- 1Key Laboratory of Pesticide Toxicology and Application Technique, College of Plant Protection, Shandong Agricultural University, Tai'an, China
- 2Eco-environment and Plant Protection Research Institute, Shanghai Academy of Agricultural Sciences, Shanghai, China
Non-target-site resistance (NTSR) to herbicides is a worldwide concern for weed control. However, as the dominant NTSR mechanism in weeds, metabolic resistance is not yet well-characterized at the genetic level. For this study, we have identified a shortawn foxtail (Alopecurus aequalis Sobol.) population displaying both TSR and NTSR to mesosulfuron-methyl and fenoxaprop-P-ethyl, yet the molecular basis for this NTSR remains unclear. To investigate the mechanisms of metabolic resistance, an RNA-Seq transcriptome analysis was used to find candidate genes that may confer metabolic resistance to the herbicide mesosulfuron-methyl in this plant population. The RNA-Seq libraries generated 831,846,736 clean reads. The de novo transcriptome assembly yielded 95,479 unigenes (averaging 944 bp in length) that were assigned putative annotations. Among these, a total of 29,889 unigenes were assigned to 67 GO terms that contained three main categories, and 14,246 unigenes assigned to 32 predicted KEGG metabolic pathways. Global gene expression was measured using the reads generated from the untreated control (CK), water-only control (WCK), and mesosulfuron-methyl treatment (T) of R and susceptible (S). Contigs that showed expression differences between mesosulfuron-methyl-treated R and S biotypes, and between mesosulfuron-methyl-treated, water-treated and untreated R plants were selected for further quantitative real-time PCR (qRT-PCR) validation analyses. Seventeen contigs were consistently highly expressed in the resistant A. aequalis plants, including four cytochrome P450 monooxygenase (CytP450) genes, two glutathione S-transferase (GST) genes, two glucosyltransferase (GT) genes, two ATP-binding cassette (ABC) transporter genes, and seven additional contigs with functional annotations related to oxidation, hydrolysis, and plant stress physiology. These 17 contigs could serve as major candidate genes for contributing to metabolic mesosulfuron-methyl resistance; hence they deserve further functional study. This is the first large-scale transcriptome-sequencing study to identify NTSR genes in A. aequalis that uses the Illumina platform. This work demonstrates that NTSR is likely driven by the differences in the expression patterns of a set of genes. The assembled transcriptome data presented here provide a valuable resource for A. aequalis biology, and should facilitate the study of herbicide resistance at the molecular level in this and other weed species.
Introduction
Agrestal weeds reduce crop yields worldwide by 34%, on average, and are thus a considerable threat to food security (Oerke, 2005). Herbicides remain, by far, the most effective tools for weed control. However, weed control failure caused by herbicide resistance is an increasing and critical global problem. Herbicide resistance in weeds is now widely recognized as the consequence of the adaptive evolution of large and genetically variable weed populations to the intensive selection pressure exerted by applied herbicides (Jasieniuk et al., 1996; Powles and Yu, 2010). Because resistant weeds can survive herbicide applications through a variety of mechanisms (Powles and Yu, 2010; Beckie and Tardif, 2012; Délye et al., 2013), a better understanding of these mechanisms is crucial to devise strategies for more efficient weed control.
Currently, the mechanisms of resistance to herbicides may be divided into two broad categories: target-site resistance (TSR) and non-target-site resistance (NTSR). TSR, which arises from the overproduction of the target enzyme or from structural changes in the herbicide binding site, is now well-characterized in weeds (Délye et al., 2013). NTSR arises by any mechanism that reduces the concentration of herbicide reaching the target site (Preston, 2004; Yuan et al., 2007; Powles and Yu, 2010; Délye, 2013; Délye et al., 2013). An enhanced rate of herbicide metabolism (hereafter, “metabolic resistance”) is by far the dominant NTSR mechanism (Yu and Powles, 2014b). It often involves cytochrome P450 monooxygenases (CytP450s), glutathione S-transferases (GSTs), glucosyltransferases (GTs), ATP-binding cassette (ABC) transporters, as well as other enzyme systems, such as oxidases and hydrolases, which can also metabolize herbicides (Preston, 2004; Délye, 2013). Compared with TSR, NTSR is understudied because its mechanisms involve multiple gene activities (Petit et al., 2010a,b). Moreover, NTSR may confer to weeds an unpredictable resistance to herbicides irrespective of their mode of action (Petit et al., 2010b), and this clearly poses a very serious threat to sustainable chemical weed management.
Shortawn foxtail (Alopecurus aequalis Sobol.) is a diploid and partly cross-pollinated (c. 40%) winter-annual grass weed that is widely distributed in some overwintering crop regions in China (Guo et al., 2016). This weed has a strong tillering capacity that enhances its competitive ability against wheat (Triticum aestivum L.) seedlings, generating yield losses of more than 50% (Tang et al., 1990; Guo et al., 2015a). As a weed, A. aequalis is most efficiently controlled by herbicide applications. The excellent efficacy and selectivity of acetyl-CoA carboxylase (ACCase)- and acetohydroxyacid synthase (AHAS)-inhibiting herbicides have led to their widespread adoption for the post-emergence control of A. aequalis, but also its subsequent evolution of resistance (Yu and Powles, 2014b). Today, in some regions of China A. aequalis has evolved a high level of resistance to many herbicides with different mechanisms of action, such as fenoxaprop-P-ethyl and mesosulfuron-methyl. The ACCase gene mutations at Ile1781, Asp2078, or Ile2041, and the AHAS gene mutations at Pro197 or Trp574, were all found to decrease the enzyme sensitivities, the main driver of resistance in A. aequalis (Guo et al., 2015b, 2016; Xia et al., 2015). Although an evolved resistance to herbicides is likely due to TSR in many cases, NTSR is now considered the predominant mode of resistance in grass weeds to the ACCase- and AHAS-inhibiting herbicides (Délye, 2013). Moreover, TSR and NTSR can evolve together under the selection pressure exerted by herbicides: hence, both may occur within the same weed species, in the same population, or even in the same individual (Délye et al., 2010; Petit et al., 2010a). Unfortunately, researchers often neglect other coexisting resistance mechanisms after identifying the presence of TSR. Not surprisingly, the NTSR mechanisms in the herbicide-resistant A. aequalis have not yet been investigated.
Whole-transcriptome sequencing (RNA-Seq) technology is a powerful tool to unravel the genetic basis of the herbicide stress response in weeds (Unver et al., 2010; An et al., 2014), and to identify the genetic differences between the resistant and sensitive plants, before and after a herbicide application (Metzker, 2010). This exceptionally accurate technology has been applied to many non-model plant species, including weeds (Gaines et al., 2014; Türktaş et al., 2015). Recently, by using RNA-Seq, several genes were identified in weeds that confer NTSR to different herbicides, such as in rye-grass (Lolium rigidum Gaudin) (Gaines et al., 2014; Duhoux et al., 2015, 2017), black-grass (Alopecurus myosuroides Huds.) (Gardin et al., 2015), American sloughgrass (Beckmannia syzigachne Steud.) (Pan et al., 2016), and flixweed (Descurainia sophia L.) (Yang et al., 2016). Nevertheless, owing to the different herbicide-use histories and weed species, the genes involved in metabolic resistance might also be different (Délye et al., 2013; Yu and Powles, 2014b). Therefore, most of the genetic mechanisms underpinning NTSR remain largely unknown among different weed species, including A. aequalis.
In our prior study an A. aequalis population with multiple resistance to mesosulfuron-methyl and fenoxaprop-P-ethyl was identified; two amino acid substitutions, Pro197Arg in the AHAS gene and Ile2041Asn in the ACCase gene, were confirmed in the individual plants of this resistant population (Guo et al., 2016). Nevertheless, CytP450s may play an important concurrent role in the fenoxaprop-P-ethyl resistance because the CytP450s inhibitor, piperonyl butoxide (PBO), can significantly reduce the GR50 (i.e., the herbicide rate causing a 50% growth reduction in plants) (Pan et al., 2015). Cross-resistance patterns were also identified and they indicated the existence of metabolic resistance in the resistant A. aequalis population (Guo et al., 2016). In the present study, the mechanism of NTSR to mesosulfuron-methyl was also confirmed by applying the CytP450 inhibitor, malathion. In particular, both an RNA-Seq transcriptome analysis and qRT-PCR experiments were conducted to identify and validate the specific genes involved in NTSR to mesosulfuron-methyl in the A. aequalis study population.
Materials and Methods
Plant Materials and Growth Conditions
The collection and storage of seeds from the resistant (R, AH18-origin) and susceptible (S, SD01-origin) A. aequalis populations were described in Guo et al. (2016). The sensitivity of these two populations was tested; a purified R population (AH18) homozygous for the ACCase and AHAS gene mutations and a confirmed S population (SD01) were both acquired (Guo et al., 2016). Prior to their planting, the seeds were germinated as described by Guo et al. (2015a). The pre-germinated seeds were sown in 12-cm-diameter, 11-cm-deep plastic pots (20 seeds per pot) containing moist loam soils. Next, the pots were randomly placed in a controlled greenhouse (natural light, 25/15°C, ~75% relative humidity), where they were watered every other day. For each pot, the weed seedlings that emerged were thinned to 15 that were evenly sized at the two- to three-leaf stage.
Sensitivity to Mesosulfuron-Methyl Following the Cytp450s Inhibitor Malathion
Whole-plant dose-response experiments were carried out to determine the GR50 values of the purified R (AH18) and confirmed S (SD01) populations to mesosulfuron-methyl in the absence and presence of malathion. The malathion used in this experiment was formulated in a mixture of a commercial solvent and an emulsifier. The mesosulfuron-methyl was applied by using a commercial formulation—30 g L−1 oil-miscible flowable concentrate (Bayer, Hangzhou, China)—diluted with distilled water.
When the A. aequalis seedlings reached the three- to four-leaf stage they were ready for use in the whole-plant dose-response experiments. These experiments were done twice in a completely randomized design that had three replicates. In each experimental repeat there was a total of 84 pots used (2 malathion rates × 2 populations × 3 replicates × 7 treatments). Malathion was applied as an active ingredient (ai), at a rate of 0 or 1,000 g ai ha−1, at 1 h before the herbicides were applied (Preston et al., 1996). Mesosulfuron-methyl application rates of 0, 3, 9, 27, 81, 243, and 729 g ai ha−1 were used to treat the R plants, while rates of 0, 0.04, 0.11, 0.33, 1, 3, and 9 g ai ha−1 were used on the S plants. These herbicide application rates were adopted to represent the GR50 value for each population in the absence of CytP450 inhibitors. The control plants were treated with the mixture (commercial solvent and emulsifier) used to formulate the malathion. All the herbicides were applied by using an air-compressed, moving-nozzle cabinet sprayer which delivered 450 L ha−1 of water at a pressure of 0.275 MPa, via a flat-fan nozzle positioned 50 cm above the foliage. After being treated, the experimental plants were returned to the controlled greenhouse. Three weeks later, their aboveground shoots were harvested and oven-dried at 80°C for 72 h and their dry weight data were recorded.
