- 1Key Laboratory of the Ministry of Education for Medicinal Resources and Natural Pharmaceutical Chemistry, National Engineering Laboratory for Resource Development of Endangered Crude Drugs in Northwest of China, College of Life Sciences, Shaanxi Normal University, Xi’an, China
- 2School of Geography and Life Science, Qinghai Normal University, Xining, China
- 3Department of Life Sciences, Shaanxi XueQian Normal University, Xi’an, China
Real time quantitative PCR (RT-qPCR or qPCR) has been extensively applied for analyzing gene expression because of its accuracy, sensitivity, and high throughput. However, the unsuitable choice of reference gene(s) can lead to a misinterpretation of results. We evaluated the stability of 10 candidates – five traditional housekeeping genes (UBC21, GAPC2, EF-1α4, UBQ10, and UBC10) and five novel genes (SAND1, FBOX, PTB1, ARP, and Expressed1) – using the transcriptome data of Gentiana macrophylla. Common statistical algorithms ΔCt, GeNorm, NormFinder, and BestKeeper were run with samples collected from plants under various experimental conditions. For normalizing expression levels from tissues at different developmental stages, GAPC2 and UBC21 had the highest rankings. Both SAND1 and GAPC2 proved to be the optimal reference genes for roots from plants exposed to abiotic stresses while EF-1α4 and SAND1 were optimal when examining expression data from the leaves of stressed plants. Based on a comprehensive ranking of stability under different experimental conditions, we recommend that SAND1 and EF-1α4 are the most suitable overall. In this study, to find a suitable reference gene and its real-time PCR assay for G. macrophylla DNA content quantification, we evaluated three target genes including WRKY30, G10H, and SLS, through qualitative and absolute quantitative PCR with leaves under elicitors stressed experimental conditions. Arbitrary use of reference genes without previous evaluation can lead to a misinterpretation of the data. Our results will benefit future research on the expression of genes related to secoiridoid biosynthesis in this species under different experimental conditions.
Introduction
Gentiana macrophylla Pall. is a well-known medicinal plant in the Gentianaceae family. Its dried roots, ‘Qinjiao,’ have been used in traditional Chinese medicine for over 2000 years, usually as an ingredient in numerous formulae. The biological and pharmacological effects of Qinjiao include stomachic, choleretic, and antihepatotoxic activities (Wang and Lou, 1987; Ji et al., 2002). Secoiridoids are its dominant active constituents, especially gentiopicrin (gentiopicroside). With the development of molecular biology tools, Gentiana is now being used to study the molecular pathways of secondary metabolites and key related genes.
Improving our understanding of gene expression patterns can provide insight into complex biological processes, such as signaling and metabolic pathways (Marino et al., 2008). Quantitative real-time PCR (qPCR) is the most sensitive method for detecting both high and low levels of expression. This technique is used for clinical diagnoses, analyses of gene expression in specific tissues, and research projects that involve complex experiments and a large number of genes (Gachon et al., 2004; Nicot et al., 2005). There are mainly two kinds of qPCR assays in use: relative quantification and absolute quantification. Relative quantification compares expression of the target gene to that of one or more reference genes within the same sample. Reference genes should be consistently expressed across the samples being surveyed (Sellars et al., 2007). Absolute quantification determines the exact copy concentration of target gene by relating the cycle threshold (Ct) value to a standard curve (Yu et al., 2005). This method can accurately quantify the number of template copies in a known amount of starting sample. A set of guidelines, the Minimum Information for Publication of Quantitative Real-time PCR Experiments (MIQE) has been developed to improve the reproducibility and reliability of qPCR experiments (Bustin et al., 2009). Currently in plant research, validated and qualitative RT-qPCR protocols are still rare. Keyser et al. (2013) build the protocol can be implemented on all plant species to assure accurate quantification of gene expression.
Classic housekeeping genes that encode 18S rRNA, ubiquitin, actin, β-tubulin, and glyceraldehyde-3-phosphate dehydrogenase (GAPC or GAPDH) are commonly used as internal controls for such analyses of plants. However, those genes were chosen in the pre-genomic era because of their known or suspected roles in basic cellular processes. Although they were assumed to have uniform expression in all types of samples and under all experimental conditions (Czechowski et al., 2005), more recent examinations have demonstrated that, for many species and treatments, the expression of these genes is, in fact, highly variable in different testing environments (Czechowski et al., 2005; Jain et al., 2006). In contrast, several new reference genes with very stable expression have been identified through microarray, transcriptome, and genome-wide sequencing analyses that have focused on a range of species, e.g., humans, Escherichia coli, and Arabidopsis thaliana (hereafter, Arabidopsis; Czechowski et al., 2005; Maccoux et al., 2007; Zhou et al., 2011). Furthermore, the rapid introduction of genomes and transcriptome datasets has provided a high-throughput approach for identifying sets of novel reference genes (Zhuang et al., 2015). For example, 40 candidates have been mined from datasets for the Brassica napus transcriptome, and 14 have been selected for further qPCR analysis with different tissues and under various experimental treatments (Wang et al., 2014). Expressed sequence tag (EST) databases have been screened to find three novel reference genes and eight traditional housekeeping genes that are stably expressed in different tissues/organs and developing seeds from four cultivars of Vernicia fordii (Han et al., 2012). Transcriptome sequence data in Fagopyrum esculentum have revealed that Expressed protein of unknown function (Expressed1 or Exp1), SAND family protein (SAND), and clathrin adapter complex subunit family protein (CACS) are the most stably expressed genes in different structures of that plant (Demidenko et al., 2011). All of these reports demonstrate the importance of screening and identifying novel reference genes from EST databases, transcriptome data, microarray analysis, and cDNA libraries (Kumar et al., 2011). The success of qPCR analyses with G. macrophylla is still limited because of inappropriate choices made for reference genes. Identifying more reliable genes to use with that method would benefit future transcription-level studies of G. macrophylla development and metabolic pathways, such as for secoiridoid biosynthesis. Transcriptome profiling has been performed for genes expressed in the roots, leaves, and floral parts of this species, and numerous unigenes have been assigned to secondary-metabolite pathways (Hua et al., 2014). This provides a wealth of resources for our screening reference genes.
The object of the research described here was to characterize genes that might be suitable for transcript normalization in G. macrophylla plants at different developmental stages or when subjected to abiotic stresses. Expression profiles for 10 candidate genes SAND1, F-box family protein (FBOX), Ubiquitin-conjugating enzyme 21 (UBC21), Polypyrimidine tract-binding protein 1 (PTB1), GAPC2, Actin-related protein (ARP), Elongation factor 1-alpha 4 (EF-1α4), Polyubiquitin 10 (UBQ10), Ubiquitin-conjugating enzyme 10 (UBC10), and Exp1 – were examined in leaves and roots from plants (1-year-old seedlings) exposed to elicitor stress inducers (silver nitrate, copper sulfate, arachidonic acid, ammonium citrate, salicylic acid, or methyl jasmonate); roots, leaves, and stems collected at 1-year-old seedlings; or whole plants sampled at the two-leaf, four-leaf, or six-leaf stage of development. The stability of expression for these genes was then evaluated by the GeNorm (Vandesompele et al., 2002), NormFinder (Andersen et al., 2004), BestKeeper (Pfaffl et al., 2004), and comparative ΔCt methods (Silver et al., 2006).
In this study, the validity of using the two housekeeping genes – SAND1 and EF-1α4 – as reference genes to normalize qPCR gene expression data from the G. macrophylla is tested. Expression levels of WRKY transcription factor (WRKY30), geraniol 10-hydroxylase (G10H), and secologanin synthases (SLS) gene are determined in a sample set of leaves from plants undergoing abiotic stress. Finally, the direct comparison of the expression profiles by using relative and absolute qPCR procedures enables us to determine if consistent results can be achieved. As transcription factors, the WRKY proteins are involved in responses to biotic and abiotic stresses, and in developmental processes (Ulker and Somssich, 2004). Our digital expression (DGE) database showed that the members of WRKY family from G. macrophylla can positively response the elicitors stress (Hua et al., 2014). Secoiridoids, such as gentiopicroside in G. macrophylla, are derived from secologanin, which originates from isopentenyl diphosphate via the iridoid pathway (van der Fits and Memelink, 2000). Its biosynthesis in plants might involve either the cytosolic mevalonic acid (MVA) or the plastidial 2-C-methyl-D-erythritol-4-phosphate (MEP) pathway for isopentenyl diphosphate (IPP) and the iridoid pathway for secologanin. Several genes encoding key enzymes in those pathways have been well studied in Catharanthus roseus (Hedhili et al., 2007). G10H and SLS have important roles in regulating monoterpenoid indole alkaloids (MIA) biosynthesis in C. roseus (Zhu et al., 2014; Bernonville et al., 2015). Taken together, the aims of this study are (i) to select appropriate reference genes to use for normalization of gene expression by qPCR in G. macrophylla, (ii) to develop and evaluate qPCR methods for these genes in medicine plant which have transcriptome data and (iii) will help further efforts to quantify DNA content or copy number, contributing to the advance of G. macrophylla molecular pathways of secondary metabolites.
