- 1 Department of Biochemistry, Biophysics and Molecular Biology, Iowa State University, Ames, IA, USA
- 2 Department of Plant Biology, Carnegie Institution for Science, Stanford, CA, USA
- 3 Bioinformatics and Computational Biology Program, Iowa State University, Ames, IA, USA
- 4 Department of Statistics, Iowa State University, Ames, IA, USA
- 5 Genome Center, University of California, Davis, CA, USA
- 6 M. J. Murdock Metabolomics Laboratory, Institute of Biological Chemistry, Washington State University, Pullman, WA, USA
- 7 Department of Biotechnology, College of Life Science and Biotechnology, Yonsei University, Seoul, Korea
- 8 Anatomy and Neurobiology, Virginia Commonwealth University, Richmond, VA, USA
- 9 Department of Biological Sciences, University of North Texas, Denton, TX, USA
- 10 Virginia Bioinformatics Institute, Virginia Polytechnic Institute and State University, Blacksburg, VA, USA
- 11 Plant Biology Division, The Samuel Roberts Noble Foundation, Ardmore, OK, USA
- 12 Division of Biology, Kansas State University, Manhattan, KS, USA
- 13 Department of Genetics, Development and Cell Biology, Iowa State University, Ames, IA, USA
Metabolomics is the methodology that identifies and measures global pools of small molecules (of less than about 1,000 Da) of a biological sample, which are collectively called the metabolome. Metabolomics can therefore reveal the metabolic outcome of a genetic or environmental perturbation of a metabolic regulatory network, and thus provide insights into the structure and regulation of that network. Because of the chemical complexity of the metabolome and limitations associated with individual analytical platforms for determining the metabolome, it is currently difficult to capture the complete metabolome of an organism or tissue, which is in contrast to genomics and transcriptomics. This paper describes the analysis of Arabidopsis metabolomics data sets acquired by a consortium that includes five analytical laboratories, bioinformaticists, and biostatisticians, which aims to develop and validate metabolomics as a hypothesis-generating functional genomics tool. The consortium is determining the metabolomes of Arabidopsis T-DNA mutant stocks, grown in standardized controlled environment optimized to minimize environmental impacts on the metabolomes. Metabolomics data were generated with seven analytical platforms, and the combined data is being provided to the research community to formulate initial hypotheses about genes of unknown function (GUFs). A public database (www.PlantMetabolomics.org) has been developed to provide the scientific community with access to the data along with tools to allow for its interactive analysis. Exemplary datasets are discussed to validate the approach, which illustrate how initial hypotheses can be generated from the consortium-produced metabolomics data, integrated with prior knowledge to provide a testable hypothesis concerning the functionality of GUFs.
Introduction
The biochemical and physiological functions of a large proportion of the 28,692 unique genes in the Arabidopsis genome are experimentally undetermined (TAIR November 2010)1. These genes fall into two categories: (1) genes whose function cannot be ascribed based upon any sequence homology [i.e., genes that either share no sequence homology to any gene in sequence databases, or share homology to genes of unknown function (GUFs)] – approximately 9000 of the annotated genes fall in this category; and (2) genes whose function can be classified, based on sequence homology, in terms of broad functional categories (e.g., phosphatase, kinase, etc.), but the exact biochemical and physiological function of the encoded protein remains elusive – approximately 15,000 genes fall in this latter category. Since completion of the sequencing of the Arabidopsis genome in 2000 (AGI, 2000), various governmental research-funding agencies have supported the development of community resources and the application of different technologies to identify biochemical and physiological functions of these GUFs. These resources include the development of large mutant collections, mutant phenotype data, global expression profiling of RNA and protein gene products, protein–protein interaction data, and identification of the location of gene products at the cellular/tissue and subcellular organelle levels (Somerville and Dangl, 2000; Shinozaki and Sakakibara, 2009; MASC, 2010). This manuscript describes the out come of a multi-disciplinary experimental system that has been developed within this context, to generate and evaluate metabolomics data as a tool for deciphering gene function in Arabidopsis.
Metabolomics is the large-scale profiling of the pool of small organic molecules (molecular weight ≤1000), which are acted upon and chemically interconverted by enzymes. Collectively these small organic molecules that are substrates and products of enzyme-catalyzed reactions define the metabolome of a biological sample (Fiehn et al., 2000; Hall et al., 2002). By identifying and quantifying the metabolome of a biological sample, metabolomics defines the steady-state levels of the intermediates of metabolic networks that constitute the sample, i.e., the metabolic phenotype. These data articulate the final expression (output) of the genome at the molecular level. Hence it follows that comparing the metabolome of a wild-type sample to that of a sample altered by a mutation at a target gene (or some other perturbation of the metabolic network) will provide clues for the function of that targeted gene, and thus help define the basis for a biological trait or biochemical phenotype associated with that allele. The strategy of globally comparing outcomes of gene expression at different molecular levels (i.e., transcriptomics and proteomics) has been at the heart of functional genomics (Steinhauser et al., 2004; Winter et al., 2007; Hruz et al., 2008; Mentzen and Wurtele, 2008; Mentzen et al., 2008). More recently metabolomics has been used to characterize specific metabolic networks, including those associated with biotic and abiotic stresses (Broeckling et al., 2005), phenylpropanoid, and isoflavonoid biosynthesis (Farag et al., 2008), glucosinolate metabolism (Wentzell et al., 2007), starch metabolism (Messerli et al., 2007), chloroplast-targeted gene products (Lu et al., 2008, 2011; Ajjawi et al., 2010), and flavonol metabolism (Yonekura-Sakakibara et al., 2008).
Because the functionality of the GUFs is by definition undefined, it is near impossible to predict the metabolites whose accumulation may be altered due to a loss-of-function allele at a GUF locus. It is therefore desirable that the analytical technology used to assess the metabolome of a mutant sample be as comprehensive as possible. However, no complete metabolite list is available for any organism. Moreover, even if such a list were available, due to the diversity of chemical and physical properties of metabolites, and the technical limitations in the dynamic range of chemical detectors available to researchers, it would be a challenging proposition to assess the entire metabolome of an organism. These technical limitations can be partially surmounted by a combination of two strategies. First, metabolomics can be conducted with different analytical detectors (e.g., mass spectrometers, fluorescence, UV/VIS absorbance, IR absorbance, NMR), and each of these detectors can be used in combination with different separation technologies [e.g., gas chromatography (GC), liquid chromatography (LC), capillary electrophoresis (CE)]. Thus, by combining different separation technologies with different detection systems, it should be possible to expand the types of metabolites that can be analyzed, and therefore cast a broader net for capturing and measuring metabolites with vastly different chemical properties. Second, in contrast to the analytical approaches in which global analyses of metabolites are conducted independent of the chemical and physical properties of the metabolites, targeted metabolite analysis can be employed. In this strategy metabolites are initially partially purified or enriched prior to analysis, increasing the sensitivity of the analysis. Therefore, by combining multiple such targeted metabolic profiling strategies with different metabolomics platforms, one gains both breadth and depth in the coverage of the samples’ metabolomes.
This manuscript reports on the assembly of a consortium of metabolomics and metabolite-profiling laboratories, which unifies the advantages offered by different analytical approaches to determine the effect of mutations in GUFs on the metabolome of the tissue. This consortium (The Arabidopsis Metabolomics Consortium) uses parallel technologies for the analysis of a large number of metabolites. In partnership with biochemists, biostatisticians, and bioinformaticists, the consortium has developed resources that can be used for generating sophisticated hypotheses regarding the metabolic and physiological functions of Arabidopsis GUFs. The cumulative data are available to the community via the project database2 (Bais et al., 2010). These data and resulting hypotheses provide the basis for additional informed and targeted experimentation necessary for the empirical validation of the biochemical and physiological functions of Arabidopsis GUFs.