Plant data obtained from the mesosulfuron-methyl dose-responses were expressed in relative terms, as a percentage of the control. The datasets from the replicate experiments were analyzed by ANOVA that used the general linear model procedure in SPSS v.19.0.0 (IBM Corporation, Armonk, USA). Because the variance among the replicated experiments was not significant (p > 0.05), the data were pooled and fitted to a non-linear regression analysis in SigmaPlot v.12.0 (Systat Software, Inc., San Jose, USA). The GR50 was determined by using a four-parameter log-logistic equation (Seefeldt et al., 1995). The fitted model was as follows:
where C is the lower limit of the response, D is the upper limit of the response, X is the herbicide application rate, and b is the slope of the curve at the GR50.
To estimate the resistance levels, the resistance index (RI) was calculated as follows:
Sample Collection
Ten seeds were randomly selected from the purified R (AH18) and confirmed S (SD01) populations, respectively, for germination and onward cultivation to maturity. Mature seeds (AH18-F1/SD01-F1) were collected from each individual plant. To study metabolic resistance, one seed from each individual-plant progeny was randomly selected to make up a 10-seed combination. The experimental design included three biological replicates of R and S for the untreated control (CK), water-only control (WCK), and mesosulfuron-methyl (T; 1 × labeled field rate, 9 g ai ha−1) treatments, for a total of eighteen 10-seed combinations of the R and S biotype.
The selected 180 seeds were germinated and cultivated to the three- to four-leaf stage under the above-mentioned experimental conditions. Leaf samples for the untreated control were collected at time point 0 (R_CK/S_CK), while those from the water-only control (R_WCK/S_WCK) and the mesosulfuron-methyl treatments (R_T/S_T) were collected 24 h after the treatments were applied. Each sample consisted of a two-leaf mixture: a 4-cm-long, newest emerging leaf, and a 3-cm-long, first fully expanded leaf (both taken from each of the 10 selected plants). A total of 18 samples were thus collected (3 biological replicates × 3 treatments × 2 populations). All of these samples were frozen immediately in liquid nitrogen to avoid RNA degradation and/or induction of plant responses to wounding; they were then pulverized for the total RNA extraction. The remaining seeds (AH18-F1/SD01-F1) from each individual-plant progeny were used in parallel to those for RNA-Seq—they were likewise planted, cultivated to the three- to four-leaf stage, and used as plant materials in the subsequent validation experiment by quantitative real-time reverse transcription polymerase chain reaction (qRT-PCR).
RNA Extraction, cDNA Library Preparation, and Illumina Sequencing
The total RNAs from the 18 samples were extracted with Transzol Up (TransGen Biotech, Beijing, China) according to the manufacturer's protocol and they were treated with DNase I (Takara, Beijing, China). Total RNA degradation and contamination were monitored on 1% agarose gels. RNA purity was checked using a NanoPhotometer® spectrophotometer (IMPLEN, West Lake Village, USA), and the RNA concentration was measured using a Qubit RNA Assay Kit and a Qubit 2.0 Fluorometer (Life Technologies, Gaithersburg, USA). The RNA integrity was assessed with a RNA Nano 6000 Assay Kit as part of the Agilent Bio analyzer 2,100 system (Agilent Technologies, Palo Alto, USA). The highest-quality RNA samples were selected for preparing the cDNA library.
The cDNA library construction and the Illumina sequencing were both performed by Annoroad Gene Technology Co., Ltd (Beijing, China). Briefly, the sequencing libraries were generated via a NEBNext Ultra™ RNA Library Prep Kit for Illumina (NEB, Ipswich, USA) following the manufacturer's instructions. A total of 3 μg of RNA per sample was used as the input material for the RNA-sample preparations. Poly (A) mRNA was purified from total RNA by using poly-T oligo-attached magnetic beads, and then fragmented into short sequences. These cleaved RNA fragments were transcribed into first-strand cDNA by a Moloney Murine Leukemia Virus (M-MuLV) Reverse Transcriptase (RNase H-) and by random hexamer primers; this step was followed by a second-strand cDNA synthesis in a reaction buffer which included dNTPs, DNA polymerase I, and RNase H. After end-repair and adapter-ligation, the products were amplified through PCR and purified by using a QIAquick PCR Purification Kit (QIAGEN, Valencia, USA) to construct the cDNA library. The cDNA libraries were sequenced on the Illumina Hiseq™ 4000 platform (Illumina Inc., San Diego, USA) and 150-bp paired-end reads were generated.
RNA-Seq Data Filtering, de novo Transcriptome Assembly, and Gene Functional Annotation
Raw image data files from the Illumina Hiseq™ 4000 were transformed to raw reads by the Consensus Assessment of Sequence and Variation (CASAVA, v.1.8.2) base recognition program, and then stored in FASTQ-formatted files. Raw reads containing the adapter poly-N (>5% of the unknown sequences designated as “N”) and the low-quality reads (>15% bases with a quality value <20) were filtered out by in-house Perl scripts to obtain high-quality, clean reads. All downstream analyses were based only on these clean reads of high quality.
A de novo transcriptome assembly was accomplished in Trinity v.20140717 (Grabherr et al., 2011) under its default parameter values, by which the transcripts and unigenes—the longest transcript of a set of transcripts that appears to originate from the same transcription locus—were obtained. To assess the accuracy of assembly, all of the unigenes were matched to the sequences of an annual model plant, false-brome (Brachypodium distachyon [L.] Beauv.), using the BLAST-Like Alignment Tool (BLAST) with E ≤ 1e-20. The assembled transcripts were translated into all six possible open reading frames (ORFs) through TransDecoder v.20140717, and their proper translation was defined as the one that gave the longest amino acid sequence (Saha et al., 2002).
To obtain comprehensive, functional information regarding the sequences, gene function was annotated through publicly accessible databases—NCBI non-redundant protein sequences (Nr), NCBI non-redundant nucleotide sequences (Nt), Universal Protein Resource (UniProt), protein family (PFAM), and orthologous groups and functional annotation (eggNOG)—by means of local BLAST programs (NCBI, NIH, Bethesda, USA) which used a significance threshold of E < 1e-5. Meanwhile, the software programs HMMER v.3.1b1 (Finn et al., 2011), signalIP v.4.1 (Petersen et al., 2011), and TMHMM v.2.0 (Krogh et al., 2001) in Trinotate were run to identify the protein domains, to predict the signal peptides, and to locate the trans membrane regions, respectively.
Next, the gene ontology (GO, http://www.geneontology.org/) annotation for the unigenes was implemented with Blast2go (Conesa et al., 2005), set to an E-value cutoff = 1e-5. The GO functional classifications were performed using WeGO software (http://wego.genomics.org.cn/cgi-bin/wego/index.pl) (Ye et al., 2006). The pathway assignments were carried out by sequence searches against the Kyoto Encyclopedia of Genes and Genomes database (KEGG, http://www.kegg.jp/), which used the KEGG Automatic Annotation Server (KAAS, http://www.genome.jp/kegg/kaas/) set to an E-value threshold < 1e-10.
Identification and Analysis of the Differentially Expressed Genes (DEGs)
The assembled transcriptome was used as the reference database, and the clean reads were mapped back onto the reference transcriptome by Bowtie 2 v.2.2.3 (Langmead and Salzberg, 2012). The read count for each gene in each sample was obtained from the mapping results by RSEM (RNA-Seq by Expectation Maximization) (Li and Dewey, 2011); then, the mapped read counts for each transcript were normalized, to eliminate the effects of the sequencing depth and gene length on the gene expression levels, by RPKM (Reads Per Kilobase Millon Mapped Reads) (Mortazavi et al., 2008). In this way, the gene expression levels were determined for each sample and could be directly compared. Next, the differences in the expression abundance of each gene per transcript between sample pairs were calculated by using DESeq 2 v.1.4.5 (Love et al., 2014). Each resulting p-value was adjusted to a q-value, following the Benjamini-Hochberg procedure for controlling the false discovery rate (Storey and Tibshirani, 2003). Those genes with a q < 0.05 and |log2(fold change)| ≥ 1 were identified as DEGs.
Expression differences were compared between the untreated R and untreated S at time point 0 (R_CK and S_CK), between R and S at 24 h after the water control treatment (R_WCK and S_WCK), and between R and S at 24 h after the mesosulfuron-methyl treatment (R_T and S_T). Expression differences were also compared within R and S among the untreated (CK), water-only control (WCK), and mesosulfuron-methyl treatments (T). The DEGs were later analyzed by GO and a KEGG enrichment analysis. Both analyses were implemented with a hyper geometric test (Young et al., 2010), in which the p-value was adjusted for multiple comparisons as the q-value, and the background data are the genes in the whole genome. Those GO or KEGG terms with a q < 0.05 were considered as significantly enriched.
Selection and qRT-PCR Validation of the Candidate Metabolic Resistance Contigs
To select candidate contigs for metabolic resistance, three factors were simultaneously considered: their statistical significance, their magnitude of expression differences, and their annotations related to known herbicide metabolism genes and signaling functions. The expression level of each contig was first measured by qRT-PCR in the original RNA samples that were used in the RNA-Seq experiment. For the normalization of gene expression and to ensure the reliability of the qRT-PCR data, the ubiquitin (UBQ, JN599096.1) and glyceraldehyde-3-phosphate dehydrogenase genes (GAPDH, JN599100.1) were used as the internal control genes (Petit et al., 2012). Gene-specific primers were designed according to the sequences of the selected unigenes in Primer Premier v.5.0 (Premier Biosoft International, Palo Alto, USA). The sequences of these primers are listed in Supplementary Table S4.
The qRT-PCR was performed in 96-well plates on the Bio-Rad CFX96 Touch™ real-time PCR system (Bio-Rad, Richmond, USA), which used the TransStart® Top Green qPCR SuperMix (TransGen Biotech, Beijing, China). Each reaction was conducted in a 20-μL mixture—consisting of 10 μL of 2 × TransStart® Top Green qPCR SuperMix, 0.4 μL of forward primer, 0.4 μL of reverse primer, 0.4 μL of cDNA, and 8.8 μL of RNase-free ddH2O—with four replicates per cDNA sample. The qRT-PCR programs consisted of a 2-min incubation phase at 95°C, followed by 45 cycles, with each cycle consisting of a 10-s incubation at 95°C, a 15-s incubation at 60°C, and a 20-s incubation at 72°C. At the end of the amplification cycle, melting curve analyses were performed to confirm the specificity of qRT-PCR primers. The amplification efficiencies were derived from a standard curve generated by a 10-fold serial dilution points of cDNA (Pfaffl, 2001). A similar amplification efficacy (87.6–99.7%) for the target and internal control genes was observed. The fold-change in gene expression (as 2−ΔCt) was calculated by using the comparative Ct method (Schmittgen and Livak, 2008), relative to the S samples, where ΔCt = [Ct target gene-Ct mean of the two internal control genes]; three biological replicates were obtained. Statistical analysis of the qRT-PCR data was conducted using the Student's t-test (p < 0.05) procedure of SPSS software.
