- 1Institute of Agricultural Sciences (ICA), Spanish National Research Council (CSIC), Madrid, Spain
- 2Escuela Técnica Superior de Ingeniería Agronómica, Alimentaria y de Biosistemas (ETSIAAB), Universidad Politécnica de Madrid, Madrid, Spain
- 3Centre for Artificial Intelligence, University College London, London, United Kingdom
- 4Environment and Agronomy Department, National Institute for Agricultural and Food Research and Technology (INIA), Spanish National Research Council (CSIC), Madrid, Spain
Crop protection is a key activity for the sustainability and feasibility of agriculture in a current context of climate change, which is causing the destabilization of agricultural practices and an increase in the incidence of current or invasive pests, and a growing world population that requires guaranteeing the food supply chain and ensuring food security. In view of these events, this article provides a contextual review in six sections on the role of artificial intelligence (AI), machine learning (ML) and other emerging technologies to solve current and future challenges of crop protection. Over time, crop protection has progressed from a primitive agriculture 1.0 (Ag1.0) through various technological developments to reach a level of maturity closelyin line with Ag5.0 (section 1), which is characterized by successfully leveraging ML capacity and modern agricultural devices and machines that perceive, analyze and actuate following the main stages of precision crop protection (section 2). Section 3 presents a taxonomy of ML algorithms that support the development and implementation of precision crop protection, while section 4 analyses the scientific impact of ML on the basis of an extensive bibliometric study of >120 algorithms, outlining the most widely used ML and deep learning (DL) techniques currently applied in relevant case studies on the detection and control of crop diseases, weeds and plagues. Section 5 describes 39 emerging technologies in the fields of smart sensors and other advanced hardware devices, telecommunications, proximal and remote sensing, and AI-based robotics that will foreseeably lead the next generation of perception-based, decision-making and actuation systems for digitized, smart and real-time crop protection in a realistic Ag5.0. Finally, section 6 highlights the main conclusions and final remarks.
1 Linking crop protection to the technological evolution of agriculture
Crop protection involves a large number of critical farming activities with a decisive impact on the viability and sustainability of agriculture. Throughout history, humans have developed new methods and practices to protect their crops. From ancient times to about 1950, agriculture 1.0 employed a large workforce to manually control crop pests (i.e., plant diseases, weeds and other plagues, both vertebrate and invertebrate), which produced low yields but in sufficient quantity to feed the population. In the late 1950s, agriculture 2.0 began with the use of synthetic pesticides and specialized machines to control the common crop pests. At that stage, agriculture evolved towards the economic edge, aiming to produce more food at a cheaper price, i.e., towards a more industrialized agriculture. At the end of the 20th century, agriculture 3.0 emerged with the idea of using new technologies and data-driven modeling as essential tools to take decisions and manage cropping systems. This disruptive concept led to the origin of precision agriculture, in which telematics, global navigation satellite systems (GNSS), machinery guidance, and sensing devices aimed to optimize the crop protection tasks, to reduce costs and environmental impacts of pesticides, and to improve food quality. What followed was a further step in the integration of geo-spatial technologies, computer sciences and digitization into the agricultural process, where sensors, mobile telephony, embedded systems, cloud computing, internet of things (IoT) and big data were incorporated on board of autonomous machinery, smart sprayers and actuators to facilitate the application of the precision crop protection paradigm within the concept of agriculture 4.0 (Zhai et al., 2020). Continuing this evolution, Agriculture 5.0 (Ag5.0) will promote a new era of intelligent crop management with automatized decision making processes, unmanned operations and progressively less human intervention supported by the latest Artificial Intelligence (AI) systems, advanced robotics, and powerful Machine Learning (ML) algorithms (Saiz-Rubio and Rovira-Más, 2020).
Modern agriculture will face in the next decades two immense challenges never seen in previous generations. The first one is the impact of climate change in agricultural systems (Hoegh-Guldberg et al., 2019), which causes destabilization of farming practices (Mulla et al., 2020) and irregular crop seasons due to excessive heat and water scarcity in large productive areas (Piao et al., 2019); (Falkland and White, 2020), which inevitably leads to the emergence of new invasive pests or the increased severity of existing ones. The second one is to produce food for a growing human and animal population, while ensuring food security by using fewer agrochemicals and imposing strict controls at all stages of the agricultural supply chain (van Dijk et al., 2020). In view on this imminent future, Ag5.0 must offer creative solutions based on AI, ML algorithms and other technological innovations that continuously interact with the crop and its environment, which will require undoubtedly transdisciplinary studies and interdisciplinary collaborations, where precision crop protection becomes a key discipline in the Ag5.0 revolution by implementing new procedures and strategies to drastically reduce the use of agrochemicals in the control of diseases, weeds and plagues.
2 The stages of precision crop protection: Perception, analysis and actuation
The use of new technologies in crop protection aims at detecting and identifying the symptoms or problems caused by crop pests (Behmann et al., 2015), followed by a site-specific application of a chemical or mechanical control action. This process comprisesthe three main stages for pursuing a precision crop protection strategy, as follows (Figure 1): 1) perception, 2) analysis and, optionally (but recommendable) decision-making, and 3) actuation. The perception stage involves field inspection andacquisition of plant information (e.g., crop and/or weed imaging) through a sensor or camera mounted on an on-ground or a remotely-sensed platform, while the actuation stage consists on the application of a prescribed site-specific treatment with a smartequipment usually assisted by a GNSS receiver. The necessary link between perception and actuation is the analysis stage, which consists of in-depth evaluation of digital crop data by using diverse data-driven techniques and identifying targeting areas of crops with problems associated to diseases, weeds and plagues. The analysis stage also often includes the generation of management zones and treatment/prescription maps following a decision-making process, e.g. based on the outcomes of a decision support system (DSS).