Results
Analytical Platforms
The rationale of the Arabidopsis Metabolomics Consortium (see footnote 2) is to combine parallel analytical outputs from five laboratories that conduct metabolite-profiling studies on aliquots of the identical plant material, and thus maximize the portion of the metabolome that can be interrogated. In combination, the analytical laboratories generate metabolite abundance data for about 1500 metabolites (Table 1). Approximately two-thirds of these data were obtained from four non-targeted metabolomics approaches, each of which utilized different analytical platforms: gas chromatography time-of-flight mass spectrometry (GC–TOFMS; Fiehn group), ultra-high pressure liquid chromatography–quadrupole time-of-flight mass spectrometry (UHPLC–QTOFMS; Sumner group), capillary electrophoresis mass spectrometry (CE–MS; Shulaev group), and liquid chromatography mass spectrometry (LC–MS; Shulaev group). These non-targeted approaches capture abundance information on metabolites involved in primary metabolism, including amino acids, organic acids, fatty acids, alcohols, carbohydrates, nucleosides, and secondary metabolites, including chalcones, flavonoids, flavonoid O-glycosides, glucosinolates, and terpenoids. In addition to the non-targeted approaches, the Consortium also used five targeted profiling methods to measure levels of glycerolipids, fatty acids, cuticular waxes, phytosterols/tocopherols, and chlorophylls/carotenoids. Currently, of the approximate 1500 analytes that are routinely detected, approximately 730 are chemically defined (Table 1; Table S1 in Supplementary Material).
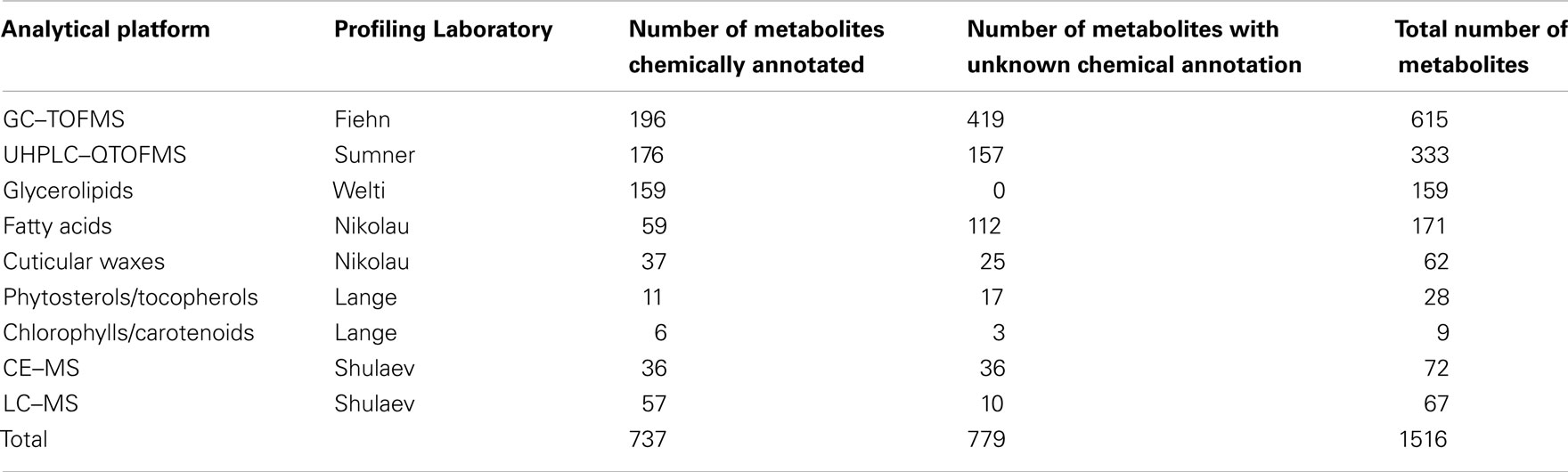
Table 1. Summary of metabolites/compounds identified by the analytical laboratories in the Arabidopsis Metabolomics Consortium.
Merging data from such multiple analytical platforms requires considerable care and attention to detail. Issues that were encountered include inconsistent naming of samples, and metabolites or analytes, inconsistent organization of the tabular data, and the need to accurately label and distinguish analytes whose chemical identities were not established. The latter is particularly an issue in establishing the degree of redundancy in the analyte abundance data generated by the independent platforms. Overcoming these organizational issues were primarily managed by a single “gatekeeper” who manually curated data prior to entry and release on the project database. In all cases, such inconsistencies were clarified by direct feedback from the analytical lab responsible for generating the data for each analytical platform.
In addition to these organizational and managerial issues, combining data from independent platforms required normalization of the data to ensure consistency in the data-structure, and thus provide users a basis for extracting useful knowledge from the integrated datasets. For example, some platforms reported analyte abundances as integrated peak areas, whereas others had the ability to report concentrations per biomass dry weight. The issues presented by this complexity were overcome by integrating two normalization protocols. First, each analytical platform normalized the data relative to an internal chemical standard, which was added to the tissue to a known concentration prior to extraction. The chemical nature of this internal standard was specific to each analytical platform, and all metabolite and analyte peaks were normalized relative to this spiked standard. Second, each platform conducted parallel analyses on aliquots the same mutant and wild-type tissue samples, and for each analyte the relative ratio of its abundance in the two tissue samples was calculated. The statistical evaluation of the entire metabolome used these ratio data to calculate a statistical distance measure that was invariant to arbitrary scaling of each metabolite. The specific distance measure used was primarily the Canberra distance and variance-weighted distance (Dixon, in preparation), which is invariant to arbitrary scaling of each analyte.
As an initial evaluation of the strategy to combine datasets, the Consortium conducted a Pilot Study that evaluated the metabolomes of 18 Arabidopsis mutants (Table S2 in Supplementary Material), combining metabolite abundance data gathered from these different analytical platforms. Figure 1 integrates the resulting dataset identifying the number of metabolites whose abundance was significantly altered in each mutant as revealed by each analytical platform. These analyses establish that by combining datasets, access to substantially larger portion of the metabolome was gained than was possible with any single platform individually. In addition, each analytical platform revealed significantly altered metabolites in most of the mutants analyzed and the platform that revealed the majority of the altered compounds differed among the individual mutants. For example, the fatty acid platform detected the largest number of significant metabolite changes in the At2g42560 mutant (SALK_063167), whereas the cuticular wax platform revealed the largest number of metabolite differences in the At5g19070 mutant (SALK_074697). This enhanced capability therefore provides considerably more metabolic information concerning the functionality of the gene that has been targeted for analysis.
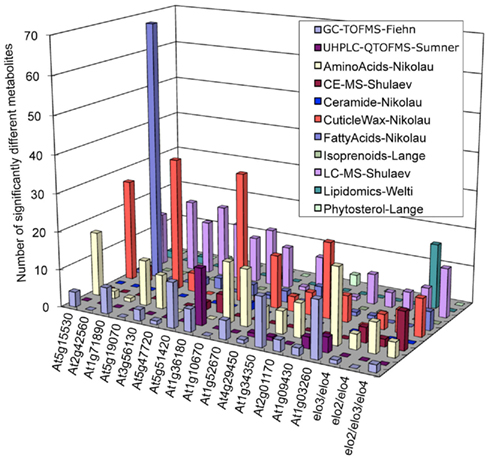
Figure 1. Distribution of significantly altered metabolites among different mutants as detected by different analytical platforms (identified in the insert).
