- 1Department of Forest Biomaterials, North Carolina State University, Raleigh, NC, USA
- 2National Renewable Energy Laboratory, Biosciences Center, Golden, CO, USA
Optimizing the use of lignocellulosic biomass as the feedstock for renewable energy production is currently being developed globally. Biomass is a complex mixture of cellulose, hemicelluloses, lignins, extractives, and proteins; as well as inorganic salts. Cell wall compositional analysis for biomass characterization is laborious and time consuming. In order to characterize biomass fast and efficiently, several high through-put technologies have been successfully developed. Among them, near infrared spectroscopy (NIR) and pyrolysis-molecular beam mass spectrometry (Py-mbms) are complementary tools and capable of evaluating a large number of raw or modified biomass in a short period of time. NIR shows vibrations associated with specific chemical structures whereas Py-mbms depicts the full range of fragments from the decomposition of biomass. Both NIR vibrations and Py-mbms peaks are assigned to possible chemical functional groups and molecular structures. They provide complementary information of chemical insight of biomaterials. However, it is challenging to interpret the informative results because of the large amount of overlapping bands or decomposition fragments contained in the spectra. In order to improve the efficiency of data analysis, multivariate analysis tools have been adapted to define the significant correlations among data variables, so that the large number of bands/peaks could be replaced by a small number of reconstructed variables representing original variation. Reconstructed data variables are used for sample comparison (principal component analysis) and for building regression models (partial least square regression) between biomass chemical structures and properties of interests. In this review, the important biomass chemical structures measured by NIR and Py-mbms are summarized. The advantages and disadvantages of conventional data analysis methods and multivariate data analysis methods are introduced, compared and evaluated. This review aims to serve as a guide for choosing the most effective data analysis methods for NIR and Py-mbms characterization of biomass.
Introduction for Biomass Chemical Composition
Biomass is a complicated mixture of organic and inorganic compounds. It is mainly composed of cellulose, hemicelluloses and lignins, as well as minor components, such as proteins, extractives, ash, and other nonstructural mineral materials. Because of its renewable nature and chemical composition, biomass is an attractive feedstock for energy and chemical products (Ragauskas et al., 2006; Himmel et al., 2007; Wei et al., 2009; Sluiter et al., 2010). In order to provide an effective guide for feedstock selection and process development, it is very important to measure biomass chemical composition accurately and efficiently (Sluiter et al., 2010; Templeton et al., 2010; Daystar et al., 2013). In this paper, we will review the use of two high-throughput techniques, near infrared spectroscopy (NIR) and pyrolysis-molecular beam mass spectrometry (Py-mbms) in biomass characterization. The advantages and disadvantages of different data analysis methods, including band/peak assignment, tools for spectral treatments and resolution enhancement and multivariate data analysis methods, are introduced, compared and evaluated. Selected research publications are reviewed and categorized as “case studies” according to the ways they analyzed data and the specific biomass properties that are evaluated.
Conventional Biomass Characterization Relevant to Biofuel Production
Traditional biomass compositional analysis, based on two-stage sulfuric acid hydrolysis followed by gravimetric and instrumental analysis, has been used to measure lignin and carbohydrates for more than 100 years. These methods have been used by researchers for studies of wood materials, animal food, human health, bioenergy production, and many other areas related to biomaterials. The history and uses of these methods were reviewed in detail elsewhere (Sluiter et al., 2010). The analytical uncertainty for different methods was also evaluated by statistical analysis and reported as the standard deviation of measurement for each component (Templeton et al., 2010). Other wet chemical techniques also include: acidolysis, thioacidolysis, nitrobenzene oxidation, transesterification, acetyl bromide method, orcinol method, Van Soest method, etc. Routine procedures, a number of less common methods, and new analytical methods developed for research purposes in the field of wood chemistry are described in books (Browning, 1967; Sjöström and Alén, 1999). These techniques quantify important chemical structure biomass, but they are time consuming and laborious.
Separately, combustion-related properties are of interest for the utilization of biomass in biofuel and biopower production. There are three types of combustion-related properties: morphological, physical, and chemical properties (Braadbaart and Poole, 2008). Traditional fuel analysis of biomass includes ultimate analysis, proximate analysis, and thermogravimetric analysis. In addition, ash composition and sulfur can be determined and used to predict fuel indices, especially for slagging behavior, aerosol formation, and corrosion related risks (Obernberger, 2014).
Use of Spectroscopic Tools in Biomass Characterization as High Throughput Techniques
Spectroscopic methods, such as Fourier transform infrared spectroscopy (FTIR), NIR, Raman spectroscopy (Raman), and nuclear magnetic resonance (NMR), are widely used to measure functional groups and chemical bonds in biomass. These measurements are faster and more convenient than most conventional chemical methods used for biomass characterization and fuel analysis. Besides, since there is no degradative chemical treatment used during analysis, the information gained from these tools is more representative of the chemical structures in original biomass. However, there are some drawbacks for using these spectroscopic tools. For example, data interpretation for FTIR, Raman, and NMR is relatively complicated, sample preparation can be complex, and due to the mixed nature of biomass, peak assignment usually suffers from the overlap of many compounds. A good summary of spectroscopic tools used as high throughput techniques in biomass study can be found in a recent review (Lupoi et al., 2014).
High Throughput Techniques Coupled with Multivariate Statistical Analysis
Because of many chemical features included in a single spectrum, it is challenging to elucidate data directly for a group of samples. Therefore, multivariate analysis (MVA) tools have been widely used in spectroscopic data analysis (Jin and Xu, 2011; Smith-Moritz et al., 2011; Xu et al., 2013; Lupoi et al., 2014). Among them, the two multivariate tools that have been widely used are: (1) Principal component analysis (PCA), and (2) Partial least square (PLS).
Principal component analysis is mainly used for identifying outliers, sample comparison, and screening. It relies on projecting original samples variables on several (usually <six) reconstructed variables which are representative of original sample variation. Those reconstructed variables are known as principal components (PCs). Samples described with PCs can be plotted in scores plot, in which similar samples cluster together while samples different from each other are separated in two-, three-, or n-dimensional coordinates. Together with the scores plot, PCA loadings plot allows for the determination of important chemical features responsible for the sample grouping. In the loadings plot, variables with large values are highly correlated with sample grouping (Sykes et al., 2009).
Partial Least Square is used to build prediction correlation models between spectral data and the property of interest. In the application of NIR and Py-mbms, spectral data is regarded as “predictors” for the biomass properties of interest. The properties of a new sample can then be estimated using a PLS model built from spectral data taken on a set of similar samples with known characteristics. In this way, time consuming experiments for new samples could be eliminated. Regression coefficients are generated and can be used to relate chemical features in the spectra to the specific sample properties (Labbe et al., 2006).
In summary, multivariate tools used in spectroscopic data analysis have three functions: (1) comparing sample similarities and differences and discovering outliers; (2) building prediction models between spectroscopic data and biomass properties of interest; and (3) discovering correlations between property data and spectral data.
Biomass Characterization by NIR Spectroscopy
Near infrared spectroscopy is normally considered to be in the range of electromagnetic spectrum from 12,000 to 4000 cm-1 (Smith-Moritz et al., 2011). This wavelength region has two major advantages: first, the speed of spectral acquisition is high, which facilitates the real-time data collection for process control; secondly, the wide applicability to a diverse ranges of materials with little or no sample preparation (Schwanninger et al., 2011). This allows NIR to be effective for online monitoring and quality control of a wide variety of product properties and manufacturing processes (Workman, 2001; Kelley et al., 2004a; Tsuchikawa, 2007; Jin and Xu, 2011). Because of this, NIR has been extensively used as a high-throughput method to determine chemical, physical, mechanical, and fuel properties of woody biomass during the past 20 years.
However, there are some disadvantages to NIR. Although NIR absorption spectra have similar patterns to those in the mid-IR, they have wider separation, more anti-symmetry, and weaker intensity due to the fact that it is the combination and overtone bands from fundamental vibrations involved in NIR region. Therefore, the interpretation of NIR spectra are much harder than mid-IR (Schwanninger et al., 2011; Lupoi et al., 2014).
The utility of band assignments depends on the purpose of specific research or application. There is ongoing discussion around the necessity of interpreting NIR spectra in detail. Chemical/physical information contained in the NIR spectra can be used for detailed analysis (Schwanninger et al., 2011). However, it is not necessary to fully understand the chemical details for NIR to be useful for quantitative analysis. If NIR is used as a fast tool in distinguishing samples and in building prediction models for biomass properties, the detailed assignments are generally not needed. Statistical analysis for extracting useful information is essential for this purpose (Xu et al., 2013). Meaningful scientific insight of structural information could be better gained with the help of both statistical analysis and band assignments.
