- 1School of Metallurgy and Environment, Central South University, Changsha, China
- 2School of Minerals Processing and Bioengineering, Central South University, Changsha, China
- 3College of Life Sciences, Central China Normal University, Wuhan, China
- 4School of Life Sciences, Central South University, Changsha, China
- 5State Key Laboratory of Hybrid Rice, College of Life Sciences, Wuhan University, Wuhan, China
Pseudometallophytes are excellent models to study microevolution and local adaptation to soil pollution, as they can grow both on metalliferous and contrasting non-metalliferous soils. Although, there has been accumulating evidence for the effects of edaphic conditions and geographical isolation on the genetic structure of pesudometallophytes, it is still a difficult problem in evolutionary biology to assess their relative importance. In this study, we investigated the spatial patterns of genetic variability, population differentiation and genetic groups in pseudometallophyte Commelina communis with 12 microsatellite loci. Eight metallicolous and six non-metallicolous populations of C. communis were sampled from cupriferous sites and surrounding non-contaminated areas in China. Neither significant reduction in genetic diversity nor apparent founder and bottleneck effects were observed in metallicolous populations of C. communis. Based on Bayesian and Neighbor-Joining clustering analyses and a principal coordinates analysis, all sampled populations were found to be mainly separated into three genetic groups, corresponding well to their geographical locations rather than edaphic origins. Moreover, a significant and strong correlation between population genetic divergence and geographical distance were detected by Mantel test (r = 0.33; P < 0.05) and multiple matrix regression with randomization (MMRR; βD = 0.57, P < 0.01). However, the effect of copper concentration on genetic patterns of C. communis was not significant (MMRR; βE = -0.17, P = 0.12). Our study clearly demonstrated that the extreme edaphic conditions in metalliferous areas had limited effects on the genetic variability in C. communis. Geographic distance played a more important role in affecting the genetic structure of C. communis than soil composition did. In C. communis, the geographically disjunctive populations on metalliferous soils had multiple origins and evolved independently from nearby non-metallicolous populations.
Introduction
Environmental stress experienced by living organisms plays an important role in local adaptation and evolution of new species (Hoffman and Parsons, 1991; Parsons, 2005; Nevo, 2011). Soils contaminated with high concentrations of heavy metals (metalliferous soils) are toxic and restrictive habitats for plants due to phytotoxicity (Antonovics et al., 1971; Wu, 1990; Ernst, 1996). The harsh edaphic conditions in metalliferous areas therefore have been considered as the major environmental determinants of plant survival and distribution (Cain, 1944; Rajakaruna, 2004). However, some plant species (known as metallophytes) that have evolved heavy metal-tolerance can colonize, survive, and reproduce in such conditions without suffering toxicity (Antonovics et al., 1971; Simon, 1978; Macnair, 1987; Baker et al., 2010). Pseudometallophytes, i.e., species growing on both metalliferous and non-metalliferous soils have been found to be the dominant plants in heavy metal contaminated areas (Ernst, 2006). Exploring the genetic variation and differentiation between metallicolous (M) and non-metallicolous (NM) populations in pseudometallophytes provides us an ideal opportunity to understand the processes of plant local adaptation and microevolution in the context of environmental pollution (Linhart and Grant, 1996; Vekemans and Lefèbvre, 1997; Mengoni et al., 2000, 2001; Meyer et al., 2010).
Genetic variation at intra- and inter-population level have been considered as the preconditions of plant local adaptation or evolution (Bone and Farres, 2001; Jiménez-Ambriz et al., 2006; Słomka et al., 2011). The extremely edaphic conditions on metal-contaminated areas can exert strong selection pressure and significantly influence the levels of genetic diversity within and among populations of pseudometallophytes (Macnair et al., 2000; Assunção et al., 2003; Dubois et al., 2003). In initial M populations, the number of metal tolerant individuals is assumed to be small (Macnair, 1987; Al-Hiyaly et al., 1993) and recently established populations on the metalliferous soils therefore may experience strong founder and bottleneck effects (Lefèbvre and Vernet, 1990; Linhart and Grant, 1996; Vekemans and Lefèbvre, 1997; Pollard et al., 2002). In multiple previous studies, significant reduction in genetic variability has been detected in populations of pseudometallophytes from metal contaminated sites (Nordal et al., 1999; Mengoni et al., 2001; Deng et al., 2007; Meyer et al., 2010; Babst-Kostecka et al., 2014). However, opposite results were also observed in some other pseudometallophytes, where the levels of genetic diversity were similar in both NM and M populations (Mengoni et al., 2000, 2006; Pauwels et al., 2005; Baumbach and Hellwig, 2007; Bizoux et al., 2008; Wójcik et al., 2013) or even higher in populations established in metal-contaminated soils (Słomka et al., 2011; Dresler et al., 2015). Such contrasting patterns suggest that soil composition is an important factor affecting the patterns of genetic variation in pesudometallophytes, but not the only one. To better understand the effects of edaphic conditions on the genetic variation in pesudometallophytes, other additional factors that can possibly influence the initial founder effects should be investigated, such as the level of selection pressure, the level of gene flow among populations, the successive mutation events, the geographic distribution patterns, and the plant reproductive systems (Ye et al., 2012; Babst-Kostecka et al., 2014). So far, it is still unclear how these factors, either individually or jointly, come into play during the colonization of pseudometallophytes.
Generally, geography plays an important role in population genetic divergence and speciation. The greater physical distance among populations will lead to the stronger internal genetic differentiation (Rieseberg and Willis, 2007). Heavy metal contaminated sites are featured for their patchy distributions and usually surrounded by non-contaminated mainland (Lefèbvre and Vernet, 1990). In such a case, populations from metalliferous soils should have closer genetic relationships with geographically adjacent NM populations than other distant conspecific M populations. Therefore, the hypothesis of multiple and independent origins for M populations of pesudometallophytes has been proposed (Schat et al., 1996; Mengoni et al., 2001; Pauwels et al., 2005; Quintela-Sabarís et al., 2010). However, extremely edaphic conditions can also cause the development of population differentiation (Bone and Farres, 2001). Under heavy metal stress, a small geographical interval between M and NM populations can result in significant genetic divergence or even sympatric speciation (Linhart and Grant, 1996; Rajakaruna, 2004). Although, both edaphic conditions and geography should contribute to shaping the spatial population structure of pesudometallophytes, it is still difficult to assess their relative importance on this event.
Commelina communis L. (Commelinaceae), commonly known as dayflower, is distributed extensively throughout China except for a few provinces, such as Qinghai, Hainan, Xinjiang, and Tibet (Hong and DeFillipps, 2000; Huang, 2001). This species can grow on both cupriferous habitats and surrounding non-metalliferous areas and has been considered as a typical pseudometallophyte (Tang et al., 1997, 1999, 2001; Ye et al., 2012). In the previous study conducted by Ye et al. (2012), the populations of C. communis from both metalliferous and nearby non-metalliferous soils mainly clustered into two groups, corresponding well to edaphic types rather than geographic locations (Ye et al., 2012). Cu contamination instead of geographic distance therefore had been considered as the major factor influencing the genetic structure of C. communis. However, it should be noted that the sampling sites for M populations in the previous research were relatively close and mainly concentrated in eastern China (Ye et al., 2012), the potential effect of geography might be underestimated.
To accurately evaluate the effects of edaphic conditions and geographical factors on the microevolutionary processes of pesudometallophytes, multiple replicates of M and NM populations separated by large geographic distances should be analyzed. In this study, 14 C. communis populations were sampled from three main regions in China, covering a much broader ranges of geographic distribution than the previous study (Ye et al., 2012). Twelve highly polymorphic microsatellite loci were used to estimate the genetic diversity and population structure of C. communis (Jarne and Lagoda, 1996; Sunnucks, 2000; Zhang and Hewitt, 2003; Selkoe and Toonen, 2006). The specific aims of this study were to: (1) assess the genetic variability and differentiation among populations of C. communis established in metalliferous and non-metalliferous soils; (2) test the relative importance of geographic distance and soil composition on shaping genetic differentiation of C. communis populations; (3) explore whether C. communis populations established in metalliferous and non-metalliferous soils had a single or multiple independent origins.
