Why do plants grow the way that they do? According to Aristotle, there are four kinds of causes, or four fundamentally different ways of answering “why” questions such as this (Aristotle, 1984; Falcon, 2012). In reductionist science, answers to “why” questions typically relate to one of the first three of Aristotle's causes, regarding changes in substances (material cause), in form (formal cause) and in the effects of external influences (efficient cause). This is reflected in much functional-structural pant modeling (FSPM), where “structural” aspects of plant architecture are clearly concerned with formal causes and internal “functional” aspects, such as hormones and transported nutrient are clearly concerned with material causes (Sievänen et al., 2000; Prusinkiewicz, 2004; Yan et al., 2004; Godin and Sinoquet, 2005; Fourcaud et al., 2008; Hanan and Prusinkiewicz, 2008; Vos et al., 2010). The environmental aspects, such as light, soil water and nutrients, pests and pathogens that are also often included in such FSPM and interact with both function and structure are clearly concerned with efficient causes. However, Aristotle's fourth kind of cause, final cause, seems to be less considered in reductionist science in general, and in FSPM in particular.
Final causes concern the aim or purpose being served by the object of interest, a plant in our case. In other words, discussion of final causes concerns answering the question of why a plant grows the way it does by reference to the purpose of that growth. Such answers could take the form of “The plant is growing like that because it is trying to maximize its light interception,” for example. In science, such a response may lead to accusations of anthropomorphism, which can be defined as the attribution of human qualities to things other than humans, with a connotation that such attribution is erroneous and problematic (Horowitz, 2007). If Pavlov (1927) wrote that animals should be “studied as purely physiological facts, without any need to resort to fantastic speculations as to the existence of any possible subjective state in the animal which may be conjectured on analogy with ourselves,” then it would seem an even greater sin to explain the behavior of plants as “purposeful,” or in terms of what they are trying to achieve with that behavior? However, evolutionary theory provides a clear rationale for the value of explanations of behavior in terms of the purpose of that behavior, as long as it can be seen as having an evolutionary advantage, and thus having been selected for by evolutionary processes. So we can rephrase our “final cause” response more carefully, “The plant is growing like that because that is an ecological strategy that has evolved over time due to the fact that it tends to maximize the plant's light interception.” But how can we know whether a growth strategy has indeed evolved over time to maximize light interception (or any other function that contributes to evolutionary success)?
The dynamic structural development of a plant can be seen as a strategy for exploiting the limited resources available within its environment, such as light, soil water and nutrients, and we would expect that evolution would lead to efficient growth strategies that reduce resource costs while maximizing resource acquisition. No one growth strategy will be optimal in all environments; which strategies of structural development are most effective will depend on how the resources on which the plant depends are distributed through both time and space. The relative advantage of a plant's growth strategies will also depend on how its architecture influences factors such as dispersal of seeds and pollen, the impacts of herbivoury and drought stress, the efficiency of water transport, biomechanical support, and resistance to wind, along with how much it costs to produce and maintain the structures that comprise its architecture (Küppers, 1989; Gartner, 1995). Therefore, if we are to shed light on Aristotle's final cause and start to understand why plants have evolved different strategies of structural development, we need to understand the various costs and benefits of different growth strategies in different environments (Farnsworth and Niklas, 1995; Lynch, 1995).
There is a long history of modeling plants in order to investigate the costs and benefits of different structural growth strategies (e.g., Shinozaki et al., 1964; Honda and Fisher, 1979; Johnson and Thornley, 1987; Niklas, 1999; West et al., 1999; Takenaka et al., 2001; Falster and Westoby, 2003; King et al., 2003). However, many potentially important aspects of plant growth and function have not been represented in these models, largely due to computational constraints and limitations in modeling technology. As simplifications of reality, no model can possibly include all aspects of reality. Nonetheless, recent years have seen the development of a new generation of plant models that include more of these previously neglected aspects, such as the explicit topology and spatial geometry of the plant structure; the way that the plant architecture develops dynamically over time by changes in existing components and the addition of new ones; the feedbacks between plant structure, function, and environment that also change with time as the plant grows and the environment changes; the way that the distribution of resources within a plant's environment varies with time and space; and competition between individuals within plant populations and communities. It is this “new generation” of models that are often known as functional-structural plant models (FSPMs) or “virtual plants” (Sievänen et al., 2000; Prusinkiewicz, 2004; Yan et al., 2004; Godin and Sinoquet, 2005; Fourcaud et al., 2008; Hanan and Prusinkiewicz, 2008; Vos et al., 2010).