NIR Band Assignment and Data Processing
In NIR analysis, data points are usually collected in reflectance form (R) and converted to log10(1/R) form, which is equivalent to an absorbance spectrum.
As stated above, knowledge regarding band assignment is important for the understanding of chemical structures in biomass and there are several references on NIR band assignments (Tsuchikawa et al., 2003; Schwanninger et al., 2011; Via et al., 2013). Commonly assigned vibrations in the NIR spectra of woody biomass include (Schwanninger et al., 2011):
(1) 1370–1471 nm: First and second overtones of O–H stretching vibrations from free or weakly bonded O–H in carbohydrates and first overtones of C–H, Caromatic–H stretching vibrations, such as first overtone of O–H stretching in free OH group or OH group with a weak H-bond from cellulose, xylan, and glucomannan (1386, 1414, 1428, 1471, 1477–1484), first overtone of O–H stretching in phenolic hydroxyl groups from extractive or lignin (1410, 1447, 1448), first overtone of C–H stretching and bending in aromatic associated C–H from lignin (1417, 1440).
(2) 1471–1632 nm: First overtone of O–H stretching from strong O–H bonded group, semi-crystalline and crystalline region of cellulose (1473–1632) or intramolecular H-bond in glucomannan (1471, 1493).
(3) 1666–2000 nm: First overtone of aliphatic and aromatic C–H stretching vibrations and O–H combination bands from extractives/lignin (e.g., 1668, 1674, 1684, 1726), hemicellulose (e.g., 1720, 1724), cellulose (e.g., 1723, 1731), which are overlapped with each other and water band (e.g., 1887–2000).
(4) ABOVE 2000 nm: Assignment in this region is difficult due to high number of possibilities for the coupling of vibrations.
There are a number of well-established NIR spectra preprocessing techniques that can be used to achieve resolution enhancement and to more precisely locate band position. Methods for spectral data preprocessing include: (1) smoothing and derivatization (Denoyer and Dodd, 2002; Rousset et al., 2011) such as using the algorithm based method used by Savitzky and Golay (1964), (2) calculation of differential spectra (Rousset et al., 2011), and (3) Fourier self de-convolution, curve fitting (Ozaki et al., 2001) with more advanced techniques involving PCA (Fackler and Schwanninger, 2010) and two dimensional correlation analysis (Ozaki et al., 2001; Schwanninger et al., 2011).
Among those preprocessing methods, derivatives are widely used to reduce the impact of overlapping peaks and baseline variation. However, there is a concern that generating derivatives can possibly generate false information. Both the shape of the spectrum and the data processing algorithms have an impact on band shape and location. Differences between the location of the bands between the raw and the second derivative spectrum can be more than 20 cm-1 (5 nm). Researchers have also reported that the second derivative form was not always more precise than the normal form for the prediction of lignin in wood (Michell, 1995; Xu et al., 2013). Therefore, when spectral data is processed with the second derivative, possible peak shifts should be taken into consideration. The same consideration is also important for deriving conclusions from processing spectra of PCA and regression coefficients from PLS (Schwanninger et al., 2011).
NIR Spectroscopy Coupled with PCA
The primary application of NIR coupled with PCA is to classify biomass samples of various origins or from different pretreatments without conducting laborious traditional wet chemistry techniques on all samples. Related areas of this application are summarized below:
(1) Related to species/plant fractions (Michell, 1995; Kelley et al., 2004a; Labbe et al., 2008a,b; Nkansah et al., 2010);
(2) Related to genetic engineering of feedstock crops (Baillères et al., 2002; Sandak and Sandak, 2011; Zhou et al., 2011);
(3) Related to chemical/thermal/biological treatments (Kelley et al., 2004b; Yang et al., 2007; Houghton et al., 2009; Krongtaew et al., 2010).
For example, in order to evaluate the impact of biomass pretreatments (including acid and alkaline pretreatments, some in combination with hydrogen peroxide) on the change of cell wall compositions of wheat and oat straw, FT-NIR was utilized to characterize raw and pretreated straw (Krongtaew et al., 2010). Second derivatives from NIR absorption bands were generated and evaluated to show the changes in properties related to biomass recalcitrance during subsequent bioethanol production. These properties include the change of lignin, hemicelluloses; as well as amorphous, semi-crystalline, and crystalline regions of cellulose moieties of pretreated sample. PCA of derivative data was efficiently utilized to differentiate the alterations in chemical structure of straw due to different pretreatment methods as shown in Figure 1. It was demonstrated that FT-NIR coupled with PCA is a powerful tool to assess biomass digestibility, with a potential to be used in process control in the area of biomass utilization or energy conversion.
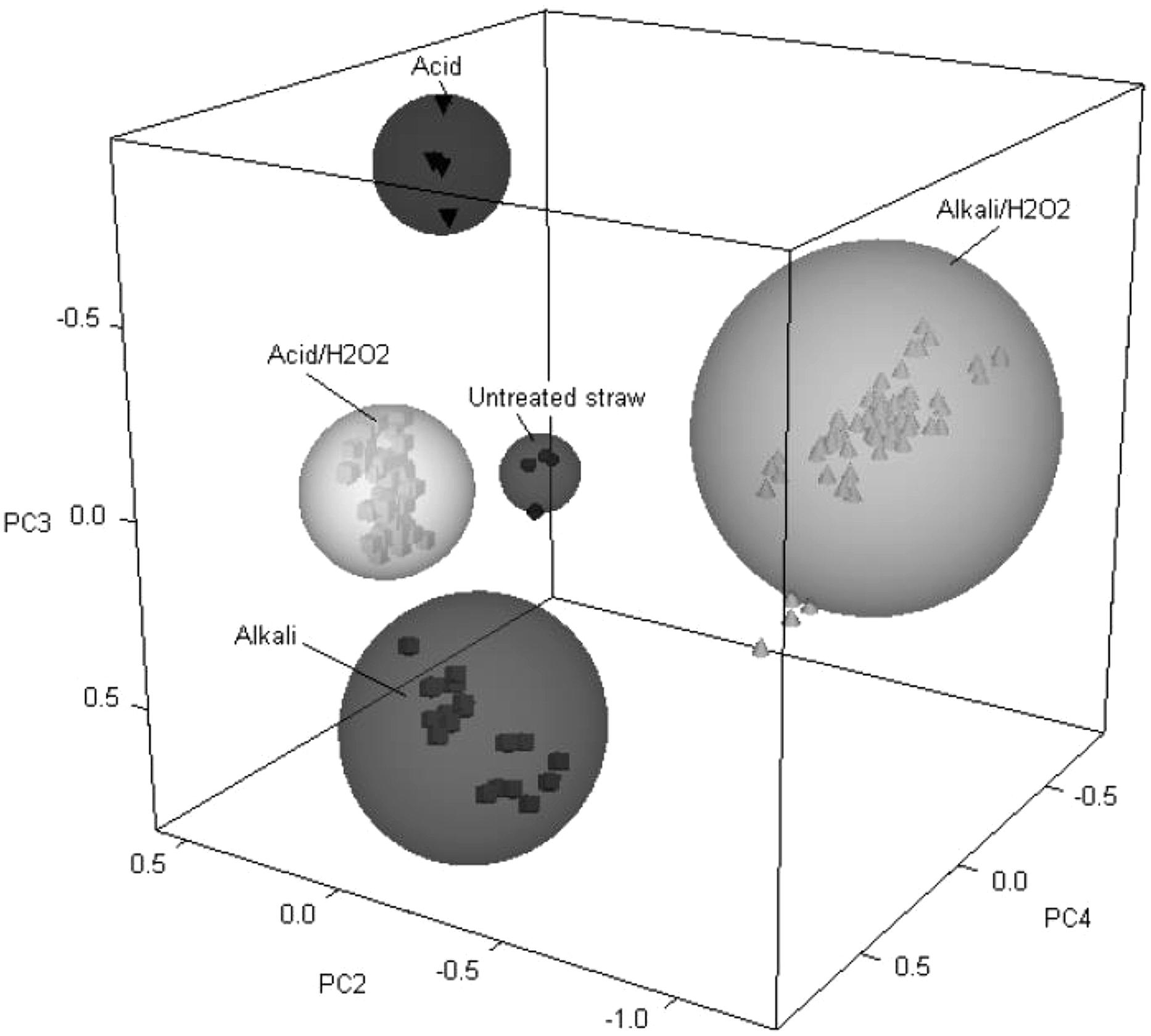
FIGURE 1. PCA scores plot of untreated wheat straw samples (•) and samples treated with acid (▼), alkali (▪), acid/H2O2 (□), and alkali/H2O2 (Δ) as reproduced from literature (Krongtaew et al., 2010).
