- 1Genome Center, University of California, Davis, Davis, CA, USA
- 2Instituto de Agricultura Sostenible, Consejo Superior Investigaciones Científicas, Córdoba, Spain
- 3Unidad de Hortofruticultura, Centro de Investigación y Tecnología Agroalimentaria de Aragón, Zaragoza, Spain
Almond kernels are a rich source of phytosterols, which are important compounds for human nutrition. The genetic control of phytosterol content has not yet been documented in almond. Association mapping (AM), also known as linkage disequilibrium (LD), was applied to an almond germplasm collection in order to provide new insight into the genetic control of total and individual sterol contents in kernels. Population structure analysis grouped the accessions into two principal groups, the Mediterranean and the non-Mediterranean. There was a strong subpopulation structure with LD decaying with increasing genetic distance, resulting in lower levels of LD between more distant markers. A significant impact of population structure on LD in the almond cultivar groups was observed. The mean r2-value for all intra-chromosomal loci pairs was 0.040, whereas, the r2 for the inter-chromosomal loci pairs was 0.036. For analysis of association between the markers and phenotypic traits five models were tested. The mixed linear model (MLM) approach using co-ancestry values from population structure and kinship estimates (K model) as covariates identified a maximum of 13 significant associations. Most of the associations found appeared to map within the interval where many candidate genes involved in the sterol biosynthesis pathway are predicted in the peach genome. These findings provide a valuable foundation for quality gene identification and molecular marker assisted breeding in almond.
Introduction
Almond is the most important source of nut tree oils worldwide and is well ranked among the oil crops (http://faostat.fao.org). Kernel quality has become an important criterion for selecting modern almond cultivars (Socias i Company et al., 2008). Almond breeding has been until recently focused on selecting self-compatible and late-blooming cultivars with fruits of a high physical quality (Socias i Company et al., 2012). Consequently, very little information on the chemical evaluation of the almond kernel has been reported and the studies on the chemical components of the almond kernel and their variability are scarce (Socias i Company et al., 2008). Incorporation of such parameters in the evaluation of new crosses would be of special relevance in determining the possible commercial and industrial uses of the kernels, since their specific use depends primarily on its chemical composition (Socias i Company et al., 2008). Among the chemical parameters evaluated in recent years, the most important have been fatty acid composition (Kodad et al., 2011), tocopherol content (Kodad et al., 2006), and phytosterol content (Fernández-Cuesta et al., 2012a).
In fruit trees, classical breeding takes a lot of time and effort, especially for field management and observations of field trials (Socias i Company, 1998). The development of DNA-based technologies enables breeders to target specific traits much more effectively than using classical breeding techniques.
Phytosterols or plant sterols are products of the isoprenoid biosynthetic pathway naturally present in plants and occurring exclusively in the cytoplasm. They resemble mammalian cholesterol, both in their chemical structure and their biological function (Piironen et al., 2000). The role of phytosterols in plant growth and developmental processes such as cell division (Lindsey et al., 2003), embryogenesis (Clouse, 2000), anti-inflammatory (Bouic, 2001), and anti-oxidation activities (van Rensburget et al., 2000) is well known. Indeed, plant sterols reduce intestinal cholesterol absorption and, subsequently, LDL cholesterol (Plat and Mensink, 2005), which has been recognized since two decades ago as one of the main risk factors of cardio-vascular diseases, the leading cause of mortality in western countries (Castelli, 1984). Since plant sterols are structurally related to cholesterol and are incorporated into the mixed micelles in the intestinal tract, they are largely recommended to be included routinely in the human diet as they are one of the main mechanisms for cholesterol reduction (Law, 2000). In addition, phytosterol supplementation in humans can decrease significantly the risk of chronic diseases such as cardio-vascular disease, cancer or neurological disorders (Bramley et al., 2000). In almond oil, total phytosterol content represents the sum of the major components such as β-sitosterol (±72%) and Δ5-avenasterol (±15%). Other components represented in minor amounts are campesterol (±2.5%), stigmasterol (±0.8%), Δ7-campesterol (±3%), clerosterol (±1%), sitostanol (± 0.5%), Δ5, 24-stigmastadienol (±0.6%), Δ7-stigmastenol (±1.5%), and Δ7-avenasterol (±1.8%) (Fernández-Cuesta et al., 2012a).
The CITA almond germplasm collection shows a very large variability reflecting the wide genetic diversity of its accessions (≈250 accessions) from all over the world (Espiau et al., 2002). Taking this variability into account, this collection is used as the almond reference collection for the Spanish Plant Genetic Resources Network, the Spanish and European Community Plant Variety Offices. This collection has also been the origin of the CITA almond breeding program, where not only very important agronomical traits, such as late blooming and self-incompatibility, are considered, but also all aspects of almond quality (Socias i Company et al., 2012).
Molecular markers have been used extensively in almond breeding and genetic studies, and several linkage maps have been constructed for specific almond crosses to enhance efficiency of almond breeding programs (Sánchez-Pérez et al., 2007; Font i Forcada et al., 2012; Fernández i Martí et al., 2013). Linkage maps can be useful in identifying quantitative trait loci (QTL) for specific crosses and can be used for studying various traits including fruit quality, diseases resistance and physiological characteristics, among others.
As an alternative to analysis in controlled crosses, association mapping (AM) in unstructured and complex populations is now being largely applied to many crops. This approach relies on the strength of association between genetic markers and phenotype. Thus, it detects and locates genes relative to an existing map of genetic markers (Mackay and Powell, 2007). AM has been successfully applied in mapping genes involved in several traits in different plant species such as, maize (Krill et al., 2010), lettuce (Simko et al., 2009), potato (Pajerowska-Mukhtar et al., 2009), wheat (Breseghello and Sorrells, 2006), but only few studies have been carried out in fruit tree crops, such as peach (Font i Forcada et al., 2013), apple (Cevik et al., 2010), or pear (Oraguzie et al., 2010).
One of the key goals of AM is to detect marker-QTL linkage associations using plant materials routinely developed in breeding programs and that can be deployed through marker-assisted selection (MAS) in subsequent generations of cultivar development. However, AM reduces those limitations and does not require the construction of mapping populations and is performed with germplasm collections or non-structured populations. Additionally, phenotypic information available in germplasm collections might directly been used in linkage disequilibrium (LD) mapping avoiding the time required to produce F1 populations, especially in fruit trees. In general, AM is more suited for organisms with little or no pedigree information, populations with rich allelic diversity and traits with little or no selection history and controlled by many loci with small effects (Oraguzie et al., 2007). All these conditions are common in vegetatively propagated fruit trees, such as almond, with a high level of heterosis and a short breeding history (Socias i Company et al., 2012).
