- 1Forestry Research Institute, Hokkaido Research Organization, Bibai, Japan
- 2Institute of Low Temperature Science, Hokkaido University, Sapporo, Japan
- 3Graduate School of Agricultural and Life Sciences, The University of Tokyo, Tokyo, Japan
Boreal coniferous species with wide geographic distributions show substantial variation in autumn cold acclimation among populations. To determine how this variation is inherited across generations, we conducted a progeny test and examined the development of cold hardening in open-pollinated second-generation (F2) progeny of Abies sachalinensis. The F1 parents had different genetic backgrounds resulting from reciprocal interpopulational crosses between low-elevation (L) and high-elevation (H) populations: L × L, L × H, H × L, and H × H. Paternity analysis of the F2 progeny using molecular genetic markers showed that 91.3% of the fathers were located in surrounding stands of the F1 planting site (i.e., not in the F1 test population). The remaining fathers were assigned to F1 parents of the L × L cross-type. This indicates that the high-elevation genome in the F1 parents was not inherited by the F2 population via pollen flow. The timing of autumn cold acclimation in the F2 progeny depended on the cross-type of the F1 mother. The progeny of H × H mothers showed less damage in freezing tests than the progeny of other cross-types. Statistical modeling supported a linear effect of genome origin. In the best model, variation in freezing damage was explained by the proportion of maternally inherited high-elevation genome. These results suggest that autumn cold acclimation was partly explained by the additive effect of the responsible maternal genome. Thus, the offspring that inherited a greater proportion of the high-elevation genome developed cold hardiness earlier. Genome-based variation in the regulation of autumn cold acclimation matched the local climatic conditions, which may be a key factor in elevation-dependent adaptation.
Introduction
Seasonal growth cycles are well described for plant taxa distributed in boreal, sub-boreal, and temperate climates. In particular, for evergreen coniferous species, cold acclimation often occurs before temperatures drop below freezing (Aitken and Hannerz, 2001; Chuine, 2010). During this acclimation, evergreen plants shift their physiological condition from an active growth phase to a hardening phase that involves some degree of freezing tolerance. This physiological shift is responsible for a series of mechanical and biochemical changes in the cell: for example, intracellular accumulation of compatible osmolytes such as soluble sugars, accumulation of polypeptides and/or proteins, and the vesiculation of vacuoles (Fujikawa et al., 2006). The timing of cold acclimation affects fitness. A long duration of the hardy phase may help plants avoid autumn and spring freezing damage. However, it can also limit annual photosynthetic activity and growth. Therefore, because of a trade-off between growth and risk of freezing damage, the timing of phenological events can be a key driver for adaptation to cold climates. During their long evolutionary histories, conifers have acquired an adaptive schedule of cold acclimation to survive under their local environmental conditions, using changes in daylength and/or temperature as regulatory signals (Kozlowski and Pallardy, 2002). Population differences in the acclimation schedule can be observed within species that inhabit wide geographic distributions spanning diverse climatic regions. Interpopulational genetic variation in phenological traits is associated with climatic differences (Rehfeldt, 1989; Oleksyn et al., 1998). Furthermore, interpopulational genetic variation for many boreal conifers is greater during autumn cold acclimation than during spring dehardening (Aitken and Hannerz, 2001; Howe et al., 2003). It is a consistent trend that populations from high latitudes and elevations exhibit earlier development of cold hardening than those from low latitudes or elevations (Rehfeldt, 1989; Skrøppa and Magnussen, 1993; Oleksyn et al., 1998; Savolainen et al., 2004; Notivol et al., 2007; Mimura and Aitken, 2010).
Common garden trials and reciprocal transplantation experiments are powerful tools for detecting adaptive variation in plants and the drivers of natural selection. A previous study using transplanted materials of the sub-boreal conifer Abies sachalinensis Mast. (Sakhalin fir) has revealed an adaptive cline in the timing of autumn cold acclimation along an elevational gradient (Ishizuka et al., 2015). In that study, earlier development of autumn cold acclimation in trees derived from high-elevation populations than in those from low-elevation populations was detected at all transplantation sites. Modeling analysis was then conducted to examine the environmental conditions responsible for the detected physiological variation. The results have shown that the genetic variation in response to the temperature change might be an important driver of elevation-dependent adaptation (Ishizuka et al., 2015). However, the mechanisms by which the genome generates quantitative adaptive differences are not clearly understood. Quantitative traits resulting as a consequence of genome inheritance and genome effects are complex (Sykes et al., 2006; Cané-Retamales et al., 2011). Furthermore, some studies have reported epigenetic phenomena which have regulated phenological traits (Johnsen et al., 2005; Kvaalen and Johnsen, 2008). Perennial plants have a “memory” of the climate they experienced during embryogenesis. These epigenetic signals may be transmitted through several mechanisms, including cytosine methylation and histone modification, and may enhance adaptation at a population level (Johnsen et al., 2005; Kvaalen and Johnsen, 2008). Controlled crosses using mother plants that have been exposed to the same environmental conditions can be used to test the effects of genome inheritance on the regulation of the autumn cold acclimation. However, previous ecological studies have not extensively evaluated the complex effects of genome inheritance on the development of cold acclimation in trees.
We investigated the genetic basis of variation in the regulation of autumn cold acclimation using Sakhalin fir. This conifer plays an important role in the timber production in northern Japan. It is a dominant climax species in natural sub-boreal forests of the Far East, thus forming an important component of forest ecosystems (Kato, 1952). The geographic range of this species extends from eastern Siberia to Hokkaido, the northernmost island of the Japanese archipelago (Figure 1A). The elevational distribution extends from 100 to 1600 m a.s.l. This species survived in a glacial refugium in Hokkaido, and had become a dominant species by the time of the last glacial maximum, 20000 years ago (Igarashi, 1996; Tsumura and Suyama, 1998). Interpopulational variation in several traits has been observed within this elevational range, including variation in growth and survival (Goto et al., 2011; Ishizuka and Goto, 2012), resistance to biological stresses such as disease or rats (Kurahashi and Hamaya, 1981; Saho et al., 1994), and cold hardiness (Eiga and Sakai, 1984). In our recent study, we reported that clinal variation in the timing of cold acclimation appears to result from adaptation to the local climate at elevations ranging from 230 to 1200 m (Ishizuka et al., 2015).