Furthermore, the expression patterns of those contigs, whose relative expression data provided by qRT-PCR agreed with the RNA-Seq-detected profiles (i.e., the contigs whose expression levels were significantly higher in the R than in the S samples in both the RNA-Seq and qRT-PCR experiments), were also examined in the parallel plant materials—for which the seed germination, plant cultivation, and total RNA extraction followed the above-mentioned methods. Their cDNA was later obtained, and qRT-PCR was carried out using the same primers and procedure as before.
Results
Mesosulfuron-Methyl Dose-Response in the Absence and Presence of the CytP450s Inhibitor Malathion
The whole-plant dose-response experiments demonstrated that the purified R population (AH18) had evolved a high level resistance (RI = 31.15-fold) to mesosulfuron-methyl (Table 1); this was consistent with our previous report (Guo et al., 2016). When malathion alone was applied at 1,000 g ai ha−1, there were no visual effects on A. aequalis seedling growth, in either the R or S population (Figure 1). Importantly, malathion greatly reduced the resistance level of the R population when it was used before the mesosulfuron-methyl treatment. Under a malathion pretreatment of 1,000 g ai ha−1, the GR50 value of mesosulfuron-methyl decreased by 41% for the R population (Table 1, Figure 2). By contrast, malathion did not lead to any obvious increase in the susceptibility of the S population to mesosulfuron-methyl, as its GR50 decreased little (Table 1). Malathion, the P450 inhibitor, has long been used as an indicator of CytP450 involvement in metabolic resistance to AHAS-inhibiting herbicides. The observation that, in our present study, malathion caused a significant reduction in the resistance level when it was applied with the herbicide indicated the involvement of at least one CytP450 gene in the resistance mechanisms operating in the R biotype.
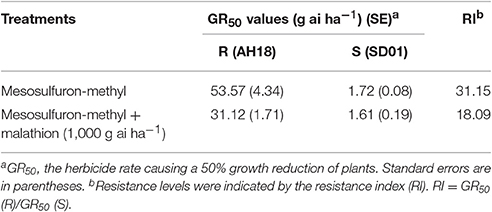
Table 1. The effects of mesosulfuron-methyl on Alopecurus aequalis growth with and without malathion pretreatment.
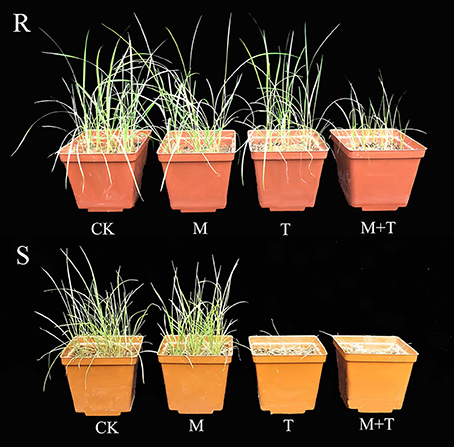
Figure 1. Photographs of R (top) and S (bottom) Alopecurus aequalis plants 42 days after treatment, showing the untreated (CK), the malathion-only control (M; 1,000 g ai ha−1), the mesosulfuron-methyl treatment (T; 9 g ai ha−1), and the effects of the malathion plus mesosulfuron-methyl (M+T; malathion was applied at 1,000 g ai ha−1 1 h prior to the mesosulfuron-methyl application).
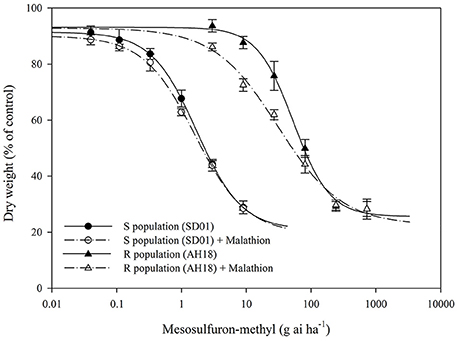
Figure 2. Dose-response curves for the dry weight of the R (AH18) and S (SD01) Alopecurus aequalis populations treated with a range of mesosulfuron-methyl doses with (+) or without 1,000 g ai ha−1 of malathion. The values are expressed as the percentage of the untreated control. Each data point is the mean ± SE of twice-repeated experiments. The lines were fitted to the mean values using “four-parameter log-logistic equation”.
Illumina Sequencing and de novo Assembly
Using the Illumina sequencing technology, a total of 975,586,352 raw sequencing reads were generated from the 18 RNA libraries (S_CK, R_CK, S_WCK, R_WCK, S_T, and W_T; each had three biological replicates). After the quality control and data cleaning, there were 831,846,736 clean reads left over, ranging from 49,270,938 to 60,784,678 per sample, for use in the de novo assembly (Table 2, Supplementary Table S1). The clean reads assembled to 179,989 transcripts, with an average length of 1,233 bp. Figure 3 shows the length distribution of these transcripts. Of the 831,846,736 clean reads sequenced, we were able to map the great majority of them (93.02%) back onto the transcripts using Bowtie 2. Among these transcripts, 43,290 unigenes >200 bp and 14,352 unigenes >500 bp, with an overall mean length of 944 bp and an N50 length of 1,857 bp, were obtained by using the longest transcript at each locus of each gene. The length distribution of these unigenes is also shown in Figure 3. B. distachyon is now intensively utilized as a model grass species in various biological studies (Draper et al., 2001). By using the BLAST search, 34.40% of the unigenes were successfully matched to the sequences of B. distachyon, at an average accuracy of 84.18%.
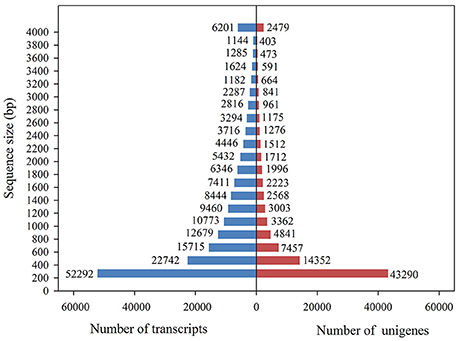
Figure 3. Overview of the transcriptome assembly data for Alopecurus aequalis, showing the size frequency distributions of the transcripts and unigenes.
Gene Functional Annotation and Classification
The numbers of unigenes annotated by the databases are summarized in Table 3. Of the 95,479 assembled unigenes, over half were annotated in at least one of the seven databases, whereas <5% had annotations in all seven databases, and the greatest sequence similarity was obtained from the Nr database (Table 3). Based on the Nr database annotations, the unigene sequences of our study species, A. aequalis, were most similar to the gene sequences from B. distachyon, Hordeum vulgare subsp. vulgare, Aegilops tauschii Coss., Triticum Urartu L., Oryza sativa L. Japonica, and Triticum aestivum L. (Figure 4).
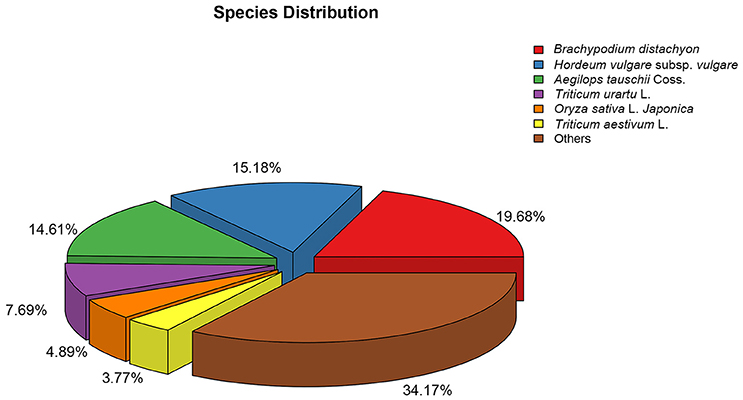
Figure 4. Species distributions of the BLASTX matches of the Alopecurus aequalis transcriptome unigenes.
To characterize the functional classifications of the annotated unigenes, GO and KEGG analyses were performed to access the distributions of functional categories. A total of 29,889 (29.29%) unigenes were annotated by the GO database; these were classified into 67 functional subgroups consisting of 23 subgroups as “biological process” (BP), 22 as “cellular component” (CC), and 22 as “molecular function” (MF) (Figure 5). In the BP category, cellular process (18,218, 60.95%) and metabolic process (17,088, 57.17%) represented the two major contributors. In the molecular function category, binding (18,469, 61.79%) and catalytic activity (16,732, 55.98%) represented the major contributors. In addition, 14,246 unigenes were assigned to 32 KEGG pathways; these unigenes were primarily involved in “carbohydrate metabolism” (1,722, 12.09%), “translation” (1,244, 8.73%), “signal transduction” (1,228, 8.62%), and “amino acid metabolism” (1,200, 8.42%)” (Figure 6).
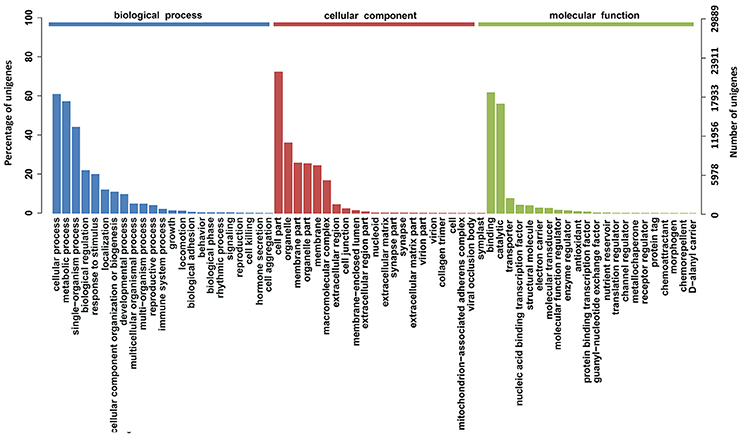
Figure 5. GO function classification of the annotated unigenes in Alopecurus aequalis. The unigenes were allocated to three categories: biological process, cellular component, and molecular function.