NIR Spectroscopy Coupled with PLS
One of the main applications of NIR coupled with PLS is to build regression models for the prediction of biomass properties, such as lignin content, S/G-lignin ratio, moisture content, heating value (Kelley et al., 2004a; Rousset et al., 2011; Schwanninger et al., 2011).
Related areas of the application of NIR coupled with PLS in existing literatures are summarized below:
(1) Prediction of cell wall components (Michell, 1995; Sanderson et al., 1996; Tucker et al., 2001; Baillères et al., 2002; Kelley et al., 2004a; Lovett et al., 2004; Yeh et al., 2004; Jin and Chen, 2007; Labbe et al., 2008b; Philip Ye et al., 2008; Wolfrum and Sluiter, 2009; Nkansah et al., 2010; Hou and Li, 2011; Sandak and Sandak, 2011; Smith-Moritz et al., 2011; Zhou et al., 2011).
For example, in order to identify specific monosaccharide outliers from a plant mutant population, FT-NIR coupled with PLS regression was utilized to analyze plant leaves of Arabidopsis (Smith-Moritz et al., 2011). Various Arabidopsis cell wall mutants were analyzed for prediction model building. PCA was performed on pre-processed and area-normalized NIR spectra, followed by calculation of the Mahalanobis distance, a linear discriminate analysis technique to identify outliers using PCA results. By using this technique, a pilot study was conducted which consisted of 550 mutant lines (3590 leaf samples), resulting in a set of 235 leaf samples as Mahalanobis outliers. Quantitative information about monosaccharide composition is gained by means of PLS modeling with known biochemical values and FT-NIR spectra. The correlation between predicted and experiment determined monosaccharide composition (mol%) of 226 rice leaf samples are shown in Figure 2 with R2 = 0.98 (Smith-Moritz et al., 2011).
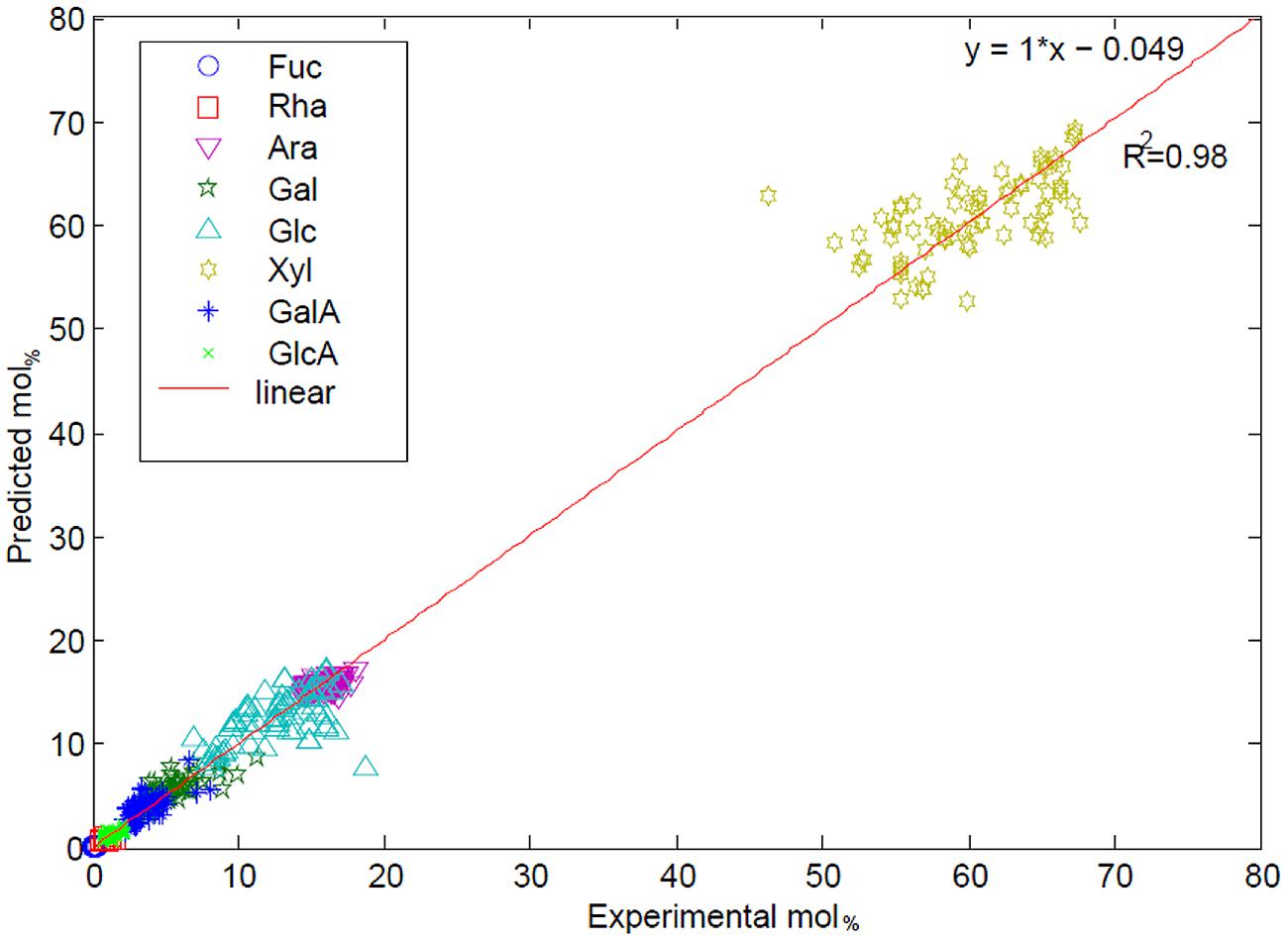
FIGURE 2. A correlation analysis predicted (PLS model of FT-NIR) versus experimentally determined monosaccharide composition (mol%) of rice leaf samples. The correlation coefficient between experimental and predicted values was calculated to be R2 = 0.98 as reproduced from literature (Agblevor et al., 1994; Smith-Moritz et al., 2011).
(2) Prediction of other physical properties (Thygesen, 1994; Hoffmeyer and Pedersen, 1995), mechanical properties (Kelley et al., 2004a; André et al., 2006), fuel properties (Lestander and Rhen, 2005; Labbe et al., 2008a).
For example, NIR coupled with PLS has been used to predict cell wall chemistry and mechanical properties of loblolly pine from different radial locations and heights of trees grown in Arkansas (Kelley et al., 2004a). Mechanical properties include three point bending test and related microfibril angle. The correlation between experimental data and predicted data from PLS modeling is very strong with correlation coefficients (r) as high as 0.80. A reduced spectral range (650–1150 nm) usually available in handheld NIR spectrometers was also demonstrated to be useful for predicting mechanical properties.
Biomass Characterization by Py-mbms
Py-mbms has been intensively used for studies of biological and synthetic macromolecules, such as wood, grasses, carbon in soil and chars. It has proved to be an efficient and powerful analytical tool (Evans and Milne, 1987; Kelley et al., 2002; Labbe et al., 2005; Magrini et al., 2007; Sykes et al., 2008; Mann et al., 2009; French and Czernik, 2010). Detailed description of this technology is available in the above references. In short, the Py-mbms is composed of a pyrolysis furnace and a free-jet mbms. Typically the furnace is preheated to 500°C before ground sample of biomass is inserted into the inert atmosphere of the furnace. Pyrolysis products from biomass in the furnace are swept out of the furnace into the mbms by an argon gas stream. Molecular fragments contained in the pyrolysis vapor are expanded in a series of vacuum chambers to be quenched; so that intermolecular collisions are prevented. A low-energy electron beam (17–23 eV) in the triple quadruple mass spectrometer is employed to produce a positive ion mass spectrum. The positive ion stream is magnified and collected by the detector.
Mass peaks were assigned to chemical fragments produced from fast pyrolysis of biomass for direct interpretation (Evans and Milne, 1987). The spectra from Py-mbms is also interpreted with the help of MVA tools, especially PLS and PCA (Hoover et al., 2002; Kelley et al., 2002, 2004b; Labbe et al., 2005; Magrini et al., 2007; Mann et al., 2009).
Py-mbms Peak Assignment and Data Processing
During data acquisition of Py-mbms, amplified positive ions from biomass pyrolysis vapor are scanned continuously; then the signal is collected by a computer. Approximate evolution time of fast pyrolysis for a sample of 4 mg is less than 1 min. During the evolution time there are typically 50 single scans collected. Biomass with larger sample size will need longer evolution time and more scans during fast pyrolysis. Together with single scan spectrum, time resolved profile and averaged spectrum can be collected by the computer acquisition software (Evans and Milne, 1987).