Materials and Methods
Plant and Soil Sampling
Eight natural copper mines located in different geographic areas of China were selected as sampling sites for M populations of C. communis (Table 1 and Figure 1). Sampled M populations were discontinuously distributed in three geographical regions (I: east-central china, II: central China, and III: north China; Figure 1). Six NM populations were also sampled from nearby non-metalliferous soils. In each population, leaves of C. communis were collected from twenty individuals separated by at least 5 m and immediately dried in silica gel for genomic DNA extraction. In addition, 10 rhizospheric soil samples were randomly collected from 5 to 15 cm in depth at each sampling site.
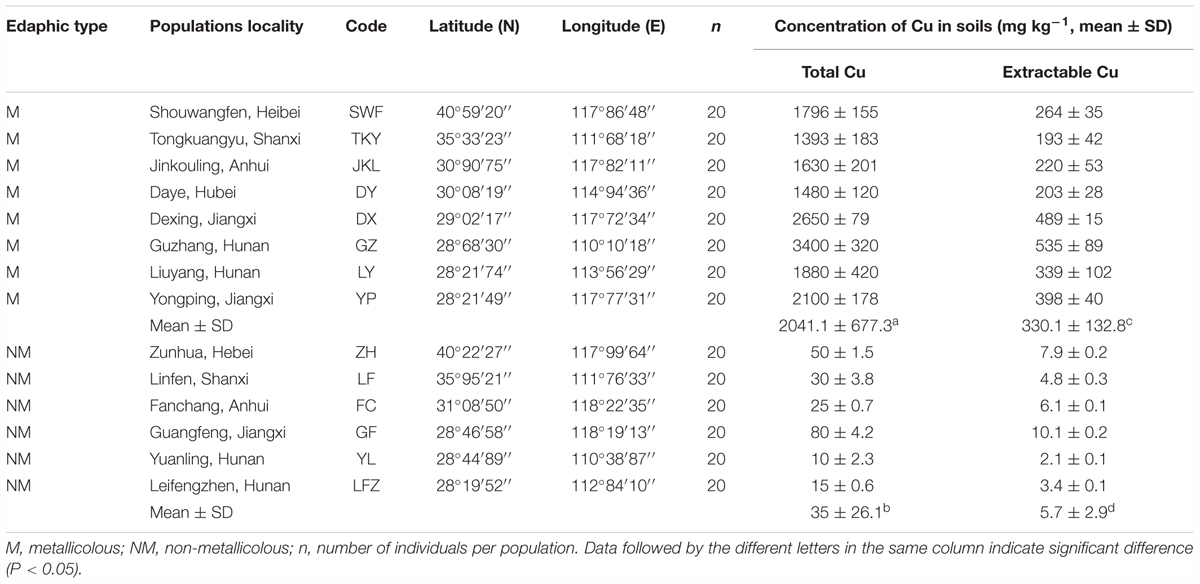
TABLE 1. Locations of 14 populations of Commelina communis, and characterizations of soils associated with plants.
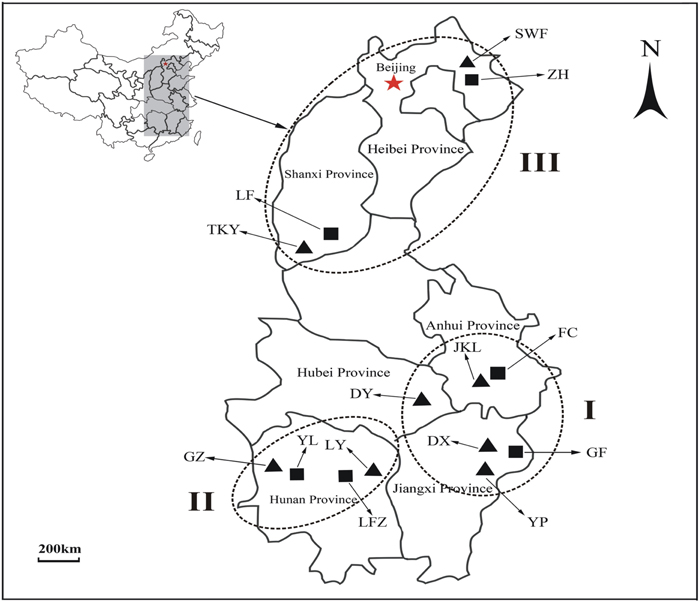
FIGURE 1. The geographical locations of 14 Commelina communis populations in this study. ▲ Metalliferous populations of C. communis; ■ non-metalliferous populations of C. communis. I, II, and III represent three different sampling groups.
Soil Chemical Analyses
Sampled soils were dried and sieved through a 2-mm mesh. The concentrations of total and extractable Cu in soils were analyzed using atomic absorption spectrometry (Hitachi, Japan). For the total Cu concentrations, prepared soils (three replicates per site) were cold-digested with HCl and HNO3 (3:1) for at least 12 h, then digested at 120°C for 2 h. For the extractable Cu concentrations, soil samples (three replicates per site) were extracted with diethylenetetraminepenta acid (DTPA) solution.
DNA Extraction and Microsatellite Analysis
The genomic DNA was extracted from dried leaves of C. communis using the DNA extraction Kit (Qiagen, Hilden, Germany). The quality of extracted DNA was measured by NanoDrop spectrophotometer (Thermo Scientific, USA).
Polymorphism was assayed on each DNA sample with 12 microsatellite markers developed in our previous study (Supplementary Table S1; Li et al., 2015b). The amplification reaction was carried out in 20 μL reaction mixture, containing 30–50 ng genomic DNA, 7.5 μL of 2×Taq PCR MasterMix (Tiangen, Beijing, China), 0.6 μM of each primer. Three fluorescent compounds (HEX, ROX or 6-FAM, Invitrogen, Carlsbad, CA, USA) were used to label the forward primers for the automated sequencers analysis (Supplementary Table S1). Amplification were carried out with an Eppendorf Mastercycler pro vapo protect thermocycler (AG, Hamburg, Germany), using the following thermocycling condition: initial denaturation at 95°C for 3 min, followed by 30 cycles of 30 s denaturation at 94°C, 30 s annealing at the optimal temperature (depending on each locus) and 1 min extension at 72°C, then 7 min at 72°C for the final extension. The ABI PRISM 3730XL DNA Sequencer (Applied Biosystems) and GeneMapper version 4.0 (Applied Biosystems) were used to separate the amplified products and determine the allele sizes, respectively.
Data Analysis
Genetic Diversity
The errors and null alleles in the microsatellite data were tested by MICROCHECKER 2.2.3 (Van Oosterhout et al., 2004). The linkage disequilibrium between all pairs of locus was checked by GENEPOP 4.0 (Raymond and Rousset, 1995). The P-values were corrected with sequential Bonferroni correction (Rice, 1989). To test for signs of positive and balancing selection on these loci, the FST-outlier approach (Beaumont and Nichols, 1996; Beaumont, 2005) implemented in LOSITAN (Antao et al., 2008) was used. The tests for outlier loci were performed using the stepwise mutation model with 100, 000 simulations and a confidence interval of 0.95. Loci that presented FST higher than the 95% confidence interval were considered candidates for divergent selection, and that presented FST lower than the 95% confidence interval were considered candidates for balancing selection. For each microsatellite locus, the observed (Ho) and expected (He) heterozygosity, the number of alleles (Na) and the Polymorphism Information Content (PIC) were calculated with PowerMarker v3.23 software (Liu, 2002). Meanwhile, the average number of alleles Nal, Ho, He in each population across all microsatellite loci were estimated with the same software. The allelic richness (Ar) and the number of ‘private’ alleles (Npr) for each population was estimated by FSTAT 2.9.3.2 (Goudet, 1995) and MICROSAT (Minch et al., 1997), respectively.
