- Key Laboratory of Resource Biology and Biotechnology in Western China, Department of Life Science, Northwest University, Xi'an, China
Background: Oxytropis ochrocephala Bunge, an indigenous locoweed species in China, poses great threats to livestock on grasslands. There is a need for further genetic study in the plants per se, for understanding the basis of its acclimation mechanism in various unfavorable environmental conditions and to implement effective control measures. Quantitative real-time reverse transcription-polymerase chain reaction (qRT-PCR) is the most commonly used method for gene expression analysis. To facilitate gene expression studies and obtain more accurate qRT-PCR data, normalization relative to stable reference genes is required. The aim of this study was to select the most stable reference genes for transcriptional analysis in O. ochrocephala.
Results: We selected 12 candidate reference genes, 18S ribosomal RNA (18S RNA), actin2/7 (ACT7), β-actin (ACTB), actin101 (ACT101), actin11 (ACT11), β-tubulin (TUB), α-tubulin (TUA), glyceraldehyde-3-phosphate dehydrogenase-1 (GAPDH1), GAPDH2, metallothionein-like protein (MET), fructose-bisphosphate aldolase (FBA) and histone H3 (HIS), from the transcriptome datasets of O. ochrocephala and determined the suitability by analyzing their expression levels when exposed to a range of abiotic stress conditions. By employing software packages including geNorm, NormFinder and BestKeeper, HIS, ACT7, and ACT101 were assessed as the most suitable set for normalization in all samples. When normalized with the most stable reference genes, the expression patterns of the three target genes were in accordance with those in the transcriptome data, indicating that the reference genes selected in this study are suitable.
Conclusions: The study provided appropriate reference genes for accurate normalization in qRT-PCR analysis in O. ochrocephala and emphasized the importance of validating reference genes for gene expression analysis under specific experimental condition. The usage of inappropriate reference gene would cause misinterpretation.
Introduction
Oxytropis ochrocephala Bunge (Fabaceae), a perennial grass species, grows aggressively on the grassland of northwestern China. It is the most widespread locoweed (the collective name for toxic Oxytropis and Astragalus species) in China. Locoweeds contain an indolizidine alkaloid swainsonine, an α-mannosidase inhibitor which causes over-accumulation of mannose rich oligosaccharide in the lysosomes and impairs the neural system of livestock, which has long been recognized as the principle for intoxication in animals (Ralphs et al., 2008). Although it has been proved that swainsonine is produced by its symbiotic fungal endophytes, the plants per se also have a great impact on the production of swainsonine (Pryor et al., 2009). Cook et al. (2013) observed that plants offer the endophytes nutrients, hormones and some other signals in influencing its capacity to produce the toxin. In addition, O. ochrocephala grows in high-altitude areas with deficient water, high soil saline content, and extreme low environmental temperature, indicating that the plant has the ability to cope with unfavorable environmental stresses. In order to better understand the role of the plant in production of swainsonine, the research on its stress response mechanism to harsh environment conditions is needed. Currently, genetic information of locoweeds for molecular biology research is limited in the public databases, which makes it more difficult for further in-depth research. A recently EST dataset of a suppressive subtraction cDNA library enriched in genes from two temperate Oxytropis species was made available in NCBI, but it only has 1245 ESTs and thus there is a need for further exploring the genetic information in Oxytropis (Chung et al., 2004; Archambault and Strömvik, 2011).
RNA sequencing (RNA-Seq), with the rapid development of the next-generation sequencing technology, has been applied prevalently on analyzing the transcriptomes of various species for a range of purposes (Wang et al., 2009; Li et al., 2015a; Stone and Storchova, 2015). The main outcome of RNA-Seq data is the identification of differentially expressed genes, while it was also used to search for reference genes. Any gene with a minimal expression level variation in every analyzed sample is considered as candidate reference gene. Meanwhile, qRT-PCR is commonly used to determine expression levels of genes and to validate transcriptomic data (Andersen et al., 2004; Caldana et al., 2007). For accurate qRT-PCR evaluation, it is necessary to select suitable reference genes as internal control under different experimental conditions because the starting material, RNA extraction, RT-PCR efficiency, and qRT-PCR efficiency can vary among experiments. In addition, gene expression can be highly tissue-specific and often varies based on the physiological status of the organism or experimental treatments. Thus, it is essential for an optimal reference gene or combination of genes for the specific experiment to normalize gene expression data.
The growing acquisition of plant genomes and transcriptomes provide a high-throughput approach to identify a set of reference genes. In the study of Czechowski et al. (2005), based on the Arabidopsis genome, hundreds of genes with stable expression levels were identified. Yang et al. (2014b) used a similar approach to mine 40 genes as candidate reference genes from the transcriptome datasets of Brassica napus and 14 reference genes were selected for further analysis with qRT-PCR in different tissues and under different experimental treatments. Reference genes are usually cellular maintenance genes, which regulate basic cellular functions and components, such as Actin (Nakayama et al., 2014), Tubulin (Zhang et al., 2013), GAPDH (Ling et al., 2014), 18S RNA (Yang et al., 2014a), Ubiquitin (UBQ) (Galeano et al., 2014), Histone (Wang et al., 2014; Yan et al., 2014). Recent studies have indicated that there is no universal reference gene for all experimental conditions and systematic expression stability analysis of reference genes under specific experimental conditions is necessary (Jian et al., 2008).
A few statistical algorithms have been developed to identify which reference gene(s) is (are) suitable for normalization under a given experiment condition for a specific species. These programs have been successfully employed to determine the stability of reference gene expression and identify stable reference genes for various plant species, such as tall fescue (Yang et al., 2015), moss (Li et al., 2015b), switchgrass (Gimeno et al., 2014), and Caragana korshinskii (Yang et al., 2014c). Because of the different algorithms, the rankings provided by different programs are often not completely identical, and Jacob et al. (2013) recommend that more than two algorithms should be used for reference gene stability evaluation.