Recent bibliographic reviews point out to Unmanned Aerial Vehicles (UAVs), innovative ML algorithms, and various robots and autonomous equipment as the most disruptive technology for each stage, respectively (Filho et al., 2020; Lima et al., 2020; Dainelli et al., 2021). UAVs are playing an important role in the perception stage due to their capability to capture crop data from large areas in a short time and with diverse types of cameras and sensors (e.g., RGB cameras, multi- and hyper- spectral sensors, thermal cameras, active sensors such as LiDAR, radar or sonar), which have led to significant progress in pest monitoring with the help of powerful analysis procedures, either by direct observation of the pest (e.g., weed patches), by diagnosis of the main symptoms of the disease (e.g., leave decay or thermal stress), or by detection of damages caused in the crop leaves and canopy (e.g., foliar losses due to a plague attack).
The analysis stage is the major challenge for many crops, probably being the bottleneck for the progress of precision crop protection. The ultimate objective of this stage is the accurate and timely detection of each crop-specific disease, weed or plague,whose complexity lies in the vast number of possible crop-pest scenarios with a diverse typology of associated symptoms, in addition to other environmental and cultural factors such as different weather conditions, soil properties, and farmers’ decisions on crop field management, which impact the type and degree of severity of pest occurrences (Oerke et al., 2012; Pätzold et al., 2020). This diversity of variables and factors can be addressed by ML methods with the ability to learn from experience (i.e., data) and integrate information from multiple sources. ML enables the analysis of massive amounts of crop and pest data over time by taking advantage of the continuous evolution of the hardware with increasingly powerful central (CPU), graphics (GPU) and tensor (TPU) processing units (Wang et al., 2019). As a result, ML can study the behavior of natural crop-pest systems by capturing and exploiting the underlying patterns in the data and build predictive/generative models accordingly for critical analytical tasks such as image classification, object detection, pattern recognition, geo-location, etc., aimed to propose solutions for complex crop protection challenges.
Finally, actuation is the task that leveraged large-scale viability of precision crop protection strategies, leading to great scientific and technological effort in the last decade to develop autonomous machinery, smart sprayers and agricultural robots that effectively implement site-specific crop management (Shafi et al., 2019; Lowenberg-DeBoer et al., 2021), either by direct treatment in real-time (Pérez-Ruiz et al., 2015) or, eventually, assisted by a prescription map (Fernández-Quintanilla et al., 2018) according to the principles established by the International Society of Precision Agriculture (ISPA, 2021).
3 ML taxonomy based on the tasks to be solved
The ML algorithms have been conventionally classified according to different criteria, based on: i) the nature of the model (full or partial probabilistic/generative model vs. discriminant model), ii) the type of reasoning applied (inductive or transductive, depending on whether the model performs a reasoning from observed training cases to general rules or the other way around, respectively), or iii) the data availability and the supervision process (unsupervised, supervised, semi-supervised and reinforcement learning). However, the extent of ML within the scope of precision crop protection is best described by an alternative criterion based on the task to be solved, which leads to an expanded taxonomy of six categories, as follows: classification, regression, clustering, anomaly detection, dimensionality reduction, and association rule learning. These six tasks can be addressed with traditional ML algorithms or, for some specific tasks mainly classification and regression, with the more advanced artificial neural network (ANN) models, which in turn also include Deep Learning (DL) algorithms (Figure 2).
3.1 Traditional ML algorithms
Traditional ML algorithms usually approach learning tasks by analyzing and interpreting input data with well-established architectures optimized for common computing resources, thus often achieving satisfactory results but with less accuracy and versatility than sophisticated ANN algorithms. Of the tasks listed above, classification is the most common in many disciplines, with well-known algorithms such as support vector machine (SVM), decision trees (DT), random forest (RF), K-Nearest Neighbor (k-NN), etc. Classification algorithms are part of the supervised learning type, aiming to categorize a certain set of structured or unstructured data in classes, being a binary classification when the objective is to predict the state of true or false, and a multi-category classification when there are more than two objective classes (Sen et al., 2020; Djafri and Gafour, 2022). These algorithms are used for predictive tasks in the fields of image analysis, video, object recognition, data mining, etc. (Kowsari et al., 2019), all of which are relevant to deal with the challenge of automatic identification, detection or classification of plant diseases, weeds and plagues. This objective also usually requires previous phases such as image/video preprocessing, segmentation and feature extraction that imply the use of other algorithms of the regression, clustering and dimensionality reduction typology.
The regression algorithms are also part of the supervised learning type and consist in relating continuous input and output variables through a function, which can be set by parametric or non-parametric approaches. In the former case, the output values are predicted by an explicit analytical formula that adjusts the known points by establishing and minimizing a cost function (e.g. linear regression) that link the input and output variables (Wei et al., 2015; Gaitán, 2020). In the latter case, a kernel function is defined to determine the prediction for the output based on similar experiences of the inputs, hence it depends on the correlation between the output and the known points surrounding the input (Čížek and Sadikoğlu, 2020). A form of regression that allows correction of overfitting is the regularization algorithms, which avoid generating low error (i.e., high accuracy) in the training but high error during the testing (Zou and Hastie, 2005). Common algorithms in this group are LASSO Regularization, Ridge Regularization and Elastic Net Regularization.