Consequently, the objective of the present study has been to perform the first whole genome AM analysis for phytosterol content in a group of 71 almond accessions to identify the genomic regions associated to this quality trait.
Materials and Methods
Plant Material and DNA Isolation
A collection of 71 almond (Prunus amygdalus Batsch) cultivars encompassing a wide range of geographic origins was used in this study (Table 1). They were selected among the whole almond pool in order to have the most representative Spanish local accessions, including 36 genotypes from all the Spanish growing regions. In addition, cultivars from different breeding programs and some foreign cultivars (USA, France, Greece, Italy, Portugal, Algeria, Argentina, Australia, Bulgaria, Tunisia and Ukraine) were also included in this study. The trees are maintained as living plants grafted on the almond × peach hybrid clonal rootstock INRA GF-677, using standard management practices (Espiau et al., 2002).
Twenty mature fruits were randomly collected from each genotype during two consecutive years. The fruit was considered mature when the mesocarp was fully dry and split along the fruit suture and the peduncle was near to complete abscission. Fruits were cracked and seed coats removed by pouring in warm water (100°C) during 5 min. Blanched kernels were dried until constant weight and ground in an electrical grinder to obtain fine flour. Almond oil was extracted from the almond flour to analyze the phytosterol content (Fernández-Cuesta et al., 2012a).
For DNA extraction, leaf samples were collected from young shoots from the upper part of each tree, frozen immediately in liquid nitrogen, and stored at -20°C. Genomic DNA was isolated following the PowerPlant DNA isolation Kit (MO BIO Laboratories, CA, USA). The DNA was quantified and diluted to 10 ng uL-1 for PCR amplifications.
Phenotypic Evaluation
Phytosterol content was analyzed in two replicates per sample following a previously described procedure for the analysis of free and esterified phytosterols (Fernández-Cuesta et al., 2012a). In short, almond flour to which an internal standard solution of cholesterol 0.1% was added to alkaline hydrolysis with potassium hydroxide 2% followed by phytosterols extraction in hexane:water (1:1.5 by vol.). Phyosterols were then derivatized for 15 min at room temperature using a commercial silylating mixture (Silan-Sterol-1, Panreac Química, Barcelona, Spain) and analyzed by gas chromatography using the analytical conditions reported by Fernández-Cuesta et al. (2012b). Kernel phytosterol content was expressed as milligrams per kilogram of kernel. Theoretical oil phytosterol content, expressed as milligrams per kilogram of kernel oil, was estimated from kernel phytosterol content and kernel oil content using the following formula: oil phytosterol content = (kernel phytosterol content × 100)/oil content.
Genotyping
Forty fluorescently labeled microsatellite (SSR) markers (Table 2) developed in other Prunus species were used to genotype the 71 accessions. These SSR have been chosen because of their high polymorphism in peach, cherry, plum, and almond, and because they represent a wide coverage of the almond genome. PCR conditions were as follow: 1×PCR buffer, 1.5 mM MgCl2, 0.2 mM dNTPs, 0.2 μM of each primer, one unit of Taq DNA Polymerase (Invitrogen, Madrid, Spain) and 20 ng of genomic DNA in a 20 μl final volume. The PCR program consisted in a denaturation for 1 min at 94°C, followed by 35 cycles of 15 s at 94°C, 15 s for the annealing temperatures for the different primers used, and 1 min at 72°C, and a final extension of 2 min at 72°C. Each reaction was repeated and analyzed twice to ensure reproducibility. PCR products were detected using ABI 3130xl Genetic Analyzer and GeneMapper (Applied Biosystems). For capillary electrophoresis detection, forward primers were labeled with 5′-fluorescence dyes PET, NED, VIC and 6-FAM and the size standard was Gene Scan™ 500 Liz® (Applied Biosystems).
Phenotype-Genotype Association Analysis
Genetic parameters such as the number of alleles per locus (A), the effective number of alleles detected per locus (Ae), the observed heterozygosity (Ho), the expected heterozygosity (He), and the Wright's fixation index (F) were obtained for comparing both heterozygozities. All parameters were estimated using the PopGene 1.31 software. Phylogenies were estimated using Neighbor joining algorithm/method (Saitou and Nei, 1987). Neighbor joining analyses were conducted with the PAUP* v.4.0b10 phylogenetics package (Swofford, 2003).
Population structure in our diverse collection of almond cultivars was addressed using the method based on the Bayesian modeling environment implemented in the software STRUCTURE 2.3.2 (Pritchard et al., 2000). The aim of this approach was the identification of population structure by clustering individuals into genetically distinguishable groups on the basis of allele frequencies. The ad hoc statistic ΔK (Evanno et al., 2005) was used to set the number of populations (K). Individual and admixture analyses were performed using STRUCTURE assuming an admixture model where the allelic frequencies were correlated. This method uses a Markov Chain Monte Carlo (MCMC) algorithm to cluster individuals into populations on the basis of multilocus genotype data (Pritchard et al., 2000). A burn-in of 20,000 and 250,000 MCMC replications seemed to be the best fit for our data at K = 3. The analysis was run for K-values ranging from two to ten inferred clusters with 20 independent runs each. The results were displayed graphically in a bar graph/chart. The low frequency alleles (considering MAF ≤ 0.05) were removed. The LD between pairs of multiallelic loci was calculated separately for loci on the same or on a different linkage group (LG), using the r2 coefficient. The statistical r2 gives an indication of both recombination and mutation (Flint-Garcia et al., 2003). The significance level of LD between loci was examined using a permutation test implemented in TASSEL software for multiallelic loci, using the “rapid permutation” option.
AM analyses were measured by using TASSEL 2.1 (Yu and Buckler, 2006). The General Linear Model (GLM, Q) and the Mixed Linear Model (MLM, Q + K) approaches were used to examine association between the phenotypic traits and DNA markers. A structured association approach could correct false associations using Q-matrix of population membership estimates. The mean value of the markers at P < 0.005 was used for determining the significance of marker-trait associations. Significant markers were declared using the Bonferroni procedure at the p < 0.00125 experimental-wide threshold. Alleles with minor frequency (MAF) lower than 5% were removed (Wilson et al., 2004).
Results
Phenotypic Variation in the Almond Germplasm
The phenotypic variability of the phytosterol content and profile was large, reflecting the wide coverage of the almond gene pool reached by the 71 cultivars studied. The distribution for total phytosterol content was left-skewed, with a range from 2776.5 to 1125.6 mg kg−1 and a mean of 1882.5 mg kg−1. Most phytosterol content traits showed a normal distribution when evaluating their frequency, indicating that this subset was not biased (Table 3). These results remark the importance of the genetic background of each accession for the phenotypic profile of its nuts.