In this study, we selected 12 candidate reference genes based on the abiotic transcriptome datasets of O. ochrocephala by RNA-Seq (unpublished data) and further validated the expression stability of these genes by qRT-PCR and evaluated them using popular software packages including geNorm (Vandesompele et al., 2002), NormFinder (Andersen et al., 2004) and BestKeeper (Pfaffl et al., 2004). Three target genes, 1-aminocyclopropane-1-carboxylate oxidase (ACO), calcium binding protein (CaBP) and cinnamate-4-monooxygenase (C4H), were used to validate the effectiveness of the selected reference genes. The three genes ACO, CaBP, and C4H represent the characteristics of stress-responsive genes in plants. Under drought stress, ACO gene is up-regulated to increase the production of ethylene which is a key plant hormone to regulate plant growth and development (Chen et al., 2005). CaBP gene is a member of the calcium signaling system, which regulates an important pathway in plant cold acclimation (Yang et al., 2010). C4H is involved in the accumulation of soluble phenylpropanoids which act as the radical scavenger, helping to reduce cell oxidative damage caused by biotic and abiotic stress (Hemm et al., 2004). This is the first report which has determined the reference genes in O. ochrocephala and the other Oxytropis species. In particular, this work provides the basis for further research in exploring the mechanism of adaption O. ochrocephala to stress environments.
Materials and Methods
Plant Materials and Stress Treatments
Seeds of O. ochrocephala were collected from Haiyuan, Ningxia province. Seeds were pre-treated with concentrated sulphuric acid for 8 min, then washed three times with distilled water and placed onto wet filter papers in Petri dishes for germination. Germinated seeds were kept growing until cotyledons emerged and then transferred into individual pots (5 × 5 × 6 cm) filled with sand and peat (1:1), and grown in the growth chamber under controlled conditions at a photo flux density of 300 μmol m−2s−1 (14/10 h, day/night period), at a relative humidity of 55–60%, and a temperature of 25 ± 2°C (Tang et al., 2007). Each group of 10 pots of 4-week-old seedlings with consensus growth status were selected and treated by drought, cold and salt stress, relatively. Three biological replicates were included for each treatment and control.
For drought treatment, 20% PEG-6000 solution (w/v, polyethylene glycol, Sangon, China) was applied to irrigate the plants in the soil every 4 h, simulating osmotic stress which is equivalent to −0.54 MPa, according to Jones and Gorham (1983); For cold treatment, seedlings were transferred into another growth chamber at 4°C with the rest growing conditions being the same. For salt treatment, 150 mM NaCl (Sangon, China) solution was applied to irrigate the plants. Entire seedlings were collected at different times (0, 3, 6, 12 h) from the onset of treatment and immediately frozen in liquid nitrogen for RNA extraction.
Total RNA Extraction and cDNA Synthesis
Total RNA was extracted according to the modified CTAB method (Gasic et al., 2004). To eliminate DNA contamination, total RNA was DNase I (Ambion, USA) treated and purified according to the manufacturer's protocol. The integrity of total RNA samples were verified by 1.2% (w/v) agarose gel electrophoresis, and the quantity and quality of RNA samples were measured with the NanoDrop ND-1000 Spectrophotometer (NanoDrop Technologies, USA).
In order to perform qRT-PCR, cDNA was synthesized by reverse transcription using 2.5 μg total RNA in a 20 μl reaction volume according to the manufacturer's instructions (Thermo Scientific, USA). The cDNA was diluted 10-fold with nuclease-free water for qRT-PCR.
Transcriptome Data Mining for Candidate Reference Genes
We performed transcriptome sequencing of O. ochrocephala using Illumina paired-end sequencing technology on an Illumina Hi-Seq™ 2000 platform for the four samples (control, drought, cold and salt). After assembly and annotation, a software package, RSEM (RNA-Seq by Expectation Maximization) (Li and Dewey, 2011), was employed to analyze the read counts, which were then converted to fragments Kilobase of exon model per millon mapped reads (FPKM values), a commonly accepted estimate for the expression level of unigenes (Trapnell et al., 2010). To estimate expression stability of each gene, the following indices of FPKM values, including mean expression value (MV), standard deviation (SD) and coefficient of variation (CV) value (dividing SD by MV) were calculated according to the methodology described by de Jonge et al. (2007). Genes with lower CV values were considered to be stably expressed and the cut-off CV value was set as lower than 0.3, suggested by Czechowski et al. (2005) and Narsai et al. (2010).
Twelve candidate reference genes were selected based on gene annotation to NR database. Sequences of the candidate reference genes were used to design primers using Primer5 software. The criteria for primer design were set as follows: melting temperature (Tm) in a range of 55–60°C, primer lengths of 18–24 bp, GC contents of 45–55% and amplicon lengths of 95–282 bp.
RT-PCR and qRT-PCR Analysis
To verify the specificity of all the primer sets, PCR was performed using pooled cDNA as templates, and the PCR products were examined by 2% (w/v) agarose gel electrophoresis. The amplicons should appear as a single band with the correct size. To confirm that the PCR product did not contain multiple amplicons of the same size, which were amplified from possibly existing several genes in a gene family, eight individual PCRs were performed for each gene and the products were sequenced. Then the genes were further analyzed by qRT-PCR.
qRT-PCR reactions were performed in 20 μl system on a Bio-Rad CFX96 Real-Time PCR system (Bio-Rad, USA) with a reaction contained 10 μl of FastStart Universal SYBR GreenMaster (Roche, Germany), 2 μl of diluted cDNA template, 1 μl of each primer (10 μM). For each gene, a no template control (NTC) was included using water instead of cDNA as template. The qRT-PCR reactions were conducted following the fast thermal cycles: 95°C for 10 min, 40 cycles at 95°C for 30 s, 58°C for 30 s, and 72°C for 30 s. After 40 cycles, the dissociation curve was performed to confirm the specificity of each primer again by heating up the product from 60°C to 95°C. The Rn (normalized reporter) threshold was automatically selected to obtain the cycle threshold (Ct) values. The final Ct value of each sample was the mean of three biological replicates and three technical replicates. The mean amplification efficiency of each primer pair was checked by the LinRegPCR program (Ruijter et al., 2009).
Data Analysis of Gene Expression Stability
Three different types of programs, geNorm (version 3.5), BestKeeper and NormFinder were used to rank the stability of the selected reference genes across all the experimental sets (Xia et al., 2014; Yang et al., 2014c; Galli et al., 2015). For geNorm and NormFinder, the raw Ct value of each gene was converted into the relative quantities by the formula 2−ΔCt (ΔCt = each corresponding Ct value - lowest Ct value). For BestKeeper, the Ct value was used to calculate the standard deviation (SD) and the coefficient of variation (CV). The most stable gene exhibits the lowest CV ± SD value. Genes with SD value greater than 1 were considered to be unacceptable as reference genes. The pairwise variation (Vn/Vn+1) between two sequential normalization factors was obtained by geNorm software for determine the optimal number of reference genes needed to normalize. The recommended cut-off threshold is 0.15, below which an additional control gene is not required for normalization (Vandesompele et al., 2002). The overall recommended rankings of the best reference genes were obtained using the results of three algorithms.