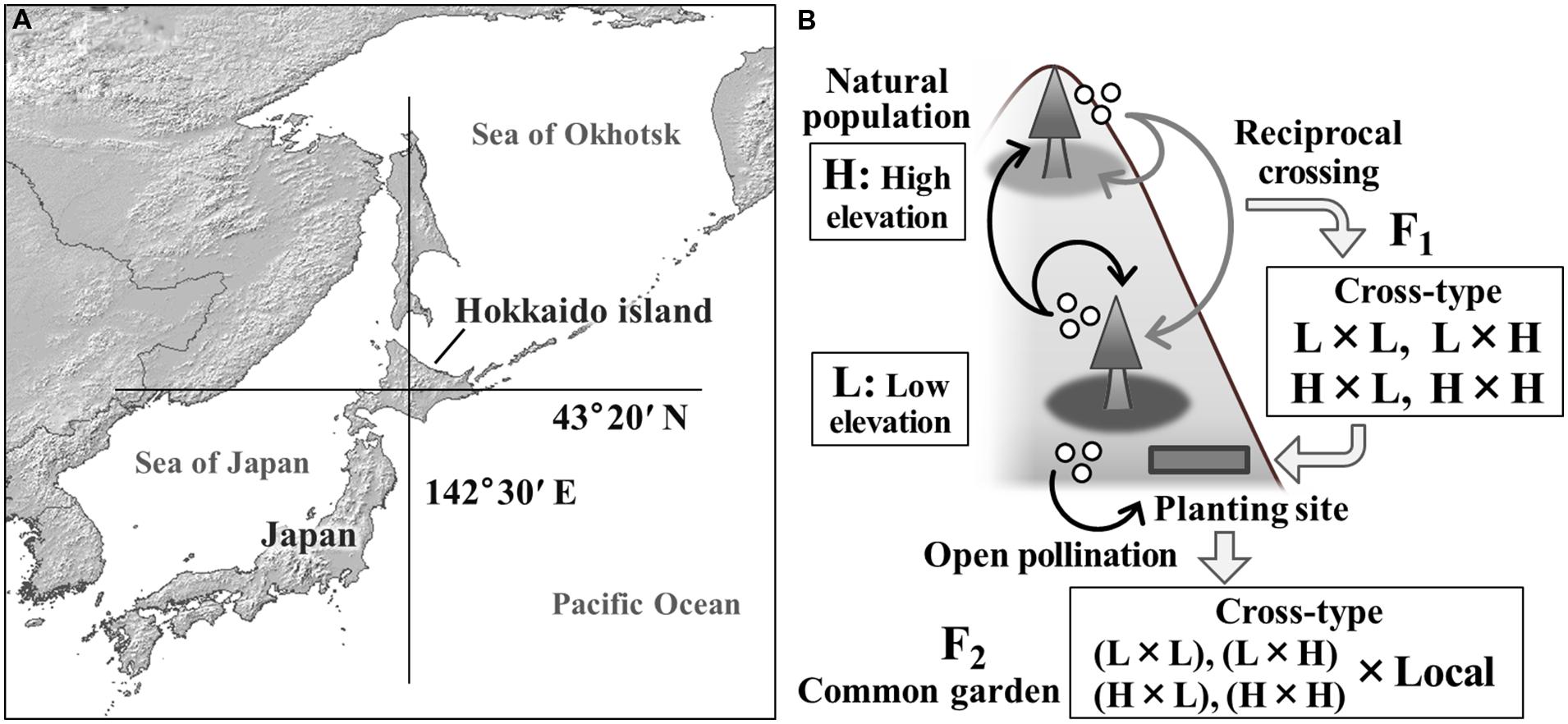
FIGURE 1. A map of the study site (A) and the experimental design using F2 progeny of interpopulational crosses of Abies sachalinensis (B). Two natural populations used for crossing were located where the latitudinal and longitudinal lines cross in panel (A). A planting site of the F1 population and a common garden trial for the F2 progeny were established in the same area. The F1 population was produced using reciprocal crossing between low-elevation (L) and high-elevation (H) populations in 1979. Open-pollinated seeds were collected from the F1 mother trees in 2009. The resulting F2 progeny had various genetic backgrounds resulting from the maternal cross-type.
In the present study, we used open pollination to obtain second-generation (F2) progeny for testing the genetic basis of variation in phenological traits. The maternal population (F1) was produced by reciprocal crosses between two distinct ecotypes: a population inhabiting low elevations and a population inhabiting high elevations. The genetic background of the maternal F1 trees varied among cross-types (low × low, hybrids between elevations, high × high), and the trees were planted at a single site in order to ensure their exposure to the same environment. Thus, we could evaluate the effects of genome inheritance by examining the traits of the F2 population. Using the F2 progeny, we conducted (1) paternity analysis based on microsatellite markers and (2) statistical modeling of freezing damage. Our objectives were to (1) measure variation in the timing of autumn cold acclimation of the F2 progeny and (2) establish whether this variation could be explained by the genomic background inherited from the low-elevation or high-elevation populations.
Materials and Methods
F1 Planting Site
We conducted this study in the University of Tokyo Hokkaido Forest (UTHF), located in central Hokkaido, northern Japan (43°20′N, 142°30′E; Figure 1A). In the UTHF, natural sub-boreal forests cover about 150 km2 of the south-western slope of Mt. Dairoku (1459 m a.s.l.), with an elevational gradient of more than 1200 m. With increasing elevation, dramatic changes occur in soil type, snow depth, and forest vegetation, including changes in the prevalence of the bamboo species, Sasa senanensis and Stigmella kurilensis (Kato, 1952; Toyooka et al., 1983; Nakata et al., 1994). However, the primary factor changing with elevation is air temperature. Based on monitoring data recorded hourly from 2009 to 2010 at 230 m, the annual and winter (October–March) mean temperatures were 6.53°C and -1.78°C, respectively. Temperatures at 1100 m were 1.75°C and -6.43°C, respectively.
Sakhalin fir, A. sachalinensis, grows from the lowland (200 m) to the high-elevation zone (1200 m) in this area. In 1979, natural populations at 400–530 m and 1100–1200 m were selected as low-elevation (L) and high-elevation (H) populations, respectively, and artificial reciprocal crossing was performed by Kurahashi (Kurahashi, 1995; Goto et al., 2011). Within each population, five and three adult trees were selected as mother (seed parents) and father trees (pollen donors), respectively (Kurahashi and Hamaya, 1981). Pollen from the three father trees in each population was pooled and used for the crosses. The generated progeny were categorized into four cross-types (female × male): L × L, L × H, H × L, and H × H (Figure 1B). We refer to these progeny as the F1 population. Seedlings of the F1 population were grown in an outdoor nursery at a lowland site of the UTHF. In 1986, the F1 individuals were transplanted to the lowland planting site (220 m; Figure 1B). At the planting site, the progeny were arrayed in grids at a spacing of 1.2 m, assigning one block (2 columns × 10 rows) to each cross-treatment. Unreplicated blocks were used to avoid contamination among treatments (Kurahashi, 1995). In total, 440 F1 seedlings were transplanted. Thus, the F1 populations had varying genetic backgrounds but were exposed to the same environmental conditions. Further information about the traits measured on the F1 trees (survival, height, diameter at breast height, leaf nitrogen content, and leaf area per weight) were provided by Goto et al. (2011).
Seed Collection from the F1 Generation
In 2009, 23 years after planting, 353 F1 trees were alive and some of them were in a reproductive stage. Our field surveys during the flowering and seed-maturing seasons showed that 22 trees flowered that year at the F1 planting site (Table 1). We collected open-pollinated seeds from the flowering trees on September 6th and 9th, 2009. We selected 13 F1 mother trees that produced a sufficient number of progeny (>20 cones) to use in this study (Table 1; see the Supplemental Table for ID-information of the mother trees). The progeny (F2 population) were grouped into four types according to the maternal cross category: L × L, L × H, H × L, and H × H (Figure 1B). Collected seeds were stored at -3°C until use.
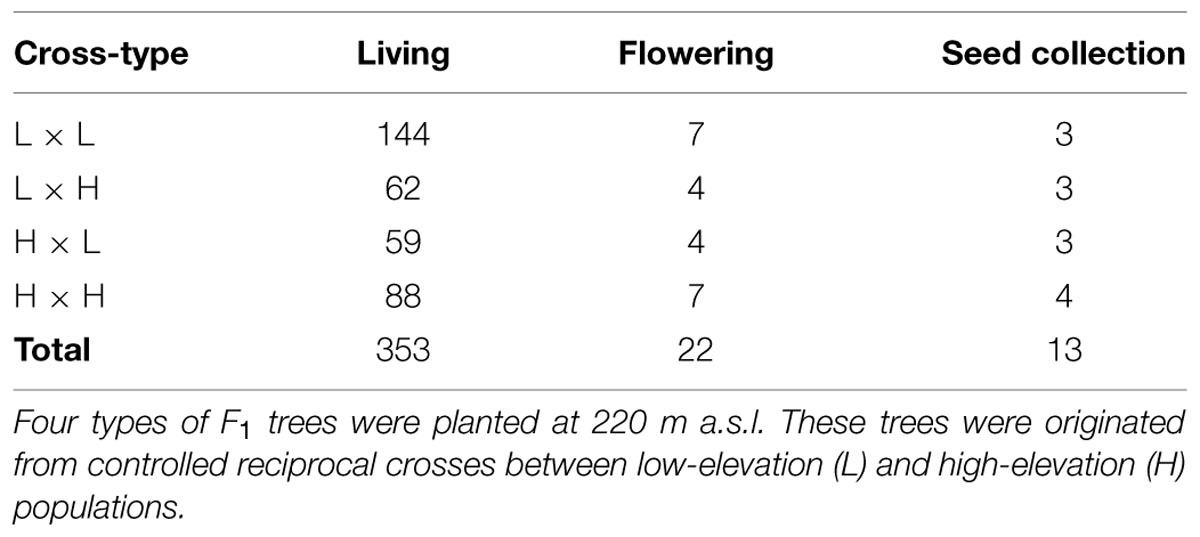
TABLE 1. Number of F1 trees of Abies sachalinensis that were living, flowering, or used to collect open-pollinated seeds in 2009.