Analysis of Metabolite Abundances
To illustrate the efficacy of the Consortium, nine series of metabolomics studies, referenced as Experiments 1–9 (E1–E9) were conducted on Arabidopsis stocks carrying T-DNA mutant alleles. These experiments included profiling mutant stocks in genes of known function (GKF) and GUFs. Thirty-nine T-DNA mutant lines were selected based on their availability and expert knowledge concerning each line that already existed within the Consortium. An additional 64 GUF lines were selected based on an association network (Lee et al., 2010), which included sequence homology data, coexpression with GKF, information mined from literature and similarity of phylogeny with GKF. Detailed information concerning the selection of these GUFs is accessible on the project database. The focus of this paper is on the results of the initial three metabolomic experiments, E1, E2, and E3, which collectively evaluated the metabolomes of 69 mutant lines; a complete list of the T-DNA mutant lines used in these experiments is identified in Table S3 in Supplementary Material and within the project database www.PlantMetabolomics.org (Bais et al., 2010). The mutants analyzed in E1, E2, and E3 were randomly placed in each of the three experiments using a random number generator to assign subjects to groups3.
Distinguishing between Genotype-Based and Environmentally Induced Changes in the Metabolome
A major goal of the Consortium is to reveal genotype-based differences in the metabolomes of the mutant stocks. However, the Consortium had to initially cope with the fact that the metabolic status of plants is altered with shifting environmental conditions, and that plants have evolved complex mechanisms to mediate alterations in gene expression and changes in rates of enzyme-catalyzed reactions. These alterations in cellular and metabolic processes manifest changes in steady-state levels of metabolic intermediates, i.e., changes in the metabolome (Nikiforova et al., 2005).
Therefore, to test whether the growth, analytical, and data interpretation pipelines could reveal genotype-based differences in the metabolome (in contrast to environmentally induced changes) as a means of validating these platforms, a Pilot Study referenced as the environmental impact experiment (EIE) “EIE2” was conducted. In this experiment, seedlings of wild-type and a T-DNA knockout line SALK_021108 (carrying a mutant allele in the GUF, At1g52670) were exposed to environmental alterations (light intensity, temperature, and desiccation stress) well beyond the boundaries of the Consortium’s standard growth conditions (Table 2). As expected, analysis of the metabolomics data obtained from this experiment indicated that each of these environmental perturbations affected the metabolome (Figure 2). Moreover, statistical analyses indicated by the visual separation of wild-type samples from mutant samples, that it is possible to distinguish the mutant metabolome from that of the wild-type irrespective of the environmental perturbation. Namely, statistical distances among mutant and wild-type samples are smaller than the distances between them. Therefore, this example illustrates that by maintaining the growth conditions within a narrow range of temperature (±2°C), illumination intensity (±10 μE m−2 s−1), and harvesting timeline (<2 min), the observed changes in the metabolome will not reflect the effect of environmental pressures on metabolism but rather reflect the consequence of genetic influence of the mutant alleles.
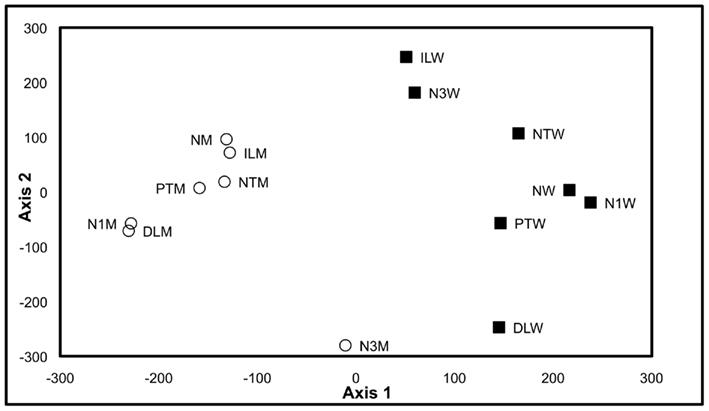
Figure 2. Multi-dimensional scaling plot of Environmental Impact Experiment. Multi-dimensional scaling plot of the data generated in the Environmental Impact Experiment reveals a clear separation of wild-type samples (filled squares) from the mutant samples (open circles). Standard “normal” growth conditions for the wild-type and At1g52670 (SALK_021108) mutant allele are denoted as NW and NM, respectively. Similarly environmental perturbations (described in Table 2) labeled on MDS plot for wild-type and mutant samples are as follows: positive temperature change (PTW and PTM), negative temperature change (NTW and NTM), decreased light intensity (DLW and DLM), increased light intensity (ILW and ILM), 1 h harvest delay (N1W and N1M), and 3 h harvest delay (N3W and N3M). This plot indicates that the metabolomes of the wild-type samples and mutant samples can be differentiated even though environmental growth conditions were perturbed beyond the normal limits of the standard growth conditions defined in the Section “Materials and Methods.”
Validation of Hypothesis Generation from Metabolomics Data
As a means of validating the accuracy of hypotheses generated from this combined metabolomics platform, the metabolome of a mutant stock whose biochemical functionality is well established was evaluated. The mutant selected for this validation experiment is a gene involved in glutathione metabolism. In plants, glutathione (GSH) plays important roles, including detoxifying photosynthetically generated hydrogen peroxide, chelating heavy metal ions and controlling cell size, and root development (Ohkama-Ohtsu et al., 2008). This validation experiment used the mutant stock (SALK_078745), which carries a T-DNA insertion knockout in At5g37830 that encodes for 5-oxoprolinase (5OPase). This enzyme catalyzes the conversion of 5-oxoproline (5OP) to glutamate (Glu), and was initially discovered in plants in 1976 (Mazelis and Pratt, 1976). The involvement of 5OPase in the degradation of GSH in Arabidopsis was established by Ohkama-Ohtsu et al. (2008) who characterized this mutant. Inclusion of this GKF insertion line in the metabolomics analyses has allowed the Consortium to test its ability to generate an accurate hypothesis. Further these analyses provide potentially new information as to the consequences of knocking out OXP1 on the metabolome of plants.
Ratio plot analysis of the metabolomics data from SALK_078745 mutant identifies the hyper- and hypo-accumulating metabolites, and visualizes the error associated with the ratio calculation for each metabolite (Figure 3). Statistical analysis (Student’s t-test) of the log-transformed abundance data reveal 129 metabolites that are significantly different between the oxp1 mutant and the wild-type. Adjusting for a false discovery rate from multiple hypothesis testing based on the Benamini and Hochberg (1995) algorithm, reduced this difference to nine metabolites that have a p-value less than 0.05. Four of these nine metabolites are annotated as unknown, leaving five chemically defined metabolites, which are oxoproline, melibiose, succinic acid, malic acid, and 4-benzyloxy-n-butyl-glucosinolate. Seven of these significantly altered metabolites hyper-accumulate and the other two hypo-accumulate in the mutant, with oxoproline displaying the largest abundance change (Table 3). The fact that oxoproline displays the largest change in abundance in the mutant recapitulates the prior finding that At5g37830 encodes for 5OPase (Ohkama-Ohtsu et al., 2008). Moreover, the additional metabolic changes revealed by these analyses are an indication of the pleiotropic consequence of altering glutathione metabolism and these can be further explored by mapping the chemically defined metabolites on to metabolic pathways.
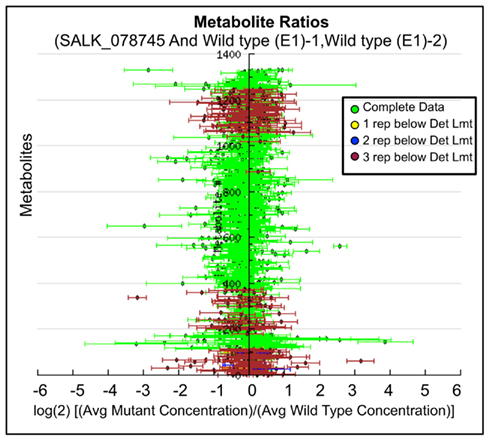
Figure 3. Log-ratio plot of the metabolome of the oxp1 (SALK_078745) mutant. The y-axis plots individual metabolites. The x-axis plots log-transformed relative ratio of abundance of each metabolite in the mutant sample normalized to the levels of that metabolite in the wild-type control sample. The calculation of SE is described in the Section “Materials and Methods.”