Validation of Reference Genes
The expression pattern of three target genes ACO, CaBP, and C4H were analyzed using the most stable and least stable reference genes after normalization across three experimental sets, drought stress (for ACO), cold stress (for CaBP) and salt stress (for C4H). To validate the results, the expression levels of the target genes in qRT-PCR were compared with the FPKM values in RNA-seq data in each sample (Figure S2). The sequences of these genes were obtained from the transcriptome data (Table S3). The amplification efficiencies of the target genes were also estimated by the LinRegPCR program. The average Ct value was calculated from three biological and technical replicates and used for relative expression analyses. The relative expression data were calculated according to the 2−ΔΔCT method and presented as fold change (Livak and Schmittgen, 2001).
Results
Identification of Putative Reference Genes Based on Transcriptome Datasets
Based on the FPKM value, we selected 12 genes with low CV values (below 0.3) (18S, ACT101, ACTB, ACT7, ACT11, GAPDH1, GAPDH2, TUB, TUA, MET, FBA, and HIS) and these genes have been reported as stable genes in other species. The sequences of the 12 reference genes were obtained from the transcriptome data. Gene symbol, gene length and function description were shown in Table 1. We used full length unigene sequences from transcriptome data to design the specific primers for qRT-PCR. Primer pairs were designed to avoid the conserved domains or at the untranslated region (UTR) of each gene. For the amplified sequences of these homolog genes, nucleotide identities were relatively lower, around 30–50% range. Amino acid identities among homologous genes were less than 30%, suggesting less functional identity of the amplified fragment (Table S1).
Expression Profiles of Reference Genes
The primer specificities were confirmed by agarose gel electrophoresis, sequencing and melting curves, which showed the single amplicon of the expected size and the single peak melting curves (Figure S1, sequencing result not shown). The qRT-PCR products ranged from 95 to 282 bp. The amplification efficiencies of these reference genes were spanning from 1.8 to 2, and the linear R2 (correlation coefficients) ranged from 0.995 to 0.999. The primer sequences and relevant amplification information were presented in Table 2.
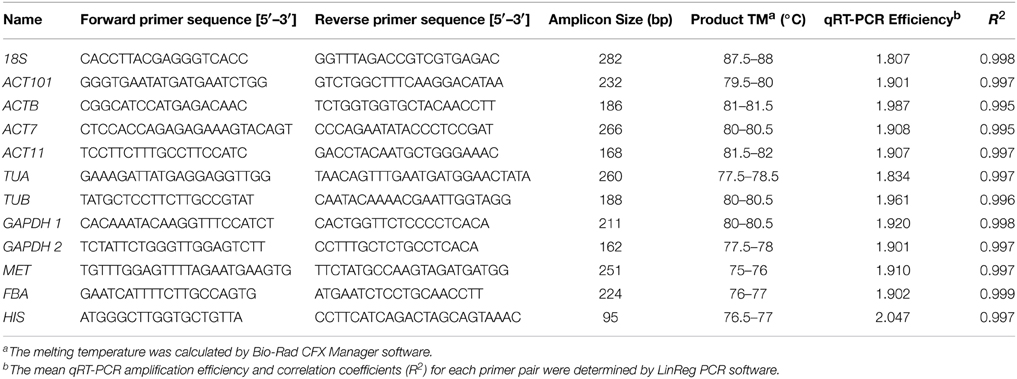
Table 2. List of primer sequences of the candidate reference genes and their amplification efficiencies.
To quantify overall differences in expression levels of the 12 reference genes, the median Ct value for each gene under total groups was calculated. The mean Ct values of the 12 candidate reference genes revealed a minimum of 15.93 ± 2.56 (18S) (mean ± SD) and a maximum of 30.34 ± 2.20 (MET) for highest and lowest expression levels, which showed the expression level of MET was almost 44-fold lower than 18S. The genes with higher SD of Ct values indicated more variable expression compared to these with lower SD. HIS showed the smallest variation in gene expression (23.37 ± 0.79), while 18S with the most variable levels of expression (Figure 1).
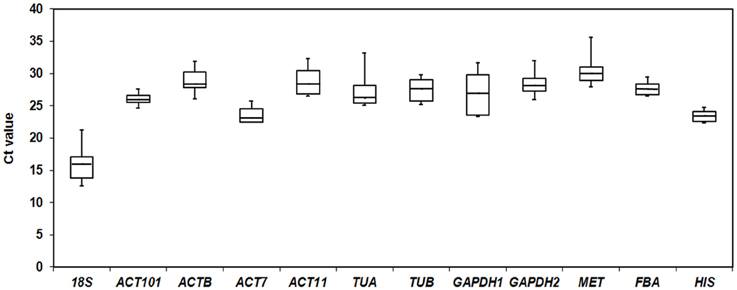
Figure 1. Cycle threshold (Ct) values of 12 candidate reference genes across all samples. The final Ct value of each sample was the mean of three biological and technical replicates. Box graph indicates the interquartile range. A line across the box showed as the median. Lower and upper dashes represent the maximum and minimum values.
geNorm Analysis
Average pairwise expression ratios (M) of the 12 reference genes were evaluated using software geNorm. M value is negatively correlated with gene stability, and below 1.5 is considered to be stable expression (Hellemans et al., 2007). As determined by the geNorm, ACT101, and HIS were the most stable reference genes in total samples. In contrast, TUA and 18s were the least stable reference genes. Under each subset of the treatment, the two best reference genes in drought stress were ACT101 and HIS with the lowest M value. The most preferred genes for normalization in the cold stress were ACT7 and HIS. As for the salt stress, GAPDH2 and HIS were the most stable reference genes. In general, HIS was the most stable gene in total or single treatment (Figure 2).