The average levels of Cu concentration and genetic variation between M and NM populations were compared with independent- t-test in the SPSS statistical package (IBM, Corp., Armonk, NY, USA). To determine whether there was a significant association between the geographic location and genetic diversity in C. communis populations, multiple regression analyses were performed in the SPSS with latitude and longitude as covariates and M-NM as fixed factor. Complementarily, we performed paired t-test to compare levels of genetic diversity between nearby pairs of populations established in metalliferous and non-metalliferous soils (SWF-ZH, TKY-LF, JKL-FC, DX-GF, GZ-YL, and LY-LFZ).
Population Genetic Structure
To identify the patterns of genetic structure in C. communis, a Bayesian model-based clustering, regardless of an individual’s geographic origin, was performed in STRUCTURE to infer the number of potential genetic clusters (K) (Pritchard et al., 2000). The clustering was conducted with the admixture model and correlated allele frequencies using the 100, 000 burn-in time periods and 1, 000, 000 Markov Chain Monte Carlo (MCMC) iterations. The different number of clusters (K) from 1 to 14 was tested with 10 independent runs. The most likely number of K was estimated from the posterior probability of the log-likelihood distribution Pr (X|K) (Pritchard et al., 2000) and its second-order rate of change (ΔK) (Evanno et al., 2005).
To assess the hierarchical partitioning of genetic variation in C. communis, an analysis of molecular variance (AMOVA) was performed with Arlequin 3.5.1 (Excoffier and Lischer, 2010). Two alternative population groups were examined: (1) a arrangement based on geographical locations in east-central China (Group I: JKL, DY, DX, YP, FC, GF) vs. sites in central China (Group II: GZ, LY, YL, LFZ) vs. sites in north China (Group III: SWF, TKY, ZH, LF; Figure 1); (2) a arrangement based on edaphic types with Cu-contaminated sites (Group M: SWF, TKY, JKL, DY, DX, GZ, LY, YP) vs. non-contaminated sites (Group NM: ZH, LF, FC, GF, YL, LFZ; Figure 1). In each analysis, 1, 000 permutations were used to test the significance levels of variance components. To test the correlation between the genetic (Pairwise FST) and geographic distances among populations of C. communis, the Mantel test was conducted in Arlequin 3.5.1 with 10, 000 permutations (Mantel, 1967; Excoffier and Lischer, 2010). Pairwise FST values for each population comparison were calculated with FSTAT 2.9.3.2 (Goudet, 1995). The geographic distances between populations were measured as the straight-line distances according to their coordinates. Moreover, multiple matrix regression with randomization (MMRR) was used to explicitly test the role of environment (soil composition) vs. geography on shaping observed patterns of genetic differentiation. MMRR was implemented with 10, 000 permutations in R with the MMRR function script (Wang, 2013). The regression analysis was based on distance matrices of genetic and geographical and a matrix of dissimilarity in copper concentration between the studied populations.
Phylogeographic Relationships among Populations
To explore the genetic relationships among C. communis populations, a Neighbor-joining (NJ) tree was constructed with Cavalli-Sforza’s and Edwards chord distance (Dc) based on microsatellite allele frequencies (Takezaki and Nei, 1996; Goldstein and Pollock, 1997). Bootstrap (10, 000 replicates) was performed to evaluate the node robustness. In addition, a principal coordinates analysis (PCoA) based on pairwise genetic difference was implemented with GenALEx version 6.5 (Peakall and Smouse, 2012) to validate the naturally occurring genetic clusters.
Bottleneck Analysis
When a population undergoes a recent bottleneck, its reduced effective population size exhibits a correlated reduction in allele numbers and heterozygosity at polymorphic loci. However, the number of alleles in bottlenecked population is more severely affected than heterozygosity, i.e., the allelic diversity is reduced faster than the heterozygosity. Therefore, the bottlenecked population is predicted to show an excess of heterozygosity. To detect the evidence for apparent bottleneck events in populations of C. communis, the program BOTTLENECK v 1.2.02 was used (Cornuet and Luikart, 1996; Piry et al., 1999). The two-phase mutation model (TPM) that has been considered as the best model for microsatellite data in BOTTLENECK was adopted in our study (Di Rienzo et al., 1994; Piry et al., 1999). The proportion of single step mutation events was set to 95%, with a variance of 12 (Piry et al., 1999). Wilcoxon sign-rank test was used to test the significant heterozygosity excess (Cornuet and Luikart, 1996; Piry et al., 1999).
Results
Characterization of Soil Conditions
An overview of geographical locations and soil characteristics for the 14 C. communis populations were presented in Table 1 and Figure 1. The average concentrations of total and extractable Cu in the metalliferous soils were significantly higher than those in non-metalliferous soils (Table 1). Compared to non- metalliferous areas, the average Cu concentrations was almost 50 times higher at the metalliferous sites (Table 1). In addition, large variances in Cu concentrations among the metalliferous sites were also detected. The highest values were reached up to 3400 ± 320 mg kg-1 for the total Cu and 535 ± 89 for the extractable Cu mg kg-1 at population GZ, whilst the lowest concentrations of total and extractable Cu were 1393 ± 183 and 193 ± 42 mg kg-1, respectively at population TKY.
Polymorphism of Microsatellite Loci
Over the 12 SSR loci, no evidence of null alleles or scoring error was detected using MICROCHECKER. Deviations from linkage equilibrium were not significant after Bonferroni corrections (P > 0.0001). A total of 135 different alleles were found across all the 14 populations of C. communis. The number of alleles among polymorphic loci ranged from four to 28, and the average value per locus was 11.25 (Supplementary Table S1). For each locus, the observed (Ho) and expected (He) heterozygosity ranged from 0.552 to 0.905 and 0.567 to 0.913, with a mean value of 0.764 and 0.771, respectively (Supplementary Table S1). The PIC values of the microsatellite loci varied from 0.535 to 0.901 in our study (Supplementary Table S1). LOSITAN analyses revealed that no loci were under directional or balancing selection (Supplementary Figure S1).
Population Genetic Diversity
Commelina communis showed high levels of genetic diversity (Table 2). The mean number of alleles (Nal) for M and NM populations of C. communis ranged from 1.833 (TKY) to 6.500 (DY) and 2.500 (LFZ) to 5.167 (GF), respectively. No significant associations between genetic diversity and population latitude (P = 1.0) and longitude (P = 0.9) were detected by multiple regression analyses. The parameters of average gene diversity indices were relatively high in most populations from metalliferous soils, with the average values of 3.558 for Ar, 0.440 for Ho and 0.555 for He (Table 2). There were no significant differences between M and NM populations in average Ar (t = 0.03, P = 0.976), Ho (t = 1.04, P = 0.319), and He (t = -0.23, P = 0.823; Table 2). Paired t-test considering nearby populations established in metalliferous and non-metalliferous soils did not show either significant difference in Ar (t = -1.12, P = 0.312), Ho (t = 1.23, P = 0.272), or He (t = -1.33, P = 0.239). Private alleles were detected in both M and NM populations of C. communis and no significant differences were observed in average Npr between M and NM populations (t = 1.84, P = 0.091; Table 2). However, the total number of private alleles within M populations (24) was almost fourfold that within NM populations (7). The populations with most private alleles were DY (7) and JKL (5) (Table 2).
Population Genetic Structure
The overall pairwise FST value was 0.325 (95% confidence interval: 0.213–0.443). Except three pairs of populations (FC and JKL, GF and YP, LFZ and LY), all the values of pairwise FST were significantly different from 0 (P < 0.05; Supplementary Table S2). The Mantel test indicated that there was a positive correlation between the genetic and geographical distance (r = 0.33; P < 0.05), indicating that geography is an important factors affecting the genetic structure of C. communis. Moreover, the results of MMRR revealed that the genetic distance had a significant correlation with geographic distance (βD = 0.57, P < 0.01). However, the effect of environmental factor (copper concentration) on genetic patterns of C. communis was not significant (βE = -0.17, P = 0.12; Figure 2).
