- 1State Key Laboratory of Vegetation and Environmental Change, Institute of Botany, Chinese Academy of Sciences, Beijing, China
- 2Department of Life Science, University of Chinese Academy of Sciences, Beijing, China
- 3Chinese Academy of Meteorological Sciences, Beijing, China
Climate change often induces shifts in plant functional traits. However, knowledge related to sensitivity of different functional traits and sensitive indicator representing plant growth under hydrothermal change remains unclear. Inner Mongolia grassland is predicted to be one of the terrestrial ecosystems which are most vulnerable to climate change. In this study, we analyzed the response of four zonal Stipa species (S. baicalensis, S. grandis, S. breviflora, and S. bungeana) from Inner Mongolia grassland to changing temperature (control, increased 1.5, 2, 4, and 6°C), precipitation (decreased 30 and 15%, control, increased 15 and 30%) and their combined effects via climate control chambers. The relative change of functional traits in the unit of temperature and precipitation change was regarded as sensitivity coefficient and sensitive indicators were examined by pathway analysis. We found that sensitivity of the four Stipa species to changing temperature and precipitation could be ranked as follows: S. bungeana > S. grandis > S. breviflora > S. baicalensis. In particular, changes in leaf area, specific leaf area and root/shoot ratio could account for 86% of the changes in plant biomass in the four Stipa species. Also these three measurements were more sensitive to hydrothermal changes than the other functional traits. These three functional indicators reflected the combination of plant production capacity (leaf area), adaptive strategy (root/shoot ratio), instantaneous environmental effects (specific leaf area), and cumulative environmental effects (leaf area and root/shoot ratio). Thus, leaf area, specific leaf area and root/shoot ratio were chosen as sensitive indicators in response to changing temperature and precipitation for Stipa species. These results could provide the basis for predicting the influence of climate change on Inner Mongolia grassland based on the magnitude of changes in sensitive indicators.
Introduction
Global warming that is caused by increased concentrations of CO2 and other greenhouse gases is likely to increase global mean temperature by 1.0–3.7°C at the end of this century based on the most recent projections (IPCC, 2013). Meanwhile, global warming is projected to shift precipitation patterns and exacerbate extreme climate events (Tramblay et al., 2012). Consequently, plant growth, distribution, adaptive strategy and productivity (Sherry et al., 2008; Xu et al., 2013, 2014; Maréchaux et al., 2015), even the structure and function of ecosystem (Bai et al., 2004; Albert et al., 2011; Craine et al., 2012; Wilcox et al., 2015), have been dramatically affected by global warming. Therefore, taking adaptive countermeasures has become a global focus (IPCC, 2013; Palumbi et al., 2014). But if we want to scientifically implement adaptive strategies, we must firstly understand the effects of global warming on plants at every level (physiological ecology, structure, and function, etc.), and then determine how global warming affects plants. Meanwhile we urgently need to examine sensitivity degree of different plants to climate change and determine how to create and use a sensitivity index reflecting whole plant growth (Brunner et al., 2015). These analyses could provide a scientific basis for quantitatively evaluating the responses of terrestrial ecosystems to climate change and to mitigating that change effectively.
Temperature and precipitation are important climatic factors related to plant growth, but changes in those factors result in various effects on many plant functional traits (Xu et al., 2013; Springate and Kover, 2014; Maréchaux et al., 2015). In general, changes in temperature and precipitation mainly affect plants' production capacity and adaptive strategies. For example, moderate warming could enhance plants' production capacity, including plant development and biomass accumulation (Wu et al., 2011; Xu et al., 2013). However, warming also suppresses total production and root production of some herbaceous plants (Hoeppner and Dukes, 2012) or has little effect on plant biomass of Caragana microphylla (Xu et al., 2014). Mild drought has no significant effect on plant growth, but extreme drought would reduce plant height (PH), leaf area (LA) or photosynthetic ability, and even inhibits a plant's production capacity (Mumson et al., 2013; Sapeta et al., 2013). Additionally, a plant often shifts its adaptive strategies in response to the limitations produced by temperature or precipitation to maintain growth (Yang et al., 2010; Comas et al., 2013). For example, early-blooming plant species could prolong their growing season under night warming in semi-arid grasslands (Xia and Wan, 2012), some plants increase their root/shoot ratio (R/S) in response to decreased temperatures in Inner Mongolian grassland (Fan et al., 2009), and some plants also increase the ratio of root biomass or decreased the ratio of leaf and stem biomass to allocate more resources to roots under water deficit conditions (Yin et al., 2005; Guo et al., 2007). Increases in root/leaf ratio and specific leaf area (SLA) were also found in Populus tremula and Caragana microphylla to maintain its individual moisture balance and individual growth, especially under extreme drought (Yin et al., 2005; Xu et al., 2014).
However, the combined effects of temperature and precipitation on a plant are different from their individual effects. Numerous studies have found that sensitivity of plants to high temperature could be improved, and their tolerance to warming stress would be reduced by drought (Xu and Zhou, 2006; Hoeppner and Dukes, 2012). For instance, warming benefits the growth of herbaceous plants, especially leguminous plants, but seasonal variations in rainfall amount could decrease sensitivity of herbs to warming (Hopkins and Del Prado, 2007). Nighttime warming could result in a significant decrease in plant biomass, LA and SLA of Leymus chinensis; however, these adverse effects are strengthened by water deficits (Xu and Zhou, 2006). These previous studies have elucidated the effects of climate change on a large number of plant functional traits (Sherry et al., 2008; Hoeppner and Dukes, 2012), but relationship of the changes in various functional traits and which trait is relatively more vulnerable in response to climate change are neglected. Several studies have reported that plant leaves were sensitive to environmental change because leaf is the main place where photosynthesis and respiration occur (Catoni and Gratani, 2014; Salgado-Negret et al., 2015). Similarly, plants mainly shifted resource allocation to roots to maintain the individual growth ratio under drought and warming conditions (Bret-Harte et al., 2001; Xu et al., 2014). So we can see that plant leaf and root mass partitioning might respond significantly to climate change to a certain degree, but quantitative analysis for the degree of sensitivity of these functional traits in response to temperature and precipitation remains unknown. Currently only a limited suite of countermeasures are available to develop adaptive strategies of plant species as they relate to climate change. As a consequence, a better understanding of which trait is more sensitive and indicates the response of whole plant growth to climate change can provide new insights into how plants adapt to a changing world.
The temperate grasslands of Inner Mongolia, China are an important part of the Eurasian grassland biome. These grasslands support diverse plant and animal species and play important roles in providing ecological services and socio-economic development of the region (Kang et al., 2007). Temperatures have increased substantially in this region during the past 50 years (Wan et al., 2009) and summer precipitation is predicted to increase or decrease in different grassland types (Cholaw et al., 2003). Recently numerous studies also indicated that dominant plant species in Inner Mongolia have faced species alteration and degradation in recent years (Luo et al., 2012; Zhang et al., 2013). Therefore, this region has already been documented as being very sensitive and relatively fragile to global climate change (Niu and Wan, 2008; Sui et al., 2013). Stipa species serve as the dominant plants of zonal grassland communities and present an ecological alternating distribution along a changing precipitation gradient from east to west in Inner Mongolia. This distribution reflects local plant-environment interactions that demonstrate the various adaptabilities of Stipa species to environmental change over a long evolutionary time scale. Of these species, S. baicalensis, S. grandis, S. breviflora, and S. bungeana are the dominant species in the arid and semiarid grassland of Inner Mongolia and are mainly distributed in meadow steppe, typical steppe, desert steppe and warm-temperate typical steppe, respectively (Qi et al., 2010; Hu et al., 2013, 2014). These four Stipa species distribute in various regions in Inner Mongolia grassland with different demand of temperature and precipitation, their sensitivity degree to hydrothermal changes are not well understood.
