- 1National Key Laboratory of Crop Genetics and Germplasm Enhancement, National Center for Soybean Improvement, Nanjing Agricultural University, Nanjing, China
- 2Key Laboratory of Plant Resources Conservation and Sustainable Utilization, South China Botanical Garden, Chinese Academy of Sciences, Guangzhou, China
Soybean mosaic virus (SMV) is a member of Potyvirus genus that causes severe yield loss and destroys seed quality in soybean [Glycine max (L.) Merr.]. It is important to explore new resistance sources and discover new resistance loci to SMV, which will provide insights to improve breeding strategies for SMV resistance. Here, a genome-wide association study was conducted to accelerate molecular breeding for the improvement of resistance to SMV in soybean. A population of 165 soybean mutants derived from two soybean parents was used in this study. There were 104 SNPs identified significantly associated with resistance to SC7, some of which were located within previous reported quantitative trait loci. Three putative genes on chromosome 1, 9, and 12 were homologous to WRKY72, eEF1Bβ, and RLP9, which were involved in defense response to insect and disease in Arabidopsis. Moreover, the expression levels of these three genes changed in resistance and susceptible soybean accessions after SMV infection. These three putative genes may involve in the resistance to SC7 and be worthy to further research. Collectively, markers significantly associated with resistance to SC7 will be helpful in molecular marker-assisted selection for breeding resistant soybean accessions to SMV, and the candidate genes identified would advance the functional study of resistance to SMV in soybean.
Introduction
Soybean [Glycine max (L.) Merr.] provides abundant protein for humans and is also one of the world important oil crops. Soybean mosaic virus (SMV) is a member of Potyvirus genus and causes severe yield loss and destroy seed quality (Adams et al., 2005). In the United States, according to disease reactions by resistant cultivars (Buffalo, Davis, Kwanggyo, Marshall, Ogden, and York) and two susceptible cultivars (Clark and Rampage), 98 isolates SMV strains had been classified into seven strains and named G1 to G7 (Cho and Goodman, 1979, 1982). Resistance loci, Rsv1, Rsv3, and Rsv4 had been mapped to chromosomes 13, 14, and 2, respectively (Hayes et al., 2000, 2004; Gore et al., 2002; Wang et al., 2010). Rsv1 in cultivar PI 96983 was resistant to G1–G6 but susceptible to G7 (Cho and Goodman, 1982); Rsv3 was resistant to strains G5–G7 and Rsv4 to G1–G7 (Hayes et al., 2000; Jeong et al., 2002). Based on SMV isolates reaction to a serious of soybean differentials, SMV had been classified into 21 strains in China and named SC1 to SC21 (Wang et al., 2003; Guo et al., 2005; Li et al., 2010). SC7 is a virulent strain that prevalent in Huang-Huai-Hai valleys in China (Wang et al., 2003). Yang et al. (2013) fine-mapped two dominant genes resistant to SC3, SC6, SC7, and SC17 near Rsv1 locus. Wang et al. (2011) fine-mapped resistance gene to SMV strain SC8 in Kefeng No.1 which was in the neighbor of Rsv4. Yan et al. (2015) fine-mapped the resistance gene Rsc7 in Kefeng No.1 to a region of approximately 158 kb on chromosome 2 that is between SSR marker BARCSOYSSR_02_0621 and BARCSOYSSR_02_0632. Due to different hosts and cultivars use in identifying SMV strains, the relationship between SC1-SC21 in China and G1–G7 in United States is unknown.
Nucleotide binding sites (NBS) and leucine rich repeat (LRR) domain were contained in many plant disease resistance genes. There were 19 genes contain NBS-LRR domain in Rsv1 locus and at least 10 different Rsv1 alleles have been found in various landraces, such as Rsv1 in PI 96983, Rsv1-d in FT-10, Rsv1-h in Suweon 97, Rsv1-k in Kwanggyo, Rsv1-m in Marshall, Rsv1-n in PI 507389, Rsv1-r in Raiden, Rsv1-s in LR1, Rsv1-t in Ogden, and Rsv1-y in York (Buss et al., 1989; Chen et al., 1991, 2001, 2002; Ma et al., 1995, 2003; Silva et al., 2004; Tucker et al., 2009; Shao et al., 2014). Many resistance gene loci were mapped by use of cross between narrow genetic resources based on resistance and susceptible accessions. Though this position method was effective, only limited gene loci have been mapped. Therefore, new method is needed to explore new resistant genes in soybean. Compared with linkage analysis, much more alleles and broader heritable diversities could be identified in genome-wide association analyses (GWAS) (Mackay, 2001).
Genome-wide association study is a powerful technique that enables researchers to exploit natural variations and locate candidate genes in the genome (Korte and Farlow, 2013). In recent years, with the development of the technology of sequencing, a large number of SNP markers were developed. Combining high-quality of SNP markers and phenotypic resources, GWAS has been successfully applied in many crops. In rice, a substantial number of loci were identified performed by GWAS for 14 agronomic traits that potentially important for rice production and improvement (Huang et al., 2010). In maize, Wang X. et al. (2016) used a GWAS of maize drought tolerance at the seedling stage and identified 83 genetic variants, which were resolved to 42 candidate genes. According to the SNP signal, ZmVPP1 was identified contributing to this trait (Wang X. et al., 2016). In soybean, Zhang et al. (2014) combined genome-wide association analysis and linkage analysis identified GmACP1 gene that related to P efficiency. Nineteen SNPs and five haplotypes for soybean yield components based on 1536 SNPs were identified by GWAS (Hao et al., 2012). Moreover, GWAS can provide a comparatively higher resolution in terms of mapping the genomic position of interested genes or QTLs because it can be applied to germplasm collections or naturally occurring populations (Shirasawa et al., 2013).
Resistance to SMV can be qualitative or quantitative. Qualitative resistance is controlled by a pair of single dominant genes and confers complete resistance to some SMV strains but susceptibility to others. Quantitative resistance is controlled by multiple genes in soybean (Zhi et al., 2005), is more broad-spectrum and durable than qualitative resistance, but it confers just partial resistance to SMV.
In our study, a genome-wide association analysis was performed to a diverse panel of 165 soybean mutants genotyped with the 355 K SoySNP array and phenotyped with resistance to SMV strain of SC7. The objective of this study was to identify candidate genes significantly associated with resistance in development to SC7. These results will be helpful for cloning of SC7 resistance genes and applying in molecule marker-assisting breeding.
Materials and Methods
Plant Materials and SMV Strains
Soybean mutant population was derived from two parental accessions Nannong 86-4 and Nannong 94-16 through compound chemical (ethyl methanesulfonate, EMS) and physical(60Coγ) mutagenesis (Han et al., 2007, 2008). After years of investigating of phenotypic traits, we finally obtained 165 soybean mutants, among which 93 mutants were from Nannong 86-4 and 72 mutants were from Nannong 94-16. These mutants have extensive variations in leaf, stem, flower, seed shape, protein, oil contents, etc. (Han et al., 2007, 2008). SMV strain SC7 was conserved on highly SMV susceptible cultivar Nannong 1138-2 and the viruliferous leaves were frozen in -80°C refrigerator. All the plant materials and SC7 strain were provided by the National Center for Soybean Improvement, Nanjing Agricultural University, Nanjing, China.
Resistance Evaluation
The seedlings of 165 soybean accessions for association analysis were planted in round plastic pots (diameter × depth: 31 cm × 24 cm) filled with sand in an aphid-free greenhouse. About 45 seeds per each accession were planted in a pot. The planting dates were in May 2015, September 2015, May 2016, and September 2016 at the Jiangpu Experimental Station of Nanjing Agricultural University (32°12′N and 118°37′48″E) and these four experiments were denominated as environment 1, environment 2, environment 3, and environment 4. All accessions were planted with three biological replications and a randomized complete-block design was used for all field trials. After germination, the weak seedlings were pulled out. About 40 soybean plants per each genotype were inoculated with SMV strains of SC7 in every replication.
By using mortars and pestles, the fresh leaves of Nannong 1138-2 infected with SC7 were independently grinded in 0.01 mol/L sodium phosphate buffer (pH 7.2–7.4). Seedlings were manually inoculated with the inoculum by rubbing primary leaves at the V1 stage and inoculated again a week later. Disease symptoms were observed from day 7 to 1 month after inoculation. Resistant accessions were symptomless reaction to SMV infection and susceptible accessions were showed systematic mosaic leaves. The rate of susceptible plants to the total inoculated plants of each accession was defined as the disease rate (DR). The standard was according to Pu et al. (1983), DR of resistant accession was less than 10% and susceptible accession was greater than 10%.
Phenotypic Data Analysis
Statistical analysis of the phenotypic data including descriptive statistics, analysis of variance (ANOVA), and broad heritability were using R software (R Development Core Team, 2015). The best linear unbiased prediction (BLUP) model in R (lme4 package) was used to estimate variation of year, line, and location components. The formula of linear unbiased prediction model was according to Merk et al. (2012)
Yik indicates trait investigated, μ is total mean, Gi is genotypic effect of ith, Yk is effect of kth year, GYik suggested genotype × year interaction, 𝜀ik is residual error. The value of BLUP was produced from BLUP model with random effect and the BLUPs consisted of a new phenotype data was used for GWAS.
Broad-sense heritability (h2) of DR was calculated by using the method according to Kim et al. (2014) with the formula as below:
in the formula, is genotype variance,
is interaction variance of genotype and year,
is variance of error components; n represent years; and r represent replications.