The fact that FSPMs represent a large number of potentially-important interacting processes in a dynamic way and at a high degree of detail would seem to make them a perfect tool for investigating the costs and benefits of different structural growth strategies, and thus providing insight into the final cause of plant growth strategies. Indeed many models that could be termed FSPMs have been employed to investigate the relative advantages of varying below- and above-ground structural growth strategies (e.g., Pearcy and Yang, 1996; Colasanti and Hunt, 1997; Dunbabin et al., 2003; Pearcy et al., 2005; Sterck et al., 2005; Clark and Bullock, 2007; Pagès, 2011). However, the strength of FSPMs, their dynamic realism, is also their weakness, because it makes them relatively complex and computationally demanding. It can take a relatively long time to run even a single FSPM growth simulation, and an FSPM typically contains a large number of growth-strategy-defining parameters, meaning that to run simulations for all combinations of all values of all parameters of interest becomes a major computational challenge. One approach is to use a relatively complex and realistic FSPM but only attempt to evaluate a limited subset of all possible strategies (e.g., Dunbabin et al., 2003; Pagès, 2011), and the other is to use a simpler FSPM but explore a more comprehensive set of strategies (e.g., Niklas, 1994, 1999). However, probably neither of these would really satisfy Aristotle in his search for a final cause of real plant growth; for that we need a thorough and comprehensive search through a wide range of growth strategies with a model that is flexible and detailed enough to capture the most important aspects of real plant growth.
A promising option for moving forward is to employ evolutionary optimization algorithms (Fogel, 1994; Ashlock, 2006). Such algorithms provide a computationally efficient means of exploring a wide range of possibilities in search of optimal solutions. In addition, marrying evolutionary algorithms with FSPMs would also appear to be a perfect way to explore the optimality of plant structures and growth strategies from an evolutionary perspective, in order to deepen our understanding of the relationships between evolution, ecosystems, individual plants, and genes (Prusinkiewicz, 2000). Earlier use of evolutionary algorithms with models of plant structure were aimed at evolving better above-ground plant forms based on aesthetic criteria (McCormack, 1993; Jacob, 1994; Traxler and Gervautz, 1996; McCormack, 2004); these representations of plant structure were relatively simple and abstract and contained little realistic representation of biological processes. In more recent times, more biologically-motivated questions of ecological theory and above-ground plant competition at the level of individual plants and plant populations have been tackled with a combination of structural plant models and evolutionary computation, but still at a relatively abstract level (Bornhofen and Lattaud, 2006, 2007, 2009; Kennedy, 2010; Bornhofen et al., 2011). These examples only highlight the huge potential for using sophisticated evolutionary computation with more detailed and realistic FSPMs. While the potential focus of such FSPMs is almost limitless (above-ground, below-ground, herbs, shrubs, trees…), the way that an evolutionary algorithm can be combined with a FSPM to investigate the final cause of plant growth can be explained in quite general terms (Figure 1).
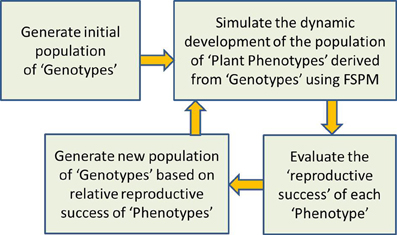
Figure 1. General schema of how an evolutionary algorithm can be combined with a FSPM to investigate the final cause or “evolutionary purpose” of plant growth. The FSPM to be used would have a number of parameters that define its growth strategy, and it is assumed that these parameters represent genetic information that can change with evolution. First an initial “population” of “genotypes” is generated, with each “genotype” consisting of a different set of values for all growth strategy parameters. In step two, the “phenotypic” realization of each “genotype” is simulated with runs of the FSPM, each one corresponding to a set of growth strategy parameters. In step three, the relative reproductive success of each phenotype is determined; this could be based on the final size of the plant for example, with larger plants assumed to produce more seed and pollen and thus be more likely to contribute genes to following generations, all else being equal. In step four, these measures of relative reproductive success are used to generate a new population of genotypes; for example, the genotype of each new seed would be based on the genotype of one or two randomly selected “parent phenotypes,” with the chance of a simulated plant being chosen as a parent depending on its size. Step two is now applied to the new population of genotypes, resulting in a new population of phenotypes, and so the process continues until a specified number of generations have elapsed, or until some other criterion indicating sufficient evolution is satisfied.