Plant functional traits represent the internal or external characteristics that plants adapt to and interact with the environment. Changes in plant functional traits can express the sensitivity of plants and ecosystem in response to the external environment (Craine et al., 2002; de Bello et al., 2010; Salgado-Negret et al., 2015). Several studies have also indicated that function and structure of grassland ecosystems are more susceptible to the responses of dominant plants to climate change (Hou et al., 2013; Xu et al., 2014; Yuan et al., 2015). Therefore, using climate control chambers, the main objective of this study was to examine sensitivity of different plant functional traits in response to temperature and precipitation change. This study analyzed those traits in four dominant Stipa species (S. baicalensis, S. grandis, S. breviflora, and S. bungeana) from the grasslands of Inner Mongolia. There are three hypotheses were tested: (1) as decrease in water can strengthen the adverse effects of warming to some plant growth (Xu and Zhou, 2006; Hopkins and Del Prado, 2007; Hoeppner and Dukes, 2012), the sensitivity of plant functional traits in response to temperature may be enhanced by changes in precipitation to a certain degree, and vice versa; (2) Previous studies have shown that S. baicalensis is dominant species in arid and semiarid grassland of Inner Mongolia and mainly distributes in meadow steppe with sufficient precipitation and stable growth, S. grandis and S. breviflora respectively distribute in typical steppe and desert steppe with developed root system, S. bungeana is a constructive species in warm-temperate typical steppe with a relative scarce of precipitation and a warm climate and has a higher demand of the cooperative effects of hydrothermal factors (Qi et al., 2010; Hu et al., 2013, 2014). So among the sensitivity of the four Stipa species to hydrothermal changes, S. bungeana may be the most sensitive species, followed by S. breviflora and S. grandis, and S. baicalensis was the least sensitive species; (3) Plant leaves are sensitive to environmental change as leaf is the main place where photosynthesis and respiration occur (Catoni and Gratani, 2014; Salgado-Negret et al., 2015), and plants primarily maintain individual growth via altering root mass partition under drought and warming conditions (Bret-Harte et al., 2001; Xu et al., 2014), so LA and R/S of Stipa species may be more sensitive indicators among functional traits to combined effects of temperature and precipitation in this study.
Materials and Methods
Plant Culture
Seedlings of Stipa species were used to study their functional traits in response to anticipated climate change. During the year prior to the experiment, seeds were collected from local steppe habitats in Inner Mongolia, China. Seeds of S. baicalensis, S. grandis, S. breviflora, and S. bungeana were collected from a natural meadow steppe in Hulun Buir (49°13′N, 119°45′E), a typical steppe in Xilinhot (43°38′N, 116°42′E), a desert steppe in Siziwang Banner (41°43′N, 111°52′E) and a sandy grassland in Ordos (39°50′N, 109°59′E), respectively (Table 1). Each Stipa plant was the dominant species of each respective site. Seeds from each species were sterilized in a 5% potassium permanganate solution for 8 min and then rinsed before sowing. Sowing was performed in plastic pots (10.9 cm in diameter, 9.5 cm in height, and 0.71 L in volume) with plastic film on the bottom as a seepage control measure. Each plastic pot was filled with 0.58 kg of air-dried soil that was retrieved from the local surface soil (0–30 cm) of the four corresponding plants. All pots were initially placed in a naturally illuminated glasshouse (day/night temperature of 26–28°C/18–20°C, maximum photosynthetic photon flux density of 1000 μmol·m−2.s−1) until the third leaf emerged (3 weeks after sowing). Then, the plants were transferred into climate control chambers (RXZ-500D, The Southeast Instruments Inc., Ningbo, China) and subjected to a respective 3-month warming and precipitation change treatment as described below.

Table 1. The geographical information related to seed collections for the four Stipa species with average temperature and precipitation data from June to August during 1978–2007 (30 years).
Experimental Design
The warming and precipitation change experiments were conducted at the greenhouse in the Institute of Botany, Chinese Academy Sciences, Beijing, China. Thirty-year (1978–2007) monthly average temperature and precipitation of the growing season (June, July, and August) for the four sites were used as baselines (Table 1). Based on future climate change scenarios, two factors were designed in this experiment: five temperature patterns including T0 (normal temperature, local monthly average temperature), T1.5 (C), T2.0 (C), T4.0 (C), and T6.0 (C); and five precipitation patterns, including W0 (normal precipitation, local monthly average precipitation), W−30% (W0−30%), W−15% (W0−15%), W+15% (W0+15%), and W+30% (W0+30%). For each species, five climate control chambers were used for five temperature treatments and with separate precipitation treatments within each chamber as a split plot. That is, for each temperature treatment, five different irrigation treatments were used with six replicates (six pots, each with four plants) for each species. This created 150 plots in total for each species. Precipitation was added to the pots by a sprayer at approximately 17:00 every 3 days, as described in our previous similar experiments (Xu and Zhou, 2006; Xu et al., 2014). The pots were randomly placed into each chamber, and moved stochastically within each layer (exchanging pot positions from center to edge and vice versa) every 3 days and between two layers every week to avoid the effects of an uneven distribution of environmental conditions. The pots were also transferred between the five chambers every 2 weeks to minimize any difference between growth chambers, except for desired treatments of temperature and water regimes (Qaderi et al., 2012; Xu et al., 2014).
Plant Harvest
At the end of the experiment, three categories of functional traits (morphological characteristics: PH, the number of leaves (LN), LA, biomass: aboveground biomass (AB), belowground biomass (BB), total biomass (TB), and growth index: SLA, leaf mass ratio (LAR), R/S) of each species under each treatment were measured. PH and LN of each plant were determined prior to harvest. Harvested plants were further separated into stems, roots, green leaves and dead leaves. LA per plant was measured with a WinFOLIA system for root/leaf analysis (WinRhizo, Régent Instruments, Quebec, Canada). Separated items were then oven-dried at 80°C to a constant weight to obtain biomass. AB summed from the biomass of stems, green leaves and dead leaves was added to BB to obtain total biomass. Three replicates of LA in one temperature and precipitation treatment were used to measure other physiological indices, so only three replicates of biomass within one treatment were obtained. The following plant growth indices were calculated according to Poorter (1999). SLA (m2·kg−1), LAR (m2·kg−1), and R/S were expressed as leaf area/its mass, leaf area/plant total biomass, root biomass/aboveground biomass, respectively.
Sensitivity Analysis
Sensitivity refers to the degree of response of a system to climate change; however, this response may be favorable or detrimental (IPCC, 2013). If y ecosystem is a function in a variety of exposure xi, y = f (x1, x2,…, xn) (xi is the attribute value of y in the i). The degree of sensitivity is generally regarded as the relative change of y ecosystem under the change of exposure xi within a certain range (Luers, 2005; Cariboni et al., 2007; Cui et al., 2009). Therefore, the sensitivity coefficient can be expressed as Δy/Δxi. In this study, compared to control temperature and precipitation treatment, the sensitivity of plant functional traits to changing temperature and precipitation could be regarded as the relative change of the functional traits in the unit of temperature and precipitation change. The greater the absolute value of the sensitivity coefficient, the greater the sensitivity of functional trait is to hydrothermal change. The sensitivity coefficient (SC) to changing temperature or precipitation is expressed as:
Where i is the various temperature or precipitation treatments; j is the various functional traits; r is the number of functional traits, where r = 9; m is different plant species; n is the number of temperature or precipitation treatment except for control treatment, where n = 4; Δxim is the variation of temperature or precipitation in treatment i for species m; xim is the value of temperature or precipitation in treatment i for species m; x0m is the value of temperature or precipitation in the control treatment for species m; Δyijm is the relative variation of functional trait j under treatment i for species m; yijm is the value of functional trait j under treatment i for species m; y0jm is the value of functional trait j under the control treatment for species m. SCijm is the sensitivity of functional trait j to temperature or precipitation treatment i for species m; and SCjm is the sensitivity of functional trait j for species m, SCm is the sensitivity of species m.