F2 Common Garden Trial
We conducted a common garden trial of the F2 progeny. Before the trial, viable seeds were selected from all collected seeds using soft X-ray photography (Softex, Kanagawa, Japan). In the spring of 2010, the viable seeds were subjected to a seed stratification treatment and were sown into the UTHF nursery (230 m a.s.l.; Figure 1A). One seed was placed in each 4-cm grid of a 1.0 m × 1.2 m block to maintain the identity of all germinated seedlings. The four blocks were separated by buffer spaces. To avoid edge effects for the F2 progeny, control seeds were also sown at the edge of the rows and columns. In total, 2112 seeds were sown in this trial (four blocks × 22 rows × 24 columns). The seedlings were raised until the end of the second growing season. As shown in Table 2, the cross-type differences of the mother of the F2 progeny were reflected in some functional traits, such as the germination rates and 2-year heights.
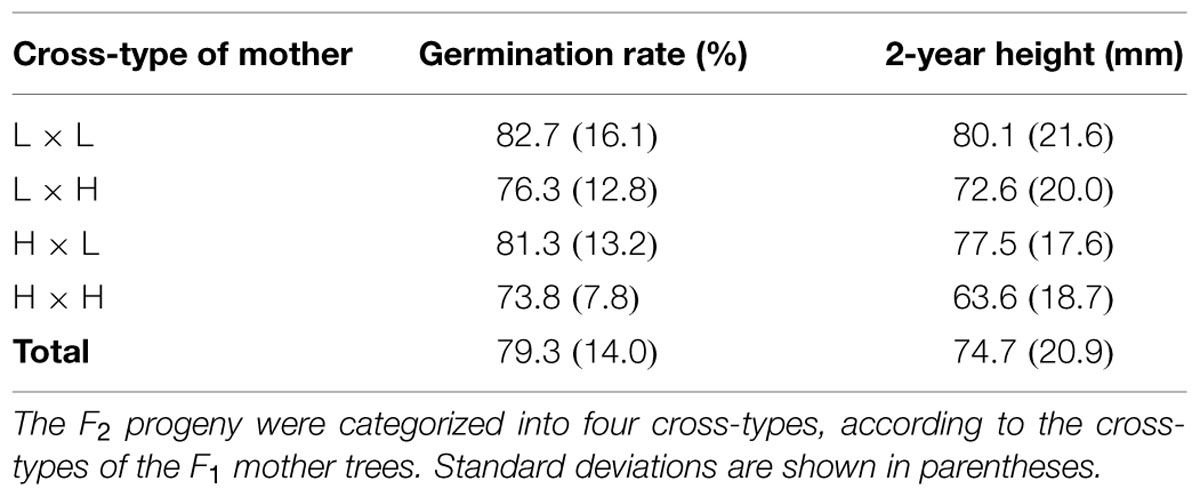
TABLE 2. Germination rates and 2-year heights of F2 progeny in a common garden trial at 230 m a.s.l.
Genotyping using SSR Markers
To estimate the genome composition of the F2 progeny, we performed molecular genetic analysis using nuclear microsatellite (nSSR) and chloroplast microsatellite (cpSSR) markers. In the present study, we used four nSSRs: As08, As16, As32 (Lian et al., 2007), and NFH 15 (Hansen et al., 2005), and three cpSSRs: pt30204, pt71936 (Vendramin et al., 1996), and pt30249 (Liepelt et al., 2001).
Samples selected for SSR genotyping consisted of the 22 flowering F1 trees described in Table 1 plus 80 of their F2 progeny. These 80 F2 progeny were derived from seeds collected in 2009, and were composed of 16 progeny from each of five F1 trees selected across the cross-types (Table 3). These seedlings were germinated in an indoor growth chamber.
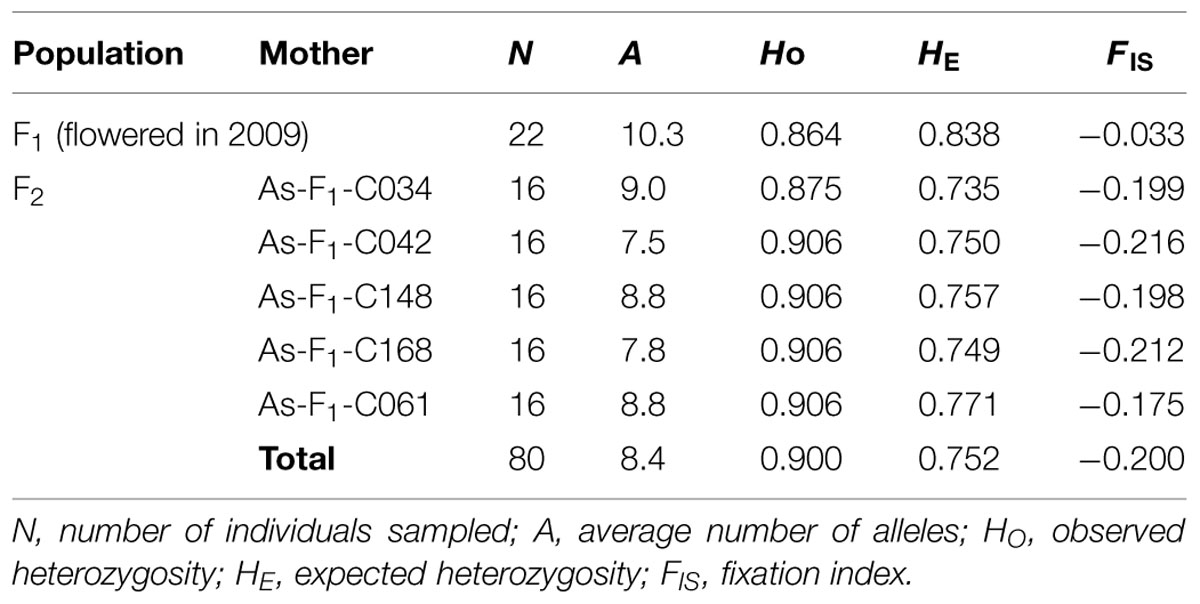
TABLE 3. Genetic diversity statistics of the F1 trees that flowered in 2009 and their open-pollinated F2 progeny.
For the DNA extractions, foliage was collected from the 22 candidate F1 trees and the 80 F2 progeny. DNA was extracted using the DNeasy Plant Mini Kit (Qiagen, Ltd., Crawley, UK), and the PCR reactions were performed using the Multiplex PCR Kit (Qiagen, Ltd., Crawley, UK) following the protocol of Lian et al. (2007). The PCR products were sequenced using an Applied Biosystems 3130xl Genetic Analyzer (Life Technologies, Carlsbad, CA, USA). Genotyping of all samples was performed based on the length of each sequenced fragment using Peak Scanner ver. 1.0 software from Applied Biosystems. Genetic diversity statistics were calculated by GenAlEx ver. 6.5 (Peakall and Smouse, 2012).
Paternity Analysis
We considered the 22 flowering F1 trees as candidate pollen parents of the F2 progeny because no other trees were flowering at the F1 planting site. However, trees outside of the F1 population may have also served as pollen parents because the F1 planting site was surrounded by mature artificial forests. The origin of these artificial forests was a local lowland forest (200–300 m) that was different from the forests used to create the F1 population.