SNP Genotyping and Genome-Wide Association Analysis
One hundred and sixty-five soybean mutants were genotyped by the 355 K SoySNP array (Wang J. et al., 2016). There were totally 282,469 SNPs were identified and the minor allele frequencies (MAFs) of these SNPs were evaluated by PLINK 1.07. After SNPs with MAFs < 0.05 were filtered out, 61,543 were left and used in genome-wide association analysis.
Difference models, including General Linear Model (GLM), GLM with Q (population structure), GLM with K (kinship), Mix Linear Model (MLM) with Q + K were selected and compared for the best fit model. The Bonferroni threshold as P ≤ (1/61543) or –Log10P ≥ 4.79 was used to define significant marker-trait associations.
Population Structure Analysis
STRUCTURE 2.3.4 based on Bayesian model was adopted to analysis the population structure and relatedness in soybean. The length of burn-in period was set as 10,000 and number of Markov chain Monte Carlo (MCMC) replications after burn-in were set as 100,000. Hypothetic number of subpopulations (K) were set from 1 to 10 with three independent replications of operation. To evaluate the suitable K in this population, log likelihood of the LnP(D) and an ad hoc statistic Δk based on the second-order rate of change of LnP(D) were analyzed for successive k (Evanno et al., 2005).
The 61,543 SNPs with MAFs < 0.05 were used to construct a neighbor-joining (NJ) phylogenetic tree with 1,000 bootstrap steps in Tassel 5.2.3. These SNPs were also used in PCA (principal component analysis) by PLINK 1.07 and population stratification was marked by different colors and shapes in a multidimensional scaling plot.
Putative Genes Annotation and the Expression Analysis
In the GWAS results, we searched all the genes in the linkage disequilibrium (LD) decay distance of significantly associated SNPs and soybean genome annotation was referred to Glyma.Wm82.a1.v1.11. Based on the soybean genomic annotations and bioinformatics, we predicted some putative genes related to resistance to SC7 within the LD decay distance of associated SNPs. Meanwhile, the amino acid sequences of the candidate genes in soybean response to pathogens stress were used to perform a BLASTP analysis in Arabidopsis.
To elucidate the expression of putative resistance genes, quantitative real-time PCR (qRT-PCR) was used to analysis their expression in the resistance and susceptible accessions, respectively. According to the average DR of four environments, we selected the extreme resistance and susceptible accessions, namely NJAU_M001 and NJAU_M082, respectively. The soybean seedlings were grown in an aphid-free greenhouse, and three replicated biological samples of leaves were collected in liquid nitrogen at 0, 2, 3, and 4 days after SC7 inoculating or mock inoculating. Total RNA of processed leaves were isolated with kit (TaKaRa, Japan). First strand cDNA synthesis kit (TaKaRa, Japan) was used to synthesis cDNA. With soybean tubulin gene (GenBank Number: AY907703) as an internal control, qRT-PCR was performed using ABI 7500 system (Applied Biosystems, United States) with following conditions: denaturing at 95°C for 5 min; 40 cycles at 95°C for 15 s, 60°C for 60 s. The fold changes of expression levels for each gene was calculated as ΔΔCT value. All the experiments were performed in three biological and three technical replicates.
Results
Phenotypic Variation of Disease Rate in Soybean
Descriptive statistics, ANOVA, and broad heritability of DR in four environments were used to analyze the phenotypic data (Table 1). The average DRs in 165 soybean mutants were 0.68–0.70, which was visually showed on the box plots (Figure 1). As showed in Figure 2, DR of this population was stabled and without much difference among four environments. Coefficient of variation was ranged from 20.0 to 29.0%, indicating significant variation of DR among 165 soybean mutants. Moreover, the ANOVA suggested that the genotype effect and genotype × environmental interaction effects significantly influence DR (P ≤ 0.01). Broad-sense heritability for the DR was 60.6% in this population, which was a little lower than 86.4% previous reported by Yan et al. (2015). This result showed that the trait of DR can be stably inherited in soybean and some resistance accessions can be used in breeding.

TABLE 1. Descriptive statistics, analysis of variance (ANOVA) and broad heritability of disease rate in four environments.
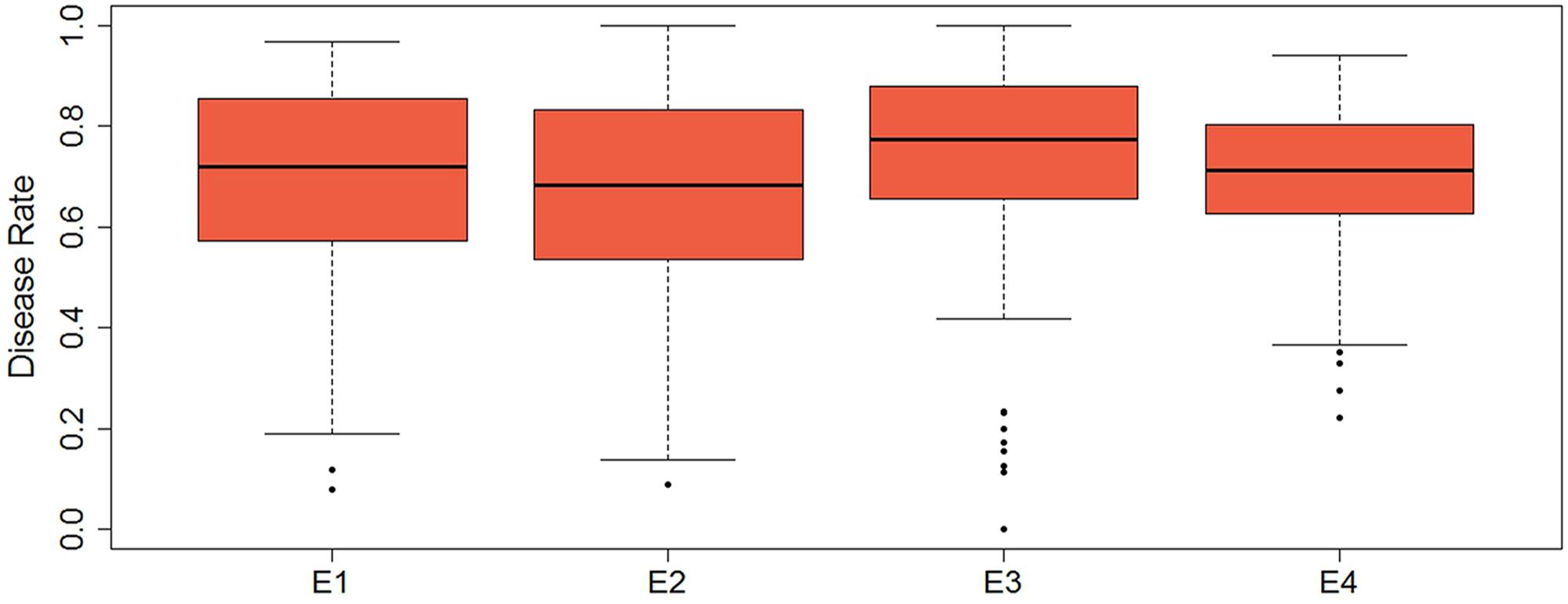
FIGURE 1. Box plot of the distribution of disease rate based on four environments data. The bold line indicates the median value, the red shaded area represents the lower and upper quartiles, and the dotted line range from edge line to upper edge line. Black dots were abnormal value.
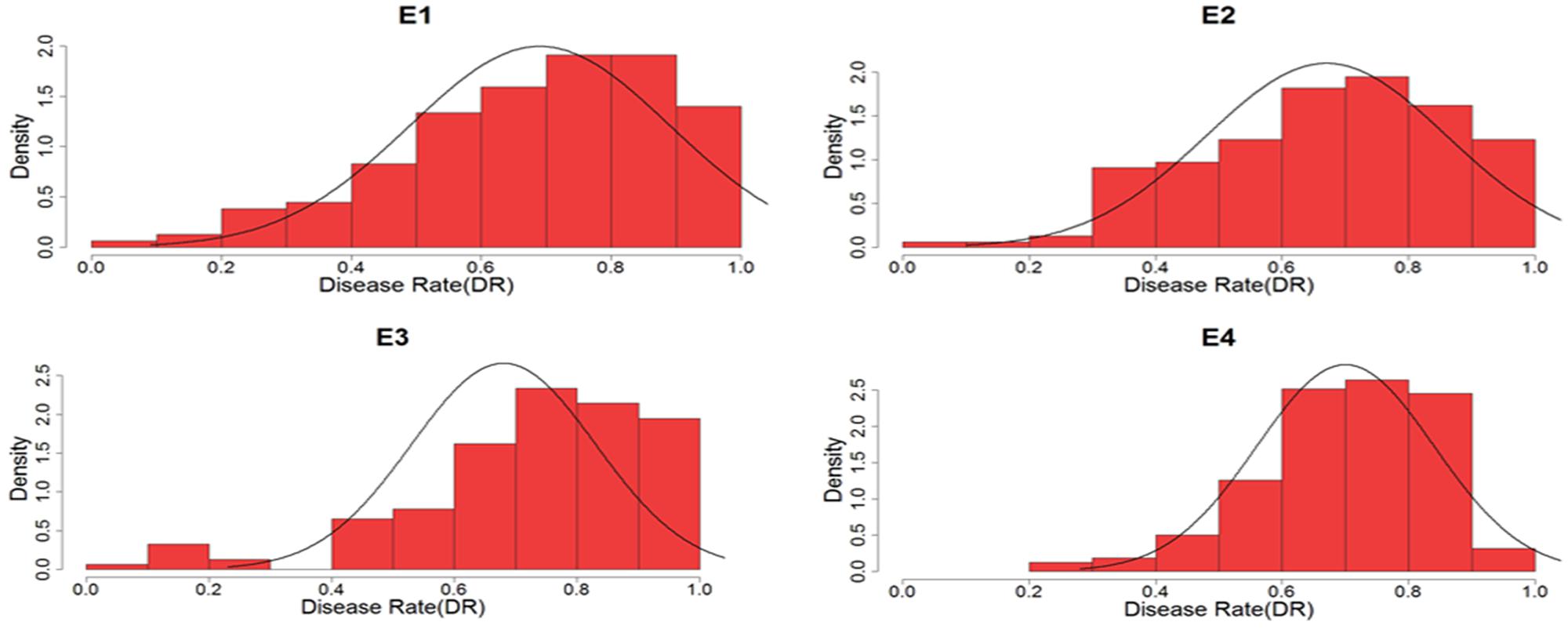
FIGURE 2. Density distribution of disease rate to soybean mosaic virus (SMV) strain of SC7 in four environments. E, environment.