Similarly, SC to combined effects of temperature and precipitation is expressed as:
Where i is the various combined temperature and precipitation treatments; j is the various functional traits; m is different plant species; n is the number of combined temperature and precipitation treatment except for control treatment, where n = 16; Δgim is the variation of combined temperature and precipitation in treatment i for species m; Tim is temperature in treatment i for species m; T0m is temperature in the control treatment for species m; Pim is precipitation in treatment i for species m; P0m is precipitation in control treatment for species m; Δy′ijm is the relative variation of functional trait j under treatment i for species m; y′ijm is the value of functional trait j under treatment i for species m; y′0jm is the value of functional trait j under control combined temperature and precipitation treatment (T0W0) for species m. SC′ijm is the sensitivity of functional trait j to combined temperature and precipitation treatment i for species m; and SC′jm is the sensitivity of functional trait j to combined effects of temperature and precipitation for species m.
The average SC of morphological characteristics to combined effects of temperature and precipitation is computed as the average SC of PH, LN, and LA. Likewise, the average SC of biomass and growth index to combined effects of temperature and precipitation are respectively determined as the average SC of biomass (AB, BB, TB) and growth indices (SLA, LAR, R/S). Moreover, in all combined treatments of temperature and precipitation, we compare the maximum SC of every functional trait to determine which trait is the most sensitive to hydrothermal change in every species.
Statistical Analysis
Values of plant morphological characteristics, biomass allocation, and growth index are subjected to an ANOVA with a General Linear Model (GLM)-Full Factorial Mode to test the main effects of warming, changing precipitation and their interaction.
A pathway analysis is chosen to analyze the main indicators affecting changes in plant biomass and the direct or indirect effects of main indicators to plant biomass. Based on a multiple regression, a pathway analysis can break the correlation coefficient riy up into direct path coefficient (direct effect of independent variable to dependent variable) and indirect path coefficient (indirect effect of independent variable to dependent variable). Firstly the main indicators affecting changes in plant biomass are filtered from functional traits by a stepwise multiple linear regression analysis. Stepwise regression is a technique for choosing variables to include in a multiple regression model, which not only guarantees the validity and importance of the chosen variables but also reduces additional error introduced by the redundant variables. In stepwise regression, the variables ending up in the final equation represent the best combination of independent variables to predict the dependent variable. In this study, we make PH, LA, LN, SLA, LAR, and R/S as independent variable xm and TB as dependent variable y. Independent variable xm and dependent variable y are analyzed by stepwise multiple linear regression analysis to filter the main factors xi affecting dependent variable y. Then for independent variable xi and dependent variable y, pathway analysis defines the indirect path coefficient (Qiy) between xi and y is calculated as:
Where Qiy is indirect path coefficient of some independent variable xi to dependent variable y; Qijy is indirect path coefficient of some independent variable xi to dependent variable y via independent variable xj. Pjy is direct path coefficient between variable xj and dependent variable y, and Piy is direct path coefficient between variable xi and dependent variable y, which are standardized coefficients in linear stepwise regression. rij is simple correlation coefficient between variable xi and variable xj, and riy is simple correlation coefficient between independent variable xi and dependent variable y, which are computed by Pearson correlation analysis.
A multiple regression analysis is used to study the correlation of plant functional traits with their local temperature and precipitation for the four Stipa species. For one of the species, the average temperature and precipitation data from June to August in seed collection zone are used as independent variable and data of PH, LA, LN, AB, BB, TB, SLA, LAR, and R/S are used as dependent variable. The correlation between functional traits and temperature and precipitation and goodness of fit of multiple regressions are determined by adjusted R square. All statistical analyses are performed using SPSS 17.0 software (SPSS Inc., Chicago, IL, USA). All statistical significances were denoted at P < 0.05 unless otherwise noted.
Results
Sensitivity of Plant Functional Traits to Changing Precipitation
Changing temperature and precipitation alone significantly affected all the morphological characteristics and biomasses in the four Stipa species. However, the growth indices were affected differently (Table 2). For S. grandis and S. bungeana, changing temperature and precipitation significantly exerted interactive effects on every functional trait except for LN and PH (P = 0.100 and 0.242, df = 16). Functional traits of S. breviflora were also significantly affected by the interactive effects of changing temperature and precipitation except for AB, LN and LAR (P = 0.242, 0.178, and 0.226, df = 16). In addition, significant interactive effects occurred only in BB, TB, R/S and LAR of S. baicalensis (P-values were 0.000, df = 16, Table 2).
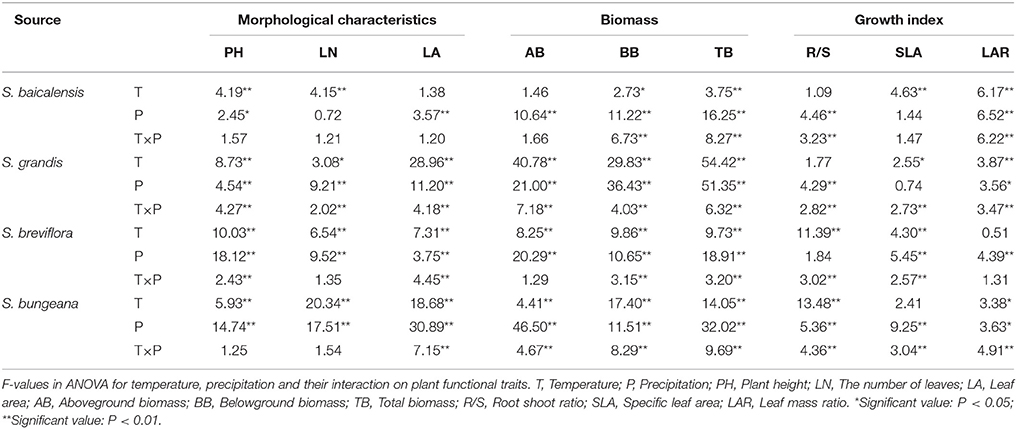
Table 2. Analysis of variance for Stipa species under different temperature and precipitation treatments.
Under different temperature treatments, sensitivity coefficients of plant functional traits to precipitation change were different in the four Stipa species. Compared to T0, increased temperature enhanced the sensitivity of functional traits to precipitation, but excessively high temperature reduced the sensitivity (Figure 1). For S. baicalensis, as temperature increased, PH, LN and AB all reached the maximum sensitivity to precipitation under T1.5 treatment, but LA and the other functional traits (BB, TB, SLA, LAR and R/S) reached the maximum SC respectively under T6.0 treatment and T2.0 treatment. For S. grandis, when temperature increased at T6.0, every functional trait (except for PH, AB, and LAR) had larger SC to precipitation than under the other temperature treatments. For S. breviflora, the traits that were the most sensitive to precipitation were LA and BB respectively under T6.0 and the other increased temperature treatments (T1.5, T2.0, T4.0). For S. bungeana, R/S had the maximum SC to precipitation under all temperature treatments (Figure 1).