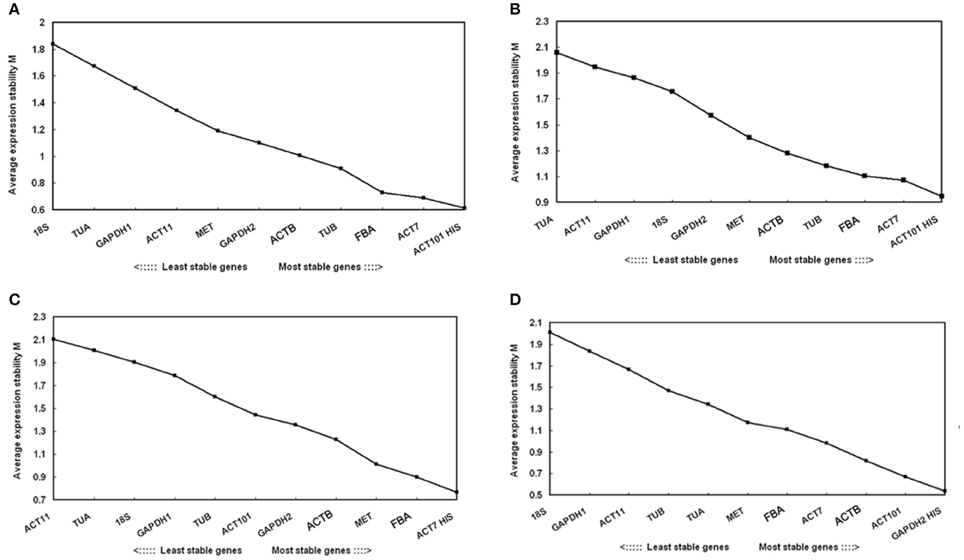
Figure 2. Expression stability values (M) of 12 candidate reference genes calculated by geNorm. Lower M values indicate more stable expression. Ranking of the gene expression stability was performed in all the samples and each abiotic stress samples. The least stable genes were on the left and the most stable genes on the right. (A) All tested samples (B) Drought stress (C) Cold stress (D) Salt stress.
The optimal number of the reference genes required for accurate normalization was determined by pairwise variation (Vn/Vn+1). In the subsets of drought and cold stress, the V2/3 value was below 0.15 (0.144 and 0.107, respectively), which suggested that two reference genes should be used for normalization. In the salt treatment subset, three reference genes were sufficient for accurate normalization, as the V3/4 value was lower than 0.15. When total samples were considered, the pairwise variationV7/8 value was the lowest (0.165) but still above 0.15 (Figure 3).
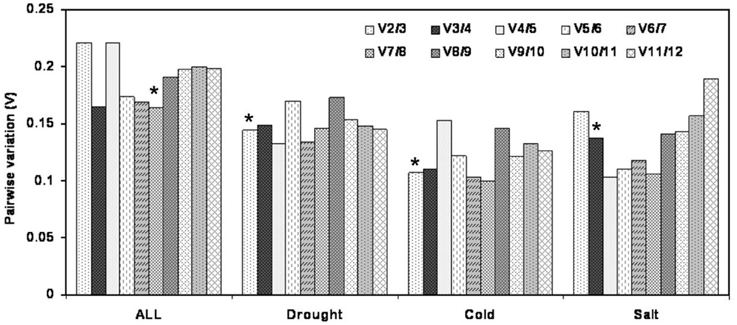
Figure 3. Pairwise variation (V) of 12 candidate reference genes calculated by geNorm to determine the optimal number of reference genes for accurate normalization. Asterisk indicates the optimal number of reference genes required for normalization.
NormFinder Analysis
NormFinder ranks genes based on the stability value for each reference gene. More stable gene expression has the lower stability value. In the subset of drought stress, TUB and ACTB were the most stable. In cold stress, HIS and ACT101 were the most stable. In salt stress, the top three stably expressed genes were GAPDH2, ACT101, and HIS. When evaluated the total experimental samples, ACT7 and FBA were the top ranked genes (Table 3). In contrast, TUA was the least stable reference gene under drought stress, ACT11 was the least stable under cold stress and 18S was the least stably expressed gene under salt stress. The rank in NormFinder was slightly different from that in geNorm. Genes considered as the most stable by geNorm (ACT101 and HIS) ranked third and forth by NormFinder, respectively.
BestKeeper Analysis
The BestKeeper program determines the stability ranking of the reference genes based on the CV ± SD values. In the drought stress set, HIS (2.17 ± 0.51) and ACT101 (2.55 ± 0.67) with lowest CV ± SD values were identified as stable genes, which were consistent with the results of geNorm. In the cold stress set, only ACT7 and HIS showed SD < 1, which were considered as the most stable genes. In the salt stress treatment, HIS (2.24 ± 0.51), GAPDH2 (3.13 ± 0.85), and ACTB (3.26 ± 0.90) were identified as the best reference genes for normalization (Table 4).
Reference Gene Validation
Data generated by the three algorithms across different experimental sets were further compared (Table 5). The best and worst ranked candidate reference genes were selected for normalizing three target genes ACO, CaBP, and C4H under different experimental conditions. Under drought treatment, the expression level of ACO was up-regulated 2.8-fold when normalized using the two stable genes (HIS and ACT101), while the expression level was overestimated (232-fold) when normalized using the least stable combination (TUA and 18S). When HIS and ACT101 were used as the reference genes, the expression level of ACO was generally identified with the expression profile in RNA-seq (Figure S2). In the same way, under cold stress, the expression level of CaBP was 3.4-fold higher than control when using the most stable reference genes (ACT7 and HIS) as the internal control. By contrast, the expression pattern of CaBP was down-regulated when the least stable genes were used (ACT11 and TUA). Based on the result of geNorm, three reference genes were needed for normalization under salt stress condition. The stable gene combination (GAPDH2, HIS, and ACT101) was used to analyze the transcript abundance of C4H under salt stress. C4H was 3.4-fold up-regulated evaluated by the stable genes and there was a bias when the worst genes (18S+GAPDH1+ACT11) were used for normalization (Figure 4).