Population Structure Analysis, Linkage Disequilibrium, and Minor Allele Frequencies of Soybean Mutants
To evaluate the population structure and relatedness among 165 soybean mutants, we performed STRUCTURE, PCA, and constructed a NJ tree (Figure 3). In STRUCTURE results, LnP(D) was minimum at K = 1 and increased close to the maximum with the increased of K (Figure 3A). The ad hoc quantity (Δk) showed a much higher possibility at K = 2 than at K = 3–10 among three replications in the program, suggested that the population can be clustered into two major subpopulations (Figures 3B,C): Subpopulation I and Subpopulation II. This result was further supported by the NJ tree and PCA (Figures 3D,E). Subpopulation I contains 93 accessions including the parent Nannong 86-4. And Subpopulation II contains 72 accessions including the parent Nannong 94-16. These results confirmed that accessions from the same ancient species have a close relationship with each other. Additionally, the Q-matrix at K = 2 calculated by STRUCTURE was used for subsequent genome-wide association analysis.
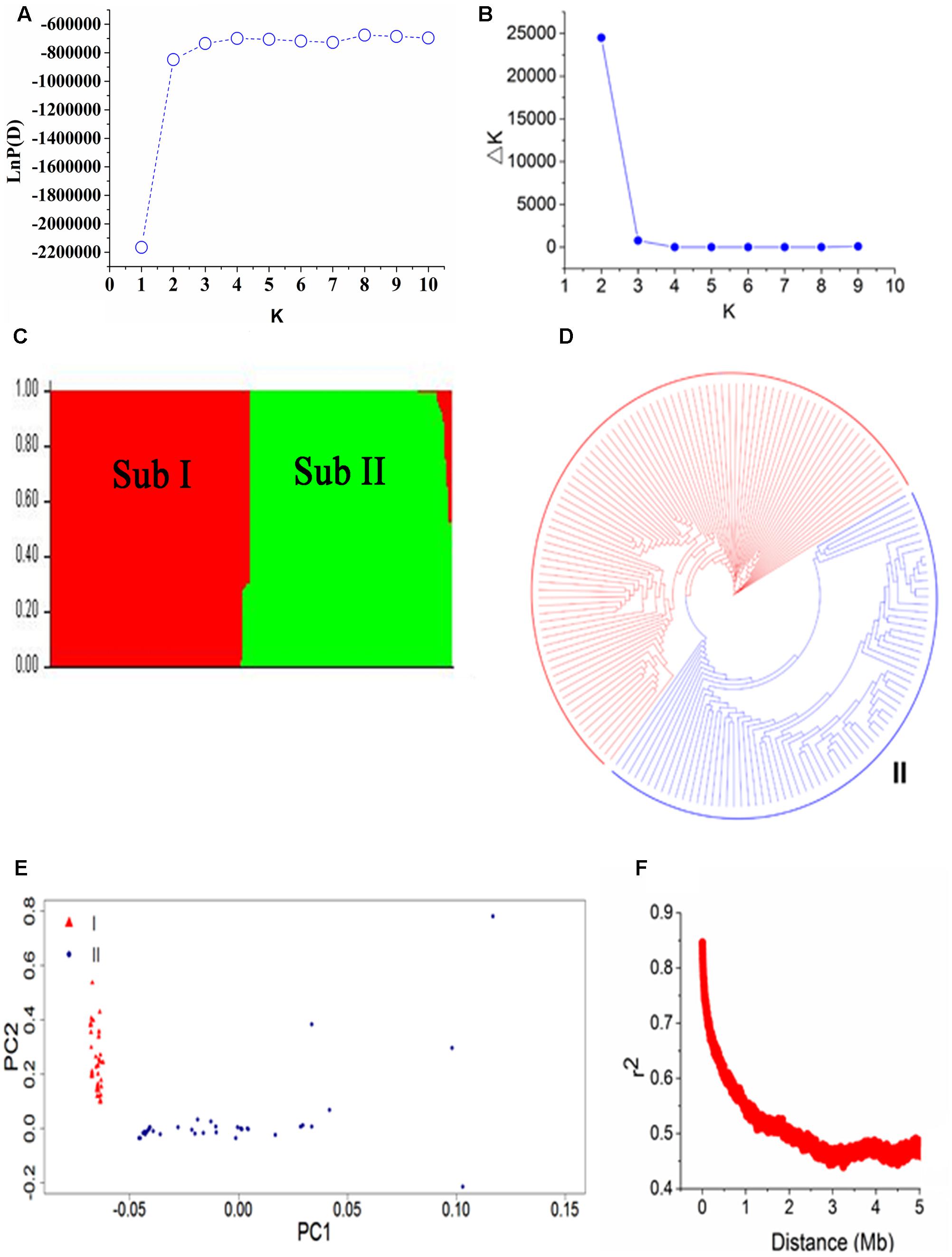
FIGURE 3. Population structure and linkage disequilibrium (LD) decay of 165 soybean accessions. (A,B) LnP(D) and Δk based three repeats of STRUCTURE analysis. (C) The population structure estimated by STRUCTURE, there are two colored segments and each colored segment represents the percentage of the individual in the population. Sub means subpopulation. (D) A neighbor-joining tree of the 165 accessions that can be divided into two subpopulations. (E) Principal component analysis (PCA) of 165 soybean accessions, triangle in red represent Subpopulation I and dot with solid in blue is Subpopulation II. (F) LD decay based pair-wise r2 values in 5000-kb.
Linkage disequilibrium decay distance is an index for the suitable number of markers used in GWAS. A higher LD value indicates the need for a smaller number of markers and a lower LD value means the need for more markers. The LD decay plot based on pair-wise r2 values in 5000-kb was showed in Figure 3F. We found an obvious LD decay in this population. The average r2 values in the population were 0.506 in this study. The physical distance corresponding to half maximum of r2 values was 1 Mb.
Because this soybean mutant population was mutagenic from two parent line (Nannong 86-4 and Nannong 94-16) and the probability of many accessions mutate in same point were very low. Approximately four fifths SNPs with MAFs less than 0.05 have been filtered out, and only 61,543 SNPs were left and used in this study. The distribution of the minor allelic frequencies of 61,543 SNPs were observed in Figure 4 with continued distribution from 0.05 to 0.50. The average of MAF value of the 61,543 SNPs was 0.32 and 69.10% of the SNPs (42,528/61,543) had a MAF value between 0.30 to 0.45.
Genome-Wide Association Analysis Reveals SNPs Significantly Associated with DR
To reveal SNPs significantly associated with DR, the genome-wide association analysis were performed. Difference models, including GLM, GLM with Q, GLM with K, MLM with Q + K were selected and compared for the best fit model. Results showed that MLM with Q + K excessive corrected observed P-value which lead to observed P-value less than expected P-value and no significantly associated SNP was identified (Figure 5). QQ plot of GWAS in GLM was same as GLM + K model which showed K matrix did not affect the result. Moreover, QQ plot of GLM model was much closer to diagonal line, suggesting that this model was more suitable than GLM + Q and MLM with Q + K models. Therefore, GLM model was selected in Tassel 5.2.3 (Bradbury et al., 2007) software in this study.
