- 1Triticeae Research Institute, Sichuan Agricultural University, Chengdu, China
- 2State Key Laboratory of Crop Genetics of Disease Resistance and Disease Control, Sichuan Agricultural University, Chengdu, China
- 3College of Agronomy, Sichuan Agricultural University, Chengdu, China
Stripe rust (also known as yellow rust), caused by the pathogen Puccinia striiformis f. sp. tritici (Pst), is a common and serious fungal disease of wheat (Triticum aestivum L.) worldwide. To identify effective stripe rust resistance loci, a genome-wide association study was performed using 152 wheat landraces from the Yellow and Huai River Valleys in China based on Diversity Arrays Technology and simple sequence repeat markers. Phenotypic evaluation of the degree of resistance to stripe rust at the adult-plant stage under field conditions was carried out in five environments. In total, 19 accessions displayed stable, high degrees of resistance to stripe rust development when exposed to mixed races of Pst at the adult-plant stage in multi-environment field assessments. A marker–trait association analysis indicated that 51 loci were significantly associated with adult-plant resistance to stripe rust. These loci included 40 quantitative trait loci (QTL) regions for adult-plant resistance. Twenty identified resistance QTL were linked closely to previously reported yellow rust resistance genes or QTL regions, which were distributed across chromosomes 1B, 1D, 2A, 2B, 3A, 3B, 4A, 4B, 5B, 6B, 7A, 7B, and 7D. Six multi-trait QTL were detected on chromosomes 1B, 1D, 2B, 3A, 3B, and 7D. Twenty QTL were mapped to chromosomes 1D, 2A, 2D, 4B, 5B, 6A, 6B, 6D, 7A, 7B, and 7D, distant from previously identified yellow rust resistance genes. Consequently, these QTL are potentially novel loci for stripe rust resistance. Among the 20 potentially novel QTL, five (QDS.sicau-2A, QIT.sicau-4B, QDS.sicau-4B.2, QDS.sicau-6A.3, and QYr.sicau-7D) were associated with field responses at the adult-plant stage in at least two environments, and may have large effects on stripe rust resistance. The novel effective QTL for adult-plant resistance to stripe rust will improve understanding of the genetic mechanisms that control the spread of stripe rust, and will aid in the molecular marker-assisted selection-based breeding of wheat for stripe rust resistance.
Introduction
Wheat (Triticum aestivum L.) is an important food crop worldwide (Juliana et al., 2017) that is persistently threatened by attack from diverse rapidly evolving pathogens (Riaz et al., 2018). Among these biotic stresses, stripe rust caused by the pathogen Puccinia striiformis f. sp. tritici (Pst) is a major global threat to wheat production (Wellings, 2011; Kumar et al., 2016), especially in China (Wan et al., 2007; McIntosh et al., 2018). The five-leading wheat-producing provinces in China are Henan, Hebei, Shandong, Jiangsu, and Anhui, which contribute more than 60% of the national production (Wan et al., 2007). The first four provinces mentioned include the Yellow and Huai River Valleys, which are the main wheat-growing regions and have a unique stripe rust epidemic system (Chen and Kang, 2017). Analysis of the genetic diversity of wheat landraces from the Yellow and Huai River Valleys in China will provide information important for breeding of disease resistance in wheat.
Since the widespread stripe rust epidemic of the 1950s, extensive research has been conducted into the epidemiology and management of this disease. To date, 80 yellow rust resistance (Yr) genes have been permanently named in wheat, including the recently mapped Yr79 (Feng et al., 2018) and Yr80 (Nsabiyera et al., 2018), and 67 stripe rust resistance genes have been temporarily designated, including all-stage resistance (also termed seedling resistance) and adult-plant resistance (APR) (Wang and Chen, 2017). Although these Yr genes have been identified in diverse wheat accessions, the race specificity of seedling resistance genes limits their efficacy against pathotypes (Kankwatsa et al., 2017). In contrast, APR is generally considered to be durable, but APR genes represent a minority of known resistance genes (Kankwatsa et al., 2017; Yuan et al., 2018). Therefore, enhancing the resistance of adult plants to cope with evolving races of Pst is the preferred strategy for resistance breeding. Although traditional breeding has substantially improved wheat cultivars, the practices are time-consuming and of low efficiency (Liu W.Z. et al., 2017). However, breeding for resistance is the most cost-effective and eco-sustainable approach to prevent disease-related yield losses (Kumar et al., 2016; Juliana et al., 2018; Singh et al., 2018). The development of molecular markers linked to Yr genes or quantitative trait loci (QTL) can facilitate marker-assisted selection and improve the efficiency of breeding disease-resistant wheat (Miedaner and Korzun, 2012; Ayana et al., 2018).
Genome-wide association study (GWAS) shows potential advantages over traditional QTL mapping and linkage analysis, such as enhanced resolution and broader allele coverage, as well as being less time-consuming and much more cost effective (Olukolu et al., 2016). A GWAS is a powerful approach that can capture trait loci and utilize linkage disequilibrium (LD) to examine marker–trait associations (MTAs) and identify novel genes associated with complex quantitative phenotypic variation (Yang et al., 2015; Liu W.Z. et al., 2017). This technique has been successfully applied to elucidate the genetic architecture of disease resistance in a variety of plant species, such as Arabidopsis (Rajarammohan et al., 2018), rice (Korinsak et al., 2018), maize (Rashid et al., 2018), grain sorghum (Adeyanju et al., 2015), and soybean (Passianotto et al., 2017). In wheat, GWAS has been used to study complex agronomic traits (Liu W.Z. et al., 2017; Sun et al., 2017), leaf rust (Gao et al., 2016), and stem rust (Kankwatsa et al., 2017; Edae et al., 2018). In addition, GWAS has enabled verification of stripe rust resistance and identification of the underlying resistance genes in wheat (Juliana et al., 2018).
In this research, we used a population of 152 landraces of wheat grown in the Yellow and Huai River Valleys to address the following three objectives: (a) to evaluate the adult-plant responses to stripe rust infection in multiple environments under field conditions, (b) to assess the genetic diversity of the selected wheat landraces based on Diversity Arrays Technology (DArT) and simple sequence repeat (SSR) markers, and (c) to identify genomic regions associated with stripe rust resistance in these wheat landraces using a mixed linear model approach and to discover potential novel genes and/or QTL for stripe rust resistance.
Materials and Methods
Plant Materials
In total, 152 wheat landraces from the Yellow and Huai River Valleys of China were used in this study. The panel of accessions originated from five Chinese provinces, namely Shandong (52), Henan (45), Hebei (26), Shaanxi (15), and Jiangsu (14). The seeds used in this study were sourced from the Chinese Academy of Agricultural Sciences (germplasm numbers are preceded by the abbreviation ZM). Details on the landraces are provided in Supplementary Table S1.
Genotypic Analysis
Genomic DNA was extracted from a single plant for each of the accessions using the cetyl trimethyl ammonium bromide method (Stewart and Via, 1993). Samples of genomic DNA from each accession were subjected to selective genotyping using the DArT-seq1 platform. All accessions were also genotyped using 135 SSR markers with 865 polymorphic allele variations, which were detected based on the published sequences of Röder et al. (1998), Pestsova et al. (2000), Sourdille et al. (2001), Somers et al. (2004), and the GrainGenes 2.0 database2.
The association mapping marker dataset was filtered using the following criteria: monomorphic markers and markers with >10% missing data or minor allele frequency (MAF) < 5% were omitted (Liu W.Z. et al., 2017). After applying these filtering criteria, 7,136 DArT-seq markers and 610 SSR markers were considered for the GWAS. Of the 7,136 DArT-seq markers that satisfied this criterion, 5,457 were positioned on the consensus genetic map. The polymorphic information content (PIC) and Shannon–Weaver diversity index (H′) were calculated for each DArT-seq and SSR marker using the formulae and respectively, where pi represents the proportion of the population carrying the ith allele (Botstein et al., 1980). A cluster analysis was performed using the neighbor-joining algorithm, and the shared-allele distance was used to determine the genetic structure of the accessions using PowerMarker v3.25 (Liu and Muse, 2005). The neighbor-joining tree was visualized using FigTree v1.4.3 (Muleta et al., 2017).
Population Structure and Linkage Disequilibrium Analysis
A population structure analysis was performed using STRUCTURE v2.3.4 (Liu W.Z. et al., 2017). The dataset comprised 7,746 high-quality markers (MAF ≥ 5% and missing data ≤ 10%), including 7,136 DArT-seq and 610 SSR markers. Ten runs were performed with a K-value range of 1–10 using the admixture and correlated allele frequencies model with a burn-in of 100,000 iterations and Monte Carlo Markov Chain of 100,000 iterations (Liu W.Z. et al., 2017). The default settings were used for all other parameters. The optimal K-value was selected using the ΔK method (Evanno et al., 2005) (Supplementary Figure S1). Kinship among the 152 accessions was estimated using 7,746 markers with TASSEL v3.0 (Bradbury et al., 2007). Q and K were used in the mixed linear model as covariates to eliminate the moderately significant P-values that showed a breach of the expected distribution (Bulli et al., 2016). The LD between all pairs of markers was calculated using TASSEL v3.0 (Bradbury et al., 2007). The LD values across the known genetic distance for each chromosome of the 152 accessions were also estimated using TASSEL v3.0 with 5,457 DArT-seq markers (Evanno et al., 2005; Bradbury et al., 2007; Liu W.Z. et al., 2017). The mean r2 values over different genetic distances were also estimated for the whole genome. The LD decay plot was generated using r2 values and the genetic map distance between markers. The genetic distance at which the LD decay curve intersected with the critical r2 value was used as a threshold to determine the confidence intervals of significant QTL.
Phenotyping and Phenotypic Data Analysis
Accessions were evaluated for APR against stripe rust by artificial inoculation with mixed Pst races in five field trials, which were performed in two locations of Sichuan Province. One trial was performed at Chongzhou (CZ; 30°33′N, 103°39′E) over three consecutive growing seasons (2015–2017) and the second trial was performed at Mianyang (MY; 31°23′N, 104°49′E) over two consecutive growing seasons (2015–2016). The different year-location combinations were defined as “environments.” The five environments were designated 15CZ, 16CZ, 17CZ, 15MY, and 16MY. In all of the test environments, all accessions were evaluated in three non-replicated rows. In total, 60 seeds of each accession were sown by hand in three rows with the 0.1 m inter-plant spacing in beds 2.0 m long, and the 0.3 m inter-row spacing (approximately 20 plants per row). Seeds of the susceptible cultivar ‘SY95-71,’ which is a Sichuan winter-wheat line susceptible to almost all Chinese Pst races, were sown every 20th row. Seeds of an additional susceptible cultivar, ‘Taichung 29,’ which is a Chinese commercial cultivar susceptible to almost all Chinese Pst races, were sown as spreader rows around each plot to ensure sufficient and homogenous distribution of Pst across the trials. The susceptible and spreader rows were inoculated approximately 1 month after planting with urediniospores of seven uniformly mixed Pst isolates prevalent in China (CYR 32, CYR 33, CYR 34, Shuiyuan 4, Shuiyuan 5, Shuiyuan 7, and Guinong 22–14). The aims of inoculating these Pst isolates in a mixture in the field were to screen for wheat accessions that exhibited broad-spectrum resistance, and to distinguish accessions that exhibited such resistance to stripe rust at the adult stage.