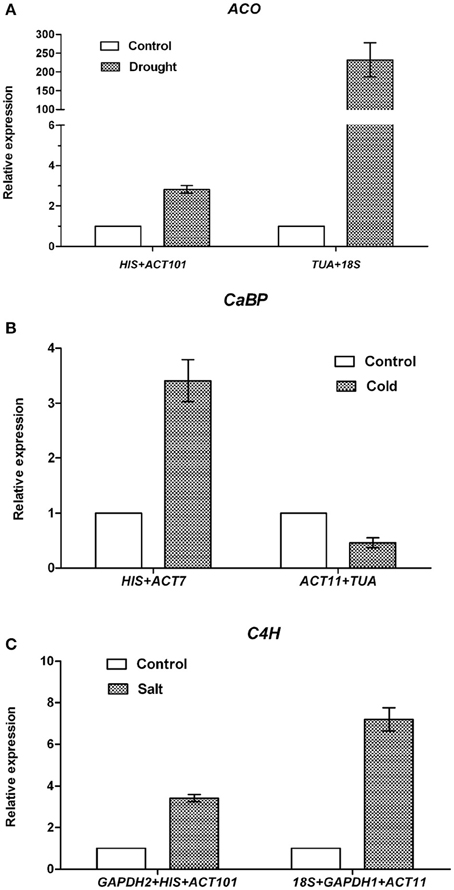
Figure 4. Normalized expression level of ACO, CaBP and C4H. (A) Relative quantification of ACO expression using by the best stable genes (ACT101 and HIS), and the least stable genes (TUA and 18S) under drought treatment. (B) Relative quantification of CaBP expression by the best stable genes (HIS and ACT7), and the least stable genes (ACT11 and TUA) under cold treatment. (C) Relative quantification of C4H expression by the best stable genes (GAPDH2, HIS, and ACT101), and the least stable genes (18S, GAPDH1, and ACT11) under salt treatment. The average Ct value was calculated from three biological and technical replicates and used for relative expression analyses. Error bars indicate standard errors.
Discussion
An accurate and careful expression analysis of stress related genes can bring valid information to reveal more details about the transcriptional networks in stress response process. Therefore, it is required that any transcriptional study be based on accurate selection of suitable reference genes for normalization to ensure the accuracy of the analysis. To date, few studies have undertaken a comparison and selection of reference genes in O. ochrocephala or even in any Oxytropis species. As a result, this hinders relevant work in characterization of genes involved in stress responses in O. ochrocephala. We had performed the first large-scale transcriptome data for O. ochrocephala (unpublished), consisting of 118,596 unigenes, which served as the source of the reference gene selection. The study demonstrated that the RNA-Seq data are useful source for candidate reference genes screening and represented an important strategy for large-scale reference gene selection for non-model plants. By using three different computer programs, the 12 candidate reference genes exhibited various performance in their stability in O. ochrocephala.
Amongst protein-coding genes, HIS was indicated as a good potential internal control under drought treatment. This result was consistent with a previous study in strawberry, which confirmed that HIS gene was the most stable reference genes under osmotic stresses and salt stress (Galli et al., 2015). However, in liverwort, as evaluated by geNorm and NormFinder, HIS gene showed poor expression stability except for the group involving hormone treatment (Saint-Marcoux et al., 2015). The result demonstrates that in specific species reference genes are regulated differently, each species may have its own stably expressed genes which should be determined for each subset of experimental conditions. MET and FBA were relatively uncommon reference genes compared with the frequently used ones (commonly used). Several reports have indicated that the expression levels of traditional reference genes vary considerably and the newly discovered reference genes perform better than the traditional reference genes in Arabidopsis (Dekkers et al., 2012), Brassica napus L. (Yang et al., 2014b), and tea (Hao et al., 2014). While in this study, neither FBA nor MET ranked the top three most stable genes under tested experimental conditions. The commonly used reference genes outperformed the novel ones in O. ochrocephala. Moreover, based on transcriptome datasets, more novel and stable reference gene could be identified from other O. ochrocephala samples in the further study.
The actin gene(s), the most frequently used reference gene (Zhong et al., 2011; Galli et al., 2013), also showed poor consistency in Brassica napus L, soybean and peach (Tong et al., 2009; Ma et al., 2013; Yang et al., 2014b). It is notable that among the four actin genes tested in our study, ACT101 and ACT2/7 ranked head of ACTB and ACT11. Similarly, gene expression levels varied between the homologous genes TUA and TUB, and between GAPDH1 and GAPDH2. In the present study, the commonly used reference gene TUA and GAPDH1 displayed variable expression pattern but TUB and GAPDH2 were more stable in each subset. This result was consistent with an earlier studies in soybean, in that the expression of Actin2/7 was also more stable than Actin11 in different tissues (Jian et al., 2008) and similarly in rice, UBQ5 was the most stably expressed, whereas UBQ10 exhibited the least stable expression in a given set of tissue samples (Jain et al., 2006). These results suggest that the expression pattern and stability of members from the same gene family may be variable in the same experiment. For these homolog genes, the fluctuated Ct values suggest different levels of expression and they have the same CDS (coding sequence) from different gene loci. This highlights the need to ensure primer specificity at the outset of any gene expression experiment because of the similarity of coding sequence in the genes within the same gene family. In our experiment, primer sets were designed in the UTR to avoid the conserved domain, which enabled the gene specific amplification (Table S2).
Our results indicated that 18S was the least stable reference gene in almost all the experimental condition. The difference of Ct between 18S and HIS (stable gene) was 7, suggesting over 20-fold of gene expression between the two genes. When 18S was validated as a reference gene for normalization the target genes ACO, the expression pattern was obviously overestimated, consistent with findings in broomrape (González-Verdejo et al., 2008) and rice (Bevitori et al., 2014). According to Jain et al. (2006), the reason why 18S is not suitable for normalization in real-time PCR analysis is the excessive high expression level compared with the target genes, which make it difficult to analyze the qRT-PCR data by subtracting the baseline. Nonetheless, in the absence of better choices, 18S is still frequently used as the reference genes for normalizing gene expression because it is independent of developmental stages and external stimuli (Gantasala et al., 2013; Huang et al., 2014).
In order to avoid the erroneous data that may be triggered by using single reference gene, normalization with multiple reference genes is becoming the common way. To evaluate the optimal number of genes required for normalization, geNorm showed V7/8 was the lowest value in total treatment samples. However, using seven reference genes to normalize is not feasible in practice. The V value (pairwise variation) threshold 0.15 should not be considered as an absolute value but rather a suggested one. Several reports have even reported higher V values in some species (De Ketelaere et al., 2006; Wan et al., 2010) and it is dependent on the consideration of the research purpose. Vandesompele et al. (2002) proposed that at least three genes are required for more reliable normalization. Considering these results, three top ranked reference genes HIS, ACT7, and ACT101 were appropriate for gene expression normalization under drought, cold and salt conditions. Besides, using different reference genes may lead to opposite or misunderstanding results in normalization of target genes.
To conclude, the current work presented here shows that the transcriptome data provide an effective approach to identify a set of reference genes and the reference genes, HIS, ACT7, and ACT101, were stably expressed under abiotic stress treatments, which should facilitate future studies on gene expression in O. ochrocephala.