Average spectra are also known as spectral “fingerprints.” Spectral fingerprints gained at analytical pyrolysis temperature of 500–550°C and the molecular beam free jet expansion represent primary products from biomass pyrolysis. Studies shown that at this temperature range, molecular structure of the original biomass is well preserved and there is no interaction observed among organic components during pyrolysis, although inorganics may alter the pyrolysis pathways of the carbohydrates (Evans and Milne, 1987). Thus, with known peak assignment, spectral “fingerprints” generated could be used to depict the molecular structure of chemical composition in biomass. A summary of important peak assignment in biomass is shown in Table 1 (Evans and Milne, 1987; Sykes et al., 2008). Characteristic spectral fingerprints of whole biomass samples and separated constituents of biomass are shown in Figure 3 (Evans and Milne, 1987).

TABLE 1. Peak assignments associated with Py-mbms spectrum for Populus wood based on literature (Evans and Milne, 1987; Sykes et al., 2008).
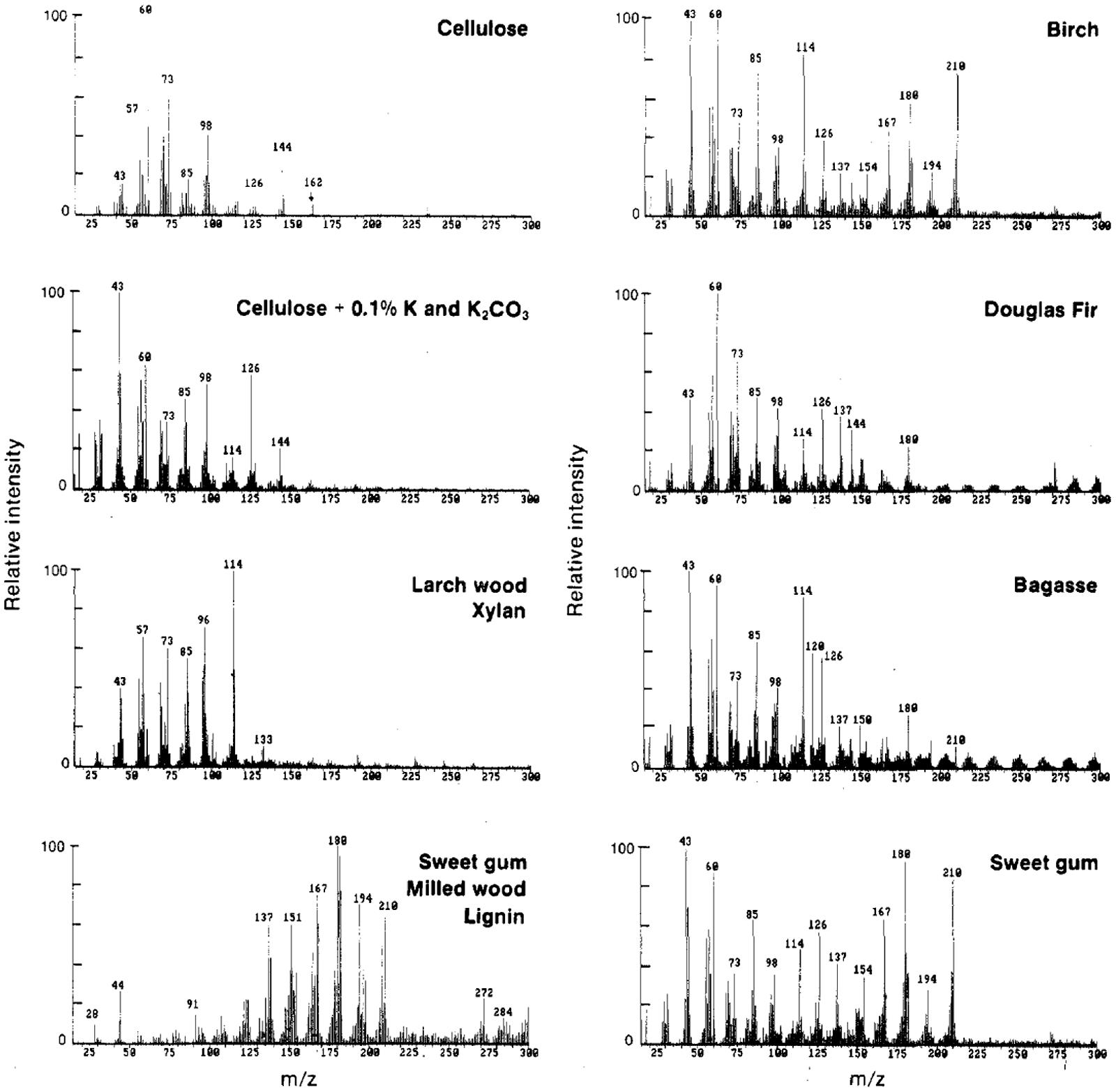
FIGURE 3. Characteristic mass spectral patterns of primary pyrolysis products for several whole biomass samples and for separated constituents of biomass (Evans and Milne, 1987).
Pyrolysis-molecular beam mass spectrometry has been successfully applied in many biomass-related studies, including the research of cellulose, cellulose with inorganics, many woods, xylan, milled wood lignin, bagasse (Evans and Milne, 1987), herbaceous biomass under different storage environments (Agblevor et al., 1994), hardwood sawdust and its torrefaction products (Nimlos et al., 2003), and poplar grown under different nitrogen conditions (Sykes et al., 2009).
For example, in the study of bark phenolysis conducted by Alma and Kelley, bark and its phenolysis products from Calabrian pine, Lebanon cedar, acacia, and European chestnut were characterized using Py-mbms (Alma and Kelley, 2002). From the results of Py-mbms averaged spectra, it was shown that bark (1) has less common lignin peaks at m/z 180, 194, 210 assigned to coniferyl alcohol/vinylsyringol, 4-propenylsyringol/ferulic acid, and sinapyl alcohol, respectively; (2) has unique triplet of peaks at m/z of 96, 97, 98 assigned to furans; and (3) has more phenols, such as peaks at m/z of 110, 124, 150, and 164 assigned to catechol, guaiacol, vinyl guaiacol, and isoeugenol. In softwood bark, extractives and lignin dimers can be identified at m/z of 298, 300, 302, and 272 assigned to didehydroabeitic acid, dehydroabeitic acid, abeiticacid, and lignin dimer, respectively (Alma and Kelley, 2002). These results are consistent with known differences between bark and wood.
Selected Peaks From Py-mbms Raw Data
As summarized above, certain Py-mbms peaks can be unambiguously assigned to specific biomass components. Lignin fragments are particularly easy to identify. Because of this, Klason lignin content of biomass can be directly estimated from Py-mbms spectral fingerprints. Firstly, spectral fingerprints of samples are area/mean normalized for the mass of the original sample. Then, the total intensity of lignin related peaks from the normalized spectrum is calculated. After that, a correction factor is calculated by dividing the known Klason lignin value by the summed intensity of a NIST standard material. The correction factor can be used to convert the total intensity of lignin related peaks to Klason lignin content (Davis and Lagutaris, 2002; Sykes et al., 2008, 2009; Ziebell et al., 2013). Similarly, S/G ratios were determined by dividing the sum of S-lignin peaks by the sum of G-lignin peaks excluding peaks associated with both S and G fragments (Davis and Lagutaris, 2002; Sykes et al., 2008, 2009; Mann et al., 2009; Ziebell et al., 2013).
For example, corrected lignin values and S/G-lignin ratio were determined from Py-mbms for 800 greenhouse-grown poplar trees grown under atmosphere containing different amount of nitrogen (Sykes et al., 2009). Lignin contents ranged from 13 to 28% whereas S/G ranged from 0.5 to 1.5. It was shown that the variations in cell wall composition were larger in the plants grown under high nitrogen conditions than those grown under low nitrogen conditions.
Similarly, “within-tree” variability in lignin content and S/G ratio with increasing height and increasing ring for poplars was determined by Py-mbms (Sykes et al., 2008). Wood disks from seven different poplar trees, which were seven years old, were sampled at five different heights of 0.3, 0.6, 1.2, 1.8, and 2.4 m from base to stem. Samples were collected from the north side of each wood disk taken at height of 1.2 m to study difference between growth rings. According to results from Py-mbms, ring effect on lignin content was significant while the effect of height was small. Higher S/G ratio was observed with increasing ring size, whereas lignin content decreased. S/G ratio was determined for switchgrass grown under different environment using the same methodology (Mann et al., 2009).