Stripe rust disease severity (DS), which was recorded as the percentage leaf area showing disease symptoms, was evaluated three times between the early and late dough stages. The first evaluation was performed when ‘SY95-71’ and ‘Taichung 29’ displayed DS values of at least 80%, and was followed by two additional evaluations at 7 days intervals. Resistance to stripe rust was measured using the “Rules for monitoring and forecasting wheat stripe rust (Puccinia striiformis West.)” (National Standard of the People’s Republic of China, GB/T 15795-2011). Infection type (IT) was visually scored on a 0–4 scale described by Bariana and McIntosh (1993) as follows: “0” = immune (no visible uredia); 0; = near immune (necrotic or chlorotic flecks without sporulation); 1 = highly resistant (small uredia with necrosis); 2 = moderately resistant (small to medium uredia with necrosis and chlorosis); 3 = moderately susceptible (medium-sized uredia with chlorosis); and 4 = highly susceptible (large uredia without chlorosis). Accessions with IT 0–2 were classified as resistant and those with IT 3–4 as susceptible.
Descriptive statistics and analysis of variance of stripe rust IT and DS data from the field experiments were performed using QTL IciMapping v4.1 (Meng et al., 2015; Yuan et al., 2018). Broad-sense heritability (H2) estimates were calculated for each environment as: H2 = δg2/(δg2 + δe2), where δg and δe are estimates of genetic and environmental variances, respectively (Lu et al., 2016). To eliminate the environmental impact on stripe rust responses, the best linear unbiased prediction (BLUP) values (Piepho et al., 2008) were calculated using a mixed model procedure (PROC MIXED) with SAS v8.1 (SAS Institute Inc., Cary, NC, United States). Pearson’s correlation coefficients among environments were calculated to evaluate the consistency levels of stripe rust IT and DS values across the environments.
GWAS for Stripe Rust
To identify loci associated with responses to stripe rust, a GWAS was performed using 7,746 high-quality markers, including 7,136 DArT-seq markers and 610 SSR markers, phenotypic data (IT and DS) from the five environments, and the BLUP values. The MTAs were identified using the mixed linear model, which incorporated the coefficients Q and K used in the adult-plant stage estimates of IT and DS with TASSEL v3.0 (Yu et al., 2006; Chen et al., 2017). The loci with significant MTAs had a -log10(P) threshold of 3. The DArT-seq and SSR markers were combined into a single putative QTL if they resided within a confidence interval of ±1.11 cM based on the standard critical threshold r2 = 0.3, in accordance with the method of Maccaferri et al. (2015b). We also compared the locations of significant QTL in the GWAS with those of previously reported Yr genes, including 80 formally named Yr genes (Yr1–80) and 67 temporarily designated Yr genes, and 332 mapped QTL were projected onto the integrated map that included DArT, SSR, and SNP markers using BioMercator v4.2 (Chen et al., 2017; Cheng et al., 2019). Comparison of DArT-seq marker positions was also carried out using the Wheat consensus genetic map v4.03 and IWGSC RefSeq v1.04 with BLAST+ v2.7.1 (Camacho et al., 2009).
Results
Genetic Diversity and Population Structure
In total, we identified 7,746 polymorphic markers (MAF ≥ 5% and missing data ≤ 10%) among the 152 wheat accessions. These markers were unevenly distributed among the three subgenomes and chromosomes of wheat. Of the polymorphic markers, 2,523, 3,506, and 1,717 markers were mapped to the A, B, and D subgenomes, respectively. Chromosome 3B contained the greatest number of markers (808), whereas chromosome 4D contained the fewest number of markers (97) (Supplementary Figure S2 and Supplementary Table S2). The MAF, gene diversity, and PIC indices were used to evaluate the extent of genetic variation among the 152 accessions. The three genetic diversity indices exhibited consistent trends that showed the population of wheat landraces contained high genetic diversity (Supplementary Figure S3).
The data for the three genetic diversity indices are presented in Supplementary Table S2. Genome-specific analyses of Nei’s (1973) genetic distance were significantly consistent among these indices. Chromosome 6A showed the greatest MAF, gene diversity, and PIC values of 0.267, 0.351, and 0.280, respectively, whereas chromosome 4A showed the lowest values of 0.196, 0.281, and 0.233, respectively. Among the three subgenomes, subgenome D showed the greatest MAF, gene diversity, and PIC values (0.232, 0.321, and 0.261, respectively), whereas subgenome A exhibited the lowest values (0.227, 0.313, and 0.255, respectively). The genome-wide means of the three indices for the 7,746 polymorphic markers were 0.230, 0.318, and 0.259, respectively (Supplementary Table S2 and Supplementary Figure S3).
A neighbor-joining phylogenetic analysis based on shared allele distances showed that the 152 landraces exhibited a high degree of genetic relatedness (Supplementary Figure S4). Based on the greatest ΔK value using the 7,746 polymorphic markers, the 152 accessions were divided into two subgroups, Gp1 and Gp2 (Supplementary Table S1 and Figure 1B). Subgroup Gp1 contained 75 accessions, which were predominantly from Henan, Shaanxi, and Jiangsu Province. Subgroup Gp2 contained 77 accessions, which were predominantly from Shandong and Hebei Provinces. The geographical distribution of the subgroups is shown in Figure 1D. Based on the heat map for the IT and DS values of the 152 accessions, highly resistant accessions were clustered in Gp1, and the majority of the accessions in Gp1 were characterized by low IT and DS values (Supplementary Table S1 and Figures 1C, 2B,D). The mean IT values in the five environments for the five provinces ranged from 1.41 to 3.31, and the mean DS values ranged from 15.99 to 56.45%. The lowest IT and DS values were observed for Shaanxi Province (0.67–2.27 and 5.07–27.33%, respectively), whereas the greatest values were observed for Hebei Province (3.00–3.15 and 26.63–75.96%, respectively) (Table 1). The Fast Ward distance-based hierarchical clustering method, which explains the genetic structure of the population, revealed similar genetic variation among the accessions (Figure 1A). Kinship coefficients for the 152 accessions calculated with TASSEL v3.0 using the 7,746 polymorphic markers ranged from 0 to 1, with an average of 0.48. The kinship coefficients in Gp1 ranged from 0.52 to 1, with an average of 0.80, and those in Gp2 ranged from 0.51 to 1, with an average of 0.84.
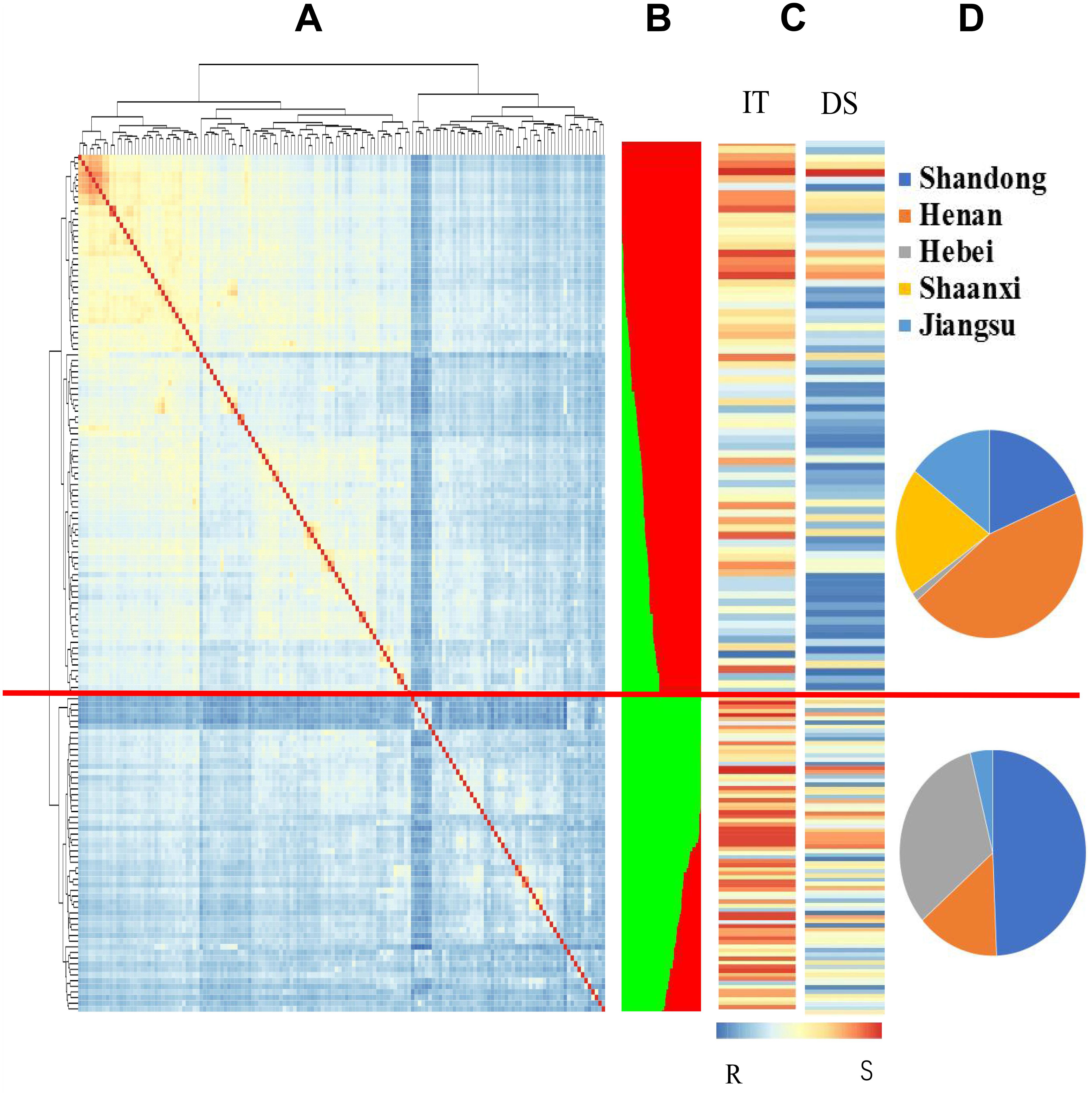
Figure 1. Relationship of population structure and genetic relatedness analysis of 152 Chinese Yellow and Huai River Valleys Wheat Zone landraces between population sub-clustering and stripe rust resistance. (A) Estimated the distance of hierarchical clustering for the accessions using Fast Ward grouping algorithm and heat map showing the kinship and phylogenetic relations. The same phylogeny was shown on the left and above the heatmap. Orange represents high kinship relations while blue colors shows continuous weaker relations. (B) Population structure summary plot (k = 2) of membership coefficients using STRUCTURE v.2.3.4. Each individual accession partitioned into colored segments, with the area of each segment representing the proportion. Two given sub-populations are represented: red Gp1; green Gp2. The red horizontal lines separated the 152 Chinese Yellow and Huai River Valleys Wheat Zone landraces into two subgroups according to structure membership coefficients. (C) The bar chart displayed IT and DS blue to white to red lines indicate reactions to Pst changed from resistance to intermediate to susceptibility to stripe rust. (D) Frequency of population structure subgroups in geographical regions of origin. From the top to the bottom, they are subgroups 1 and 2.