Materials and Methods
Plant Materials and Experimental Conditions
Seeds of Gentiana macrophylla collected from Taibai County, Shaanxi Province, China, were soaked overnight in running tap water. After sonicated for 30 min and 24 h of gibberellin treatment, they were scattered on soil and germinated in the greenhouse (20 ± 2°C, natural lighting). The roots, stems, and leaves were sampled from 1-year-old (6- to 7-cm-tall) plants. Whole plant tissues were also collected from young seedlings (1- to 2-cm-tall) at the two leaves (2L), four leaves (4L), and six leaves (6L) stages. The effects of abiotic stress on gene expression were monitored by foliage spraies on 1-year-old plants with 0.92 mM AgNO3 (Ag), 200 μM CuSO4 (Cu), 10 mg L-1 arachidonic acid (AA), 200 μM ammonium citrate (AC), 200 μM salicylic acid (SA), or 200 μM methyl jasmonate (MeJA), and samples (root and leaf) were collected separately after 6 h of stress treatment. All tissues tested from each experimental condition were flash-frozen in liquid nitrogen and stored at –80°C.
Total RNA Extraction and cDNA Synthesis
Total RNA was isolated with a Polysaccharide and Polyphenols Plant Extract Total RNA (centrifugal column type) Kit according to the manufacturer’s instructions (BioTake, Beijing, China). RNA was treated with RNase-free DNase I (TaKaRa, Dalian, China) to remove genomic DNA. The RNA integrity was checked on a 1% agarose gel. The quantity and quality of the total RNA samples were assessed by recording absorbance at 260/280 nm and 260/230 nm with a NanoDrop ND-1000 spectrophotometer (Thermo Scientific, Wilmington, DE, USA). Only RNA samples with a 260/280 ratio of 1.8 to 2.1 and a 260/230 ratio >2.0 were used for subsequent analyses. Total RNA (1 μg) was reverse-transcribed with a PrimeScriptTM RT Reagent Kit (TaKaRa, Dalian, China) in a 20-μL reaction volume according to the manufacturer’s protocol. All cDNA samples were diluted at 1:5 with RNase-free water and stored at –80°C.
Reference Genes Selection and Primer Design
We performed transcriptome sequencing of G. macrophylla using Illumina paired-end sequencing technology on an Illumina Hi-SeqTM 2000 platform for the four samples (flowers, stems, leaves, and roots; Hua et al., 2014). To ensure the reliability and correctness of target prediction, we applied two steps to predict the potential reference genes of G. macrophylla. The first step was based on Arabidopsis sequences used as queries for BLASTn and tBLASTx against the G. macrophylla transcriptome, which had been uploaded in the BioEdit (Hall, 1999) local database. Second step, G. macrophylla genes used as queries for BLAST one by one through the Tair1, the highest Arabidopsis ortholog sequences were recorded at Table 1. Candidate reference genes of G. macrophylla were shown in Table 1. The primers were designed according to NCBI Primer-BLAST2. Gene characteristics and primer sequences are presented in Table 1.
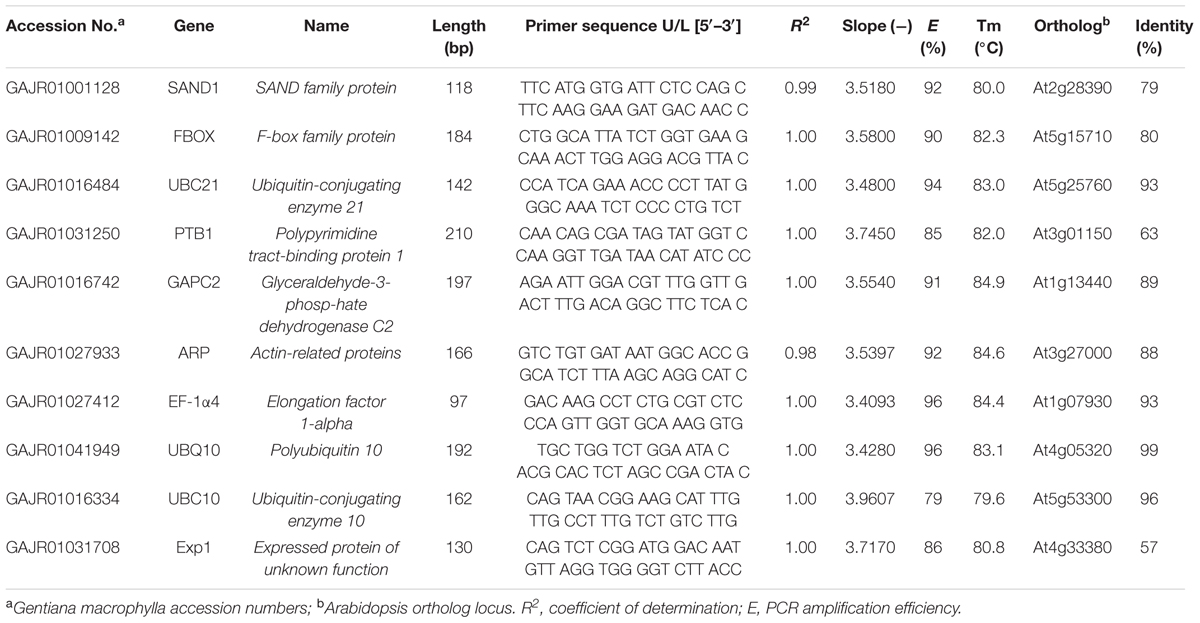
TABLE 1. Candidate reference genes, primer sequences, and characteristics of PCR amplifications in Gentiana macrophylla.
Test Conditions for qPCR and Analysis of Data
Reactions to assess the range of expression for our 10 candidate reference genes were performed in triplicate with SYBR® Premix Ex TaqTMII (TaKaRa, USA) and the Roche LightCycler® 96 system (Roche Diagnostics GmbH). Each sample was tested in three independent replicates with a total reaction volume of 25 μL that contained 0.5 μL of each primer (diluted to 10 mM) plus 12.5 μL of SYBR® Premix Ex TaqTM II, 9.5 μL of ddH2O, and 2 μL of template. Conditions for qPCR included an initial 95°C for 30 s, then 45 cycles of 95°C for 5 s and 60°C for 30 s, followed by a final melting curve analysis.
To determine how different statistical algorithms compared in their ability to select the most stable reference genes, we applied RefFinder (Xie et al., 2012). This web3-based comprehensive tool utilizes the currently available algorithms GeNorm, NormFinder, BestKeeper, and comparative ΔCt. It assigns an appropriate weight to each gene and calculates a geometric (Geo) mean for overall ranking of all potential reference genes. We used GenEx6 software (MultiD Analysis4) to obtain the optimal number of reference genes by calculating their values of Accumulated Standard Deviation (Acc. SD).
Absolute and Relative Quantitation Method
The G. macrophylla transcription factor WRKY30 and two key enzymes genes (G10H and SLS) in gentiopicroside pathway were assessed in the present study for ten potential endogenous genes suitability in qPCR. The PCR-amplifications were performed under conditions of 94°C for 2 min; then 30 cycles of 94°C for 30 s, 60°C for 30 s, and 72°C for 30 s; followed by a final extension step at 72°C for 10 min. Each sample with a total reaction volume of 50 μL that contained 1 μL of each primer (diluted to 10 mM) plus 25 μL of Taq PCR master Mix (Takera, Dalian, China), 21 μL of ddH2O, and 2 μL of template. Afterward, 50 μL of each PCR product was run on a 1% agarose gel for confirmation. Amplified products of the correct (predicted) size were excised from the agarose gels and purified with an E.Z.N.A.TM Gel Extraction Kit (OMEGA, USA). All of them were sequencing carried out by Shanghai Biological Engineering Company. All sequencing results were alignment with G. macrophylla transcriptome database confirmed to be the expected amplicon.
DNA (PCR product) concentration was estimated by measuring the absorbance at 260 nm as described above. DNA copy number was calculated according to the following formula (Godornes et al., 2007):
6.02 × 1023 (molecules/mole) Avogadro’s number
660 Da Average weight of a single base pair.
Each of the purified DNA was diluted with sterile deionized water to obtain a standard series differing by 10-fold.
After qPCR reaction, the values of threshold cycles are achieved. From the slope of a standard curve, PCR amplification efficiency (E) can be calculated according to the equation as follow (Leong et al., 2007):
Where the “slope” is that of the linear regression of Log (target concentration) versus threshold cycle (Ct; Gonçalves et al., 2005).
Each standard curve is established by plotting the Ct on the Y-axis and the natural log of concentration (copies/μL) on the X-axis, and the equation y = slope × x + b, coefficient of determination (R2) and percentage of variance in copy numbers were achieved (Xue et al., 2014). Primers used for analyzing genes expression, plus information about the standard curves, R2 and E are shown in Table 2.