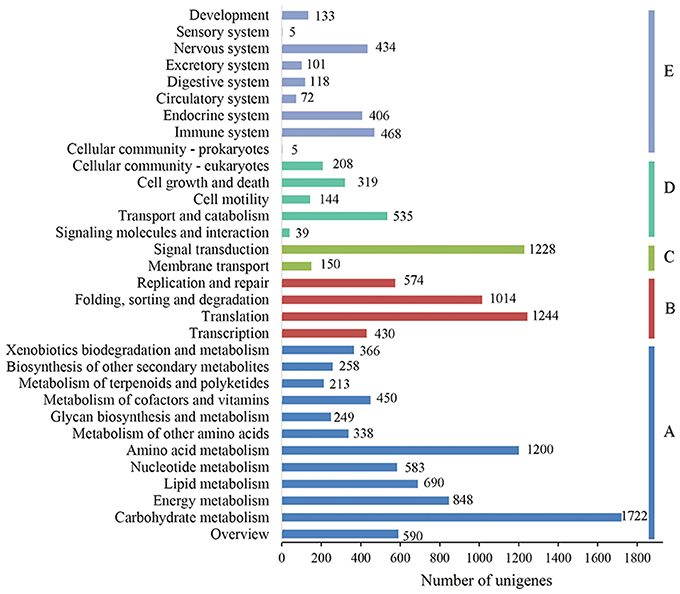
Figure 6. KEGG function classification results of the annotated unigenes in Alopecurus aequalis. The y-axis lists the various KEGG pathways. The x-axis indicates the number of genes. According to their participation in these KEGG pathways, the unigenes were divided into five color-coded groups: metabolism (A), genetic information processing (B), environmental information processing (C), cellular processes (D), and organismal systems (E).
Differential Gene Expression and Functional Analysis
Constitutive differential expression (i.e., q < 0.05 and |log2(fold change)|≥1) was apparent between the untreated R and S samples, such that 5,840 contigs were constitutively up-regulated in R, and 5,648 contigs were up-regulated in S (Figure 7A). Differential expression between R and S in all three treatments was evident for 5,654 contigs (Figure 7B). When comparing the mesosulfuron-methyl treatment with the water-only control, a total of 5,549 contigs were up-regulated in R, and 5,649 contigs were up-regulated in S. However, considering only those contigs up-regulated by the mesosulfuron-methyl treatment revealed an overlap of 409 contigs between the R and S populations, including contigs putatively annotated as 10 CYPs, 1 GST, 4 GTs, and 2 ABC transporters (Supplementary Table S2). DEGs between the mesosulfuron-methyl treatments and the untreated control were also identified: there were 5,837 contigs up-regulated and 4,492 contigs down-regulated in R_T relative to R_CK, as well as 8,561 contigs up-regulated and 6,859 contigs down-regulated in S_T relative to S_CK (Figure 7A).
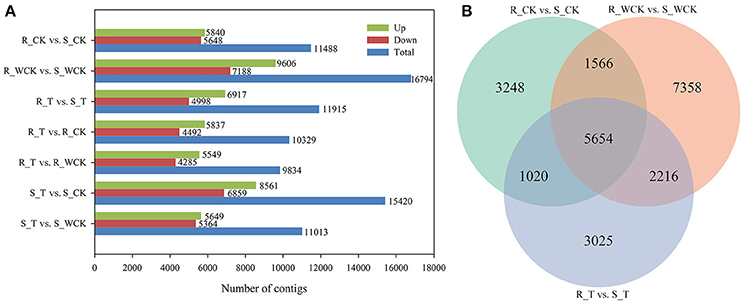
Figure 7. The statistics of the DEGs between the Alopecurus aequalis treatment groups. (A) The number of DEGs between the different groups. (B) Venn diagram showing the number of DEGs between R (AH18) and S (SD01) in the three treatment comparisons.
To further characterize the function of the DEGs, we conducted GO enrichment analyses. In total, there were 11,915 DEGs found between the R_T and S_T samples; these were classified into 57 functional groups, namely 22 groups as BP, 17 groups as CC, and 18 groups as MF (Supplementary Figure S1). Among these DEGs, the GO terms of “cellular process” (1587, 13.32%), “metabolic process” (1200, 12.59%), and “single-organism process” (1202, 10.09%)” in the BP category, “cell part” (1912, 16.05%)” in the CC category, and those of “binding” (1691, 14.19%)” and “catalytic” (1691, 13.00%) in the MF category, were all significantly enriched between the R and S population samples.
We also conducted a detailed KEGG enrichment analysis of the DEGs between the R and S populations for each of the three treatments (Supplementary Figures S2–S4). The DEGs between the mesosulfuron-methyl-treated R and S samples were significantly enriched in the 12 pathways (Table 4). Specifically, 17 up-regulated genes were enriched in the “metabolism of xenobiotics by CytP450” pathway, and 16 up-regulated genes were enriched in the “drug metabolizing-cytochrome CytP450” pathway. Notably, the genes involved in the “metabolism by cytochrome CytP450” changed significantly in the KEGG pathway analysis; this result suggested that the CytP450 genes could play a key role in the metabolic resistance to mesosulfuron-methyl in A. aequalis.
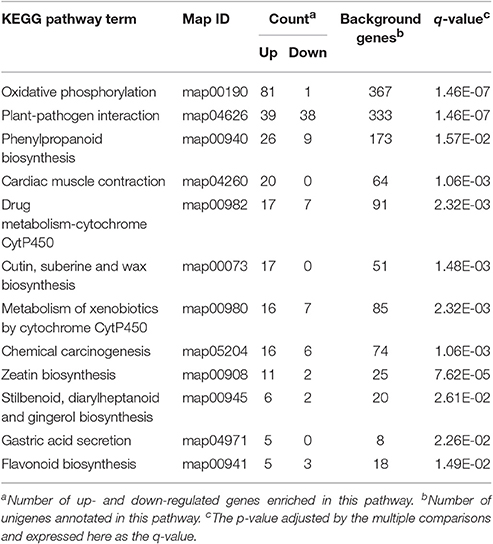
Table 4. The 12 enriched KEGG pathway terms of the DEGs between mesosulfuron-methyl treated R (AH18) and S (SD01) Alopecurus aequalis populations.
Selection of Candidate Metabolic Resistance Contigs
Considering the contigs differentially expressed between the R and S populations, those having predicted annotations related to metabolism and signaling pathways are more likely to be involved in conferring metabolic mesosulfuron-methyl resistance (Gaines et al., 2014). Therefore, we selected those overlapping genes up-regulated in all three comparative groups—i.e., R_T relative to S_T, R_T relative to R_WCK, and R_T relative to R_CK—which had such predicted annotations (Supplementary Table S2). We also selected those up-regulated genes annotated as related to metabolism that had a significantly different expression between R and S in all three treatments (Supplementary Table S3). In particular, the overlapping genes having |log2(fold change)| values in R_T relative to S_T exceeding those of the other two comparable groups (i.e., R_WCK vs. S_WCK and R_CK vs. S_CK) were identified (Supplementary Table S3). In addition, contigs were also chosen that had predicted annotations related to oxidation (e.g., oxidase and peroxidase), hydrolysis (e.g., esterase and hydrolase), and plant stress physiology (e.g., response to plant hormone stimulus) in the above-mentioned comparative groups, along with those contigs with the largest fold-change expression differences but of an unknown function (i.e., no assigned annotation). Based on these criteria, a total of 31 contigs were selected as candidate genes that might confer mesosulfuron-methyl resistance to A. aequalis (Table 5). Of these contigs, eight were annotated to the CytP450 family, five to the GST family, five to GT family, and two to the ABC transporter family, with the remainder (11) annotated to other families.
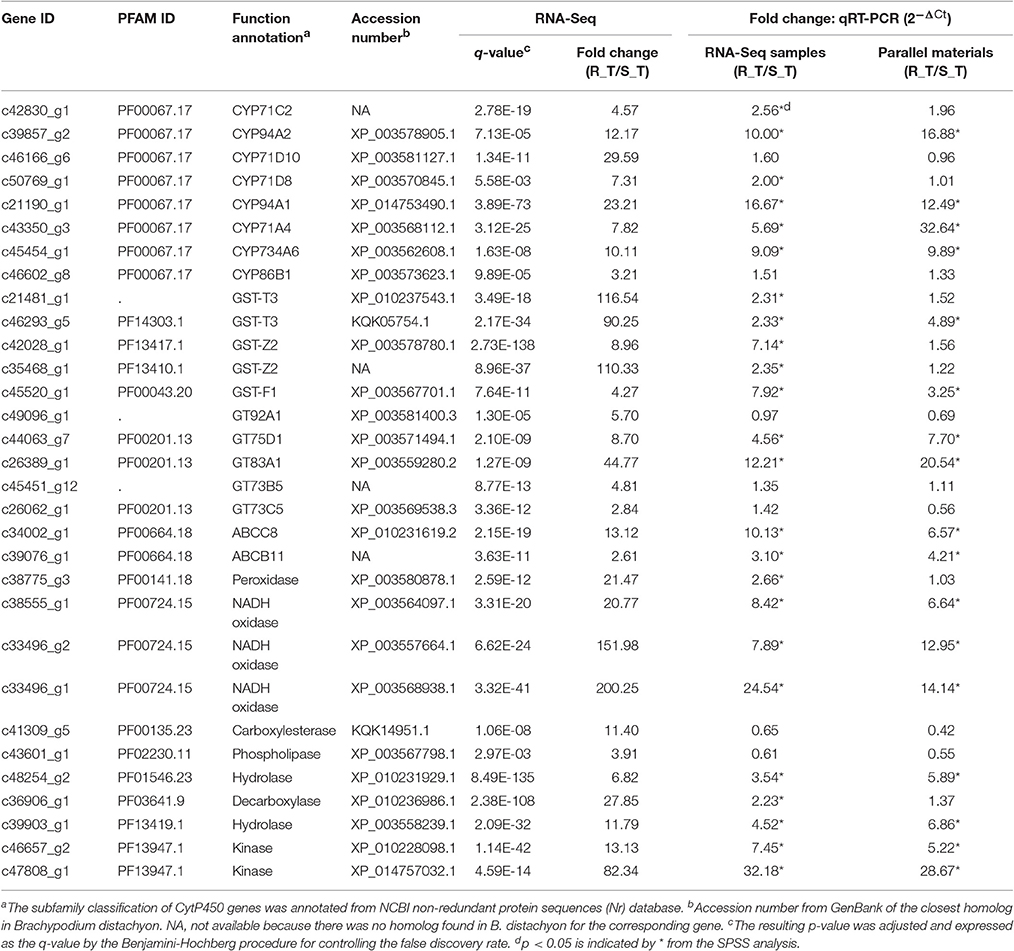
Table 5. Selection and identification of the up-regulated contigs annotated and related to metabolic resistance in Alopecurus aequalis via RNA-Seq and qRT-PCRs (2−ΔCt).
qRT-PCR Validation of the Candidate Metabolic Resistance Contigs
The expression levels of the 31 candidate contigs were first validated by qRT-PCR using the original RNA samples (i.e., R_T and S_T). The relative expression data provided by the qRT-PCR showed that 24 of the 31 candidate contigs exhibited significantly higher expression levels in the R (AH18) than in the S (SD01) population samples (Table 5).
The expression patterns of the 24 candidate contigs were evaluated further using the parallel A. aequalis plant materials. These parallel samples had a genetic background identical to the RNA-Seq samples. Based on the results of the RNA-Seq and the two rounds of the qRT-PCR validations, a total of 17 candidate contigs were expressed significantly higher in the R (AH18) than in the S (SD01) population samples (Table 5, Figure 8, Supplementary Figure S5). These 17 contigs possibly encoded proteins with homology to four CytP450s (CYP94A1, CYP94A2, CYP71A4, and CYP734A6), two GSTs (GST-T3 and GST-F1), two GTs (GT75D1 and GT83A1), two ABC transporters (ABCC8 and ABCB11), three oxidases, two hydrolases, and two kinases. Although some variation among individual plants was observed, the average expression levels of the candidate contigs provided by qRT-PCR were consistent with the RNA-Seq expression data, thus revealing little deviation in the fold change (Figure 8).