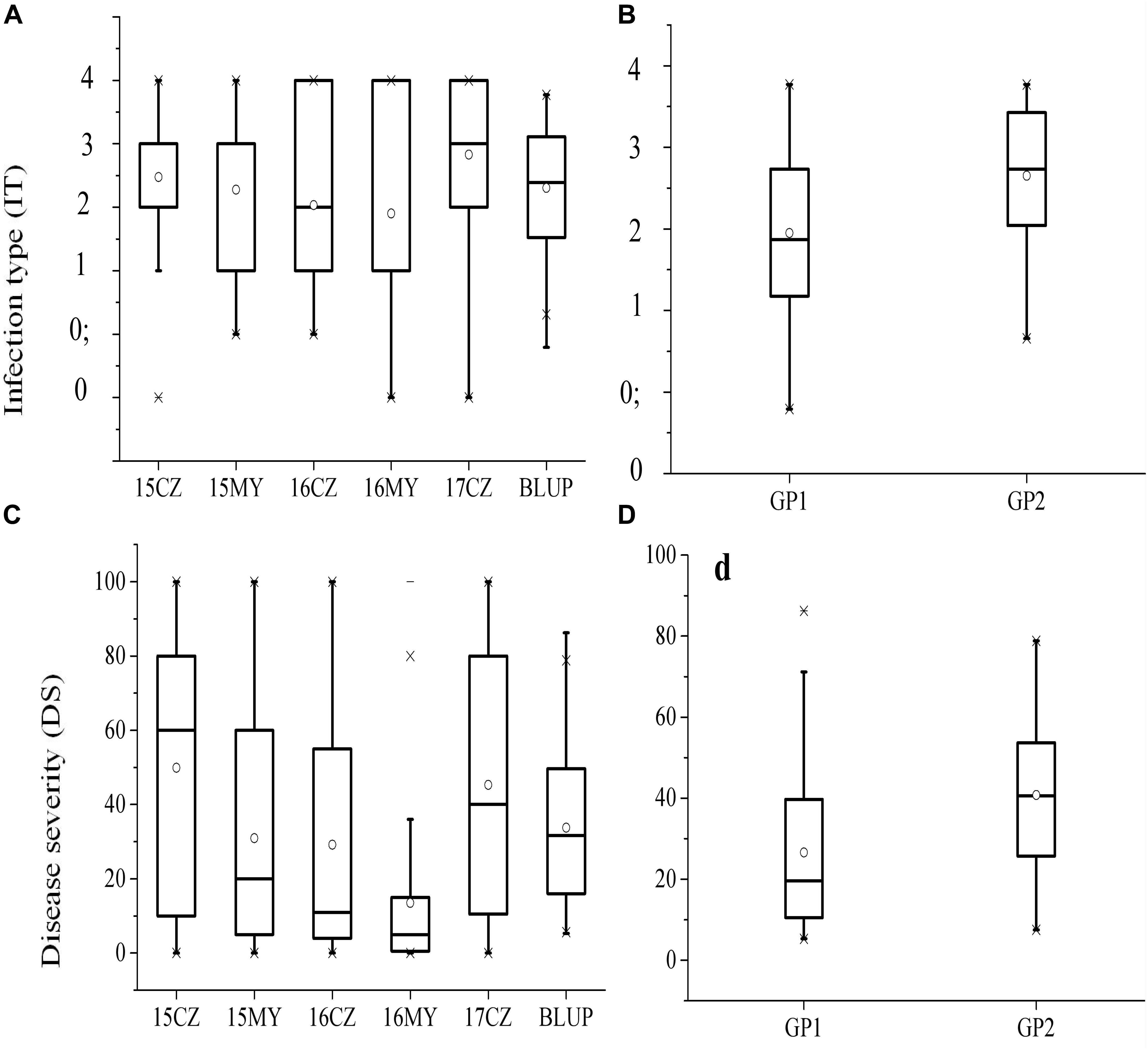
Figure 2. Box plot for distributions of IT (A) and DS (C) evaluated at the adult-plant stage in five environments; Mean, median, and range of IT (B) and DS (D) variation in each of the two subgroups at the adult-plant stage. Solid horizontal lines show medians. The circle signs the mean, the top and bottom box edges show the 25th to 75th percentiles of the total data, and the outer outliers.
Across the 152 accessions, the genome-wide LD generally declined with genetic distance (cM). Pairwise DArT-seq markers showed a significant LD (P < 0.001), which was illustrated by the scatter of pairwise LD r2 values (Supplementary Figure S5). The baseline intersected with the smoothing spline curve at 1.11 cM based on the standard critical r2 = 0.3, which was used to estimate the QTL coverage regions with inter-marker genetic distance confidence intervals of ±1.11 cM from the peak of the significant associations.
Phenotypic Assessment and H2 Estimation
The responses of the 152 wheat landraces to Pst were assessed in the five environments (3 years at CZ, and 2 years at MY). The phenotypic data used for the GWAS comprised the IT and DS values. Based on the IT data, two accessions (1.32%) were highly resistant (R, IT 0–1) to the mixed races of Pst across all environments at the adult-plant stage, whereas 17 accessions (11.18%) were highly susceptible (S, IT = 4). Based on the mean DS values, broad variation was exhibited among the 152 accessions in each environment, ranging from 8 to 57%. In total, 11.84% (mean DS < 20%) of accessions were highly resistant and 32.24% were highly susceptible (mean DS > 80%) to the mixed races of Pst across all environments at the adult-plant stage (Supplementary Table S1). The means for IT and DS ranged from 1.90 to 2.83 and 13.52 to 49.90%, respectively, within the environments (Figures 2A,C). Individual subpopulations showed different degrees of stripe rust resistance, with Gp1 showing the lowest mean BLUP values for IT (1.95) and DS (26.60%) (Figures 2B,D), which indicated the influence of APR genes. The means for IT and DS for the accessions originating from Shaanxi Province were greater than those from Hebei Province (Table 1). The phenotypic variation of IT and DS across the five environments was validated by phenotypic distributions based on BLUP values. In general, we identified 19 accessions with stable high-level resistance to stripe rust across all environments at the adult-plant stage, with low IT (0–2), DS (<20%), and BLUP (<1.20 for IT and <10.00 for DS) values (Supplementary Table S3). These accessions are promising sources of stripe rust resistance to exploit in breeding programs. Analysis of variance revealed statistically significant (P < 0.01) differences among the accessions in both the individual locations and across the locations in the five environments. The H2 values for stripe rust IT and DS, calculated across the five environments, were 81 and 86%, respectively, and collectively ranged from 77 to 86% (Table 2). The relatively high H2 estimates indicated environmental variation was limited compared with phenotypic variation across the five environments. The Pearson’s correlation coefficients for IT and DS responses to stripe rust ranged from 0.388 to 0.687 (Table 3). The Pearson’s correlation coefficients for stripe rust IT and DS among the multiple locations over multiple growing years averaged 0.574 and 0.541, respectively. Average correlations between years within locations were 0.571 and 0.632 for IT, and 0.493 and 0.611 for DS at CZ and MY, respectively. The correlation coefficients between IT and DS within the same environment ranged from 0.393 to 0.893 (Table 3).
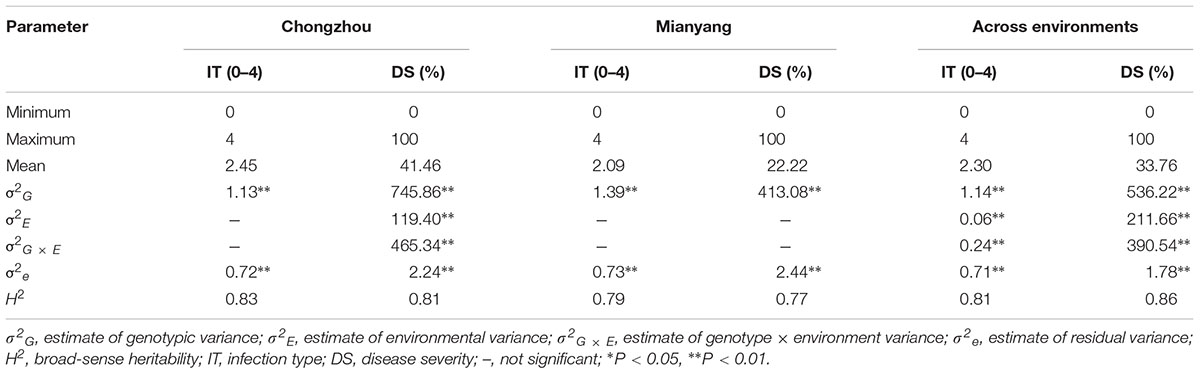
Table 2. Estimates of variance components and heritability of IT and DS of stripe rust at adult-plant stage for the 152 Chinese Yellow and Huai River Valleys Wheat Zone landraces in five environments.