Py-mbms Coupled with PCA
Pyrolysis-molecular beam mass spectrometry coupled with PCA provides a fast analytical method to distinguish a large number of biomass samples. It has been used to study biomass compositional variations due to species (Evans and Milne, 1987; Agblevor et al., 1994; Alma and Kelley, 2002; Kelley et al., 2004b), genetic engineering (Labbe et al., 2005; Davis et al., 2006), different growth environments (Mann et al., 2009; Sykes et al., 2009), thermal (Nimlos et al., 2003)/chemical (Alma and Kelley, 2002; Kelley et al., 2004b)/biological (Kelley et al., 2002; Arantes et al., 2009) treatments, and various storage/collection (Agblevor et al., 1994) methods.
For example, Py-mbms coupled with PCA has been used to measure the overall composition between and within a series of original and transgenic aspens (Labbe et al., 2005). Two clones were transformed with GRP-iaaM gene (N1-17-26 and N1-2-1) and GRP-iaaM/35S-ACCase (N2-4-9 and N2-5-5). PCA analysis was conducted for data analysis with an attempt to identify chemical differences between the modified and control aspens. Figure 4 shows PCA scores plots with four replicate samples from five different aspen samples. Figure 4A shows a plot of PC1 versus PC2, while Figure 4B shows a plot of PC2 versus PC3. In Figure 4A, there is clear separation between the two N1 samples while two N2 samples are indistinguishable. Moreover, two N2 samples are clearly separated from each other along PC3 as shown in Figure 4B. The loadings from PCA are shown in Figure 5. Using PC1 loadings as an example, C5 carbohydrates (m/z 85 and 114) and lignin (m/z 137, 180, 210, and 272) are highlighted for PC1. This suggests there are more C5 sugars and less lignin in controls than those in N1 and N2 samples (Labbe et al., 2005).
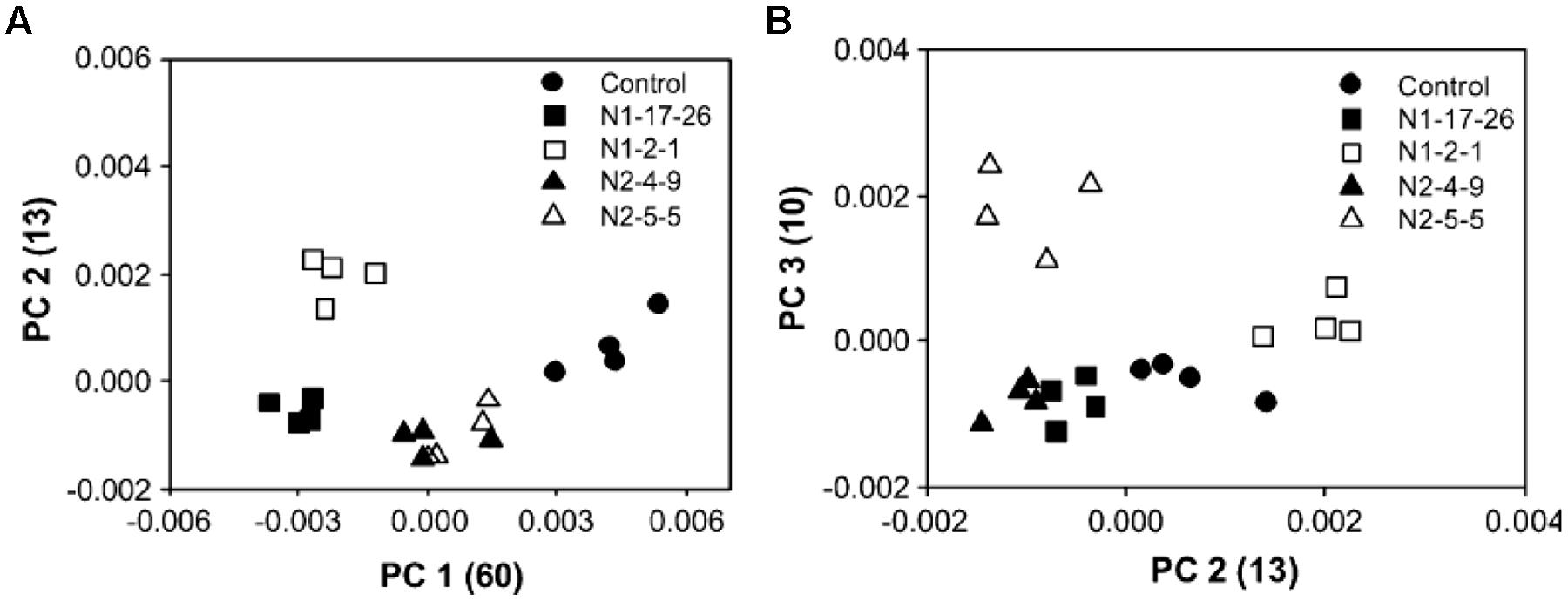
FIGURE 4. Scores plot of PCA of Py-mbms data for original and transgenic aspens; (A) PC1 versus PC2; (B) PC2 versus PC3; N1 samples are clearly separated from control samples in (A) while two N2 samples are not distinguishable; in (B) two N2 samples are clearly separated by PC3 as reproduced from literature (Labbe et al., 2005).
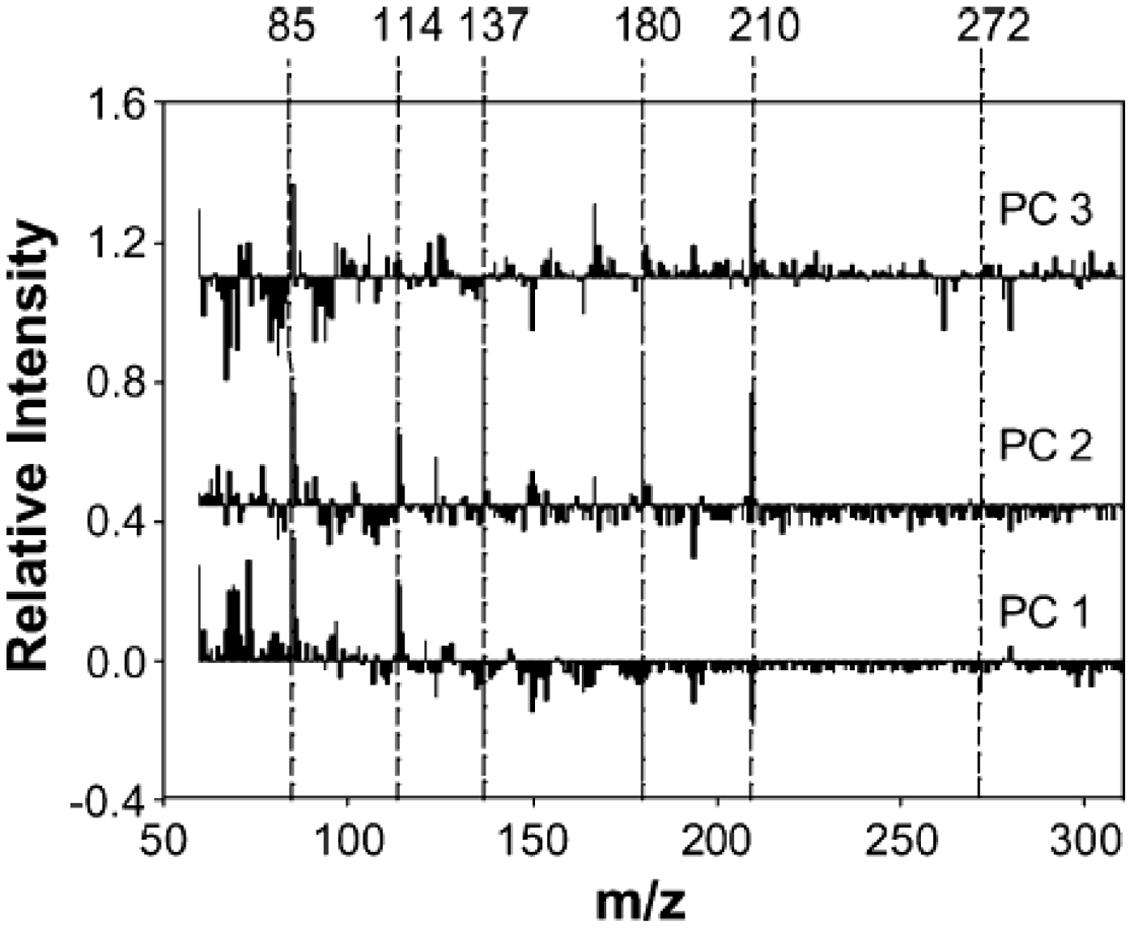
FIGURE 5. Loadings from PCA of Py-mbms data for original and transgenic aspens; from top to bottom: PC3, PC2, PC1; C5 carbohydrates (m/z 85 and 114) and lignin (m/z 137, 180, 210, and 272) are highlighted for PC1 as reproduced from literature (Labbe et al., 2005).