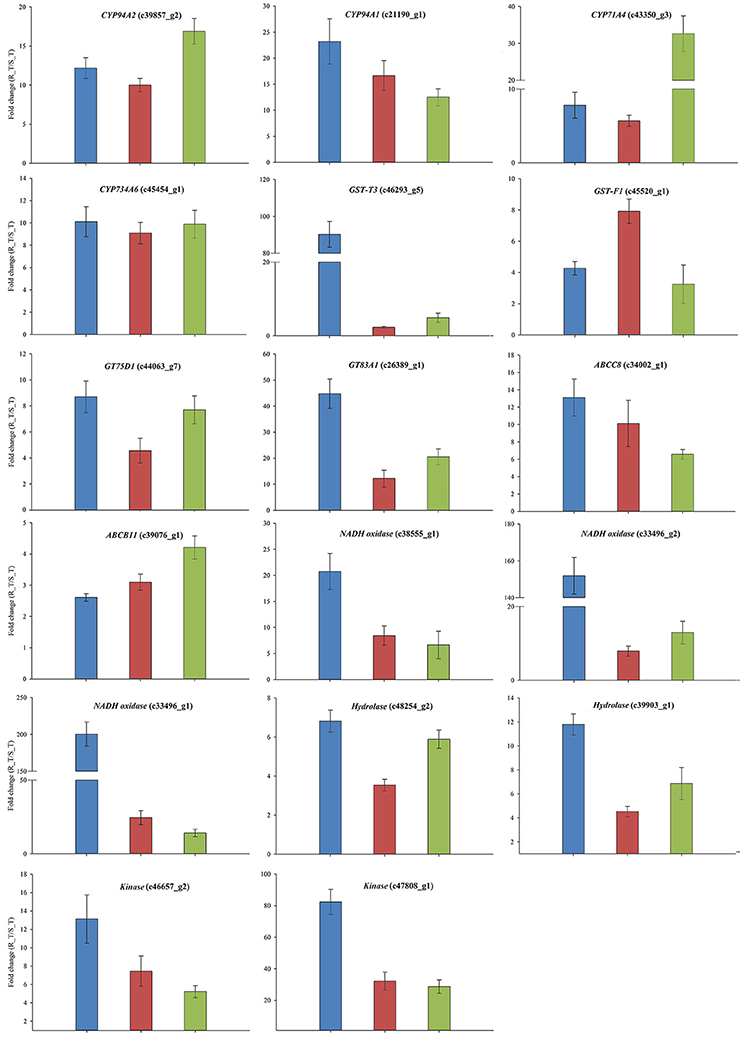
Figure 8. The qRT-PCR validations of the 17 genes that had a consistently up-regulated expression in the R Alopecurus aequalis samples. Blue bars represent the RNA-Seq; red bars represent the qRT-PCR validation that used the RNA-Seq samples; and green bars represent the qRT-PCR validation that used the parallel plant materials. Both UBQ and GAPDH were used as the internal control genes. Means and their SEs from three biological replicates are shown.
Discussion
This study aimed to identify the genes present in A. aequalis in response to mesosulfuron-methyl, so as to establish a transcriptomic resource for subsequent studies. Our previous study confirmed that the R A. aequalis population (AH18) has evolved a high level of resistance to fenoxaprop-P-ethyl and mesosulfuron-methyl. Two amino acid substitutions, Ile2041Asn in the ACCase gene and Pro197Arg in the AHAS gene, were identified in the individual plants of the R population. Furthermore, the NTSR mechanism may take part in the resistance to fenoxaprop-P-ethyl by A. aequalis. (Guo et al., 2016). Here, we demonstrated that the resistance to mesosulfuron-methyl of the R A. aequalis population is also partly due to a NTSR mechanism, because the use of malathion largely reversed this resistance (Table 1, Figures 1, 2). It is well-established that malathion, a cytochrome P450 inhibitor, is able to inhibit the metabolism of the sulfonylurea herbicide, thus reversing a metabolism-based resistance (Christopher et al., 1994; Yu and Powles, 2014a). Similarly, malathion has been used recently to examine metabolism-based resistance to the AHAS-inhibiting herbicide tribenuron-methyl in a resistant D. sophia population (Yang et al., 2016). Together, these results support the hypothesis that NTSR co-exists with TSR in resistant plants, as was clearly demonstrated for herbicide-resistant Lolium rigidum populations (Han et al., 2016).
Additionally, the area from which the R A. aequalis population was collected had received the fenoxaprop-P-ethyl application 7–8 years earlier than that of mesosulfuron-methyl. The ACCase-inhibiting herbicide, fenoxaprop-P-ethyl, probably “pre-selected” for NTSR to the AHAS-inhibiting herbicide mesosulfuron-methyl (Délye et al., 2011). The underlying genes of the R population involved in metabolism-based resistance, as triggered by mesosulfuron-methyl, are likely also playing an important role in the NTSR to fenoxaprop-P-ethyl. Therefore, a RNA-Seq study was warranted for the R A. aequalis population (AH18) to better understand metabolic resistance evolution in this weed species.
RNA-Seq technology is a useful tool for studying NTSR mechanisms, although the transcriptomes of most weed species have not yet been fully sequenced. In recent years, RNA-Seq has been successfully applied to identify the genes involved in NTSR to the AHAS-inhibiting herbicides in two grass and one broadleaf weed species—L. rigidum (Gaines et al., 2014), A. myosuroides (Gardin et al., 2015), and D. sophia (Yang et al., 2016)—which generated 19,623, 180,117, and 84,085 assembled contigs with an average size of 448, 616, and 839 bp, respectively. Nevertheless, only a handful of herbicide-metabolizing and resistance-endowing genes have been identified in these weed species (Yang et al., 2016). Moreover, the NTSR mechanisms are expected to differ both among and within weed species (Délye et al., 2013). Therefore, we performed the RNA-Seq analyses twice: once for the de novo assembly of the transcriptome of A. aequalis, and again for the identification of the candidate contigs in response to the mesosulfuron-methyl in the multiple-herbicide-resistant A. aequalis population. Owing to the development of the Illumina Hiseq 4,000 platform, our reference A. aequalis transcriptome had a similar number of assembled unigenes (95 479), but of longer average size (944 bp), when compared with those reported by projects using other weed species (Peng et al., 2010; Riggins et al., 2010; Yang et al., 2013; An et al., 2014; Leslie and Baucom, 2014; Chen et al., 2016; Duhoux et al., 2017).
A commercial formulation of mesosulfuron-methyl was used in our experiments because any allele(s) that enables an individual plant to withstand herbicide action should be strongly selected by recurrent applications, of both the herbicide molecules and the formulations associated with the herbicides, under realistic field conditions (Délye, 2013). In addition, seeds of the R population were collected from Anhui and those of the S population were collected from Shandong (Guo et al., 2016). Because of the differences in the genetic background of the two populations, and also in the application of herbicides, both the constitutive and herbicide-induced DEGs whose functional annotation involved metabolic resistance between the two biotypes were selected. Subsequently, all the contigs were confirmed by qRT-PCR of the RNA-Seq samples and again by the parallel plant materials. Hence, the genes consistently over-expressed in the R plants may contribute importantly to the NTSR. The 17 genes identified here support the hypothesis that NTSR in A. aequalis is largely driven by the differences in the expression patterns of a set of genes between the R and S plants.
Candidate Genes in the CytP450, GST, and GT Families
In our study, four CytP450 genes (CYP94A1, CYP94A2, CYP71A4, and CYP734A6) were identified as major candidates for metabolic resistance. The biochemical role of CytP450-mediated herbicide metabolism has been well-established in resistant L. rigidum populations, and several distinct CytP450 isoforms were shown to be involved in metabolic resistance to ACCase- and AHAS-inhibiting herbicides (Christopher et al., 1994; Preston et al., 1996; Preston and Powles, 1998; Busi et al., 2011; Duhoux and Délye, 2013; Gaines et al., 2014). Several CytP450 genes, such as CYP71AK2 and CYP72A254, were reported to show a high expression level in a multiple-herbicide-resistant E. phyllopogon population (Iwakami et al., 2014b), while CYP94A1 was implicated in the process of plant defense against chemical injury in the common vetch (Vicia sativa L.) (Benveniste et al., 2005). The CYP734A family typically functions in the brassinosteroid catabolism of plants, and brassinosteroids are known to protect plant crops against toxicity from herbicides (Ohnishi et al., 2006; Xia et al., 2009). Transgenic Arabidopsis (Arabidopsis thaliana) expressing either of the two CytP450 genes, CYP81A12 or CYP81A21, confers resistance to AHAS inhibitors bensulfuron and penoxsulam (Iwakami et al., 2014a). Moreover, many CytP450s conferring herbicide resistance have been identified in several major crop species, such as CYP81A6 in rice (O. sativa) (Pan et al., 2006), CYP71C6v1 in wheat (T. aestivum) (Wen-Sheng et al., 2006), CYP71A10 in soybean (Glycine max [Linn.] Merr.) (Siminszky et al., 1999), and CYP76B1 in Jerusalem artichoke (Helianthus tuberosus L.) (Didierjean et al., 2002). Importantly, it has been demonstrated that the purified recombinant protein expressed by the T. aestivum CYP71C6v1 gene could specifically metabolize three herbicides, namely chlorsulfuron, triasulfuron, and metsulfuron-methyl, via the phenyl ring hydroxylase (Wen-Sheng et al., 2006; Xiang et al., 2006). The CytP450 genes that we identified in the R A. aequalis population may also confer potential resistance to multiple herbicides, but this hypothesis will require empirical testing for its validation.