For paternity analysis, we first inferred paternity of the F2 progeny using four nSSRs. Using CERVUS 3.0 (Kalinowski et al., 2007), we compared the nSSRs genotypes of the 80 F2 progeny to their known seed parents (mothers) and 22 candidate F1 pollen parents (fathers). The most likely father was estimated using maximum-likelihood assignment from the possible allele combinations (genotypes) of the parents. When none of the potential F1 donors were assigned as fathers, we considered pollen contamination and genotyping errors as possible causes. Genotyping errors can lead to inflated estimates of outside pollen flow (Slavov et al., 2005). We set the genotyping error to 1% with an 80% confidence level for the 10000 cycle-simulations for the paternity assignment.
After assigning fathers using CERVUS, we performed a simple exclusion procedure using three cpSSRs. The F2 progeny and the assumed F1 pollen parents were required to have matching cpSSR haplotypes (Abies cpSSRs are paternally inherited; Vendramin and Ziegenhagen, 1997). We assumed that CERVUS assigned the correct pollen parent when the genotypes of the F2 progeny and assigned pollen parent matched for all three cpSSR markers (considering genotyping errors). When a complete match was not found, we assumed the pollen came from outside of the F1 planting site.
Combining the results from CERVUS and the exclusion procedure, we estimated the proportion of selfed seeds, seeds sired by the F1 population, and seeds sired by fathers outside the studied F1 population. We also estimated the composition of the F1 trees among four cross-types, based on the genotyping results.
Autumn Freezing Test
We conducted freezing tests on the F2 progeny three times during the autumn season using the method of Eiga and Sakai (1984). The potted seedlings were placed outdoors just before the test. Three freezing tests were conducted at approximately 2-week intervals: on 12 and 26 October, and 11 November (referred to as Tests 1, 2, and 3). Each freezing test was performed at the Institute of Low Temperature Science, Hokkaido University. The potted seedlings were placed in a dark chamber kept at 5°C. After an overnight incubation, the temperature was lowered at a rate of 2°C per hour until the target temperature was reached. The target temperature was maintained for 4 h, and then increased at a rate of 2°C per hour to 5°C. For Test 1, the target temperature was -15°C. For Tests 2 and 3, the target temperatures were -15 and -30°C. The seedlings were kept in the growth chamber for 14 days with a 12-h photoperiod (the photosynthetic photon flux density was 100 μmol m-2 s-1 during the day). We measured freezing damage using the visual scoring method of Lindgren and Hallgren (1993). Freezing damage was scored using six classes of needle discoloration (brown needles) as follows: 0 (no damage), 1 (1–20% of needles were discolored), 2 (21–40% discolored), 3 (41–60% discolored), 4 (61–80% discolored), and 5 (81–100% discolored). For each temperature at each time point, we used 10 F2 seedlings from each F1 mother tree. For some maternal trees with fewer seedlings, we used seven seedlings. In total, 605 seedlings were used in the freezing test (121 seedlings × 5 test conditions).
Data Analysis
Because no freezing damage was observed in the -15°C treatment in Tests 2 and 3, we excluded these data from subsequent analysis. Therefore, three test conditions were defined: T1 involved freezing at -15°C in Test 1, T2 involved freezing at -30°C in Test 2, and T3 involved freezing at -30°C in Test 3. For each condition, we used a nested ANOVA to study the effect of maternal cross-type and the effect of mother trees on freezing damage in the F2 progeny, using the following model:
where Yijk is the freezing damage score of the k-th progeny (k; 1-10) of the j-th mother tree (j; 1-4) in the i-th cross-type (i; 1-4), μ is the general mean, Ci is the effect of the i-th cross-type, Mj (Ci) is the effect of the j-th mother tree nested in the i-th cross-type, and Eijk is the residual error.
We also evaluated the effect of low-elevation and high-elevation plant genomes on freezing damage using the following statistical model that includes all test conditions. We assumed that freezing damage in the F2 progeny is affected by the genetic inheritance, test conditions, and mother tree. Therefore, we constructed the full model with all assumed effects, as follows:
where Yijkl is the freezing damage score of the k-th progeny of the j-th mother tree in the i-th cross-type under the l-th test condition (l; 1-3), μ, Ci and Mj (Ci) are the same as in Model 1, and Tl is the effect of the l-th test condition. In this full model, we regard the model element C as representing the effect of the plant genome (genetic effect). Then, in the analysis, C, T, and their interaction (C × T) are treated as fixed effects, whereas M (C) and its interaction with T are treated as random effects. Because damage is an ordinal variable (Ishizuka et al., 2015), we used an ordered probit mixed model (Lee, 1992). Specifically, we used the cumulative link mixed model (CLMM) function in the “ordinal” package of R 3.1.2 (R Development Core Team, 2014). CLMM uses a maximum likelihood approach with the Laplace approximation and adaptive Gauss-Hermite quadrature (see Christensen, 2014).
We then assessed which types of variables were appropriate for the effect of the plant genome (C) to describe the freezing damage score. Therefore, we used the following four types of the genetic effects as C in Models 2–5:
Model 2: Linear effect (0 for L × L; 0.25 for L × H and H × L; 0.5 for H × H)
Model 3: Categorical effect (L × L; L × H; H × L; H × H)
Model 4: Categorical interaction effect (“NoHyb” for L × L and H × H; “HybLH” for L × H; “HybHL” for H × L)
Model 5: Linear effect + interaction effect (0 for L × L; 0.25 + α for L × H; 0.25 - α for H × L; 0.5 for H × H).
In Model 2, the proportion of high-elevation genome inherited from the maternal parent was used. Numeric values indicating the linear effect of the maternal genome origin were assigned to C. The highest value was assigned to the H × H cross-type, whereas the lowest value, 0, was assigned to the L × L cross-type. Model 2 should show a good fit if freezing damage was closely related to the amount of high-elevation genome inherited from the maternal trees (i.e., quantitative trait). In Model 3, C was set to one of four categorical values indicating the cross-type of the maternal trees. Model 3 should fit well if the maternal origin is important, but the proportion of high-elevation genome is not. Model 4 included a genome interaction effect. As described above, C was set to one of three categorical values: NoHyb, HybLH, or HybHL. In Model 5, we included both the cross-type linear effect and genome interaction effect. C was partitioned into the combination of the variables used in Models 2 and 4.
We used a model selection procedure to exclude the variables that did not improve the model fit. The Akaike Information Criterion (AIC) was used to consider goodness-of-fit and number of parameters (Johnson and Omland, 2004). Within each model (Models 2–5), a backward stepwise procedure from the full model was performed to determine the most effective variable sets. Then, we compared the AIC values among all candidate models and selected the model with the lowest AIC value as the best model for explaining freezing damage in the F2 progeny. All statistical analyses in this study were conducted using R 3.1.2 (R Development Core Team, 2014).
Results
Paternity Analysis
All seven SSR markers were polymorphic. For the nSSR markers, the average number of alleles per locus (N) was 15.0, ranging from 7 for As08 to 21 for As16 and NFH15. For the cpSSR markers, the numbers of haplotypes of Pt30141, Pt30204, and Pt71936 were 15, 13, and 7, respectively. The genotypes of the 22 F1 candidate pollen parents differed from each other (see Supplemental Table). Genetic diversity statistics for the four nSSR markers are summarized for the F1 and F2 materials in Table 3. The genetic characteristics of the F2 progeny were consistent among their maternal groups. The average number of alleles in the F2 progeny was smaller than that in the F1 trees, which was caused by maternal sharing (13 out of 353 F1 trees; Table 1). In contrast, their observed heterozygosity (HO) was higher in the F2 progeny than in the F1 trees, resulting in a negative fixation index (FIS).
Paternity analysis using four nSSR markers showed that 12 F2 progeny had a father from the candidate F1 pollen parent group. By adding the results of cpSSR analysis, seven F2 progeny out of 12 were not excluded. For these seven F2 progeny, the cross-type of all of the assigned F1 fathers was L × L. There were no progeny with genotypes composed solely of maternal alleles, excluding the possibility of selfing. Moreover, most of the F2 progeny had novel alleles in comparison with the candidate F1 pollen donors. Allowing for genotyping errors, the proportions of selfing, mating between F1 trees, and mating with outside trees were 0, 8.7 (7 samples), and 91.3% (73 samples), respectively.