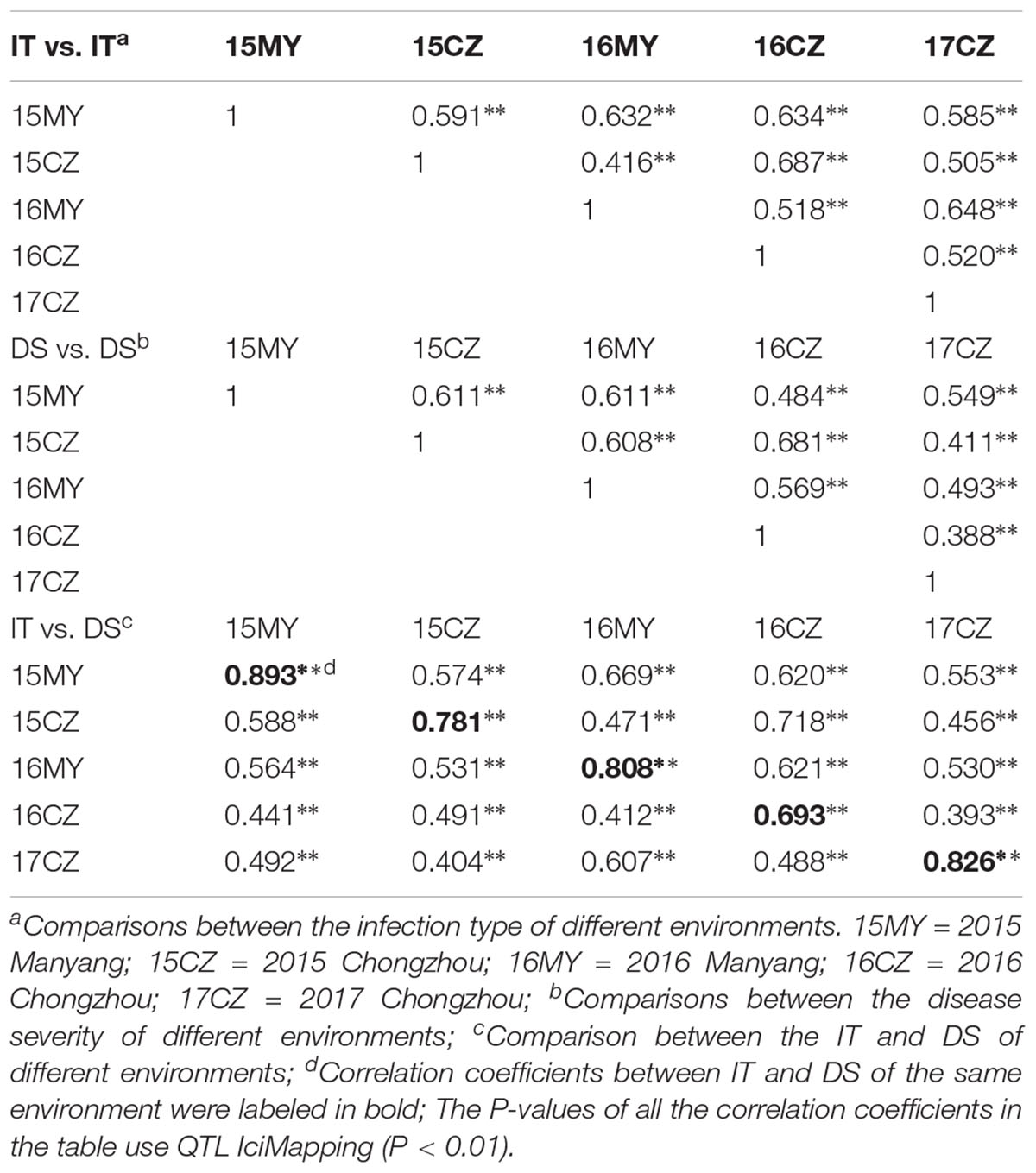
Table 3. Correlation coefficients for IT and DS response to APR of 152 Chinese Yellow and Huai River Valleys Wheat Zone landraces in five environments.
Candidate Genes Associated With Pst
Using 7,746 polymorphic markers, a GWAS was performed for stripe rust IT and DS after exposure to mixed Pst isolates within the five environments and for BLUP values at the adult-plant stage based on the mixed linear model. A total of 51 markers within 40 distinct QTL located on all 16 chromosomes were determined to be significantly associated (P < 0.001) with APR (Table 4). Unique QTL were located on chromosomes 2D, 3A, 4A, and 6D, and more than one QTL on the other chromosomes. The phenotypic variance explained by each of these MTAs ranged from 7.44 to 17.70%. Detailed information on the 40 putative resistance QTL is presented in Table 4.
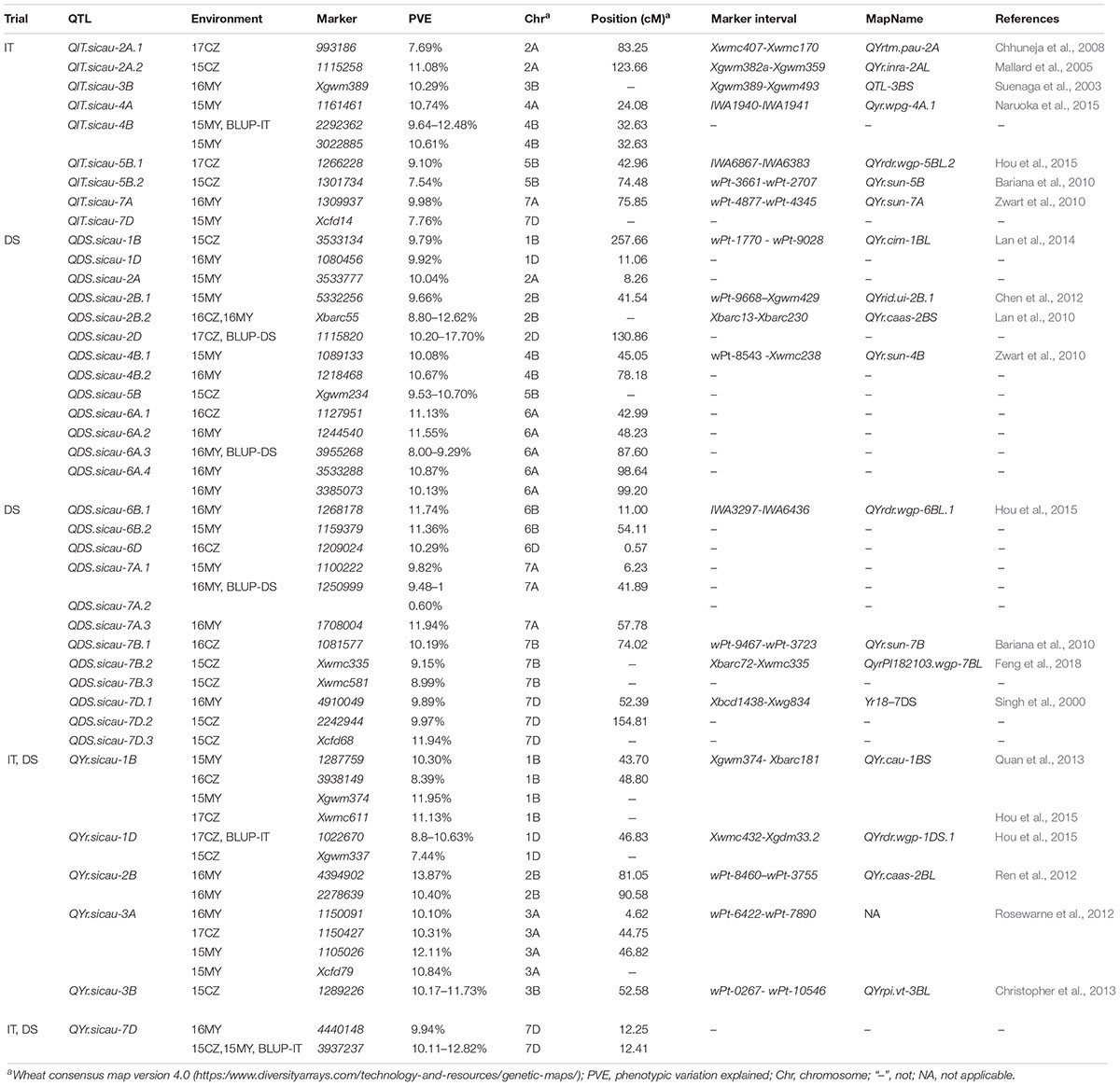
Table 4. Association of the 7746 markers at adult-plant stage of putative QTL to previously reported Yr genes and QTL.
Of the 40 QTL, nine QTL detected on seven chromosomes were associated with IT, explaining 7.54–12.48% of the phenotypic variation, and 25 QTL detected on chromosomes 1A, 3A, 4B, 6B, and 6D were associated with DS, explaining 8.00–12.62% of the phenotypic variation. In total, six of the 40 QTL were identified as associated with both IT and DS.
Twenty potentially novel QTL or Yr genes were associated with adult-plant responses, which were located on 11 chromosomes and explained 2.63–17.70% of the phenotypic variance (Table 5). In particular, QYr.sicau-7D was significantly associated with both IT and DS. QDS.sicau-2A, QIT.sicau-4B, QDS.sicau-4B.2, QDS.sicau-6A.3, and QYr.sicau-7D were significantly associated with adult-plant responses in four or more environments as well as with BLUPs, and explained 2.69–12.82% of the phenotypic variation. All of these novel loci are strong candidates to aid in development of cultivars with increased resistance to stripe rust at the adult-plant stage.
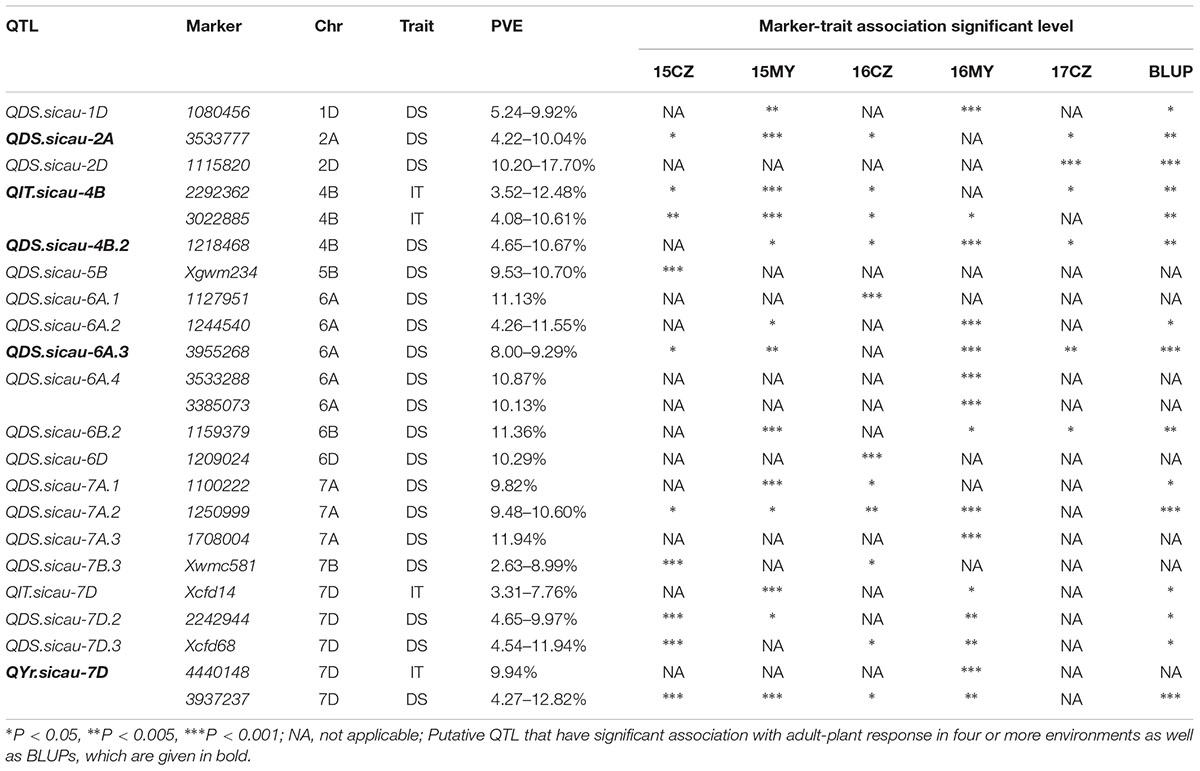
Table 5. Potentially novel QTL or Yr genes associated with stripe rust resistance to five environments and BLUPs at the adult-plant stage.