TABLE 2. The standard curve formula, coefficient of determination (R2) and PCR amplification efficiency (E) performed in qPCR assays.
Relative quantitation analysis of expression data for target gene was conducted according to the 2-ΔΔCt method (Erickson et al., 2007).
Results
PCR Amplification Specificity and PCR Efficiency of Candidate Reference Genes
The products of RT-qPCR reactions were sequenced and shown to be identical to the sequence accessions in Table 1. Primer specificities (Table 1) were confirmed by single-peak melting curves for the qPCR products (Figure 1), based on the presence of a single band of the correct size for each pair. The melting temperatures (Tm) of the PCR products ranged from 79.6°C for UBC10 to 84.9°C for GAPC2 (Table 1). Primers that displayed coefficients of correlation >0.98 and efficiencies between 79 and 94% were selected for the next round of qPCR (Table 1).
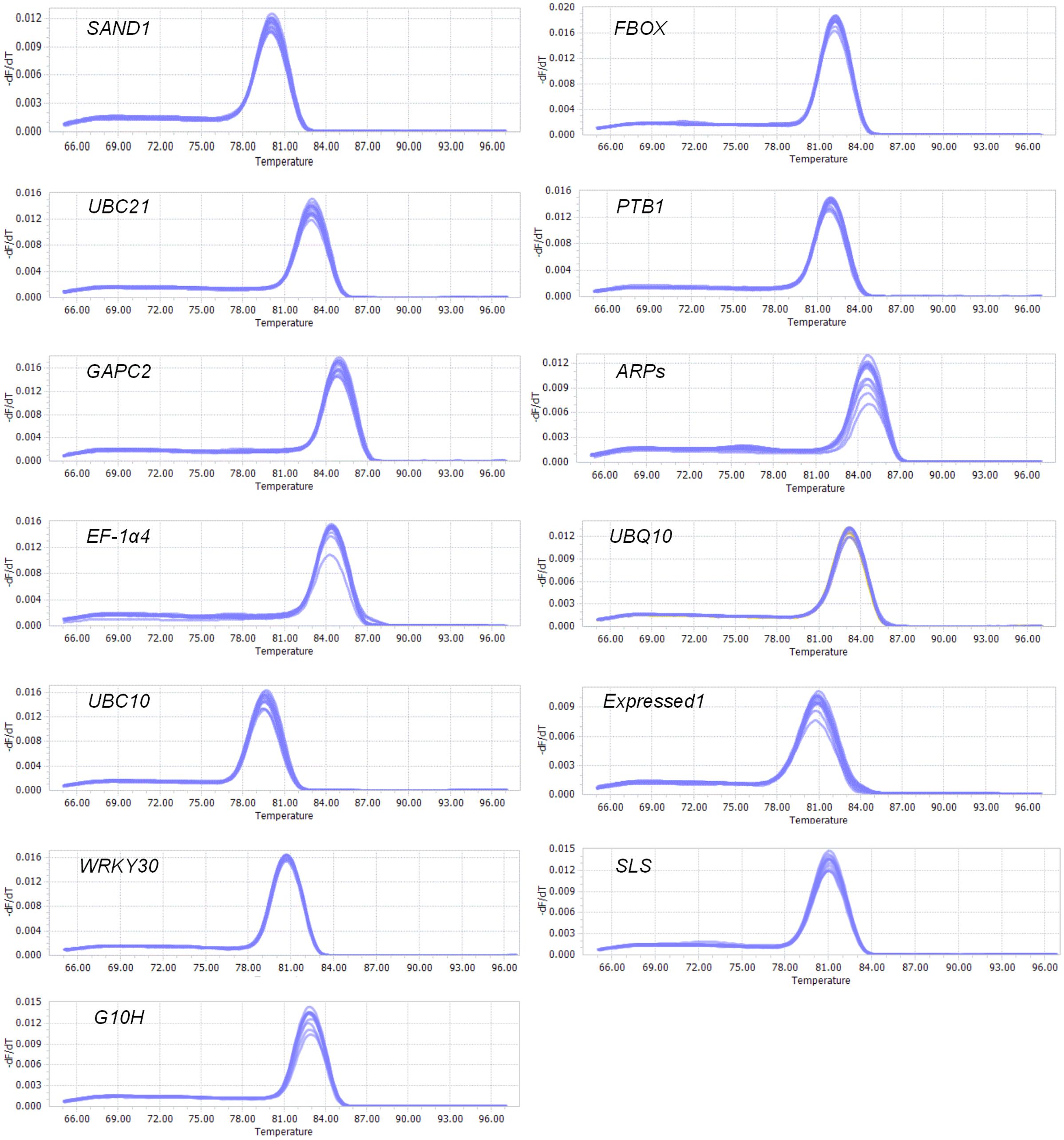
FIGURE 1. Melting curves for genes. Melting temperatures were visualized by plotting negative derivative of change in fluorescence divided by change in temperature relative to temperature [-(d/dT) Fluorescence].
Data were analyzed for experiments covering either plant developmental stages or abiotic stress responses. Four sample subsets were examined: tissues from various stages, leaves from plants exposed to abiotic stress, roots from those stressed plants, and a combination of data from all experimental conditions (all samples). The expression levels of housekeeping genes and transcript accumulations are shown in Figure 2. Transcripts of UBQ10 were most abundant in roots from stressed plants (median cycle threshold, or Ct, value of 20.4) while those levels were lowest in the sample set for developmental stages (Ct of 27.6). Expression was low in all four sample subsets for Exp1. Overall, Ct values ranged from 19.22 for UBC10 in stems to 32.9 for Exp1 at 4 L. Most of those values were between 22.0 and 28.0. These results indicated that none of the selected genes had stable expression among samples, thereby demonstrating how important it is to evaluate the suitability of reference gene(s) for normalizing expression under given experimental conditions when analyzing G. macrophylla.
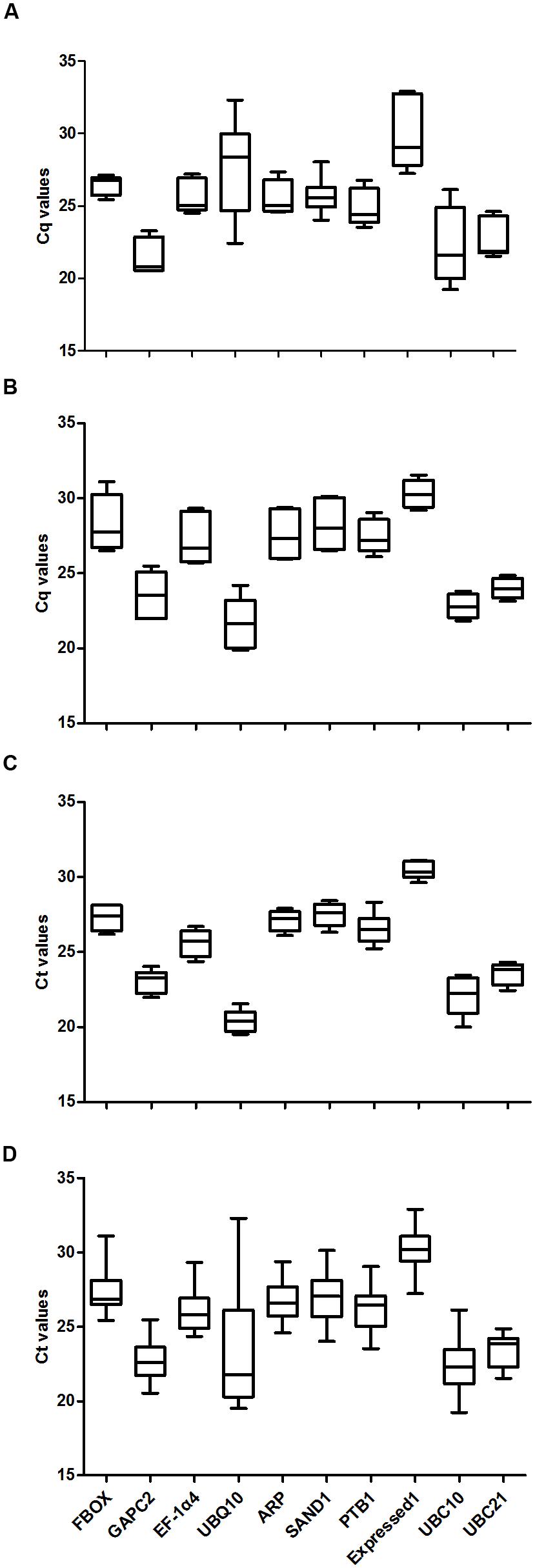
FIGURE 2. Average Ct values for 10 candidate reference genes. (A) developmental stages, (B) leaves from stress-treated plants, (C) roots from stress-treated plants, (D) Data from all experimental conditions combined.