In a recent study illustrating the potential of this approach, we explored below-ground plant structural optimality by linking an evolutionary optimization algorithm with a dynamic root growth FSPM (Renton et al., 2012; Renton and Poot, 2013, unpublished) in a Tool for Analysis of Root Structures Incorporating Evolution of Rooting Strategies (TARSIERS). This study extended on previous studies by including a relatively detailed representation of root structure and spatial and temporal variations in resource distributions, applied to a realistic case study situation—perennial plants growing on shallow soils in seasonally dry environments. The approach was able to simulate reasonable patterns of evolution of structural growth strategies that converged toward the specialized root system morphologies that have been observed in species restricted to these types of habitats, and which are likely to enhance access to water resources in cracks in the underlying rock (Poot and Lambers, 2003a,b, 2008; Poot et al., 2008, 2012). The study showed how adding an evolutionary perspective to FSPMs could provide insights into both evolutionary processes and the ecological costs and benefits of different plant growth strategies.
As computing technology and modeling methodologies continue to advance, the computational difficulties of applying comprehensive and realistic evolutionary algorithms to detailed and realistic models of plant structure and function will continue to be overcome. While the realm of possibilities will keep expanding, the challenge will continue to be to design plant models that are simple enough for evolutionary optimization to be computationally feasible, yet flexible enough to allow a range of structural development strategies to be explored and realistic enough to capture the essential characteristics of interest. Within current FSPMs, the representation of the interactions between functional processes and structural development can be relatively simple and empirical (Renton et al., 2005a,b, 2007) or more mechanistic, realistic, detailed, and thus complex (Allen et al., 2005; Costes et al., 2008; Lopez et al., 2008); it is likely that relatively simple approaches will be of most use for integration into evolutionary simulations in the foreseeable future, although the use of “super-computing” facilities could potentially allow evolutionary optimization to be applied to even very complex and detailed FSPMs. The approaches developed will give insights into both evolutionary processes and the ecological costs and benefits of different plant growth strategies. The strategies considered could include both fixed strategies, which do not depend on the environment encountered by an individual plant, and plastic strategies, that do adapt to the encountered environment. By showing how plant architectural strategies have evolved to meet the requirements of certain specific environments, they will also help understand and predict how these strategies are likely to function or adapt as environments change in the future. If, as Dobzhansky (1973) wrote, “nothing in biology makes sense except in the light of evolution,” then it is essential to add an evolutionary perspective to FSPM, which addresses Aristotle's fourth and final cause in addition to his first three causes addressed by the structural, functional, and environmental perspectives already commonly used in FSPM. This will help to provide a more complete answer to the question of why plants grow the way they do.
Acknowledgments
The author acknowledges the contribution of Pieter Poot in their joint research on simulating the evolution of rooting strategies in extreme environments, which has informed this current article.
References
Allen, M. T., Prusinkiewicz, P., and DeJong, T. M. (2005). Using L-systems for modeling source-sink interactions, architecture and physiology of growing trees: the L-PEACH model. New Phytol. 166, 869–880. doi: 10.1111/j.1469-8137.2005.01348.x
Aristotle. (1984). Complete Works of Aristotle, Vol. 1. The Revised Oxford Translation. Princeton, NJ: Princeton University Press.
Ashlock, D. (2006). Evolutionary Computation for Modeling and Optimization. New York, NY: Springer-Verlag.
Bornhofen, S., and Lattaud, C. (2006). Life history evolution of virtual plants: trading off between growth and reproduction. Lect. Notes Comput. Sci. 4193, 808–817. doi: 10.1007/11844297_82
Bornhofen, S., and Lattaud, C. (2007). “Evolution of virtual plants interacting with their environment,” in Proceedings of the 9th International Conference on Virtual Reality (VRIC'07), (Laval), 172–176.
