- 1Center for Agricultural Resources Research, Institute of Genetics and Developmental Biology, Chinese Academy of Sciences, Shijiazhuang, China
- 2The College of Life Science, University of Chinese Academy of Sciences, Beijing, China
- 3College of Biology and Engineering, Hebei University of Economics and Business, Shijiazhuang, China
- 4State Key Laboratory of Plant Cell and Chromosome Engineering, Chinese Academy of Sciences, Beijing, China
- 5Anyang Academy of Agricultural Sciences, Anyang, China
High-density genetic linkage maps are essential for precise mapping quantitative trait loci (QTL) in wheat (Triticum aestivum L.). In this study, a high-density genetic linkage map consisted of 6312 SNP and SSR markers was developed to identify QTL controlling kernel size and weight, based on a recombinant inbred line (RIL) population derived from the cross of Shixin828 and Kenong2007. Seventy-eight putative QTL for kernel length (KL), kernel width (KW), kernel diameter ratio (KDR), and thousand kernel weight (TKW) were detected over eight environments by inclusive composite interval mapping (ICIM). Of these, six stable QTL were identified in more than four environments, including two for KL (qKL-2D and qKL-6B.2), one for KW (qKW-2D.1), one for KDR (qKDR-2D.1) and two for TKW (qTKW-5A and qTKW-5B.2). Unconditional and multivariable conditional QTL mapping for TKW with respect to TKW component (TKWC) revealed that kernel dimensions played an important role in regulating the kernel weight. Seven QTL-rich genetic regions including seventeen QTL were found on chromosomes 1A (2), 2D, 3A, 4B and 5B (2) exhibiting pleiotropic effects. In particular, clusters on chromosomes 2D and 5B possessing significant QTL for kernel-related traits were highlighted. Markers tightly linked to these QTL or clusters will eventually facilitate further studies for fine mapping, candidate gene discovery and marker-assisted selection (MAS) in wheat breeding.
Introduction
Bread wheat (Triticum aestivum L.) is one of the leading cereal crops worldwide, which plays a crucial role in sustaining food security. The genetic improvement of three yield components, i.e., productive spikes per unit area, kernel number per spike and kernel weight, contributed a great deal to the increase of wheat yield level and alleviation of food crisis in the last decades (Sayre et al., 1997). Among the three yield components, kernel weight showed the highest heritability (Alexander et al., 1984), and selection for this component in the early generations of breeding was highly effective (Xiao and He, 2003; Brown et al., 2009). For instance, thousand kernel weight (TKW) of Chinese wheat mini core collection (262 accessions) increased from a mean 31.5 g in 1940s to 44.64 g in 2000s, representing a 2.19 g increase in each decade (Wang L. et al., 2012).
Thousand kernel weight is closely associated with kernel size traits, such as kernel length (KL), kernel width (KW), and kernel diameter ratio (KDR) (Campbell et al., 1999; Dholakia et al., 2003). And kernel size traits usually contribute to yield by affecting the TKW and can also be associated with milling and processing (Osborne and Anderssen, 2003). Therefore, improving kernel weight and size is a prime breeding target for wheat yield potential and end use quality.
Thousand kernel weight and kernel size are complex quantitative traits controlled by multiple genes and significantly influenced by the environment (Giura and Saulescu, 1996; Ammiraju et al., 2001). Quantitative trait loci (QTL) mapping is a key approach to understand the genetic architecture of kernel traits. Great progress has been made in identifying major QTL and isolating underlying genes for kernel weight and size in cereal crops, such as rice, maize (Li et al., 2010) and barley (Ayoub et al., 2002), etc. For rice, in particular, lots of genes controlling the kernel size and weight have been characterized, like GS3 (Fan et al., 2006), GS5 (Li et al., 2011), qGL3 (Zhang X. et al., 2012), GW2 (Song et al., 2007), and GW8 (Wang S. et al., 2012). Recently, Xu et al. (2018) reported that the OsMKKK10-OsMKK4-OsMAPK6 signaling pathway positively regulates grain weight and size in rice. Results of these studies revealed that grain yield was controlled by genes related to kernel size traits.
Compared with rice, the molecular cloning of genes associated with kernel weight and size has lagged behind in wheat. Up to date, none gene/QTL associated with kernel weight and size have been cloned in wheat via the map-based cloning approach. And most of them, such as TaCwi-A1 (Ma et al., 2012), TaCKX6-D1 (Zhang L. et al., 2012), TaGS1 (Guo et al., 2013), TaGS5 (Ma et al., 2016), and TaGW2 (Su et al., 2011) were cloned through homologous cloning. Although multiple major and stable QTL controlling kernel shape and size were identified on chromosomes 2A (Wu et al., 2015), 2D (Breseghello and Sorrells, 2007; Ramya et al., 2010; Cui et al., 2016), 4A (Cui et al., 2016), 5A (Wu et al., 2015), 5B (Breseghello and Sorrells, 2007; Ramya et al., 2010; Li et al., 2015) and 6A (Zhang et al., 2013), they were defined in a relatively large chromosome interval due to the limited numbers of markers.
Single nucleotide polymorphism (SNP) is the most abundant type of molecular markers. During the past 5 years, increasing numbers of SNPs have been discovered in hexaploid wheat. For example, Cavanagh et al. (2013) released a consensus genetic map with 7504 SNP markers from the Wheat9K SNP array using a combination of seven mapping populations. Wang et al. (2014) mapped 46,977 SNP markers from the Wheat90K array to the wheat genetic map using a combination of eight mapping populations. Winfield et al. (2016) documented a consensus map with 56,505 SNP markers from the Wheat820K array, using three independent bi-parental populations. Recently, using Wheat660K array, Cui et al. (2017) released a high-density genetic map with 119,566 markers (including 119,001 SNP markers) based on an individual mapping population. Using the high-throughput SNP genotyping, more and more wheat QTL for kernel traits have been high-solution mapped.
In the present study, a high-density genetic linkage map based on iSelect 90K SNP and SSR markers was constructed using the Shixin828/Kenong2007 recombinant inbred line (RIL) population. Both unconditional and conditional QTL analysis were conducted to investigate the underlying genetic basis of TKW and kernel size as well as to dissect the genetic relationships between them at QTL level. The information obtained from this study could provide further insights into the genetic factors that influence kernel traits.
Materials and Methods
Plant Materials and Field Trials
An F6:7 RIL population derived from a cross between Shixin828 (SX828) and Kenong2007 (KN2007) (denoted by SK-RIL) was developed in this study. SX828 was released in 2005 and has been one of the major cultivars in the North China Plain in the last decade. It has superior photosynthesis characteristics during filling stage (Bi et al., 2010), thus shows higher grain-filling rate and larger kernel than most other commercial cultivars. KN2007, on the other hand, is a small kernel line derived from Kenong9204 (Cui et al., 2011). In the present study, 163 SK-RILs were used for SNP and SSR genotyping, genetic linkage analysis and QTL detection.
The SK-RILs and their parents were evaluated in eight environments: 2014–2015 in Shijiazhuang (37°53′N, 114°41′E, altitude 54 m) with high nitrogen (HN) trial; 2015–2016 in Shijiazhuang with both HN and low nitrogen (LN) trials; 2016–2017 in Shijiazhuang with both HN and LN trials, Anyang (35°12′N, 113°37′E, altitude 77 m) with HN trial, Beijing (40°06′N, 116°24′E, altitude 51 m) with both HN and LN trials. These 8 environments were designated as E1, E2, E3, E4, E5, E6, E7, and E8, respectively. The soil nitrate-nitrogen (N) contents within the 0–20 cm layer in each environment were measured after harvest (Supplementary Table S1). In each HN plot, 300 kg ha-1 of diamine phosphate and 225 kg ha-1 of urea were applied before sowing, and 150 kg ha-1 of urea was applied at the elongation stage every year. In the LN plots, no nitrogen fertilizer was applied throughout the growing period. The materials were planted in randomized complete blocks with two replications for each of the 8 environments. Each block contained two rows that were 2 m long and 0.25 m apart and 40 seeds were evenly planted in each row. All of the recommended agronomic practices were followed in each of the trials except for the nitrogen fertilization treatment described above.