Pyrolysis-molecular beam mass spectrometry had been also used to study the impact of storage environment on herbaceous material. Weathered and unweathered fractions of three types of herbaceous biomass after storage at 18 different conditions for 6–9 months were analyzed by Py-mbms coupled with PCA (Agblevor et al., 1994). Two major trends in the data were shown by PCA (factor analysis): major clusters were distinguished by relative nitrogen contents between switchgrass and the other two herbaceous biomass samples; subgroups of weathered and unweathered materials are clearly separated as subgroups within the major clusters. According to the variance diagram (similar to loadings plot), lower amount of carbohydrates constituted the major chemical difference between weathered and unweathered samples (Agblevor et al., 1994). This observation is consistent with results from traditional wet chemical analysis and Py-GC/MS.
In some cases, there is no separation of clusters in PCA scores plot. This indicates that there is no comprehensive difference among samples for the specific chemical features included in those particular PCs.
For example, three transgenic clones of populous wood were analyzed by Py-mbms, GC/MS, and traditional wet chemical techniques to screen for possible variations in cell wall composition due to genetic engineering (Davis et al., 2006). Various Bacillus thuringiensis (Bt) gene-containing constructs were used to transform poplar genotypes. Transgenic poplar was then compared with non-transgenic control. PCA results showed that there were generally no distinct groupings of individual transgenic lines or non-transgenic controls, indicating no significant differences in cell wall composition between control and transgenic poplars (Davis et al., 2006).
Py-mbms Coupled with PLS
One of the primary applications of Py-mbms has been the development of prediction models for biomass compositional properties. Results from conventional methods of cell wall compositional analysis were used as references to build calibration models with capability for predicting the composition for future samples. As a result, laborious wet chemistry techniques can be eliminated. PLS regression is widely used in this arena for both woody (Tuskan et al., 1999; Labbe et al., 2005) and herbaceous biomass (Agblevor et al., 1994; Kelley et al., 2004b; Mann et al., 2009).
For example, the effectiveness of NIR and Py-mbms in predicting cell wall composition of various agricultural residues was tested (Kelley et al., 2004b). Forty-one samples from 14 species with known content of lignin and six individual sugars were analyzed by NIR and Py-mbms. Prediction models were built between spectral data from both techniques and cell wall compositional data. Correlation coefficient and root mean square error data for each calibration and validation model was presented and compared. Good correlations between the predicted and measured value of major components (lignin, glucose, xylose, and mannose) were obtained (correlation coefficients of both calibration and validation model are above 0.80 for both NIR and Py-mbms), while correlations for minor sugars (mannose, galactose, arabinose, and rhamnose) were not as good. A summary of PLS prediction of chemical composition from Py-mbms is presented in Table 2. According to the author, more samples for specific feedstocks are needed for building improved models. This work also did a thorough comparison between NIR and Py-mbms (Kelley et al., 2004b).
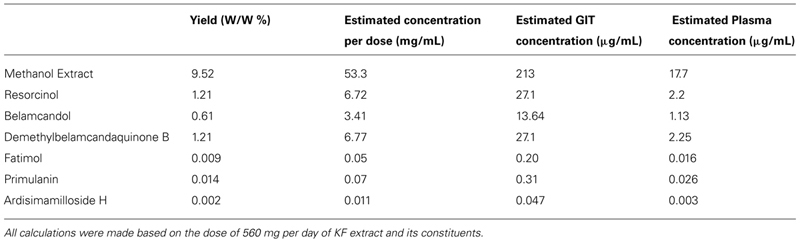
TABLE 2. Summary of the PLS-2 predictions of chemical composition from Py-mbms (six PCs; Kelley et al., 2004b).
Other than being used to predict cell wall composition of biomass, PLS has been applied in predicting other biomass properties and processing parameters. The acidic phenolysis condition of bark (Alma and Kelley, 2002), weight loss during fungal degradation of spruce (Kelley et al., 2002) and carbon content/fraction of different soils (Hoover et al., 2002; Magrini et al., 2007) were also predicted by Py-mbms coupled with PLS.
For example, NIR and Py-mbms were utilized to monitor the chemical changes of wood undergoing brown-rot degradation. In this case, spruce blocks were infected by Postia placenta or Glaoeophyllum trabeum for 0, 2, 4, 8, and 16 weeks (Kelley et al., 2002). Weight losses over the time period were monitored and recorded. PLS models were built to predict weight loss. Strong correlation between recorded weight loss and predicted weight were obtained (correlation coefficients of calibration model reached 0.98, while those of test model reached 0.96 for both NIR and Py-mbms). The regression coefficients for PLS model from Py-mbms data show that weight loss during decay is positively correlated to carbohydrates (m/z 85, 114, and 126) and negatively correlated to monomethoxylated lignin fragments (m/z 123, 138, and 151; Kelley et al., 2002).
Conclusion
Compared to traditional techniques in biomass characterization, high-throughput analytical techniques, such as NIR and Py-mbms have been proved to be efficient tools in exploring the chemical features of different biomass samples with minimal sample preparation. These high-throughput techniques coupled with MVA have been demonstrated to be efficient in identifying outliers, comparing samples (using PCA), and building prediction models (using PLS). Both NIR and Py-mbms coupled with MVA could be used not only for characterizing the cell wall chemistry, but also for predicting other chemical, physical, mechanical, and fuel properties. In comparison with Py-mbms, NIR has the advantages of low cost and simple instrumentation, field-portable, and nondestructive, whereas Py-mbms provides superior information of molecular structural information.
Thus, we recommend that NIR and Py-mbms coupled with MVA should be widely employed for biomass characterization. Additional fundamental work on assigning NIR vibrations band and Py-mbm speaks for modified biomass or biomass related products are recommended since current assignment are mainly based on the study of unmodified biomass. Lack of assignments for new bands/peaks in modified biomass limit the application of these two techniques in exploring the fundamental changes of chemical composition of modified biomass. Also, comparison and correlation between analytical results from Py-GC/MS and Py-mbms should be encouraged because of the important similarity and differences in these two techniques are critical for using those techniques for the characterization of biomass molecular structure.
Conflict of Interest Statement
The authors declare that the research was conducted in the absence of any commercial or financial relationships that could be construed as a potential conflict of interest.
Acknowledgments
This project is supported by the project of Southeastern Partnership for Integrated Biomass Supply Systems (IBSS). The IBSS project is supported by Agriculture and Food Research Initiative Competitive Grant No. 2011-68005-30410 from the USDA National Institute of Food and Agriculture. Hui Wei and Michael E. Himmel are sponsored by the Center for Direct Catalytic Conversion of Biomass to Biofuels (C3Bio), an Energy Frontier Research Center funded by the US Department of Energy, Office of Science, Office of Basic Energy Sciences under Award Number DE-SC0000997, and also by Laboratory Directed Research & Development (LDRD) program at the National Renewable Energy Laboratory (NREL). Experimental data in figures and tables were generated with the help of Robert Sykes and Mark Davis at NREL. This work was conducted as part of the BioEnergy Science Center (BESC). The BESC is a US Department of Energy Bioenergy Research Center supported by the Office of Biological and Environmental Research in the DOE Office of Science.
References
Agblevor, F. A., Evans, R. J., and Johnson, K. D. (1994). Molecular-beam mass-spectrometric analysis of lignocellulosic materials 1. Herbaceous biomass. J. Anal. Appl. Pyrolysis 30, 125–144. doi: 10.1016/0165-2370(94)00808-6
Alma, M. H., and Kelley, S. S. (2002). The application of pyrolysis-molecular beam mass spectrometry for characterization of bark phenolysis products. Biomass Bioenergy 22, 411–419. doi: 10.1016/S0961-9534(02)00018-1
André, N., Labbé, N., Rials, T., and Kelley, S. (2006). Assessment of wood load condition by near infrared (NIR) spectroscopy. J. Mater. Sci. 41, 1879–1886. doi: 10.1007/s10853-006-4433-6.
Arantes, V., Qian, Y. H., Kelley, S. S., Milagres, A. M. F., Filley, T. R., Jellison, J.,et al. (2009). Biomimetic oxidative treatment of spruce wood studied by pyrolysis-molecular beam mass spectrometry coupled with multivariate analysis and C-13-labeled tetramethylammonium hydroxide thermochemolysis: implications for fungal degradation of wood. J. Biol. Inorg. Chem. 14, 1253–1263. doi: 10.1007/s00775-009-0569-6
Baillères, H., Davrieux, F., and Ham-Pichavant, F. (2002). Near infrared analysis as a tool for rapid screening of some major wood characteristics in a eucalyptus breeding program. Ann. For. Sci. 59, 479–490. doi: 10.1051/forest:2002032
Braadbaart, F., and Poole, I. (2008). Morphological, chemical and physical changes during charcoalification of wood and its relevance to archaeological contexts. J. Archaeol. Sci. 35, 2434–2445. doi: 10.1016/j.jas.2008.03.016
Browning, B. L. (1967). Methods of Wood Chemistry. NY: Interscience Publishers, a Division of John Wiley & Sons.