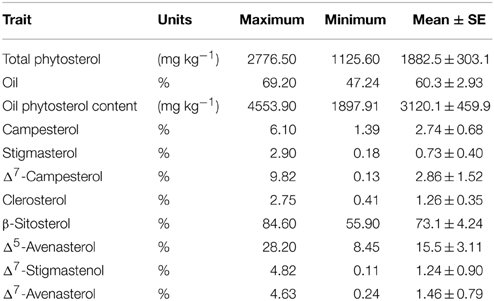
Table 3. Units, minimum, maximum, and mean values for the phytosterol traits evaluated in 71 almond cultivars (average of 2 years of study).
SSR Analysis of the Accessions
Amplification of the 40 SSR loci was successful in all the almond genotypes, producing well-defined and reproducible bands. The primers produced a total of 501 different alleles, with an average of 13.9 alleles per locus, with sizes from 86 to 302 bp. The marker EPDCU5100 showed only two alleles whereas the marker CPPCT053 produced 23 different alleles (Table 2). All primers produced a maximum of two bands per genotype, according to the diploid level of almond. The observed heterozygosity ranged from 0.24 (BPPCT030) to 0.94 (CPPCT040), with an average of 0.66 across all 40 SSRs. Ho and He values were compared with the fixation index (F) which was on the average 0.11, ranging from −0.14 (EPDCU5100) to 0.52 (BPPCT010). The high F-values observed corresponding to high homozygosity, particularly in individuals with only one band, suggests the presence of null alleles (Brookfield, 1996). The F-value was positive in 33 and negative in 7 SSR loci, thus indicating the high level of heterozygosis in the cultivars studied, as it would be expected in an allogamous species such as almond. It was shown that the SSRs developed in other Prunus species such as peach, sweet cherry or Japanese plum, can be effectively used for evolutionary and fingerprinting studies in almond, confirming the high level of synteny within the Prunus species (Mnejja et al., 2010).
Clustering Analysis of the Genotypes
Clustering analysis based on Neighbor-joining essentially allowed the detection of three major clusters of different size, further subdivided in other small clusters (Figure 1). A close relationship could be established for some of these groups with their geographical origin. The first cluster (blue) contained only Spanish accessions (36 in total). This group comprises only cultivars from Spain with representative accessions such as “Marcona,” “Desmayo Largueta,” “Atocha” or “Castilla.” Some new releases from different Spanish breeding programs, such as “Mardía” and “Marta,” were also grouped in this cluster. The accessions from the two Spanish archipelagos (Canary Islands and Majorca), such as “Padre Santo,” “Dura de Tijarafe,” “Vivot,” “Garondès” and “Vinagrilla,” were equally clustered in this first group. The second group (red) appeared to be much diversified, with 20 accessions, including a pool of Mediterranean accessions: two from North Africa (“Constantini” and “Zahaf”), four from France (“Belle d'Aurons,” “Lauranne,” “Ferragnès,” and “Ferraduel”), four from Italy (“Tuono,” “Supernova,” “Rachele,” and “Filippo Ceo”), five from Greece (“Picantilli,” “Tsotouliu,” “Truito,” “Symmetrikji,” and “Phyllis”), and five from Portugal (“Cosa Nova,” “Molar de Fuzeta,” “Carreirinha,” “Raposa,” and “Rameira”). The third group (green) clustered accessions from many different countries, although all of them have in common not being strictly Mediterranean. This group included one accession from Bulgaria (“Exinograd”), three from Crimea (“Yaltinskij,” “Primorskij,” and “Sovietskij”), two from Argentina (“Marcona Argentina” and “Emilito”), one from Australia (“Chellastone”) and eight from the United States (“I.X.L.,” “Texas,” “Thompson,” “Tioga,” “Peerless,” “Tokyo,” “Mono,” and “Tardy Nonpareil”).
Population Structure and Linkage Disequilibrium Analysis
The co-dominant nature of the 40 molecular markers was used to analyze the structure of the populations using a Bayesian approach. The number of subpopulations (K) tested ranged between 2 and 10 (Figure 2) with 20 runs for each K using MCMC replications which showed evident knees at K = 3 (Evanno et al., 2005). The level of partitioning corresponded to a very strong differentiation into two major groups. The first group contained accessions from Mediterranean countries, mostly from Spain, but also from Italy, France, Portugal, Greece and North Africa, while the second group contained accessions from non-Mediterranean countries (including America, Australia and Eastern Europe). The proportion of genotypes assigned to each population was not symmetric, indicating that population structure exists (Pritchard et al., 2000).
Extent of genome-wide LD was evaluated through pairwise comparisons among the 40 marker loci and the 71 almond germplasm accessions studied (Figures 3, 4). After removing low frequency alleles (MAF = 0.05), the results showed a high level of LD up to 20 cM, which dissipated at farther distances. The overall LD for all cultivars was 0.034 in the region from 0 to 10 cM, 0.079 from 10 to 20 cM, 0.036 from 20 to 30 cM, and 0.027 after 30 cM. These results were lower if the cultivars were separated in two different groups, Mediterranean and non-Mediterranean. Thus, the range of LD spaced every 10 cM was 0.061, 0.087, 0.045, and 0.032 for Mediterranean cultivars, and 0.058, 0.079, 0.039, and 0.028 for non-Mediterranean cultivars. A high level of LD up to 20 cM was observed for the whole ensemble of accessions when they were split in a Mediterranean and a non-Mediterranean group. The total r2-value for intra-chromosomal loci pairs was 0.040 and the unlinked markers pairs showed a similar percentage of significant LD in Mediterranean and non-Mediterranean cultivars (values of 0.091 and 0.073, respectively). Regarding the total r2-value for inter-chromosomal loci pairs was 0.036 for the whole ensemble, 0.082 for the Mediterranean accessions, and 0.062 for the non-Mediterranean accessions. The overall level of LD detected was low, which could be mostly likely due to poor marker coverage.
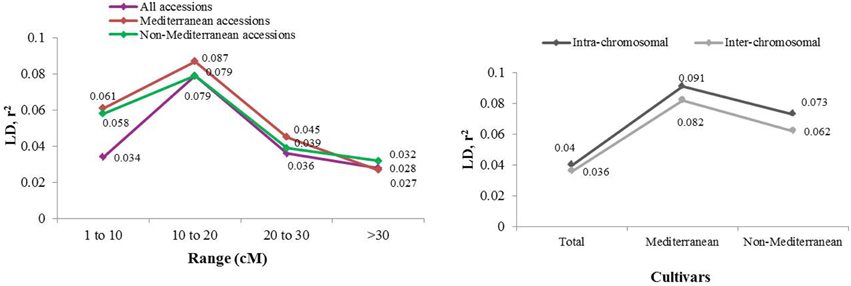
Figure 4. LD based on r2, averaged for map distance classes and germplasm groups based on population structure analysis in the STRUCTURE.