Autumn Freezing Test and Model Selection
Freezing damage scores in the F2 progeny ranged from 0 to 5 for treatment T1 (-15°C in Test 1) and treatment T2 (-30°C in Test 2; Figure 2). In T1, the most frequent damage scores for the progeny belonging to the L × L and H × H cross-types were 5 (30.0%) and 0 (45.2%), respectively. For the hybrid cross-types (L × H and H × L), a score of 1 was most common (26.7% for L × H and 36.7% for H × L). In T2, the most frequent damage score for the H × H cross-type was 1 (51.6%). In contrast, high damage scores (4 and 5) were often observed for the L × L, L × H, and H × L cross-types. In T3 (-30°C in Test 3), most progeny showed no damage, regardless of the cross-type (Figure 2C).
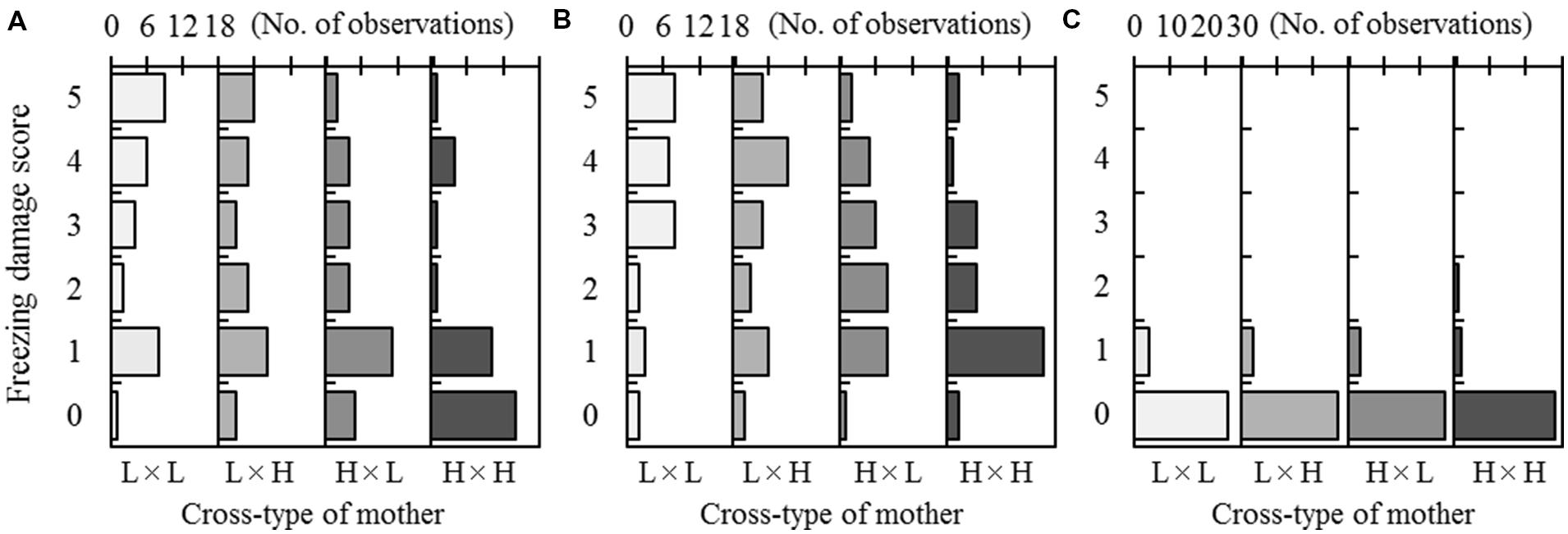
FIGURE 2. Freezing damage of F2 progeny after a freezing test at -15°C in Test 1 (A), -30°C in Test 2 (B), or -30°C in Test 3 (C). Three freezing tests were conducted in autumn at a 2-week interval. The freezing damage scores range from 5 (complete needle discoloration) to 0 (no discoloration). Observed frequencies were shown by arraying the cross-type of each mother (on the lower x-axis). The maximum scale of the number of observations (on the top x-axis) was set to 18 for each of the four cross-types in Tests 1 and 2, and set to 30 for each of the four cross-types in Test 3.
The ANOVA indicated a significant difference among mother trees within cross-types [M (C)] for treatment T1 (Table 4). For treatment T2, a significant difference was detected among maternal cross-types (C), but not among mother trees [M (C)]. For treatment T3, however, statistical analysis was not possible due to limited variation in freezing damage score, as shown in Figure 2.
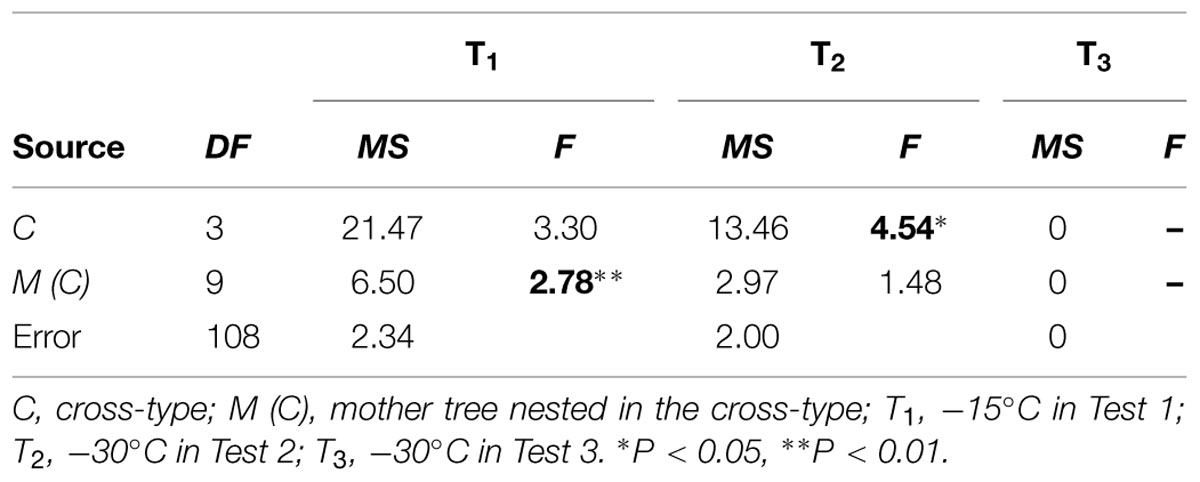
TABLE 4. ANOVA of freezing damage in the F2 progeny for each test condition, including degrees of freedom (DF), mean square (MS) and F-value (F).
Model selection for each of the four candidate models (Models 2–5) indicated that the interaction terms C × T and M (C) × T should be excluded from Models 2, 3, and 5 (Table 5). In Model 4, the C effect was also excluded. This indicates the effect of the mother trees assumed in Model 4 (i.e., non-hybrid vs. hybrid) was not useful for predicting freezing damage in the F2 progeny. A model comparison based on AIC values revealed that Model 2, which included the cross-type linear effect, was the best model (Table 5). The effects included in this model were C, T, and M (C). The estimated coefficients of these variables are shown in Table 6. A significant negative effect was observed for C, indicating that the freezing damage tended to be lower in the progeny that inherited a higher proportion of the high-elevation genome.
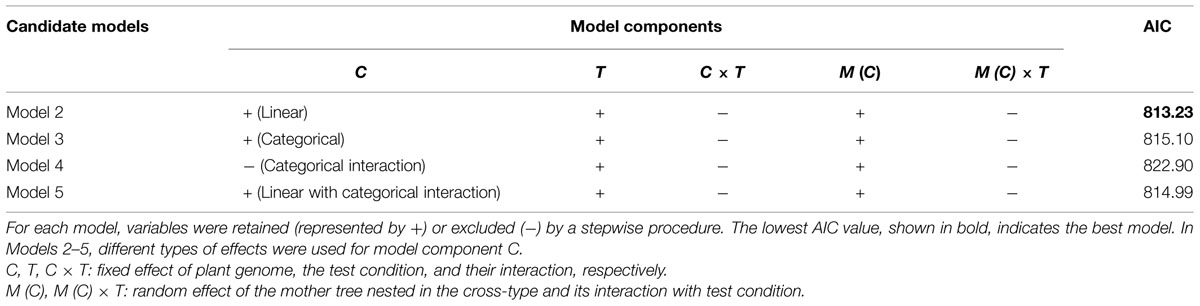
TABLE 5. Candidate model components and Akaike Information Criterion (AIC) values after model selection.