Within the two previous categories, the ensemble algorithms are the combination of predictions from various ML techniques applied to a single model improving predictive performance (Sagi and Rokach, 2018). In classification, an ensemble of classifiers is generally more accurate than the individual classifiers that compose it. Individual decisions are combined by weighted or unweighted votes in the classification of new examples (Hooftman et al., 2022), which allows a good balance between performance and computational cost (Telikani et al., 2022). The ensemble algorithms in regression improve accuracy while reduce bias and variance errors, avoiding over-adjustment when results deserve extra training (Ren et al., 2016). Some outstanding algorithms in this group are adaBoost, bootstrap aggregation (Bagging), category boosting (CatBoost), extremely randomized trees, gradient boosting machines (GBM), RF, stacked generalization (Stacking).
The clustering algorithms are part of the unsupervised and semi-supervised learning methods, which allow grouping the data into sets of similar objects to maximize the intra-cluster similarity and minimize inter-cluster similarity (Ezugwu et al., 2022). The partitional clustering applies techniques to obtain a single partition by an objective function of the input data, in a fixed number of clusters, using iterative relocation clusters and resulting in the best configuration of the total number of executions (Nanda and Panda, 2014). The hierarchical clustering performs the division of data (root node) by a sequence of nested partitions, known as tree type structures (dendrograms). This approach follows a type of pattern agglomerated (from bottom to top) or by divisive clustering (from top to bottom), with no need to define the number of clusters in advance (Murtagh and Contreras, 2012).
The dimensionality reduction algorithms transform a high-dimensional data set into a representative lower-dimensional subset, as not all data features may be equally relevant for the problem at hand, greatly reducing computational complexity (Xu et al., 2019). This technique is widely used for data preprocessing, by two different ways: i) feature selection, in which the input features are combined to obtain a new dataset with a smaller number of new variables that retain the original information based on the input components and projection, and ii) feature extraction, in which the most relevant features of the original dataset are kept by removing those features that contribute little or nothing to the output features (Chhikara et al., 2020).
The anomaly detection algorithms try to find patterns, outliers or some kind of exception in the data that do not conform to the expected behavior (Chandola et al., 2009), by mean of a function that decide about the detection of an unknown or heterogeneous novelty present in the datasets with a class imbalance (Guansong et al., 2022). Isolation Forest, One-Class SVM, and PCA-Based Anomaly Detection are the most common algorithms to detect anomalies with application in crop protection.
The association rule learning algorithms serve to find regularities present in parts of the dataset (descriptive rules) and generalize the dataset to enable predictions on new data (predictive rules) (Fürnkranz and Kliegr, 2015). These algorithms can identify an association rule in the form A→B, based on the indicators support, confidence and lift. Support from A→B is the percentage of all items in A and B. Confidence is the percentage of A and B by the percentage of A. Lift indicates the probability of B occurring since A has occurred (Hashimoto et al., 2018). Within this category, the algorithms Apriori and Eclat are the most popular.
3.2 Artificial neural networks and deep learning models
The ANN algorithms are highly customizable and flexible computing models roughly inspired by biological neural networks, based on creating connected networks of simple processing units (neurons) that together can learn complex patterns and solve undefined problems. The ANNs works as universal approximators for any mathematical function, whose learning process is based on training from large datasets through sequential computations until accurate patterns are obtained. Then, when new patterns are presented, ANNs are able to predict them. These algorithms are mainly applied in tasks of classification and regression, e.g. in approximation functions (i.e. mapping multiple inputs to a single output), pattern classification (i.e. identification of new patterns through association and pattern recognition), associative memories (i.e. pattern recognition from limited information in the subset of data), and generation of new significant patterns, which can help in the reconstruction of patterns with greater characteristics (Schmidhuber, 2015).
Neural networks with two or more layers are the conceptual basis to generate DL models, whose progress has been spectacular in recent years in all disciplines, even in precision crop protection (Ferentinos, 2018; Kamilaris and Prenafeta-Boldú, 2018; Xia et al., 2018; Farooq et al., 2019; Hasan et al., 2021; Rakhmatulin et al., 2021; Allmendinger et al., 2022; Tugrul et al., 2022; Rai et al., 2023). DL algorithms transform data to construct complex concepts in a hierarchical structure with several levels of abstraction, so that the higher levels are composed of the characteristics of the lower levels (LeCun et al., 2015). The great potential of DL in many fields employing image analysis is allowing small data sets to be fitted to pre-trained models with different data, reducing training time and optimizing hardware resources (Kamilaris and Prenafeta-Boldú, 2018). DL covers different approaches suited to specific problems, for example, convolutional neural networks (CNNs) are used in computer vision and image classification, recurrent neural networks (RNNs) are used for prediction and language modelling, autoencoder is used in dimensionality reduction, and generative adversarial networks (GANs) are used in the generation of new images (Sarker, 2021).
CNN architectures for image classification is the most common application of DL in precision crop protection. The CNN algorithms find the features of objects of interest by self-learning from the image data, in contrast to traditional ML algorithms that require the user to establish such features (Hong et al., 2020). Performance of CNNs varies depending of number of parameters and convolutional layers (network depth), which in turn is directly constrained by the power of the available computing resources (Table 1). A broader application of CNN-based classifiers is object detection, which overcomes the issue of visual recognition in multi-class domains and object labelling in computer vision. Examples of CNN architectures for object detection and classification implemented in crop protection include Region-based Convolutional Neural Network (R-CNN) (Girshick et al., 2014), Fast R-CNN (Girshick, 2015), Faster R-CNN (Ren et al., 2015), You Only Look Once (YOLO) (Redmon et al., 2016), Single Shot Detector (SSD) (Liu et al., 2016), Feature Pyramid Networks (FPN) (Lin et al., 2017a), RetinaNet (Lin et al., 2017b) and Mask R-CNN (He et al., 2017).