Phenotypic Evaluation and Statistical Analysis
Five representative plants in the center of the second row were randomly sampled at physiological maturity for phenotypic evaluation. Kernel traits including KL, KW, KDR, and TKW were evaluated for 200 random kernels from all tiller spikes of each representative plants using the Seed Counting and Analysis System of WSeen SC-G Instrument (Zhejiang, China)1. The spike number per plant (SNPP) and kernel number per spike (KNPS) were also investigated in the 8 environments detailed above. SNPP was determined by the mean of the five representative plants, while KNPS was determined by the mean of the main spikes of the five representative plants.
The analysis of variance (ANOVA) and the calculation of phenotypic data correlation coefficients between all investigated traits were performed with SPSS 20.0 (SPSS, Chicago, IL, United States)2. Broad sense heritability () of the corresponding traits was estimated based on the following formula: = VG/VP; where VG and VP are the genetic variance and phenotypic variance, respectively. The conditional phenotypic values of TKW with respect to TKW component (TKWC) were evaluated using QGAStation 2.03 according to Zhu (1995) and Fan et al. (2015). The raw data from each environment were assembled as follows: the first column represented the block (replications), the second column represented the genotype (163 SK-RILs), and the following columns were trait data, specifically the TKWC and TKW. ‘Conditional Final’ was conducted and the output file provided information on conditional phenotypic values of y(TKW|TKWC), which indicates TKW conditioned on TKWC (for example, TKW| KL means TKW was conditioned on KL). Both unconditional and conditional phenotypic values were used for QTL mapping analyses.
Genotyping and Linkage Map Construction
The 163 SK-RILs together with their two parents were genotyped using the Illumina iSelect 90K SNP Array containing 81,587 wheat SNP markers from CapitalBio Corporation (Beijing, China)4. In addition, 225 SSR markers were used to anchor the linkage groups into specific chromosomes better. Marker allele frequency < 0.3 or containing > 10% missing data were rejected. The remaining markers were binned based on the pattern of segregation in the SK-RILs using the BIN function of IciMapping 4.1.5 Unique markers from each bin with least missing data were further identified and sorted into groups using the MAP function of IciMapping 4.1, with a LOD score of 3.5 and a recombination fraction of 0.3 using the Kosambi mapping function. Groups were ordered with Kosambi mapping function of JoinMap v. 4.0 software using a LOD score ≥ 3 after preliminary analysis using a LOD score ranging from 2 to 10. MapChart2.26 was used to draw the genetic linkage map. For the redundant loci that showed co-segregation in the 163 SK-RILs, only one unique informative marker is shown. The short chromosome arms are on the top.
QTL Analysis
The SK-RIL population derived genetic linkage map was used to screen QTL in this study. The average trait phenotypic values of two replicates under each environment (E1–E8) were used for individual environment QTL analysis. The inclusive composite interval mapping (ICIM) performed with IciMapping 4.1 was conducted to detect putative additive QTL. Moreover, Multi-environment Traits (MET) analysis was employed for combined QTL analysis across environments to verify the QTL identified in the individual environment and evaluate the QTL × environment interactions. The conditional phenotypic values evaluated by QGAStation 2.0 were used for conditional QTL mapping analysis. The missing phenotypic data were deleted using the ‘Deletion’ command. The walking speed for all QTL was 1.0 cM, and the P-value inclusion threshold was 0.001. The threshold LOD scores were calculated using 1000 permutations with a type I error of 0.05 (Doerge and Churchill, 1996; Li et al., 2007). A suggestive QTL with an average LOD value > 2.5 in a data set was shown. A QTL with an average LOD value > 3.0 and average phenotypic variance contribution > 10% was defined as a major QTL, and one showing significance in at least four environments sets was considered a stable QTL (Lander and Kruglyak, 1995; Cui et al., 2016).
Results
High-Density Genetic Linkage Map Construction
Genotyping of the SX828/KN2007 RIL population with the iSelect 90K SNP array resulted in 10,638 (13.04%) polymorphic markers. In addition to the SNP markers, 225 SSR markers were also used for the linkage analysis. After removing ambiguous and unlinked markers, a genetic linkage map with 6312 markers (including 6130 SNP markers and 182 SSR markers) was constructed, which was within 2672 unique loci, spanning 3049.4 cM in length with an average marker density of 1.1 cM/locus, covering 21 wheat chromosomes (Table 1, Supplementary Table S2, and Supplementary Figure S1). Of the 6130 SNP markers, 6118 (99.8%) were best hits to 4100 Chinese Spring (CS) sequence contigs, with 1.5 polymorphic markers per contig. In total, 79.8% of the contigs had coincident physical and genetic positions, while 16.6% of the contigs were mapped to the homoeologous chromosomes such as 1A in physical position but 1B in the SK-RIL genetic map, and the rest of 3.6% were disordered (Supplementary Table S3). All together, of the 6312 high-quality polymorphic markers, 2565 (40.6%) were localized to the A genome spanning 1059.1 cM with an average marker density of 0.41 cM/locus, 2919 (46.2%) were mapped to the B genome covering 1140.7 cM with an average marker density of 0.39 cM/locus, and 828 (13.2%) were mapped to the D genome spanning 849.6 cM with an average marker density of 1.03 cM/locus. 86.8% of the markers in total mapped to the A and B genomes, revealed higher polymorphisms of the A and B genomes than that of the D genome. The 6312 markers distributed unevenly on the 21 chromosomes, ranging from 18 on chromosome 4D to 790 on chromosome 5B. Chromosomes 1B, 2D, and 5D were integrated by two linkage groups, respectively. Ten gaps (>20 cM) were found on chromosomes 1D, 2B (2), 3B, 4B, 5D, 6D, 7B and 7D (2) (Supplementary Table S2 and Supplementary Figure S1).
Phenotypic Variation and Correlation Analysis
The phenotypic values of the SK-RILs and parents are shown in Table 2. In all eight tested environments, the parent SX828 exhibited higher TKW and longer kernel than that of the other parent KN2007, while KN2007 had wider kernel than SX828. All of the investigated traits manifested continuous segregation in the RIL population, and the most absolute values of skewness and kurtosis for all investigated traits were less than 1.0, indicating normal distribution and involvement of multiple genes for these traits. Strong transgressive segregation exceeding the limits of both parents was observed, suggesting that alleles with positive effects were distributed between the two parents. High value of broad sense heritability () for all the investigated traits suggested that genetic factor played an important role in the formation of these traits (Table 2).
Pearson’s coefficients of correlation were calculated for all the traits based on the average data of the eight environments (Supplementary Table S4). TKW was significantly and positively correlated with KL and KW. The correlation coefficient of KW-TKW (r = 0.696, P < 0.01) was higher than that of KL-TKW (r = 0.592, P < 0.01), suggesting that KW should be the main contributor to the increased grain weight. Furthermore, SNPP was significantly and positively correlated to KDR but negatively correlated to KW, while KNPS was significantly negatively correlated to KL, TKW and SNPP.