Ranking of Candidates and Determination of Optimal Reference Genes
Four algorithms were used to analyze 10 housekeeping genes and four sample subsets across 16 experimental conditions. An integration tool calculated the Geomean of each gene across GeNorm, NormFinder, BestKeeper, and ΔCt methods. From this, the M values (gene expression stability value, via GeNorm) and SD values (stability values, NormFinder) are presented in Table 3. The reference genes were ranked according to their comprehensive stability in Table 4.
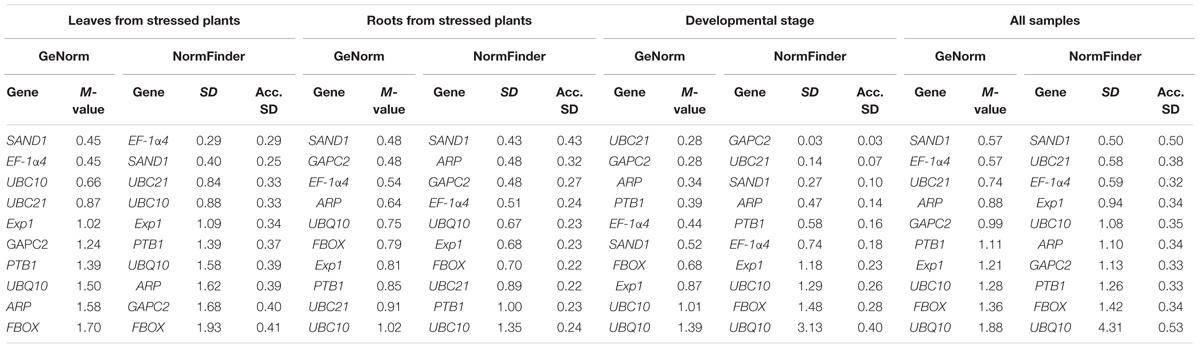
TABLE 3. Stability rankings of candidate genes based on GeNorm and NormFinder for four sampling subsets (Acc. SD, accumulated standard deviation).
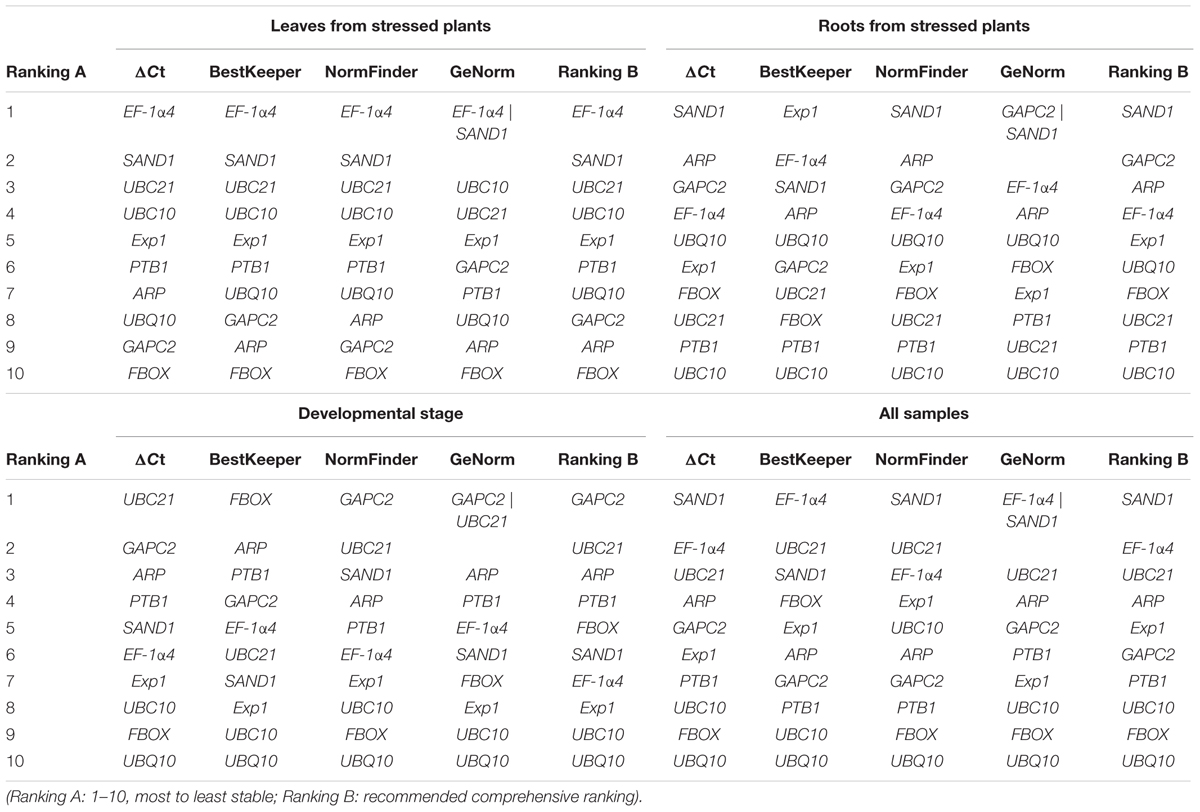
TABLE 4. Stability rankings by RefFinder of candidate reference genes from four sampling subsets representing different experimental conditions.
When all samples were considered, the best genes for RT-qPCR normalization in G. macrophylla were EF-1α4 (M = 0.57, SD = 0.59), UBC21 (M = 0.74, SD = 0.58), and SAND1 (M = 0.57, SD = 0.50). The least suitable overall were FBOX (M = 1.36, SD = 1.42) and UBQ10 (M = 1.88, SD = 4.31). However, those rankings changed when the samples were classified into three different groups, i.e., leaves from stressed plants, roots from stressed plants, and developmental stage. The data subset for leaves from stressed plants indicated that the most stable reference genes were EF-1α4 (M = 0.45, SD = 0.29) and SAND1 (M = 0. 45, SD = 0.40) while the least stable were ARP (M = 1.58, SD = 1.62) and FBOX (M = 1.70, SD = 1.93).
For the group of developmental stage samples, the first choice for most accurate normalization was UBC21 (M = 0.28, SD = 0.14), followed by GAPC2 (M = 0.28, SD = 0.03). In contrast, UBC10 (M = 1.01, SD = 1.29) and UBQ10 (M = 1.39, SD = 3.13) were the least suitable for use as references (Table 3). For roots from stressed plants, the best choices were SAND1 (M = 0.48, from GeNorm; SD = 0.43, from NormFinder), GAPC2 (M = 0.48; GeNorm), and ARP (SD = 0.48; NormFinder). These findings again provided evidence that reference genes must be carefully selected to match the experimental conditions under which a gene is being evaluated.
The GeNorm and NormFinder algorithms available in the GenEx package also allow one to determine the optimal number of control genes to use in normalization processes based on calculations of Acc. SD values for all 10 genes under every experimental condition were computed here by NormFinder and the tested data are shown in Table 3. When three reference genes were used for the subset that combined all samples, the lowest Acc. SD value was achieved, i.e., 0.3231 (Figure 3A). For leaves from stressed plants, the optimal number of reference genes was two, for a minimum Acc. SD value of 0.2481 (Figure 3B). By comparison, evaluations of expression in roots from stressed plants were most accurate when six (Acc. SD value of 0.6390) to seven (0.7518) reference genes were used (Figure 3C). Finally, we found it most remarkable that only one gene was needed to provide qPCR normalization for genes from samples in the developmental stage subset (Figure 3D).
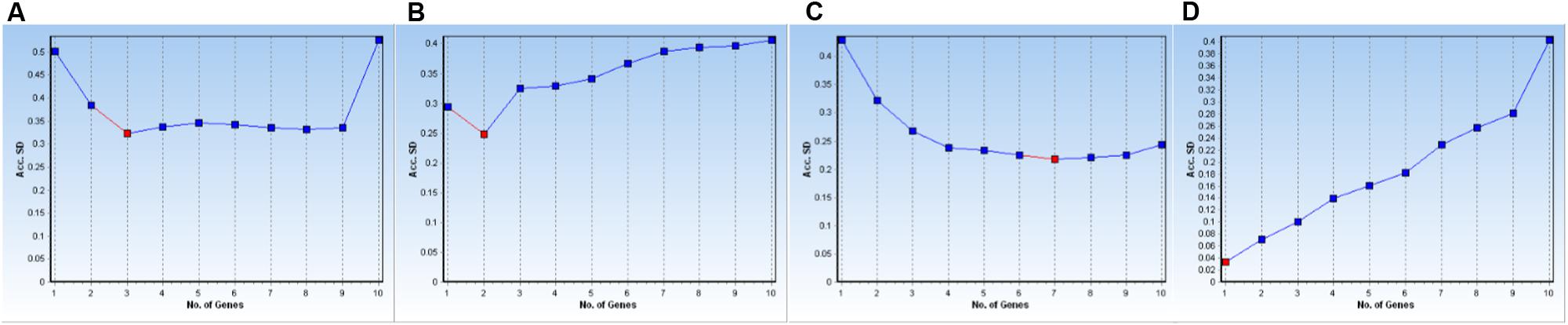
FIGURE 3. Determination of optimal number of reference genes for normalization based on Acc. SD calculations. Data were analyzed by NormFinder for various data subsets of experimental conditions: (A) all samples, (B) leaves from stressed plants, (C) roots from stressed plants, and (D) samples from developmental stages.