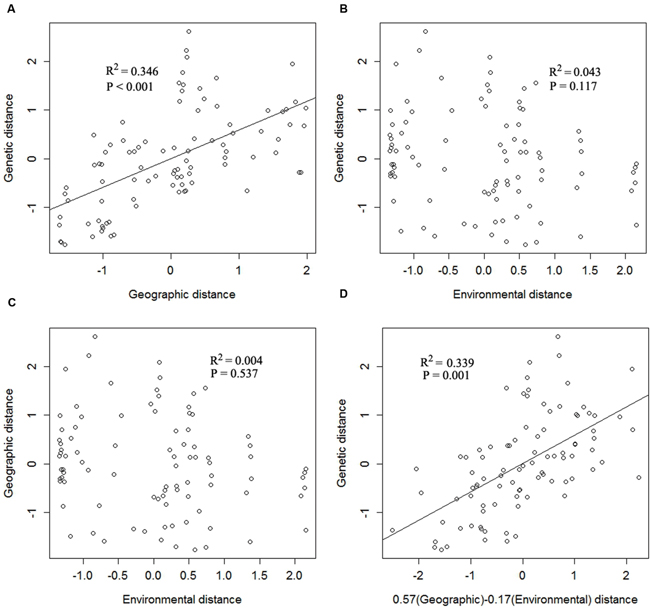
FIGURE 2. Multiple matrix regression with randomization analysis (MMRR) scatter plots showing the relationships of (A) geographical and genetic distance, (B) environmental and genetic distance, (C) environmental and geographical distance, and (D) the inferred multiple regression for the effects of geographical (βD = 0.57) and environmental distances (βE = -0.17) on genetic distance.
The number of subpopulations (K) was identified based on maximum likelihood and ΔK values. A clear peak in the value of ΔK was detected at K = 3 (Figures 3A,B). In general, three gene pools were evident. The north China populations were predominantly composed of a single gene pool, whereas the other two gene pools were mostly detected in the east-central China populations and the central China populations (Figure 3C). In addition, a general picture of admixture genotypes also emerged in our analysis and the ancestry of several genotypes in the 14 populations could be traced to more than one gene pool (Figure 3C). Several genotypes form one population shared alleles with other populations, indicating that these populations likely maintained frequent gene flow (Figure 3C). The PCoA results supported the findings from STRUCUTRE, demonstrating that genetic clustering of populations was in concordance with their geographic origins (Figure 4). However, there was an overlap of population LFZ from central China with population JKL from east-central China, indicating that intensive gene flow might occur between these two populations (Figure 4). The relationships between populations in the NJ tree showed that populations sampled from the metalliferous and non-metalliferous sites were not clearly separated, but divided into three distinct clusters, broadly corresponding to three geographical areas (Figure 5). All the populations located in east-central China formed one cluster (cluster I). The populations from central China and north China comprised another two major clusters (cluster II and cluster III). C. communis populations with close geographic distances had relatively low genetic divergence (Figure 5).
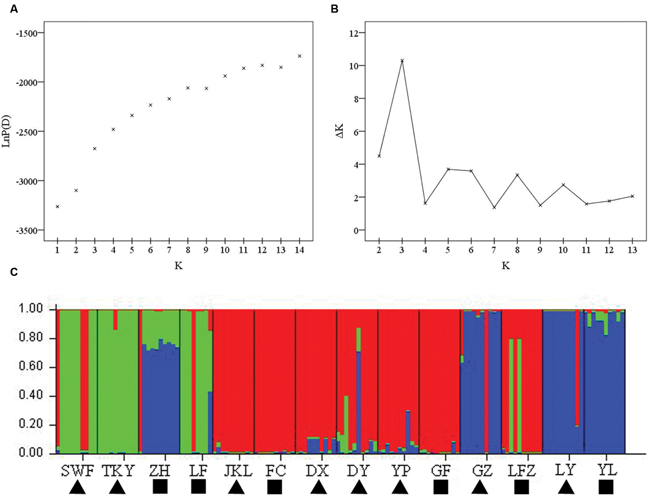
FIGURE 3. The Bayesian analysis results of C. communis based on SSR data. (A) Plot of mean posterior probability LnP(D) values of each K; (B) STRUCTRE estimation of the number of populations for K ranging 1–14 by delta K-value (ΔK); (C) the result of the assignment of individuals for K = 3. Individuals are represented as thin vertical lines partitioned into segments corresponding to the inferred genetic cluster as indicated by color and populations were marked on the bottom. The abbreviations of population name are same as Table 1.
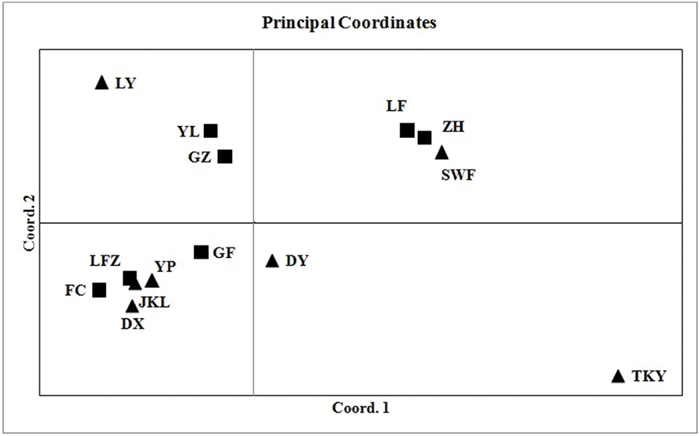
FIGURE 4. Principal coordinates analysis illustrating genetic differences among 14 populations of C. communis. Coordinate 1 is accounted for 42.57% and coordinate 2 for 28.77% of the total variation among populations. The abbreviations of population name are same as Table 1.
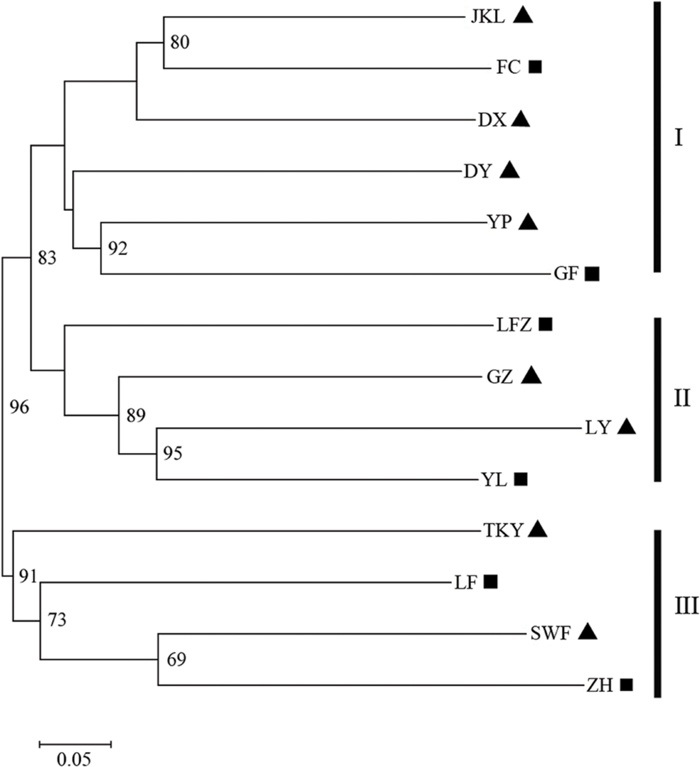
FIGURE 5. The Neighbor-joining (NJ) tree of C. communis accessions based on Cavalli-Sforza’s chord distance. Number at nodes represent the frequency (expressed as a percentage) at which a node occurred among 10, 000 replications. Bootstrap values higher than 60% from 1000 replicates are shown above the branches. The abbreviations of population name are same as Table 1.
The results of AMOVA showed that most of the genetic variability occurred within populations of C. communis (61.51 and 64.16% of total variance for geographic and edaphic grouping, respectively), although variation among subpopulations were significant in both groupings (P < 0.001; Table 3). Grouping of the individual samples according to geographical distribution allowed 10.47% of the total variation (P < 0.001) to be accounted for by differences among the three geographic origins (Table 3A). Edaphic separations, however, showed little contribution to the total genetic variation (2.26%, P = 0.95; Table 3B).