Bornhofen, S., and Lattaud, C. (2009). Competition and evolution in virtual plant communities: a new modeling approach. Nat. Comput. 8, 349–438. doi: 10.1007/s11047-008-9089-5
Bornhofen, S., Barot, S., and Lattaud, C. (2011). The evolution of CSR life-history strategies in a plant model with explicit physiology and architecture. Ecol. Model. 222, 1–10. doi: 10.1016/j.ecolmodel.2010.09.014
Clark, B., and Bullock, S. (2007). Shedding light on plant competition: modelling the influence of plant morphology on light capture (and vice versa). J. Theor. Biol. 244, 208–217. doi: 10.1016/j.jtbi.2006.07.032
Colasanti, R. L., and Hunt, R. (1997). Resource dynamics and plant growth: a self-assembling model for individuals, populations and communities. Funct. Ecol. 11, 133–145. doi: 10.1046/j.1365-2435.1997.00088.x
Costes, E., Smith, C., Renton, M., Guédon, Y., Prusinkiewicz, P., and Godin, C. (2008). MAppleT: simulation of apple tree development using mixed stochastic and biomechanical models. Funct. Plant Biol. 35, 936–950. doi: 10.1071/FP08081
Dobzhansky, T. (1973). Nothing in biology makes sense except in the light of evolution. Am. Biol. Teach. 35, 125–129. doi: 10.2307/4444260
Dunbabin, V., Diggle, A., and Rengel, Z. (2003). Is there an optimal root architecture for nitrate capture in leaching environments? Plant. Cell. Environ. 26, 835–844. doi: 10.1046/j.1365-3040.2003.01015.x
Falcon, A. (2012). “Aristotle on Causality,” in The Stanford Encyclopedia of Philosophy (Winter 2012 Edn.), ed E. N. Zalta. Available online at: http://plato.stanford.edu/archives/win2012/entries/aristotle-causality/. (Accessed June 26, 2013).
Falster, D. S., and Westoby, M. (2003). Plant height and evolutionary games. Trends Ecol. Evol. 18, 337–343. doi: 10.1016/S0169-5347(03)00061-2
Farnsworth, K. D., and Niklas, K. J. (1995). Theories of optimization, form and function in branching architecture in plants. Funct. Ecol. 9, 355. doi: 10.2307/2389997
Fogel, D. B. (1994). An introduction to simulated evolutionary optimization. IEEE Trans. Neural Netw. 5, 3–14. doi: 10.1109/72.265956
Fourcaud, T., Zhang, X., Stokes, A., Lambers, H., and Korner, C. (2008). Plant growth modelling and applications: the increasing importance of plant architecture in growth models. Ann. Bot. 101, 1053–1063. doi: 10.1093/aob/mcn050
Gartner, B. L. (ed.). (1995). Plant Stems: Physiology and Functional Morphology. London: Academic Press.
Godin, C., and Sinoquet, H. (2005). Functional-structural plant modelling. New Phytol. 166, 705–708. doi: 10.1111/j.1469-8137.2005.01445.x
Hanan, J., and Prusinkiewicz, P. (2008). Foreword: studying plants with functional-structural models. Funct. Plant Biol. 35, i–iii. doi: 10.1071/FPv35n10_FO
Honda, H., and Fisher, J. B. (1979). Ratio of tree branch lengths: the equitable distribution of leaf clusters on branches. PNAS 76, 3875–3879. doi: 10.1073/pnas.76.8.3875
Horowitz, A. (2007). “Anthropomorphism,” in Encyclopedia of Human-Animal Relationships: A Global Exploration of Our Connections With Animals, ed M. Bekoff (Westport, CT: Greenwood Publishing Group Incorporated), 60–66.
Jacob, C. (1994). “Genetic L-System programming,” in Parallel Problem Solving from Nature – PPSN III Lecture Notes in Computer Science, eds Y. Davidor, H.-P. Schwefel, and R. Männer (Springer: Berlin; Heidelberg), 333–343. Available online at: http://link.springer.com/chapter/10.1007/3-540-58484-6_277. (Accessed January 29, 2013).
Johnson, I. R., and Thornley, J. H. M. (1987). A model of shoot: root partitioning with optimal growth. Ann. Bot. 60, 133–142.