To obtain comprehensive rankings of these genes as suitable references, we utilized RefFinder and integrated the results from our four algorithms. As shown in Table 3, EF-1α4 and SAND1 (leaves), SAND1 and GAPC2 (roots), GAPC2 and UBC21 (developmental stages), and SAND1 and EF-1α4 (all samples) were the most stable while the least appropriate were ARP and FBOX (leaves), PTB1 and UBC10 (roots), UBC10 and UBQ10 (developmental stages), and FBOX and UBQ10 (all samples).
Validation of Selected Reference Genes
Here, the validity of using the two stable genes, SAND1 and EF-1α4, as reference genes to normalize real-time RT-PCR gene expression data from the G. macrophylla was tested. Expression patterns of three target genes (WRKY30, G10H, and SLS) in a sample set of leaves from stressed plants and mRNAs were quantified using relative and absolute real-time RT-PCR procedures. Standard curves of the above three target genes primer pairs were established, respectively, to evaluate the amplification efficiency, and melting curves were used to check the within-species-specificity of each qPCR reactions (Figure 1). The data in Table 2 indicated that the R2 values of the three primer sets for the standard curves were >0.98 and the estimated amplification efficiencies (E) were between 81 and 90%.
The expression of WRKY30 (P < 0.001) significantly up-regulated treatment with AA when normalization with SAND1 or EF-1α4. The expression of G10H was sharply increased at Cu but was relatively lower in the AA and SA stress. Normalization with the most stable genes indicated that SLS expression was down-regulated in SA and AA samples when compared with Ag and Cu samples. When target gene expression calculated using relative quantification was more similar to that of the absolute procedure when the stable reference genes were used (Figure 4).
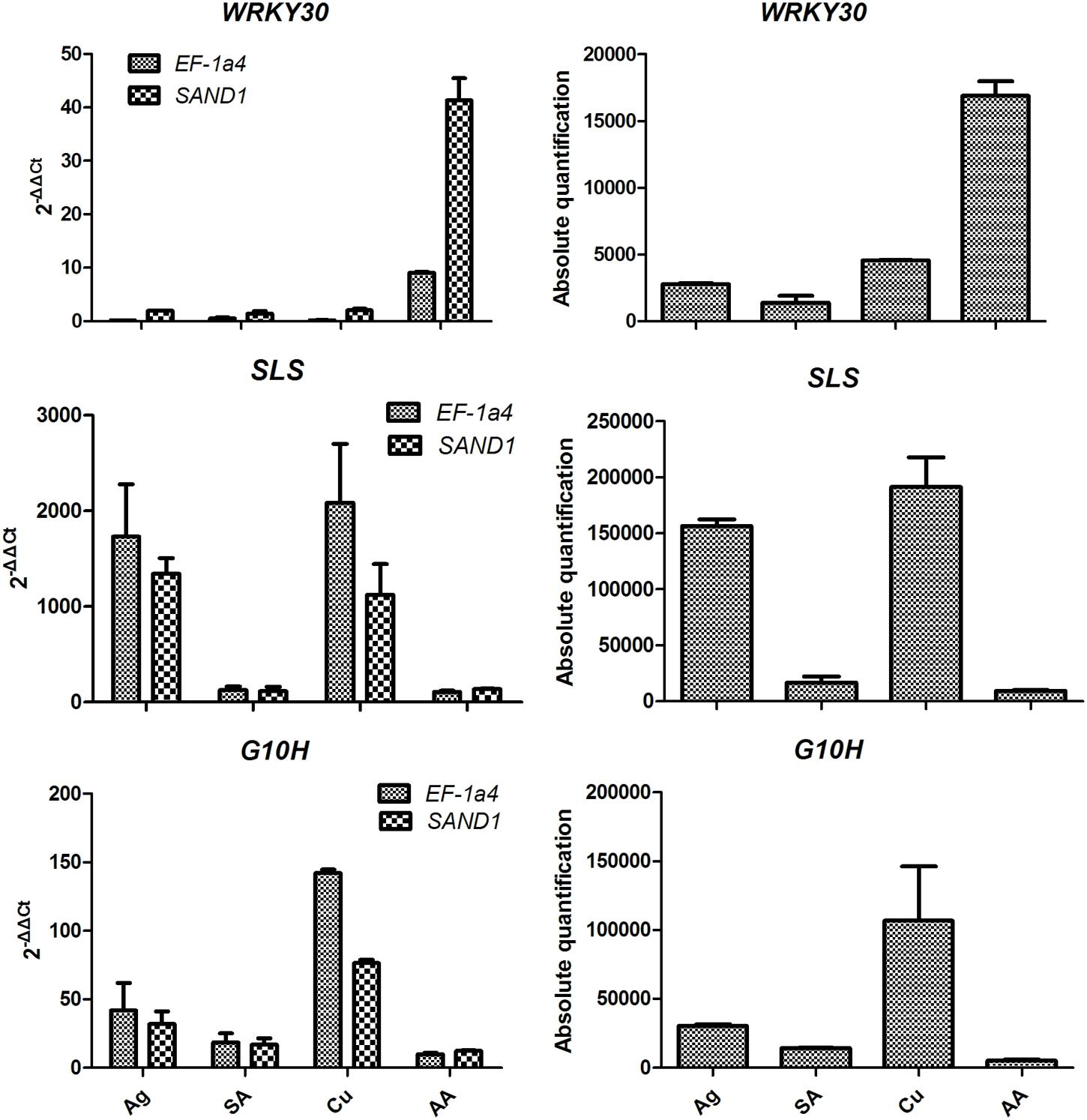
FIGURE 4. Transcript levels of WRKY30 transcription factor and two key enzyme genes (SLS and G10H) in Gentiana macrophylla when selected reference genes were used for normalization. Error bars shows mean standard error calculated from two biological replicates. Comparison of target genes expression profiles relative to the two most stable reference genes (EF-1a4 and SAND1) in samples with leaves from stressed plants. Ag, silver nitrate; SA, salicylic acid; Cu, copper; AA, arachidonic acid.
Discussion
With the rapid development of next-generation sequencing technology, RNA sequencing (RNA-Seq) has been applied primarily to analyze the transcriptomes of various species. Although the main outcome has been the identification of differentially expressed genes, these RNA-Seq data are also used to search for reference genes (Zhuang et al., 2015). We previously examined large-scale transcriptome data for G. macrophylla that comprised 42,918 unigenes (Hua et al., 2014). That initial search served as our resource for selecting reference genes. For any species, it is crucial that one carefully choose the most stable reference gene or internal control gene in order to avoid misinterpreting the results from expression analyses. In the research presented here, we examined four groups of homologous genes (EF-1α, GAPC, ACT, and SAND) and investigated their levels of expression under different experimental conditions. The genes with the highest rankings overall for stable expression were SAND1 and GAPC2. We also chose eight other reference genes, including four traditional housekeeping genes (UBC21, EF-1α4, UBQ10, and UBC10) and four novel genes (FBOX, PTB1, ARP, and Exp1) and compared their levels of expression with those of orthologs from Arabidopsis. These 10 reference genes were evaluated in different tissues from G. macrophylla and under several types of abiotic stress. Our findings demonstrated that RNA-Seq data sets are useful resources when screening suitable candidates, and our results represent an important strategy for large-scale selection of reference genes when studying a non-model plant species (Zhuang et al., 2015).
The statistical algorithms GeNorm, NormFinder, and BestKeeper were developed as aids in selecting stably expressed reference genes for use with qPCR when normalizing expression. For GeNorm, an optimal number of reference genes was selected out of a larger group of candidates based on M-values. In contrast, NormFinder evaluates the expression stability of individual reference genes and takes into account intra- and intergroup variations for normalization while BestKeeper analyzes variabilities in the expression of candidate reference genes by calculating fluctuations in Ct values. All three methods utilize different strategies that can produce contrasting results (Mallona et al., 2010; Wang et al., 2014). For example, in Arabidopsis, ACT2, EF-1a4, and UBQ10 are ranked immediately after the top three reference genes identified via GeNorm but are ranked lower by NormFinder (Remans et al., 2008). In the current research, FBOX proved to be most stably expressed according to BestKeeper but was ranked ninth by GeNorm and seventh by NormFinder. Instead, the ΔCt, NormFinder, and GeNorm methods recommended GAPC2 and UBC21 as most appropriate for normalizing expression during developmental stages. For roots from stressed plants, the top three reference genes were SAND1, ARP, and GAPC2 per GeNorm and ΔCt while BestKeeper ranked SAND1 in third place, ARP in fourth, and GAPC2 as sixth for the same tissue. When the data subset for all samples was studied, EF-1a4 was recognized as the most stably expressed by GeNorm but was ranked third by NormFinder. None of these algorithms identified a single gene as being the most stably expressed under all of our experimental conditions, and individual rankings for each gene differed among algorithms (Klie and Debener, 2011). Therefore, the results from all four methods be considered together when determining which reference genes are most suitable for qPCR normalizations (Wang et al., 2014).