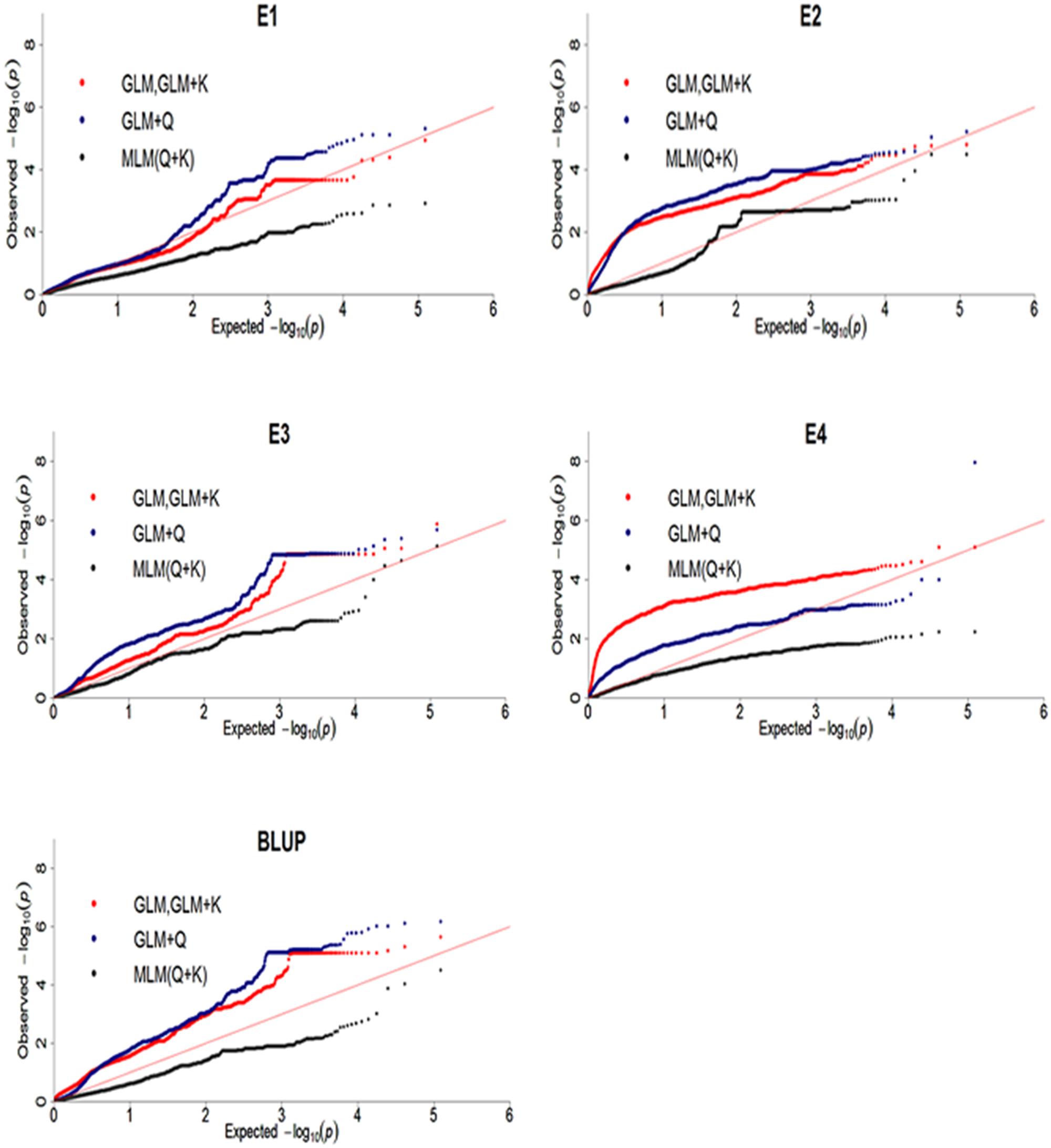
FIGURE 5. Quantile-quantile plots of three genome-wide association study (GWAS) models for disease rate in four environments and BLUP. E, environment; BLUP, best linear unbiased prediction.
There were totally 104 SNPs significantly associated with DR identified in four environments and BLUP (Table 2). Among these significant SNPs, 52 SNPs were repetitively detected in both E3 and BLUP on chromosome 12 (Figure 6). The rest SNPs were only detected in a single environment, which may be due to the influences of environments. On chromosome 9, two regions were found to be significantly associated with DR. Among these two regions, one region extending 1.6 Mb which contains 19 significant SNPs was overlapped with one QTL SCN 39-5 (soybean cyst nematode) (Wu et al., 2009). The other region of chromosome 9, approximately 3.7 Mb long with 27 significant SNPs contains one QTL Sclero 8-3 that resists to Sclerotinia Stem Rot (Vuong et al., 2008). Similar to chromosome 9, on chromosome 12, there were also two regions identified with strong signals associated with DR, which include 20 SNPs and 30 SNPs distributed in 111 and 170 kb physical area, respectively. Several QTLs reported to response to biotic stress, such as Phytoph 9-1 (Wang et al., 2010), Corn earworm 2-2 (Rector et al., 1999), Pod borer 1-1 (Zhao et al., 2008), and SCN 10-5 (Qiu et al., 1999) were mapped to this region. Additionally, Several SNPs distributed on other chromosomes, such as chromosome 1, 3, 5, 10, 13, and 15. In the vicinity of these identified SNPs, there were some QTLs reported to response to biotic stress. For example, SCN 19-1, SCN 19-2, SCN 19-4, SCN 33-7, SCN 44-5, SCN 18-1, and SCN 37-1 have been reported to resistant to soybean cyst nematode (Yue et al., 2001; Guo et al., 2006; Vuong et al., 2010; Jiao et al., 2015); SDS 13-1 was associated with resistant to soybean fungi disease sudden death syndrome (SDS) (Abdelmajid et al., 2012); Japanese beetle resistance 1-4 was associated with herbivory pest Japanese beetles resistance (Yesudas et al., 2010); Phytoph 5-3 was related to Phytophthora sojae infection (Wu et al., 2011); Rag 3-2 associated with aphid resistance(Zhang et al., 2009); Asian Soybean Rust 2-4 resisted to fungal pathogen Phakopsora pachyrhizi (Harris et al., 2015); Peanut root-knot nematode 2-2 was associated with resistance to peanut root-knot nematode in soybean (Tamulonis et al., 1997).
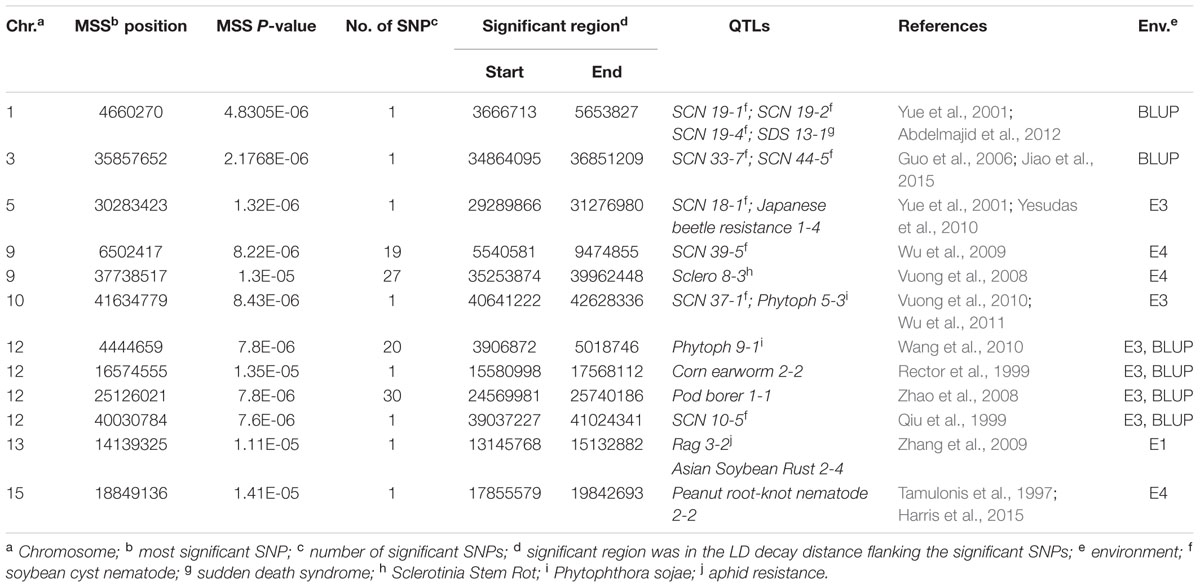
TABLE 2. SNPs loci associated with disease rate (DR) identified via a genome-wide association study (GWAS) in 165 soybean accessions.