Putative Additive QTL for Kernel Traits
A total of 78 putative additive QTL associated with KL, KW, KDR, and TKW were detected in the eight individual environments (Table 3 and Supplementary Table S5). It was found that these QTL were distributed across all wheat chromosomes except for 3D, 4D, and 7D. Of these, 32 QTL were mapped to the A genome, 30 to the B genome and 16 to the D genome. These QTL individually explained 3.95–16.57% of the phenotypic variance with LOD value ranging from 2.60 to 10.59. Thirty-three QTL (42.31%) were reproducibly detected in at least two environments. Sixteen QTL (20.51%) individually accounted for more than 10% of the phenotypic variance with an average LOD value > 3.0 (defined as major QTL); three of these (qKW-2D.1, qKDR-2D.1 and qTKW-5B.2) also showed stability across more than four environments and were characterized as major and stable QTL. All of the six stable QTL identified in the individual environment were verified in combined analysis across eight environments (Table 3).
Eighteen QTL for KL were identified on chromosomes 1A, 1D, 2A, 2B (3), 2D, 3A (2), 3B, 4A, 5B (3), 6B (2), 7A and 7B, respectively, with individual QTL explaining 4.14–16.56% of the phenotypic variance. Of these QTL, 14 carried the favorable alleles increasing KL from SX828. Two stable QTL, qKL-2D and qKL-6B.2, individually showed significant effects in six and five environments and exhibited 4.27–10.14% and 4.89–12.25% of the phenotypic variance, respectively. Favorable alleles of the two QTL were contributed by SX828.
Twenty QTL for KW were detected on chromosomes 1A (2), 1D, 2D (2), 3A, 3B (2), 4A, 4B (3), 5A, 5B, 5D, 6A (2), 6D and 7B (2), respectively, with individual QTL explaining 4.63–15.51% of the phenotypic variance. Alleles increasing KW at 12 loci were derived from the parent KN2007. For qKW-2D.1, a major and stable QTL exhibiting 7.01–18.82% of the phenotypic variance with LOD value of 3.59–10.25, the favorable allele was derived from KN2007. For qKW-4B.1 and qKW-5B which were repeatedly identified in at least three different environments, the alleles increasing KW were from SX828.
Nineteen QTL for KDR were identified on chromosomes 1D (3), 2B (2), 2D (2), 3A, 4A (2), 5A (3), 5B (2), 6A (3) and 6B respectively, with individual QTL explaining 4.17–16.57% of the phenotypic variance. Of these, 10 QTL were repeatedly identified in at least two different environments. Except for qKDR-2B.1, all of the alleles that increased KDR in these loci came from SX828. qKDR-2D.1, co-localized with qKL-2D and qKW-2D.1 and flanked by markers Xcfd168 and BobWhite_c7149_371, was identified in all eight environments and explained 13.78–18.22% of the variation in KDR with LOD value of 7.83–12.81.
Twenty-one QTL for TKW were detected on chromosomes 1A (2), 1B, 2A (2), 2B, 2D (3), 3A (3), 3B, 4B, 5A, 5B (3), 6A, 6D and 7A, respectively, with individual QTL explaining 3.95-15.34% of the phenotypic variance. qTKW-5A and qTKW-5B.2 were significant in at least four environments, individually exhibiting 4.60–8.07% and 10.04–24.41% of the phenotypic variance, respectively. qTKW-5B.2, a major and stable QTL, was co-localized with qKL-5B.1 and the favorable allele from SX828 simultaneously increased TKW and KL.
Clusters With Co-located QTL for Kernel-Related Traits
Overview of the identified QTL for kernel traits in this study, 7 QTL clusters (C1–C7) comprising 17 QTL were scattered on chromosomes 1A (two clusters), 2D, 3A, 4B and 5B (two clusters) (Table 4 and Supplementary Figure S1). Among the clusters, QTL for TKW were always identified together with QTL for kernel size and shape, except for C3 where only QTL for KL, KW, and KDR were co-located.
C3 was positioned on chromosome 2D with co-existed qKL-2D, qKW-2D.1, and qKDR-2D.1. The stable QTL qKL-2D was detected in six environments, with SX828-derived alleles increasing KL and KDR. The major and stable QTL qKW-2D.1 was reproducibly identified in seven environments, with the favorable alleles from KN2007 increasing KW. The stable QTL qKDR-2D.1 showed significance in all eight environments. Interestingly, the positive alleles of co-located QTL for KL and KW in C3 were derived from opposite parent, which may result in no significant QTL for TKW identified.
In the other six clusters, the positive alleles of the co-located QTL for KL/KW/KDR and TKW were consistently contributed by single parent. For C1 and C2 on chromosome 1A, qTKW-1A.1 and qTKW-1A.2 were reproducibly identified in different environments, while QTL for KW in the two clusters were significant in only one environment. Favorable alleles of those QTL were all from KN2007. For C4 on chromosome 3A, a major QTL qTKW-3A.1 was clustered with a QTL qKW-3A, with the KN2007-derived alleles simultaneously increasing KW and TKW. For C5 on chromosome 4B, qKW-4B.1 was co-localized with qTKW-4B, with the favorable alleles from SX828 increasing KW and TKW. For C6 on chromosome 5B, qKW-5B showed significance in three environments and clustered with qKDR-5B.1 and qTKW-5B.1, with favorable alleles from SX828. For C7 on chromosome 5B, qKL-5B.1, qKDR-5B.2 and qTKW-5B.2 were clustered. The major and stable QTL qTKW-5B.2 was expressed in five environments, with alleles from SX828 simultaneously increasing KL, KDR, and TKW.
Conditional QTL for TKW With Respect to TKWC
To dissect the genetic effects of TKWC on the expression of QTL for the TKW that were detected in the aforementioned 7 clusters, conditional QTL mapping analysis for the TKW was conducted with respect to TKWC.
Unconditional and conditional QTL mapping results revealed that KL, KW, and KDR played different roles in the expression of QTL for TKW detected in C1-C7 (Table 5). qTKW-1A.1, qTKW-3A.1, and qTKW-4B.1 were found to be related to the variation of all three elements. qTKW-1A.2 and qTKW-5B.1 were entirely contributed by KW and KDR and partially contributed by KL. qTKW-5B.2 was found entirely due to the variation of KL and in part to the variation of KW and KDR. qTKW-2D.4 was a new QTL for TKW only detected in conditional QTL analysis (Table 5). When TKW conditional on the KL (TKW| KL), i.e., removing off the effect of KL on TKW, the additive effect of qTKW-2D.4 was derived from KN2007. Likewise, using the conditional data of TKW| KW, the additive effect of qTKW-2D.4 was derived from SX828.
Discussion
High-Density Genetic Linkage Map Construction
SNP markers enable construction of high-density genetic linkage maps and identification of QTL for complex agronomic traits in crop plants. In the present study, a genetic linkage map was constructed comprising 6130 SNP markers and 182 SSR markers (Table 1, Supplementary Table S2, and Supplementary Figure S1). It is noteworthy that 951 (15.5%) SNP markers of iSelect 90K array were newly mapped (Supplementary Table S6). Order of the SNP in the present genetic map was in good agreement with that in the recently released wheat genome assembly (Supplementary Table S3). Notably, the high number (10,638) of polymorphic SNP markers between SX828 and KN2007 is comparable to Gao et al. (2015) and Zhai et al. (2016), who detected 7514 and 11,646 polymorphic SNPs between the two parental lines by iSelect 90K array, respectively. The relatively high SNP polymorphism in our mapping population confirmed the genetic divergence between the two parental lines. The genetic length of this map is 3049.4 cM, similar to the reported maps in hexaploid wheat (Somers et al., 2004; Wu et al., 2015; Zhai et al., 2016). In consequence, the current genetic map based on iSelect 90K array would be good enough for QTL mapping.