The ideal reference gene shows a constant level of expression that does not vary by organ or tissue type and is also not influenced by the treatment that is applied (Remans et al., 2008). However, numerous studies have shown that no gene is always permanently and stably expressed. Therefore, reference genes must be evaluated for each plant species and for each experimental setup (Hruz et al., 2011). Our results here indicated that, when performing expression analysis with genes from G. macrophylla, SAND1/EF-1α4 are the most appropriate for all samples combined; EF-1a4/SAND1 and SAND1/GAPC2 are the most stably expressed gene pairs in leaves and roots, respectively, from stressed plants; and GAPC2/UBC21 should be used as reference genes when examining expression during various developmental stages.
Stürzenbaum and Kille (2001) and Dean et al. (2002) have stipulated that EF-1α is a good invariant control. Earlier studies with Chrysanthemum and Caragana intermedia also showed that this elongation factor is the most stable reference gene for leaf tissue under stress treatment (Gu et al., 2011; Zhu et al., 2013). Similarly, we found here that EF-1α4 was more stably expressed in leaves from stressed plants but was less stably expressed in roots from stressed plants or in tissues at various developmental stages.
The SAND family protein is involved in vacuolar fusion at the tethering/docking stage in yeast (Wang et al., 2003) and also participates in endosomal traffic in Caenorhabditis elegans (Poteryaev et al., 2007). Our examination of stability revealed that SAND1 was the most stable reference gene in the all-sample data subset as well as in roots and leaves from stressed plants. It also proved to be a better candidate internal control gene in G. macrophylla. In Caragana intermedia, SAND exhibits stable expression across an assortment of tissues under different abiotic stress conditions (Zhu et al., 2013). Furthermore, this gene is one of the most stably expressed in different tissues and organs of citrus genotypes (Mafra et al., 2012).
Ubiquitin conjugation is a protein modification that occurs in a multistep reaction. It sequentially involves an E1 enzyme (ubiquitin-activating enzyme), an E2 enzyme (ubiquitin-conjugating), and an E3 enzyme (ubiquitin ligase). Both UBC21 and UBC10 are in the E2 class (Vierstra, 2003; Kraft et al., 2005). In all four of our data subsets, UBC21 was more stable than UBC10. In citrus under viral stress, the pairing of UBC21/UPL7 is the most stable, followed by UBC9 (Mafra et al., 2012). UBC21 has been shown to be stably expressed in sample sets of Arabidopsis (Czechowski et al., 2005). Finally, UBC10 in Cocos nucifera is a stable reference gene for all stress treatments and endosperm developmental stages (Xia et al., 2014).
Our overall rankings placed ARP, Exp1, and GAPC2 at the fourth, fifth, and sixth positions when all samples were considered. The actin-related proteins are members of an actin family that accumulate in the nucleus (Weber et al., 1995; Harata et al., 2000). Some ARPs are clearly involved in cytoskeletal functions. This is based on two related models in which actin and/or ARPs function as conformational switches that control either the activity or the assembly of chromatin-remodeling machines (Boyer and Peterson, 2000). To the best of our knowledge, ARPs have not previously been used as reference genes for accurate normalization of gene expression data. However, ARP ranked third or fourth for our other data subsets except for leaves from stressed plants. That performance was much better than UBQ10, UBC10, and FBOX, which have traditionally been used as stable reference genes in many plants. GAPDH (GAPC) encodes a glycolytic enzyme that commonly serves as an internal control (albeit without testing) across different species (Li et al., 2012). In our results, GAPC2 ranked eighth for all samples combined but was ranked first for developmental stages, sixth for leaves from stressed plants, and second for roots from stressed plants. By contrast, this gene has been reported as very unstable in the buds, seeds, and various other organs of leafy spurge (Euphorbia esula; Chao et al., 2012).
As stable housekeeping genes in several plant species (Jain et al., 2006), including Arabidopsis thaliana (Hanna et al., 2010), UBQs function in response to adverse environments (Fort et al., 1985; Bhatia et al., 1994), making them atypical for those roles. For example, expression of four UBQ genes is significantly changed in different tissues and is especially high in the flowers and fruits of Citrus japonica (Hu et al., 2014). Similar to our findings here, UBQ10 shows unstable expression across sample pools for Glycine max (Jian et al., 2008) and Oryza sativa (Jain et al., 2006).
An ortholog of At4g33380, Exp1 was moderately stable in our expression analysis. That gene is a good reference in Arabidopsis (Czechowski et al., 2005). Another very stable gene in Arabidopsis, FBOX, is highly expressed in the roots and shoots in response to Cd and Cu treatments (Remans et al., 2008). However, expression of this gene is quite unstable in seeds of leafy spurge during the germination phase (Chao et al., 2012). We also found that FBOX was one of the least stable candidate gene under the conditions and subsets tested here. Therefore, all of these reports demonstrate again that the expression of these 10 candidate reference genes can be species-specific and can also vary according to the experimental environment.
The traditional Chinese medicine of the plant G. macrophylla is derived from secoiridoid active compounds, especially gentiopicroside, which are abundant in these plants, and have broad biological and pharmacological effects. Elicitors have been used to increase the production or to induce de novo synthesis of secondary metabolites in plants. Such treatment could lead to substantial changes in the cellular metabolism (Moreno et al., 1996). The activities of several key enzymes always involved in the biosynthesis of secondary metabolite. Finally, two enzymes genes (G10H and SLS) and one transcription factor gene (WRKY30) were used to confirm the suitability of the reference genes identified here. The biosynthesis of secologanin consists of a number of steps in which the first committed step is the hydroxylation of geraniol to 10-hydroxygeraniol by the enzyme G10H (Moreno et al., 1996). The loganic acid is converted to secologanin via SLS (Pan et al., 2015). The WRKY genes function involved in developmental processes as well as plant responses to biotic and abiotic stresses (Ulker and Somssich, 2004). In our G. macrophylla leaves, When SAND1 was used as a reference, target gene expression was more similar to that of the absolute method than when EF-1α4 was used as a reference. This study indicates that the use of SAND1 and EF-1α4 for studying relative gene expression patterns in G. macrophylla elicitor stressed samples will give appropriate results. Therefore, it is possible that the novel reference gene identified here can outperform commonly used housekeeping genes. This provided more evidence that the incorrect use of reference genes without validation can reduce precision or produce misleading results.
Based on the outcome of our evaluation, we conclude that SAND1 and EF-1α4 is the most appropriate reference gene for expression analysis when tissue types under various abiotic stress conditions. Our results also demonstrate that no gene can act as a universal reference and they highlight the importance of systematically examining expression under each set of experimental conditions (Gutierrez et al., 2008). We also identified novel reference genes that outperform the housekeeping genes commonly used in G. macrophylla and we showed that some of the latter type could be inadequate for transcript normalization under certain experimental conditions (Mafra et al., 2012). In summary, the optimal choice of internal controls for qPCR studies should be tailored to a particular species and be suitable for the particular experimental conditions that are under consideration.
Author Contributions
YiH carried out the experimental design. YiH and YaH collected samples and helped on harvest. YaH and HY performed the experiments and analysis. YiH, WH, and ZW prepared the manuscript and coordinated its revision. All authors read and revised the manuscript, provided helpful discussions and approved its final version.
Conflict of Interest Statement
The authors declare that the research was conducted in the absence of any commercial or financial relationships that could be construed as a potential conflict of interest.
Acknowledgment
The research was supported by The National Key Technology R&D program (2012BAI29B03).