Author Contributions
Designed and performed and the experiments: HZ, LW; Analyzed data and wrote the paper: HZ, YF; Conducted the experiments: YF, WH; Contributed reagents/materials/fund support: WH, YW.
Conflict of Interest Statement
The authors declare that the research was conducted in the absence of any commercial or financial relationships that could be construed as a potential conflict of interest.
Acknowledgments
This work was supported by Special Fund for Agro-scientific Research in The Public Interest (Grant No. 201203062), Natural Science Foundation of China (Grant No. 31402133), Special Fund for Scientific Research from Shaanxi Provincial Department of Education (Grant No. 14JK1725), and Key Laboratory Fund for Scientific Research from Shaanxi Provincial Department of Education (Grant No. 14JS099).
Supplementary Material
The Supplementary Material for this article can be found online at: http://journal.frontiersin.org/article/10.3389/fpls.2015.00475
References
Andersen, C. L., Jensen, J. L., and Ørntoft, T. F. (2004). Normalization of real-time quantitative reverse transcription-PCR data: a model-based variance estimation approach to identify genes suited for normalization, applied to bladder and colon cancer data sets. Cancer Res. 64, 5245–5250. doi: 10.1158/0008-5472.CAN-04-0496
Archambault, A., and Strömvik, M. V. (2011). PR-10, defensin and cold dehydrin genes are among those over expressed in Oxytropis (Fabaceae) species adapted to the arctic. Funct. Integr. Genomics 11, 497–505. doi: 10.1007/s10142-011-0223-6
Bevitori, R., Oliveira, M. B., Grossi-de-Sa, M. F., Lanna, A. C., da Silveira, R. D., and Petrofeza, S. (2014). Selection of optimized candidate reference genes for qRT-PCR normalization in rice (Oryza sativa L.) during Magnaporthe oryzae infection and drought. Genet. Mol. Res. 13, 9795–9805. doi: 10.4238/2014.November.27.7
Caldana, C., Scheible, W. R., Mueller-Roeber, B., and Ruzicic, S. (2007). A quantitative RT-PCR platform for high-throughput expression profiling of 2500 rice transcription factors. Plant Methods 3:7. doi: 10.1186/1746-4811-3-7
Chen, Y. F., Etheridge, N., and Schaller, G. E. (2005). Ethylene signal transduction. Ann. Bot. 95, 901–915. doi: 10.1093/aob/mci100
Chung, M., Gelembiuk, G., and Givnish, T. J. (2004). Population genetics and phylogeography of endangered Oxytropis campestris var. chartacea and relatives: arctic-alpine disjuncts in eastern North America. Mol. Ecol. 13, 3657–3673. doi: 10.1111/j.1365-294X.2004.02360.x
Cook, D., Grum, D. S., Gardner, D. R., Welch, K. D., and Pfister, J. A. (2013). Influence of endophyte genotype on swainsonine concentrations in Oxytropis sericea. Toxicon 61, 105–111. doi: 10.1016/j.toxicon.2012.10.018
Czechowski, T., Stitt, M., Altmann, T., Udvardi, M. K., and Scheible, W. R. (2005). Genome-wide identification and testing of superior reference genes for transcript normalization in Arabidopsis. Plant Physiol. 139, 5–17. doi: 10.1104/pp.105.063743
de Jonge, H. J., Fehrmann, R. S., de Bont, E. S., Hofstra, R. M., Gerbens, F., Kamps, W. A., et al. (2007). Evidence based selection of housekeeping genes. PLoS ONE 2:e898. doi: 10.1371/journal.pone.0000898
De Ketelaere, A., Goossens, K., Peelman, L., and Burvenich, C. (2006). Technical note: validation of internal control genes for gene expression analysis in bovine polymorphonuclear leukocytes. J. Dairy Sci. 89, 4066–4069. doi: 10.3168/jds.S0022-0302(06)72450-X
Dekkers, B. J., Willems, L., Bassel, G. W., van Bolderen-Veldkamp, R. P., Ligterink, W., Hilhorst, H. W., et al. (2012). Identification of reference genes for RT–Qpcr expression analysis in Arabidopsis and tomato seeds. Plant Cell Physiol. 53, 28–37. doi: 10.1093/pcp/pcr113
Galeano, E., Vasconcelos, T. S., Ramiro, D. A., Martin, V. D. F. D., and Carrer, H. (2014). Identification and validation of quantitative real-time reverse transcription PCR reference genes for gene expression analysis in teak (Tectona grandis L.f.). BMC Res. Notes 7:464. doi: 10.1186/1756-0500-7-464
Galli, V., Borowski, J. M., Perin, E. C., Messias, R. D. S., Labonde, J., Pereira, I. D. S., et al. (2015). Validation of reference genes for accurate normalization of gene expression for real time-quantitative PCR in strawberry fruits using different cultivars and osmotic stresses Gene 554, 205–214. doi: 10.1016/j.gene.2014.10.049
Galli, V., da Silva Messias, R., dos Anjos e Silva, S. D., and Rombaldi, C. V. (2013). Selection of reliable reference genes for quantitative real-time polymerase chain reaction studies in maize grains. Plant Cell Rep. 32, 1869–1877. doi: 10.1007/s00299-013-1499-x
Gantasala, N. P., Papolu, P. K., Thakur, P. K., Kamaraju, D., Sreevathsa, R., and Rao, U. (2013). Selection and validation of reference genes for quantitative gene expression studies by real-time PCR in eggplant (Solanum melongena L). BMC Res. Notes 6:312. doi: 10.1186/1756-0500-6-312
Gasic, K., Hernandez, A., and Korban, S. S. (2004). RNA extraction from different apple tissues rich in polyphenols and polysaccharides for cDNA library construction. Plant Mol. Biol. Rep. 22, 437–438. doi: 10.1007/BF02772687
Gimeno, J., Eattock, N., Van, D. A., and Blumwald, E. (2014). Selection and validation of reference genes for gene expression analysis in switchgrass (Panicum virgatum) using quantitative real-time RT-PCR. PLoS ONE 9:e91474. doi: 10.1371/journal.pone.0091474