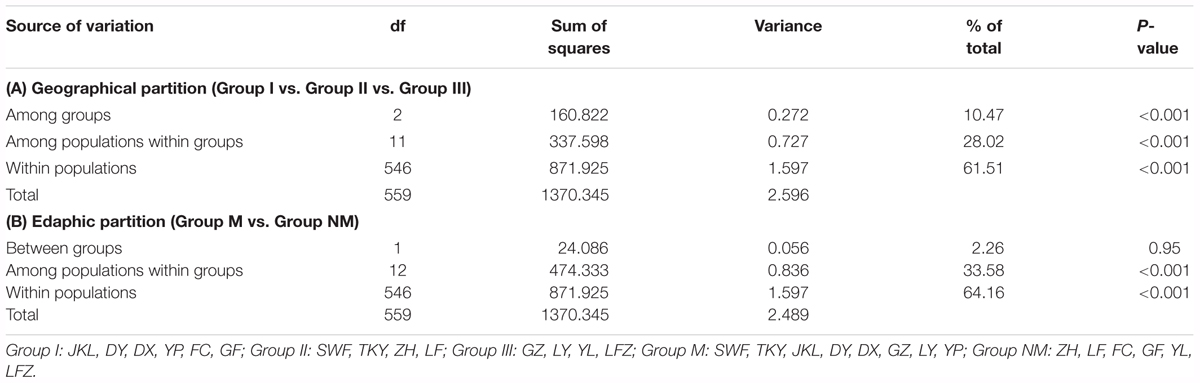
TABLE 3. The results of analysis of molecular variance (AMOVA) for C. communis populations based on SSR data (under two alternative grouping of populations: geographical and strictly edaphic).
Genetic Evidence for Population Bottlenecks
Bottleneck analysis under the TPM showed that there were no significant heterozygosity excesses in the 14 C. communis populations (P > 0.05, Table 4), indicating that all populations sampled from metalliferous areas did not experience apparent founder and bottleneck effects during their recent colonization.
Discussion
Our data showed that severe soil conditions at metalliferous areas did not significantly affect the levels of genetic variability in C. communis. The populations of C. communis from metalliferous soils had not undergone any apparent founder and bottleneck events during their colonization history. All sampled C. communis populations mainly clustered into three groups, corresponding well to their geographical locations rather than edaphic origins. Geographically distant M populations showed closer genetic similarity to their nearby NM populations than to each other. Both Mantel and MMRR analyses suggested that geographic distance played a more important role in determining the population structure of C. communis than soil composition did. Moreover, all Cu-contaminated populations branched into separated genetic groups, supporting the hypothesis of multiple and independent origins of M populations in C. communis.
Microsatellite Variation in Populations of C. communis
Heavy metal tolerance is a fundamental condition for pseudometallophytes to survive on metalliferous soils. The evolutionary scenario assumes that metal-tolerant genotypes initially have low frequencies in natural populations (Al-Hiyaly et al., 1993); therefore, significant reduction in genetic diversity should be expected in the founder populations on metalliferous areas (Lefèbvre and Vernet, 1990; Wu, 1990). In many pseudometallophyte species, e.g., Biscutella laevigata, Arabidopsis halleri, Silene paradoxa and Sedum alfredii, the levels of genetic diversity in M populations were significantly reduced due to strong founder and bottleneck effects (Mengoni et al., 2001; Deng et al., 2007; Meyer et al., 2010; Ye et al., 2012; Babst-Kostecka et al., 2014). However, contrary to the aforementioned assumption, the levels of genetic diversity were not significantly reduced in M populations of C. communis (Table 2). For example, the DY population originating from Cu-contaminated sites had the highest number of private alleles as well as the highest value of genetic diversity indices (Table 2). Moreover, in contrast to other studies (Pauwels et al., 2006; Deng et al., 2007; Meyer et al., 2010; Ye et al., 2012), our large-scale investigation indicated that all populations sampled from Cu-contaminated sites did not experience apparent founder and bottleneck effects (Table 4). These results suggested that the selective pressure caused by Cu contamination might be not perceivably stronger for C. communis populations on metalliferous soils, or its effect on genetic variability had been balanced by other factors.
Gene flow has been considered as an important factor affecting the genetic variability of M populations in pseudometallophytes (Muller et al., 2004; Pauwels et al., 2005; Bizoux et al., 2008). The spatial genetic structure of C. communis could be significantly determined by the interplay and balance between local genetic drift and gene flow. The flowers of C. communis lack nectaries, and generalist pollinators, such as flies and bees, have been reported as their main pollinators (Faden, 1992, 2000; Ushimaru et al., 2007; Li et al., 2015a). With the aid of insect pollination, the levels of gene flow between M populations and adjacent NM populations of C. communis could be significantly increased and the initial founder and bottleneck effects therefore might be partly eroded by the successive gene flow. In the present study, shared ancestry in C. communis populations was detected by STRUCTURE (Figure 3C). Several populations shared alleles with other populations in spite of their far geographic distance. For example, individuals from population ZH located in north-China had inferred ancestry from east-central and central China. Similarly, mixed inferred ancestry was also found in population LFZ (Figure 3C). The population LFZ from central China had a close genetic relationship with east-central China populations (Figure 4). There was an overlap of population LFZ from non-metalliferous soils with metalliferous population JKL from east-central China (Figure 4), indicating that intensive gene flow might occur. Moreover, the AMOVA results showed that geography only accounted for 10% of the total genetic variation in our study (Table 3). Thus, all these results suggested that gene flow might play an important role in maintaining high genetic diversity in populations of C. communis. In addition, frequent nuclear DNA mutations in the M populations also could increase the population genetic diversity and reverse the initial founder and bottleneck effects (Mengoni et al., 2001; Dresler et al., 2015). Of all C. communis populations, the DY population sampled from Cu contaminated soils had the greatest number of private alleles. In addition, the M opulations with higher number of private alleles usually showed higher level of genetic variability in our study (Table 2), suggesting that genomic mutations may enhance the levels of genetic diversity in M populations of C. communis. However, we have to keep in mind that our study was based on only a small set of randomly chosen microsatellites. Because SSRs are neutral markers (Supplementary Figure S1) and may also be in disequilibrium with Cu selected gene, such mutations could be just replenishing genetic diversity in neutral regions of the genome and the necessary mutations for coping with Cu might be still at low frequency in M populations. Therefore, the overall genomic effect of the bottleneck might be small and quickly erased by gene flow from nearby NM populations or from other M populations. It also should be pointed out that C. communis is an out-crossing species, which can produce a lot of seeds every year. Each plant produces 5–80 bisexual flowers and each fruit has 2 elliptic, or 1 elliptic and 2 semi-elliptic, or 4 semi-elliptic seeds (Hong and DeFillipps, 2000). Because the mature seeds easily drop on the ground from the dehiscent fruits (Li et al., 2015a), the buried seeds in the soil therefore could be stored and form a seed bank. Seed banks have been suggested as the genetic reservoirs, which can maintain genotypes overtime and contain significantly larger amounts of genetic diversity than expected (Mandák et al., 2012). However, genetic consequences of a seed bank may vary among species and/or populations as suggested by Mahy et al. (1999), and more detailed studies are needed in the future to assess the importance of the seed bank in shaping genetic diversity and structure in C. communis.