The number of markers on each genome was uneven. Markers for the A (40.6%) and B (46.2%) genomes were more abundant than those for the D genome, consistent with previous studies (Somers et al., 2004; Chao et al., 2009; Wang et al., 2014), and this is attributed to the low level of polymorphism in the D genome of hexaploid wheat. D genome is a recent evolutionary addition to the hexaploid wheat genome and there has been limited gene flow Aegilops tauschii Coss. and T. aestivum (Dubcovsky and Dvorak, 2007), possibly explaining the low polymorphism rate.
Response of QTL for Kernel Traits to Divergent Nitrogen Supply
Nitrogen (N) is an essential mineral nutrient required by crop plants. Sufficient N supply usually resulted in increased productive tillers, kernel number per spike and grain yield, which are frequently accompanied with smaller kernel size and lower kernel weight (Sinclair and Jamieson, 2006; Makino, 2011). In our study, SNPP were negatively correlated with KW, and KNPS exhibited significant negative correlation with KL and KW (Supplementary Table S4). Most values of kernel size and kernel weight of SK-RILs under LN supplies were higher than those under HN supplies, which is in agreement with the negative correlation between grain size and nitrogen supplies (Habash et al., 2007; Makino, 2011).
Genomic regions detected under a specific nitrogen treatment are more probably involved in wheat adaptation to the corresponding environment (Laperche et al., 2007; Fan et al., 2015). It can be seen that five stable QTL (qKL-2D, qKL-6B.2, qKW-2D.1, qKDR-2D.1 and qTKW-5B.2) for kernel traits were expressed in both HN and LN environments (Table 3 and Supplementary Tables S5, S7), indicating that the expression of these QTL were less sensitive to nitrogen supply. 36 QTL including one stable QTL qTKW-5A were only detected under HN condition means that the expression of these QTL were induced by HN supply (Supplementary Tables S5, S7). Seventeen QTL were expressed only under LN condition indicates that these QTL were adapting to nitrogen constraint (Supplementary Table S5). Notably, the qTKW-5A is valuable in obtaining higher grain weight under the sufficient N supply management, and the allele from SX828 may increase the nitrogen uptake and use efficiency (Supplementary Table S7).
Pleiotropic Cluster on Chromosomes 2D
Associative traits are prone to share regions with significant QTL. Several genetic loci simultaneously controlling KL and KW were identified on chromosome 2D in cluster 3 (Table 4 and Figure 1). These loci have also been highlighted in other studies. Campbell et al. (1999) firstly reported a RFLP marker linked to KL on 2D, later Breseghello and Sorrells (2006) detected a KL-associated 2D marker Xgwm539, which is close to qKL-2D.1 in our study. However, Ramya et al. (2010) reported that Xgwm539 was linked to QTkw.ncl-2D.2 and QKw.ncl-2D.2. In our study, QTL in cluster 3 were only for KL and KW with no significant effect on TKW (Table 4, Supplementary Table S5, and Supplementary Figure S1). Nevertheless, when the influence of KL or KW on TKW was excluded, a stable QTL (qTKW-2D.4) for TKW was detected (Table 5), suggesting a tension or tradeoff between the two kernel dimensions. This finding is not at all accidental. In previous QTL analyses, increasing effects of QTL on respective traits have frequently been supplied by different parents in an opposite manner (Liu et al., 2014; Cheng et al., 2017). A comparison of the QTL on chromosome 2D detected in the present study to those identified in previous studies showed that these important QTL were located at an approximately equivalent or adjacent chromosomal region (Supplementary Figure S2). Moreover, Rht8, one of gibberellin-responsive (GAR) dwarfing genes tightly linked with WMC503 and XGWM261 (Korzun et al., 1998; Du et al., 2018), was mapped on chromosome 2DS (Supplementary Figure S1). There was a large interval between cluster 3 and WMC503/XGWM261. Thus, we suppose there is no linkage between the C3 (Table 4) and Rht8 in our study. This region of chromosome 2D should be good donor for improving kernel size, to which should be paid more attention in wheat breeding programs.
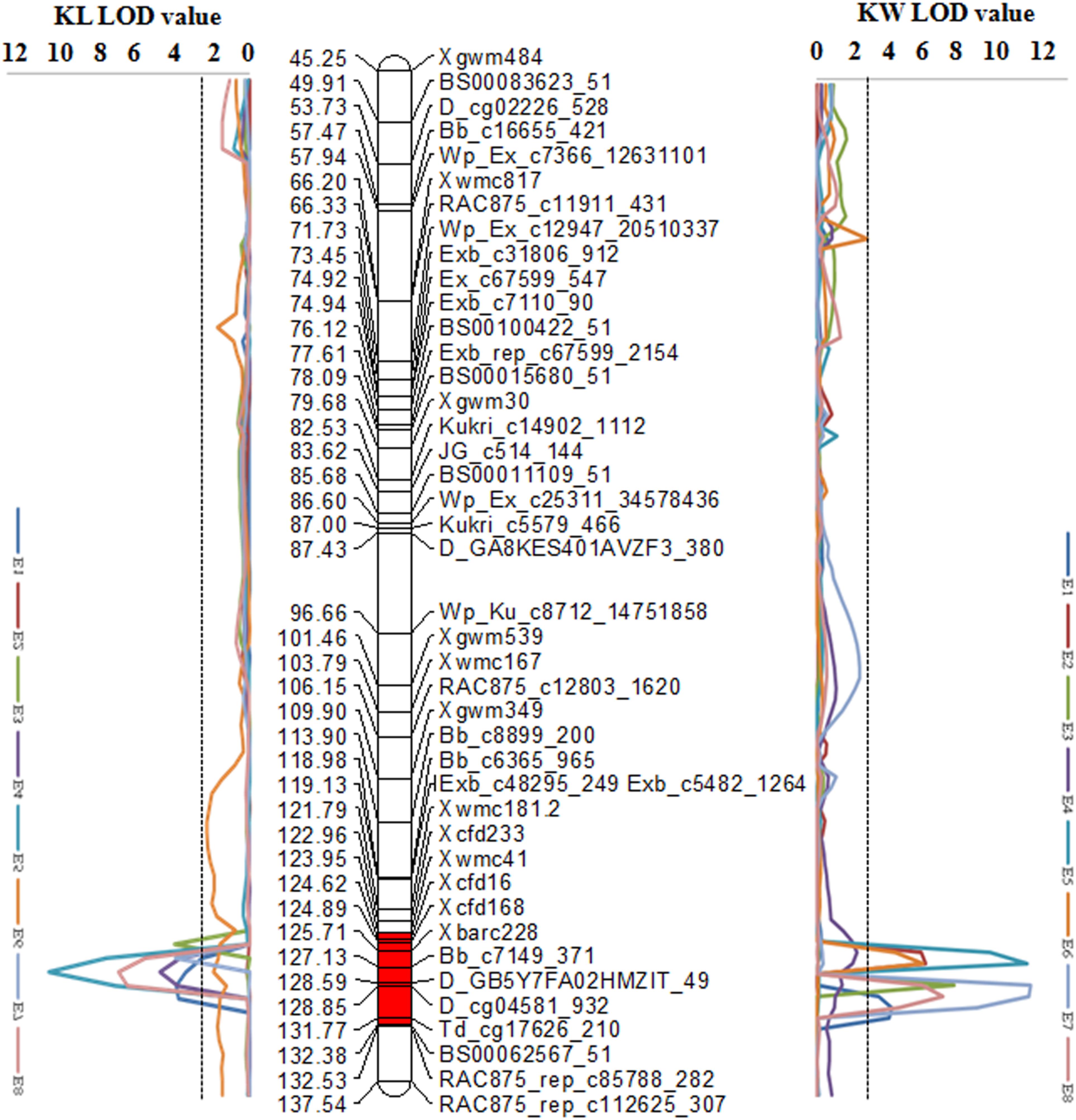
FIGURE 1. The LOD value of QTL on chromosome 2D for kernel length and kernel width in eight environments. The LOD value of black dashed line is 2.5. The map on the left is the LOD value of QTL for kernel length in eight environments; the map on the middle is the high-resolution genetic linkage map of chromosome 2D; the map on the right is the LOD value of QTL for kernel width in eight environments.