Footnotes
- ^ http://www.arabidopsis.org/index.jsp
- ^ http://www.ncbi.nlm.nih.gov/tools/primer-blast/
- ^ http://fulxie.0fees.us/?type=reference
- ^ http://www.gene-quantification.de/genex.html
References
Andersen, C. L., Jensen, J. L., and Orntoft, T. F. (2004). Normalization of real-time quantitative reverse transcription-PCR data: a model-based variance estimation approach to identify genes suited for normalization, applied to bladder and colon cancer data sets. Cancer Res. 64, 5245–5250. doi: 10.1158/0008-5472.CAN-04-0496
Bernonville, T. D. D., Foureau, E., Parage, C., Lanoue, A., Clastre, M., Londono, M. A., et al. (2015). Characterization of a second secologanin synthase isoform producing both secologanin and secoxyloganin allows enhanced de novo assembly of a Catharanthus roseus transcriptome. BMC Genomics 16:619. doi: 10.1186/s12864-015-1678-y
Bhatia, P., Taylor, W. R., Greenberg, A. H., and Wright, J. A. (1994). Comparison of glyceraldehyde-3-phosphate dehydrogenase and 28S-ribosomal RNA gene expression as RNA loading controls for northern blot analysis of cell lines of varying malignant potential. Anal. Biochem. 216, 223–226. doi: 10.1006/abio.1994.1028
Boyer, L. A., and Peterson, C. L. (2000). Actin-related proteins (Arps): conformational switches for chromatin-remodeling machines? Bioessays 22, 666–672. doi: 10.1002/1521-1878(200007)22:7<666::AID-BIES9>3.3.CO;2-P
Bustin, S. A., Benes, V., Garson, J. A., Hellemans, J., Huggett, J., Kubista, M., et al. (2009). The MIQE guidelines: minimum information for publication of quantitative real-time PCR experiments. Clin. Chem. 55, 611–622. doi: 10.1373/clinchem.2008.112797
Chao, W. S., Dogramaci, M., Foley, M. E., Horvath, D. P., and Anderson, J. V. (2012). Selection and validation of endogenous reference genes for qRT-PCR analysis in leafy spurge (Euphorbia esula). PLoS ONE 7:e42839. doi: 10.1371/journal.pone.0042839
Czechowski, T., Stitt, M., Altmann, T., Udvardi, M. K., and Scheible, W. R. (2005). Genome-wide identification and testing of superior reference genes for transcript normalization in Arabidopsis. Plant Physiol. 139, 5–17. doi: 10.1104/pp.105.063743
Dean, J. D., Goodwin, P. H., and Hsiang, T. (2002). Comparison of relative RT-PCR and northern blot analyses to measure expression of β-1,3-glucanase in Nicotiana benthamiana infected with Colletotrichum destructivum. Plant Mol. Biol. Rep. 20, 347–356. doi: 10.1007/BF02772122
Demidenko, N. V., Logacheva, M. D., and Penin, A. A. (2011). Selection and validation of reference genes for quantitative real-time PCR in buckwheat (Fagopyrum esculentum) based on transcriptome sequence data. PLoS ONE 6:e19434. doi: 10.1371/journal.pone.0019434
Erickson, H. S., Albert, P. S., Gillespie, J. W., Wallis, B. S., Rodriguez-Canales, J., Linehan, W. M., et al. (2007). Assessment of normalization strategies for quantitative RT-PCR using microdissected tissue samples. Lab. Invest. 87, 951–962. doi: 10.1038/labinvest.3700659
Fort, P., Marty, L., Piechaczyk, M., Sabrouty, S. E., Dani, C., Jeanteur, P., et al. (1985). Various rat adult tissues express only one major mRNA species from the glyceraldehyde-3-phosphate-dehydrogenase multigenic family. Nucleic Acids Res. 13, 1431–1442. doi: 10.1093/nar/13.5.1431
Gachon, C., Mingam, A., and Charrier, B. (2004). Real-time PCR: what relevance to plant studies? J. Exp. Bot. 55, 1445–1454. doi: 10.1093/jxb/erh181
Godornes, C., Leader, B. T., Molini, B. J., Centurion-Lara, A., and Lukehart, S. A. (2007). Quantitation of rabbit cytokine mRNA by real-time RT-PCR. Cytokine 38, 1–7. doi: 10.1016/j.cyto.2007.04.002
Gonçalves, S., Cairney, J., Maroco, J., Oliveira, M. M., and Miguel, C. (2005). Evaluation of control transcripts in real-time RT-PCR expression analysis during maritime pine embryogenesis. Planta 222, 556–563. doi: 10.1007/s00425-005-1562-0
Gu, C., Chen, S., Liu, Z., Shan, H., Luo, H., Guan, Z., et al. (2011). Reference gene selection for quantitative real-time PCR in Chrysanthemum subjected to biotic and abiotic stress. Mol. Biotechnol. 49, 192–197. doi: 10.1007/s12033-011-9394-6
Gutierrez, L., Mauriat, M., Guénin, S., Pelloux, J., Lefebvre, J. F., Louvet, R., et al. (2008). The lack of a systematic validation of reference genes: a serious pitfall undervalued in reverse transcription-polymerase chain reaction (RT-PCR) analysis in plants. Plant Biotechnol. J. 6, 609–618. doi: 10.1111/j.1467-7652.2008.00346.x
Hall, T. A. (1999). BioEdit: a user-friendly biological sequence alignment editor and analysis program for Windows 95/98/NT. Nucl. Acids Symp. Ser. 41, 95–98.
Han, X. J., Lu, M. Z., Chen, Y. C., Zhan, Z. Y., Cui, Q. Q., and Wang, Y. D. (2012). Selection of reliable reference genes for gene expression studies using Real-Time PCR in tung tree during seed development. PLoS ONE 7:e43084. doi: 10.1371/journal.pone.0043084
Hanna, L., Seong Jeon, Y., Jeong Hwan, L., Wanhui, K., Seung Kwan, Y., Heather, F., et al. (2010). Genetic framework for flowering-time regulation by ambient temperature-responsive miRNAs in Arabidopsis. Nucleic Acids Res. 38, 3081–3093. doi: 10.1093/nar/gkp1240
Harata, M., Oma, Y., Tabuchi, T., Zhang, Y., Stillman, D. J., and Mizuno, S. (2000). Multiple actin-related proteins of Saccharomyces cerevisiae are present in the nucleus. J. Biochem. 128, 665–671. doi: 10.1093/oxfordjournals.jbchem.a022799
Hedhili, S., Courdavault, V., Giglioli-Guivarc’h, N., and Gantet, P. (2007). Regulation of the terpene moiety biosynthesis of Catharanthus roseus terpene indole alkaloids. Phytochem. Rev. 6, 341–351. doi: 10.1007/s11101-006-9021-5
Hruz, T., Wyss, M., Docquier, M., Pfaffl, M. W., Masanetz, S., Borghi, L., et al. (2011). RefGenes: identification of reliable and condition specific reference genes for RT-qPCR data normalization. BMC Genomics 12:156. doi: 10.1186/1471-2164-12-156
Hu, Y., Chen, H., Luo, C., Dong, L., Zhang, S. W., He, X. H., et al. (2014). Selection of reference genes for real-time quantitative PCR studies of kumquat in various tissues and under abiotic stress. Sci. Hortic. 174, 207–216. doi: 10.1016/j.scienta.2013.12.003
Hua, W. P., Zheng, P., He, Y. H., Cui, L. J., Kong, W. W., and Wang, Z. Z. (2014). An insight into the genes involved in secoiridoid biosynthesis in Gentiana macrophylla by RNA-seq. Mol. Biol. Rep. 41, 4817–4825. doi: 10.1007/s11033-014-3352-x
Jain, M., Nijhawan, A., Tyagi, A. K., and Khurana, J. P. (2006). Validation of housekeeping genes as internal control for studying gene expression in rice by quantitative real-time PCR. Biochem. Biophys. Res. Commun. 345, 646–651. doi: 10.1016/j.bbrc.2006.04.140
Ji, L., Liao, Z., and Sun, H. (2002). A survey of Gentian plants of the Tibetan traditional herb medicines. Acta Biol. Plateau Sin. 15, 243–250.
Jian, B., Liu, B., Bi, Y., Hou, W., Wu, C., and Han, T. (2008). Validation of internal control for gene expression study in soybean by quantitative real-time PCR. BMC Mol. Biol. 9:59. doi: 10.1186/1471-2199-9-59
Keyser, E. D., Desmet, L., Bockstaele, E. V., and Riek, J. D. (2013). How to perform RT-qPCR accurately in plant species? A case study on flower colour gene expression in an azalea (Rhododendron simsii hybrids) mapping population. BMC Mol. Biol. 14:13. doi: 10.1186/1471-2199-14-13
Klie, M., and Debener, T. (2011). Identification of superior reference genes for data normalisation of expression studies via quantitative PCR in hybrid roses (Rosa hybrida). BMC Res. Notes 4:518. doi: 10.1186/1756-0500-4-518
Kraft, E., Stone, S. L., Ma, L., Su, N., Gao, Y., Lau, O.-S., et al. (2005). Genome analysis and functional characterization of the E2 and RING-type E3 ligase ubiquitination enzymes of Arabidopsis. Plant Physiol. 139, 1597–1611. doi: 10.1104/pp.105.067983
Kumar, V., Sharma, R., Trivedi, P. C., Vyas, G. K., and Khandelwal, V. (2011). Traditional and novel references towards systematic normalization of qRT-PCR data in plants. Aust. J. Crop Sci. 5, 1455–1468.