Davis, M. F., and Lagutaris, R. M. (2002). Comparison of syringyl/guaiacyl (S/G) ratios measured by solid state 13C NMR, pyrolysis molecular beam mass spectrometry and thioacidolysis. Abstr. Pap. Am. Chem. Soc. 223, U126–U126.
Davis, M. F., Tuskan, G. A., Payne, P., Tschaplinski, T. J., and Meilan, R. (2006). Assessment of Populus wood chemistry following the introduction of a Bt toxin gene. Tree Physiol. 26, 557–564. doi: 10.1093/treephys/26.5.557
Daystar, J. S., Venditti, R. A., Gonzalez, R., Jameel, H., Jett, M., and Reeb, C. W. (2013). Impacts of feedstock composition on alcohol yields and greenhouse gas emissions from the NREL thermochemical ethanol conversion process. Bioresources 8, 5261–5278.
Denoyer, L. K., and Dodd, J. G. (2002). “Smoothing and derivatives in spectroscopy,” in Handbook of Vibrational Spectroscopy, eds J. M. Chalmers and P. R. Griffiths (Chichester: John Wiley & Sons Ltd.), 12.
Evans, R. J., and Milne, T. A. (1987). Molecular characterization of the pyrolysis of biomass 1. Fundamentals. Energy Fuels 1, 123–137. doi: 10.1021/ef00002a001
Fackler, K., and Schwanninger, M. (2010). Polysaccharide degradation and lignin modification during brown rot of spruce wood: a polarised Fourier transform near infrared study. J. Near Infrared Spectrosc. 18, 403–416. doi: 10.1255/jnirs.901
French, R., and Czernik, S. (2010). Catalytic pyrolysis of biomass for biofuels production. Fuel Process. Technol. 91, 25–32. doi: 10.1016/j.fuproc.2009.08.011
Himmel, M. E., Ding, S. Y., Johnson, D. K., Adney, W. S., Nimlos, M. R., Brady, J. W.,et al. (2007). Biomass recalcitrance: engineering plants and enzymes for biofuels production. Science 315, 804–807. doi: 10.1126/science.1137016
Hoffmeyer, P., and Pedersen, J. G. (1995). Evaluation of density and strength of Norway spruce wood by near infrared reflectance spectroscopy. Holz Roh Werkstoff 53, 165–170. doi: 10.1007/BF02716418
Hoover, C. M., Magrini, K. A., and Evans, R. J. (2002). Soil carbon content and character in an old-growth forest in northwestern Pennsylvania: a case study introducing pyrolysis molecular beam mass spectrometry (py-mbms). Environ. Pollut. 116, S269–S275. doi: 10.1016/s0269-7491(01)00258-5
Hou, S., and Li, L. (2011). Rapid characterization of woody biomass digestibility and chemical composition using near-infrared spectroscopy. J. Integr. Plant Biol. 53, 166–175. doi: 10.1111/j.1744-7909.2010.01003.x
Houghton, T. P., Stevens, D. M., Pryfogle, P. A., Wright, C. T., and Radtke, C. W. (2009). The effect of drying temperature on the composition of biomass. Appl. Biochem. Biotechnol. 153, 4–10. doi: 10.1007/s12010-008-8406-x
Jin, L., and Xu, Q. (2011). Application of near infrared spectroscopy and multivariate analysis in the forest products industry. Adv. Mater. Res. (Durnten-Zurich, Switz.) 236–238, 1098–1102. doi: 10.4028/www.scientific.net/AMR.236-238.1098
Jin, S., and Chen, H. (2007). Near-infrared analysis of the chemical composition of rice straw. Ind. Crops Prod. 26, 207–211. doi: 10.1016/j.indcrop.2007.03.004
Kelley, S., Rials, T., Snell, R., Groom, L., and Sluiter, A. (2004a). Use of near infrared spectroscopy to measure the chemical and mechanical properties of solid wood. Wood Sci. Technol. 38, 257–276. doi: 10.1007/s00226-003-0213-215
Kelley, S. S., Rowell, R. M., Davis, M., Jurich, C. K., and Ibach, R. (2004b). Rapid analysis of the chemical composition of agricultural fibers using near infrared spectroscopy and pyrolysis molecular beam mass spectrometry. Biomass Bioenergy 27, 77–88. doi: 10.1016/j.biomboie.2003.11.005
Kelley, S. S., Jellison, J., and Goodell, B. (2002). Use of NIR and pyrolysis-mbms coupled with multivariate analysis for detecting the chemical changes associated with brown-rot biodegradation of spruce wood. FEMS Microbiol. Lett. 209, 107–111. doi: 10.1111/j.1574-6968.2002.tb11117.x
Krongtaew, C., Messner, K., Ters, T., and Fackler, K. (2010). Characterization of key parameters for biotechnological lignocellulose conversion assessed by ft-NIR spectroscopy. Part I. Qualitative analysis of pretreated straw. Bioresources 5, 2063–2080.
Labbe, N., Harper, D., and Rials, T. (2006). Chemical structure of wood charcoal by infrared spectroscopy and multivariate analysis. J. Agric. Food Chem. 54, 3492–3497. doi: 10.1021/jf053062n
Labbe, N., Lee, S. H., Cho, H. W., Jeong, M. K., and Andre, N. (2008a). Enhanced discrimination and calibration of biomass NIR spectral data using non-linear kernel methods. Bioresour. Technol. 99, 8445–8452. doi: 10.1016/j.biortech.2008.02.052
Labbe, N., Ye, X. P., Franklin, J. A., Womac, A. R., Tyler, D. D., and Rials, T. G. (2008b). Analysis of switchgrass characteristics using near infrared spectroscopy. Bioresources 3, 1329–1348.
Labbe, N., Rials, T. G., Kelley, S. S., Cheng, Z. M., Kim, J. Y., and Li, Y. (2005). FT-IR imaging and pyrolysis-molecular beam mass spectrometry: new tools to investigate wood tissues. Wood Sci. Technol. 39, 61–77. doi: 10.1007/s00226-004-0274-0
Lestander, T. A., and Rhen, C. (2005). Multivariate NIR spectroscopy models for moisture, ash and calorific content in biofuels using bi-orthogonal partial least squares regression. Analyst 130, 1182–1189. doi: 10.1039/B500103J
Lovett, D. K., Deaville, E. R., Mould, F., Givens, D. I., and Owen, E. (2004). Using near infrared reflectance spectroscopy (NIRS) to predict the biological parameters of maize silage. Anim. Feed Sci. Technol. 115, 179–187. doi: 10.1016/j.anifeedsci.2004.02.007
Lupoi, J. S., Singh, S., Simmons, B. A., and Henry, R. J. (2014). Assessment of lignocellulosic biomass using analytical spectroscopy: an evolution to high-throughput techniques. Bioenergy Res. 7, 1–23. doi: 10.1007/s12155-013-9352-1
Magrini, K. A., Follett, R. F., Kimble, J., Davis, M. F., and Pruessner, E. (2007). Using pyrolysis molecular beam mass spectrometry to characterize soil organic carbon in native prairie soils. Soil Sci. 172, 659–672. doi: 10.1097/ss.0b013e3180d0a3a5
Mann, D. G. J., Labbe, N., Sykes, R. W., Gracom, K., Kline, L., Swamidoss, I. M.,et al. (2009). Rapid assessment of lignin content and structure in switchgrass (Panicum virgatum L.) grown under different environmental conditions. Bioenergy Res. 2, 246–256. doi: 10.1007/s12155-009-9054-x
Michell, A. J. (1995). Pulpwood quality estimation by near-infrared spectroscopic measurements on eucalypt woods. Appita J. 48, 425–428.
Nimlos, M., Brooking, E., Looker, M. J., and Evans, R. J. (2003). Biomass torrefaction studies with a molecular beam mass spectrometer. Abstr. Pap. Am. Chem. Soc. 226, U536–U536.