We previously mapped TaGS2-D1 to the same region on 2DL in the KJ-RIL map (Cui et al., 2014) and found that TaGS2-D1 from KN9204 had positive effects on KW, KDR and TKW (Cui et al., 2016). Therefore, we used IN10, one functional marker of GS2, to genotype SX828 and KN2007 and detected two banding patterns. KN2007 and KN9204 displayed identical banding pattern while SX828 and J411 were the same (Supplementary Figure S3), which implied that KN2007 might share the same chromosome interval of 2D as KN9204, thus the C3 on chromosome 2D of this study (Table 4) might harbor the similar QTL related with kernel size on chromosome 2D of the KJ-RIL population (Cui et al., 2016). As KN2007 was derived from KN9204 and the favorable allele qKW-2D was donated by KN2007, the accomplishing of whole genome sequence assembly of KN9204 (not published) will benefit fine-mapping of this major QTL cluster.
QTL for TKW on Chromosome 5B and the Prediction of Candidate Genes
Another notable cluster C7 on chromosomes 5B consisted of a range of QTL affecting KL, KDR and TKW (Table 4). The results of conditional QTL mapping in the present study also indicated that qTKW-5B.2 was entirely explained by KL and partially contributed by KW (Table 5). In addition, qTKW-5B.2 was detected when conditional analysis was conducted on SNPP and KNPS (Supplementary Table S8). These data suggested that the expression of qTKW-5B.2 was primarily dependent on variation of KL. A number of QTL for TKW and kernel size on chromosome 5B have been reported in previous studies (Groos et al., 2003; Breseghello and Sorrells, 2006). Among them, QTkw.ncl-5B.2 and QTw-5B.1 associated with Xgwm213 were located on the 5BL (Ramya et al., 2010); QTkw5B.1-12 and QKw5B.1-12 were located on 5B (Li et al., 2015). Sarma et al. (2000) reported two possible loci affecting flowering time on the long arm of the 5B chromosome (Vrn-B1 and Eps). Tóth et al. (2003) found that Vrn-B1 was closely linked to the SSR marker Xgwm604 and Eps-5BL2 was linked to the SSR locus Xwmc73. However, Xgwm604 and Xwmc73 were no polymorphism in our SK-RILs (Supplementary Figure S4). Thus, we supposed this loci should not be association with flowering time in our study. After comparing the position of the marker intervals, qTKW-5B.2 identified in the SK-RIL population was considered preliminarily as a new QTL for its large distance from other reported QTL. Therefore, further fine mapping of the putative pleiotropic QTL in this interval is of great value.
qTKW-5B.2 was repeatedly identified using various softwares based on different mathematical models (Table 6 and Figure 2). The peak position of this QTL was found in the BS00050775_51 – IAAV3126 overlapping confidence interval of 0.7 cM. Based on the genome sequence assembly of T. aestivum cv. Chinese Spring7, the overlapping confidence intervals of BS00050775_51 - IAAV3126 spanned 45.41 Mb (5B: 236261222 – 5B: 281675808) in physical position (Supplementary Table S3) with 357 predicted protein coding genes in wheat (Supplementary Figure S5). This information is very valuable for future high-resolution mapping and map-based cloning of qTKW-5B.2.
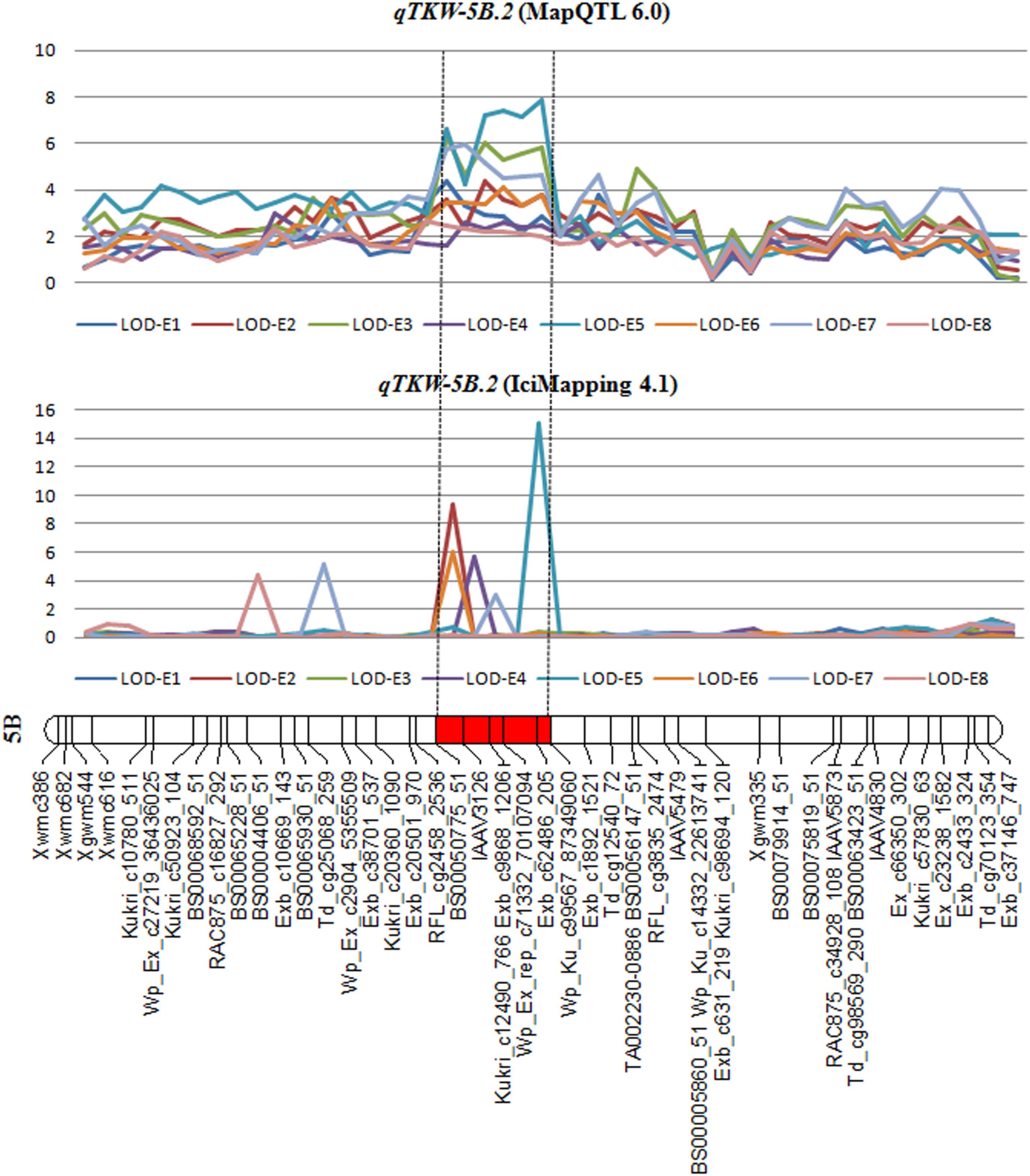
FIGURE 2. Overlapping confidence interval of QTL for TKW based on MapQTL 6.0, IciMapping 4.1 and QTLNetwork 2.0. The segment in red is the overlapping confidence interval of qTKW-5B.