Association Mapping and Allelic Effect
We tested five models in TASSEL to determine associations and also to account for the influence of population structure by comparing their ability to reduce the inflation of false positive associations. The P-values were plotted in a cumulative fashion for each model and the distribution examined. According to Stich et al. (2008) the distribution of P-values ideally should follow a uniform distribution with less deviation from the expected P-values.
The association analysis using the GLM approach (being the naïve model), Q-model and P-model, detected a large number of associations between the markers and phenotypes. The Q-model (GLM with Q-matrix as correction for population structure) showed 30 associations between markers and traits (results not shown). It appears that these models may not have accounted for the heterogeneity of the genetic background, which may have resulted in false positive associations.
The K-model (MLM with K-matrix as correction for population structure) and QK-model (MLM with Q-matrix and K-matrix as correction for population structure) showed good fit for the P-values (P < 0.001), while the other models were characterized by the excess of small P-values (abundance of spurious associations) (Figure 5). These two latter models showed high uniform distribution of P-values.
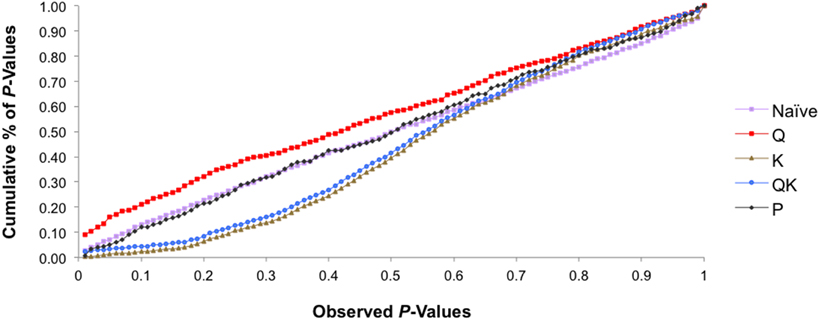
Figure 5. Comparison of different genome wide association study (GWAS) models. Cumulative distribution of P-values was computed from the DNA markers and phenotypes for the different association models.
Taking into account the performance of the different models, only results from the K-model are showed and discussed here since this appeared to have controlled better population structure and kinship relationships. Thirteen significant associations were detected between seven SSRs and eight phytosterol traits (Table 4). The BPPCT011 locus was associated with total phytosterol, stigmasterol and Δ7-stigmastenol contents. The EPDCU5100 and BPPCT030 markers were associated with β-sitosterol and stigmasterol, respectively. The CPPCT008 and UDP96-003 markers were associated with clerosterol and Δ7-stigmastenol and with total phytosterol and Δ7-avenasterol, respectively. Finally, the markers CPPCT047 and EPDCU3392 were associated with Δ7-campesterol and Δ7-stigmastenol, and with campesterol and Δ7-stigmastenol, respectively. The percentage of phenotypic variation explained by these markers ranged between 73.8 and 46.1%, with UDP96-003 having the maximum value and EPDCU5100 the minimum value. The P-values showing the level of significance of the associations between SSR markers and phytosterol traits are shown in Figure 5. Some associations were observed in the same regions where QTL had previously been identified (Table 4).
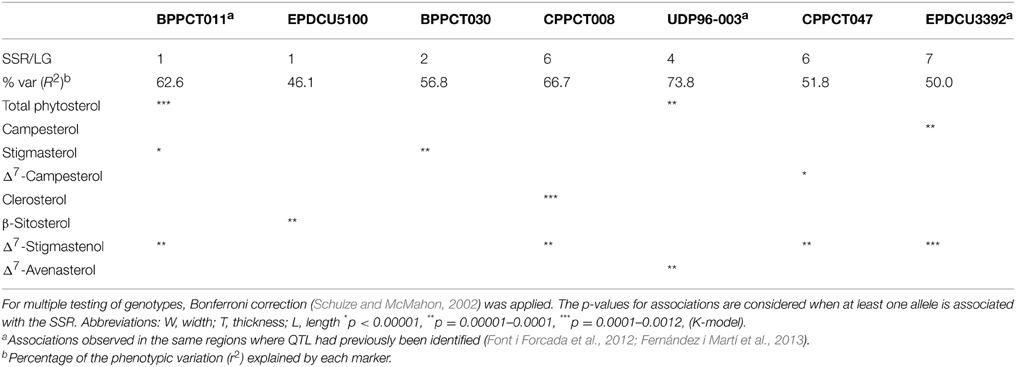
Table 4. Statistical significance of the p-values and associations observed between markers and phytosterol content of almond cultivars.
Discussion
Phytosterol content in plants is a complex trait known to be controlled by both major and minor genetic factors (Amar et al., 2008). As far as we know, this is the first time that the phytosterol content is genetically studied in almond, and also it represents the second AM analysis in this nut crop (Font i Forcada et al., 2015). However, there have so far been few studies on genotypic effects and QTL mapping phytosterols in other species. The few studies that have been documented are in sunflower (Haddadi et al., 2012; Merah et al., 2012) and Brassica napus L. (Amar et al., 2008; Shia, 2014).
The mean allele number found in this study, 13.9, was slightly lower than 17.2 obtained by Fernández i Martí et al. (2009), but very similar to 14.6 reported by Elhamzaoui et al. (2012), and much higher than 4.7 obtained by Martínez-Gómez et al. (2003). One possible reason for this discrepancy in the mean number of alleles (17.2 vs. 13.9) could be the inclusion of wild genotypes by Fernández i Martí et al. (2009), resulting in more alleles, some of which novel, whereas only domesticated germplasm was used in the present study, possibly having narrowed their genetic base. The average heterozygosity value of 0.66 is lower than 0.72 obtained by Fernández i Martí et al. (2009), but slightly higher than other values reported in almond, 0.62 by Elhamzaoui et al. (2012) and 0.59 by Martínez-Gómez et al. (2003).
The two main genetic groups identified by the Bayesian analysis corresponded to the Mediterranean and non-Mediterranean gene pools. These two groups could also be further substructured based on geographical origin. These clusters, however, could also be associated with local adaptation, diversifying selection, familial relatedness, or combinations thereof (Yu et al., 2006). Many species have undergone a long and complex period of domestication and breeding with limited gene flow, and this could be expected in the structure of this complex population (Sharbel et al., 2000). Hence, despite the diversity observed in almond, genetic bottlenecks may have occurred during almond dissemination (Fernández i Martí et al., 2015). The presence of population stratification and unequal distribution of alleles could result in non-functional, spurious associations (Pritchard and Rosenberg, 1999). In order to understand the distribution of genetic diversity in the almond cultivars, the model-based clustering approach was implemented in STRUCTURE to infer population structure. Thus, the 71 accessions could be separated in two different pools, the Mediterranean and non-Mediterranean, which are in line with those obtained by Fernández i Martí et al. (2015), where only 17 SSRs were used. In addition, in that study the cultivars from Europe also grouped separately from the Asian, American and Australian cultivars.