Discussion
LD Decay and Population Structure
Population structure is an important factor that influences LD (Flint-Garcia et al., 2003). Assessment of population structure is extremely important before conducting a GWAS to avoid spurious associations (Yu et al., 2006). In the present study, STRUCTURE analysis divided the 152 wheat accessions into two subgroups on the basis of genotype data. The Q and K method was used in the GWAS analysis, and some false negative MTAs were eliminated (Liu Y.X. et al., 2017).
Response of Adult-Stage Wheat Landraces to Stripe Rust
In this study, we evaluated responses to stripe rust across five environments. The statistically significant (P < 0.001) differences observed in different environments were most likely the result of variation in environmental variables (temperature and rainfall) and the Pst race composition in each environment. Some accessions showed lower IT and DS values in one environment compared with those in a different environment. In this situation, BLUP values were obtained across locations and years, with genotypes considered as fixed effects in this model (Liu W.Z. et al., 2017; Zhang et al., 2018). Thus, to increase the reliability of the results, MTAs were considered relevant only when the parameters were significant in two or more environments, as well as in the multi-environment BLUP analysis.
Identification and mapping of stripe rust resistance genes have been conducted since the 1960s (Lupton and Macer, 1962). The majority of stripe rust resistance genes have been identified in common wheat (Chen, 2013). Chinese landraces, such as ‘Pingyuan 50,’ which may possess potentially useful loci for race-specific and race-non-specific resistance, have been investigated (Lan et al., 2010). In the current study, MTAs were not associated with multiple environments when applying a stringent significance level in each environment. When applying P < 0.001, only QYr.sicau-7D, located in the QTL region between 4440148 and 3937237, was highly significantly associated with IT and DS in three environments as well as with BLUPs at the adult-plant stage (Table 4). When applying the less stringent significance criterion of P < 0.005, five additional loci (QDS.sicau-6A.3, QDS.sicau-7A.2, QYr.sicau-1D, QYr.sicau-4B.1, and QYr.sicau-4B.2) were significantly associated with IT and/or DS in two or more environments as well as with BLUPs at the adult-plant stage. Three of the five loci (QDS.sicau-6A.3, QYr.sicau-4B.1, and QYr.sicau-4B.2) were potentially novel APR loci. The QTL QYr.sicau-4B.1 and QYr.sicau-4B.2 were identical to QIT.sicau-4B and QDS.sicau-4B.2, respectively, and were significantly associated with IT and DS (Table 5 and Supplementary Table S4). Seven additional loci (QIT.sicau-2B.1, QDS.sicau-3B, QIT.sicau-5B, QDS.sicau-5D, QIT.sicau-6A, QDS.sicau-6D.1, and QDS.sicau-7A) were significantly associated (P < 0.05) with IT and/or DS in four or more environments as well as with BLUPs at the adult-plant stage (Table 4 and Supplementary Table S4). The other loci that showed significant associations with IT and DS in single environments should be treated with caution because they were instable in different environments and susceptible to some Pst races.
Multigenic and Pleiotropic Effects Revealed by GWAS
Multigenic effects were observed in the current study, and IT and DS were significantly associated with multiple markers. Six QTL (QYr.sicau-1B, QYr.sicau-1D, QYr.sicau-2B, QYr.sicau-3A, QYr.sicau-3B, and QYr.sicau-7D) were highly significantly associated (P < 0.001) with IT and DS. For example, QYr.sicau-1B, QYr.sicau-1D, QYr.sicau-2B, QYr.sicau-3A, and QYr.sicau-7D were associated with two or more markers, identified as Xgwm374, Xwmc611, 1287759, and 3938149; 1022670 and Xgwm337; 4394902 and 2278639; 1150091, 1150427, 1105026, and Xcfd79; and 4440148 and 3937237, respectively (Table 4). Of 20 potentially novel QTL, five (QDS.sicau-2A, QIT.sicau-4B, QDS.sicau-4B.2, QDS.sicau-6A.3, and QYr.sicau-7D) were significantly (P < 0.05) associated with IT and/or DS in four or more environments, as well as with BLUPs, at the adult-plant stage (Tables 4, 5). The phenotypic variation explained by these five loci ranged from 9.29 to 12.82%. Therefore, the five QTL may have large effects on APR, particularly QIT.sicau-4B and QYr.sicau-7D, which contained multiple markers. These five QTL were used to research the potential molecular functions of the significant markers and the putative QTL.
Association of Significant Resistance Loci With Previously Published Yr Genes or QTL
Using the 152 accessions from the Yellow and Huai River Valleys, 40 putative QTL were detected that were significantly (P < 0.001) associated with APR to stripe rust caused by a mixture of prevalent Pst races. Of these QTL, 20 had been previously published. QIT.sicau-5B.1, QYr.sicau-1B, and QYr.sicau-1D were identified in winter wheat. The markers located near QYrdr.wgp-5BL.2 were IWA6383 and IWA6867, and QIT.sicau-5B.1 was flanked by the QTL region in the same interval. QYr.sicau-1B was significantly associated with markers 1287759, 3938149, Xgwm374, and Xwmc611, which were previously published in winter wheat and located in an interval containing Xgwm374 and Xwmc611 (Quan et al., 2013; Hou et al., 2015). QYrdr.wgp-1DS.1 was flanked by Xgwm353, Xgdm33b, Xgwm337, and Xwmc432. In the present study, QYr.sicau-1D was located close to this QTL region. Thus, these two QTL could be identical (Hou et al., 2015). QIT.sicau-5B.2 and QDS.sicau-6B.1 were consistently identified in the wheat cultivars ‘Janz’ and ‘Kukri,’ respectively (Bariana et al., 2010). The QTL QYrtm.pau-2A was previously mapped to a 3.6-cM interval between Xwmc407 and Xwmc170, whereas QIT.sicau-2A.1 from the present study was mapped proximal to this QTL (Bariana et al., 2010). The QTL QIT.sicau-2A.2 was identified in the interval between Xgwm382a to Xgwm359 (Mallard et al., 2005). QIT.sicau-3B, identified in bread wheat, is associated with Xgwm389 on chromosome 3BS and is considered to affect Yr30 (Suenaga et al., 2003). QIT.sicau-4A, also reported as Qyr.wpg-4A.1, is located in the interval between IWA1940 to IWA1941 (Naruoka et al., 2015). QIT.sicau-7A is inherited from a synthetic hexaploid parent (CPI133872) and is located on the distal part of chromosome 7AS. QDS.sicau-1B reduced the stripe rust DS value. Closely linked markers suggest that the 1BL locus has pleiotropic and multigenic effects on the APR gene Lr46/Yr29 (Lan et al., 2014). Stripe rust resistance QTL on chromosome 2B were previously reported (Ramburan et al., 2004; Guo et al., 2008; Carter et al., 2009). QDS.sicau-2B.1 may be a major locus derived from IDO444 (Chen et al., 2012). The SSR marker Xbarc55, which is closely linked to QDS.sicau-2B.2, may be useful to improve wheat stripe rust resistance (Lan et al., 2010). Chromosome 4BL was identified as containing many QTL (Rosewarne et al., 2013) and was the most affected by segregation distortion. QDS.sicau-4B.1, also reported as QYr.sun-4B, is located in a 4.3-cM interval containing wPt-8543 and Xwmc238 (Zwart et al., 2010) but was not detected in the present GWAS analysis. Chromosomes 7B and 7D have been reported to be associated with stripe rust resistance responses (Pink and Law, 1985). A QTL region on chromosome 7B reduces pustule density, which is a rust disease component (Muhammad et al., 2005). QDS.sicau-7B.1 is flanked by wPt-9467 and wPt-3723 in QYr.sun-7B (Bariana et al., 2010). QDS.sicau-7B.2, which is located close to marker Xwmc335, was identified in QyrPI182103.wgp-7BL as being linked to Xbarc72 and flanked by the Yr79 locus (Feng et al., 2018). QDS.sicau-7D.1 was identified by the Yr18 gene, and the region contributed by the cultivar ‘Opata 85’ was observed to reduce DS by almost half in all trials (Singh et al., 2000). QYr.sicau-2B is contributed by the common wheat cultivar ‘Naxos’ (Ren et al., 2012) in the marker interval XwPt-8460 to XwPt-3755, which was significantly associated with IT and DS, and these resistant loci were tagged by 4394902 and 2278639. The QTL QYr.sicau-3A, which was significantly associated with IT and DS, was flanked by wPt-6422 and wPt-7890 in an ‘Avocet’ × ‘Pastor’ wheat population (Rosewarne et al., 2012). Christopher et al. (2013) reported previously that QYrpi.vt-3BL, which is located between markers wPt-0267 and wPt-10546, explained 10.17–11.73% of the phenotypic variation. On the basis of the genetic locations of QYrpi.vt-3BL and QYr.sicau-3B on chromosome 3BL in the present study, these two QTL might be closely linked or located in the same chromosomal region.
Novel Stripe Rust Resistance Loci
A total of 20 potentially novel QTL that are significantly (P < 0.001) associated with IT and/or DS were detected in the field at the adult-plant stage. The relative positions and details of the previously mapped QTL and Yr genes on the integrated map are shown in Figure 3 and Supplementary Table S5. These represent potentially novel resistance loci because no QTL or genes are reported in the same interval (Table 5). However, allelism tests are required to determine which represent alleles of previously mapped genes and which represent novel Yr genes.
