, Bes-Rastrollo M. Physical activity intensity and type 2 diabetes: isotemporal substitution models in the “seguimiento universidad de navarra” (SUN) cohort. J Clin Med. (2021) 10(13):2744. doi: 10.3390/jcm10132744
58. Cleven L, Krell-Roesch J, Nigg CR, Woll A. The association between physical activity with incident obesity, coronary heart disease, diabetes and hypertension in adults: a systematic review of longitudinal studies published after 2012. BMC Public Health. (2020) 20(1):726. doi: 10.1186/s12889-020-08715-4
59. Lai TF, Liao Y, Hsueh MC, Yen HY, Park JH, Chang JH. Substituting sedentary time with physical activity in youngest-old to oldest-old community-dwelling older adults: associations with body composition. Front Public Health. (2022) 10:837213. doi: 10.3389/fpubh.2022.837213
60. Martin A, Fitzsimons C, Jepson R, Saunders DH, van der Ploeg HP, Teixeira PJ, et al. Interventions with potential to reduce sedentary time in adults: systematic review and meta-analysis. Br J Sports Med. (2015) 49(16):1056–63. doi: 10.1136/bjsports-2014-094524
61. Ciriminna S, Veronese N, Cannizzo C, D’Aleo A, Ganci A, Montana Lampo SE, et al. Effectiveness of physical activity interventions in sedentary people during COVID-19 lockdown: a systematic review of randomized controlled trials. Sustainability. (2024) 16(3):1331. doi: 10.3390/su16031331
62. Kenkhuis MF, Klingestijn M, Fanshawe AM, Breukink SO, Janssen-Heijnen MLG, Keulen ETP, et al. Longitudinal associations of sedentary behavior and physical activity with body composition in colorectal cancer survivors up to 2 years post treatment. J Cancer Res Clin Oncol. (2023) 149(7):4063–75. doi: 10.1007/s00432-022-04267-9
Keywords: body composition, visceral adipose tissue, physical activity, sedentary time, obesity, prediabetes
Citation: Torres-Carballo M, Galmes-Panades AM, Arias-Fernández M, Huguet-Torres A, Abbate M, Fresneda S, Sánchez-Rodríguez C, Yañez AM and Bennasar-Veny M (2025) Isotemporal substitution of sedentary time with physical activity and sleeping time: associations with body composition among individuals with prediabetes. Front. Sports Act. Living 7:1579962. doi: 10.3389/fspor.2025.1579962
Received: 19 February 2025; Accepted: 24 March 2025;
Published: 7 April 2025.
Edited by:
Shengdi Lu, Shanghai Jiao Tong University, ChinaReviewed by:
Yuhan Li, University of Illinois at Urbana-Champaign, United StatesZitong Zhang, Icahn School of Medicine at Mount Sinai, United States
Chunyi Zhang, University of Texas MD Anderson Cancer Center, United States
Copyright: © 2025 Torres-Carballo, Galmes-Panades, Arias-Fernández, Huguet-Torres, Abbate, Fresneda, Sánchez-Rodríguez, Yañez and Bennasar-Veny. This is an open-access article distributed under the terms of the Creative Commons Attribution License (CC BY). The use, distribution or reproduction in other forums is permitted, provided the original author(s) and the copyright owner(s) are credited and that the original publication in this journal is cited, in accordance with accepted academic practice. No use, distribution or reproduction is permitted which does not comply with these terms.
*Correspondence: A. M. Galmes-Panades, YWluYS5nYWxtZXNAdWliLmVz
†These authors have contributed equally to this work