LD depends on a combination of many factors, such as the origin of the population, the selected set of accessions, the analyzed genomic region, the molecular marker system, and the presence of unidentified subpopulations. However, our LD results are very similar to those obtained by Fernández i Martí et al. (2015) (r2 = 0.04 for intra-chromosomal and r2 = 0.03 for inter-chromosomal), as well as in another self-incompatible Prunus species, such as sweet cherry (r2 = 0.028) (Arunyawat et al., 2012). Although LD in general decays more rapidly in out-crossing species than in selfing species, since recombination may be less effective in selfing species (Nordborg and Tabare, 2002), the level of LD decay observed in our study is comparable to the decay found in a self-compatible Prunus species such as peach (Cao et al., 2012; Font i Forcada et al., 2013). Population structure influences the magnitude and pattern of LD in almond, as in other species, such as Arabidopsis thaliana (Ostrowski et al., 2006), maize (Remington et al., 2001), and barley (Comadran et al., 2009). In the presence of LD, it will be possible to identify genetic regions (if LD extends to a distance of several cM) or genes (if LD decays quickly, in a few thousand base pairs) associated with a particular trait of interest by genome-wide associations or by individual SNPs (Single Nucleotide Polymorphisms) or SNP haplotypes within a candidate gene (Malysheva-Otto et al., 2006), respectively.
A previous study (Fernández i Martí et al., 2015) showed a strong subpopulation structure and LD decaying with increasing genetic linkage distance using only 17 SSR. Although the number of markers used in the present study was relatively low (40 SSR), our associations represent a first attempt of identification of candidate genes and provide strong evidence for the AM of phytosterol content in almond. Correction for the confounding effects of population structure present in plant populations is essential for AM because the complex population structure may cause spurious correlations (Pritchard et al., 2000). In our almond germplasm, we tested five models in TASSEL, and among them, K-model seems to have controlled population structure and kinship relationships better to eliminate the possible spurious associations. Results from this analysis have been corrected to minimize spurious associations leading to less inflated type I error (Yu et al., 2006).
Among the 40 SSR loci associated with phytosterol traits by association analysis (13 significant associations), many markers previously identified by linkage mapping were included (Font i Forcada et al., 2012). For example, out of the eight SSR markers identified for phytosterol traits in this study, three of them (BPPCT011, UDP96-003, and EPDCU3392) were significantly associated (seven significant associations) or located near regions where QTL had previously been identified in previous genetic studies (Font i Forcada et al., 2012). Furthermore, these seven associations were distributed among LG1, LG4, and LG7.
The complex traits detected in this study revealed that several putative genomic regions are involved in the expression of these phenotypes. To confirm the candidate genes and the marker location for the phytosterol traits, we screened the genes that belong to the plant sterol biosynthesis pathway and that are close to the associated markers found here using the browser Prunus persica genome sequence data (http://www.rosaceae.org). Interestingly, all the associations found in this work and located on LG1, LG2, LG4, LG6, and LG7 appeared to map within the interval where many candidate genes involved in the sterol biosynthesis pathway are predicted in the peach genome. For example, at the upper region of the LG1 and close to marker EPDCU5100, two genes (ppa022710m and ppa001844m), were identified at the same position than our EPDCU5100 marker and it might be responsible for the enzymatic reactions of cycloartenol synthase 2, SMT1.
This SSR marker was found to be associated with β-sitosterol analyzed here. Hence, the location surrounding this SSR might be considered as a good candidate region with genes controlling these phytosterols. Additionally, five more candidate genes also located on LG1 have been identified to control limiting steps in the biosynthesis of the end product sterol in plant cells (ppa012513m, ppa003821m, ppa011030m, ppa005590m, and ppa003991m). 24-Methylene sterol, cytochrome P450, Δ24-sterol reductase (DWF1), and squaleneepoxidase (SEQ1) appeared to be within the interval flanked by the two SSRs BPPCT011 and CPPCT053, which are associated in our study with contents of total phytosterol, and of stigmasterol and Δ7-stigmastenol.
Our analysis from genome scanning also found a candidate gene, ppa1027202m, strongly correlated with all the sterol traits analyzed here. This gene spans along the region where PMS40, CPDCT045 and UDP96-003 map on LG4 and it has been associated with the cytochrome P450, which encodes the sterol C-22 sterol desaturase. Finally, the results of the associations performed on LG7 were confirmed by the presence of another candidate gene, which is associated with C14 sterol reductase and ergosterol biosynthesis ERG4 (ppa007403m). This gene (scaffold 7: 14,682,911) is physically close to the SSR EPDCU3392 in the peach genome.
Almond has a poorly developed genomic infrastructure as compared to other Rosaceae species (peach, apple, strawberry, sweet cherry, etc…). With the application of new sequencing technologies, the availability of thousands of high-throughput single nucleotide polymorphisms (SNP) and various genomes of this family already sequenced (Velasco et al., 2010; Shulaev et al., 2011; Verde et al., 2012), yet relying on a set of SSR markers for identifying genes and/or QTL could be considered as a limiting factor. The application of NGS technologies and bioinformatic tools to generate high frequency SNPs still remains unexplored. Hence, the use of high-density genetic linkage maps based on SNP markers in genome mapping and phenotypic selection is still very limited in almond germplasm and breeding populations. Certainly, the use of such a small number of expressed sequence tags (EST) and microsatellite (SSR) might have somehow disadvantaged deeper conclusions in the present work, but our results match very well with most of the predicted genes found during the peach genome sequence project. Moreover, SSRs still remain very attractive for breeding purposes and they have proven superior power than other markers for genetic analysis (Khan and Korban, 2012). In addition, and as documented in the few studies on AM in Prunus, such as peach (Cao et al., 2012; Font i Forcada et al., 2013) and sweet cherry (Ganopoulos et al., 2011), the authors used mostly SSR markers for carrying out their findings. Marker numbers ranged in those studies from 15 SSRs in Ganopoulos et al. (2011) to 40 SSR in Font i Forcada et al. (2013) and 53 SSR in Cao et al. (2012). Thus, the 40 SSR markers used along our analysis may appear adequate for AM in almond.