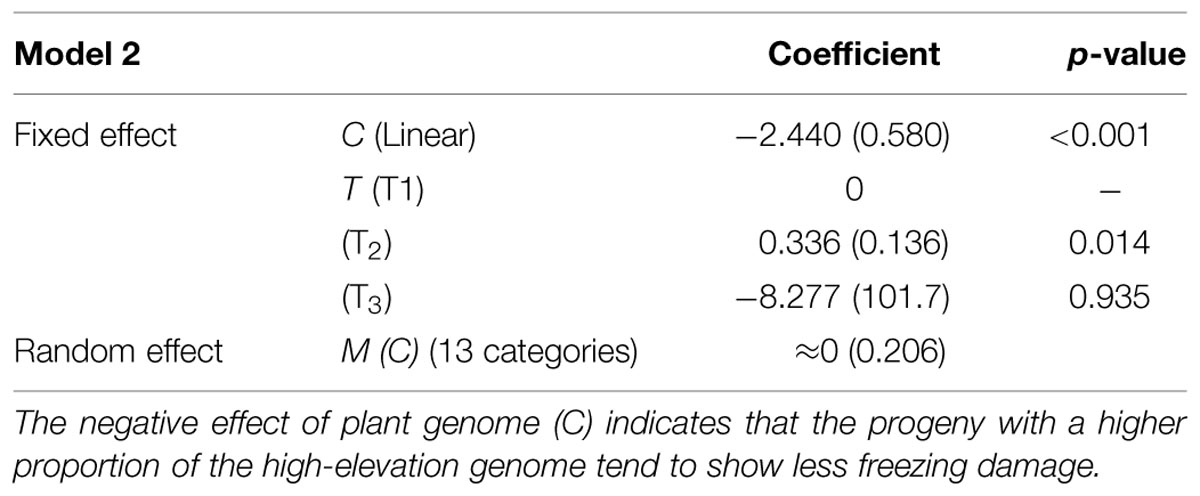
TABLE 6. Estimated parameters for Model 2, the best model among all candidate models (Models 2–5) for predicting freezing damage in the F2 progeny (standard errors are in parentheses).
Discussion
Freezing damage differed among seedlings of A. sachalinensis early in the study period, but was barely observed in the final test conducted in November (Figure 2). This demonstrates the development of freezing tolerance over time. In addition, freezing damage differed among maternal cross-types of the F2 progeny, even though the mother trees were grown in the same environment (Figure 2). Genetic variation in autumn phenology was relatively clear. Seedlings that inherited a greater proportion of the high-elevation genome displayed earlier cold acclimation. We used visual scoring, but alternative quantitative evaluations (e.g., by chlorophyll fluorescence or electrolytic leakage) are also used as indicators of cold hardiness (Lindgren and Hallgren, 1993; Ehlert and Hincha, 2008). Although quantitative evaluation is a powerful tool, the accuracy and efficiency of these alternative measures may be compromised if the plants have small leaves. In the present study, most seedlings developed thin needles, making a quantitative measurement difficult. In contrast, discoloration occurred evenly, making differences in freezing damage clear and comparable among seedlings. In addition, there is a strong correlation (r = 0.762) between our scoring method and chlorophyll fluorescence (evaluated by the Fv/Fm value) in A. sachalinensis (Ishizuka et al., 2015). Thus, our evaluation method is sufficient for detecting phenotypic variation associated with cold acclimation in this species.
In studies of other boreal conifers, genetic variation in autumn phenology and associations with the climate of origin indicated adaptation to the local climate (Rehfeldt, 1989; Skrøppa and Magnussen, 1993; Oleksyn et al., 1998; Savolainen et al., 2004; Notivol et al., 2007; Mimura and Aitken, 2010). In our previous study using reciprocally transplanted materials of A. sachalinensis, we found clinal variation in the timing of autumn cold acclimation along an elevational gradient (Ishizuka et al., 2015). Here, we further evaluated the effects of genetic background of the trees using molecular markers and statistical models to improve our understanding of the local adaptation and the evolution of autumn cold acclimation.
Paternity Analysis
The combined use of nSSR and cpSSR markers made it possible to assign pollen parents to A. sachalinensis seedlings. We assigned 22 candidate F1 pollen parents to the F2 progeny with consideration for genotyping error and assignment confidence (Slavov et al., 2005). We assumed that cryptic gene flow had little effect because the genetic origin of the F1 trees was different from that of the artificial forests surrounding the F1 planting site. Therefore, when the paternity analysis found no possible fathers among the candidates, we assumed that the pollen parent was outside of the F1 planting site, and presumably derived from the surrounding mature plantations.
The small paternal contribution from the F1 trees (8.7%) indicated a high level of pollen flow from outside pollen sources (91.3%). High levels of pollen contamination have been reported to be a severe problem in seed orchard management (Wheeler and Jech, 1992). When a seed orchard is young, the proportion of outside pollen flow tends to be extremely high. For example, Ozawa et al. (2009) performed paternity analysis of collected seeds in a young Pinus densiflora seed orchard, and detected 82% outside pollen flow. The results of that study suggested that the amount of pollen brought to the female strobili from the male strobili within the seed orchard was markedly lower than that of immigrant pollen brought from surrounding mature forests. In the present study, only 6.2% of the F1 parents were flowering due to their young age (29 years old) and small size (Table 1). As suggested by Ozawa et al. (2009), the amount of pollen from the F1 planting site must be lower than that from the surrounding mature plantations. Therefore, the results obtained by paternity analysis are reasonable.
If the timing of pollen shed from surrounding plantations and the receptivity of female strobili of the F1 trees are mismatched, outside pollination will occur rarely. Indeed, Sasaki (1983) reported that the flowering phenology of A. sachalinensis at the plantation forest at 1100 m was delayed by approximately 2 weeks compared with that at 530 m. If the difference in flowering phenology is genetically controlled, the timing of female flowering of F1 trees derived from a crossing among high-elevation parents may differ from that of male flowering of local (low-elevation) trees. However, the flowering phenology of the F1 trees which were planted at the same environment overlapped, as described by Goto et al. (2011). These results suggest that environmental factors, rather than ontogenetic control, strongly affected the flowering phenology of A. sachalinensis (Sasaki, 1983; Goto et al., 2011). Therefore, it is not surprising that outside pollen flow was predominant at our F1 planting site.
Evidence of frequent outcrossing was also seen in the genetic parameters obtained using nSSRs. Observed heterozygosity (HO) increases and the fixation index (FIS) decreases when mating occurs between local and non-local populations (Hamrick and Godt, 1996). In our study, HO of the F2 was higher than that of natural stands (Lian et al., 2008; Table 2). A large decrease in FIS was also detected between the F1 and F2 generations, although there were only small differences within the F2 (Table 2). The negative FIS in F2 indicated that the chances of mating between an F1 mother and its relatives were low. These results support our conclusions concerning pollen flow from outside the F1 planting site.
We concluded that only a few F1 fathers contributed to the F2 progeny because of the high levels of pollen flow from the surrounding mature plantations. Moreover, the F1 trees that were assigned as fathers (seven progeny) were all derived from the L × L cross-type. This indicates that the high-elevation plant genome from the F1 population was not inherited by the F2 population to any great degree.
Autumn Freezing Test and Model Selection
Our modeling analysis revealed that the regulation of autumn cold acclimation could be well explained by the linear effect of the genome origin. The model using the expected proportion of high-elevation genome as a fixed effect was selected as the best model (Table 5). In this model, variation in autumn cold acclimation was explained by variation in the maternal genome composition. The F2 progeny that inherited a greater proportion of the high-elevation genome showed earlier cold acclimation.