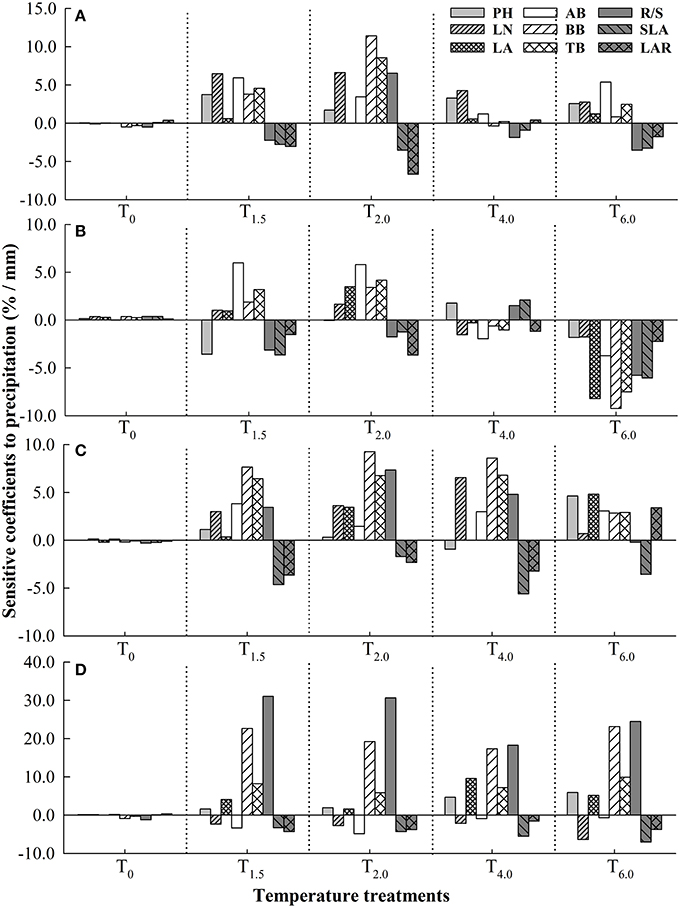
Figure 1. Sensitivity coefficients of plant functional traits to precipitation under different temperature treatments in four Stipa species. (A) S. baicalensis, (B) S. grandis, (C) S. breviflora, (D) S. bungeana. T0, T1.5, T2.0, T4.0, T6.0 denote increasing temperature by 0°C, 1.5°C, 2.0°C, 4.0°C, and 6.0°C, respectively, relative to mean temperature at the local site over 30 years (1978–2007).
Under T0 treatment, all the four species had low sensitivity to precipitation. As temperature increased, S. bungeana had larger sensitivity to precipitation than the other three species, but S. grandis was the most non-sensitive one among the four species. S. breviflora was similarly sensitive to precipitation with S. baicalensis when temperature increased slightly (T1.5, T2.0) and was more susceptible to precipitation than S. baicalensis as temperature increased largely (T4.0, T6.0; Table 3).
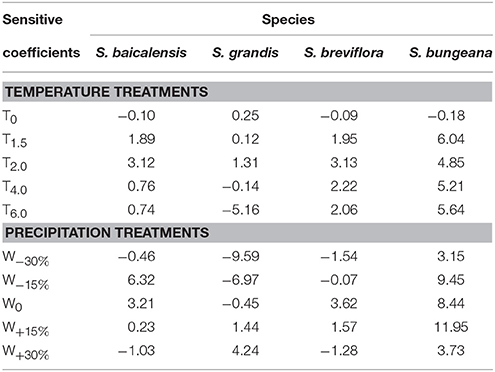
Table 3. Average sensitivity coefficients of functional traits to precipitation under different temperature treatments (%/mm) and average sensitivity coefficients of functional traits to temperature under different precipitation treatments (%/°C) in the four Stipa species.
Sensitivity of Plant Functional Traits to Changing Temperature
Increased or decreased precipitation with a certain degree strengthened sensitivity of plant functional traits to changing temperature in the four Stipa species (Figure 2). Under W0, LN, AB, BB, and R/S were respectively the most sensitive trait to changing temperature in S. baicalensis, S. grandis, S. breviflora, and S. bungeana. Changes in precipitation altered the sensitivity of plant functional traits to temperature. For S. baicalensis, R/S and LN respectively had the most sensitivity to temperature when precipitation increased and decreased greatly (W+30% and W−30%). For S. grandis and S. breviflora, BB and SLA respectively were most susceptible to temperature under increased precipitation treatments. For S. bungeana, R/S was the most sensitive trait to temperature when precipitation decreased or increased slightly, and BB had the maximum SC when precipitation significantly increased (Figure 2).
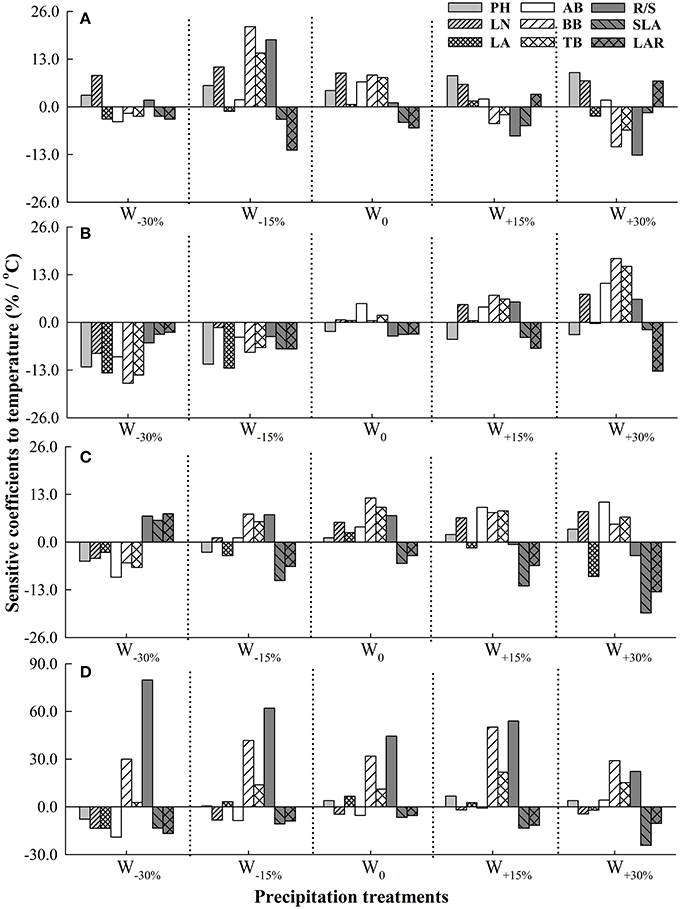
Figure 2. Sensitivity coefficients of plant functional traits to temperature under different precipitation treatments in four Stipa species. (A) S. baicalensis, (B) S. grandis, (C) S. breviflora, (D) S. bungeana. W−30%, W−15%, W0, W+15%, W+30% denote −30%, −15%, 0, +15%, and +30% of precipitation relative to mean precipitation in the local site over 30 years (1978–2007).
Under all precipitation treatments except for W+30% treatment, S. bungeana was the most sensitive one to temperature among the four species. Under W0, sensitivity of the four species to temperature was ranked as: S. bungeana > S. breviflora > S. baicalensis > S. grandis. As precipitation deceased, S. bungeana had the most sensitivity and S. grandis was the most non-sensitivity to temperature. But S. baicalensis had the minimum SC to temperature compared to other three species as precipitation increased (Table 3).
Sensitivity of Stipa Species
For all combined treatments of temperature and precipitation, the sensitivities of biomass in the four Stipa species were the maximum among all plant functional traits. The growth index was more sensitive than morphological characteristics to hydrothermal changes in S. baicalensis, but the opposite result was observed in the other three species (Figure 3). Compared to control hydrothermal treatment (T0W0), the average SC of all functional traits in the four species to hydrothermal change was ranked as: S. bungeana (0.09) > S. grandis (0.08) > S. breviflora (0.04) > S. baicalensis (−0.02).
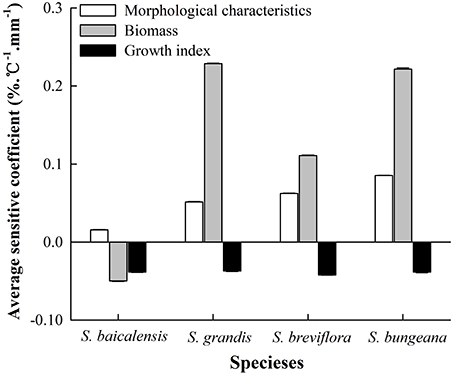
Figure 3. Average sensitivity coefficients of functional traits to combined effects of changing temperature and precipitation in the four Stipa species.