It is noteworthy that an international consortium, in which our group participate, has recently been created in 2014 to sequence the whole genome of the almond cultivar “Texas” for intended release at the end of 2015 (Almond International Consortium). This advancement in genome sequencing will offer important new possibilities for SNP discovery and genome wide association studies (GWAS) in this nut crop. The cost of growing and maintaining tree crops until they reach maturity is very high, thus any effort to carry out early selection will be highly desirable to reduce orchard costs at the seedling stage.
Conclusion
In the present study, we identified seven markers associated with phytosterol content in almond. It has also been shown that wide genetic variation exists for kernel sterol contents in this nut crop. Our results showed that AM could be highly useful to detect significant complex traits of interest. Since this is the first genetic analysis for phytosterol content in almond, we suggest that these results provide an insight into the genetic architecture of important fruit quality traits for almond and this new genetic information would offer an efficient platform for classical and MAS breeding in this Prunus species.
Author Contributions
CF and AF designed the study and performed the molecular and statistical analysis. LV conducted the phytosterol analysis. RS provided the material from the almond germplasm collection. CF, RS, and AF discussed the results and drafted the manuscript. All authors have read and approved the final manuscript.
Conflict of Interest Statement
The authors declare that the research was conducted in the absence of any commercial or financial relationships that could be construed as a potential conflict of interest.
Acknowledgments
This work was supported by the Research Group A12 of Aragón (Spain) and the Marie Curie fellowship to AM.
References
Amar, S., Becker, H. C., and Mollers, C. (2008). Genetic variation and genotype × environment interactions of phytosterol content in three doubled haploid populations of winter rapeseed. Crop Sci. 48, 1000–1006. doi: 10.2135/cropsci2007.10.0578
Arunyawat, U., Capdeville, G., Decroocq, V., and Mariette, S. (2012). Linkage disequilibrium in French wild cherry germplasm and worldwide sweet cherry germplasm. Tree Genet. Genomes 8, 737–755. doi: 10.1007/s11295-011-0460-9
Bouic, P. J. D. (2001). The role of phytosterols and phytosterolins in immune modulation: a review of the past 10 years. Curr. Opin. Clin. Nutr. Metab. Care 4, 471–475. doi: 10.1097/00075197-200111000-00001
Bramley, P. M., Elmadfa, I., Kafatos, A., Kelly, F. J., Manio, Y., Roxborough, H. E., et al. (2000). Vitamin E. J. Sci. Food Agric. 80, 918–938. doi: 10.1002/(SICI)1097-0010(20000515)80:7<913::AID-JSFA600>3.0.CO;2-3
Breseghello, F., and Sorrells, M. E. (2006). Association mapping of kernel size and milling quality in wheat (Triticum aestivum L.) cultivars. Genetics 172, 1165–1177. doi: 10.1534/genetics.105.044586
Brookfield, J. F. Y. (1996). A simple new method for estimating null allele frequency from heterozygote deficiency. Mol. Ecol. 5, 453–455. doi: 10.1111/j.1365-294X.1996.tb00336.x
Cao, K., Wang, L., Zhu, G., Fang, W., Chen, C., and Luo, J. (2012). Genetic diversity, linkage disequilibrium, and association mapping analyses of peach (Prunus persica) landraces in China. Tree Genet. Genomes 8, 975–990. doi: 10.1007/s11295-012-0477-8
Castelli, W. P. (1984). Epidemiology of coronary heart disease: the Framingham study. Am. J. Med. 76, 4–12. doi: 10.1016/0002-9343(84)90952-5
Cevik, V., Ryder, C. D., Popovich, A., Manning, K., King, G. J., and Seymour, G. B. (2010). A FRUITFULL-like gene is associated with genetic variation for fruit flesh firmness in apple (Malus domestica Borkh.). Tree Genet. Genomes 6, 271–279. doi: 10.1007/s11295-009-0247-4
Clouse, S. D. (2000). Plant development: a role for sterols in embryogenesis. Curr. Biol. 10, R601–R604. doi: 10.1016/S0960-9822(00)00639-4
Comadran, J., Thomas, W. T. B., van Eeuwijk, F. A., Ceccarelli, S., Grando, S., Stanca, A. M., et al. (2009). Patterns of genetic diversity and linkage disequilibrium in a highly structured Hordeum vulgare association-mapping population for the Mediterranean basin. Theor. Appl. Genet. 119, 175–187. doi: 10.1007/s00122-009-1027-0
Elhamzaoui, A., Oukabli, A., Charafi, J., and Moumni, M. (2012). Assessment of genetic diversity of Moroccan cultivated almond (Prunus dulcis Mill D.A. Webb) in its area of extreme diffusion using SSR markers. Am. J. Plant Sci. 3, 1294–1303. doi: 10.4236/ajps.2012.39156
Espiau, M. T., Ansón, J. M., and Socias i Company, R. (2002). The almond germplasm bank of Zaragoza. Acta Hort. 591, 275–278.
Evanno, G., Regnaut, S., and Goudet, J. (2005). Detecting the number of clusters of individuals using the software STRUCTURE: a simulation study. Mol. Ecol. 14, 2611–2620. doi: 10.1111/j.1365-294X.2005.02553.x
Fernández-Cuesta, A., Aguirre-González, M. R., Ruiz-Méndez, M. V., and Velasco, L. (2012b). Validation of a method for the analysis of phytosterols in sunflower seeds. Eur. J. Lipid Sci. Technol. 114, 325–331. doi: 10.1002/ejlt.201100138
Fernández-Cuesta, A., Kodad, O., Socias i Company, R., and Velasco, L. (2012a). Phytosterol variability in almond germplasm. J. Am. Soc. Hort. Sci. 137, 343–348.
Fernández i Martí, A., Alonso, J. M., Espiau, M. T., Rubio-Cabetas, M. J., and Socias i Company, R. (2009). Genetic diversity in Spanish and foreign almond germplasm assessed by molecular characterization with simple sequence repeats. J. Am. Soc. Hort. Sci. 134, 535–542.