Kennedy, M. C. (2010). Functional-structural models optimize the placement of foliage units for multiple whole-canopy functions. Ecol. Res. 25, 723–732. doi: 10.1007/s11284-009-0658-6
King, J., Gay, A., Sylvester-Bradley, R., Bingham, I., Foulkes, J., Gregory, P., et al. (2003). Modelling cereal root systems for water and nitrogen capture: towards an economic optimum. Ann. Bot. 91, 383–390. doi: 10.1093/aob/mcg033
Küppers, M. (1989). Ecological significance of above-ground architectural patterns in woody plants: a question of cost-benefit relationships. Trends Ecol. Evol. 4, 375–379. doi: 10.1016/0169-5347(89)90103-1
Lopez, G., Favreau, R. R., Smith, C., Costes, E., Prusinkiewicz, P., and DeJong, T. M. (2008). Integrating simulation of architectural development and source-sink behaviour of peach trees by incorporating Markov chains and physiological organ function submodels into L-PEACH. Funct. Plant Biol. 35, 761–771. doi: 10.1071/FP08039
McCormack, J. (2004). “Aesthetic evolution of L-Systems revisited,” in Applications of Evolutionary Computing Lecture Notes in Computer Science, eds G. R. Raidl, S. Cagnoni, J. Branke, D. W. Corne, R. Drechsler, Y. Jin et al., (Springer: Berlin; Heidelberg), 477–488. Available online at: http://link.springer.com/chapter/10.1007/978-3-540-24653-4_49. (Accessed January 24, 2013).
McCormack, J. (1993). “Interactive evolution of L-system grammars for computer graphics modelling,” in Complex Systems: From Biology to Computation, eds D. G. Green, and T. R. J. Bossomaier (Amsterdam: IOS Press), 118–130.
Niklas, K. J. (1994). Morphological evolution through complex domains of fitness. Proc. Natl. Acad. Sci. U.S.A. 91, 6772–6779. doi: 10.1073/pnas.91.15.6772
Pagès, L. (2011). Links between root developmental traits and foraging performance. Plant Cell Environ. 34, 1749–1760. doi: 10.1111/j.1365-3040.2011.02371.x
Pavlov, I. P. (1927). “Lecture II,” in Conditioned Reflexes: An Investigation of the Physiological Activity of the Cerebral Cortex (Courier Dover Publications). Available online at: http://psychclassics.yorku.ca/Pavlov/lecture2.htm
Pearcy, R. W., Muraoka, H., and Valladares, F. (2005). Crown architecture in sun and shade environments: assessing function and trade-offs with a three-dimensional simulation model. New Phytol. 166, 791–800. doi: 10.1111/j.1469-8137.2005.01328.x
Pearcy, R. W., and Yang, W. (1996). A three-dimensional crown architecture model for assessment of light capture and carbon gain by understory plants. Oecologia 108, 1–12. doi: 10.1007/BF00333208
Poot, P., Bakker, R., and Lambers, H. (2008). Adaptations to winter-wet ironstone soils: a comparison between rare ironstone Hakea (Proteaceae) species and their common congeners. Aust. J. Bot. 56, 574–582. doi: 10.1071/BT08155
Poot, P., Hopper, S. D., and van Diggelen, J. M. H. (2012). Exploring rock fissures: does a specialized root morphology explain endemism on granite outcrops? Ann. Bot. 110, 291–300. doi: 10.1093/aob/mcr322
Poot, P., and Lambers, H. (2003a). Are trade-offs in allocation pattern and root morphology related to species abundance? A congeneric comparison between rare and common species in the south-western Australian flora. J. Ecol. 91, 58–67. doi: 10.1046/j.1365-2745.2003.00738.x
Poot, P., and Lambers, H. (2003b). Growth responses to waterlogging and drainage of woody Hakea (Proteaceae) seedlings, originating from contrasting habitats in south-western Australia. Plant Soil 253, 57–70. doi: 10.1023/A:1024540621942
Poot, P., and Lambers, H. (2008). Shallow-soil endemics: adaptive advantages and constraints of a specialized root-system morphology. New Phytol. 178, 371–381. doi: 10.1111/j.1469-8137.2007.02370.x
Prusinkiewicz, P. (2000). Simulation modeling of plants and plant ecosystems. Commun. ACM 43, 84–93. doi: 10.1145/341852.341867
Prusinkiewicz, P. (2004). Modeling plant growth and development. Curr. Opin. Plant Biol. 7, 79–83. doi: 10.1016/j.pbi.2003.11.007
Renton, M., Hanan, J., and Burrage, K. (2005a). Using the canonical modelling approach to simplify the simulation of function in functional-structural plant models. New Phytol. 166, 845–857. doi: 10.1111/j.1469-8137.2005.01330.x
Renton, M., Kaitaniemi, P., and Hanan, J. (2005b). Functional-structural plant modelling using a combination of architectural analysis, L-systems and a canonical model of function. Ecol. Model. 184, 277–298. doi: 10.1016/j.ecolmodel.2004.10.008
Renton, M., and Poot, P. (2013). “Simulating the evolution of optimal rooting strategies in shallow soils and extreme climates,” in Proceedings of the 7th International Conference on Functional-Structural Plant Models, eds R. Sievänen, E. Nikinmaa, C. Godin, A. Lintunen, and P. Nygren (Saariselkä; Vantaa: Finnish Society of Forest Science). Available online at: http://www.metla.fi/fspm2013/proceedings
Renton, M., Poot, P., and Evers, J. B. (2012). “Simulation of optimal rooting strategies: what's the best way to find a wet crack?” in Proceedings of the Fourth International Symposium on Plant Growth Modeling and Applications (PMA12) (Shanghai: IEEE Computer Society).