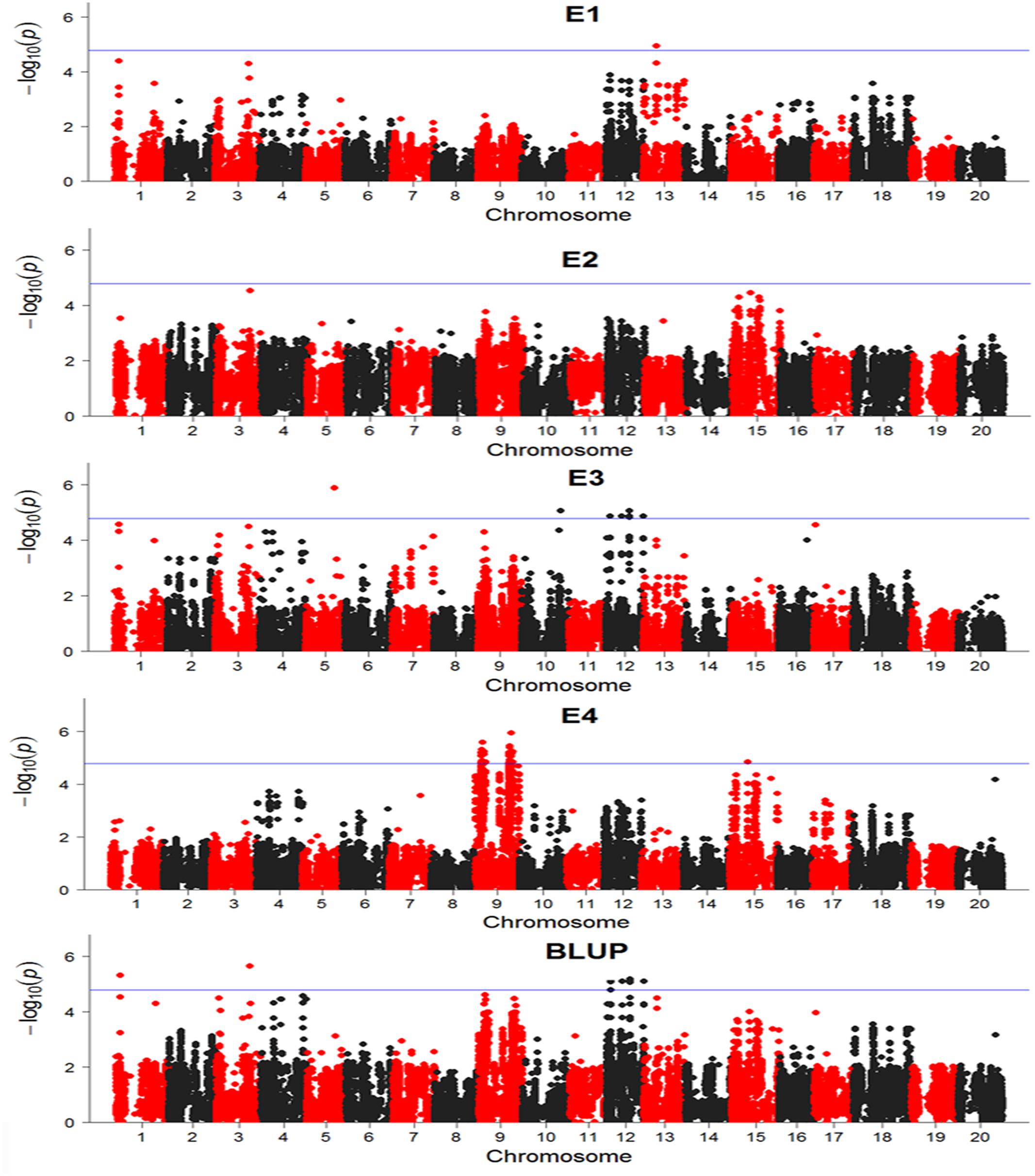
FIGURE 6. Manhattan plots base on General Linear Model (GLM) model in GWAS results. The horizontal line indicates a significant association signals (-log10P ≥ 4.79). E, environment; BLUP, best linear unbiased prediction.
These results showed that SNPs significantly associated with DR were also mapped to the disease and pest resistance loci reported before. It suggested that these loci might be common in the resistance to biotic stress and that these loci were valuable for marker-assisting selection in breeding.
Identification of Putative Genes and the Expression Analysis
To annotate the SNPs significantly associated with resistance to SC7, we searched the 1 Mb (LD decay distance) flanked regions of these SNPs to find putative genes. Then we utilized the soybean genome annotation information and performed BLASTP against Arabidopsis genome to annotate the putative genes (Table 3). On chromosome 1, Glyma.01G043300 was a putative gene which was homologous to WRKY72 in Arabidopsis. The latter was reported to contribute to the basal immunity and defense against root-knot nematodes (Bhattarai et al., 2010). Moreover, AtWRKY72 can also utilize SA-independent defense mechanisms (Bhattarai et al., 2010). On chromosome 9, Glyma.09g063800 was an eukaryotic translation elongation factor EF1B and contained guanine nucleotide exchange domain (Andersen et al., 2000) and a GST C-terminal domain (Dixon et al., 2002). Glyma.09g063800 was homologous to eEF1Bβ in Arabidopsis. eEF1Bβ was reported to facilitate potato virus X infection and interacted with PVX TGBp1 (Hwang et al., 2015). In soybean, Luan et al. (2016) reported that knocked down the four GmEF1B homologs Glyma.13g073200, Glyma.02g276600, Glyma.14g039100, and Glyma.04g195100 enhanced resistance against the virulent G7 strain of SMV. On chromosome 12, we identified a receptor-like protein Glyma.12g233700 which was homologous to AtRLP9 in Arabidopsis. AtRLP9 was reported to co-regulate with Rust-Induced Secreted Protein (RISP), which was strong and specific induced by an avirulent strain of Melampsora larici-populina (Rinaldi et al., 2007). The gene expression analysis in response to downy mildew showed that AtRLP9 was distributed in pathology-related group which also contained AtRLP52, AtWRKY72, and other NBS-LRR class disease resistance protein (Coker et al., 2015). AtRLP52 was found to be involved in the resistance response (Ramonell et al., 2005). Therefore, these three genes (Glyma.01G043300, Glyma.09g063800, and Glyma.12g233700) were identified as putative genes related to SC7 resistance.
Expression of putative genes were analyzed through qRT-PCR at 0, 2, 3, and 4 days after SC7 or mock inoculated in a resistance (NJAU_M001) accession and a susceptible (NJAU_M082) accession. As shown in Figure 7, the expression of Glyma.01G043300 significantly decreased with SC7 inoculating than mock inoculating, which was consistent in both the resistance and susceptible accessions. On the contrary, the Glyma.09g063800 was significantly up-regulated in the SMV resistance NJAU_M001 soybean at 2, 3, and 4 days. And the expression level was the highest at 2 days after SC7 inoculating compared with mock inoculating in the same time. Meanwhile, in SMV susceptible soybean NJAU_M082, the expression of Glyma.09g063800 increased slightly at 0, 2, and 3 days and significantly increased at 4 days after SC7 inoculating compared with mock inoculating. The expression pattern of Glyma.12g233700 was different in the SMV resistant and susceptible soybean accessions. The expression levels of Glyma.12g233700 up-regulated in the SMV resistant soybean NJAU_M001, and decreased in SMV susceptible soybean NJAU_M082 after SMV inoculating compared with mock inoculating. These expression analyses indicate the three putative genes were induced by the infection of SC7, which should provide an important basis for further functional study of these SMV putative resistance genes.
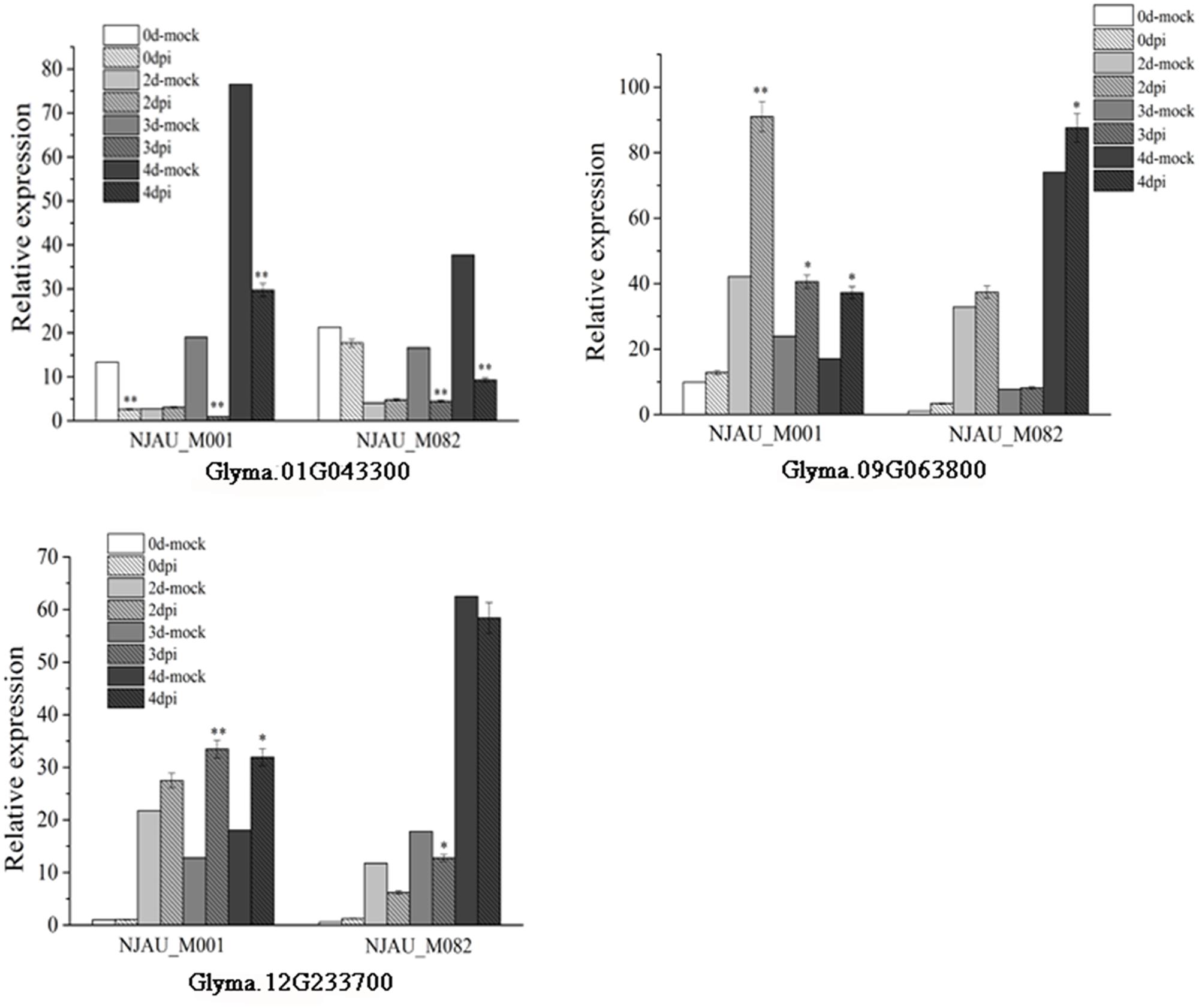
FIGURE 7. Expression analysis of the three putative genes in SMV resistant soybean NJAU_M001 and SMV susceptible soybean NJAU_M082. In the legend, 0, 2, 3, and 4 days-mock represent 0, 2, 3, and 4 days after inoculating with 0.01 mol/L sodium phosphate buffer, respectively. Dpi means days past inoculated with SC7. Error bar indicate the standard deviation. Results are representative of three biological replicates. ∗, significant at P ≤ 0.05; ∗∗, significant at P ≤ 0.01.