Fernández i Martí, A., Font i Forcada, C., Kamali, K., Rubio-Cabetas, M. J., Wirthensohn, M., and Socias i Company, R. (2015). Molecular analyses of evolution and population structure in a worldwide almond [Prunus dulcis (Mill.) D.A. Webb syn. P. amygdalus Batsch] pool assessed by microsatellite markers. Genet. Resour. Crop Evol. 62, 205–219. doi: 10.1007/s10722-014-0146-x
Fernández i Martí, A., Font i Forcada, C., and Socias i Company, R. (2013). Genetic analysis for physical nut traits in almond. Tree Genet. Genomes 9, 455–465. doi: 10.1007/s11295-012-0566-8
Flint-Garcia, S. A., Thornsberry, J. M., and Buckler, E. S. (2003). Structure of linkage disequilibrium in plants. Annu. Rev. Plant. Biol. 54, 357–374. doi: 10.1146/annurev.arplant.54.031902.134907
Font i Forcada, C., Espiau, M. T., Ansón, J. M., Socias i Company, R., and Fernández i Martí, A. (2015). Association mapping analysis for chemical and physical traits in almond. PLoS ONE 10:e0127656. doi: 10.1371/journal.pone.0127656
Font i Forcada, C., Fernández i Martí, A., and Socias i Company, R. (2012). Mapping quantitative trait loci for kernel composition in almond. BMC Genet. 13:47. doi: 10.1186/1471-2156-13-47
Font i Forcada, C., Oraguzie, N., Igartua, E., Moreno, M. A., and Gogorcena, Y. (2013). Population structure and marker-trait associations for pomological traits in peach and nectarine cultivars. Tree Genet. Genomes 9, 331–349. doi: 10.1007/s11295-012-0553-0
Ganopoulos, I., Kazantzis, K., Chatzicharisis, I., Karayiannis, I., and Tsaftaris, A. (2011). Genetic diversity structure and fruit trait associations in Greek sweet cherry cultivars using microsatellite based (SSR/ISSR) and morpho-physiological markers. Euphytica 181, 237–251. doi: 10.1007/s10681-011-0416-z
Haddadi, P., Ebrahimi, A., Langlade, N., Yazdi-Samadi, B., Berger, M., Calmon, A., et al. (2012). Genetic dissection of tocopherol and phytosterol in recombinant inbred lines of sunflower through quantitative trait locus analysis and the candidate gene approach. Mol. Breed. 29, 717–729. doi: 10.1007/s11032-011-9585-7
Khan, M. A., and Korban, S. S. (2012). Association mapping in forest trees and fruit crops. J. Exp. Bot. 63, 4045–4060. doi: 10.1093/jxb/ers105
Kodad, O., Estopañán, G., Juan, T., Mamouni, A., and Socias i Company, R. (2011). Tocopherol concentration in almond oil: genetic variation and environmental effect under warm conditions. J. Agric. Food Chem. 59, 6137–6141. doi: 10.1021/jf200323c
Kodad, O., Socias i Company, R., Prats, M. S., and López-Ortiz, M. C. (2006). Variability in tocopherol concentrations in almond oil and its use as a selection criterion in almond breeding. J. Hort. Sci. Biotechnol. 81, 501–507.
Krill, A. M., Kirst, M., Kochian, L. V., Buckler, E. S., and Hoekenga, O. A. (2010). Association and linkage analysis of aluminum tolerance genes in maize. PLoS ONE 5:e9958. doi: 10.1371/journal.pone.0009958
Law, M. (2000). Plant sterol and stanol margarines and health. Br. Med. J. 320, 861–864. doi: 10.1136/bmj.320.7238.861
Lindsey, K., Pullen, M. L., and Topping, J. F. (2003). Importance of plant sterols in pattern formation and hormone signaling. Trends Plant Sci. 8, 1360–1385. doi: 10.1016/j.tplants.2003.09.012
Mackay, I., and Powell, W. (2007). Methods for linkage disequilibrium mapping in crops. Trends Plant Sci. 12, 57–63. doi: 10.1016/j.tplants.2006.12.001
Malysheva-Otto, V., Ganal, W., and Roder, S. (2006). Analysis of molecular diversity, population structure and linkage disequilibrium in a worldwide survey of cultivated barley germplasm (Hordeum vulgare L.). BMC Genet. 7:6. doi: 10.1186/1471-2156-7-6
Martínez-Gómez, P., Arulsekar, S., Potter, D., and Gradziel, T. M. (2003). An extended interspecific gene pool available to peach and almond breeding as characterized using simple sequence repeat (SSR) markers. Euphytica 131, 313–322. doi: 10.1023/A:1024028518263
Merah, O., Langlade, N., Alignan, M., Roche, J., Pouilly, N., Lippi, Y., et al. (2012). Genetic analysis of phytosterol content in sunflower seeds. Theor. Appl. Genet. 125, 1589–1601. doi: 10.1007/s00122-012-1937-0
Mnejja, M., Garcia-Mas, J., Audergon, J. M., and Aruìs, P. (2010). Prunus microsatellite transportability across rosaceous crops. Tree Genet. Genomes 6, 689–700. doi: 10.1007/s11295-010-0284-z
Nordborg, M., and Tabare, S. (2002). Linkage disequilibrium: what history has to tell us. Trends Genet. 18, 83–90. doi: 10.1016/S0168-9525(02)02557-X
Oraguzie, N. C., Whitworth, C. J., Brewer, L., Hall, A., Volz, R. K., Bassett, H., et al. (2010). Relationships of PpACS1 and PpACS2 genotypes, internal ethylene concentration and fruit softening in European (Pyrus communis) and Japanese (Pyrus pyrifolia) pears during cold air storage. Plant Breed. 129, 219–226. doi: 10.1111/j.1439-0523.2009.01684.x
Oraguzie, N. C., Wilcox, P. L., Rikkerink, E. H. A., and de Silva, H. N. (2007). “Linkage disequilibrium,” in Association Mapping in Plants, eds N. C. Oraguzie, E. H. A. Rikkerink, S. E. Gardiner, and H. N. de Silva (New York, NY: Springer), 11–39.
Ostrowski, M. F., David, J., Santoni, S., McKhann, H., Reboud, X., Le Corre, V., et al. (2006). Evidence for a large-scale population structure among accessions of Arabidopsis thaliana: possible causes and consequences for the distribution of linkage disequilibrium. Mol. Ecol. 15, 1507–1517. doi: 10.1111/j.1365-294X.2006.02865.x
Pajerowska-Mukhtar, K., Stich, B., Achenbach, U., Ballvora, A., Lubeck, J., Strahwald, J., et al. (2009). Single nucleotide polymorphisms in the allene oxide synthase 2 gene are associated with field resistance to late blight in populations of tetraploid potato cultivars. Genetics 181, 1115–1127. doi: 10.1534/genetics.108.094268
Piironen, V., Lindsay, D. G., Miettinen, T. A., Toivo, J., and Lampi, A. M. (2000). Plant sterols: biosynthesis, biological function and their importance to human nutrition. J. Sci. Food Agr. 80, 939–966. doi: 10.1002/(SICI)1097-0010(20000515)80:7<939::AID-JSFA644>3.0.CO;2-C
Plat, J., and Mensink, R. P. (2005). Plant stanol and sterol esters in the control of blood cholesterol levels: mechanism and safety aspects. Am. J. Cardiol. 96, 15–22. doi: 10.1016/j.amjcard.2005.03.015
Pritchard, J. K., and Rosenberg, N. A. (1999). Use of unlinked genetic markers to detect population stratification in association studies. Am. J. Hum. Genet. 65, 220–228. doi: 10.1086/302449
Pritchard, J. K., Stephens, M., Rosenberg, N. A., and Donnelly, P. (2000). Association mapping in structured populations. Am. J. Hum. Genet. 67, 170–181. doi: 10.1086/302959
Remington, D. L., Thornsberry, J. M., Matsuoka, Y., Wilson, L. M., Whitt, S. R., Doebley, J., et al. (2001). Structure of linkage disequilibrium and phenotypic associations in the maize genome. Proc. Natl. Acad. Sci. U.S.A. 98, 11479–11484. doi: 10.1073/pnas.201394398
Saitou, N., and Nei, M. (1987). The neighbor-joining method: a new method for reconstructing phylogenetic trees. Mol. Biol. Evol. 4, 406–425.