4 Scientific impact and relevant contributions of ML in precision crop protection
An extensive bibliometric study of the Scopus database (www.scopus.com) revealed 107 traditional ML algorithms and 18 ANN models applied in all disciplines between 2010 and 2022, of which 105 and 17 algorithms, respectively, have been implemented in precision crop protection objectives with diverse degree of contribution in the domains of crop diseases, weeds and plagues (Table 2). SVM topped the list of traditional algorithms applied in precision crop protection objectives with >1,700 publications, followed by linear regression (LR) and Stacking with >1,500 publications each one. Principal Component Analysis (PCA), RF and DT are other algorithms with high impact reaching more than 1,100 publications each. A four group of relevant algorithmsis formed by Bagging, logistic regression (LoR), k-NN and k-means clustering, which appear in more than 500 publications of precision crop protection. Some algorithms rank relatively high in terms of their use in precision crop protection in comparison to all disciplines (PCP/All), such as k-NN, simple linear iterative clustering (SLIC), stacking and stepwise discriminant analysis (SDA) (>10% PCP/All), or in comparison to precision agriculture (PCP/PA), such as Gaussian Mixture Regression (GMR) (>70% PCP/PA). Among the ANN models, convolutional neural networks (CNNs) are by far the most widely used in precision crop protection with >1,200 publications, mainly focused on detecting and classifying crop diseases, weeds or plagues with image-based technology, with ResNet, GoogLeNet and VGGNet being the most applied models, and to a lesser extent LeNet and Xception models (Figure 3).
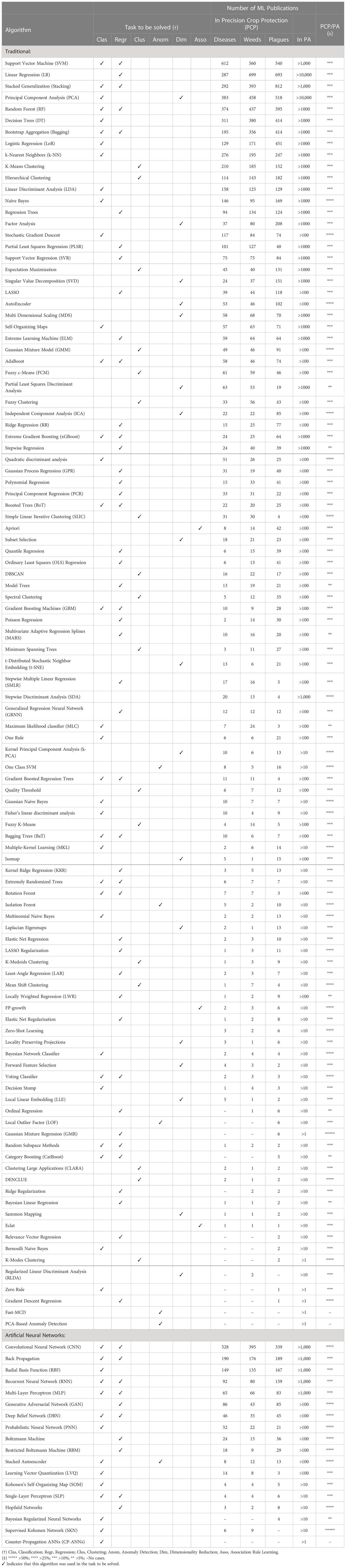
Table 2 Numbers publications of machine learning algorithms according to the proposal taxonomy (source Scopus).
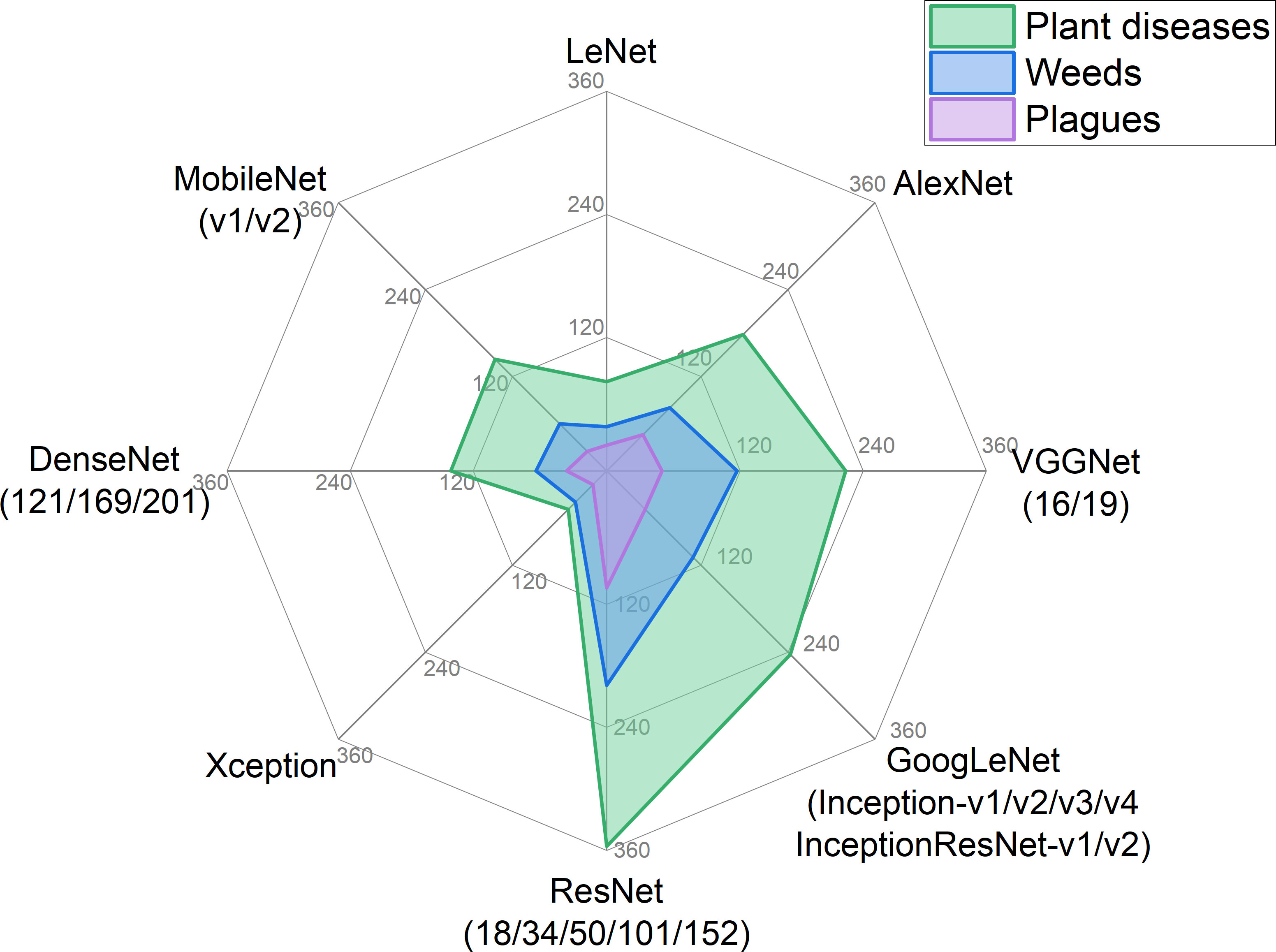