The GSTs and GTs are two other key families of enzymes involved in herbicide detoxification. The biochemical role of GST and GT in the NTSR to herbicides is now well-established (Brazier-Hicks and Edwards, 2005; Cummins et al., 2011, 2013). Prior studies indicated that GSTs and GTs were associated with NTSR to the ACCase- and AHAS-inhibiting herbicides in L. rigidum (Gaines et al., 2014; Duhoux et al., 2015). In the present study, two GST genes (GST-T3 and GST-F1) and two GT genes (GT83A1 and GT75D1) were identified as associated with the metabolic resistance of A. aequalis. The predicted GST-F1 gene shows a high sequence homology with A. myosuroides (86%, UniProt accession No. Q9ZS17). Another study showed GST-F1 plays a central role in the multiple-herbicide resistance of A. myosuroides, and that the expression of its orthologous GST-F1 in a multiple-resistant L. rigidum population also showed enhancement (Cummins et al., 2013). Both the Tau (U) and Phi (F) classes of GSTs play roles in the herbicide detoxification performed by plants for several compounds (Cummins et al., 2011). Some GST genes conferring herbicide tolerance were also identified in several crop species, such as TaGSTU4 in wheat (T. aestivum) (Thom et al., 2002), GST I, and GST-27 in maize (Zea mays L.) (Karavangeli et al., 2005), and GmGSTU21 and GmhGS in soybean (G. max) (Skipsey et al., 2005). Additionally, the GT75D1 found in the present study shares a 73% sequence identity with the predicted 5-O-glucosyltransferase gene of B. distachyon (GenBank accession No. XP_003571494.1). Coincidentally, an A. myosuroides population, one resistant to multiple classes of herbicides, due in part to the elevated expression of CytP450s and GSTs active in herbicide detoxification, displayed higher O-glucosyltransferase activity than did the herbicide-sensitive populations of A. myosuroides (Brazier et al., 2002). Similarly, GT gene activities, for example GTA triggered by the application of AHAS-inhibiting herbicides, were observed in A. thaliana (Manabe et al., 2007), and two GT genes were shown to glycosylate the primary major bentazone metabolite, 6-hydroxybentazone, in the herbicide-tolerant G. max (Leah et al., 1992). Together, these findings suggest to us that the GST-F1 and GT75D1 genes up-regulated in the R A. aequali population may be associated with its mesosulfuron-methyl resistance. However, the respective roles of GST-T3 and GT83A1 remain unknown, and more investigative work is needed to elucidate their putative roles in herbicide resistance.
Candidate Genes in the ABC Transporter Family
Plant ABC transporters, one of the most diverse gene families, are implicated in the detoxification of xenobiotics, which include herbicides (Rea, 2007). However, in contrast to the CytP450, GST, and GT gene families which confer herbicide resistance through metabolism, the ABC transporters detoxify herbicides by sequestering the herbicides and their metabolites (Yuan et al., 2007). In the present study, the two contigs c34002_g1 and c39076_g1 were annotated to ABCC8 and ABCB11, respectively, and so they may be associated with the mesosulfuron-methyl resistance. Similar to our result, an ABCC1, which could play an important role in the metabolic resistance to tribenuron-methyl, was identified in the resistant D. sophia population (Yang et al., 2016); an ABCB11 was also identified and found up-regulated in a resistant B. syzigachne population having NTSR to fenoxaprop-P-ethyl (Pan et al., 2016). Indeed, there are considerable lines of evidence for the involvement of ABC transporters in protecting plant tissues from toxin-induced damage. Two ABCC transporters, AtABCC1 and AtABCC2, were reported to mediate the tolerance of arsenic and arsenic-based herbicides in A. thaliana (Song et al., 2010). An ABC transporter, AtOPT6, showed an association with herbicide resistance in A. thaliana (Cagnac et al., 2004). Finally, the overexpression of AtPgp1, a multidrug resistance family member, and that of its garden pea homolog psNTP9, was able to confer resistance to multiple herbicides in A. thaliana (Windsor et al., 2003). Therefore, it is likely that ABCC8 and ABCB11 participate in driving metabolic resistance to mesosulfuron-methyl.
Candidate Herbicide-Resistance Genes in Other Gene Families
In this study, we found five further genes possibly associated with mesosulfuron-methyl metabolic resistance in A. aequalis. These genes are annotated in three major enzyme families functioning in the herbicide degradation process: hydrolases, oxidases, and peroxidases. Hydrolases can cleave the herbicide molecule, after which the cleaved molecule is transformed into a more hydrophilic metabolite by the oxidases and peroxidases (Délye, 2013). In addition, the increased expression of oxidases and peroxidases could effectively protect plant cells against the oxidative damage caused by a herbicide's action, which may also contribute to herbicide resistance (Délye, 2013).
Additionally, we selected two up-regulated contigs potentially encoding proteins with homology to kinase involved in response to the stimulus of salicylic acid (a plant hormone). Salicylic acid is a signaling molecule involved with local plant defense reactions at infection sites and the induction of systemic resistance (Durner et al., 1997). Plants can withstand various biotic and abiotic stresses in variable environments by having evolved complicated networks of stress detection, signaling, and response pathways which trigger both general and specific responses, often with an adjustment of the response over time (Délye, 2013). As a powerful abiotic stress, herbicides are known to trigger some of these pathways depending on the specific type of study, the herbicide, and the plant species involved (Das et al., 2010; Unver et al., 2010). NTSR is clearly part of an abiotic stress response, but the extent of this involvement varies among genotypes. Importantly, hormones participate in optimizing plant responses to abiotic stresses (Peleg and Blumwald, 2011), and yet there are few reports of such responses involving NTSR. The up-regulated genes annotated as hormone-related in this study may well be related to the mesosulfuron-methyl-induced stress response pathways. Considering the complexity of most plant defense mechanisms, further rigorous and comprehensive studies are required.
Optimization of Methods
In this study, the RNA-Seq experimental design consisted of three treatments: an untreated control, a water-only control, and a mesosulfuron-methyl treatment. The objectives of using two controls were to measure the effects on transcription due specifically to the mesosulfuron-methyl treatment, and to control for any transcriptional effects from the spraying of plants with water (the solvent for mesosulfuron-methyl). According to our results, more DEGs were evidently observed due to mesosulfuron-methyl treatment relative to the untreated control than due to mesosulfuron-methyl treatment relative to the water-only control (Figure 7A). Hence, the DEGs triggered specially by mesosulfuron-methyl may play a more important role in the NTSR of A. aequalis. An earlier RNA-Seq experiment likewise established two controls—an untreated control and adjuvant-only control—to eliminate the potential effects on transcription due to the adjuvant (Gaines et al., 2014). By contrast, many other published reports pay more attention to the comparison between the untreated control and the herbicide treatment, which neglects the possible effects of the solvent or adjuvant on the RNA-Seq results (e.g., Yang et al., 2013, 2016; An et al., 2014; Chen et al., 2016).
Conclusion
A multiple-resistant A. aequalis population (AH18) possessing TSR and NTSR to mesosulfuron-methyl and fenoxaprop-P-ethyl was identified. The TSR mechanism was clearly demonstrated in our previous work, while the NTSR mechanism was explored in the current study. Taken together, the results indicate this latter mechanism is likely due to CytP450-, GST-, and GT-mediated integrated metabolic resistance, in combination with an ABC transporter-mediated sequestration of the herbicide metabolites. Additional genes, whose functional annotation is related to oxidation, hydrolysis, and even plant stress physiology, may also contribute to the NTSR. All the candidate genes identified in this study were triggered by mesosulfuron-methyl, and so it may also be responsible for the NTSR to fenoxaprop-P-ethyl. The current study is but a first step toward elucidating the mechanism behind NTSR in A. aequalis. Functional characterizations, ideally including transgenic expression and over-expression in a model plant species, B. distachyon, and key-gene knockouts in the R A. aequalis population, are now necessary to define the biochemical role of the candidate genes in herbicide metabolism and resistance in plants.
Data Accessibility
The raw Illumina sequence reads have been deposited in the NCBI Sequence Read Archive (SRA) database with accession number SRP106664 including SRX2792093 for SD01 (S) and SRX2792094 for AH18 (R).
Author Contributions
NZ and JW designed the research. NZ, WL, and SB performed the experimental work and the data analysis. WG, GY, FW, and WTL provided helpful suggestions for the data analysis and during the manuscript preparation. NZ and JW wrote the paper. All authors edited and reviewed the manuscript.
Funding
This research was funded by the Special Fund for Agro-scientific Research in the Public Interest (No. 201303031).
Conflict of Interest Statement
The authors declare that the research was conducted in the absence of any commercial or financial relationships that could be construed as a potential conflict of interest.
Acknowledgments
We thank the editor Michael Deyholos and reviewers for their useful and insightful comments on drafts of the manuscript. We also thank the Charlesworth Group for their professional English language editing services.