Genetic Differentiation between M and NM Populations of C. communis
Previous studies have shown that edaphic conditions (soil metal contamination) exert more influence than geographic distance on the genetic structure of pseudometallophytes (Deng et al., 2007; Parisod and Christin, 2008; Słomka et al., 2011; Ye et al., 2012; Babst-Kostecka et al., 2014). In contrast to previous studies, our investigation showed that the population structure of C. communis was mainly affected by geography rather than copper exposure. A significantly high level of genetic variability was found to be derived from the group of geographic partitions (P < 0.001), while that derived from the group of contaminated vs. non-contaminated populations was not significant (P = 0.95; Table 3). Both Mantel and MMRR analyses also indicated that the geographic distance rather than soil composition had a strong influence on genetic differentiation among populations of C. communis (Figure 2). In addition, M and NM populations were not clearly separated, but divided into three distinct clusters, broadly corresponding well with their geographical locations rather than edaphic types. Geographically adjacent C. communis populations showed relatively low genetic divergence, compared to populations far apart (Figure 5; Supplementary Table S2). Therefore, our data indicated that geography exerted more impact than copper exposure on the genetic structure of C. communis. Ye et al. (2012), however, suggested that Cu contamination played a more important role in shaping the population structure of C. communis than geographic distance. It should be noted that the average of total Cu concentrations (5404 ± 2151) and their extractable fractions (1196 ± 688) at metalliferous sites in Ye et al. (2012) are much higher than those in our study (Table 1), which may increase diverging selection and reduce gene flow between their study M and NM populations of C. communis. Moreover, ecogeographic distance has long been regarded as the most important factors to affect the gene flow in plants (Rieseberg and Willis, 2007). Although, we did find evidence of genetic differentiation between populations of C. communis likely due to the geographic distance, this is not an impervious barrier. Both STRUCTURE and PCoA results indicated a possible gene flow among populations in different regions (Figures 3 and 4). The population structure of C. communis was analyzed at a relatively small geographic scale in the previous research, concentrated and mainly limited in eastern China (Ye et al., 2012). The relatively close geographic distances among M populations of C. communis may mask the contribution of geography (Ye et al., 2012).
Because the metalliferous sites are often fragmented, the hypothesis of multiple and independent origins of M populations has been proposed and validated in some pseudometallophytes (Schat et al., 1996; Vekemans and Lefèbvre, 1997; Mengoni et al., 2001; Pauwels et al., 2005; Quintela-Sabarís et al., 2010). For C. communis, the geographically disjunctive M populations in our study clearly distributed into three distinct genetic clusters rather than one (Figures 3–5). All populations of C. communis from Cu-contaminated soils have closer genetic relationships with geographically adjacent NM populations than other distant conspecific M populations (Figures 4 and 5). Thus, our data indicated that the widely geographic distribution of M populations in C. communis today more likely evolved independently from nearby NM populations rather than from one single tolerant ancestral population (Ye et al., 2012).
Conclusion
This study clearly demonstrated that the extreme edaphic conditions in metalliferous areas had limited effects on the genetic variability in C. communis. The populations of C. communis from metal contaminated sites have not experienced apparent historical founder and bottleneck events during their recent colonization. The high levels of genetic diversity in the M populations of C. communis may be resulted from extensive gene flow between neighboring NM populations or geographically close M populations, high frequency of genetic mutations, and/or a high number of tolerant plants in the initially established populations. The patterns of genetic structure in C. communis were primarily influenced by geographic distance rather than soil composition. Thus, we speculate that geography plays a more important role than edaphic condition in shaping the genetic structure of C. communis. In addition, the widely distributed M populations of C. communis may have evolved independently from multiple origins.
Author Contributions
JL and WZ designed the study. HX and YS collected the data. JL, LT, YG, YL analyzed the data. JL and WZ drafted the manuscript. LS, RY, and XW critically revised the manuscript. All authors read and approved the final version of the manuscript.
Funding
This research was supported by the National Natural Science Foundation of P. R. China (Grant No. 31100173, 31000106, 31200382, 31470230, 51274268, and 50904080) and the China Postdoctoral Science Foundation (Grant No. 2015M582348, 2013M540643, and 2012M521562).
Conflict of Interest Statement
The authors declare that the research was conducted in the absence of any commercial or financial relationships that could be construed as a potential conflict of interest.
Acknowledgments
We thank Binbin Du, Huang Yu for their help in the field, and Jinming Chen for helpful discussion and advice. We also thank the reviewers for their helpful comments and suggestions.
Supplementary Material
The Supplementary Material for this article can be found online at: http://journal.frontiersin.org/article/10.3389/fpls.2016.01085
FIGURE S1 | Assessment of outlier SSR loci using LOSTIAN software at the 95% threshold. In red, the confidence area for candidate loci potentially under positive selection; in grey, the confidence area for neutral loci; in yellow, the confidence area for candidate loci potentially under balancing selection.
References
Al-Hiyaly, S. A. K., McNeilly, T., Bradshaw, A. D., and Mortimer, A. M. (1993). The effect of zinc contamination from electricity pylons. Genetic constraints on selection for zinc tolerance. Heredity 70, 22–32. doi: 10.1038/hdy.1993.4
Antao, T., Lopes, A., Lopes, R., Beja-Pereira, A., and Luikart, G. (2008). LOSITAN: a workbench to detect molecular adaptation based on a FST-outlier method. BMC Bioinformatics 9:323. doi: 10.1186/1471-2105-9-323
Antonovics, J., Bradshaw, A. D., and Turner, R. G. (1971). Heavy metal tolerance in plants. Adv. Ecol. Res. 7, 1–85. doi: 10.1016/S0065-2504(08)60202-0
Assunção, A. G. L., Bookum, W. M., Nelissen, H. J. M., Vooijs, R., Schat, H., and Ernst, W. H. O. (2003). Differential metal-specific tolerance and accumulation patterns among Thlaspi caerulescens populations originating from different soil types. New Phytol. 159, 411–419. doi: 10.1046/j.1469-8137.2003.00819.x
Babst-Kostecka, A. A., Parisod, C., Godé, C., Vollenweider, P., and Pauwels, M. (2014). Patterns of genetic divergence among populations of the pseudometallophyte Biscutella laevigata from southern. Plant Soil 383, 245–256. doi: 10.1007/s11104-014-2171-0
Baker, A. J. M., Ernst, W. H. O., Van der Ent, A., Malaisse, F., and Ginocchio, R. (2010). “Metallophytes: the unique biological resource, its ecology and conservational status in Europe, Central Africa and Latin America,” in Ecology of Industrial Pollution, eds L. C. Batty and K. B. Hallberg (New York, NY: Cambridge University Press), 7–54.
Baumbach, H., and Hellwig, F. H. (2007). Genetic differentiation of metallicolous and non-metallicolous Armeria maritime (Mill.) Willd. taxa (Plumbaginaceae) in Central Europe. Plant Syst. Evol. 269, 245–258. doi: 10.1007/s00606-007-0593-3
Beaumont, M. A. (2005). Adaptation and speciation: what can FST tell us? Trends Ecol. Evol. 20, 435–440. doi: 10.1016/j.tree.2005.05.017
Beaumont, M. A., and Nichols, R. A. (1996). Evaluating loci for use in the genetic analysis of population structure. Proc. R. Soc. B Biol. Sci. 263, 1619–1626. doi: 10.1098/rspb.1996.0237
Bizoux, J. P., Daïnou, K., Raspé, O., Lutts, S., and Mahy, G. (2008). Fitness and genetic variation of Viola calaminaria, an endemic metallophyte: implications of population structure and history. Plant Biol. 10, 684–693. doi: 10.1111/j.1438-8677.2008.00077.x
Bone, E., and Farres, A. (2001). Trends and rates of microevolution in plants. Genetica 11, 165–182. doi: 10.1023/A:1013378014069
Cornuet, J. M., and Luikart, G. (1996). Description and power analysis of two tests for detecting recent population bottlenecks from allele frequency data. Genetics 144, 1119–1127.
Deng, J., Liao, B., Ye, M., Deng, D., Lan, C., and Shu, W. (2007). The effects of heavy metal pollution on genetic diversity in zinc/cadmium hyperaccumulator Sedum alfredii populations. Plant Soil 297, 83–92. doi: 10.1007/s11104-007-9322-5
Di Rienzo, A., Peterson, A. C., Garza, J. C., Valdes, A. M., Slatkin, M., and Freimer, N. B. (1994). Mutational processes of simple-sequence repeat loci in human populations. Proc. Natl. Acad. Sci. U.S.A. 91, 3166–3170. doi: 10.1073/pnas.91.8.3166
Dresler, S., Tyrka, M., Szeliga, M., Ciurab, J., Wielboc, J., Wójcika, M., et al. (2015). Increased genetic diversity in the populations of Echium vulgare L. colonizing Zn-Pb waste heaps. Biochem. Syst. Ecol. 60, 28–36. doi: 10.1016/j.chemosphere.2010.12.081
Dubois, S., Cheptou, P. O., Petit, C., Vekemans, X., Meerts, P., Poncelet, M., et al. (2003). Genetic structure and mating systems of metalliferous and nonmetalliferous populations of Thlaspi caerulescens. New Phytol. 157, 633–641. doi: 10.1046/j.1469-8137.2003.00684.x
Ernst, W. H. O. (1996). “Phytotoxicity of heavy metals,” in Fertilizers and Environment, ed. C. Rodrigues-Barrueco (Dordrechett: Kluwer Academic Publishers), 423–430.