Using BS00050775_51 as a probe, the 163 SK-RILs were divided into two groups, one group with allele from SX828 and the other with allele from KN2007, to perform mean comparison regarding TKW. The positive allele of qTKW-5B.2 from SX828 increased TKW value of 2.1–3.5 g, indicating a tremendous potential for its application in wheat molecular breeding programs designed to increase grain output (Supplementary Figure S6).
Other Important Clusters Associated With Kernel Traits
Genes and QTL for multiple kernel shape and size are usually linked or show pleiotropic effects. Several genetic loci simultaneously controlling KW and TKW were identified in the C1 on chromosome 1A in our study (Table 4). This result is in consistence with the report of Ramya et al. (2010), who detected a QTL controlling TKW between markers Xwmc24 and Xgwm357 in the same genetic region.
Cluster 4, simultaneously facilitated KW and TKW, was mapped on chromosome 3A, and most likely corresponds to TaGS5 (Ma et al., 2016). TaGS5, an ortholog of rice grain size gene OsGS5, is associated with kernel width and kernel weight in wheat. OsGS5 which encodes a putative serine carboxypeptidase, promotes cell division by regulating cell cycle genes resulting in large grain size generated by an increased cell number (Li et al., 2011). The high homology of OsGS5 and TaGS5-3A suggests a similar function in wheat.
QTL for KW and TKW were co-located in cluster 5 on chromosome 4B. The same genomic region was found associated with QTL for KL, KW and TKW in the Chinese winter wheat line Yanda1817 (Wu et al., 2015). Kumar et al. (2016) also identified a QTL (QTL-15) in the same region related to KW, KA (kernel area) and TKW, and proposed this genomic region could harbor an ortholog of rice gene GS3 (Huang et al., 2013) which encodes a putative protein phosphatase with a Kelch-like repeat domain (OsPPKL1) and has positive effect on kernel size and weight (Zhang X. et al., 2012). Thus, this region is noteworthy for genetic improvement of kernel weight and size in wheat.
Conclusion
A high-density genetic linkage map was constructed for the Shixin828/Kenong2007 RIL population using the iSelect 90K SNP array, which is in good accordance with the recently released CS wheat genome assembly. This genetic map was proved powerful for mapping QTL of kernel size and weight. As results, two important QTL clusters on chromosomes 2D and 5B that are associated with TKW and kernel size were identified by unconditional and conditional analyses. These QTL clusters could serve as target regions for fine mapping and MAS in wheat breeding.
Author Contributions
QS, WZ, and JuL designed the research. QS, WZ, LL, and XlZ conducted genotyping of the SK-RIL population. QS, WZ, XlZ, LL, LZ, NZ, LS, XX, GL, JjL, DM, JJ, XuZ, CY, YT, and JmL conducted phenotyping of the SK-RIL population. QS, XlZ, NZ, WZ, ZL, and JuL analyzed the data and wrote the paper. WZ and JuL had primary responsibility for final content. All authors read and approved the final manuscript.
Funding
This research was supported by grants from the National Key Research and Development Program of China (2017YFD0100600), the National Natural Science Foundation of China (31671673), the Science and Technology Service Network Initiative Program of the Chinese Academy of Sciences (KFJ-STS-ZDTP-024), Hebei Provincial Science and Technology Research and Development Project (16226320D), and China Agriculture Research System (CARS-03).
Conflict of Interest Statement
The authors declare that the research was conducted in the absence of any commercial or financial relationships that could be construed as a potential conflict of interest.
Supplementary Material
The Supplementary Material for this article can be found online at: https://www.frontiersin.org/articles/10.3389/fpls.2018.01484/full#supplementary-material
Footnotes
- ^http://hzwseen.foodmate.net/
- ^http://en.wikipedia.org/wiki/SPSS
- ^http://ibi.zju.edu.cn/software/qga/
- ^http://www.capitalbio.com
- ^http://www.isbreeding.net
- ^http://www.biometris.nl/uk/Sofware/MapChart/
- ^https://urgi.versailles.inra.fr/download/iwgsc/IWGSC_RefSeq_Assemblies/v1.0/
References
Alexander, W. L., Smith, E. L., and Dhanasobhan, C. (1984). A comparison of yield and yield component selection in winter wheat. Euphytica 33, 953–961. doi: 10.1007/BF00021926
Ammiraju, J. S., Dholakia, B. B., Santra, D. K., Singh, H., Lagu, M. D., Tamhankar, S. A., et al. (2001). Identification of inter simple sequence repeat (ISSR) markers associated with seed size in wheat. Theor. Appl. Genet. 102, 726–732. doi: 10.1007/s001220051703
Ayoub, M., Symons, S., Edney, M., and Mather, D. (2002). QTLs affecting kernel size and shape in a two-rowed by six-rowed barley cross. Theor. Appl. Genet. 105, 237–247. doi: 10.1007/s00122-002-0941-1
Bi, C., Bai, Z., Li, C., Zheng, J., and Tang, G. (2010). Effects of planting density on photosynthetic characteristics and grain yield of a wheat cultivar Shixin828. Acta Agric. Boreali Sin. 25, 165–169.
Breseghello, F., and Sorrells, M. E. (2006). Association mapping of kernel size and milling quality in wheat (Triticum aestivum L.) cultivars. Genetics 172, 1165–1177. doi: 10.1534/genetics.105.044586
Breseghello, F., and Sorrells, M. E. (2007). QTL analysis of kernel size and shape in two hexaploid wheat mapping populations. Field Crops Res. 101, 172–179. doi: 10.1016/j.fcr.2006.11.008
Brown, T. A., Jones, M. K., Powell, W., and Allaby, R. G. (2009). The complex origins of domesticated crops in the Fertile Crescent. Trends Ecol. Evol. 24, 103–109. doi: 10.1016/j.tree.2008.09.008
Campbell, K. G., Bergman, C. J., Gualberto, D. G., Anderson, J. A., Giroux, M. J., Hareland, G., et al. (1999). Quantitative trait loci associated with kernel traits in a soft × hard wheat cross. Crop Sci. 39, 1275–1285. doi: 10.2135/cropsci1999.00111
Cavanagh, C. R., Chao, S., Wang, S., Huang, B. E., Stephen, S., Kiani, S., et al. (2013). Genome-wide comparative diversity uncovers multiple targets of selection for improvement in hexaploid wheat landraces and cultivars. Proc. Natl. Acad. Sci. U.S.A. 110, 8057–8062. doi: 10.1073/pnas.1217133110
Chao, S., Zhang, W., Akhunov, E., Sherman, J., Ma, Y., Luo, M. C., et al. (2009). Analysis of gene-derived SNP marker polymorphism in US wheat (Triticum aestivum L.) cultivars. Mol. Breed. 23, 23–33. doi: 10.1007/s11032-008-9210-6
Cheng, R., Kong, Z., Zhang, L., Xie, Q., Jia, H., Yu, D., et al. (2017). Mapping QTLs controlling kernel dimensions in a wheat inter-varietal RIL mapping population. Theor. Appl. Genet. 130, 1405–1414. doi: 10.1007/s00122-017-2896-2
Cui, F., Fan, X., Chen, M., Zhang, N., Zhao, C., Zhang, W., et al. (2016). QTL detection for wheat kernel size and quality and the responses of these traits to low nitrogen stress. Theor. Appl. Genet. 129, 469–484. doi: 10.1007/s00122-015-2641-7
Cui, F., Fan, X., Zhao, C., Zhang, W., Chen, M., Ji, J., et al. (2014). A novel genetic map of wheat: utility for mapping QTL for yield under different nitrogen treatments. BMC Genet. 15:57. doi: 10.1186/1471-2156-15-57
Cui, F., Zhang, N., Fan, X., Zhang, W., Zhao, C., Yang, L., et al. (2017). Utilization of a Wheat660K SNP array-derived high-density genetic map for high-resolution mapping of a major QTL for kernel number. Sci. Rep. 7:3788. doi: 10.1038/s41598-017-04028-6
Cui, Z., Zhang, F., Chen, X., Li, F., and Tong, Y. (2011). Using in-season nitrogen management and wheat cultivars to improve nitrogen use efficiency. Soil Sci. Soc. Am. J. 75, 976–983. doi: 10.2136/sssaj2010.0117
Dholakia, B. B., Ammiraju, J. S. S., Singh, H., Lagu, M. D., Röder, M. S., Rao, V. S., et al. (2003). Molecular marker analysis of kernel size and shape in bread wheat. Plant Breed. 122, 392–395. doi: 10.1046/j.1439-0523.2003.00896.x
Doerge, R. W., and Churchill, G. A. (1996). Permutation tests for multiple loci affecting a quantitative character. Genetics 142, 285–294.