Sánchez-Pérez, R., Howad, W., Dicenta, F., Arús, P., and Martínez-Gómez, P. (2007). Mapping major genes and quantitative trait loci controlling agronomics traits in almond. Plant Breed. 126, 310–318. doi: 10.1111/j.1439-0523.2007.01329.x
Schulze, T. G., and McMahon, F. J. (2002). Genetic association mapping at the crossroads: which test and why? Overview and practical guidelines. Am. J. Med. Genet. 114, 1–11. doi: 10.1002/ajmg.10042
Sharbel, T. F., Haubold, B., and Olds, T. (2000). Genetic isolation by distance in Arabidopsis thaliana: bio- geography and postglacial colonization of Europe. Mol. Ecol. 9, 2109–2118. doi: 10.1046/j.1365-294X.2000.01122.x
Shia L. Teh. (2014). Genetic Variation and Inheritance of Phytosterol and Oil Content in Winter Oilseed Rape (Brassica napus L.). Ph.D. dissertation, Faculty of Agricultural Sciences, Georg-August-Universität Göttingen, Germany.
Shulaev, V., Sargent, D. J., Crowhurst, R. N., Mockler, T. C., Folkerts, O., Delcher, A. L., et al. (2011). The genome of woodland strawberry (Fragaria viscera). Nat. Genet. 43, 109–116. doi: 10.1038/ng.740
Simko, I., Pechenick, D. A., McHale, L. K., Truco, M. J., Ochoa, O. E., Michelmore, R. W., et al. (2009). Association mapping and marker-assisted selection of the lettuce dieback resistance gene Tvr 1. BMC Plant Biol. 9:135. doi: 10.1186/1471-2229-9-135
Socias i Company, R. (1998). Fruit tree genetics at a turning point: the almond example. Theor. Appl. Genet. 96, 588–601. doi: 10.1007/s001220050777
Socias i Company, R., Alonso, J. M., Kodad, O., and Gradziel, T. M. (2012). “Almond,” in Fruit Breeding, Handbook of Plant Breeding, Vol. 8, eds M. L. Badenes and D. Byrne (Heidelberg: Springer-Verlag), 697–728.
Socias i Company, R., Kodad, O., Alonso, J. M., and Gradziel, T. M. (2008). Almond quality: a breeding perspective. Hort. Rev. 34, 197–238. doi: 10.1002/9780470380147.ch3
Stich, B., Mohring, J., Piepho, H. P., Heckenberger, M., Buckler, E. S., and Melchinger, A. E. (2008). Comparison of mixed-model approaches for association mapping. Genetics 178, 1745–1754. doi: 10.1534/genetics.107.079707
Swofford, D. L. (2003). PAUP*, Phylogenetic Analysis using Parasimony (*and Other Methods). Sunderland, MA: Sinauer Associates.
van Rensburget, S. J., Daniels, W. M., van Zyl, J. M., and Taljjard, J. J. (2000). A comparative study of the effects of cholesterol, beta-sitosterol, beta-sitosterolglucoside, dehydroepian- drosterone, sulphate and melatonin on in vitro lipid peroxidation. Metab. Brain Dis. 15, 257–265. doi: 10.1023/A:1011167023695
Velasco, R., Zharkikh, A., Affourtit, J., Dhingra, A., Cestaro, A., Kalyanaraman, A., et al. (2010). The genome of the domesticated apple (Malus domestica Borkh). Nat. Genet. 42, 833–839. doi: 10.1038/ng.654
Verde, I., Bassil, N., Scalabrin, S., Gilmore, B., Lawley, C. T., Gasic, K., et al. (2012). Development and evaluation of a 9K SNP array for peach by internationally coordinated SNP detection and validation in breeding germplasm. PLoS ONE 7:e35668. doi: 10.1371/journal.pone.0035668
Wilson, L. M., Whitt, S. R., Ibañez, A. M., Rocheford, T. R., Goodman, M. M., and Buckler, E. S. (2004). Dissection of maize kernel composition and starch production by candidate gene association. Plant Cell 16, 2719–2733. doi: 10.1105/tpc.104.025700
Yu, J. M., and Buckler, E. S. (2006). Genetic association mapping and genome organization of maize. Curr. Opin. Biotechnol. 17, 155–160. doi: 10.1016/j.copbio.2006.02.003
Keywords: Prunus amygdalus, genetic variability, sterol content, population structure, linkage disequilibrium, SSR markers, candidate genes
Citation: Font i Forcada C, Velasco L, Socias i Company R and Fernández i Martí Á (2015) Association mapping for kernel phytosterol content in almond. Front. Plant Sci. 6:530. doi: 10.3389/fpls.2015.00530
Received: 26 May 2015; Accepted: 29 June 2015;
Published: 09 July 2015.
Edited by:
Jaime Prohens, Universitat Politècnica de València, SpainReviewed by:
Sergio Lanteri, DISAFA - University of Turin, ItalyDaniela Marone, Centre of Cereal Research - CRA-CER, Foggia, Italy
Copyright © 2015 Font i Forcada, Velasco, Socias i Company and Fernández i Martí. This is an open-access article distributed under the terms of the Creative Commons Attribution License (CC BY). The use, distribution or reproduction in other forums is permitted, provided the original author(s) or licensor are credited and that the original publication in this journal is cited, in accordance with accepted academic practice. No use, distribution or reproduction is permitted which does not comply with these terms.
*Correspondence: Ángel Fernández i Martí, Genome Center, University of California, 451 Health Sciences Dr., Davis, Davis, CA 95616, USA,YWZlcm5hbmRlem1hcnRpQHVjZGF2aXMuZWR1