Figure 3 Number of publications of CNN architectures commonly used in the three domains of precision crop protection (crop diseases, weeds and crop plagues) from 2010 to 2022 (source: Scopus). Figure compiled with the conjunction of “CNN architecture” and each of the three crop protection domains (crop diseases, weeds and crop plagues) as search criteria within the article title, abstract and keywords.
A temporal analysis on ML-based publications shows that the adoption of ML algorithms has increased steadily year on year across all disciplines over the last decade (Figure 4A), which in turn is boosting the development of precision crop protection strategies (Figure 4B). Comparing the trends in both figures, peak values were reached in the last year in all cases, with classification and regression tasks being the most common by far in the group of traditional ML algorithms (55% and 29% across all cases and 47% and 41% in precision crop protection, respectively), followed by clustering, anomaly detection and dimensionality reduction tasks in the case of all disciplines, with considerably less impact (11%, 3% and 2%, respectively), and a negligible value for association rule learning. However, the dimensionality reduction algorithms were much more widely used in precision crop protection (11%) than the other three categories. In the case of ANN algorithms, their use has increased significantly in the last five years, counting 29,956 (Figure 4A) and 759 new publications (Figure 4B) in 2022 across all disciplines and in precision crop protection, respectively. Compared to the traditional ML algorithms, ANN algorithms remain at the highest rates since 2018 across all disciplines, but still do not exceed traditional classification algorithms in precision crop protection, although they did overcome dimensionality reduction algorithms in 2019 and regression algorithms in 2022.
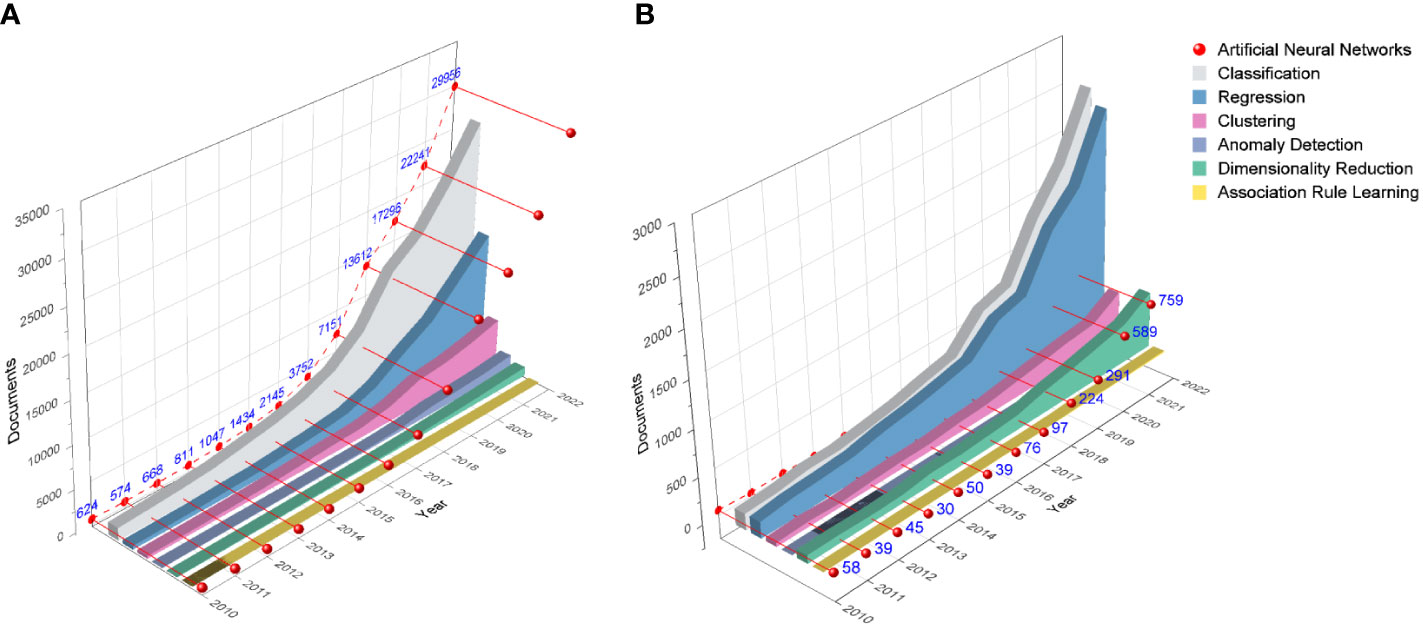
Figure 4 Publications trends (2010 – 2022) of traditional ML algorithms (colored solid areas) and ANNs (dashed red line) in all disciplines (A), and for precision crop protection applications (B), according to the proposed taxonomy (source: Scopus).