Du, Y., Chen, L., Wang, Y., Yang, Z., Saeed, I., Hu, Y., et al. (2018). The combination of dwarfing genes Rht4 and Rht8 reduced plant height, improved yield traits of rainfed bread wheat (Triticum aestivum L.). Field Crops Res. 215, 149–155. doi: 10.1016/j.fcr.2017.10.015
Dubcovsky, J., and Dvorak, J. (2007). Genome plasticity a key factor in the success of polyploid wheat under domestication. Science 316, 1862–1866. doi: 10.1126/science.1143986
Fan, C., Xing, Y., Mao, H., Lu, T., Han, B., Xu, C., et al. (2006). GS3, a major QTL for grain length and weight and minor QTL for grain width and thickness in rice, encodes a putative transmembrane protein. Theor. Appl. Genet. 112, 1164–1171. doi: 10.1007/s00122-006-0218-1
Fan, X., Cui, F., Zhao, C., Zhang, W., Yang, L., Zhao, X., et al. (2015). QTLs for flag leaf size and their influence on yield-related traits in wheat (Triticum aestivum L.). Mol. Breed. 35:24. doi: 10.1007/s11032-015-0205-9
Gao, F., Wen, W., Liu, J., Rasheed, A., Yin, G., Xia, X., et al. (2015). Genome-wide linkage mapping of QTL for yield components, plant height and yield-related physiological traits in the Chinese wheat cross Zhou 8425B/Chinese Spring. Front. Plant Sci. 6:1099. doi: 10.3389/fpls.2015.01099
Giura, A., and Saulescu, N. N. (1996). Chromosomal location of genes controlling grain size in a large grained selection of wheat (Triticum aestivum L.). Euphytica 89, 77–80. doi: 10.1007/BF00015722
Groos, C., Robert, N., Bervas, E., and Charmet, G. (2003). Genetic analysis of grain protein-content, grain yield and thousand kernel weight in bread wheat. Theor. Appl. Genet. 106, 1032–1040. doi: 10.1007/s00122-002-1111-1
Guo, Y., Sun, J., Zhang, G., Wang, Y., Kong, F., Zhao, Y., et al. (2013). Haplotype, molecular marker and phenotype effects associated with mineral nutrient and grain size traits of TaGS1a in wheat. Field Crops Res. 154, 119–125. doi: 10.1016/j.fcr.2013.07.012
Habash, D. Z., Bernard, S., Schondelmaier, J., Weyen, J., and Quarrie, S. A. (2007). The genetics of nitrogen use in hexaploid wheat: N utilisation, development and yield. Theor. Appl. Genet. 114, 403–419. doi: 10.1007/s00122-006-0429-5
Huang, R., Jiang, L., Zheng, J., Wang, T., Wang, H., Huang, Y., et al. (2013). Genetic bases of rice grain shape: so many genes, so little known. Trends Plant Sci. 18, 218–226. doi: 10.1016/j.tplants.2012.11.001
Korzun, V., Roeder, M. S., Ganal, M. W., Worland, A. J., and Law, C. N. (1998). Genetic analysis of the dwarfing gene (Rht8) in wheat, Part I. Molecular mapping of Rht8 on the short arm of chromosome 2D of bread wheat (Triticum aestivum L.). Theor. Appl. Genet. 96, 1104–1109. doi: 10.1007/s001220050845
Kumar, A., Mantovani, E. E., Seetan, R., Soltani, A., Echeverrysolarte, M., Jain, S., et al. (2016). Dissection of genetic factors underlying wheat kernel shape and size in an Elite × Nonadapted cross using a high density SNP linkage map. Plant Genome 9, 2–22. doi: 10.3835/plantgenome2015.09.0081
Lander, E., and Kruglyak, L. (1995). Genetic dissection of complex trait: guidelines for interpreting and reporting linkage results. Nat. Genet. 11, 241–247. doi: 10.1038/ng1195-241
Laperche, A., Brancourt-Hulmel, M., Heumez, E., Gardet, O., Hanocq, E., Devienne-Barret, F., et al. (2007). Using genotype × nitrogen interaction variables to evaluate the QTL involved in wheat tolerance to nitrogen constraints. Theor. Appl. Genet. 326, 399–415. doi: 10.1007/s00122-007-0575-4
Li, H. H., Ye, G. Y., and Wang, J. K. (2007). A modifed algorithm for the improvement of composite interval mapping. Genetics 175, 361–374. doi: 10.1534/genetics.106.066811
Li, Q., Lin, L., Yang, X., Warburton, M. L., Bai, G., Dai, J., et al. (2010). Relationship, evolutionary fate and function of two maize co-orthologs of rice GW2 associated with kernel size and weight. BMC Plant Biol. 10:143. doi: 10.1186/1471-2229-10-143
Li, Q., Zhang, Y., Liu, T., Wang, F., Liu, K., Chen, J., et al. (2015). Genetic analysis of kernel weight and kernel size in wheat (Triticum aestivum L.) using unconditional and conditional QTL mapping. Mol. Breed. 35, 1–15. doi: 10.1007/s11032-015-0384-4
Li, Y., Fan, C., Xing, Y., Jiang, Y., Luo, L., Sun, L., et al. (2011). Natural variation in GS5 plays an important role in regulating grain size and yield in rice. Nat. Genet. 43, 1266–1269. doi: 10.1038/ng.977
Liu, Y., Wang, L., Sun, C., Zhang, Z., Zheng, Y., and Qiu, F. (2014). Genetic analysis and major QTL detection for maize kernel size and weight in multi-environments. Theor. Appl. Genet. 127, 1019–1037. doi: 10.1007/s00122-014-2276-0
Ma, D., Yan, J., He, Z., Wu, L., and Xia, X. (2012). Characterization of a cell wall invertase gene TaCwi-A1 on common wheat chromosome 2A and development of functional markers. Mol. Breed. 29, 43–52. doi: 10.1007/s11032-010-9524-z
Ma, L., Li, T., Hao, C., Wang, Y., Chen, X., and Zhang, X. (2016). TaGS5-3A, a grain size gene selected during wheat improvement for larger kernel and yield. Plant Biotechnol. J. 14, 1269–1280. doi: 10.1111/pbi.12492
Makino, A. (2011). Photosynthesis, grain yield and nitrogen utilization in rice and wheat. Plant Physiol. 155, 125–129. doi: 10.1104/pp.110.165076
Osborne, B. G., and Anderssen, R. S. (2003). Single-kernel characterization principles and applications. Cereal Chem. J. 80, 613–622. doi: 10.1094/CCHEM.2003.80.5.613
Ramya, P., Chaubal, A., Kulkarni, K., Gupta, L., Kadoo, N., Dhaliwal, H. S., et al. (2010). QTL mapping of 1000-kernel weight, kernel length, and kernel width in bread wheat (Triticum aestivum L.). J. Appl. Genet. 51, 421–429. doi: 10.1007/BF03208872