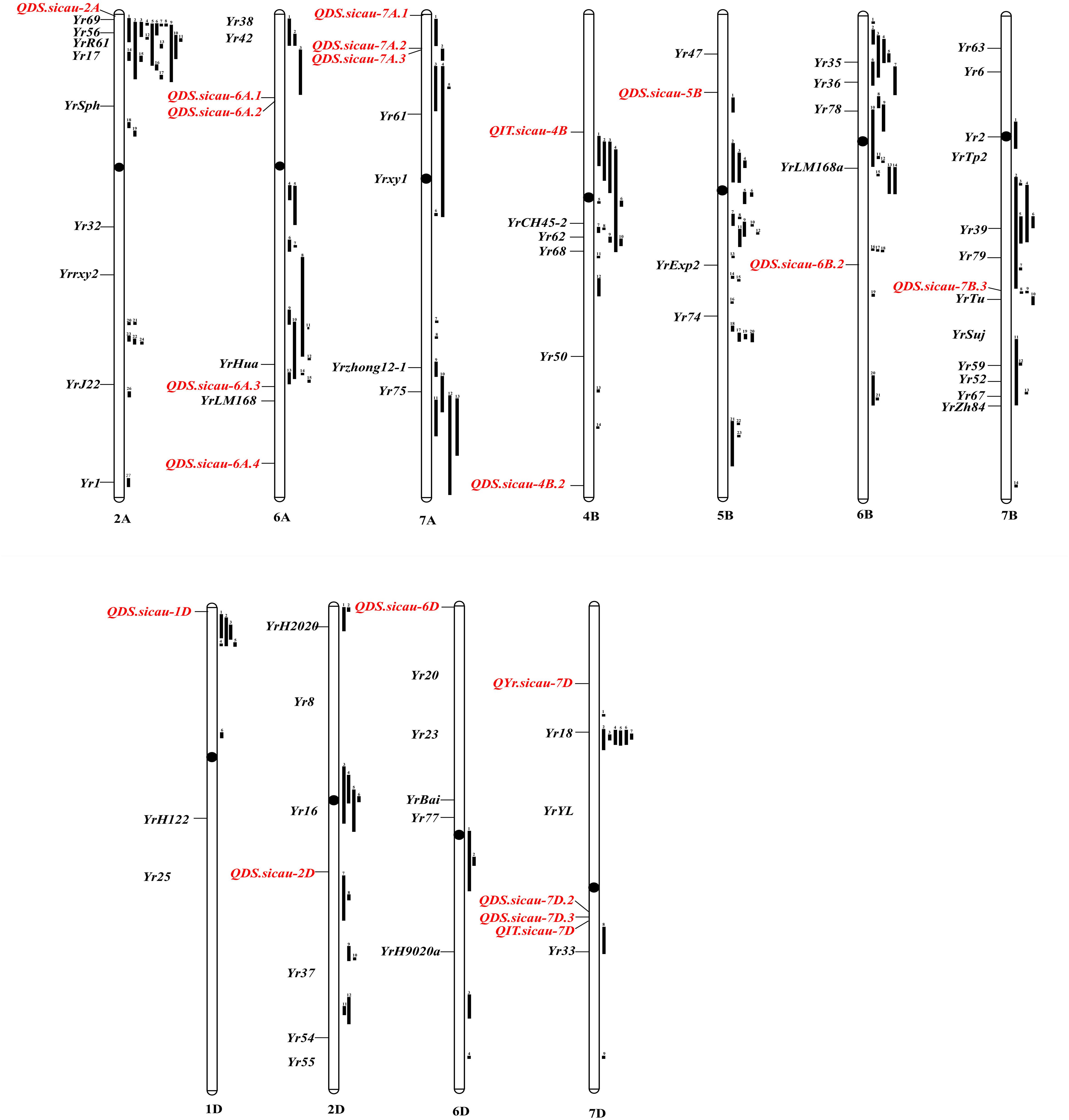
Figure 3. Chromosomal positions of loci associated with IT and/or DS to Pst identified in this study relative to positions of previously mapped QTL and Yr genes. The relative lengths of all chromosomes are standardized same. Loci identified in this study are highlighted in red. Previously mapped QTL (black bar) is on right side of the chromosomes and Yr genes (black) for stripe rust resistance is on left side of the chromosomes. All positions are approximations, and thus could be served as guidelines for future research. The relationships between loci markers identified in this study and the previously mapped QTL and Yr genes are described (Table 4 and Supplementary Table S5).
The subgenome A contained eight QTL, which were located on chromosomes 2A, 6A, and 7A. Of these QTL, six explained 10.04–11.94% of the phenotypic variance. QDS.sicau-2A was identified on the distal 3.81% of the short arm of chromosome 2A and was linked to previously reported QTL (Maccaferri et al., 2015b). However, 3533777 was located in a different linkage region. Therefore, QDS.sicau-2A is a potentially novel QTL. There are no genetic maps available to compare relative distances; therefore, the identity of QDS.sicau-2A needs to be confirmed using an allelism test. QDS.sicau-6A.1 and QDS.sicau-6A.2 were assigned to the wheat chromosome 6AS. Currently, there are no genetic maps available to compare relative distances between both 1127951 and 1244540 and the flanking markers for these previously reported QTL. Therefore, more work is required to determine whether QDS.sicau-6A.1 and QDS.sicau-6A.2 are novel. QDS.sicau-6A.4 was located on chromosome 6AL and assigned to the distal 15.97% of the long arm; this QTL may be a novel APR locus because there is no previously reported QTL in this region. QDS.sicau-7A.2 and QDS.sicau-7A.2 were assigned to the distal 5.22 and 7.31% of the short arm of wheat chromosome 7A, respectively. Many QTL have been previously reported on chromosome 7AS (Zwart et al., 2010; Rosewarne et al., 2012; Maccaferri et al., 2015a; Liu Y.X. et al., 2017), but not in this chromosomal region.
In subgenome B, one QTL was located on each of the chromosomes 5B, 6B, and 7B, and two QTL on chromosome 4B. Four out of five QTL explained 10.61–11.36% of the phenotypic variance. QIT.sicau-4B was located on chromosome 4BS, which harbors a number of previously reported QTL (Agenbag et al., 2012; Liu Y.X. et al., 2017), but these differ from QIT.sicau-4B. QDS.sicau-4B.2 was assigned to the distal 3.98% of the long arm of chromosome 4B, which to the best of our knowledge does not overlap with the position of any known APR gene; therefore, this QTL may represent a novel resistance locus. QDS.sicau-5B, which was located on the short arm of chromosome 5B, may be a novel QTL because only Yr47 and QYr.uga-5B, which are not located in this region, have been published (Hao et al., 2011). QDS.sicau-6B.2 was located on 6BL, and the previously published QTL (William et al., 2006; Rosewarne et al., 2012; Liu Y.X. et al., 2017) differ from QDS.sicau-6B.2.
Seven QTL were located in subgenome D, with one QTL each located on chromosomes 1D, 2D, and 6D, and four QTL were located on chromosome 7D. The foremost of these QTL was QYr.sicau-7D, which was highly significantly associated with IT and DS in three environments as well as with BLUPs at the adult-plant stage. In addition, four out of seven QTL explained 10.20–17.70% of the phenotypic variance. QYr.caas-2DL and Yr54 were previously published (Basnet et al., 2014), but differ from QDS.sicau-2D detected in the present study. Thus, QDS.sicau-2D is likely a novel stripe rust resistance locus. QDS.sicau-6D, which was assigned to the distal 0.01% of the short arm of chromosome 6D, represents a novel resistance locus because no race-specific genes have been published in this chromosomal region. QDS.sicau-7D.3 was mapped in proximity to the centromere of chromosome 7DL. There are no reports of any significant associations with stripe rust responses in this chromosomal region (Boukhatem et al., 2002). The confidence interval of QYr.sicau-7D tagged by DArT markers 4440148 and 3937237 did not overlap with the position of a previously published Yr gene or QTL, and thus it is likely a novel stripe rust resistance locus.
Author Contributions
LL carried out the experiments, analyzed the data, and drafted the manuscript. FY, CY, XY, YC, YqW, YW, JL, and MD performed the experiments. JW, QJ, WL, JM, and YmW analyzed the data. YL revised the manuscript. YZ participated in the design of the experiments. GC formulated the questions, designed and carried out the experiments, analyzed the data, and revised the manuscript. All authors read and approved the final version.
Funding
This work was supported by the National Key Research and Development Program of China (2017YFD0100900, 2016YFD0100100, and 2016YFD0102000).
Conflict of Interest Statement
The authors declare that the research was conducted in the absence of any commercial or financial relationships that could be construed as a potential conflict of interest.
Acknowledgments
The authors thank Prof. Qiuzhen Jia (Plant Protection Research Institute, Gansu Academy of Agricultural Sciences, Lanzhou, China) for providing the stripe rust isolates. Prof. Lilui Li and Xiuquan Li (Chinese Academy of Agricultural Sciences) for plant materials (Chinese wheat landraces) support.
Supplementary Material
The Supplementary Material for this article can be found online at: https://www.frontiersin.org/articles/10.3389/fpls.2019.00596/full#supplementary-material
Footnotes
- ^http://www.diversityarrays.com/
- ^http://wheat.pw.usda.gov/GG2
- ^https://www.diversityarrays.com/technology-and-resources/genetic-maps/
- ^http://www.wheatgenome.org/
References
Adeyanju, A., Little, C., Yu, J. M., and Tesso, T. (2015). Genome-wide association study on resistance to stalk rot diseases in grain sorghum. G3 5, 1165–1175. doi: 10.1534/g3.114.016394
Agenbag, G. M., Pretorius, Z. A., Boyd, L. A., Bender, C. M., and Prins, R. (2012). Identification of adult plant resistance to stripe rust in the wheat cultivar Cappelle-Desprez. Theor. Appl. Genet. 125, 109–120. doi: 10.1007/s00122-012-1819-5
Ayana, G. T., Ali, S., Sidhu, J. S., Gonzalez-Hernandez, J. L., Turnipseed, B., and Sehgal, S. K. (2018). Genome-wide association study for spot blotch resistance in hard winter wheat. Front. Plant Sci. 9:926. doi: 10.3389/fpls.2018.00926
Bariana, H. S., Bansal, U. K., Schmidt, A., Lehmensiek, A., Kaur, J., Miah, H., et al. (2010). Molecular mapping of adult plant stripe rust resistance in wheat and identification of pyramided QTL genotypes. Euphytica 176, 251–260. doi: 10.1007/s10681-010-0240-x
Bariana, H. S., and McIntosh, R. A. (1993). Cytogenetic studies in wheat. xv. location of rust resistance genes in VPM1 and their genetic linkage with other disease resistance genes in chromosome 2A. Genome 36, 476–482. doi: 10.1139/g93-065
Basnet, B. R., Singh, R. P., Ibrahim, A. M. H., Herrera-Foessel, S. A., HuertaEspino, J., Lan, C., et al. (2014). Characterization of Yr54 and other genes associated with adult plant resistance to yellow rust and leaf rust in common wheat Quaiu 3. Mol. Breed. 33, 385–399. doi: 10.1007/s11032-013-9957-2
Botstein, D., White, R. L., Skolnick, M., and Davis, R. W. (1980). Construction of a genetic linkage map in man using restriction fragment length polymorphisms. Am. J. Hum. Genet. 32, 314–331.