Because of epigenetic phenomena, such as maternal effects and ‘after’ effects, the prediction of phenotypic values for quantitative traits can be a complex process (Howe et al., 2003; Johnsen et al., 2005; Kvaalen and Johnsen, 2008; Cané-Retamales et al., 2011). In some cases, the performance of the progeny is subjected to a significant epigenetic effect (Johnsen et al., 2005; Kvaalen and Johnsen, 2008). An experiment with Picea abies revealed that autumn phenological traits (date of bud set) was related to the daylength and/or thermal conditions during fertilization and seed maturation (Johnsen et al., 2005). Moreover, the performance of the progeny may be influenced by phenomena related to epistasis (Sykes et al., 2006). Sykes et al. (2006) used the crossbred progeny derived from a specific combination of crosses in P. taeda, and successfully measured the effects of genomic interactions in the chemical contents of woody materials. In the present study, however, the model analysis indicated that epigenetic phenomena and the cross-type-dependent maternal effect were not likely to have played a major role (Table 5). In comparison with models considering such effects, the model including only the effect of genome origin showed the best fit. This result could be partly explained by the fact that all maternal trees were exposed to the same environmental conditions. Moreover, in the absence of a specific cross-type effect, it would be reasonable to expect that the autumn cold acclimation of A. sachalinensis would show a linear pattern based on the proportion of low-elevation and high-elevation plant genomes.
This interpopulational regulation of autumn phenology may be one of the factors driving the local adaptation of conifers (Aitken and Hannerz, 2001; Howe et al., 2003). For A. sachalinensis, clinal variation in the timing of autumn cold acclimation along an elevational gradient is one of the elevation-dependent adaptive traits. Each population adapts to the local climate, with a trade-off between the avoidance of freezing damage and extension of the growth period (Ishizuka et al., 2015). The genome-based control of phenological traits may be the mechanism responsible for the elevation-dependent adaptation of this species.
The genetic control of ecologically relevant traits is important in forest management. Potential genetic changes in future generations must be taken into consideration, particularly considering projections of rapid global warming (Jump and Penuelas, 2005; Aitken et al., 2008; Ishizuka and Goto, 2012). If a target species shows strong genetic variation that is larger than the phenotypic plasticity, it may be important to redistribute the genes that confer optimal performance in the changing climate (Aitken et al., 2008). For A. sachalinensis, it may be difficult to track rapid climate change because of the mismatch of locally adapted autumn phenology. However, the presence of adaptive genes and their potential for climatic adaptation have not been sufficiently examined. Future studies may be needed to evaluate this subject in detail by applying powerful molecular techniques, such as the analysis of quantitative trait loci and/or genome scanning.
Conflict of Interest Statement
The authors declare that the research was conducted in the absence of any commercial or financial relationships that could be construed as a potential conflict of interest.
Acknowledgment
The authors are deeply grateful to Glenn Howe for critical comments on the manuscript.
Supplementary Material
The Supplementary Material for this article can be found online at: http://journal.frontiersin.org/article/10.3389/fpls.2015.00890
References
Aitken, S. N., and Hannerz, M. (2001). “Genecology and gene resource management strategies for conifer cold hardiness,” in Conifer Cold Hardiness, eds F. J. Bigras and S. J. Columbo (Dordrecht: Kluwer Academic Publishers), 23–53.
Aitken, S. N., Yeaman, S., Holliday, J. A., Wang, T. L., and Curtis-McLane, S. (2008). Adaptation, migration or extirpation: climate change outcomes for tree populations. Evol. Appl. 1, 95–111. doi: 10.1111/j.1752-4571.2007.00013.x
Cané-Retamales, C., Mora, F., Vargas-Reeve, F., Perret, S., and Contreras-Soto, R. (2011). Bayesian threshold analysis of breeding values, genetic correlation and heritability of flowering intensity in Eucalyptus cladocalyx under arid conditions. Euphytica 178, 177–183. doi: 10.1007/s10681-010-0292-y
Christensen, R. H. B. (2014). Ordinal – Regression Models for Ordinal Data. R Package Version 2015.1-21. Available at: https://www.cran.r-project.org/package=ordinal/ [Accessed February 15, 2015].
Chuine, I. (2010). Why does phenology drive species distribution? Philos. Trans. R. Soc. B Biol. Sci. 365, 3149–3160. doi: 10.1098/rstb.2010.0142
Ehlert, B., and Hincha, K. D. (2008). Chlorophyll fluorescence imaging accurately quantifies freezing damage and cold acclimation responses in Arabidopsis leaves. Plant Methods 4, 12. doi: 10.1186/1746-4811-4-12
Eiga, S., and Sakai, A. (1984). Altitudinal variation in freezing resistance of Saghalien fir (Abies sachalinensis). Can. J. Bot. 62, 156–160. doi: 10.1139/b84-025
Fujikawa, S., Ukaji, N., Nagao, M., Yamane, K., Takezawa, D., and Arakawa, K. (2006) “Functional role of winter-accumulating proteins from mulberry tree in adaptation to winter-induced stresses,” in Cold Hardiness in Plants: Molecular Genetics, Cell Biology and Physiology, eds T. H. H. Chen, M. Uemura, and S. Fujikawa (Oxfordshire: CABI Publishers), 181–202.
Goto, S., Iijima, H., Ogawa, H., and Ohya, K. (2011). Outbreeding depression caused by intraspecific hybridization between local and nonlocal genotypes in Abies sachalinensis. Restor. Ecol. 19, 243–250. doi: 10.1111/j.1526-100X.2009.00568.x
Hamrick, J. L., and Godt, M. J. W. (1996). Effects of life history traits on genetic diversity in plant species. Philos. Trans. R. Soc. Lon. B Biol. Sci. 351, 1291–1298. doi: 10.1098/rstb.1996.0112
Hansen, O. K., Vendramin, G. G., Sebastiani, F., and Edwards, K. J. (2005). Development of microsatellite markers in Abies nordmanniana (Stev.) Spach and cross-species amplification in the Abies genus. Ecol. Notes 5, 784–787. doi: 10.1111/j.1471-8286.2005.01062.x
Howe, G. T., Aitken, S. N., Neale, D. B., Jermstad, K. D., Wheeler, N. C., and Chen, T. H. H. (2003). From genotype to phenotype: unraveling the complexities of cold adaptation in forest trees. Can. J. Bot. 81, 1247–1266. doi: 10.1139/b03-141
Igarashi, Y. (1996). A late glacial climatic reversion in Hokkaido, northeast Asia, inferred from the Larix pollen record. Quat. Sci. Rev. 15, 989–995. doi: 10.1016/S0277-3791(96)00005-4
Ishizuka, W., and Goto, S. (2012). Modeling intraspecific adaptation of Abies sachalinensis to local altitude and responses to global warming, based on a 36-year reciprocal transplant experiment. Evol. Appl. 5, 229–244. doi: 10.1111/j.1752-4571.2011.00216.x
Ishizuka, W., Ono, K., Hara, T., and Goto, S. (2015). Use of intraspecific variation in thermal responses for estimating an elevational cline in the timing of cold hardening in a sub-boreal conifer. Plant Biol. 17, 177–185. doi: 10.1111/plb.12214
Johnsen,Ø, Dæhlen, O. G., Østreng, G., and Skrøppa, T. (2005). Daylength and temperature during seed production interactively affect adaptive performance of Picea abies progeny. New Phytol. 168, 589–596. doi: 10.1111/j.1469-8137.2005.01538.x
Johnson, J. B., and Omland, K. S. (2004). Model selection in ecology and evolution. Trends Ecol. Evol. 19, 101–108. doi: 10.1016/j.tree.2003.10.013
Jump, A. S., and Penuelas, J. (2005). Running to stand still: adaptation and the response of plants to rapid climate change. Ecol. Lett. 8, 1010–1020. doi: 10.1111/j.1461-0248.2005.00796.x
Kalinowski, S. T., Taper, M. L., and Marshall, T. C. (2007). Revising how the computer program CERVUS accommodates genotyping error increases success in paternity assignment. Mol. Ecol. 16, 1099–1106. doi: 10.1111/j.1365-294X.2007.03089.x
Kato, R. (1952). The vegetation of the Tokyo University Forest in Hokkaido (in Japanese with English summary). Bull. Tokyo Univ. For. 43, 1–18.