Hypothesis Generation from Metabolomics Data for Genes of Unknown Functions
To illustrate how the integrated metabolomics data can be coupled with prior knowledge to provide a substantive hypothesis concerning the functionality of a GUF, a specific example of a hypothesis generated from the Consortium’s metabolomics analyses is discussed. This example is the metabolomics analysis of the mutant stock SALK_092408 in the gene At4g29540. The GO Molecular Function (Berardini et al., 2004) annotation associated with At4g29540 is “transferase activity,” and TAIR annotates this gene as a “bacterial transferase hexapeptide repeat-containing protein; similar to bacterial transferase hexapeptide repeat-containing protein” (TAIR, August 2010). Sequence comparison analysis reveals that the protein encoded by At4g29540 shares low sequence identity (28% at the translated amino acid level) with the E. coli lpxA gene that is involved in Lipid A biosynthesis (Raetz and Whitfield, 2002). Lipid A is the glucosamine-based phospholipid domain of the lipopolysaccharide that makes up the outer monolayer of the outer membrane of most Gram-negative bacteria. Although there have been scattered reports that Lipid A may occur in plants (Raetz and Whitfield, 2002; Armstrong et al., 2006), it is generally believed that Lipid A is a molecule characteristic only of Gram-negative bacteria (Raetz and Whitfield, 2002; Raetz et al., 2007). In silico analysis has revealed that the Arabidopsis genome contains distant homologs of six Lipid A biosynthetic genes (lpxA, At4g29540; lpxB, At2g04560; lpxC, At1g25210; lpxD, At4g05210; lpxK, At3g20480; and kdtA, At5g03770; Raetz and Whitfield, 2002; Liu et al., 2003). Further bioinformatics analysis of plant genomes reveals that in addition to the above listed homologs, the Arabidopsis genome contains additional 4 paralogs of lpxC (At1g24793, At1g24880, At1g25054, and At1g25141), and 1 additional paralog of lpxD (At4g21220). To better understand the role of these Lipid A biosynthetic homologs in plants, the metabolome of the At4g29540 mutant was profiled and characterized.
Analysis of the SALK_092408 mutant allele (lpxA-homolog, At4g29540) was done similarly to the analysis of oxp1 mutant. False discovery rate adjusted p-values of t-test analyses failed to identify significant changes in any single metabolite abundances in the aerial tissues of this mutant relative to the wild-type (p-value of <0.1; data not shown). Therefore, to allow for the parallel analysis of all collected metabolite abundance data, a distance matrix calculation (see Materials and Methods for equation) was used to generate a statistical basis for comparing the metabolomes of all mutants evaluated within the E3 experiment. This experiment analyzed the metabolomes of 25 mutants, which included mutants in 12 GUFs, and 13 mutants whose functionality was defined by some prior experimentation and annotated with GO molecular function terms.
The statistical distances among the mutant metabolomes is represented by a hierarchical tree, which visualizes the relative metabolic differences among the 25 mutants (Figure 4). This representation indicates for example that the mutation in the GUF At1g35710 is inconsequential to the metabolome of Arabidopsis, as it maps closest to the wild-type. In contrast, the mutation in gene At1g36180, which encodes for one of the two acetyl-CoA carboxylases genes involved in the biosynthesis of fatty acids and/or malonyl-CoA derived secondary metabolites generates the largest change in the Arabidopsis metabolome. Interestingly, the metabolome that is most similar to this gene is associated with the mutant in At5g07990, which encodes flavonoid 3′-hydroxylase, an enzyme involved in the biosynthesis of malonyl-CoA derived flavonoids (Winkel-Shirley, 2001; Tohge et al., 2007). Further validation of this metabolome-based clustering approach for revealing similarities in gene functions is the finding that At1g09430 and At4g25000 are closely associated. The former encodes one of the ATP-citrate lyase subunits (Fatland et al., 2002), and the latter encodes an alpha-amylase gene involved in starch metabolism (Smith et al., 2005). The significance of this close association between these two metabolomes lies in the finding that knocking-down ATP-citrate lyase activity results in the hyper-accumulation of starch (Fatland et al., 2005). Although this association was surprising when first described, it is further substantiated with the current broader analysis of the metabolomes associated with mutations in each of these two genes.
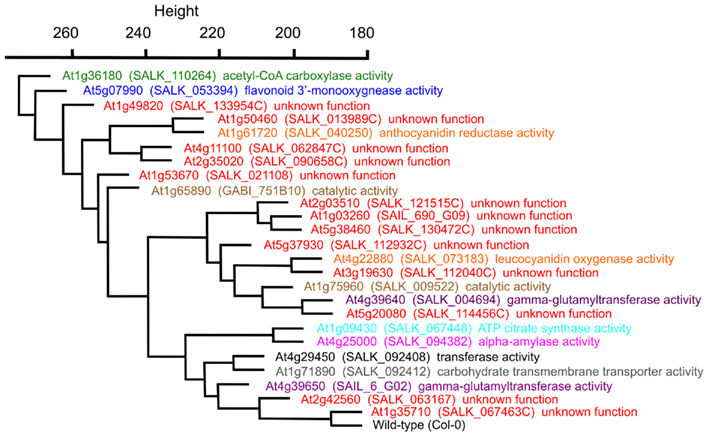
Figure 4. Hierarchical cluster diagram of mutant alleles from Experiment 3 (E3). The dissimilarity between a pair of genes was computed from the mutant metabolomes using a variance-weighted Manhattan distance measure described in the Section “Materials and Methods,” and this distance measurement was used to generate the cluster diagram. The specific mutant allele used to characterize each gene locus, and the GO Molecular Function term that annotates each locus is identified.
In terms of the Arabidopsis lpxA-homolog, At4g29540, the metabolome of this mutant is most similar to At1g71890. The GO molecular function annotation for this latter gene is: “carbohydrate transmembrane transporter activity, sucrose:hydrogen symporter activity, sugar:hydrogen symporter activity,” and it has also been implicated as a transporter of biotin (Ludwig et al., 2000). The similarities in the metabolomes of At4g29540 and At1g71890 mutants implicate a similar metabolic function for these two genes, but that interconnection is not necessarily apparent from the current datasets.
The parallel collection of morphological data within the Consortium, revealed that the At4g29540 (SALK_092408; lpxA-2) mutant showed a subtle visible growth phenotype; namely the failure to develop root hairs (Figure 5). This root hair phenotype recapitulates in a second, independently isolated mutant stock (lpxA-1), which occurs in the Wassilewskija (Ws) ecotype background, isolated from the T-DNA insertion collection made available at The Arabidopsis Knockout Facility at the University of Wisconsin-Madison (Sussman et al., 2000). To ensure that this phenotype was caused by the mutations at the At4g29540 locus, a genetic allelism test was performed by intercrossing the lpxA-2 and lpxA-1 alleles. The recovery of the root hair phenotype in the heteroallelic plants that carry both the lpxA-2 and lpxA-1 alleles establish that the lack of At4g29540-functionality is the cause of this phenotype.
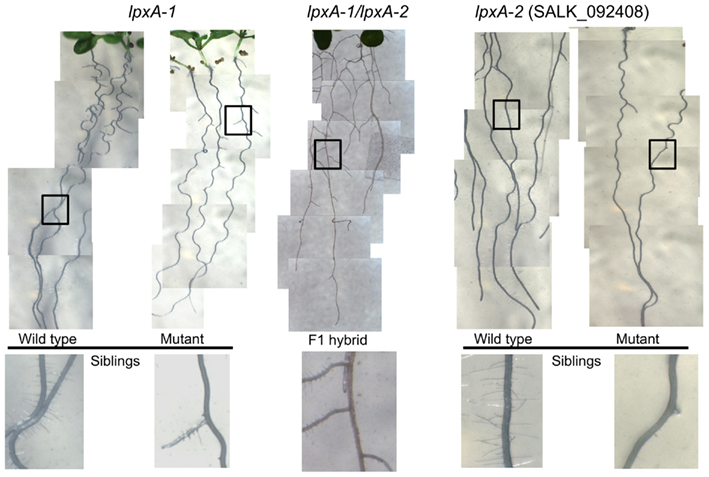
Figure 5. Root phenotype analysis of lpxA-1 and lpxA-2 (SALK_092408) alleles. The heteroallelic cross of lpxA-1 and lpxA-2 (middle) recapitulates the loss of root hair phenotype.