Nkansah, K., Dawson-Andoh, B., and Slahor, J. (2010). Rapid characterization of biomass using near infrared spectroscopy coupled with multivariate data analysis. Part 1: yellow-poplar (Liriodendron tulipifera L.). Bioresour. Technol. 101, 4570–4576. doi: 10.1016/j.biortech.2009.12.046
Obernberger, I. (2014). Strategy for the application of novel characterization methods for biomass fuels: case study of straw. Energy Fuels 28, 1041–1052. doi: 10.1021/ef402249x
Ozaki, Y., Šašiè, S., and Jiang, J. (2001). Review: how can we unravel complicated near infrared spectra?—recent progress in spectral analysis methods for resolution enhancement and band assignments in the near infrared region. J. Near Infrared Spectrosc. 9, 63–95. doi: 10.1255/jnirs.295
Philip Ye, X., Liu, L., Hayes, D., Womac, A., Hong, K., and Sokhansanj, S. (2008). Fast classification and compositional analysis of cornstover fractions using Fourier transform near-infrared techniques. Bioresour. Technol. 99, 7323–7332. doi: 10.1016/j.biortech.2007.12.063
Ragauskas, A. J., Williams, C. K., Davison, B. H., Britovsek, G., Cairney, J., Eckert, C. A.,et al. (2006). The path forward for biofuels and biomaterials. Science 311, 484–489. doi: 10.1126/science.1114736
Rousset, P., Davrieux, F., Macedo, L., and Perre, P. (2011). Characterisation of the torrefaction of beech wood using NIRS: combined effects of temperature and duration. Biomass Bioenergy 35, 1219–1226. doi: 10.1016/j.biombioe.2010.12.012
Sandak, J., and Sandak, A. (2011). Fourier transform near infrared assessment of biomass composition of shrub willow clones (Salix sp.) for optimal bio-conversion processing. J. Near Infrared Spectrosc. 19, 309–318. doi: 10.1255/jnirs.950
Sanderson, M. A., Agblevor, F., Collins, M., and Johnson, D. K. (1996). Compositional analysis of biomass feedstocks by near infrared reflectance spectroscopy. Biomass Bioenergy 11, 365–370. doi: 10.1016/S0961-9534(96)00039-6
Savitzky, A., and Golay, M. J. E. (1964). Smoothing + differentiation of data by simplified least squares procedures. Anal. Chem. 36:1627. doi: 10.1021/ac60214a047
Schwanninger, M., Rodrigues, J. C., and Fackler, K. (2011). A review of band assignments in near infrared spectra of wood and wood components. J. Near Infrared Spectrosc. 19, 287–308. doi: 10.1255/jnirs.955
Sjöström, E., and Alén, R. (1999). Analytical Methods in Wood Chemistry, Pulping, and Papermaking. Berlin Heidelberg: Springer-Verlag. doi: 10.1007/978-3-662-03898-7
Sluiter, J. B., Ruiz, R. O., Scarlata, C. J., Sluiter, A. D., and Templeton, D. W. (2010). Compositional analysis of lignocellulosic feedstocks 1. Review and description of methods. J. Agric. Food Chem. 58, 9043–9053. doi: 10.1021/jf1008023
Smith-Moritz, A. M., Chern, M., Lao, J., Sze-To, W. H., Heazlewood, J. L., Ronald, P. C.,et al. (2011). Combining multivariate analysis and monosaccharide composition modeling to identify plant cell wall variations by Fourier transform near infrared spectroscopy. Plant Methods 7:26. doi: 10.1186/1746-4811-7-26
Sykes, R., Kodrzycki, B., Tuskan, G., Foutz, K., and Davis, M. (2008). Within tree variability of lignin composition in Populus. Wood Sci. Technol. 42, 649–661. doi: 10.1007/s00226-008-0199-0
Sykes, R., Yung, M., Novaes, E., Kirst, M., Peter, G., and Davis, M. (2009). “High-throughput screening of plant cell-wall composition using pyrolysis molecular beam mass spectroscopy,” in Biofuels, ed. J. R. Mielenz (New York, NY: Humana Press), 169–183.
Templeton, D. W., Scarlata, C. J., Sluiter, J. B., and Wolfrum, E. J. (2010). Compositional analysis of lignocellulosic feedstocks. 2. Method uncertainties. J. Agric. Food Chem. 58, 9054–9062. doi: 10.1021/jf100807b
Thygesen, L. (1994). Determination of dry matter content and basic density of Norway spruce by near infrared reflectance and transmittance spectroscopy. J. Near Infrared Spectrosc. 2, 127–135. doi: 10.1255/jnirs.39
Tsuchikawa, S. (2007). A review of recent near infrared research for wood and paper. Appl. Spectrosc. Rev. 42, 43–71. doi: 10.1080/05704920601036707
Tsuchikawa, S., Murata, A., Kohara, M., and Mitsui, K. (2003). Spectroscopic monitoring of biomass modification by light-irradiation and heat treatment. J. Near Infrared Spectrosc. 11, 401–405. doi: 10.1255/jnirs.391
Tucker, M., Nguyen, Q., Eddy, F., Kadam, K., Gedvilas, L., and Webb, J. (2001). Fourier transform infrared quantitative analysis of sugars and lignin in pretreated softwood solid residues. Appl. Biochem. Biotechnol. 91–93, 51–61. doi: 10.1385/ABAB:91-93:1-9,51
Tuskan, G., West, D., Bradshaw, H. D., Neale, D., Sewell, M., Wheeler, N.,et al. (1999). Two high-throughput techniques for determining wood properties as part of a molecular genetics analysis of hybrid poplar and loblolly pine. Appl. Biochem. Biotechnol. 77–79, 55–65. doi: 10.1385/abab:77:1-3,55
Via, B. K., Adhikari, S., and Taylor, S. (2013). Modeling for proximate analysis and heating value of torrefied biomass with vibration spectroscopy. Bioresour. Technol. 133, 1–8. doi: 10.1016/j.biortech.2013.01.108
Wei, H., Xu, Q., Taylor, L. E., Baker, J. O., Tucker, M. P., and Ding, S. Y. (2009). Natural paradigms of plant cell wall degradation. Curr. Opin. Biotechnol. 20, 330–338. doi: 10.1016/j.copbio.2009.05.008
Wolfrum, E., and Sluiter, A. (2009). Improved multivariate calibration models for corn stover feedstock and dilute-acid pretreated corn stover. Cellulose 16, 567–576. doi: 10.1007/s10570-009-9320-2
Workman, J. J. Jr. (2001). Infrared and Raman spectroscopy in paper and pulp analysis. Appl. Spectrosc. Rev. 36, 139–168. doi: 10.1081/ASR-100106154
Xu, F., Yu, J. M., Tesso, T., Dowell, F., and Wang, D. H. (2013). Qualitative and quantitative analysis of lignocellulosic biomass using infrared techniques: a mini-review. Appl. Energy 104, 801–809. doi: 10.1016/j.apenergy.2012.12.019
Yang, Z., Jiang, Z. H., Fei, B. H., and Qin, D. C. (2007). Discrimination of wood biological decay by soft independent modeling of class analogy (SIMCA) pattern recognition based on principal component analysis. Spectrosc. Spectral Anal. 27, 686–690.
Yeh, T.-F., Chang, H.-M., and Kadla, J. F. (2004). Rapid prediction of solid wood lignin content using transmittance near-infrared spectroscopy. J. Agric. Food Chem. 52, 1435–1439. doi: 10.1021/jf034874r
Zhou, G., Taylor, G., and Polle, A. (2011). FTIR-ATR-based prediction and modelling of lignin and energy contents reveals independent intra-specific variation of these traits in bioenergy poplars. Plant Methods 7:9. doi: 10.1186/1746-4811-7-9
Keywords: biomass characterization, lignocellulosic biofuel, near infrared spectroscopy, pyrolysis molecular beam, mass spectrometry, multivariate data analysis, high throughput, chemometrics
Citation: Xiao L, Wei H, Himmel ME, Jameel H and Kelley SS (2014) NIR and Py-mbms coupled with multivariate data analysis as a high-throughput biomass characterization technique: a review. Front. Plant Sci. 5:388. doi: 10.3389/fpls.2014.00388
Received: 16 May 2014; Accepted: 22 July 2014;
Published online: 07 August 2014.
Edited by:
Miguel Vega-Sanchez, Lawrence Berkeley National Laboratory, USAReviewed by:
Benjamin Dawson-Andoh, West Virginia University, USAJason Lupoi, University of Queensland, USA
Jakub Sandak, Istituto per la Valorizzazione del Legno e delle Specie Arboree del Consiglio Nazionale delle Ricerche, Italy
Copyright © 2014 Xiao, Wei, Himmel, Jameel and Kelley. This is an open-access article distributed under the terms of the Creative Commons Attribution License (CC BY). The use, distribution or reproduction in other forums is permitted, provided the original author(s) or licensor are credited and that the original publication in this journal is cited, in accordance with accepted academic practice. No use, distribution or reproduction is permitted which does not comply with these terms.
*Correspondence: Stephen S. Kelley and Li Xiao, Department of Forest Biomaterials, North Carolina State University, Raleigh, NC 27606, USA e-mail:c3NrZWxsZXlAbmNzdS5lZHU=;bHhpYW8zQG5jc3UuZWR1