These positive indicators on the growing impact of ML in precision crop protection are supported by numerous applications and case studies outlined in detail in quite a few recent scientific reviews (Behmann et al., 2015; Liakos et al., 2018; Muppala and Guruviah, 2020; Chadha et al., 2021; Saleem et al., 2021). An in-depth analysis of some relevant publications reveals key challenges addressed by diverse image-based or sensor technology together with ML algorithms in the specific domains of crop diseases (Table 3), weeds (Table 4) and plagues (Table 5), as discussed hereunder.
In recent literature, one major goal is to study slight alterations in crop spectral information or other sensory components (e.g., odors or flavors) associated with pathogen infestations or with damages caused by a plague attack (Tables 3, 5). This is generally done with on-ground measurements of plant leaves or canopies collected by hyperspectral cameras, field spectroradiometers or other portable sensors (e.g., e-nose sensor), and analyzing the spectral signatures or sensor data with ML classification and/or regression algorithms, aiming to discriminate between healthy and infested plants at the earliest possible stages or to model/predict the spectral response of infested plants. Dimensionality reduction algorithms (e.g., PCA, PLS-DA) is also often applied to transform large datasets into a lower dimensional space to facilitate further analysis. This approach was used at the disease domain, e.g., for early stage classification of anthracnose crown rot disease (by Colletotrichum fungus) in strawberry crop with SDA, FLDA and k-NN algorithms (Lu et al., 2017), classifying pre- and post- symptomatic fungal infestations of late blight (Phytophthora infestans) in potato leaves with PLS-DA and RF algorithms (Gold et al., 2020), monitoring the rate of fungal powdery mildew (Erysiphe graminis) disease in wheat with PLSR, SVR and RFR algorithms (Feng et al., 2022), and pre-symptomatic detection of tobacco mosaic virus in tobacco leaves with PLS-DA, RF, SVM, BPNN, ELM and LS-SVM (Zhu et al., 2017); while at the plague domain was used, e.g., for predicting and classifying oat aphids (Rhophalosiphum padi) number in wheat cultivation with ANNs models applied to NIR and e-nose data (Fuentes et al., 2021), and spectralmodelling of cotton plants against fall armyworm (Spodoptera frugiperda) attacks with RF, XGBoost, Naïve Bayes, LoR, SVM, MLP and k-NN algorithms (Ramos et al., 2022). These tools have also shown effective in other more complex scenarios dealing with hyperspectral discrimination of various diseases or other stresses/deficiencies that may cause similar symptomatology, such as fungal Rhizoctonia root and crown rot (Rhizoctonia solani) diseases in sugar beet leaves with PLS, RF, k-NN, and SVM (Barreto et al., 2020), bacterial spots (Xanthomonas vesicatoria) disease among other fungal diseases (late blight and target) in tomato leaves with PCA and k-NN algorithms (Lu et al., 2018), fungal laurel wilt (Raffaelea lauricola) and Phytophthora root rot diseases in avocado trees with ANN-based MLP and RBF models (De Castro et al., 2015), and laurel wilt disease against N and Fe nutrient deficiencies in avocado leaves with DT and MLP (Abdulridha et al., 2018).
At the domain of weed science (Table 4), field hyperspectral technology have been routinely tested to find the best spectral regions or vegetation indices to discriminate between weeds and crops at different phenological stages (Peña-Barragán et al., 2006; Basinger et al., 2020), generally with the aim of extrapolating results for remote sensing applications (Gómez-Casero et al., 2010; de Castro et al., 2012) in the context of site-specific weed management. Moreover, ML algorithms have recently dealt with challenging issues such as: 1) discrimination of multiple weed species with similar spectral response, such as Barnyard grass (Echinochloa crusgalli) and weedy rice (Oryza sativa) in rice crops with RF, SVM and SPA (Zhang et al., 2019b), Convolvulus arvensis, Rumex, and Cirsium arvense in maize crops with PCA, k-NN and RF (Gao et al., 2018), six Amaranthus species with SVM, DT and Naïve Bayes (Sohn et al., 2021) and Cyperus esculentus clones and morphologically similar weeds with RF, regularized LoR and PLS-DA (Lauwers et al., 2020), or 2) differentiation of herbicide- resistant and susceptible Palmer amaranth (Amaranthus palmeri) plants, Kochia (Kochia scoparia) plants or Johsongrass (Sorghum halepense) plants with MLC and FLDA (Reddy et al., 2014), SVM with RBF kernel (Nugent et al., 2018), and k-NN, RF and SVM with FLDA (Huang et al., 2022), respectively.