Therefore, a focused metabolomics analysis was performed upon roots, applying the targeted platforms of lipidomics, fatty acids, amino acids, and ceramides. This analysis revealed major differences in the fatty acid and ceramide profiles of the mutant roots. Specifically, an increase in the abundance of fatty acids with odd-numbered carbon atoms and a distinctive accumulation of ceramides that contain 2-hydroxy-fatty acids with odd-numbered carbon atoms (i.e., 19, 21, 23, 25 carbons; Figure 6).
Genetic complementation was used to explore the possible functionality of At4g29540 in catalyzing an acyl-transferase reaction analogous to the lpxA-catalyzed reaction in lipid A biosynthesis. The codon-optimized At4g29540 cDNA lacking the N-terminal organelle-targeting sequence was expressed with the vector pUC57 into the E. coli strain SM101, which harbors the temperature sensitive lpxA-2(ts) allele that leads to a lethal phenotype at 42°C (Galloway and Raetz, 1990). The SM101 strain expressing the At4g29540 cDNA grew at 42°C, whereas the control strain transformed with the empty pUC57 vector failed to grow at this non-permissive temperature (Figure 7). Therefore, this genetic complementation experiment demonstrates that At4g29540-encoded protein has the capacity to catalyze an acyl-transferase reaction that is required in the first step of lipid A biosynthesis in E. coli.
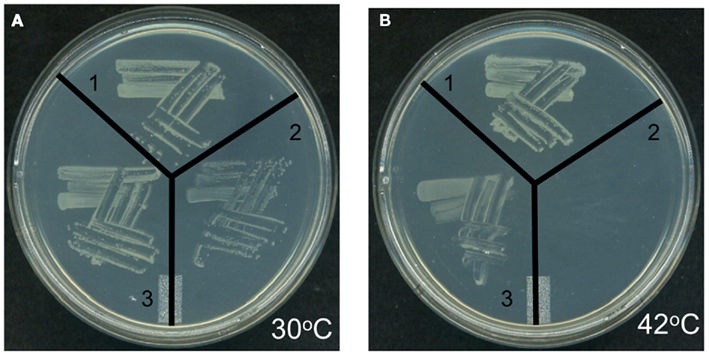
Figure 7. Genetic complementation of E. coli temperature sensitive lpxA-2(ts) allele with AtlpxA (At4g29540). (A) E. coli strains carrying the wild-type LpxA allele (strain SM105) (1), or the temperature sensitive lpxA-2(ts) allele (strain SM101) were transformed with the empty pUC57 vector (2) and recombinant pUC57 vector expressing the At4g29540 cDNA (3). These strains were grown at the permissive temperature, 30°C (A) and non-permissive temperature, 42°C (B). Complementation is evidenced by the fact that the lpxA-2(ts) strain expressing the At4g29540 cDNA grew at 42°C, whereas this mutant strain transformed with the control empty vector failed to grow at this non-permissive temperature.
These combined datasets lead to the following hypotheses: (1) At4g29540 catalyzes an acyl-transferase reaction in Arabidopsis, as evidenced by its ability to complement the E. coli lpxA mutant strain SM101; and (2) the inability of At4g29540 mutant to form root hairs is due either to the lack of metabolite product(s) that require At4g29540-functionality, or to the accumulation of the alternative metabolite(s) that accumulate in the absence of this functionality. These are testable hypotheses that could not have been formulated in the absence of the metabolomics data generated by the Consortium. Thus, integrating metabolomics data with other biological data, leads to the formulation of detailed hypotheses that can be further explored by the research community.
For example, similar analyses of the other Arabidopsis homologs of the Lipid A biosynthetic genes (i.e., At1g24793, At1g24880, At1g25054, At1g25141, At1g25210, At2g04560, At3g20480, At4g05210, At4g21220, and At5g03770) would further test whether these occur in a common pathway in Arabidopsis, as they occur in bacteria. If so, it is expected that the metabolomes of these latter mutants will mirror the metabolomic changes that are detected in the At4g29540 mutant. Indeed, recent detailed analyses of the subcellular location of these Arabidopsis protein homologs, and analyses of mutants in these genes indicate that they are part of a mitochondrial lipid metabolism pathways that may generate a Lipid X molecule, which itself is a known intermediate in the biosynthesis of Lipid A in bacteria (Li et al., 2011). Although the final lipid product of this Arabidopsis pathway is still to be identified, the collected datasets indicate a connection between mitochondrial lipid metabolism, ceramide metabolism, and root hair formation.
Discussion
By combining the analytical capabilities of six metabolomics laboratories, this consortium has the ability to assess the accumulation of about 1500 metabolite analytes of Arabidopsis, and of these, 730 metabolites are accurately annotated relative to their chemical identity. Analysis of the altered metabolites in each Arabidopsis mutant illustrates that continuing the use of these diverse analytical platforms will ensure that important metabolic differences for a diversity of different GUFs are captured. The PlantMetabolomics database provides downloadable data to researchers to allow independent evaluation and generation of their own hypotheses, autonomous of the consortium’s interpretation. Also provided are tools for additional processing of the data that can aid interpretation by researchers, particularly those who are not familiar with metabolomics data. Specifically, the database can generate metabolite abundance ratio plots between any particular mutant and the appropriate wild-type controls, clustering analyses, multi-dimensional scaling (MDS) capabilities, principle component analysis plots, and random forest classifiers (Spearman, 1987; Breiman, 2001; Seber, 2008). Therefore hypotheses concerning the functionality of the GUFs can be constructed based on these statistical visualization outputs. Additional resources available in the website include metadata, detailing the protocols for metabolite extraction and analysis, metabolite annotation pages that link to other databases, and search tools based on metabolite names and pathway annotations. Thus, the database allows the research community to be involved in interpreting the outcomes of the metabolomics data generated by this consortium. The exemplary analyses provided herein demonstrate how a community user can utilize the consortium data, and database functionalities to generate a hypothesis concerning GUFs. Analogous analysis of a GKF tested the soundness of the generated hypothesis and this outcome should provide confidence to the community users on validity of the generalized approach.
The use of metabolomics data to reveal gene functionality is of particular significance in the case of GUFs that fail to generate a readily visible morphological phenotype in the mutant state. Similar efforts have focused on determining the metabolomes of genes encoding for chloroplast-targeted proteins (Lu et al., 2008, 2011; Ajjawi et al., 2010) and mutants that present an altered starch metabolism phenotype (Messerli et al., 2007). The analyses conducted within this consortium indicate that such silent mutations will present an altered metabolome. The GUFs used in these analyses were preselected on the basis that they presented a silent mutant morphological phenotype. To date, the consortium has analyzed 69 mutant alleles (Experiments E1–E3), and none of these present an unaltered metabolome, i.e., these are not silent mutants at the level of metabolic consequence. Using a threshold log-ratio change of between −2 and +2 as an indication of altered metabolite abundance, five mutant alleles showed less than 10 changes in metabolite abundance (the minimum number of detected metabolic changes was 4 metabolites, associated with the mutation in At1g58030), 55 mutant alleles show between 10 and 30 metabolic changes, and 9 mutant alleles showed more than 30 altered metabolite abundances; the highest number of detected altered metabolites being 46.