Ernst, W. H. O. (2006). Evolution of metal tolerance in higher plants. For. Snow. Landsc. Res. 80, 251–274.
Evanno, G., Regnaut, S., and Goudet, J. (2005). Detecting the number of clusters of individuals using the software STRUCTURE: a simulation study. Mol. Ecol. 14, 2611–2620. doi: 10.1111/j.1365-294X.2005.02553.x
Excoffier, L., and Lischer, H. E. L. (2010). Arlequin suite ver 3.5: a new series of programs to perform population genetics analyses under Linux and Windows. Mol. Ecol. Resour. 10, 564–567. doi: 10.1111/j.1755-0998.2010.02847.x
Faden, R. B. (1992). Floral attraction and floral hairs in the commelinaceae. Ann. Mo. Bot. Gard. 79, 46–52. doi: 10.2307/2399808
Faden, R. B. (2000). “Floral biology of commelinacea,” in Monocots: Systematics and Evolution, eds K. L. Wilson and D. A. Morrison (Melbourne: CSIRO Publishing), 309–317.
Goldstein, D. B., and Pollock, D. D. (1997). Launching microsatellites: a review of mutation processes and methods of phylogenetic inference. J. Hered. 88, 335–342. doi: 10.1093/oxfordjournals.jhered.a023114
Goudet, J. (1995). FSTAT (version 1.2): a computer program to calculate F-statistics. J. Hered. 86, 485–486.
Hoffman, A. A., and Parsons, P. A. (1991). Evolutionary Genetics and Environmental Stress. Oxford: Oxford University Press.
Hong, D. Y., and DeFillipps, R. A. (2000). “Commelina communis,” in Flora of China, eds Z. Y. Wu and P. H. Raven (Beijing: Science Press), 36.
Jarne, P., and Lagoda, P. J. L. (1996). Microsatellites, from molecules to populations and back. Trends Ecol. Evol. 11, 424–429. doi: 10.1016/0169-5347(96)10049-5
Jiménez-Ambriz, G., Petit, C., Bourrié, I., Dubois, S., Olivieri, S., and Ronce, O. (2006). Life history variation in the heavy metal tolerant plant Thlaspi caerulescens growing in a network of contaminated and noncontaminated sites in southern France: role of gene flow, selection and phenotypic plasticity. New Phytol. 173, 199–215. doi: 10.1111/j.1469-8137.2006.01923.x
Lefèbvre, C., and Vernet, P. (1990). “Microevolutionary processes on contaminated deposits,” in Heavy Metal Tolerance in Plants: Evolutionary Aspects, ed. A. J. Shaw (Boca Raton, FL: CRC Press), 285–300.
Li, J. K., Song, Y. P., Xu, H., Zhang, Y. W., Zhu, J. Y., and Tang, L. L. (2015a). High ratio of illegitimate visitation by small bees severely weakens the potential function of heteranthery. J. Plant Ecol. 8, 213–223. doi: 10.1093/jpe/rtv021
Li, J. K., Song, Y. P., Xu, H., Zhu, J. Y., and Tang, L. L. (2015b). Development and characterization of microsatellite loci for the pseudometallophyte Commelina communis (Commelinaceae). Appl. Plant Sci. 3:1400098. doi: 10.3732/apps.1400098
Linhart, Y. B., and Grant, M. C. (1996). Evolutionary significance of local genetic differentiation in plants. Annu. Rev. Ecol. Syst. 27, 237–277. doi: 10.1146/annurev.ecolsys.27.1.237
Liu, J. (2002). POWERMARKER – A powerful Software for Marker Data Analysis. Raleigh, NC: North Carolina State University.
Macnair, M. R. (1987). Heavy metal tolerance in plants: a model evolutionary system. Trends Ecol. Evol. 2, 354–359. doi: 10.1016/0169-5347(87)90135-2
Macnair, M. R., Tilstone, G. H., and Smith, S. E. (2000). “The genetics of metal tolerance and accumulation in higher plants,” in Phytoremediation of Contaminated Soil and Water, eds N. Terry and G. Bañuelos (Boca Raton, FL: CRC Press), 235–250.
Mahy, G., Vekemans, X., and Jacquemart, A. L. (1999). Patterns of allozymic variation within Calluna vulgaris populations at seed bank and adult stages. Heredity 82, 432–440. doi: 10.1038/sj.hdy.6884990
Mandák, B., Zákravský, P., Mahelka, V., and Plačková, I. (2012). Can soil seed banks serve as genetic memory? A study of three species with contrasting life history strategies. PLoS ONE 7:e49471. doi: 10.1371/journal.pone.0049471
Mantel, N. A. (1967). The detection of disease clustering and a generalized regression approach. Cancer Res. 27, 209–220.
Mengoni, A., Barabesi, C., Gonnelli, C., Galardi, F., Gabbrielli, R., and Bazzicalupo, M. (2001). Genetic diversity of heavy metal-tolerant populations in Silene paradoxa L. (Caryophyllaceae): a chloroplast microsatellite analysis. Mol. Ecol. 10, 1909–1916. doi: 10.1046/j.0962-1083.2001.01336.x
Mengoni, A., Gonnelli, C., Galardi, F., Gabbrielli, R., and Bazzicalupo, M. (2000). Genetic diversity and heavy metal tolerance in populations of Silene paradoxa L. (Caryophyllaceae): a random amplified polymorphic DNA analysis. Mol. Ecol. 9, 1319–1324. doi: 10.1046/j.0962-1083.2001.01336.x
Mengoni, A., Selvi, F., Cusimano, N., Galardi, F., and Gonnelli, C. (2006). Genetic diversity inferred from AFLP fingerprinting in populations of Onosma echioides (Boraginaceae) from serpentine and calcareous soils. Plant Biosyst. 140, 211–219. doi: 10.1080/11263500600756660
Meyer, C. L., Kostecka, A. A., Saumitou-Laprade, P., Créach, A., Castric, V., Pauwels, M., et al. (2010). Variability of zinc tolerance among and within populations of the pseudometallophyte Arabidopsis halleri and possible role of directional selection. New Phytol. 185, 130–142. doi: 10.1111/j.1469-8137.2009.03062.x
Minch, E., Ruiz-Linares, A., Goldstein, D., Feldman, M., and Cavalli-Sforza, L. L. (1997). MICROSAT (version 1.5d): A Computer Program for Calculating Various Statistics on Microsatellite Allele Data. Stanford, CA: Stanford University.