Based on adjusted R2 in multiple regression models of plant functional traits with their local average temperature and precipitation, a faint relation of functional traits with temperature and precipitation was shown in S. baicalensis (Table 4). The correlation of LN, LA, AB, and R/S with temperature and precipitation in the four species was ranked as: S. bungeana > S. grandis > S. breviflora. S. grandis represented the maximum correlation of BB, TB and LAR with temperature and precipitation among the four species (Table 4). In general, S. bungeana and S. grandis exerted relatively stronger correlation with their local temperature and precipitation compared to S. breviflora and S. baicalensis, which were similar with their sensitivity degrees. Thus, the sensitivity of Stipa species to hydrothermal change was closely related to the hydrothermal environment in their native habitats.
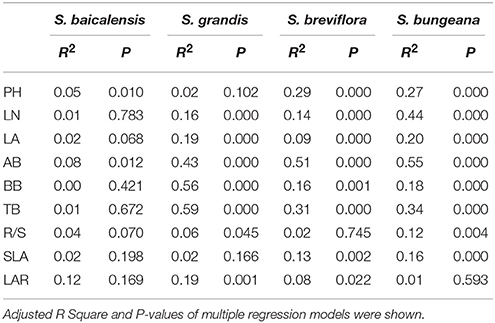
Table 4. Multiple regression analyses for plant functional traits with average temperature and precipitation data from June to August in seed collection zone of four Stipa species.
Sensitivity Indicators
Every functional trait had different sensitivity under different combined effects of temperature and precipitation. For S. baicalensis, the maximum SC of morphological characteristics, biomass and growth indices to hydrothermal changes were LN, BB, and R/S ratio, respectively (Figure 4A). LN, BB, and R/S showed significant correlations with each other, and the correlation coefficients were −0.49 (LN and BB, P = 0.000, n = 300), 0.28 (LN and R/S, P = 0.000, n = 300), and 0.13 (BB and R/S, P = 0.017, n = 300; Table 5). The sensitivity of the three traits was BB > R/S > LN. This reflected the fact that production capacity and adaptive strategy were significantly changed by changes in hydrothermal conditions; production capacity was the most sensitive.
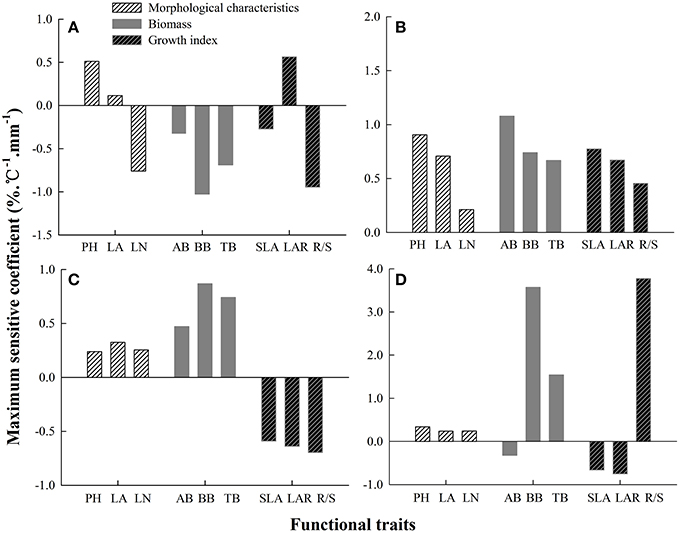
Figure 4. The maximum coefficients of the sensitivity of plant functional traits to the combined effects of changing precipitation and temperature in four Stipa species. (A) S. baicalensis, (B) S. grandis, (C) S. breviflora, (D) S. bungeana.
For S. grandis, PH, AB, and SLA were the most sensitive traits of morphological characteristics, biomass and growth indices to hydrothermal changes, respectively (Figure 4B). The sensitivity of the three traits was AB > PH > SLA. PH had significant correlation coefficient with AB (0.26) and SLA (−0.22). But AB was not significantly correlated with SLA (P = 0.216, n = 300, Table 5). Therefore, the most sensitive indicators of hydrothermal change were AB and SLA, which reflecting plants production capacity and the latter reflected adaptive strategy to the environmental changes.
For S. breviflora, the most sensitive traits of morphological characteristics, biomass and growth indices were LA, BB and R/S (Figure 4C). Their sensitivities were BB > R/S > LA. BB had significant correlation coefficients with LA (−0.58) and R/S (0.13). Production capacity and adaptive strategy were sensitive to hydrothermal changes, but production capacity was the most sensitive.
For S. bungeana, PH, BB, and R/S were the most sensitive traits of morphological characteristics, biomass and growth indices (Figure 4D). R/S was more sensitive than PH and BB. In addition, R/S was significantly correlated with PH (0.27) and BB (0.13, Table 5). Therefore, adaptive strategy (R/S) was most sensitive to hydrothermal change.
Screening in Indicating Factors of Biomass
Based on a stepwise regression analysis, LA, SLA, and R/S accounted for 86% of the variance in plant biomass of the four Stipa species. A pathway analysis showed that of the three factors, R/S had the maximum correlation coefficient with plant biomass in S. baicalensis (0.82) and S. breviflora (0.54), as well as LA in S. bungeana (0.58) and S. grandis (0.62). SLA had the maximum indirect path coefficient with plant biomass in the four Stipa species (Table 6). Additionally, LA, SLA, and R/S were correlated with the other plant functional traits (Table 5), and R/S was not significantly correlated with SLA and LA (P = 0.066 and 0.181, n = 300). Thus, LA, SLA, and R/S were representative and indicative for plant growth in the four Stipa species.
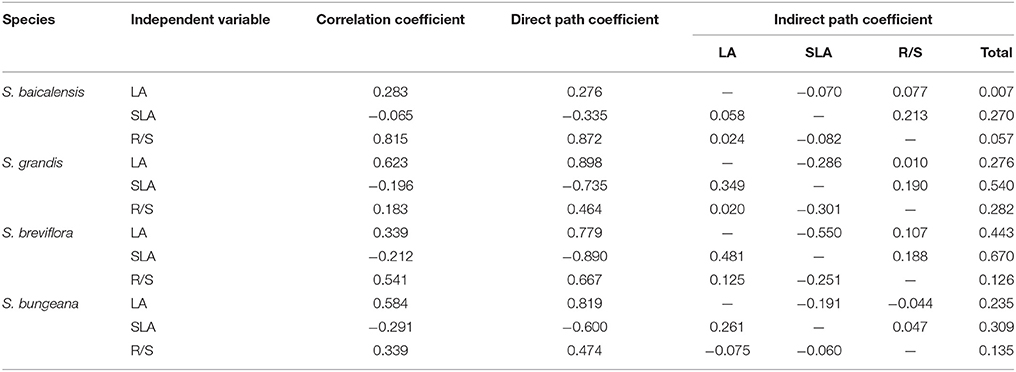
Table 6. Pathway analyses for main factors influencing plant biomass in the four Stipa species (— indicates none).
Combined with the maximum sensitivity of plant functional traits to hydrothermal change (Figure 4), the most sensitive factors indicating plant biomass were adaptive strategy (R/S) in S. baicalensis and S. bungeana, the instantaneous environmental effect (SLA) in S. grandis, and production capacity (LA) and adaptive strategy (R/S) in S. breviflora.