Disease, weed and plague detection and mapping with remote sensing have been particularly benefited from the adoption of ML algorithms (de Castro Megías et al., 2021; Lassalle, 2021; Roslim et al., 2021). In this context, proper selection of spectral and spatial image resolutions, as well as the optimal timing, is crucial to achieve satisfactory results (Peña et al., 2015; Khanal et al., 2017), which promotes the use of UAVs or manned aircrafts to the detriment of satellites in precision crop protection. Nonetheless, ML and satellite imagery can be useful in broad-scale applications, e.g., for evaluating integrated bacterial blight disease management in coffee plantations with several ecological variables (Landsat-8 surface reflectance values and VIs, relief morphometry and hydrological attributes) by using RF, SVM and Naïve Bayes (de Carvalho Alves et al., 2022), or for mapping cruciferous weed patches in multiple winter wheat fields with QuickBird satellite imagery by using MLC (de Castro et al., 2013). Thermal and hyper-spectral aerial images with capability to capture slight variations in crop temperature and in narrow spectral bands associated to certain physiological indicators, respectively are commonly used in early detection of crop diseases, such as for identifying bacterial Huanglongbing (HLB) disease in citrus trees with stepwise regression, SVM, LDA and QDA (Garcia-Ruiz et al., 2013), fungal Verticillium wilt (Verticillium dahlia) disease in olive trees with LDA and SVM (Calderón et al., 2015), bacterial Xylella fastidiosa infections in olive trees (Zarco-Tejada et al., 2018), and fungal yellow rust (Puccinia striiformis) across crop cycle in wheat with RF and CNN-based Inception-ResNet blocks (Zhang et al., 2019a). SVM with a Gaussian kernel and RF algorithms also helped to diminish the uncertainty of distinguishing trees affected by diverse biotic (i.e., infections by Xylella fastidiosa and Verticillium dahlia pathogens) and abiotic (i.e., water status) stressors that produce analogous symptoms on spectral traits in olive and almond orchards (Zarco-Tejada et al., 2021).
Most recent research in precision crop protection relies on analyzing UAV images collected with low-cost RGB cameras or multispectral imaging systems, which compromise image spectral resolution in favor of much higher spatial resolution. This ultra-high spatial resolution is particularly relevant to detect very small weed seedlings in their earliest stages, which is generally the optimal time for implementing SSWM strategies. In these scenarios, ML algorithms tackled previously unsolved challenging tasks such as: 1) distinguishing weeds outside and inside crop rows with k-NN, SVM or k-means clustering in sunflower (Pérez-Ortiz et al., 2015) and in maize (Pérez-Ortiz et al., 2016), or with an ensemble of RF trees in sunflower and cotton (De Castro et al., 2018); 2) discriminating between broad-leaved and grass weeds in sunflower and cotton by using ANN-based MLP (Torres-Sánchez et al., 2021); 3) mapping bermudagrass patches in vineyards with cover crops by using DT (de Castro et al., 2020); and 4) spectral analysis and mapping of blackgrass weed in wheat parcels by using feature selection and RF with Bayesian optimization, respectively (Su et al., 2022). In the domains of crop diseases and plagues, relevant studies with UAV multispectral imagery are mainly focused on classifying crop/tree area damaged by a disease infestation or a plague attack, e.g., detecting bacterial fire blight (Erwinia amylovora) disease in apple or in pear trees with a combination of dimensionality reduction (mRMR), anomaly detection (isolation forest) and classification (DT, RF, SVM) algorithms (Xiao et al., 2022), or by using SVM classifier with RBF (Bagheri, 2020), respectively, discriminating bacterial (banana xanthomonas wilt - BXW) and viral (banana bunchy top virus - BBTV) diseases in banana plantations with the RetinaNet model based on the ResNet50 architecture as detector and the VGG16 architecture pre-trained with the ImageNet dataset as classifier (Selvaraj et al., 2020), and classifying cotton pixels affected by two-spotted spider mite attacks with the CNN-based AlexNet algorithm (Huang et al., 2018).
Advances in CNN algorithms have greatly promoted the use of field imaging systems and proximal sensing for precision crop protection applications in the last years, as a tool to improve classification accuracy in complex crop/pest scenarios (Barbedo, 2020) and to implement real-time applications (Rakhmatulin et al., 2021). In fact, recent innovations in agricultural robotics and weeding systems are based on CNN classifiers for pest detection and classification (Oberti and Schmilovitch, 2021; Allmendinger et al., 2022; Gerhards et al., 2022; Li et al., 2022). Some recent studies in the weed domain are the classification of Chenopodium album in potato fields by comparing CNN-based GoogLeNet, VGG-16 and EfficientNet (Hussain et al., 2021) and of five different weed species in tomato fields with CNN-based RetinaNet, Faster RCNN and YOLOv7 (López-Correa et al., 2022), in the disease domain are the early detection of fungal head blight (Fusarium) disease in wheat by applying CNN-based VGG and RNN classifiers to on-ground hyperspectral images (Jin et al., 2018) and diagnosing of fungal black rot, black measles (esca) and leaf blight diseases by applying CNN-based AlexNet, MobileNets and ShuffleNet to a repository of RGB images of grape leaves for potential use in mobile devices (Tang et al., 2020), while in the plague domain are the detection and classification of multi-class plague species in trap images by using CNN-based ZF, VGG16, ResNet50 and ResNet101 (Liu et al., 2019) and the detection of Helicoverpa assulta, Spodoptera litura and Spodoptera exigua moths in pheromone trap images by comparing Faster RCNN, R-FCN ResNet, Retinanet and SSD Inception classifiers (Hong et al., 2020), among many other case studies in the three crop protection domains.