Leong, D. T., Gupta, A., Bai, H. F., Wan, G., Li, F. Y., Too, H. P., et al. (2007). Absolute quantification of gene expression in biomaterials research using real-time PCR. Biomaterials 28, 203–210. doi: 10.1016/j.biomaterials.2006.09.011
Li, Q. Q., Skinner, J., and Bennett, J. E. (2012). Evaluation of reference genes for real-time quantitative PCR studies in Candida glabrata following azole treatment. BMC Mol. Biol. 13:22. doi: 10.1186/1471-2199-13-22
Maccoux, L. J., Clements, D. N., Salway, F., and Day, P. J. (2007). Identification of new reference genes for the normalisation of canine osteoarthritic joint tissue transcripts from microarray data. BMC Mol. Biol. 8:62. doi: 10.1186/1471-2199-8-62
Mafra, V., Kubo, K. S., Alves-Ferreira, M., Ribeiro-Alves, M., Stuart, R. M., Boava, L. P., et al. (2012). Reference genes for accurate transcript normalization in citrus genotypes under different experimental conditions. PLoS ONE 7:e31263. doi: 10.1371/journal.pone.0031263
Mallona, I., Lischewski, S., Weiss, J., Hause, B., and Egea-Cortines, M. (2010). Validation of reference genes for quantitative real-time PCR during leaf and flower development in Petunia hybrida. BMC Plant Biol. 10:4. doi: 10.1186/1471-2229-10-4
Marino, E., Borges, A. A., Borgespérez, A., and Pérez, J. A. (2008). Selection of internal control genes for quantitative real-time RT-PCR studies during tomato development process. BMC Plant Biol. 8:131. doi: 10.1186/1471-2229-8-131
Moreno, P. R. H., Poulsen, C., Heijden, R. V. D., and Verpoorte, R. (1996). Effects of elicitation on different metabolic pathways in Catharanthus roseus (L.)G.Don cell suspension cultures. Enzyme Microb. Technol. 18, 99–107. doi: 10.1016/0141-0229(95)00078-X
Nicot, N., Hausman, J. F., Hoffmann, L., and Evers, D. (2005). Housekeeping gene selection for real-time RT-PCR normalization in potato during biotic and abiotic stress. J. Exp. Bot. 56, 2907–2914. doi: 10.1093/jxb/eri285
Pan, Y. J., Jia, L., Guo, X. R., Zu, Y. G., and Tang, Z. H. (2015). Gene transcript profiles of the TIA biosynthetic pathway in response to ethylene and copper reveal their interactive role in modulating TIA biosynthesis in Catharanthus roseus. Virology 252, 813–824. doi: 10.1007/s00709-014-0718-9
Pfaffl, M. W., Tichopad, A., Prgomet, C., and Neuvians, T. P. (2004). Determination of stable housekeeping genes, differentially regulated target genes and sample integrity: BestKeeper–Excel-based tool using pair-wise correlations. Biotechnol. Lett. 26, 509–515. doi: 10.3390/ijms160612513
Poteryaev, D., Fares, H., Bowerman, B., and Spang, A. (2007). Caenorhabditis elegans SAND-1 is essential for RAB-7 function in endosomal traffic. EMBO J. 26, 301–312. doi: 10.1038/sj.emboj.7601498
Remans, T., Smeets, K., Opdenakker, K., Mathijsen, D., Vangronsveld, J., and Cuypers, A. (2008). Normalisation of real-time RT-PCR gene expression measurements in Arabidopsis thaliana exposed to increased metal concentrations. Planta 227, 1343–1349. doi: 10.1007/s00425-008-0706-4
Sellars, M. J., Vuocolo, T., Leeton, L. A., Coman, G. J., Degnan, B. M., and Preston, N. P. (2007). Real-time RT-PCR quantification of Kuruma shrimp transcripts: a comparison of relative and absolute quantification procedures. J. Biotechnol. 129, 391–399. doi: 10.1016/j.jbiotec.2007.01.029
Silver, N., Best, S., Jiang, J., and Thein, S. L. (2006). Selection of housekeeping genes for gene expression studies in human reticulocytes using real-time PCR. BMC Mol. Biol. 7:33. doi: 10.1186/1471-2199-7-33
Stürzenbaum, S. R., and Kille, P. (2001). Control genes in quantitative molecular biological techniques: the variability of invariance. Comp. Biochem. Phys. B 130, 281–289. doi: 10.1016/S1096-4959(01)00440-7
Ulker, B., and Somssich, I. E. (2004). WRKY transcription factors: from DNA binding towards biological function. Curr. Opin. Plant Biol. 7, 491–498. doi: 10.1016/j.pbi.2004.07.012
van der Fits, L., and Memelink, J. (2000). ORCA3, a jasmonate-responsive transcriptional regulator of plant primary and secondary metabolism. Science 289, 295–297. doi: 10.1126/science.289.5477.295
Vandesompele, J., De Preter, K., Pattyn, F., Poppe, B., Van Roy, N., De Paepe, A., et al. (2002). Accurate normalization of real-time quantitative RT-PCR data by geometric averaging of multiple internal control genes. Genome Biol. 3, 1–11. doi: 10.1186/gb-2002-3-7-research0034
Vierstra, R. D. (2003). The ubiquitin/26S proteasome pathway, the complex last chapter in the life of many plant proteins. Trends Plant Sci. 8, 135–142. doi: 10.1016/S1360-1385(03)00014-1
Wang, C. W., Stromhaug, P. E., Kauffman, E. J., Weisman, L. S., and Klionsky, D. J. (2003). Yeast homotypic vacuole fusion requires the Ccz1-Mon1 complex during the tethering/docking stage. J. Cell Biol. 163, 973–985. doi: 10.1083/jcb.200308071
Wang, Y., and Lou, Z. (1987). A review on the studies of the Chinese herb Qinjiao. Chin. Pharm. Bull. 22, 153–159.
Wang, Z., Chen, Y., Fang, H. D., Shi, H. F., Chen, K. P., Zhang, Z. Y., et al. (2014). Selection of reference genes for quantitative reverse-transcription polymerase chain reaction normalization in Brassica napus under various stress conditions. Mol. Gen. Genet. 289, 1023–1035. doi: 10.1007/s00438-014-0853-1
Weber, V., Harata, M., Hauser, H., and Wintersberger, U. (1995). The actin-related protein Act3p of Saccharomyces cerevisiae is located in the nucleus. Mol. Biol. Cell 6, 1263–1270. doi: 10.1091/mbc.6.10.1263
Xia, W., Liu, Z., Yang, Y., Xiao, Y., Mason, A. S., Zhao, S., et al. (2014). Selection of reference genes for quantitative real-time PCR in Cocos nucifera during abiotic stress. Botany 92, 179–186. doi: 10.1139/cjb-2013-0212
Xie, F., Xiao, P., Chen, D., Xu, L., and Zhang, B. (2012). miRDeepFinder: a miRNA analysis tool for deep sequencing of plant small RNAs. Plant Mol. Biol. 80, 75–84. doi: 10.1007/s11103-012-9885-2
Xue, B., Guo, J., Que, Y., Fu, Z., Wu, L., and Xu, L. (2014). Selection of suitable endogenous reference genes for relative copy number detection in sugarcane. Int. J. Mol. Sci. 15, 8846–8862. doi: 10.3390/ijms15058846
Yu, Y., Lee, C., Kim, J., and Hwang, S. (2005). Group-specific primer and probe sets to detect methanogenic communities using quantitative real-time polymerase chain reaction. Biotechnol. Bioeng. 89, 670–679. doi: 10.1002/bit.20347
Zhou, K., Zhou, L., Lim, Q., Zou, R., Stephanopoulos, G., and Too, H. P. (2011). Novel reference genes for quantifying transcriptional responses of Escherichia coli to protein overexpression by quantitative PCR. BMC Mol. Biol. 12:18. doi: 10.1186/1471-2199-12-18
Zhu, J., Wang, M., Wen, W., and Yu, R. (2014). Biosynthesis and regulation of terpenoid indole alkaloids in Catharanthus roseus. Pharmacogn. Rev. 9, 24–28. doi: 10.4103/0973-7847.156323
Zhu, J., Zhang, L., Li, W., Han, S., Yang, W., and Qi, L. (2013). Reference gene selection for quantitative real-time PCR normalization in Caragana intermedia under different abiotic stress conditions. PLoS ONE 8:e53196. doi: 10.1371/journal.pone.0053196
Keywords: Gentiana macrophylla, reference gene, qPCR, transcriptome, gene expression, abiotic stress
Citation: He Y, Yan H, Hua W, Huang Y and Wang Z (2016) Selection and Validation of Reference Genes for Quantitative Real-time PCR in Gentiana macrophylla. Front. Plant Sci. 7:945. doi: 10.3389/fpls.2016.00945
Received: 08 April 2016; Accepted: 14 June 2016;
Published: 29 June 2016.
Edited by:
Fabio Marroni, Istituto di Genomica Applicata, ItalyCopyright © 2016 He, Yan, Hua, Huang and Wang. This is an open-access article distributed under the terms of the Creative Commons Attribution License (CC BY). The use, distribution or reproduction in other forums is permitted, provided the original author(s) or licensor are credited and that the original publication in this journal is cited, in accordance with accepted academic practice. No use, distribution or reproduction is permitted which does not comply with these terms.
*Correspondence: Zhezhi Wang, emhlemhpd2FuZ0BvdXRsb29rLmNvbQ==