Discussion
Because of differences in regional climate change and each species' biological characteristics, there are remarkable species-specific responses to changing temperature and precipitation (Donovan et al., 2011; Xu et al., 2014; Mowll et al., 2015). In this study, the responses of plant functional traits to combined effects of temperature and precipitation were determined for dominant species from the grasslands of Inner Mongolia. Our findings were consistent with previous results (Xu et al., 2014; Maréchaux et al., 2015); the four Stipa species had their own individual response sensitivities to hydrothermal changes (Figure 3). Of these species, S. baicalensis mainly distributes in semi-arid and sub-humid meadow steppe with a relatively sufficient water supply (Qi et al., 2010). Compared to S. bungeana, S. breviflora, and S. grandis, the correlation of plant functional traits with average temperature and precipitation of the growing season was relatively weaker in S. baicalensis (Table 4). Simultaneously S. baicalensis was the least sensitive to hydrothermal changes with an average sensitivity coefficient of −0.2, which reflected the fact that future climate change may had a little negative effect on S. baicalensis. S. bungeana was the dominant species in warm-temperate typical steppe (Hu et al., 2013) with the relatively high demand of combined temperature and precipitation conditions (Table 4). Thus, the sensitivity of S. bungeana was the strongest among the four Stipa species (Figure 3). S. grandis was most suitable to grow in the unique environment of typical grassland. In addition, it would be replaced by S. baicalensis if the habitat conditions tended to become more mesic and by S. krylovii when environmental conditions tended to be more drought prone (Qi et al., 2010). S. breviflora mainly occurs in arid and semi-arid desert steppe and has a well-developed root system and higher drought resistance (Hu et al., 2014). Additionally, plant biomass of S. grandis had higher correlation with temperature and precipitation than S. breviflora (Table 4). Therefore, S. grandis was more vulnerable than S. breviflora in response to combined effects of temperature and precipitation (Figure 3).
Under the background of climate change, changes in temperature and precipitation are simultaneous and make combined effects on plants (IPCC, 2013; Mora et al., 2013). Numerous studies have reported that slight warming could promote plant cell division and growth, and then promote an increased photosynthetic rate and plant growth (Bret-Harte et al., 2001). However, greater warming would increase evaporation (Springate and Kover, 2014), then this would further weaken the plant's supply of precipitation and so inhibit plant growth (Xu and Zhou, 2006). Therefore, the response of plants to precipitation could be altered by changing temperature, and vice versa (Qaderi et al., 2012; Xu et al., 2014). In this study, sensitivity of plant functional traits in response to changes in precipitation was strengthened by increased temperature in a certain degree (Figure 1). Additionally, sensitivities of plant functional traits to temperature were also shifted by changing precipitation (Figure 2). For example, for S. baicalensis, BB was the most susceptible trait to temperature among plant functional traits under slightly decreased precipitation (W−15%), but LN had the maximum SC to temperature under an excessive shortage of precipitation (W−30%, Figure 2). This reflected the fact that as precipitation slightly reduced, S. baicalensis might mainly increase root cell division significantly and allocate more sources to roots to response to temperature change (Bret-Harte et al., 2001). While under drought stress, this species might choose to decrease leaf cell volume/surface area and increase LN to adapt to temperature change (Schäffner, 1998).
Plant individual biomass is the final result of photosynthesis and stands at the core of the grassland research (Ehret et al., 2015), which is not only restricted by the plant's own biological characteristics, but also greatly affected by environmental factors (Myneni et al., 1997; Zhang et al., 2011). Because plant biomass is not easy to measure, it is essential to screen various indicators which can reflect changes in plant biomass and are sensitive to environmental changes among plant functional traits. These chosen indicators are important for evaluating and predicting the response and adaptation of Stipa species and grassland ecosystems to climate change. In this study, LA, R/S, and SLA accounted for 86% of the variance in plant biomass in the four Stipa species. Moreover, these three traits had some direct and indirect correlations with plant biomass (Table 6), and they were also related with the other functional traits (Table 5). To some extent, they reflected plant production capacity (LA), adaptive strategy (R/S), instantaneous environment effects (SLA) and cumulative environmental effects (LA and R/S; Poorter, 1999; Yang et al., 2010). This partly confirmed the previous results that plant leaf and root mass partitioning were more significantly affected by temperature and precipitation (Bret-Harte et al., 2001; Catoni and Gratani, 2014; Xu et al., 2014; Salgado-Negret et al., 2015). Thus, these three traits could be used as sensitive indicators to hydrothermal change in the four Stipa species analyzed here. The most sensitive indicators representing plant biomass to hydrothermal change were adaptive strategy (R/S) in S. baicalensis and S. bungeana, leaf adaptive strategy (SLA) in S. grandis, and the cumulative environmental effects (LA and R/S) in S. breviflora (Figure 4; Table 6). This suggested that the responses of plants to hydrothermal change could not be reflected by a single indicator, but a combination of multiple indicators was needed. The responses of plants to environmental factors are complicated, so screening sensitive indicators of plants in response to environmental factors (temperature, precipitation, CO2, etc.) and estimating their adaptive thresholds require further study.
Conclusions
Based on combined effects of temperature and precipitation, the three hypotheses were partly confirmed. Increased temperature to a certain degree enhanced the sensitivity of plant functional traits in response to precipitation, and vice versa. The sensitivity of the four Stipa species with specific traits to changing temperature and precipitation varied. The sensitive indicators to hydrothermal changes were LA, SLA, and R/S in the four Stipa species. This suggests that the sensitivity of Stipa plants to hydrothermal changes should be reflected by a combination of multiple indicators. Also the responses of sensitive indicators can play a crucial role in the adaptation of Stipa species to future climate change.
Author Contributions
GZ, YW, and XL conceived and designed the research. XL, YW, and XS conducted the experiment. All authors analyzed and interpreted the data. XL and GZ wrote the manuscript; all authors discussed and approved the final version.
Funding
This study was jointly funded by Chinese Academy of Sciences “Strategic Priority Research Program-Climate Change: Carbon Budget and Relevant Issues” (XDA-05050408) and China Special Fund for Meteorological Research in the Public Interest (Major projects) (GYHY201506001-3).
Conflict of Interest Statement
The authors declare that the research was conducted in the absence of any commercial or financial relationships that could be construed as a potential conflict of interest.
The Associate Editor Jian-Guo Huang declares that, despite being affiliated with the same institute as the authors Xiaomin Lv, Guangsheng Zhou, Yuhui Wang and Xiliang Song, the review process was handled objectively.
Acknowledgments
We would like to thank Zhenzhu Xu, Hui Li, Jian Song, Yanling Jiang, Bingrui Jia, Liping Tan, Jun Chen, Tao Liu, Hui Wang, Yaohui Shi, and Zhixiang Yang for their loyal support and assistance during the experiment.
Abbreviations
PH, Plant height; LN, The number of leaves; LA, Leaf area; AB, Aboveground biomass; BB, Belowground biomass; TB, Total biomass; R/S, Root/shoot ratio; SLA, Specific leaf area; LAR, Leaf mass ratio; SC, Sensitivity coefficient.