Boukhatem, N., Baret, P. V., Mingeot, D., and Jacquemin, J. M. (2002). Quantitative trait loci for resistance against yellow rust in two wheat derived recombinant inbred line populations. Theor. Appl. Genet. 104, 111–118. doi: 10.1007/s001220200013
Bradbury, P. J., Zhang, Z. W., Kroon, D. E., Casstevens, T. M., Ramdoss, Y., and Buckler, E. S. (2007). Tassel: software for association mapping of complex traits in diverse samples. Bioinformatics 23, 2633–2635. doi: 10.1093/bioinformatics/btm308
Bulli, P., Zhang, J. L., Chao, S. M., Chen, X. M., and Pumphrey, M. (2016). Genetic architecture of resistance to stripe rust in a global winter wheat germplasm collection. G3 6, 2237–2253. doi: 10.1534/g3.116.028407
Camacho, C., Coulouris, G., Avagyan, V., Ma, N., Papadopoulos, J., Bealer, K., et al. (2009). Blast+: architecture and applications. BMC Bioinform. 10:421. doi: 10.1186/1471-2105-10-421
Carter, A. H., Chen, X. M., Garland-Campbell, K., and Kidwell, K. K. (2009). Identifying QTL for high-temperature adult-plant resistance to stripe rust (Puccinia striiformis f. sp. tritici) in the spring wheat (Triticum aestivum L.) cultivar ‘Louise’. Theor. Appl. Genet. 119, 1119–1128. doi: 10.1007/s00122-009-1114-2
Chen, H., Semagn, K., Iqbal, M., Moakhar, N. P., Haile, T., N’Diaye, A., et al. (2017). Genome-wide association mapping of genomic regions associated with phenotypic traits in Canadian western spring wheat. Mol. Breed. 37:141. doi: 10.1007/s11032-017-0741-6
Chen, J. L., Chu, C. G., Souza, E. J., Guttieri, M. J., Chen, X. M., Xu, S., et al. (2012). Genome-wide identification of QTL conferring high-temperature adult-plant (HTAP) resistance to stripe rust (Puccinia striiformis f. sp. tritici) in wheat. Mol. Breed. 29, 791–800. doi: 10.1007/s11032-011-9590-x
Chen, X. M. (2013). High-temperature adult-plant resistance, key for sustainable control of stripe rust. Am. J. Plant Sci. 4, 608–627. doi: 10.4236/ajps.2013.43080
Chen, X. M., and Kang, Z. S. (2017). ““Introduction: history of research, symptoms, taxonomy of the pathogen, host range, distribution, and impact of stripe rust,”,” in X. M. Chen and Z. S. Kang, eds Stripe Rust (Berlin: Springer), 1–34. doi: 10.1007/978-94-024-1111-9
Cheng, Y. K., Yao, F. J., Ye, X. L., Jiang, Q. T., Li, W., Deng, M., et al. (2019). Construction of linkage map of the meta quantitative trait loci (MQTL) on stripe rust resistance in wheat (Triticum aestivum L.). Acta Phytopathol. Sinica doi: 10.13926/j.cnki.apps.000292
Chhuneja, P., Kaur, S., Garg, T., Ghai, M., Kaur, S., Prashar, M., et al. (2008). Mapping of adult plant stripe rust resistance genes in diploid a genome wheat species and their transfer to bread wheat. Theor. Appl. Genet. 116,313–324.
Christopher, M. D., Liu, S., Hall, M. D., Marshall, D. S., Fountain, M. O., Johnson, J. W., et al. (2013). Identification and mapping of adult-plant stripe rust resistance in soft red winter wheat cultivar ‘USG 3555’. Plant Breed. 132, 53–60. doi: 10.1111/pbr.12015
Edae, E. A., Pumphrey, M. O., and Rouse, M. N. (2018). A genome-wide association study of field and seedling response to individual stem rust pathogen races reveals combinations of race-specific genes in North American spring wheat. Front. Plant Sci. 9:52. doi: 10.3389/fpls.2018.00052
Evanno, G., Regnaut, S., and Goudet, J. (2005). Detecting the number of clusters of individuals using the software structure: a simulation study. Mol. Ecol. 14, 2611–2620. doi: 10.1111/j.1365-294X.2005.02553.x
Feng, J. Y., Wang, M. N., See, D. R., Chao, S. M., Zheng, Y. L., and Chen, X. M. (2018). Characterization of novel gene Yr79 and four additional QTL for all-stage and high-temperature adult-plant resistance to stripe rust in spring wheat PI 182103. Phytopathology 108, 737–747. doi: 10.1094/PHYTO-11-17-0375-R
Flint-Garcia, S. A., Thornsberry, J. M., and Buckler, E. S. (2003). Structure of linkage disequilibrium in plants. Ann. Rev. Plant Biol. 54, 357–374. doi: 10.1146/annurev.arplant.54.031902.134907
Gao, L., Turner, M. K., Chao, S., Kolmer, J., and Anderson, J. A. (2016). Genome wide association study of seedling and adult plant leaf rust resistance in elite spring wheat breeding lines. PLoS One 11:e0148671. doi: 10.1371/journal.pone.0148671
Guo, Q., Zhang, Z. J., Xu, Y. B., Li, G. H., Feng, J., and Zhou, Y. (2008). Quantitative trait loci for high-temperature adult-plant and slow-rusting resistance to Puccinia striiformis f. sp. tritici in wheat cultivars. Phytopathology 98, 803–809. doi: 10.1094/PHYTO-98-7-0803
Hao, Y. F., Chen, Z. B., Wang, Y. Y., Bland, D., Buck, J., Brown-Guedira, G., et al. (2011). Characterization of a major QTL for adult plant resistance to stripe rust in US soft red winter wheat. Theor. Appl. Genet. 123, 1401–1411. doi: 10.1007/s00122-011-1675-8
Hou, L., Chen, X. M., Wang, M. N., See, D. R., Chao, S. M., Bulli, P., et al. (2015). Mapping a large number of QTL for durable resistance to stripe rust in winter wheat Druchamp using SSR and SNP markers. PLoS One 10:e0126794. doi: 10.1371/journal.pone.0126794
Juliana, P., Singh, R. P., Singh, P. K., Crossa, J., Huerta-Espino, J., Lan, C. X., et al. (2017). Genomic and pedigree-based prediction for leaf, stem, and stripe rust resistance in wheat. Theor. Appl. Genet. 130, 1–16. doi: 10.1007/s00122-017-2897-1
Juliana, P., Singh, R. P., Singh, P. K., Poland, J. A., Bergstrom, G. C., and Huerta-Espino, J. (2018). Genome-wide association mapping for resistance to leaf rust, stripe rust and tan spot in wheat reveals potential candidate genes. Theor. Appl. Genet. 131, 1405–1422. doi: 10.1007/s00122-018-3086-6
Kankwatsa, P., Singh, D., Thomson, P. C., Babiker, E. M., Bonman, J. M., Newcomb, M., et al. (2017). Characterization and genome-wide association mapping of resistance to leaf rust, stem rust and stripe rust in a geographically diverse collection of spring wheat landraces. Mol. Breed. 37:113. doi: 10.1007/s11032-017-0707-8
Korinsak, S., Tangphatsornruang, S., Pootakham, W., Wanchana, S., Plabpla, A., Jantasuriyarat, C., et al. (2018). Genome-wide association mapping of virulence gene in rice blast fungus Magnaporthe oryzae using a genotyping by sequencing approach. Genomics doi: 10.1016/j.ygeno.2018.05.011
Kumar, S., Archak, S., Tyagi, R. K., Kumar, J., Vikas, V. K., Jacob, S. R., et al. (2016). Evaluation of 19,460 wheat accessions conserved in the Indian national genebank to identify new sources of resistance to rust and spot blotch diseases. PLoS One 12:e0175610. doi: 10.1371/journal.pone.0167702
Lan, C. X., Liang, S. S., Zhou, X. C., Zhou, G., Lu, Q. L., Xia, X. C., et al. (2010). Identification of genomic regions controlling adult-plant stripe rust resistance in Chinese landrace Pingyuan 50 through bulked segregant analysis. Phytopathology 100, 313–318. doi: 10.1094/PHYTO-100-4-0313
Lan, C. X., Rosewarne, G. M., Singh, R. P., Herrerafoessel, S. A., Huertaespino, J., Basnet, B. R., et al. (2014). QTL characterization of resistance to leaf rust and stripe rust in the spring wheat line francolin#1. Mol. Breed. 34, 789–803. doi: 10.1007/s11032-014-0075-6
Liu, K., and Muse, S. V. (2005). PowerMarker: an integrated analysis environment for genetic marker analysis. Bioinformatics 21, 2128–2129.
Liu, Y. X., Lin, Y., Gao, S., Li, Z., Ma, J., Deng, M., et al. (2017). A genome-wide association study of 23 agronomic traits in Chinese wheat landraces. Plant J. 91, 861. doi: 10.1111/tpj.13614
Liu, W. Z., Maccaferri, M., Bulli, P., Rynearson, S., Tuberosa, R., Chen, X. M., et al. (2017). Genome-wide association mapping for seedling and field resistance to Puccinia striiformis f. sp. tritici in elite durum wheat. Theor. Appl. Genet. 130, 649–667. doi: 10.1007/s00122-016-2841-9
Lu, Q., Zhang, M. C., Niu, X. J., Wang, C. H., Xu, Q., Feng, Y., et al. (2016). Uncovering novel loci for mesocotyl elongation and shoot length in Indica rice through genome-wide association mapping. Planta 243, 645–657. doi: 10.1007/s00425-015-2434-x
Lupton, F. G. H., and Macer, R. C. F. (1962). Inheritance of resistance to yellow rust (Puccinia glumarum) in seven varieties of wheat. Br. Mycol. Soc. 45, 21–45. doi: 10.1016/s0007-1536(62)80032-1
Maccaferri, M., Zhang, J. L., Bulli, P., Abate, Z., Chao, S. M., Cantu, D., et al. (2015a). A genome-wide association study of resistance to stripe rust (Puccinia striiformis f. sp. tritici) in a worldwide collection of hexaploid spring wheat (Triticum aestivum L.). G3 5, 449–465. doi: 10.1534/g3.114.014563
Maccaferri, M., Ricci, A., Salvi, S., Milner, S. G., Noli, E., Martelli, P. L., et al. (2015b). A high-density, SNP-based consensus map of tetraploid wheat as a bridge to integrate durum and bread wheat genomics and breeding. Plant Biotechnol. J. 13, 648–663. doi: 10.1111/pbi.12288
Mallard, S., Gaudet, D., Aldeia, A., Abelard, C., Besnard, A. L., Sourdille, P., et al. (2005). Genetic analysis of durable resistance to yellow rust in bread wheat. Theor. Appl. Genet. 110, 1401–1409. doi: 10.1007/s00122-005-1954-3
McIntosh, R., Mu, J. M., Han, D. J., and Kang, Z. S. (2018). Wheat stripe rust resistance gene Yr24/Yr26: a retrospective review. Crop J. 6, 321–329. doi: 10.1016/j.cj.2018.02.001
Meng, L., Li, H. H., Zhang, L. Y., and Wang, J. K. (2015). QTL IciMapping: integrated software for genetic linkage map construction and quantitative trait locus mapping in biparental populations. Crop J. 3, 269–283. doi: 10.1016/j.cj.2015.01.001
Miedaner, T., and Korzun, V. (2012). Marker-assisted selection for disease resistance in wheat and barley breeding. Phytopathology 102, 560–566. doi: 10.1094/PHYTO-05-11-0157