González-Verdejo, C. I., Die, J. V., Nadal, S., Jiménez-Marín, A., Moreno, M. T., and Román, B. (2008). Selection of housekeeping genes for normalization by real-time RT–PCR: analysis of Or-MYB1 gene expression in Orobanche ramosa development. Anal. Biochem. 379, 176–181. doi: 10.1016/j.ab.2008.05.003
Hao, X., Horvath, D. P., Chao, W. S., Yang, Y., Wang, X., and Xiao, B. (2014). Identification and evaluation of reliable reference genes for quantitative real-time PCR analysis in Tea Plant (Camellia sinensis (L.) O. Kuntze). Int. J. Mol. Sci. 15, 22155–22172. doi: 10.3390/ijms151222155
Hellemans, J., Mortier, G., De, P. A., Speleman, F., and Vandesompele, J. (2007). qBase relative quantification framework and software for management and automated analysis of real-time quantitative PCR data. Genome Biol. 8:R19. doi: 10.1186/gb-2007-8-2-r19
Hemm, M. R., Rider, S. D., Ogas, J., Murry, D. J., and Chapple, C. (2004). Light induces phenylpropanoid metabolism in Arabidopsis roots. Plant J. 38, 765–778. doi: 10.1111/j.1365-313X.2004.02089.x
Huang, L. K., Yan, H., Jiang, X., Zhang, X., Zhang, Y., Huang, X., et al. (2014). Evaluation of candidate reference genes for normalization of quantitative RT-PCR in switchgrass under various abiotic stress conditions. Bioenergy Res. 7, 1201–1211. doi: 10.1007/s12155-014-9457-1
Jacob, F., Guertler, R., Naim, S., Nixdorf, S., Fedier, A., Hacker, N. F., et al. (2013). Careful selection of reference genes is required for reliable performance of RT-qPCR in human normal and cancer cell lines. PLoS ONE 8:e59180. doi: 10.1371/journal.pone.0059180
Jain, M., Nijhawan, A., Tyagi, A. K., and Khurana, J. P. (2006). Validation of housekeeping genes as internal control for studying gene expression in rice by quantitative real-time PCR. Biochem. Biophys. Res. Commun. 345, 646–651. doi: 10.1016/j.bbrc.2006.04.140
Jian, B., Liu, B., Bi, Y., Hou, W., Wu, C., and Han, T. (2008). Validation of internal control for gene expression study in soybean by quantitative real-time PCR. BMC Mol. Biol. 9:59. doi: 10.1186/1471-2199-9-59
Jones, R. W., and Gorham, J. (1983). “Osmoregulation,” in Physiological Plant Ecology III, eds O. L. Lange, P. S. Nobel, C. B. Osmond, and H. Ziegler (Berlin: Springer-Verlag), 35–58.
Li, B., and Dewey, C. N. (2011). RSEM: accurate transcript quantification from RNA-Seq data with or without a reference genome. BMC Bioinformatics 12:323. doi: 10.1186/1471-2105-12-323
Li, H., Yao, W., Fu, Y., Li, S., and Guo, Q. (2015a). De novo assembly and discovery of genes that are involved in drought tolerance in Tibetan Sophora moorcroftiana. PLoS ONE 10:e111054. doi: 10.1371/journal.pone.0111054
Li, X. S., Zhang, D. Y., Li, H. Y., Gao, B., Yang, H. L., Zhang, Y. M., et al. (2015b). Characterization of reference genes for RT-qPCR in the desert moss Syntrichia caninervis in response to abiotic stress and desiccation/rehydration. Front. Plant Sci. 6:38. doi: 10.3389/fpls.2015.00038
Ling, H., Wu, Q., Guo, J., Xu, L., and Que, Y. (2014). Comprehensive selection of reference genes for gene expression normalization in sugarcane by real time quantitative RT-PCR. PLoS ONE 9:e97469. doi: 10.1371/journal.pone.0097469
Livak, K. J., and Schmittgen, T. D. (2001). Analysis of relative gene expression data using real-time quantitative PCR and the 2-ΔΔCT method. Methods 25, 402–408. doi: 10.1006/meth.2001.1262
Ma, S., Niu, H., Liu, C., Zhang, J., Hou, C., and Wang, D. (2013). Expression stabilities of candidate reference genes for RT-qPCR under different stress conditions in soybean. PLoS ONE 8:e75271. doi: 10.1371/journal.pone.0075271
Nakayama, T. J., Rodrigues, F. A., Neumaier, N., Marcelino-Guimaraes, F. C., Farias, J. R., de Oliveira, M. C., et al. (2014). Reference genes for quantitative real-time polymerase chain reaction studies in soybean plants under hypoxic conditions. Genet. Mol. Res. 13, 860–871. doi: 10.4238/2014.February.13.4
Narsai, R., Ivanova, A., Ng, S., and Whelan, J. (2010). Defining reference genes in Oryza sativa using organ, development, biotic and abiotic transcriptome datasets. BMC Plant Biol. 10:56. doi: 10.1186/1471-2229-10-56
Pfaffl, M. W., Tichopad, A., Prgomet, C., and Neuvians, T. P. (2004). Determination of stable housekeeping genes, differentially regulated target genes and sample integrity: BestKeeper–Excel-based tool using pair-wise correlations. Biotechnol. Lett. 26, 509–515. doi: 10.1023/B:BILE.0000019559.84305.47
Pryor, B., Creamer, R., Shoemaker, R., McLain-Romero, J., and Hambleton, S. (2009). Undifilum, a new genus for endophytic Embellisia oxytropis and parasitic Helminthosporium bornmuelleri on legumes. Botany 87, 178–194. doi: 10.1139/B08-130
Ralphs, M. H., Creamer, R., Baucom, D., Gardner, D. R., Welsh, S. L., Graham, J. D., et al. (2008). Relationship between the endophyte Embellisia spp. and the toxic alkaloid swainsonine in major locoweed species (Astragalus and Oxytropis). J. Chem. Ecol. 34, 32–38. doi: 10.1007/s10886-007-9399-6
Ruijter, J., Ramakers, C., Hoogaars, W., Karlen, Y., Bakker, O., Moorman, A. F. M., et al. (2009). Amplification efficiency: linking baseline and bias in the analysis of quantitative PCR data. Nucleic Acids Res. 37, e45–e45. doi: 10.1093/nar/gkp045
Saint-Marcoux, D., Proust, H., Dolan, L., and Langdale, J. A. (2015). Identification of reference genes for real-time quantitative PCR experiments in the Liverwort Marchantia polymorpha. PLoS ONE 10:e118678. doi: 10.1371/journal.pone.0118678