References
Albert, K. R., Ro-Poulsen, H., Mikkelsen, T. N., Michelsen, A., van der Linden, L., and Beier, C. (2011). Effects of elevated CO2, warming and drought episodes on plant carbon uptake in a temperate heath ecosystem are controlled by soil water status. Plant Cell Environ. 34, 1207–1222. doi: 10.1111/j.1365-3040.2011.02320.x
Bai, Y. F., Han, X. G., Wu, J. G., Chen, Z. Z., and Li, L. H. (2004). Ecosystem stability and compensatory effects in the Inner Mongolia grassland. Nature 431, 181–184. doi: 10.1038/nature02850
Bret-Harte, M. S., Shaver, G. R., Zoerner, J. P., Johnstone, J. F., Wagner, J. L., Chavez, A. S., et al. (2001). Developmental plasticity allows Betula nana to dominate tundra subjected to an altered environment. Ecology 82, 18–32. doi: 10.1890/0012-9658(2001)082[0018:DPABNT]2.0.CO;2
Brunner, I., Herzog, C., Dawes, M. A., Arend, M., and Sperisen, C. (2015). How tree roots respond to drought. Front. Plant Sci. 6:511. doi: 10.3389/fpls.2015.00511
Cariboni, J., Gatelli, D., Liska, R., and Saltelli, A. (2007). The role of sensitivity analysis in ecological modelling. Ecol. Model. 203, 167–182. doi: 10.1016/j.ecolmodel.2005.10.045
Catoni, R., and Gratani, L. (2014). Variations in leaf respiration and photosynthesis ratio in response to air temperature and water availability among Mediterranean evergreen species. J. Arid Environ. 102, 82–88. doi: 10.1016/j.jaridenv.2013.11.013
Cholaw, B., Cubasch, U., Lin, Y. H., and Ji, L. R. (2003). The change of North China climate in transient simulations using the IPCCSRES A2 and B2 scenarios with a coupled atmosphere-ocean general circulation model. Adv. Atmos. Sci. 20, 755–766. doi: 10.1007/BF02915400
Comas, L. H., Becker, S. R., Cruz, V. V., Byrne, P. F., and Dierig, D. A. (2013). Root traits contributing to plant productivity under drought. Front. Plant Sci. 4:442. doi: 10.3389/fpls.2013.00442
Craine, J. M., Nippert, J. B., Elmore, A. J., Skibbe, A. M., Hutchinson, S. L., and Brunsell, N. A. (2012). Timing of climate variability and grassland productivity. Proc. Natl. Acad. Sci. U.S.A. 109, 3401–3405. doi: 10.1073/pnas.1118438109
Craine, J. M., Tilman, D., Wedin, D., Reich, P., Tjoelker, M., and Knops, J. (2002). Functional traits, productivity and effects on nitrogen cycling of 33 grassland species. Funct. Ecol. 16, 563–574. doi: 10.1046/j.1365-2435.2002.00660.x
Cui, S. H., Li, F. Y., Huang, J., and Yu, Y. X. (2009). Review of sensitivity research on the context of global change. Adv. Earth Sci. 24, 1033–1041. doi: 10.3321/j.issn:1001-8166.2009.09.010
de Bello, F., Lavorel, S., Díaz, S., Harrington, R., Cornelissen, J. H. C., Bardgett, R. D., et al. (2010). Towards an assessment of multiple ecosystem processes and services via functional traits. Biodivers. Conserv. 19, 2873–2893. doi: 10.1007/s10531-010-9850-9
Donovan, L. A., Maherali, H., Caruso, C. M., Huber, H., and De Kroon, H. (2011). The evolution of the worldwide leaf economics spectrum. Trends Ecol. Evol. 26, 88–95. doi: 10.1016/j.tree.2010.11.011
Ehret, M., Buhle, L., Grass, R., Lamersdorf, N., and Wachendorf, M. (2015). Bioenergy provision by an alley cropping system of grassland and shrub willow hybrids: biomass, fuel characteristics and net energy yields. Agroforest. Syst. 89, 365–381. doi: 10.1007/s10457-014-9773-7
Fan, J. W., Wang, K., Harris, W., Zhong, H. P., Hu, Z. M., Han, B., et al. (2009). Allocation of vegetation biomass across a climate-related gradient in the grasslands of Inner Mongolia. J. Arid Environ. 73, 521–528. doi: 10.1016/j.jaridenv.2008.12.004.
Guo, W., Li, B., Zhang, X., and Wang, R. (2007). Architectural plasticity and growth responses of Hippophae rhamnoides and Caragana intermedia seedlings to simulated water stress. J. Arid Environ. 69, 385–399. doi: 10.1016/j.jaridenv.2006.10.003
Hoeppner, S. S., and Dukes, J. S. (2012). Interactive responses of old-field plant growth and composition to warming and precipitation. Global Change Biol. 18, 1754–1768. doi: 10.1111/j.1365-2486.2011.02626.x
Hopkins, A., and Del Prado, A. (2007). Implications of climate change for grassland in Europe: impacts, adaptations and mitigation options: a review. Grass Forage Sci. 62, 118–126. doi: 10.1111/j.1365-2494.2007.00575.x
Hou, Y. H., Zhou, G. S., Xu, Z. Z., Liu, T., and Zhang, X. S. (2013). Interactive effects of warming and increased precipitation on community structure and composition in an annual forb dominated desert steppe. PLoS ONE 8:e70114. doi: 10.1371/journal.pone.0070114.t001
Hu, H. L., Liu, Y. Z., Li, Y. K., Lu, D. X., and Gao, M. (2014). Use of the N-alkanes to estimate intake, apparent digestibility and diet composition in sheep grazing on Stipa breviflora desert steppe. J. Integr. Agr. 13, 1065–1072. doi: 10.1016/S2095-3119(13)60502-X
Hu, X. W., Zhou, Z. Q., Li, T. S., Wu, Y. P., and Wang, Y. R. (2013). Environmental factors controlling seed germination and seedling recruitment of Stipa bungeana on the Loess Plateau of northwestern China. Ecol. Res. 28, 801–809. doi: 10.1007/s11284-013-1063-8
IPCC (2013). “Climate change 2013: the physical science basis,” in Contribution of Working Group I to the Fifth Assessment Report of the Intergovernmental Panel on Climate Change, eds T. F. Stocker, D. Qin, G. K. Plattner, M. Tignor, S. K. Allen, J. Boschung, et al. (New York, NY: Cambridge University Press), 3–14.
Kang, L., Han, X. G., Zhang, Z. B., and Sun, O. J. (2007). Grassland ecosystems in China: review of current knowledge and research advancement. Philos. Trans. R. Soc. B. 362, 997–1008. doi: 10.1098/rstb.2007.2029
Luers, A. L. (2005). The surface of vulnerability: an analytical framework for examining environmental change. Global Environ. Chang. 15, 214–223. doi: 10.1016/j.gloenvcha.2005.04.003
Luo, G. P., Han, Q. F., Zhou, D. C., Li, L., Chen, X., Li, Y., et al. (2012). Moderate grazing can promote aboveground primary production of grassland under water stress. Ecol. Complex. 11, 126–136. doi: 10.1016/j.ecocom.2012.04.004
Maréchaux, I., Bartlett, M. K., Sack, L., Baraloto, C., Engel, J., Joetzjer, E., et al. (2015). Drought tolerance as predicted by leaf water potential at turgor loss point varies strongly across species within an Amazonian forest. Funct. Ecol. 29, 1268–1277. doi: 10.1111/1365-2435.12452
Mora, C., Frazier, A. G., Longman, R. J., Dacks, R. S., Walton, M. M., Tong, E. J., et al. (2013). The projected timing of climate departure from recent variability. Nature 502, 183–187. doi: 10.1038/nature12540
Mowll, W., Blumenthal, D. M., Cherwin, K., Smith, A., Symstad, A. J., Vermeire, L. T., et al. (2015). Climatic controls of aboveground net primary production in semi-arid grasslands along a latitudinal gradient portend low sensitivity to warming. Oecologia 177, 959–969. doi: 10.1007/s00442-015-3232-7
Mumson, S. M., Muldavin, E. H., Brlnap, J., Peters, D. P. C., Anderson, J. P., Reiser, M. H., et al. (2013). Regional signatures of plant response to drought and elevated temperature across a desert ecosystem. Ecology 94, 2030–2041. doi: 10.1890/12-1586.1