By focusing subsequent studies on these altered biochemical changes that are the consequence of a specific mutation, one has the ability to work backward from the metabolome to the mutant gene and extract knowledge concerning the functionality of that gene. Extracting biological significance of the altered metabolome relies on the accurate chemical annotation of the altered metabolites. Although the fact that more than half of the detected metabolome is chemically undefined limits this capability, the illustrated examples validate this approach. In the instance of the At5g37830 locus, our “blind” analyses rediscovered the known functionality of this gene by revealing the hyper-accumulation of its substrate, 5-oxoproline, as one of the few metabolic changes associated with mutations in this gene. In the second illustrative example, mutations in At4g29540, revealed metabolic and morphological alterations, and these provide a guide to experimentalists to enable mapping relationships between biochemical and physiological responses of the mutant, and provide a means for formulating more constrained hypotheses relative to the functionality of the GUF.
Materials and Methods
Genetic Material
Seed stocks of Arabidopsis thaliana mutants used in these studies, in ecotype Col-0 background (Alonso et al., 2003), were obtained from ABRC. These stocks were propagated at Iowa State University, and stocks homozygous for the mutant allele were generated (Tables S2 and S3 in Supplementary Material).
Plant Growth Conditions
Standardized plant growth conditions were used throughout these studies to assess the effect of mutations on the metabolome of Arabidopsis. Details of these growth conditions are provided in the Supplementary Material and Plantmetabolomics.org. Arabidopsis seedlings were grown in Petri dishes on defined sterile growth medium. This growth medium contained mineral salts supplemented with defined vitamin-mix and 0.1% (w/v) sucrose. Growth conditions (temperature, illumination, and humidity) were strictly controlled and maintained within strict limits to minimize environmentally induced alterations in metabolism. Specifically, plants were grown for 16-days under constant illumination (50 ± 10 μE m−2 s−1), constant temperature of 24 ± 2°C, and at 100% relative humidity.
The exception to standard growth regime was used in the Pilot Study EIE. In this experiment, plants were transferred to different environments (at different temperatures or illumination levels) during the last 24-h of growth (i.e., 16-days after transfer to the standard growth-room; Table 2). Dishes were transferred from standard growth condition to different environmental growth rooms, where the temperature was above (29°C) or below (19°C) the standard condition. Alternatively, the dishes were placed at different levels of illumination by moving either closer (higher light intensity of 84 μE m−2 s−1) or further away (lower light intensity of 22 μE m−2 s−1) from the light source while ensuring the temperature remained at the standard condition.
Harvesting Plant Materials for Metabolomics Analysis
On the 16th day after transfer to the growth-room, aerial portions of plants were harvested (growth stage 1.08–1.12 as defined by Boyes et al., 2001), and frozen immediately by immersion in liquid nitrogen, stored at −80°C, freeze dried for 48 h, powderized using 12–15 stainless steel balls for 2 min and aliquoted into tubes. For two of the targeted metabolomics analyses platforms, cuticular waxes and lipidomics, metabolites were immediately extracted from harvested tissue. Plates were harvested one at a time, and the entire harvest was conducted under the same illumination level as the growth conditions within a period of less than 45-s per dish.
To assess the effect of delayed harvesting following opening the dishes (i.e., lowering the humidity level), plant tissues were harvested from dishes that were grown in the standard growth regime and were harvested following a 45-s, 1 and 3-h delay after lids were removed; in this experiment (Harvest Delay in Table 2), tissues were quenched either by immersion in liquid nitrogen and processed or extracted immediately, as detailed above. All plant materials stored at −80°C were shipped via overnight carriers to the different analytical laboratories while packaged in dry-ice.
E. coli Complementation Assay
The AtlpxA (At4g29540) cDNA was synthesized by GeneScript USA Inc. (Piscataway, NJ, USA), codon-optimized for protein production in E. coli. N-terminal modification to remove the 32 codons that encode an organelle-targeting peptide was conducted via PCR. The amplified fragment was ligated into HindIII/EcoRI-digested pUC57 and transformed into both E. coli strain SM101 [which carries the lpxA-2(ts) allele] and SM105 (which is the progenitor wild-type for strain SM101), both obtained from E. coli Genetic Stock Center (New Haven, CT, USA). E. coli stains, SM105, SM101 + At4g29540, and SM101 + pUC57 were grown in LB medium containing 1 mM IPTG (to induce expression of the AtlpxA cDNA) at 30°C (the permissive temperature) and 42°C (the non-permissive temperature).
Metabolomics Analytical Platforms
Generally nine different analytical platforms were utilized to assess the metabolome of harvested tissues. Four platforms utilized non-targeted metabolomics analysis: GC–TOFMS, UPHLC–QTOFMS, CE–MS, and LC–MS. The other platforms targeted analysis to specific classes of metabolites. These focused on identification of glycerolipids, fatty acids, amino acids, ceramides, cuticular waxes, phytosterols and tocopherols, chlorophylls, and carotenoids. Details of the extraction protocols and analytical methods for each of these platforms are provided in the Supplementary Materials and are available on PlantMetabolomics.org.
Data Compilation and Dissemination
The project data are stored in a web-based analysis system4 that allows users to actively search, visualize, and download the data. Known metabolites are annotated with their chemical formula, SMILES notation (Weininger, 1988), molecular weight, and links to other databases. Links to chemical, pathway, and genomic information from KEGG, ARACYC, MetNetDB, and PubChem illuminate a metabolite’s role in a plant’s metabolic network. The database is based on the minimal information of a metabolomic experiment, MIAMet (Bino et al., 2004) standards to capture complete annotation of experiments and includes metadata for the experiments along with metabolite abundance data.
Statistical Analysis
In general, 6 separate batches of plant material (biological replicates) were sent to each analytical laboratory. Missing values caused by failed analyses were ignored in the statistical evaluation. Measurements below the detection limit were replaced by 1/2 of the estimated detection limit. The log-ratio, log2(mt/wt), was calculated for each metabolite; where mt and wt are the average metabolite abundances in the mutant and wild-type, respectively. The standard error (se) of the log-ratio was calculated using a delta-method approximation, se log-ratio = 1/ln 2 where semt and sewt are the standard errors of the average mutant and wild-type metabolite abundances.
Integrated analysis of data from all metabolomic platforms was based on averages over the biological replicates, the dissimilarity between a pair of mutants was computed using a variance-weighted Manhattan distance measure (Dixon et al., in preparation).
where Yki is the abundance of metabolite k in genotype I. The term
estimates the SD of the difference in abundance. One property of this distance measure, useful for the analysis of metabolomic data, is its invariance to multiplicative rescaling of a metabolite (Dixon et al., in preparation). That is, the contribution of metabolite k is the same no matter whether Yki is a peak area, a relative abundance, or an absolute concentration, so long as each quantity can be converted into another by multiplying by a constant, e.g., concentration = constant × abundance. Classical MDS was used to visualize the pairwise distance matrix of mutant pairs in two dimensions. The distances between points in the MDS plot are the best two-dimensional approximation to all pairs of distances in the distance matrix. Hierarchical clustering of genes based on the metabolomes of mutant strains was performed using the average linkage algorithm. The distance matrix computation, MDS, and hierarchical clustering were performed in R.
To determine significantly altered compounds in the Pilot Study mutants, metabolite data was analyzed in the following manner: Log-transformed concentrations were used to calculate Pearson’s correlation coefficient (r) between replicates. Any replicate whose correlation coefficient was less than 0.7 with at least half of all other replicates was removed from further analysis. Median values of the concentrations of all the detected metabolites in each replicate for each mutant line were averaged and the mean of the median values was used to scale the concentration levels of the compounds in the replicates. Student’s t-test was performed to identify significantly altered metabolites in each of the mutant lines compared to wild-type. To control the false discovery rate from multiple hypothesis testing, p-values from the t-tests were further adjusted by the Benamini and Hochberg (1995) algorithm. Adjusted p-value of less than 0.05 and fold change of either greater or less than 2 as the cutoff to define significantly altered metabolites.