Kozlowski, T. T., and Pallardy, S. G. (2002). Acclimation and adaptive responses of woody plants to environmental stresses. Bot. Rev. 68, 270–334. doi: 10.1663/0006-8101(2002)068[0270:AAAROW]2.0.CO;2
Kurahashi, T. (1995). Growth pattern of the F1 trees derived from reciprocal crosses between low and high elevation populations in Abies sachalinensis. Rep. JSPS KAKENHI 04806020, 44–49.
Kurahashi, A., and Hamaya, T. (1981). Variation of morphological characters and growth response of Sakhalin fir (Abies sachalinensis) in different altitude (in Japanese with English summary). Bull. Tokyo Univ. For. 71, 101–151.
Kvaalen, H., and Johnsen, Ø (2008). Timing of bud set in Picea abies is regulated by a memory of temperature during zygotic and somatic embryogenesis. New Phytol. 177, 49–59.
Lee, J. (1992). Cumulative logit modelling for ordinal response variables: applications to biomedical research. Bioinformatics 8, 555–562. doi: 10.1093/bioinformatics/8.6.555
Lian, C. L., Goto, S., and Hogetsu, T. (2007). Microsatellite markers for Sachalin fir (Abies sachalinensis Masters). Mol. Ecol. Notes 7, 896–898. doi: 10.1111/j.1471-8286.2007.01741.x
Lian, C. L., Goto, S., Kubo, T., Takahashi, Y., Nakagawa, M., and Hogetsu, T. (2008). Nuclear and chloroplast microsatellite analysis of Abies sachalinensis regeneration on fallen logs in a subboreal forest in Hokkaido. Jpn. Mol. Ecol. 17, 2948–2962. doi: 10.1111/j.1365-294X.2008.03802.x
Liepelt, S., Kuhlenkamp, V., Anzidei, M., Vendramin, G. G., and Ziegenhagen, B. (2001). Pitfalls in determining size homoplasy of microsatellite loci. Mol. Ecol. Notes 1, 332–335. doi: 10.1046/j.1471-8278.2001.00085.x
Lindgren, K., and Hallgren, J. E. (1993). Cold acclimation of Pinus contorta and Pinus sylvestris assessed by chlorophyll fluorescence. Tree Physiol. 13, 97–106. doi: 10.1093/treephys/13.1.97
Mimura, M., and Aitken, S. N. (2010). Local adaptation at the range peripheries of Sitka spruce. J. Evol. Biol. 23, 249–258. doi: 10.1111/j.1420-9101.2009.01910.x
Nakata, M., Tanaka, H., and Yagi, H. (1994). Altitudinal changes in vegetation and soils on Mt. Dairoku, central Hokkaido, Japan (in Japanese with English summary). Jpn. J. Ecol. 44, 33–47.
Notivol, E., Garcia-Gil, M. R., Alia, R., and Savolainen, O. (2007). Genetic variation of growth rhythm traits in the limits of a latitudinal cline in Scots pine. Can. J. Forest Res. 37, 540–551. doi: 10.1139/X06-243
Oleksyn, J., Modrzynski, J., Tjoelker, M. G., Zytkowiak, R., Reich, P. B., and Karolewski, P. (1998). Growth and physiology of Picea abies populations from elevational transects: common garden evidence for altitudinal ecotypes and cold adaptation. Funct. Ecol. 12, 573–590. doi: 10.1046/j.1365-2435.1998.00236.x
Ozawa, H., Watanabe, J., Chen, H., Isoda, K., and Watanabe, A. (2009). The impact of phonological and artificial factors on seed quality in a nematode-resistant Pinus densiflora seed orchard. Silvae Genetica 58, 145–152.
Peakall, R., and Smouse, P. E. (2012). GenAlEx 6.5: genetic analysis in Excel. Population genetic software for teaching and research. Bioinformatics 28, 2537–2539.
R Development Core Team (2014). R: A Language and Environment for Statistical Computing. Vienna: R Foundation for Statistical Computing.
Rehfeldt, G. E. (1989). Ecological adaptations in Douglas-fir (Pseudotsuga menziesii var. glauca) – a synthesis. Forest Ecol. Manage. 28, 203–215. doi: 10.1016/0378-1127(89)90004-2
Saho, H., Takahashi, I., and Kurahashi, A. (1994). “Relationship between the elevation of seed collecting sites and the susceptibility of Abies sachalinensis to Scleroderris lagerbergii in Hokkaido, Japan,” in Proceedings of a Joint Meeting of the IUFRO Working Party, Canker and Shoot Blight of Conifers, Vallombrosa, 224–247.
Sasaki, T. (1983). Phenology of forest trees and temperature in central Hokkaido, Japan (Japanese title is translated in English by authors). Shinrin Bunka Kenkyu 4, 77–86.
Savolainen, O., Bokma, F., Garcia-Gil, R., Komulainen, P., and Repo, T. (2004). Genetic variation in cessation of growth and frost hardiness and consequences for adaptation of Pinus sylvestris to climatic changes. Forest Ecol. Manage. 197, 79–89. doi: 10.1016/j.foreco.2004.05.006
Skrøppa, T., and Magnussen, S. (1993). Provenance variation in shoot growth components of Norway spruce. Silvae Genet. 42, 111–120.
Slavov, G. T., Howe, G. T., and Adams, T. (2005). Pollen contamination and mating patterns in a Douglas-fir seed orchard as measured by simple sequence repeat markers. Can. J. Forest Res. 35, 1592–1603. doi: 10.1139/x05-082
Sykes, R., Li, B., Isik, F., Kadla, J., and Chang, H. M. (2006). Genetic variation and genotype by environment interactions of juvenile wood chemical properties in Pinus taeda L. Ann. For. Sci. 63, 897–904. doi: 10.1051/forest:2006073
Toyooka, H., Sato, M., and Ishizuka, S. (1983). Distribution Map of the Sasa Group in Hokkaido, Explanatory Note (in Japanese). Sapporo: Forestry and Forest Products Research Institute, Hokkaido Branch, 36.
Tsumura, Y., and Suyama, Y. (1998). Differentiation of mitochondrial DNA polymorphisms in populations of five Japanese Abies species. Evolution 52, 1031–1042. doi: 10.2307/2411234
Vendramin, G. G., Lelli, L., Rossi, P., and Morgante, M. (1996). A set of primers for the amplification of 20 chloroplast microsatellites in Pinaceae. Mol. Ecol. 5, 595–598. doi: 10.1046/j.1365-294X.1996.00111.x
Vendramin, G. G., and Ziegenhagen, B. (1997). Characterization and inheritance of polymorphic plastid microsatellites in Abies. Genome 40, 857–864. doi: 10.1139/g97-811
Keywords: cold acclimation, interpopulation variation, elavational cline, phenology, Abies sachalinensis, modeling, paternity analysis
Citation: Ishizuka W, Ono K, Hara T and Goto S (2015) Influence of low- and high-elevation plant genomes on the regulation of autumn cold acclimation in Abies sachalinensis. Front. Plant Sci. 6:890. doi: 10.3389/fpls.2015.00890
Received: 31 August 2014; Accepted: 07 October 2015;
Published: 21 October 2015.
Edited by:
Glenn Thomas Howe, Oregon State University, USAReviewed by:
Jason Holliday, Virginia Polytechnic Institute and State University, USAAnne Y. Fennell, South Dakota State University, USA
Copyright © 2015 Ishizuka, Ono, Hara and Goto. This is an open-access article distributed under the terms of the Creative Commons Attribution License (CC BY). The use, distribution or reproduction in other forums is permitted, provided the original author(s) or licensor are credited and that the original publication in this journal is cited, in accordance with accepted academic practice. No use, distribution or reproduction is permitted which does not comply with these terms.
*Correspondence: Wataru Ishizuka, d2F0YXJ1LmlzaGlAZ21haWwuY29t