Myneni, R. B., Keeling, C. D., Tucker, C. J., Asrar, G., and Nemani, R. R. (1997). Increased plant growth in the northern high latitudes from 1981 to 1991. Nature 386, 698–702. doi: 10.1038/386698a0
Niu, S. L., and Wan, S. Q. (2008). Warming changes plant competitive hierarchy in a temperate steppe in northern China. J. Plant Ecol. 1, 103–110. doi: 10.1093/jpe/rtn003
Palumbi, S. R., Barshis, D. J., Traylor-Knowles, N., and Bay, R. A. (2014). Mechanisms of reef coral resistance to future climate change. Science 344, 895–898. doi: 10.1126/science.1251336
Poorter, L. (1999). Growth responses of 15 rain-forest tree species to a light gradient: the relative importance of morphological and physiological traits. Funct. Ecol. 13, 396–410. doi: 10.1046/j.1365-2435.1999.00332.x
Qaderi, M. M., Kurepin, L. V., and Reid, D. M. (2012). Effects of temperature and watering regime on growth, gas exchange and abscisic acid content of canola (Brassica napus) seedlings. Environ. Exp. Bot. 75, 107–113. doi: 10.1016/j.envexpbot.2011.09.003
Qi, Y. C., Dong, Y. S., Liu, L. X., Liu, X. R., Peng, Q., Xiao, S. S., et al. (2010). Spatial-temporal variation in soil respiration and its controlling factors in three steppes of Stipa L. in Inner Mongolia, China. Sci. China Earth Sci. 53, 683–693. doi: 10.1007/s11430-010-0039-6
Salgado-Negret, B., Canessa, R., Valladares, F., Armesto, J. J., and Perez, F. (2015). Functional traits variation explains the distribution of Aextoxicon punctatum (Aextoxicaceae) in pronounced moisture gradients within fog-dependent forest fragments. Front. Plant Sci. 6:511. doi: 10.3389/fpls.2015.00511
Sapeta, H., Costa, J. M., Lourenço, T., Maroco, J., Van Der Linde, P., and Oliveira, M. M. (2013). Drought stress response in Jatropha curcas: growth and physiology. Environ. Expe. Bot. 85, 76–84. doi: 10.1016/j.envexpbot.2012.08.012
Schäffner, A. R. (1998). Aquaporin function, structure, and expression: are there more surprises to surface in water relations? Planta 204, 131–139. doi: 10.1007/s004250050239
Sherry, R. A., Weng, E., Arnone Iii, J. A., Johnson, D. W., Schimel, D. S., Verburg, P. S., et al. (2008). Lagged effects of experimental warming and doubled precipitation on annual and seasonal aboveground biomass production in a tallgrass prairie. Global Change Biol. 14, 2923–2936. doi: 10.1111/j.1365-2486.2008.01703.x
Springate, D. A., and Kover, P. X. (2014). Plant responses to elevated temperatures: a field study on phenological sensitivity and fitness responses to simulated climate warming. Globle Change Biol. 20, 456–465. doi: 10.1111/gcb.12430
Sui, X. H., Zhou, G. S., and Zhuang, Q. L. (2013). Sensitivity of carbon budget to historical climate variability and atmospheric CO2 concentration in temperate grassland ecosystems in China. Climatic Change 117, 259–272. doi: 10.1007/s10584-012-0533-2
Tramblay, Y., Badi, W., Driouech, F., El Adlouni, S., Neppel, L., and Servat, E. (2012). Climate change impacts on extreme precipitation in Morocco. Global Planet. Change 82–83, 104–114. doi: 10.1016/j.gloplacha.2011.12.002
Wan, S. Q., Xia, J. Y., Liu, W. X., and Niu, S. L. (2009). Photosynthetic overcompensation under nocturnal warming enhances grassland carbon sequestration. Ecology 90, 2700–2710. doi: 10.1890/08-2026.1
Wilcox, K. R., Von Fischer, J. C., Muscha, J. M., Petersen, M. K., and Knapp, A. K. (2015). Contrasting above- and belowground sensitivity of three Great Plains grasslands to altered rainfall regimes. Globle Change Biol. 21, 335–344. doi: 10.1111/gcb.12673
Wu, Z., Dijkstra, P., Koch, G. W., Peuelas, J., and Hungate, B. A. (2011). Responses of terrestrial ecosystems to temperature and precipitation change: a meta-analysis of experimental manipulation. Global Change Biol. 17, 927–942. doi: 10.1111/j.1365-2486.2010.02302.x
Xia, J. Y., and Wan, S. Q. (2012). The effects of warming-shifted plant phenology on ecosystem carbon exchange are regulated by precipitation in a semi-arid grassland. PLoS ONE 7:e32088. doi: 10.1371/journal.pone.0032088
Xu, Z. Z., Shimizu, H., Ito, S., Yagasaki, Y., Zou, C. J., Zhou, G. S., et al. (2014). Effects of elevated CO2, warming and precipitation change on plant growth, photosynthesis and peroxidation in dominant species from North China grassland. Planta 239, 421–435. doi: 10.1007/s00425-013-1987-9
Xu, Z. Z., Shimizu, H., Yagasaki, Y., Ito, S., Zheng, Y. R., and Zhou, G. S. (2013). Interactive effects of elevated CO2, drought, and warming on plants. J. Plant Growth Regul. 32, 692–707. doi: 10.1007/s00344-013-9337-5
Xu, Z. Z., and Zhou, G. S. (2006). Combined effects of water stress and high temperature on photosynthesis, nitrogen metabolism and lipid peroxidation of a perennial grass Leymus chinensis. Planta 224, 1080–1090. doi: 10.1007/s00425-006-0281-5
Yang, Y. H., Fang, J. Y., Ma, W. H., Guo, D. L., and Mohammat, A. (2010). Large-scale pattern of biomass partitioning across China's grasslands. Global Ecol. Biogeogr. 19, 268–277. doi: 10.1111/j.1466-8238.2009.00502.x
Yin, C. Y., Wang, X., Duan, B. L., Luo, J. X., and Li, C. Y. (2005). Early growth, dry matter allocation and water use efficiency of two sympatric Populus species as affected by water stress. Environ. Exp. Bot. 53, 315–322. doi: 10.1016/j.envexpbot.2004.04.007
Yuan, F., Wu, J. G., Li, A., Rowe, H., Bai, Y. F., Huang, J. H., et al. (2015). Spatial patterns of soil nutrients, plant diversity, and aboveground biomass in the Inner Mongolia grassland: before and after a biodiversity removal experiment. Landscape Ecol. 30, 1737–1750. doi: 10.1007/s10980-015-0154-z
Zhang, C., Li, C. F., Chen, X., Luo, G. P., Li, L. H., Li, X. Y., et al. (2013). A spatial-explicit dynamic vegetation model that couples carbon, water, and nitrogen processes for arid and semiarid ecosystems. J. Arid. Land. 5, 102–117. doi: 10.1007/s40333-013-0146-2
Keywords: Stipa species, precipitation changes, warming, sensitive indicator, Inner Mongolia grassland
Citation: Lv X, Zhou G, Wang Y and Song X (2016) Sensitive Indicators of Zonal Stipa Species to Changing Temperature and Precipitation in Inner Mongolia Grassland, China. Front. Plant Sci. 7:73. doi: 10.3389/fpls.2016.00073
Received: 26 August 2015; Accepted: 16 January 2016;
Published: 08 February 2016.
Edited by:
Jian-Guo Huang, Chinese Academy of Sciences, ChinaReviewed by:
Eryuan Liang, Institute of Tibetan Plateau Research, Chinese Academy of Sciences, ChinaSyed Ashraful Alam, University of Helsinki, Finland and South China Botanical Garden, China
Qianqian Ma, South China Botanical Garden, China
Copyright © 2016 Lv, Zhou, Wang and Song. This is an open-access article distributed under the terms of the Creative Commons Attribution License (CC BY). The use, distribution or reproduction in other forums is permitted, provided the original author(s) or licensor are credited and that the original publication in this journal is cited, in accordance with accepted academic practice. No use, distribution or reproduction is permitted which does not comply with these terms.
*Correspondence: Guangsheng Zhou, Z3N6aG91QGliY2FzLmFjLmNu