Conflict of Interest Statement
The authors declare that the research was conducted in the absence of any commercial or financial relationships that could be construed as a potential conflict of interest.
Acknowledgments
This work was supported by the National Science Foundation (grants no. MCB 0520140 and 0820823). Additional support included: funding from the National Science Foundation Major Research Instrumentation grant no. DBI 0521587 (Ruth Welti); National Science Foundation Arabidopsis 2010 DBI0520267 (Eve S. Wurtele); The Samuel Roberts Noble Foundation for personnel support (Lloyd W. Sumner and David V. Huhman) and instrumentation purchase; Carnegie Institution for Science (Kun He, Seung Y. Rhee) and National Science Foundation grant DBI-0640769 (Seung Y. Rhee); Yun Lu for performing GC–TOFMS in the Fiehn laboratory; Agricultural Research Center at Washington State University (B. Markus Lange). The authors would also like to acknowledge the W. M. Keck Foundation for support at Iowa State University. We acknowledge the very kind support of all the collaborators listed at www.plantmetabolomics.org, who contributed Arabidopsis T-DNA tagged mutant seed stocks, in particular the late Dr. Christian R. H. Raetz (Duke University) for the seed stock carrying the lpxA-1 allele, and Dr. David J. Oliver (Iowa State University) for the seed stock carrying the oxp1 allele.
Supplementary Material
The Supplementary Material for this article can be found online at http://www.frontiersin.org/technical_advances_in_plant_science/10.3389/fpls.2012.00015/abstract
Footnotes
References
AGI. (2000). Analysis of the genome squence of the flowering plat Arabidopsis thaliana. Nature 408, 796–815.
Ajjawi, I., Lu, Y., Savage, L. J., Bell, S. M., and Last, R. L. (2010). Large-scale reverse genetics in Arabidopsis: case studies from the Chloroplast 2010 Project. Plant Physiol. 152, 529–540.
Alonso, J. M., Stepanova, A. N., Leisse, T. J., Kim, C. J., Chen, H., Shinn, P., Stevenson, D. K., Zimmerman, J., Barajas, P., Cheuk, R., Gadrinab, C., Heller, C., Jeske, A., Koesema, E., Meyers, C. C., Parker, H., Prednis, L., Ansari, Y., Choy, N., Deen, H., Geralt, M., Hazari, N., Hom, E., Karnes, M., Mulholland, C., Ndubaku, R., Schmidt, I., Guzman, P., Aguilar-Henonin, L., Schmid, M., Weigel, D., Carter, D. E., Marchand, T., Risseeuw, E., Brogden, D., Zeko, A., Crosby, W. L., Berry, C. C., and Ecker, J. R. (2003). Genome-wide insertional mutagenesis of Arabidopsis thaliana. Science 301, 653–657.
Armstrong, M. T., Theg, S. M., Braun, N., Wainwright, N., Pardy, R. L., and Armstrong, P. B. (2006). Histochemical evidence for lipid A (endotoxin) in eukaryote chloroplasts. FASEB J. 20, 2145–2146.
Bais, P., Moon, S. M., He, K., Leitao, R., Dreher, K., Walk, T., Sucaet, Y., Barkan, L., Wohlgemuth, G., Roth, M. R., Wurtele, E. S., Dixon, P., Fiehn, O., Lange, B. M., Shulaev, V., Sumner, L. W., Welti, R., Nikolau, B. J., Rhee, S. Y., and Dickerson, J. A. (2010). PlantMetabolomics.org: a web portal for plant metabolomics experiments. Plant Physiol. 152, 1807–1816.
Benamini, Y., and Hochberg, Y. (1995). Controlling the false discovery rate: a practical and powerful approach to multiple testing. J. R. Stat. Soc. Series B Stat. Methodol. 57, 289–300.
Berardini, T. Z., Mundodi, S., Reiser, L., Huala, E., Garcia-Hernandez, M., Zhang, P., Mueller, L. A., Yoon, J., Doyle, A., Lander, G., Moseyko, N., Yoo, D., Xu, I., Zoeckler, B., Montoya, M., Miller, N., Weems, D., and Rhee, S. Y. (2004). Functional annotation of the Arabidopsis genome using controlled vocabularies. Plant Physiol. 135, 745–755.
Bino, R. J., Hall, R. D., Fiehn, O., Kopka, J., Saito, K., Draper, J., Nikolau, B. J., Mendes, P., Roessner-Tunali, U., Beale, M. H., Trethewey, R. N., Lange, B. M., Wurtele, E. S., and Sumner, L. W. (2004). Potential of metabolomics as a functional genomics tool. Trends Plant Sci. 9, 418–425.
Boyes, D. C., Zayed, A. M., Ascenzi, R., Mccaskill, A. J., Hoffman, N. E., Davis, K. R., and Gorlach, J. (2001). Growth stage-based phenotypic analysis of Arabidopsis: a model for high throughput functional genomics in plants. Plant Cell 13, 1499–1510.
Broeckling, C. D., Huhman, D. V., Farag, M. A., Smith, J. T., May, G. D., Mendes, P., Dixon, R. A., and Sumner, L. W. (2005). Metabolic profiling of Medicago truncatula cell cultures reveals the effects of biotic and abiotic elicitors on metabolism. J. Exp. Bot. 56, 323–336.
Farag, M. A., Huhman, D. V., Dixon, R. A., and Sumner, L. W. (2008). Metabolomics reveals novel pathways and differential mechanistic and elicitor-specific responses in phenylpropanoid and isoflavonoid biosynthesis in Medicago truncatula cell cultures. Plant Physiol. 146, 387–402.
Fatland, B. L., Ke, J., Anderson, M. D., Mentzen, W. I., Cui, L. W., Allred, C. C., Johnston, J. L., Nikolau, B. J., and Wurtele, E. S. (2002). Molecular characterization of a heteromeric ATP-citrate lyase that generates cytosolic acetyl-coenzyme A in Arabidopsis. Plant Physiol. 130, 740–756.
Fatland, B. L., Nikolau, B. J., and Wurtele, E. S. (2005). Reverse genetic characterization of cytosolic acetyl-CoA generation by ATP-citrate lyase in Arabidopsis. Plant Cell 17, 182–203.
Fiehn, O., Kopka, J., Dormann, P., Altmann, T., Trethewey, R. N., and Willmitzer, L. (2000). Metabolite profiling for plant functional genomics. Nat. Biotechnol. 18, 1157–1161.
Galloway, S. M., and Raetz, C. R. (1990). A mutant of Escherichia coli defective in the first step of endotoxin biosynthesis. J. Biol. Chem. 265, 6394–6402.
Hall, R., Beale, M., Fiehn, O., Hardy, N., Sumner, L., and Bino, R. (2002). Plant metabolomics: the missing link in functional genomics strategies. Plant Cell 14, 1437–1440.
Hruz, T., Laule, O., Szabo, G., Wessendorp, F., Bleuler, S., Oertle, L., Widmayer, P., Gruissem, W., and Zimmermann, P. (2008). Genevestigator v3: a reference expression database for the meta-analysis of transcriptomes. Adv. Bioinformatics 2008, 420747.
Lee, I., Ambaru, B., Thakkar, P., Marcotte, E. M., and Rhee, S. Y. (2010). Rational association of genes with traits using a genome-scale gene network for Arabidopsis thaliana. Nat. Biotechnol. 28, 149–156.
Li, C., Guan, Z., Liu, D., and Raetz, C. R. (2011). Pathway for lipid A biosynthesis in Arabidopsis thaliana resembling that of Escherichia coli. Proc. Natl. Acad. Sci. U.S.A. 108, 11387–11392.