Discussion
Resistance in Development to SMV Was a Quantitative Trait That Ubiquitous in Soybean
Soybean mosaic virus is one of the serious diseases that cause severe yield loss in soybean. The spread of SMV disease is by aphid. Although chemical pesticide can kill aphid and interdict the diffuse of SMV, it can also increase the cost to the farmer and cause environment pollution. Many studies have been reported on inheritance of resistance in infection to SMV and three distinct dominant SMV resistant loci, namely Rsv1, Rsv2, and Rsv4 have been mapped in PI 96983, OX686, and V94-5152, respectively (Kiihl and Hartwig, 1979; Buzzell and Tu, 1989; Hayes et al., 2000). In addition to resistance in infection to SMV, Zhi et al. (2005) reported another genetic mechanism in resistance to SMV, which was called resistance to SMV extension or development. And the study showed that the resistance in development to Sa strains of SMV was controlled by one additive major gene plus additive-dominant polygenes (Zhi et al., 2005). Guo et al. (2012) also reported resistance genetic mechanism in development to SC3 was controlled by two equality-additive major genes. Up to now, many researchers have focused on the resistance to SMV infection, however, few studies have been focused on the resistance to SMV extension. Although the phenotypic reaction in resistance to SMV extension was not obvious than that to SMV infection, it was more extensive and durable in resistance to SMV. Accordingly, it is important to identify the loci that control the resistance to SMV extension. In this study, we treated SMV resistance as a quantitative trait and used DR which showed a roughly normal distribution (Figure 2, skewness and kurtosis as shown in Table 1) as the phenotypic data. Then we used GWAS to find putative resistance loci. These loci would provide a basis for further functional study and be applied in the breeding of resistant cultivars.
Linkage Disequilibrium in Soybean Mutant Population
Genome-wide association study was based on LD, which was different in various crops and populations, moreover, LD could be broken by historical recombinants (David et al., 2001). In maize, the LD decay was estimated to be ≤2 kb in diverse inbred lines, because maize was a kind of cross-pollination species (Michael et al., 2009; Yang et al., 2010). In rice, the LD decay distance of indica and japonica were ranged from 100 to 200 kb (Mather et al., 2007). In soybean, as a kind of self-pollination species, the LD decay distance was evaluated approximately 500 kb which was longer than other cross-pollination crops (Hyten et al., 2007). Moreover, LD was different in wild and cultivated soybeans, Wang J. et al. (2016) reported that the distance over which LD decays to half of its maximum value was 80 kb in wild soybeans and 130 kb in cultivated soybeans. In this study, the average extension of LD decay distance for the population consisting of 165 soybean mutants was estimated about 1000 kb, which was larger than the previously reported value in the natural population (Wang J. et al., 2016). The reasons for the high value LD in this study may result from the close genetic relatedness and a relatively small population size. By contrast, the natural population consists of different landraces from different geographic origins with phenotypic variations. Different geographic landraces and far genetic relatedness with each other result in a lower LD level comparing with near-isogenic accessions. The high LD level in soybean indicates the need for a small amount of markers in GWAS to identify SNPs associated with target trait, which would facilitate marker-assisted selection in breeding.
Novel Loci Identified in Resistance to SMV
Based on GLM model, we identified 104 SNPs significantly associated with resistance to SMV in four environments and BLUP. Some of these SNPs were located in or near previous reported QTLs that have been mapped by linkage analysis for resistance to SMV, soybean cyst nematode, Sclerotinia Stem Rot, fungal pathogen Phakopsora pachyrhizi, and Phytophthora sojae (Yue et al., 2001; Guo et al., 2006; Vuong et al., 2008, 2010; Wu et al., 2011; Harris et al., 2015; Jiao et al., 2015; Yan et al., 2015), suggesting these SNPs could be related with biotic stress. Nevertheless, half of these significant SNPs were only detected in a single environment. For example, on chromosome 1 and 3, some SNPs associated with resistance to SMV were detected in E1, E3, and BLUP, but only in BLUP the SNPs reached the significant level. Similarly, on chromosome 15, some SNPs in E2, E4, and BLUP were detected but few of them reached the significant level. These results may be result from a high threshold as P ≤ (1/61543) or -Log10P ≥ 4.79 set in this study. If we decreased the threshold, these SNPs would be repetitively identified in two environments at least. These results indicated that some resistance genes might exist in these genomic regions and the SNP markers we identified would helpful for assembling these genes to improve resistance to SMV.
Conclusion
In this study, the high-density SNP markers and a soybean mutant panel were used in GWAS to discover new resistance loci to SMV. In GWAS results, 104 SNPs significantly associated with DR to SMV. Many novel SNPs associated with the resistance to SMV were identified on chromosome 1, 3, 5, 9, 10, 12, 13, and 15. These novel SNPs located in or near biotic stress QTLs that previously reported. Then the genetic annotation and expression analyses identified three putative genes were relevant with resistance to SMV and were worthy of further investigation. Our results will provide insights for improving marker-assisted selection and molecular designed breeding strategies for new accession with resistance to SMV.
Author Contributions
This study was designed by HC and DY. SNP markers were analyzed by JW. ZC conducted the experiments, including phenotypic data evaluation and GWAS analysis. ZC wrote this manuscript. JW, DY, and HL revised the manuscript. FY, YY, LW, PZ, and JD provided input in experiments. All authors read and approved the final version to be published.
Conflict of Interest Statement
The authors declare that the research was conducted in the absence of any commercial or financial relationships that could be construed as a potential conflict of interest.
The reviewer PS and handling Editor declared their shared affiliation.
Acknowledgments
This work was supported in part by Ministry of Science and Technology (2016YFD0101005), Key Transgenic Breeding Program of China (2016ZX08004-003), National Natural Science Foundation of China (31370034, 31371644, and 31301342), and Jiangsu Collaborative Innovation Center for Modern Crop Production (JCIC-MCP).