Muhammad, I., Matthew, C., Maqbool, A., John, H., and David, M. (2005). Identification of QTLs for quantitative resistance to stripe rust (Puccinia striiformis f.sp. triticii) in bread wheat. Plant Pathol. J. 4, 8–13. doi: 10.3923/ppj.2005.8.13
Muleta, K. T., Rouse, M. N., Rynearson, S., Chen, X. M., Buta, B. G., and Pumphrey, M. O. (2017). Characterization of molecular diversity and genome-wide mapping of loci associated with resistance to stripe rust and stem rust in Ethiopian bread wheat accessions. BMC Plant Biol. 17:134. doi: 10.1186/s12870-017-1082-7
Naruoka, Y., Garlandcampbell, K. A., and Carter, A. H. (2015). Genome-wide association mapping for stripe rust (Puccinia striiformis f. sp. tritici) in US Pacific Northwest winter wheat (Triticum aestivum L.). Theor. Appl. Genet. 128, 1083–1101. doi: 10.1007/s00122-015-2492-2
Nei, M. (1973). Analysis of gene diversity in subdivided populations. Proc. Natl. Acad. Sci. U.S.A. 70, 3321–3323. doi: 10.1073/pnas.70.12.3321
Nsabiyera, V., Bariana, H. S., Qureshi, N., Wong, D., Hayden, M. J., and Bansal, U. K. (2018). Characterization and mapping of adult plant stripe rust resistance in wheat accession Aus27284. Theor. Appl. Genet. 131, 1–9. doi: 10.1007/s00122-018-3090-x
Olukolu, B. A., Tracy, W. F., Wisser, R., Vires, B. D., and Balint-Kurti, P. J. (2016). A genome-wide association study for partial resistance to maize common rust. Phytopathology 106, 745–751. doi: 10.1094/PHYTO-11-15-0305-R
Passianotto, A. L. D. L., Sonah, H., Dias, W. P., Belzile, F., and Abdelnoor, R. V. (2017). Genome-wide association study for resistance to the southern root-knot nematode (Meloidogyne incognita) in soybean. Mol. Breed. 37:148. doi: 10.1007/s11032-017-0744-3
Pestsova, E., Ganal, M. W., and Röder, M. S. (2000). Isolation and mapping of microsatellite markers specific for the D genome of bread wheat. Genome 43, 689–697. doi: 10.1139/gen-43-4-689
Piepho, H. P., Mohring, J., Melchinger, A. E., and Buchse, A. (2008). BLUP for phenotypic selection in plant breeding and variety testing. Euphytica 161, 209–228. doi: 10.1007/s10681-007-9449-8
Pink, D. A. C., and Law, C. N. (1985). The effect of homoeologous group-7 chromosomes upon adult plant resistance of wheat to yellow rust (Puccinia striiformis). Plant Pathol. 34, 255–262. doi: 10.1111/j.1365-3059.1985.tb01357.x
Quan, W., Hou, G. L., Chen, J., Du, Z. Y., Lin, F., Guo, Y., et al. (2013). Mapping of QTL lengthening the latent period of Puccinia striiformis in winter wheat at the tillering growth stage. Eur. J. Plant Pathol. 136, 715–727. doi: 10.1007/s10658-013-0201-z
Rajarammohan, S., Pradhan, A. K., Pental, D., and Kaur, J. (2018). Genome-wide association mapping in Arabidopsis identifies novel genes underlying quantitative disease resistance to Alternaria brassicae. Mol. Plant Pathol. 7, 1719–1732. doi: 10.1111/mpp.12654
Ramburan, V. P., Pretorius, Z. A., Louw, J. H., Boyd, L. A., Smith, P. H., Boshoff, W. H. P., et al. (2004). A genetic analysis of adult plant resistance to stripe rust in the wheat cultivar Kariega. Theor. Appl. Genet. 108, 1426–1433. doi: 10.1007/s00122-003-1567-7
Rashid, Z., Singh, P. K., Vemuri, H., Zaidi, P. H., Prasanna, B. M., and Nair, S. K. (2018). Genome-wide association study in Asia-adapted tropical maize reveals novel and explored genomic regions for sorghum downy mildew resistance. Sci. Rep. 8:366. doi: 10.1038/s41598-017-18690-3
Ren, Y., He, Z. H., Li, J., Lillemo, M., Wu, L., Bai, B., et al. (2012). QTL mapping of adult-plant resistance to stripe rust in a population derived from common wheat cultivars Naxos and Shanghai 3/Catbird. Theor. Appl. Genet. 125,1211–1221. doi: 10.1007/s00122-012-1907-6
Riaz, A., Athiyannan, N., Periyannan, S. K., Afanasenko, O., Mitrofanova, O. P., Platz, G. J., et al. (2018). Unlocking new alleles for leaf rust resistance in the vavilov wheat collection. Theor. Appl. Genet. 131, 27–144. doi: 10.1007/s00122-017-2990-5
Röder, M. S., Korzun, V., Wendehake, K., Plaschke, J., Tixier, M. H., Leroy, P., et al. (1998). A microsatellite map of wheat. Genetics 149, 2007–2023.
Rosewarne, G. M., Herrera-Foessel, S. A., Singh, R. P., Huerta-Espino, J., Lan, C. X., and He, Z. H. (2013). Quantitative trait loci of stripe rust resistance in wheat. Theor. Appl. Genet. 126, 2427–2449. doi: 10.1007/s00122-013-2159-9
Rosewarne, G. M., Singh, R. P., Huerta-Espino, J., Herrera-Foessel, S. A., Forrest, K. L., Hayden, M. J., et al. (2012). Analysis of leaf and stripe rust severities reveals pathotype changes and multiple minor QTL associated with resistance in an avocet × pastor wheat population. Theor. Appl. Genet. 124, 1283–1294. doi: 10.1007/s00122-012-1786-x
Singh, D., Ziems, L. A., Dracatos, P. M., Pourkheirandish, M., Tshewang, S., Czembor, P., et al. (2018). Genome-wide association studies provide insights on genetic architecture of resistance to leaf rust in a worldwide barley collection. Mol. Breed. 38:43. doi: 10.1007/s11032-018-0803-4
Singh, R. P., Nelson, J. C., and Sorrells, M. E. (2000). Mapping Yr28 and other genes for resistance to stripe rust in wheat. Crop Sci. 40, 1148–1155. doi: 10.2135/cropsci2000.4041148x
Somers, D. J., Isaac, P., and Edwards, K. (2004). A high-density microsatellite consensus map for bread wheat (Triticum aestivum L.). Theor. Appl. Genet. 109, 1105–1114. doi: 10.1007/s00122-004-1740-7
Sourdille, P., Tavaud, M., Charmet, G., and Bernard, M. (2001). Transferability of wheat microsatellites to diploid Triticeae species carrying the A, B and D genomes. Theor. Appl. Genet. 103, 346–352. doi: 10.1007/s00122-001-0542-4
Stewart, C. N., and Via, L. E. (1993). A rapid CTAB DNA isolation technique useful for RAPD fingerprinting and other PCR applications. Biotechniques 14, 748–750.
Suenaga, K., Singh, R. P., Huertaespino, J., and William, H. M. (2003). Microsatellite markers for genes Lr34/Yr18 and other quantitative trait loci for leaf rust and stripe rust resistance in bread wheat. Phytopathology 93, 881–890. doi: 10.1094/PHYTO.2003.93.7.881
Sun, C. W., Zhang, F. Y., Yan, X. F., Zhang, X. F., Dong, Z. D., Cui, D. Q., et al. (2017). Genome-wide association study for 13 agronomic traits reveals distribution of superior alleles in bread wheat from the Yellow and Huai valley of China. Plant Biotechnol. J. 15, 953–969. doi: 10.1111/pbi.12690
Wan, A. M., Chen, X. M., and He, Z. H. (2007). Wheat stripe rust in China. Aust. J. Agr. Res. 58, 605–619. doi: 10.1071/AR06142
Wang, M., and Chen, X. M. (2017). “Stripe rust resistance,” in Stripe Rust, eds X. M. Chen and Z. S. Kang (Berlin: Springer), 353–558. doi: 10.1007/978-94-024-1111-9
Wellings, C. R. (2011). Global status of stripe rust: a review of historical and current threats. Euphytica 179, 129–141. doi: 10.1007/s10681-011-0360-y
William, H., Singh, R., Huerta-Espino, J., Palacios, G., and Suenaga, K. (2006). Characterization of genetic loci conferring adult plant resistance to leaf rust and stripe rust in spring wheat. Genome 49, 977–990. doi: 10.1139/g06-052
Yang, H., Li, C., Lam, H. M., Clements, J., Yan, G., and Zhao, S. (2015). Sequencing consolidates molecular markers with plant breeding practice. Theor. Appl. Genet. 128, 779–795. doi: 10.1007/s00122-015-2499-8
Yu, J., Pressoir, G., Briggs, W. H., Bi, I. V., Yamasaki, M., Doebley, J. F., et al. (2006). A unified mixed-model method for association mapping that accounts for multiple levels of relatedness. Nat. Genet. 38, 203–208. doi: 10.1038/ng1702
Yuan, F. P., Zeng, Q. D., Wu, J. H., Wang, Q. L., Yang, Z. J., Liang, B. P., et al. (2018). QTL mapping and validation of adult plant resistance to stripe rust in Chinese wheat landrace Humai 15. Front. Plant. Sci. 9:968. doi: 10.3389/fpls.2018.00968
Zhang, Y. L., Liu, P., Zhang, X., Zheng, Q., Chen, M., Ge, F., et al. (2018). Multi-locus genome-wide association study reveals the genetic architecture of stalk lodging resistance-related traits in maize. Front. Plant Sci. 9:561. doi: 10.3389/fpls.2018.00611
Keywords: strip rust, adult-plant resistance, Chinese wheat landraces, genome-wide association study, Diversity Arrays Technology, simple sequence repeat
Citation: Long L, Yao F, Yu C, Ye X, Cheng Y, Wang Y, Wu Y, Li J, Wang J, Jiang Q, Li W, Ma J, Liu Y, Deng M, Wei Y, Zheng Y and Chen G (2019) Genome-Wide Association Study for Adult-Plant Resistance to Stripe Rust in Chinese Wheat Landraces (Triticum aestivum L.) From the Yellow and Huai River Valleys. Front. Plant Sci. 10:596. doi: 10.3389/fpls.2019.00596
Received: 26 November 2018; Accepted: 24 April 2019;
Published: 16 May 2019.
Edited by:
Anna Maria Mastrangelo, Research Centre for Industrial Crops, Council for Agricultural Research and Economics, ItalyReviewed by:
Marion S. Röder, Leibniz-Institut für Pflanzengenetik und Kulturpflanzenforschung (IPK), GermanyMarco Maccaferri, University of Bologna, Italy
Copyright © 2019 Long, Yao, Yu, Ye, Cheng, Wang, Wu, Li, Wang, Jiang, Li, Ma, Liu, Deng, Wei, Zheng and Chen. This is an open-access article distributed under the terms of the Creative Commons Attribution License (CC BY). The use, distribution or reproduction in other forums is permitted, provided the original author(s) and the copyright owner(s) are credited and that the original publication in this journal is cited, in accordance with accepted academic practice. No use, distribution or reproduction is permitted which does not comply with these terms.
*Correspondence: Guoyue Chen, gychen@sicau.edu.cn