Liu, D., Sun, T., and Raetz, C. (2003). Arabidopsis thaliana genes encoding orthologs of enzymes involved in Escherichia coli lipid A biosynthesis. FASEB J. 17(Suppl.), A579.
Lu, Y., Savage, L. J., Ajjawi, I., Imre, K. M., Yoder, D. W., Benning, C., Dellapenna, D., Ohlrogge, J. B., Osteryoung, K. W., Weber, A. P., Wilkerson, C. G., and Last, R. L. (2008). New connections across pathways and cellular processes: industrialized mutant screening reveals novel associations between diverse phenotypes in Arabidopsis. Plant Physiol. 146, 1482–1500.
Lu, Y., Savage, L. J., Larson, M. D., Wilkerson, C. G., and Last, R. L. (2011). Chloroplast 2010: a database for large-scale phenotypic screening of Arabidopsis mutants. Plant Physiol. 155, 1589–1600.
Ludwig, A., Stolz, J., and Sauer, N. (2000). Plant sucrose-H+ symporters mediate the transport of vitamin H. Plant J. 24, 503–509.
MASC. (2010). The Multinational Coordinated Arabidopsis thaliana Functional Genomics Project: Annual Report 2010. Madison, WI: MASC Committee.
Mazelis, M., and Pratt, H. M. (1976). In vivo conversion of 5-oxoproline to glutamate by higher plants. Plant Physiol. 57, 85–87.
Mentzen, W. I., Peng, J., Ransom, N., Nikolau, B. J., and Wurtele, E. S. (2008). Articulation of three core metabolic processes in Arabidopsis: fatty acid biosynthesis, leucine catabolism and starch metabolism. BMC Plant Biol. 8, 76. doi:10.1186/1471-2229-8-76
Mentzen, W. I., and Wurtele, E. S. (2008). Regulon organization of Arabidopsis. BMC Plant Biol. 8, 99. doi:10.1186/1471-2229-8-99
Messerli, G., Partovi Nia, V., Trevisan, M., Kolbe, A., Schauer, N., Geigenberger, P., Chen, J., Davison, A. C., Fernie, A. R., and Zeeman, S. C. (2007). Rapid classification of phenotypic mutants of Arabidopsis via metabolite fingerprinting. Plant Physiol. 143, 1484–1492.
Nikiforova, V. J., Kopka, J., Tolstikov, V., Fiehn, O., Hopkins, L., Hawkesford, M. J., Hesse, H., and Hoefgen, R. (2005). Systems rebalancing of metabolism in response to sulfur deprivation, as revealed by metabolome analysis of Arabidopsis plants. Plant Physiol. 138, 304–318.
Ohkama-Ohtsu, N., Oikawa, A., Zhao, P., Xiang, C., Saito, K., and Oliver, D. J. (2008). A gamma-glutamyl transpeptidase-independent pathway of glutathione catabolism to glutamate via 5-oxoproline in Arabidopsis. Plant Physiol. 148, 1603–1613.
Raetz, C. R., Reynolds, C. M., Trent, M. S., and Bishop, R. E. (2007). Lipid A modification systems in gram-negative bacteria. Annu. Rev. Biochem. 76, 295–329.
Raetz, C. R., and Whitfield, C. (2002). Lipopolysaccharide endotoxins. Annu. Rev. Biochem. 71, 635–700.
Seber, G. A. F. (2008). “Frontmatter,” in Multivariate Observations (Hoboken, NJ: John Wiley & Sons, Inc.), i–xx.
Shinozaki, K., and Sakakibara, H. (2009). Omics and bioinformatics: an essential toolbox for systems analyses of plant functions beyond 2010. Plant Cell Physiol. 50, 1177–1180.
Smith, A. M., Zeeman, S. C., and Smith, S. M. (2005). Starch degradation. Annu. Rev. Plant Biol. 56, 73–98.
Spearman, C. (1987). The proof and measurement of association between two things. By C. Spearman, 1904. Am. J. Psychol. 100, 441–471.
Steinhauser, D., Usadel, B., Luedemann, A., Thimm, O., and Kopka, J. (2004). CSB.DB: a comprehensive systems-biology database. Bioinformatics 20, 3647–3651.
Sussman, M. R., Amasino, R. M., Young, J. C., Krysan, P. J., and Austin-Phillips, S. (2000). The Arabidopsis knockout facility at the University of Wisconsin-Madison. Plant Physiol. 124, 1465–1467.
Tohge, T., Yonekura-Sakakibara, K., Niida, R., Wantanabe-Takahashi, A., and Saito, K. (2007). Phytochemical genomics in Arabidopsis thaliana: a case study for functional identification of flavonoid biosynthesis genes*. Pure Appl. Chem. 79, 811–821.
Weininger, D. (1988). SMILES, a chemical language and information system. 1. introduction of methodology and encoding rules. J. Chem. Inf. Comput. Sci. 28, 31–36.
Wentzell, A. M., Rowe, H. C., Hansen, B. G., Ticconi, C., Halkier, B. A., and Kliebenstein, D. J. (2007). Linking metabolic QTLs with network and cis-eQTLs controlling biosynthetic pathways. PLoS Genet. 3, e162. doi:10.1371/journal.pgen.0030162
Winkel-Shirley, B. (2001). Flavonoid biosynthesis. A colorful model for genetics, biochemistry, cell biology, and biotechnology. Plant Physiol. 126, 485–493.
Winter, D., Vinegar, B., Nahal, H., Ammar, R., Wilson, G. V., and Provart, N. J. (2007). An “electronic fluorescent pictograph” browser for exploring and analyzing large-scale biological data sets. PLoS ONE 2, e718. doi:10.1371/journal.pone.0000718
Yonekura-Sakakibara, K., Tohge, T., Matsuda, F., Nakabayashi, R., Takayama, H., Niida, R., Watanabe-Takahashi, A., Inoue, E., and Saito, K. (2008). Comprehensive flavonol profiling and transcriptome coexpression analysis leading to decoding gene-metabolite correlations in Arabidopsis. Plant Cell 20, 2160–2176.
Keywords: Arabidopsis, metabolomics, gene annotation, functional genomics, database
Citation: Quanbeck SM, Brachova L, Campbell AA, Guan X, Perera A, He K, Rhee SY, Bais P, Dickerson JA, Dixon P, Wohlgemuth G, Fiehn O, Barkan L, Lange I, Lange BM, Lee I, Cortes D, Salazar C, Shuman J, Shulaev V, Huhman DV, Sumner LW, Roth MR, Welti R, Ilarslan H, Wurtele ES and Nikolau BJ (2012) Metabolomics as a hypothesis-generating functional genomics tool for the annotation of Arabidopsis thaliana genes of “unknown function”. Front. Plant Sci. 3:15. doi: 10.3389/fpls.2012.00015
Received: 18 October 2011;
Accepted: 17 January 2012;
Published online: 10 February 2012.
Edited by:
Roger Deal, Emory University, USAReviewed by:
Kazuki Saito, Chiba University, JapanAdrian Hegeman, University of Minnesota, USA
Alisdair Fernie, Max Planck Institut for Plant Physiology, Germany
Copyright: © 2012 Quanbeck, Brachova, Campbell, Guan, Perera, He, Rhee, Bais, Dickerson, Dixon, Wohlgemuth, Fiehn, Barkan, Lange, Lange, Lee, Cortes, Salazar, Shuman, Shulaev, Huhman, Sumner, Roth, Welti, Ilarslan, Wurtele and Nikolau. This is an open-access article distributed under the terms of the Creative Commons Attribution Non Commercial License, which permits non-commercial use, distribution, and reproduction in other forums, provided the original authors and source are credited.
*Correspondence: Basil J. Nikolau, Iowa State University, 3254 Molecular Biology Building, Ames, IA 50011, USA. e-mail:ZGltbWFzQGlhc3RhdGUuZWR1