Supplementary Material
The Supplementary Material for this article can be found online at: http://journal.frontiersin.org/article/10.3389/fpls.2017.01391/full#supplementary-material
References
An, J., Shen, X., Ma, Q., Yang, C., Liu, S., and Chen, Y. (2014). Transcriptome profiling to discover putative genes associated with paraquat resistance in goosegrass (Eleusine indica L.). PLoS ONE 9:e99940. doi: 10.1371/journal.pone.0099940
Beckie, H. J., and Tardif, F. J. (2012). Herbicide cross resistance in weeds. Crop Prot. 35, 15–28. doi: 10.1016/j.cropro.2011.12.018
Benveniste, I., Bronner, R., Wang, Y., Compagnon, V., Michler, P., Schreiber, L., et al. (2005). CYP94A1, a plant cytochrome P450-catalyzing fatty acid ω-hydroxylase, is selectively induced by chemical stress in Vicia sativa seedlings. Planta 221, 881–890. doi: 10.1007/s00425-005-1503-y
Brazier, M., Cole, D. J., and Edwards, R. (2002). O-Glucosyltransferase activities toward phenolic natural products and xenobiotics in wheat and herbicide-resistant and herbicide-susceptible black-grass (Alopecurus myosuroides). Phytochemistry 59, 149–156. doi: 10.1016/S0031-9422(01)00458-7
Brazier-Hicks, M., and Edwards, R. (2005). Functional importance of the family 1 glucosyltransferase UGT72B1 in the metabolism of xenobiotics in Arabidopsis thaliana. Plant J. 42, 556–566. doi: 10.1111/j.1365-313X.2005.02398.x
Busi, R., Vila-Aiub, M. M., and Powles, S. B. (2011). Genetic control of a cytochrome P450 metabolism-based herbicide resistance mechanism in Lolium rigidum. Heredity 106, 817–824. doi: 10.1038/hdy.2010.124
Cagnac, O., Bourbouloux, A., Chakrabarty, D., Zhang, M. Y., and Delrot, S. (2004). AtOPT6 transports glutathione derivatives and is induced by primisulfuron. Plant Physiol. 135, 1378–1387. doi: 10.1104/pp.104.039859
Chen, J., Huang, H., Wei, S., Huang, Z., Wang, X., and Zhang, C. (2016). Investigating the mechanisms of glyphosate resistance in goosegrass (Elensine indica L.) by RNA-Seq technology. Plant J. 89, 407–415. doi: 10.1111/tpj.13395
Christopher, J. T., Preston, C., and Powles, S. B. (1994). Malathion antagonizes metabolism-based chlorsulfuron resistance in Lolium rigidum. Pestic. Biochem. Physiol. 49, 172–182. doi: 10.1006/pest.1994.1045
Conesa, A., Götz, S., García-Gómez, J. M., Terol, J., Talón, M., and Robles, M. (2005). Blast2GO: a universal tool for annotation, visualization and analysis in functional genomics research. Bioinformatics 21, 3674–3676. doi: 10.1093/bioinformatics/bti610
Cummins, I., Dixon, D. P., Freitag-Pohl, S., Skipsey, M., and Edwards, R. (2011). Multiple roles for plant glutathione transferases in xenobiotic detoxification. Drug Metab. Rev. 43, 266–280. doi: 10.3109/03602532.2011.552910
Cummins, I., Wortley, D. J., Sabbadin, F., He, Z., Coxon, C. R., Straker, H. E., et al. (2013). Key role for a glutathione transferase in multiple-herbicide resistance in grass weeds. Proc. Natl. Acad. Sci. U.S.A. 110, 5812–5817. doi: 10.1073/pnas.1221179110
Das, M., Reichman, J. R., Haberer, G., Welzl, G., Aceituno, F. F., et al. (2010). A composite transcriptional signature differentiates responses towards closely related herbicides in Arabidopsis thaliana and Brassica napus. Plant Mol. Biol. 72, 545–556. doi: 10.1007/s11103-009-9590-y
Délye, C. (2013). Unravelling the genetic bases of non-target-site-based resistance (NTSR) to herbicides: a major challenge for weed science in the forthcoming decade. Pest Manage. Sci. 69, 176–187. doi: 10.1002/ps.3318
Délye, C., Gardin, J. A. C., Boucansaud, K., and Chauvel, B., Petit, C. (2011). Non-target-site-based resistance should be the centre of attention for herbicide resistance research: Alopecurus myosuroides as an illustration. Weed Res. 51, 433–437. doi: 10.1111/j.1365-3180.2011.00864.x
Délye, C., Jasieniuk, M., and Le Corre, V. (2013). Deciphering the evolution of herbicide resistance in weeds. Trends Genet. 29, 649–658. doi: 10.1016/j.tig.2013.06.001
Délye, C., Michel, S., Bérard, A., Chauvel, B., Brunel, D., Guillemin, J. P., et al. (2010). Geographical variation in resistance to acetyl-coenzyme a carboxylase-inhibiting herbicides across the range of the arable weed Alopecurus myosuroides (black-grass). New Phytol. 186, 1005–1017. doi: 10.1111/j.1469-8137.2010.03233.x
Didierjean, L., Gondet, L., Perkins, R., Lau, S.-M. C., Schaller, H., O'keefe, D. P., et al. (2002). Engineering herbicide metabolism in tobacco and Arabidopsis with CYP76B1, a cytochrome P450 enzyme from Jerusalem artichoke. Plant Physiol. 130, 179–189. doi: 10.1104/pp.005801
Draper, J., Mur, L. A., Jenkins, G., Ghosh-Biswas, G. C., Bablak, P., Hasterok, R., et al. (2001). Brachypodium distachyon. A new model system for functional genomics in grasses. Plant Physiol. 127, 1539–1555. doi: 10.1104/pp.010196
Duhoux, A., Carrère, S., Duhoux, A., and Délye, C. (2017). Transcriptional markers enable identification of rye-grass (Lolium sp.) plants with non-target-site-based resistance to herbicides inhibiting acetolactate-synthase. Plant Sci. 257, 22–36. doi: 10.1016/j.plantsci.2017.01.009
Duhoux, A., Carrere, S., Gouzy, J., Bonin, L., and Délye, C. (2015). RNA-Seq analysis of rye-grass transcriptomic response to an herbicide inhibiting acetolactate-synthase identifies transcripts linked to non-target-site-based resistance. Plant Mol. Biol. 87, 473–487. doi: 10.1007/s11103-015-0292-3
Duhoux, A., and Délye, C. (2013). Reference genes to study herbicide stress response in Lolium sp.: up-regulation of P450 genes in plants resistant to acetolactate-synthase inhibitors. PLoS ONE 8:e63576. doi: 10.1371/journal.pone.0063576
Durner, J., Shah, J., and Klessig, D. F. (1997). Salicylic acid and disease resistance in plants. Trends Plant Sci. 2, 266–274. doi: 10.1016/S1360-1385(97)86349-2
Finn, R. D., Clements, J., and Eddy, S. R. (2011). HMMER web server: interactive sequence similarity searching. Nucleic Acids Res. 39, W29–W37. doi: 10.1093/nar/gkr367
Gaines, T. A., Lorentz, L., Figge, A., Herrmann, J., Maiwald, F., Ott, M. C., et al. (2014). RNA-Seq transcriptome analysis to identify genes involved in metabolism-based diclofop resistance in Lolium rigidum. Plant J. 78, 865–876. doi: 10.1111/tpj.12514
Gardin, J. A., Gouzy, J., Carrere, S., and Délye, C. (2015). ALOMYbase, a resource to investigate non-target-site-based resistance to herbicides inhibiting acetolactate-synthase (ALS) in the major grass weed Alopecurus myosuroides (black-grass). BMC Genomics 16:590. doi: 10.1186/s12864-015-1804-x
Grabherr, M. G., Haas, B. J., Yassour, M., Levin, J. Z., Thompson, D. A., Amit, I., et al. (2011). Full-length transcriptome assembly from RNA-Seq data without a reference genome. Nat. Biotechnol. 29, 644–652. doi: 10.1038/nbt.1883
Guo, W., Liu, W., Li, L., Yuan, G., Du, L., and Wang, J. (2015a). Molecular basis for resistance to fenoxaprop in shortawn foxtail (Alopecurus aequalis) from China. Weed Sci. 63, 416–424. doi: 10.1614/WS-D-14-00105.1
Guo, W., Lv, L., Zhang, L., Li, Q., Wu, C., Lu, X., et al. (2016). Herbicides cross resistance of a multiple resistant short-awn foxtail (Alopecurus aequalis Sobol.) population in wheat field. Chilean J. Agric. Res. 76, 163–169. doi: 10.4067/S0718-58392016000200005
Guo, W., Yuan, G., Liu, W., Bi, Y., Du, L., Zhang, C., et al. (2015b). Multiple resistance to ACCase and AHAS-inhibiting herbicides in shortawn foxtail (Alopecurus aequalis Sobol.) from China. Pestic. Biochem. Physiol. 124, 66–72. doi: 10.1016/j.pestbp.2015.04.006
Han, H., Yu, Q., Owen, M. J., Cawthray, G. R., and Powles, S. B. (2016). Widespread occurrence of both metabolic and target-site herbicide resistance mechanisms in Lolium rigidum populations. Pest Manage. Sci. 72, 255–263. doi: 10.1002/ps.3995
Iwakami, S., Endo, M., Saika, H., Okuno, J., Nakamura, N., Yokoyama, M., et al. (2014a). Cytochrome P450 CYP81A12 and CYP81A21 are associated with resistance to two acetolactate synthase inhibitors in Echinochloa phyllopogon. Plant Physiol. 165, 618–629. doi: 10.1104/pp.113.232843
Iwakami, S., Uchino, A., Kataoka, Y., Shibaike, H., Watanabe, H., and Inamura, T. (2014b). Cytochrome P450 genes induced by bispyribac-sodium treatment in a multiple-herbicide-resistant biotype of Echinochloa phyllopogon. Pest Manage. Sci. 70, 549–558. doi: 10.1002/ps.3572
Jasieniuk, M., Brûlé-Babel, A. L., and Morrison, I. N. (1996). The evolution and genetics of herbicide resistance in weeds. Weed Sci. 44, 176–193.
Karavangeli, M., Labrou, N. E., Clonis, Y. D., and Tsaftaris, A. (2005). Development of transgenic tobacco plants overexpressing maize glutathione S-transferase I for chloroacetanilide herbicides phytoremediation. Biomol. Eng. 22, 121–128. doi: 10.1016/j.bioeng.2005.03.001
Krogh, A., Larsson, B., Von Heijne, G., and Sonnhammer, E. L. (2001). Predicting transmembrane protein topology with a hidden Markov model: application to complete genomes. J. Mol. Biol. 305, 567–580. doi: 10.1006/jmbi.2000.4315
Langmead, B., and Salzberg, S. L. (2012). Fast gapped-read alignment with Bowtie 2. Nat. Methods 9, 357–359. doi: 10.1038/nmeth.1923
Leah, J. M., Worrall, T. L., and Cobb, A. H. (1992). Isolation and characterisation of two glucosyltransferases from Glycine max associated with bentazone metabolism. Pest Manage. Sci. 34, 81–87. doi: 10.1002/ps.2780340112
Leslie, T., and Baucom, R. S. (2014). De novo assembly and annotation of the transcriptome of the agricultural weed Ipomoea purpurea uncovers gene expression changes associated with herbicide resistance. G3 4, 2035–2047. doi: 10.1534/g3.114.013508
Li, B., and Dewey, C. N. (2011). RSEM: accurate transcript quantification from RNA-Seq data with or without a reference genome. BMC Bioinformatics 12:323. doi: 10.1186/1471-2105-12-323
Love, M. I., Huber, W., and Anders, S. (2014). Moderated estimation of fold change and dispersion for RNA-seq data with DESeq2. Genome Biol. 15, 1. doi: 10.1186/s13059-014-0550-8
Manabe, Y., Tinker, N., Colville, A., and Miki, B. (2007). CSR1, the sole target of imidazolinone herbicide in Arabidopsis thaliana. Plant Cell Physiol. 48, 1340–1358. doi: 10.1093/pcp/pcm105
Metzker, M. L. (2010). Sequencing technologies—the next generation. Nat. Rev. Genet. 11, 31–46. doi: 10.1038/nrg2626