Renton, M., Thornby, D., and Hanan, J. (2007). “Canonical modelling: An approach for intermediate-level simulation of carbon allocation in functional-structural plant models,” in Functional-Structural Plant Modelling in Crop Production, eds J. Vos, L. Marcelis, P. Visser, and J. Struik (Wageningen University, The Netherlands: Frontis/Springer), 151–164.
Shinozaki, K., Yoda, K., Hozumi, K., and Kira, T. (1964). A quantitative analysis of plant form-the pipe model theory: I. basic analyses. Japan. J. Ecol. 14, 97–105.
Sievänen, R., Nikinmaa, E., Nygren, P., Ozier-Lafontaine, H., Perttunen, J., and Hakula, H. (2000). Components of functional-structural tree models. Ann. For. Sci. 57, 399–412. doi: 10.1051/forest:2000131
Sterck, F. J., Schieving, F., Lemmens, A., and Pons, T. L. (2005). Performance of trees in forest canopies: explorations with a bottom-up functional-structural plant growth model. New Phytol. 166, 827–843. doi: 10.1111/j.1469-8137.2005.01342.x
Takenaka, A., Takahashi, K., and Kohyama, T. (2001). Optimal leaf display and biomass partitioning for efficient light capture in an understorey palm, Licuala arbuscula. Func. Ecol. 15, 660–668. doi: 10.1046/j.0269-8463.2001.00562.x
Traxler, C., and Gervautz, M. (1996). “Using genetic algorithms to improve the visual quality of fractal plants generated with csg-pl-systems,” in Proceedings of the Fourth International Conference in Central Europe on Computer Graphics and Virtual Worlds, 367–376. Available online at: http://www.cg.tuwien.ac.at/research/rendering/csg-graphs/Papers/GeneticAlgorithms.pdf. (Accessed January 24, 2013).
Vos, J., Evers, J. B., Buck-Sorlin, G. H., Andrieu, B., Chelle, M., and de Visser, P. H. B. (2010). Functional-structural plant modelling: a new versatile tool in crop science. J. Exp. Bot. 61, 2101–2115. doi: 10.1093/jxb/erp345
West, G. B., Brown, J. H., and Enquist, B. J. (1999). A general model for the structure and allometry of plant vascular systems. Nature 400, 664–667. doi: 10.1038/23251
Citation: Renton M (2013) Aristotle and adding an evolutionary perspective to models of plant architecture in changing environments. Front. Plant Sci. 4:284. doi: 10.3389/fpls.2013.00284
Received: 31 May 2013; Accepted: 10 July 2013;
Published online: 31 July 2013.
Edited by:
Katrin Kahlen, Research Center Geisenheim, GermanyReviewed by:
Jochem B. Evers, Wageningen University and Research Centre, NetherlandsCopyright © 2013 Renton. This is an open-access article distributed under the terms of the Creative Commons Attribution License (CC BY). The use, distribution or reproduction in other forums is permitted, provided the original author(s) or licensor are credited and that the original publication in this journal is cited, in accordance with accepted academic practice. No use, distribution or reproduction is permitted which does not comply with these terms.
*Correspondence:bWljaGFlbC5yZW50b25AdXdhLmVkdS5hdQ==