Muller, L. A. H., Lambaerts, M., Vangronsveld, J., and Colpaert, V. (2004). AFLP-bassed assessment of the effects of environmental heavy metal pollution on the genetic structure of pioneer populations of Suillus luteus. New Phytol. 164, 297–303. doi: 10.1111/j.1469-8137.2004.01190.x
Nevo, E. (2011). Evolution under environmental stress at macro- and microscales. Genome Biol. Evol. 3, 1039–1052. doi: 10.1093/gbe/evr052
Nordal, I., Haraldsen, K. B., Ergon, A., and Eriksen, A. B. (1999). Copper resistance and genetic diversity in Lychnis alpine (Caryophyllaceae) populations on mining sites. Folia Geobot. 34, 471–481. doi: 10.1007/BF02914923
Parisod, C., and Christin, P. A. (2008). Genome-wide association to fine scale ecological heterogeneity within a continuous population of Biscutella laevigata (Brassicaceae). New Phytol. 178, 436–447. doi: 10.1111/j.1469-8137.2007.02361.x
Parsons, P. A. (2005). Environments and evolution: interactions between stress, resource inadequacy, and energetic efficiency. Biol. Rev. Cam. Philos. Soc. 80, 589–610. doi: 10.1017/S1464793105006822
Pauwels, M., Frérot, H., Bonnin, I., and Saumitou-Laprade, P. (2006). A broad-scale analysis of population differentiation for Zn tolerance in an emerging model species for tolerance study: Arabidopsis halleri (Brassicaceae). J. Evol. Biol. 19, 1838–1850. doi: 10.1111/j.1420-9101.2006.01178.x
Pauwels, M., Saumitou-Laprade, P., Holl, A. C., Petit, D., and Bonnin, I. (2005). Multiple origin of metallicolous populations of the pseudometallophyte Arabidopsis halleri (Brassicaceae) in Central Europe: the cpDNA testimony. Mol. Ecol. 14, 4403–4414. doi: 10.1111/j.1365-294X.2005.02739.x
Peakall, R., and Smouse, P. E. (2012). GenALEx 6.5: genetic analysis in Excel. Population genetic software for teaching and research-an update. Bioinformatics 28, 2537–2539. doi: 10.1093/bioinformatics/bts460
Piry, S., Luikart, G., and Cornuet, J. M. (1999). BOTTLENECK: a computer program for detecting recent reductions in the effective population size using allele frequency data. J. Hered. 89, 502–503. doi: 10.1093/jhered/90.4.502
Pollard, A. J., Powell, K. D., Harper, F. A., and Smith, J. A. C. (2002). The genetic basis of metal hyperaccumulation in plants. Crit. Rev. Plant Sci. 21, 539–566. doi: 10.1080/0735-260291044359
Pritchard, J. K., Stephens, M., and Donnelly, P. (2000). Inference of population structure using multilocus genotype data. Genetics 155, 945–959. doi: 10.1111/j.1471-8286.2007.01758.x
Quintela-Sabarís, C., Vendramin, G. G., Castro-Fernández, D., and Fraga, M. I. (2010). Chloroplast microsatellites reveal that metallicolous populations of the Mediterranean shrub Cistus ladanifer L have multiple origins. Plant Soil 334, 161–174. doi: 10.1007/s11104-010-0368-4
Rajakaruna, N. (2004). The edaphic factor in the origin of plant species. Int. Geol. Rev. 46, 471–478. doi: 10.2747/0020-6814.46.5.471
Raymond, M., and Rousset, F. (1995). GENEPOP (version 1.2): population genetics software for exact tests and ecumenicism. J. Hered. 86, 248–249.
Rice, W. R. (1989). Analyzing table of statistical tests. Evolution 43, 223–225. doi: 10.2307/2409177
Rieseberg, L. H., and Willis, J. H. (2007). Plant speciation. Science 317, 910–914. doi: 10.1126/science.1137729
Schat, H., Vooijs, R., and Kuiper, E. (1996). Identical major gene loci for heavy metal tolerances that have independently evolved in different local populations and subspecies of Silene vulgaris. Evolution 50, 1888–1895. doi: 10.2307/2410747
Selkoe, K. A., and Toonen, R. J. (2006). Microsatellites for ecologists: a practical guide to using and evaluating microsatellite markers. Ecol. Lett. 9, 615–629. doi: 10.1111/j.1461-0248.2006.00889.x
Simon, E. (1978). Heavy metals in soils, vegetation development and heavy metal tolerance in plant populations from metalliferous areas. New Phytol. 81, 175–188. doi: 10.1111/j.1469-8137.1978.tb01616.x
Słomka, A., Sutkowska, A., Szczepaniak, M., Malec, P., Mitka, J., and Kuta, E. (2011). Increased genetic diversity of Viola tricolor L. (Violaceae) in metal-polluted environments. Chemosphere 83, 435–442. doi: 10.1016/j.chemosphere.2010.12.081
Sunnucks, P. (2000). Efficient genetic markers for population biology. Trends Ecol. Evol. 15, 199–203. doi: 10.1016/S0169-5347(00)01825-5
Takezaki, N., and Nei, M. (1996). Genetic distances and reconstruction of phylogenetic trees from microsatellite DNA. Genetics 144, 389–399.
Tang, S. R., Huang, C. Y., and Zhu, Z. X. (1997). Commelina communis L.: Copper hyperaccumulator found in Anhui Province of China. Pedosphere 7, 207–210.
Tang, S. R., Wilke, B. M., and Brooks, R. R. (2001). Heavy-metal uptake by metal-tolerant Elsholtzia haichowensis and Commelina communis from China. Commun. Soil Sci. Plant Anal. 32, 895–905. doi: 10.1081/CSS-100103915
Tang, S. R., Wilke, B. M., and Huang, C. Y. (1999). The uptake of copper by plants dominantly growing on copper mining spoils along the Yangtze River, the People’s Republic of China. Plant Soil 209, 225–232. doi: 10.1023/A:1004599715411
Ushimaru, A., Watanabe, T., and Nakata, K. (2007). Colored floral organs influence pollinator behavior and pollen transfer in Commelina communis (Commelinaceae). Am. J. Bot. 94, 249–258. doi: 10.3732/ajb.94.2.249
Van Oosterhout, C., Hutchinson, W. F., Wills, D. P. M., and Shipley, P. (2004). MICRO-CHECKER: software for identifying and correcting genotyping errors in microsatellite data. Mol. Ecol. 4, 535–538. doi: 10.1111/j.1471-8286.2004.00684.x
Vekemans, X., and Lefèbvre, C. (1997). On the evolution of heavy metal tolerant populations in Armeria maritima: evidence from allozyme variation and reproductive barriers. J. Evol. Biol. 10, 175–191. doi: 10.1046/j.1420-9101.1997.10020175.x
Wang, I. J. (2013). Examining the full effects of landscape heterogeneity on spatial genetic variation: a multiple matrix regression approach for quantifying geographic and ecological isolation. Evolution 67, 3403–3411. doi: 10.1111/evo.12134
Wójcik, M., Dresler, S., Jawor, E., Kowalczyk, K., and Tukiendorf, A. (2013). Morphological, physiological, and genetic variation between metallicolous and nonmetallicolous populations of Dianthus carthusianorum. Chemosphere 90, 1249–1257. doi: 10.1016/j.chemosphere.2012.09.068
Wu, L. (1990). “Colonization and establishment of plants in contaminated environments,” in Heavy Metal Tolerance in Plants: Evolutionary Aspects, ed. A. J. Shaw (Boca Raton: CRC Press), 269–282.
Ye, M., Liao, B., Mengoni, A., Hu, M., Luo, W. C., and Shu, W. S. (2012). Contrasting patterns of genetic divergence in two sympatic pseudo-metallophytes: Rumex acetosa L. and Commelina communis L. BMC Evol. Biol. 12:84. doi: 10.1186/1471-2148-12-84
Keywords: Commelina communis, genetic structure, heavy metal pollution, local adaptation, microsatellites, pseudometallophytes
Citation: Li J, Xu H, Song Y, Tang L, Gong Y, Yu R, Shen L, Wu X, Liu Y and Zeng W (2016) Geography Plays a More Important Role than Soil Composition on Structuring Genetic Variation of Pseudometallophyte Commelina communis. Front. Plant Sci. 7:1085. doi: 10.3389/fpls.2016.01085
Received: 25 April 2016; Accepted: 11 July 2016;
Published: 22 July 2016.
Edited by:
Renchao Zhou, Sun Yat-sen University, ChinaReviewed by:
Jacob A. Tennessen, Oregon State University, USAOctavio Salgueiro Paulo, University of Lisbon, Portugal
Joaquin Ortego, Spanish National Research Council, Spain
Copyright © 2016 Li, Xu, Song, Tang, Gong, Yu, Shen, Wu, Liu and Zeng. This is an open-access article distributed under the terms of the Creative Commons Attribution License (CC BY). The use, distribution or reproduction in other forums is permitted, provided the original author(s) or licensor are credited and that the original publication in this journal is cited, in accordance with accepted academic practice. No use, distribution or reproduction is permitted which does not comply with these terms.
*Correspondence: Weimin Zeng, emVuZ3dlaW1pbjEwMjRAMTI2LmNvbQ==