Sarma, R. N., Fish, L., Gill, B. S., and Snape, J. W. (2000). Physical characterization of the homoeologous group-5 chromosomes of wheat in terms of rice linkage blocks, and physical mapping of some important genes. Genome 43, 191–198. doi: 10.1139/g99-083
Sayre, K. D., Rajaram, S., and Fischer, R. A. (1997). Yield potential progress in short bread wheats in Northwest Mexico. Crop Sci. 37, 36–42. doi: 10.2135/cropsci1997.0011183X003700010006x
Sinclair, T. R., and Jamieson, P. D. (2006). Grain number, wheat yield and bottling beer: an analysis. Field Crops Res. 98, 60–67. doi: 10.1016/j.fcr.2005.12.006
Somers, D. J., Isaac, P., and Edwards, K. (2004). A high-density microsatellite consensus map for bread wheat (Triticum aestivum L.). Theor. Appl. Genet. 109, 1105–1114. doi: 10.1007/s00122-004-1740-7
Song, X. J., Huang, W., Shi, M., Zhu, M. Z., and Lin, H. X. (2007). A QTL for rice grain width and weight encodes a previously unknown RING-type E3 ubiquitin ligase. Nat. Genet. 39, 623–630. doi: 10.1038/ng2014
Su, Z., Hao, C., Wang, L., Dong, Y., and Zhang, X. (2011). Identification and development of a functional marker of TaGW2 associated with grain weight in bread wheat (Triticum aestivum L.). Theor. Appl. Genet. 122, 211–223. doi: 10.1007/s00122-010-1437-z
Tóth, B., Galiba, G., Fehér, E., Sutka, J., and Snape, J. W. (2003). Mapping genes affecting flowering time and frost resistance on chromosome 5B of wheat. Theor. Appl. Genet. 107, 509–514. doi: 10.1007/s00122-003-1275-3
Wang, L., Ge, H., Hao, C., Dong, Y., and Zhang, X. (2012). Identifying loci influencing 1,000-Kernel weight in wheat by microsatellite screening for evidence of selection during breeding. PLoS One 7:e29432. doi: 10.1371/journal.pone.0029432
Wang, S., Wu, K., Yuan, Q., Liu, X., Liu, Z., Lin, X., et al. (2012). Control of grain size, shape and quality by OsSPL16 in rice. Nat. Genet. 44, 950–954. doi: 10.1038/ng.2327
Wang, S., Wong, D., Forrest, K., Allen, A., Chao, S., Huang, B. E., et al. (2014). Characterization of polyploid wheat genomic diversity using a high-density 90,000 single nucleotide polymorphism array. Plant Biotechnol. J. 12, 787–796. doi: 10.1111/pbi.12183
Winfield, M. O., Allen, A. M., Burridge, A. J., Barker, G. L. A., Benbow, H. R., Wilkinson, P. A., et al. (2016). High-density SNP genotyping array for hexaploid wheat and its secondary and tertiary gene pool. Plant Biotechnol. J. 14, 1195–1206. doi: 10.1111/pbi.12485
Wu, Q. H., Chen, Y. X., Zhou, S. H., Fu, L., Chen, J. J., Xiao, Y., et al. (2015). High-density genetic linkage map construction and QTL mapping of grain shape and size in the wheat population Yanda1817 × Beinong6. PLoS One 10:e0118144. doi: 10.1371/journal.pone.0118144
Xiao, S. H., and He, Z. H. (2003). “Wheat yield and end use quality improvement in China,” in Chinese Wheat Improvement and Pedigree Analysis, ed. Q. S. Zhuang (Beijing: China Agricultural Publish Press).
Xu, R., Duan, P., Yu, H., Zhou, Z., Zhang, B., Wang, R., et al. (2018). Control of grain size and weight by the OsMKKK10-OsMKK4-OsMAPK6 signaling pathway in rice. Mol. Plant 11, 860–873. doi: 10.1016/j.molp.2018.04.004
Zhai, H., Feng, Z., Li, J., Liu, X., Xiao, S., Ni, Z., et al. (2016). QTL analysis of spike morphological traits and plant height in winter wheat (Triticum aestivum L.) using a high-density SNP and SSR-based linkage map. Front. Plant Sci. 7:1617. doi: 10.3389/fpls.2016.01617
Zhang, K., Wang, J., Zhang, L., Rong, C., Zhao, F., Peng, T., et al. (2013). Association analysis of genomic loci important for grain weight control in elite common wheat varieties cultivated with variable water and fertiliser supply. PLoS One 8:e57853. doi: 10.1371/journal.pone.0057853
Zhang, L., Zhao, Y. L., Gao, L. F., Zhao, G. Y., Zhou, R. H., Zhang, B. S., et al. (2012). TaCKX6-D1, the ortholog of rice OsCKX2, is associated with grain weight in hexaploid wheat. New Phytol. 195, 574–584. doi: 10.1111/j.1469-8137
Zhang, X., Wang, J., Huang, J., Lan, H., Wang, C., Yin, C., et al. (2012). Rare allele of OsPPKL1 associated with grain length causes extra-large grain and a significant yield increase in rice. Proc. Natl. Acad. Sci. U.S.A. 109, 21534–21539. doi: 10.1073/pnas.1219776110
Keywords: linkage analysis, SNP, SSR, QTL, kernel traits, Triticum aestivum
Citation: Su Q, Zhang X, Zhang W, Zhang N, Song L, Liu L, Xue X, Liu G, Liu J, Meng D, Zhi L, Ji J, Zhao X, Yang C, Tong Y, Liu Z and Li J (2018) QTL Detection for Kernel Size and Weight in Bread Wheat (Triticum aestivum L.) Using a High-Density SNP and SSR-Based Linkage Map. Front. Plant Sci. 9:1484. doi: 10.3389/fpls.2018.01484
Received: 04 July 2018; Accepted: 24 September 2018;
Published: 11 October 2018.
Edited by:
Anna Maria Mastrangelo, Centro di Ricerca per l’Orticoltura (CRA), ItalyReviewed by:
Jingjuan Zhang, Murdoch University, AustraliaKen Chalmers, The University of Adelaide, Australia
Copyright © 2018 Su, Zhang, Zhang, Zhang, Song, Liu, Xue, Liu, Liu, Meng, Zhi, Ji, Zhao, Yang, Tong, Liu and Li. This is an open-access article distributed under the terms of the Creative Commons Attribution License (CC BY). The use, distribution or reproduction in other forums is permitted, provided the original author(s) and the copyright owner(s) are credited and that the original publication in this journal is cited, in accordance with accepted academic practice. No use, distribution or reproduction is permitted which does not comply with these terms.
*Correspondence: Wei Zhang, weizhang@sjziam.ac.cn Junming Li, ljm@sjziam.ac.cn
†These authors have contributed equally to this work