Stone, J. D., and Storchova, H. (2015). The application of RNA-Seq to the comprehensive analysis of plant mitochondrial transcriptomes. Mol. Genet. Genomics 290, 1–9. doi: 10.1007/s00438-014-0905-6
Tang, T., Zhou, Q. P., and Li, Y. L. (2007). Study on germination condition of Oxytropis ochrocephala Bunge. Seed 9:013. doi: 10.3969/j.issn.1001-4705.2007.09.011
Tong, Z., Gao, Z., Wang, F., Zhou, J., and Zhang, Z. (2009). Selection of reliable reference genes for gene expression studies in peach using real-time PCR. BMC Mol. Biol. 10:71. doi: 10.1186/1471-2199-10-71
Trapnell, C., Williams, B. A., Pertea, G., Mortazavi, A., Kwan, G., van Baren, M. J., et al. (2010). Transcript assembly and quantification by RNA-Seq reveals unannotated transcripts and isoform switching during cell differentiation. Nat. Biotechnol. 28, 511–515. doi: 10.1038/nbt.1621
Vandesompele, J., Preter, K. D., Pattyn, F., Poppe, B., Roy, N. V., Paepe, A. D., et al. (2002). Accurate normalization of real-time quantitative RT-PCR data by geometric averaging of multiple internal control genes. Genome Biol. 3, research0034.1–research0034.11. doi: 10.1186/gb-2002-3-7-research0034
Wan, H., Zhao, Z., Qian, C., Sui, Y., Malik, A. A., and Chen, J. (2010). Selection of appropriate reference genes for gene expression studies by quantitative real-time polymerase chain reaction in cucumber. Anal. Biochem. 399, 257–261. doi: 10.1016/j.ab.2009.12.008
Wang, H. L., Chen, J., Tian, Q., Wang, S., Xia, X., and Yin, W. (2014). Identification and validation of reference genes for Populus euphratica gene expression analysis during abiotic stresses by quantitative real-time PCR. Physiol. Plant. 152, 529–545. doi: 10.1111/ppl.12206
Wang, Z., Gerstein, M., and Snyder, M. (2009). RNA-Seq: a revolutionary tool for transcriptomics. Nat. Rev. Genet. 10, 57–63. doi: 10.1038/nrg2484
Xia, W., Mason, A. S., Xiao, Y., Liu, Z., Yang, Y., Lei, X., et al. (2014). Analysis of multiple transcriptomes of the African oil palm (Elaeis guineensis) to identify reference genes for RT-qPCR. J. Biotechnol. 184, 63–73. doi: 10.1016/j.jbiotec.2014.05.008
Yan, X., Dong, X., Zhang, W., Yin, H., Xiao, H., Chen, P., et al. (2014). Reference gene selection for quantitative real-time PCR normalization in Reaumuria soongorica. PLoS ONE 9:e104124. doi: 10.1371/journal.pone.0104124
Yang, C., Pan, H., Liu, Y., and Zhou, X. (2014a). Selection of reference genes for expression analysis using quantitative real-time PCR in the pea aphid, Acyrthosiphon pisum (Harris) (Hemiptera, Aphidiae). PLoS ONE 9:e110454. doi: 10.1371/journal.pone.0110454
Yang, H., Liu, J., Huang, S., Guo, T., Deng, L., and Hua, W. (2014b). Selection and evaluation of novel reference genes for quantitative reverse transcription PCR (qRT-PCR) based on genome and transcriptome data in Brassica napus L. Gene 538, 113–122. doi: 10.1016/j.gene.2013.12.057
Yang, Q., Yin, J., Li, G., Qi, L., Yang, F., and Wang, R. (2014c). Reference gene selection for qRT-PCR in Caragana korshinskii Kom. under different stress conditions. Mol. Biol. Rep. 41, 2325–2334. doi: 10.1007/s11033-014-3086-9
Yang, T., Chaudhuri, S., Yang, L., Du, L., and Poovaiah, B. (2010). A calcium/calmodulin-regulated member of the receptor-like kinase family confers cold tolerance in plants. J. Biol. Chem. 285, 7119–7126. doi: 10.1074/jbc.M109.035659
Yang, Z. M., Chen, Y., Hu, B. Y., Tan, Z. Q., and Huang, B. T. (2015). Identification and validation of reference genes for quantification of target gene expression with quantitative real-time PCR for tall fescue under four abiotic stresses. PLoS ONE 10:e0119569. doi: 10.1371/journal.pone.0119569
Zhang, L., He, L. L., Fu, Q. T., and Xu, Z. F. (2013). Selection of reliable reference genes for gene expression studies in the biofuel plant Jatropha curcas using real-time quantitative PCR. Int. J. Mol. Sci. 14, 24338–24354. doi: 10.3390/ijms141224338
Zhong, H. Y., Chen, J. W., Li, C. Q., Chen, L., Wu, J. Y., Chen, J. Y., et al. (2011). Selection of reliable reference genes for expression studies by reverse transcription quantitative real-time PCR in litchi under different experimental conditions. Plant Cell Rep. 30, 641–653. doi: 10.1007/s00299-010-0992-8
Keywords: Oxytropis ochrocephala Bunge, reference genes, quantitative real-time PCR, transcriptome, abiotic stress
Citation: Zhuang H, Fu Y, He W, Wang L and Wei Y (2015) Selection of appropriate reference genes for quantitative real-time PCR in Oxytropis ochrocephala Bunge using transcriptome datasets under abiotic stress treatments. Front. Plant Sci. 6:475. doi: 10.3389/fpls.2015.00475
Received: 05 March 2015; Accepted: 15 June 2015;
Published: 30 June 2015.
Edited by:
Keqiang Wu, National Taiwan University, TaiwanReviewed by:
Youxiong Que, Fujian Agriculture and Forestry University, ChinaTsuyoshi Imai, NARO Institute of Fruit Tree Science, Japan
Copyright © 2015 Zhuang, Fu, He, Wang and Wei. This is an open-access article distributed under the terms of the Creative Commons Attribution License (CC BY). The use, distribution or reproduction in other forums is permitted, provided the original author(s) or licensor are credited and that the original publication in this journal is cited, in accordance with accepted academic practice. No use, distribution or reproduction is permitted which does not comply with these terms.
*Correspondence: Yahui Wei, Key Laboratory of Resource Biology and Biotechnology in Western China, Department of Life Science, Northwest University, No. 229 Tai Bai North Road, Xi'an 710069, China,d2VpeWFodWlAbnd1LmVkdS5jbg==