Footnotes
References
Abdelmajid, K. M., Ramos, L., Leandro, L., Mbofung, G., Hyten, D. L., Kantartzi, S. K., et al. (2012). The ‘PI 438489B’by ‘Hamilton’SNP-based genetic linkage map of soybean [Glycine max (L.) Merr.] identified quantitative trait loci that underlie seedling SDS resistance. J. Plant Genome Sci. 1, 18–30. doi: 10.5147/jpgs.2012.0053
Adams, M. J., Antoniw, J. F., and Fauquet, C. M. (2005). Molecular criteria for genus and species discrimination within the family Potyviridae. Arch. Virol. 150, 459–479. doi: 10.1007/s00705-004-0440-6
Andersen, G. R., Pedersen, L., Valente, L., Chatterjee, I., Kinzy, T. G., Kjeldgaard, M., et al. (2000). Structural basis for nucleotide exchange and competition with tRNA in the yeast elongation factor complex eEF1A: eEF1Bα. Mol. Cell 6, 1261–1266. doi: 10.1016/S1097-2765(00)00122-2
Bhattarai, K. K., Atamian, H. S., Kaloshian, I., and Eulgem, T. (2010). WRKY72-type transcription factors contribute to basal immunity in tomato and Arabidopsis as well as gene-for-gene resistance mediated by the tomato R gene Mi-1. Plant J. 63, 229–240. doi: 10.1111/j.1365-313X.2010.04232.x
Bradbury, P. J., Zhang, Z., Kroon, D. E., Casstevens, T. M., Ramdoss, Y., and Buckler, E. S. (2007). TASSEL: software for association mapping of complex traits in diverse samples. Bioinformatics 23, 2633–2635. doi: 10.1093/bioinformatics/btm308
Buss, G. R., Roane, C. W., Tolin, S. A., and Chen, P. (1989). Inheritance of reaction to soybean mosaic virus in two soybean cultivars. Crop Sci. 29, 1439–1441. doi: 10.2135/cropsci1989.0011183X002900060022x
Buzzell, R., and Tu, J. (1989). Inheritance of a soybean stem-tip necrosis reaction to soybean mosaic virus. J. Hered. 80, 400–401. doi: 10.1093/oxfordjournals.jhered.a110882
Chen, P., Buss, G. R., Roane, C. W., and Tolin, S. A. (1991). Allelism among genes for resistance to soybean mosaic virus in strain-differential soybean cultivars. Crop Sci. 31, 305–309. doi: 10.2135/cropsci1991.0011183X003100020015x
Chen, P., Buss, G. R., Tolin, S. A., Gunduz, I., and Cicek, M. (2002). A valuable gene in Suweon 97 soybean for resistance to soybean mosaic virus. Crop Sci. 42, 333–337. doi: 10.2135/cropsci2002.3330
Chen, P., Ma, G., Buss, G. R., Gunduz, I., Roane, C. W., and Tolin, S. A. (2001). Inheritance and allelism tests of Raiden soybean for resistance to soybean mosaic virus. J. Hered. 92, 51–55. doi: 10.1093/jhered/92.1.51
Cho, E. K., and Goodman, R. M. (1979). Strains of soybean mosaic virus: classification based on virulence in resistant soybean cultivars. Phytopathology 69, 467–470. doi: 10.1094/Phyto-69-467
Cho, E. K., and Goodman, R. M. (1982). Evaluation of resistance in soybeans to soybean mosaic virus strains. Crop Sci. 22, 1133–1136. doi: 10.2135/cropsci1982.0011183X002200060012x
Coker, T. L., Cevik, V., Beynon, J. L., and Gifford, M. L. (2015). Spatial dissection of the Arabidopsis thaliana transcriptional response to downy mildew using Fluorescence Activated Cell Sorting. Front. Plant Sci. 6:527. doi: 10.3389/fpls.2015.00527
David, E., Reich, M. C., Stacey, B., James, I., Pardis, C. S., Daniel, J. R., et al. (2001). Linkage disequilibrium in the human genome. Nature 411, 199–204. doi: 10.1038/35075590
Dixon, D. P., Davis, B. G., and Edwards, R. (2002). Functional divergence in the glutathione transferase superfamily in plants identification of two classes with putative functions in redox homeostasis in Arabidopsis thaliana. J. Biol. Chem. 277, 30859–30869. doi: 10.1074/jbc.M202919200
Evanno, G., Regnaut, S., and Goudet, J. (2005). Detecting the number of clusters of individuals using the software STRUCTURE: a simulation study. Mol. Ecol. 14, 2611–2620. doi: 10.1111/j.1365-294X.2005.02553.x
Gore, M. A., Hayes, A. J., Jeong, S. C., Yue, Y. G., Buss, G. R., and Maroof, M. A. S. (2002). Mapping tightly linked genes controlling potyvirus infection at the Rsv1 and Rpv1 region in soybean. Genome 45, 592–599. doi: 10.1139/g02-009
Guo, B., Sleper, D. A., Nguyen, H. T., Arelli, P. R., and Shannon, J. G. (2006). Quantitative trait loci underlying resistance to three soybean cyst nematode populations in soybean PI 404198A. Crop Sci. 46, 224. doi: 10.2135/cropsci2004.0757
Guo, D., Chen, H., Yang, Z., Shan, Z., Zhu, X., Chen, S., et al. (2012). Analysis of resistance inheritance to soybean mosaic virus (SMV) strain SC-3 and mapping related QTL in soybean. Soybean Sci. 31, 511–516. doi: 10.3969/j.issn.1000-9841.2012.04.001
Guo, D., Zhi, H., and Wang, Y. (2005). Identification and distribution of soybean mosaic virus strains in Middle and Northern Huang Huai Region of China. Chin. J. Oil Crop Sci. 27, 64–68. doi: 10.3321/j.issn:1007-9084.2005.04.015
Han, S. Y., Yang, M. L., Chen, Y. D., Yu, J. J., Zhao, T. J., Gai, J. Y., et al. (2008). Construction of mutant library for soybean “Nannong 94-16” and analysis of some characters. Acta Agric. Nucl. Sin. 22, 131–135. doi: 10.11869/hnxb.2008.02.0131
Han, S. Y., Zhang, H. Y., Yang, M. L., Zhao, T. J., Gai, J. Y., and Yu, D. Y. (2007). Screening of mutants and construction of mutant population in soybean “Nannong 86-4”. Acta Agron. Sin. 33, 2059–2062. doi: 10.3321/j.issn:0496-3490.2007.12.022
Hao, D., Cheng, H., Yin, Z., Cui, S., Zhang, D., Wang, H., et al. (2012). Identification of single nucleotide polymorphisms and haplotypes associated with yield and yield components in soybean (Glycine max) landraces across multiple environments. Theor. Appl. Genet. 124, 447–458. doi: 10.1007/s00122-011-1719-0
Harris, D. K., Abdel-Haleem, H., Buck, J. W., Phillips, D. V., Li, Z., and Boerma, H. R. (2015). Soybean quantitative trait loci conditioning soybean rust-induced canopy damage. Crop Sci. 55, 2589–2597. doi: 10.2135/cropsci2015.01.0058
Hayes, A. J., Jeong, S. C., Gore, M. A., Yu, Y. G., Buss, G. R., Tolin, S. A., et al. (2004). Recombination within a nucleotide-binding-site/leucine-rich-repeat gene cluster produces new variants conditioning resistance to soybean mosaic virus in soybeans. Genetics 166, 493–503. doi: 10.1534/genetics.166.1.493
Hayes, A. J., Ma, G., Buss, G. R., and Maroof, M. A. S. (2000). Molecular marker mapping of RSV4, a gene conferring resistance to all known strains of soybean mosaic virus. Crop Sci. 40, 1434–1437. doi: 10.2135/cropsci2000.4051434x
Huang, X., Wei, X., Sang, T., Zhao, Q., Feng, Q., Zhao, Y., et al. (2010). Genome-wide association studies of 14 agronomic traits in rice landraces. Nat. Genet. 42, 961–967. doi: 10.1038/ng.695
Hwang, J., Lee, S., Lee, J. H., Kang, W. H., Kang, J. H., Kang, M. Y., et al. (2015). Plant translation elongation factor 1Bbeta facilitates Potato virus X (PVX) infection and interacts with PVX triple gene block protein 1. PLOS ONE 10:e0128014. doi: 10.1371/journal.pone.0128014
Hyten, D. L., Choi, I. Y., Song, Q., Shoemaker, R. C., Nelson, R. L., Costa, J. M., et al. (2007). Highly variable patterns of linkage disequilibrium in multiple soybean populations. Genetics 175, 1937–1944. doi: 10.1534/genetics.106.069740
Jeong, S. C., Kristipati, S., Hayes, A. J., Maughan, P. J., Noffsinger, S. L., Gunduz, I., et al. (2002). Genetic and sequence analysis of markers tightly linked to the soybean mosaic virus resistance gene, Rsv3. Crop Sci. 42, 265–270. doi: 10.2135/cropsci2002.2650
Jiao, Y., Vuong, T. D., Liu, Y., Meinhardt, C., Liu, Y., Joshi, T., et al. (2015). Identification and evaluation of quantitative trait loci underlying resistance to multiple HG types of soybean cyst nematode in soybean PI 437655. Theor. Appl. Genet. 128, 15–23. doi: 10.1007/s00122-014-2409-5
Kiihl, R. A., and Hartwig, E. (1979). Inheritance of reaction to soybean mosaic virus in soybeans. Crop Sci. 19, 372–375. doi: 10.2135/cropsci1979.0011183X001900030024x
Kim, H., Xing, G., Wang, Y., Zhao, T., Yu, D., Yang, S., et al. (2014). Constitution of resistance to common cutworm in terms of antibiosis and antixenosis in soybean RIL populations. Euphytica 196, 137–154. doi: 10.1007/s10681-013-1021-0
Korte, A., and Farlow, A. (2013). The advantages and limitations of trait analysis with GWAS: a review. Plant Methods 9:29. doi: 10.1186/1746-4811-9-29
Li, Y. H., Li, W., Zhang, C., Yang, L., Chang, R. Z., Gaut, B. S., et al. (2010). Genetic diversity in domesticated soybean (Glycine max) and its wild progenitor (Glycine soja) for simple sequence repeat and single-nucleotide polymorphism loci. New Phytol. 188, 242–253. doi: 10.1111/j.1469-8137.2010.03344.x
Luan, H., Shine, M., Cui, X., Chen, X., Ma, N., Kachroo, P., et al. (2016). The potyviral P3 protein targets EF1A to promote the unfolded protein response and viral pathogenesis. Plant Physiol. 172, 221–234. doi: 10.1104/pp.16.00505
Ma, G., Chen, P., Buss, G. R., and Tolin, S. A. (1995). Genetic characteristics of two genes for resistance to soybean mosaic virus in PI486355 soybean. Theor. Appl. Genet. 91, 907–914. doi: 10.1007/bf00223899
Ma, G., Chen, P., Buss, G. R., and Tolin, S. A. (2003). Genetic study of a lethal necrosis to soybean mosaic virus in PI 507389 soybean. J. Hered. 94, 205–211. doi: 10.1093/jhered/esg059
Mackay, T. F. (2001). Quantitative trait loci in Drosophila. Nat. Rev. Genet. 2, 11–20. doi: 10.1038/35047544
Mather, K. A., Caicedo, A. L., Polato, N. R., Olsen, K. M., McCouch, S., and Purugganan, M. D. (2007). The extent of linkage disequilibrium in rice (Oryza sativa L.). Genetics 177, 2223–2232. doi: 10.1534/genetics.107.079616
Merk, H. L., Yarnes, S. C., Deynze, A. V., Tong, N., Menda, N., Mueller, L. A., et al. (2012). Trait diversity and potential for selection indices based on variation among regionally adapted processing tomato germplasm. J. Am. Soc. Hortic. Sci. 137, 427–437.