Mortazavi, A., Williams, B. A., McCue, K., Schaeffer, L., and Wold, B. (2008). Mapping and quantifying mammalian transcriptomes by RNA-Seq. Nat. Methods 5, 621–628. doi: 10.1038/nmeth.1226
Oerke, E. C. (2005). Crop losses to pests. J. Agric. Sci. 144, 31–43. doi: 10.1017/S0021859605005708
Ohnishi, T., Nomura, T., Watanabe, B., Ohta, D., Yokota, T., Miyagawa, H., et al. (2006). Tomato cytochrome P450 CYP734A7 functions in brassinosteroid catabolism. Phytochemistry 67, 1895–1906. doi: 10.1016/j.phytochem.2006.05.042
Pan, G., Zhang, X., Liu, K., Zhang, J., Wu, X., Zhu, J., et al. (2006). Map-based cloning of a novel rice cytochrome P450 gene CYP81A6 that confers resistance to two different classes of herbicides. Plant Mol. Biol. 61, 933–943. doi: 10.1007/s11103-006-0058-z
Pan, L., Gao, H., Xia, W., Zhang, T., and Dong, L. (2016). Establishing a herbicide-metabolizing enzyme library in Beckmannia syzigachne to identify genes associated with metabolic resistance. J. Exp. Bot. 67, 1745–1757. doi: 10.1093/jxb/erv565
Pan, L., Li, J., Zhang, T., Zhang, D., Dong, L., and Neve, P. (2015). Cross-resistance patterns to acetyl coenzyme A carboxylase (ACCase) inhibitors associated with different ACCase mutations in Beckmannia syzigachne. Weed Res. 55, 609–620. doi: 10.1111/wre.12170
Peleg, Z., and Blumwald, E. (2011). Hormone balance and abiotic stress tolerance in crop plants. Curr. Opin. Plant Biol. 14, 290–295. doi: 10.1016/j.pbi.2011.02.001
Peng, Y., Abercrombie, L. L., Yuan, J. S., Riggins, C. W., Sammons, R. D., Tranel, P. J., et al. (2010). Characterization of the horseweed (Conyza canadensis) transcriptome using GS-FLX 454 pyrosequencing and its application for expression analysis of candidate non-target herbicide resistance genes. Pest Manage. Sci. 66, 1053–1062. doi: 10.1002/ps.2004
Petersen, T. N., Brunak, S., von Heijne, G., and Nielsen, H. (2011). SignalP 4.0: discriminating signal peptides from transmembrane regions. Nat. Methods 8, 785–786. doi: 10.1038/nmeth.1701
Petit, C., Bay, G., Pernin, F., and Délye, C. (2010a). Prevalence of cross- or multiple resistance to the acetyl-coenzyme A carboxylase inhibitors fenoxaprop, clodinafop and pinoxaden in black-grass (Alopecurus myosuroides Huds.) in France. Pest Manage. Sci. 66, 168–177. doi: 10.1002/ps.1851
Petit, C., Duhieu, B., Boucansaud, K., and Délye, C. (2010b). Complex genetic control of non-target-site-based resistance to herbicides inhibiting acetyl-coenzyme a carboxylase and acetolactate-synthase in Alopecurus myosuroides Huds. Plant Sci. 178, 501–509. doi: 10.1016/j.plantsci.2010.03.007
Petit, C., Pernin, F., Heydel, J.-M., and Délye, C. (2012). Validation of a set of reference genes to study response to herbicide stress in grasses. BMC Res. Notes 5:18. doi: 10.1186/1756-0500-5-18
Pfaffl, M. W. (2001). A new mathematical model for relative quantification in real-time RT–PCR. Nucleic Acids Res. 29, e45–e45. doi: 10.1093/nar/29.9.e45
Powles, S. B., and Yu, Q. (2010). Evolution in action: plants resistant to herbicides. Annu. Rev. Plant Biol. 61, 317–347. doi: 10.1146/annurev-arplant-042809-112119
Preston, C. (2004). Herbicide resistance in weeds endowed by enhanced detoxification: complications for management. Weed Sci. 52, 448–453. doi: 10.1614/P2002-168B
Preston, C., and Powles, S. B. (1998). Amitrole inhibits diclofop metabolism and synergises diclofop-methyl in a diclofop-methyl-resistant biotype of Lolium rigidum. Pestic. Biochem. Physiol. 62, 179–189. doi: 10.1006/pest.1998.2382
Preston, C., Tardif, F. J., Christopher, J. T., and Powles, S. B. (1996). Multiple resistance to dissimilar herbicide chemistries in a biotype of Lolium rigidum due to enhanced activity of several herbicide degrading enzymes. Pestic. Biochem. Physiol. 54, 123–134. doi: 10.1006/pest.1996.0016
Rea, P. A. (2007). Plant ATP-binding cassette transporters. Annu. Rev. Plant Biol. 58, 347–375. doi: 10.1146/annurev.arplant.57.032905.105406
Riggins, C. W., Peng, Y., Stewart, C. N. Jr., and Tranel, P. J. (2010). Characterization of de novo transcriptome for waterhemp (Amaranthus tuberculatus) using GS-FLX 454 pyrosequencing and its application for studies of herbicide target-site genes. Pest Manage. Sci. 66, 1042–1052. doi: 10.1002/ps.2006
Saha, S., Sparks, A. B., Rago, C., Akmaev, V., Wang, C. J., Vogelstein, B., et al. (2002). Using the transcriptome to annotate the genome. Nat. Biotechnol. 20, 508–512. doi: 10.1038/nbt0502-508
Schmittgen, T. D., and Livak, K. J. (2008). Analyzing real-time PCR data by the comparative CT method. Nat. Protoc. 3, 1101–1108. doi: 10.1038/nprot.2008.73
Seefeldt, S. S., Jensen, J. E., and Fuerst, E. P. (1995). Log-logistic analysis of herbicide dose-response relationships. Weed Technol. 9, 218–227.
Siminszky, B., Corbin, F. T., Ward, E. R., Fleischmann, T. J., and Dewey, R. E. (1999). Expression of a soybean cytochrome P450 monooxygenase cDNA in yeast and tobacco enhances the metabolism of phenylurea herbicides. Proc. Natl. Acad. Sci. U.S.A. 96, 1750–1755. doi: 10.1073/pnas.96.4.1750
Skipsey, M., Cummins, I., Andrews, C. J., Jepson, I., and Edwards, R. (2005). Manipulation of plant tolerance to herbicides through co-ordinated metabolic engineering of a detoxifying glutathione transferase and thiol cosubstrate. Plant Biotechnol. J. 3, 409–420. doi: 10.1111/j.1467-7652.2005.00134.x
Song, W.-Y., Park, J., Mendoza-Cózatl, D. G., Suter-Grotemeyer, M., Shim, D., Hörtensteiner, S., et al. (2010). Arsenic tolerance in Arabidopsis is mediated by two ABCC-type phytochelatin transporters. Proc. Natl. Acad. Sci. U.S.A. 107, 21187–21192. doi: 10.1073/pnas.1013964107
Storey, J. D., and Tibshirani, R. (2003). Statistical significance for genomewide studies. Proc. Natl. Acad. Sci. U.S.A. 100, 9440–9445. doi: 10.1073/pnas.1530509100
Tang, H., Wang, X., and Shen, G. (1990). Study on the yield loss of wheat and barley caused by the infestation of Alopecurus japonicus and A. aequalis. J. Weed Sci. 1, 8–12.
Thom, R., Cummins, I., Dixon, D. P., Edwards, R., Cole, D. J., and Lapthorn, A. J. (2002). Structure of a tau class glutathione S-transferase from wheat active in herbicide detoxification. Biochemistry 41, 7008–7020. doi: 10.1021/bi015964x
Türktaş, M., Kurtoğlu, K. Y., Dorado, G., Zhang, B., Hernandez, P., and Ünver, T. (2015). Sequencing of plant genomes? a review. Turk. J. Agric. For. 39, 361–376. doi: 10.3906/tar-1409-93
Unver, T., Bakar, M., Shearman, R. C., and Budak, H. (2010). Genome-wide profiling and analysis of Festuca arundinacea miRNAs and transcriptomes in response to foliar glyphosate application. Mol. Genet. Genomics 283, 397–413. doi: 10.1007/s00438-010-0526-7
Wen-Sheng, X., Xiang-Jing, W., Tian-Rui, R., and Su-Qin, C. (2006). Purification of recombinant wheat cytochrome P450 monooxygenase expressed in yeast and its properties. Protein Expr. Purif. 45, 54–59. doi: 10.1016/j.pep.2005.07.004
Windsor, B., Roux, S. J., and Lloyd, A. (2003). Multiherbicide tolerance conferred by AtPgp1 and apyrase overexpression in Arabidopsis thaliana. Nat. Biotechnol. 21, 428–433. doi: 10.1038/nbt809
Xia, W., Pan, L., Li, J., Wang, Q., Feng, Y., and Dong, L. (2015). Molecular basis of ALS- and/or ACCase-inhibitor resistance in shortawn foxtail (Alopecurus aequalis Sobol.). Pestic. Biochem. Physiol. 122, 76–80. doi: 10.1016/j.pestbp.2014.12.019
Xia, X. J., Zhang, Y., Wu, J. X., Wang, J. T., Zhou, Y. H., Shi, K., et al. (2009). Brassinosteroids promote metabolism of pesticides in cucumber. J. Agric. Food Chem. 57, 8406–8413. doi: 10.1021/jf901915a
Xiang, W., Wang, X., and Ren, T. (2006). Expression of a wheat cytochrome P450 monooxygenase cDNA in yeast catalyzes the metabolism of sulfonylurea herbicides. Pestic. Biochem. Physiol. 85, 1–6. doi: 10.1016/j.pestbp.2005.09.001
Yang, Q., Deng, W., Li, X., Yu, Q., Bai, L., and Zheng, M. (2016). Target-site and non-target-site based resistance to the herbicide tribenuron-methyl in flixweed (Descurainia sophia L.). BMC Genomics 17:551. doi: 10.1186/s12864-016-2915-8
Yang, X., Yu, X.-Y., and Li, Y.-F. (2013). De novo assembly and characterization of the barnyardgrass (Echinochloa crus-galli) transcriptome using next-generation pyrosequencing. PLoS ONE 8:e69168. doi: 10.1371/journal.pone.0069168
Ye, J., Fang, L., Zheng, H., Zhang, Y., Chen, J., Zhang, Z., et al. (2006). WEGO: a web tool for plotting GO annotations. Nucleic Acids Res. 34, W293–W297. doi: 10.1093/nar/gkl031
Young, M. D., Wakefield, M. J., Smyth, G. K., and Oshlack, A. (2010). Gene ontology analysis for RNA-seq: accounting for selection bias. Genome Biol. 11:R14. doi: 10.1186/gb-2010-11-2-r14
Yu, Q., and Powles, S. (2014a). Metabolism-based herbicide resistance and cross-resistance in crop weeds: a threat to herbicide sustainability and global crop production. Plant Physiol. 166, 1106–1118. doi: 10.1104/pp.114.242750
Yu, Q., and Powles, S. B. (2014b). Resistance to AHAS inhibitor herbicides: current understanding. Pest Manage. Sci. 70, 1340–1350. doi: 10.1002/ps.3710
Keywords: abiotic stress, acetohydroxyacid synthase, herbicide metabolism, mesosulfuron-methyl, multiple-herbicide resistance, RNA-Seq, shortawn foxtail, weeds
Citation: Zhao N, Li W, Bai S, Guo W, Yuan G, Wang F, Liu W and Wang J (2017) Transcriptome Profiling to Identify Genes Involved in Mesosulfuron-Methyl Resistance in Alopecurus aequalis. Front. Plant Sci. 8:1391. doi: 10.3389/fpls.2017.01391
Received: 06 June 2017; Accepted: 26 July 2017;
Published: 09 August 2017.
Edited by:
Michael Deyholos, University of British Columbia, CanadaReviewed by:
Turgay Unver, Izmir International Biomedicine and Genome Center (iBG-izmir), TurkeyDouglas S. Domingues, Sao Paulo State University (UNESP), Brazil
Copyright © 2017 Zhao, Li, Bai, Guo, Yuan, Wang, Liu and Wang. This is an open-access article distributed under the terms of the Creative Commons Attribution License (CC BY). The use, distribution or reproduction in other forums is permitted, provided the original author(s) or licensor are credited and that the original publication in this journal is cited, in accordance with accepted academic practice. No use, distribution or reproduction is permitted which does not comply with these terms.
*Correspondence: Jinxin Wang, d2FuZ2p4QHNkYXUuZWR1LmNu