Michael, A., Gore, J. M. C., Robert, J., Elshire, Q. S., Elhan, S. E., Bonnie, L. H., et al. (2009). A first-generation haplotype map of maize. Science 326, 1115–1117. doi: 10.1126/science.1177837
Pu, Z., Cao, Y., Fang, C., Xue, B., and Fang, Z. (1983). Resistance in soybean varieties to six strains of soybean mosaic virus. J. Nanjing Agric. Univ. 3, 005. doi: 10.7685/j.issn.1000-2030.1983.03.006
Qiu, B., Arelli, P., and Sleper, D. (1999). RFLP markers associated with soybean cyst nematode resistance and seed composition in a ‘Peking’ × ‘Essex’ population. Theor. Appl. Genet. 98, 356–364. doi: 10.1007/s001220051080
R Development Core Team (2015). R: A Language and Environment for Statistical Computing. Vienna: R Foundation for Statistical Computing.
Ramonell, K., Berrocal-Lobo, M., Koh, S., Wan, J., Edwards, H., Stacey, G., et al. (2005). Loss-of-function mutations in chitin responsive genes show increased susceptibility to the powdery mildew pathogen Erysiphe cichoracearum. Plant Physiol. 138, 1027–1036. doi: 10.1104/pp.105.060947
Rector, B., All, J., Parrott, W., and Boerma, H. (1999). Quantitative trait loci for antixenosis resistance to corn earworm in soybean. Crop Sci. 39, 531–538. doi: 10.2135/cropsci1999.0011183X003900020038x
Rinaldi, C., Kohler, A., Frey, P., Duchaussoy, F., Ningre, N., Couloux, A., et al. (2007). Transcript profiling of poplar leaves upon infection with compatible and incompatible strains of the foliar rust Melampsora larici-populina. Plant Physiol. 144, 347–366. doi: 10.1104/pp.106.094987
Shao, Z. Q., Zhang, Y. M., Hang, Y. Y., Xue, J. Y., Zhou, G. C., Wu, P., et al. (2014). Long-term evolution of nucleotide-binding site-leucine-rich repeat genes: understanding gained from and beyond the legume family. Plant Physiol. 166, 217–234. doi: 10.1104/pp.114.243626
Shirasawa, K., Fukuoka, H., Matsunaga, H., Kobayashi, Y., Kobayashi, I., Hirakawa, H., et al. (2013). Genome-wide association studies using single nucleotide polymorphism markers developed by re-sequencing of the genomes of cultivated tomato. DNA Res. 20, 593–603. doi: 10.1093/dnares/dst033
Silva, M. F., Kiihl, R. A. S., and Almeida, Á. M. R. (2004). Inheritance of resistance to Soybean mosaic virus in FT-10 soybean. Euphytica 135, 339–343. doi: 10.1023/b:euph.0000013371.00994.52
Tamulonis, J. P., Luzzi, B. M., Hussey, R. S., Parrott, W. A., and Boerma, H. R. (1997). RFLP mapping of resistance to southern root-knot nematode in soybean. Crop Sci. 37, 1903–1909. doi: 10.2135/cropsci1997.0011183X003700060039x
Tucker, D. M., Maroof, M. A. S., Jeong, S. C., Buss, G. R., and Tolin, S. A. (2009). Validation and interaction of the soybean mosaic virus lethal necrosis allele, Rsv1-n, in PI 507389. Crop Sci. 49, 1277–1283. doi: 10.2135/cropsci2008.08.0477
Vuong, T., Diers, B., and Hartman, G. (2008). Identification of QTL for resistance to Sclerotinia stem rot in soybean plant introduction 194639. Crop Sci. 48, 2209–2214. doi: 10.2135/cropsci2008.01.0019
Vuong, T. D., Sleper, D. A., Shannon, J. G., and Nguyen, H. T. (2010). Novel quantitative trait loci for broad-based resistance to soybean cyst nematode (Heterodera glycines Ichinohe) in soybean PI 567516C. Theor. Appl. Genet. 121, 1253–1266. doi: 10.1007/s00122-010-1385-7
Wang, D., Ma, Y., Yang, Y., Liu, N., Li, C., Song, Y., et al. (2011). Fine mapping and analyses of RSC8 resistance candidate genes to soybean mosaic virus in soybean. Theor. Appl. Genet. 122, 555–565. doi: 10.1007/s00122-010-1469-4
Wang, H., Waller, L., Tripathy, S., Martin, S. K., Zhou, L., Krampis, K., et al. (2010). Analysis of genes underlying soybean quantitative trait loci conferring partial resistance to Phytophthora sojae. Plant Genome 3, 23–40. doi: 10.3835/plantgenome2009.12.0029
Wang, J., Chu, S., Zhang, H., Zhu, Y., Cheng, H., and Yu, D. (2016). Development and application of a novel genome-wide SNP array reveals domestication history in soybean. Sci. Rep. 6:20728. doi: 10.1038/srep20728
Wang, X., Wang, H., Liu, S., Ferjani, A., Li, J., Yan, J., et al. (2016). Genetic variation in ZmVPP1 contributes to drought tolerance in maize seedlings. Nat. Genet. 48, 1233–1241. doi: 10.1038/ng.3636
Wang, X. Q., Gai, J. Y., and Pu, Z. Q. (2003). Classification and distribution of strain groups of soybean mosaic virus in middle and lower Huang-Huai and Changjiang valleys. Soybean Sci. 22, 102–107. doi: 10.3969/j.issn.1000-9841.2003.04.001
Wu, X., Blake, S., Sleper, D. A., Shannon, J. G., Cregan, P., and Nguyen, H. T. (2009). QTL, additive and epistatic effects for SCN resistance in PI 437654. Theor. Appl. Genet. 118, 1093–1105. doi: 10.1007/s00122-009-0965-x
Wu, X., Zhou, B., Zhao, J., Guo, N., Zhang, B., Yang, F., et al. (2011). Identification of quantitative trait loci for partial resistance to Phytophthora sojae in soybean. Plant Breed. 130, 144–149. doi: 10.1111/j.1439-0523.2010.01799.x
Yan, H., Wang, H., Cheng, H., Hu, Z., Chu, S., Zhang, G., et al. (2015). Detection and fine-mapping of SC7 resistance genes via linkage and association analysis in soybean. J. Integr. Plant Biol. 57, 722–729. doi: 10.1111/jipb.12323
Yang, X., Gao, S., Xu, S., Zhang, Z., Prasanna, B. M., Li, L., et al. (2010). Characterization of a global germplasm collection and its potential utilization for analysis of complex quantitative traits in maize. Mol. Breed. 28, 511–526. doi: 10.1007/s11032-010-9500-7
Yang, Y., Zheng, G., Han, L., Dagang, W., Yang, X., Yuan, Y., et al. (2013). Genetic analysis and mapping of genes for resistance to multiple strains of Soybean mosaic virus in a single resistant soybean accession PI 96983. Theor. Appl. Genet. 126, 1783–1791. doi: 10.1007/s00122-013-2092-y
Yesudas, C. R., Sharma, H., and Lightfoot, D. A. (2010). Identification of QTL in soybean underlying resistance to herbivory by Japanese beetles (Popillia japonica, Newman). Theor. Appl. Genet. 121, 353–362. doi: 10.1007/s00122-010-1314-9
Yue, P., Arelli, P., and Sleper, D. (2001). Molecular characterization of resistance to Heterodera glycines in soybean PI 438489B. Theor. Appl. Genet. 102, 921–928. doi: 10.1007/s001220000453
Zhang, D., Song, H., Cheng, H., Hao, D., Wang, H., Kan, G., et al. (2014). The acid phosphatase-encoding gene GmACP1 contributes to soybean tolerance to low-phosphorus stress. PLOS Genet. 10:e1004061. doi: 10.1371/journal.pgen.1004061
Zhang, G., Gu, C., and Wang, D. (2009). Molecular mapping of soybean aphid resistance genes in PI 567541B. Theor. Appl. Genet. 118, 473–482. doi: 10.1007/s00122-008-0914-0
Zhao, G., Wang, J., Han, Y., Teng, W., Sun, G., and Li, W. (2008). Identification of QTL underlying the resistance of soybean to pod borer, Leguminivora glycinivorella (Mats.) obraztsov, and correlations with plant, pod and seed traits. Euphytica 164, 275–282. doi: 10.1007/s10681-008-9728-z
Keywords: association analysis, soybean mosaic virus, linkage disequilibrium, soybean, mutant population
Citation: Che Z, Liu H, Yi F, Cheng H, Yang Y, Wang L, Du J, Zhang P, Wang J and Yu D (2017) Genome-Wide Association Study Reveals Novel Loci for SC7 Resistance in a Soybean Mutant Panel. Front. Plant Sci. 8:1771. doi: 10.3389/fpls.2017.01771
Received: 21 June 2017; Accepted: 28 September 2017;
Published: 11 October 2017.
Edited by:
Rosa Ana Malvar, Misión Biológica de Galicia (CSIC), SpainReviewed by:
Pilar Soengas, Misión Biológica de Galicia (CSIC), SpainBernardo Ordas, Consejo Superior de Investigaciones Científicas (CSIC), Spain
Copyright © 2017 Che, Liu, Yi, Cheng, Yang, Wang, Du, Zhang, Wang and Yu. This is an open-access article distributed under the terms of the Creative Commons Attribution License (CC BY). The use, distribution or reproduction in other forums is permitted, provided the original author(s) or licensor are credited and that the original publication in this journal is cited, in accordance with accepted academic practice. No use, distribution or reproduction is permitted which does not comply with these terms.
*Correspondence: Jiao Wang, wjiao@njau.edu.cn Deyue Yu, dyyu@njau.edu.cn