5 Emerging technologies of precision crop protection in line with AG5.0
Crop protection has used technology to reinvent itself over time, with AI tools and ML algorithms being the main drivers in the last decade towards the implementation of automated, smart and precise tasks following the precision agriculture and digital Ag4.0 paradigms. While AI involves the scientific and technological research of machines that are able to perceive, reason, learn, adapt, make decisions and act rationally to meet objectives in a given environment, the advances in ML are behind the recent rise of AI in primary, industrial and service sectors. As discussed above, many of the ML algorithms have already been successfully applied in agriculture and other disciplines (Table 2), while others unprecedented in agriculture are now reaching the level of maturity needed to address new precision crop protection goals in line with emerging Ag5.0.
These goals will primarily focus on developing and exploiting two issues: 1) early detection of crop pests, and 2) autonomous real-time multitasking systems. On the one side, the former will enable the application of more effective control measurements at the optimal time before the damage provoked by a disease, weed or plague becomes too severe. The development of data-driven early detectors is particularly urgent considering the adverse effects that current climate change scenario are causing on cropping systems due to the spread of newly emerging or invasive pests (Juroszek et al., 2020; IPPC Secretariat, 2021). To this end, the implementation of Ag5.0 technologies will facilitate data fusion from various sources and tools (e.g. climate data, proximal and remote sensing, crop and soil sensors, farm management information systems, etc.) and assess the spatio-temporal occurrence and severity of the pests (Shankar et al., 2020), which will lead to improve early detectors and diagnostic algorithms (Picon et al., 2019; Ramcharan et al., 2019). On the other side, the latter aims the design of powerful autonomous systems capable of simultaneously doing the three main stages of precision crop protection in real time (see section 2), i.e. identifying occurrences of crop diseases, weeds or plagues at different spatial and temporal scales, analyzing crop and pest information, and make the decision of applying a customized site-specific management adjusted to each crop-pest scenario (Pretto et al., 2019; Birrell et al., 2020; Lottes et al., 2020).
Ag5.0 technology will tackle these and other future challenges with a multidisciplinary domain that relies on powerful ML algorithms (Liakos et al., 2018; Coulibaly et al., 2022), along with the latest technological solutions on hardware (Bustio-Martínez et al., 2022), telecommunications (Chopra et al., 2017; Ejaz et al., 2020), and robotics (Ren et al., 2020; Albiero et al., 2022), which may contribute now or in the short, medium or long term given their different degrees of maturity and use (Figure 5), as discussed below.
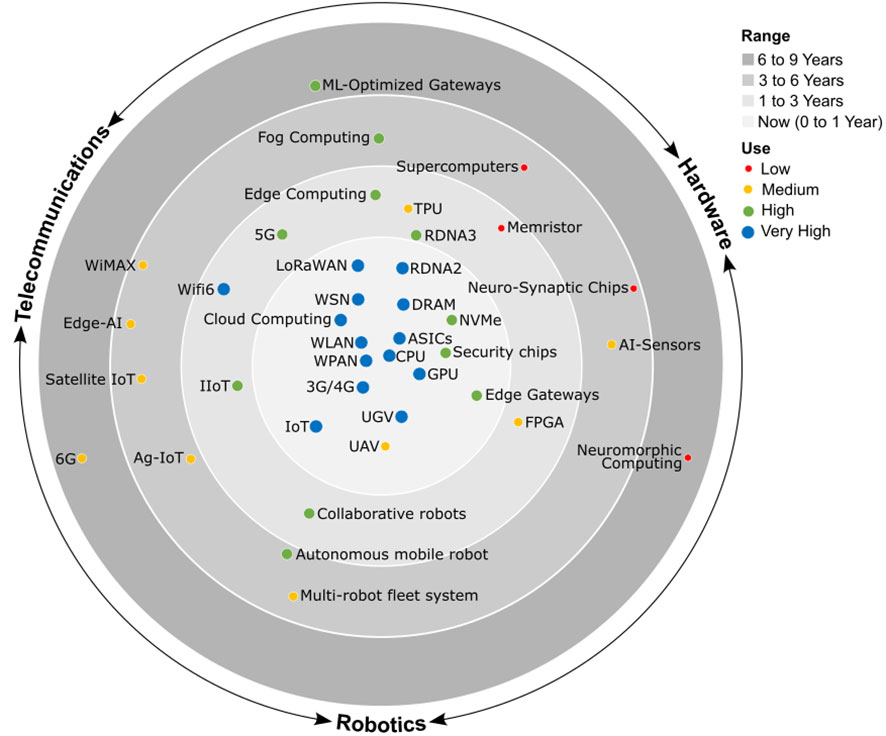
Figure 5 Multidisciplinary technological domain of Ag5.0 with a different degree of maturity and use ranging from mature technologies in the core circle to future technologies in the peripheral circle. CPU, central processing unit; GPU, graphics processing unit; TPU, tensor processing unit; DRAM, dynamic random-access memory; RDNA, radeon DNA; NVMe, non-volatile memory express; ASIC, application specific integrated circuit; FPGA, field programable gate array; LPWAN, low power wide area network; WLAN, wireless local area network; WPAN, wireless personal area network; WSN, wireless sensor network; IoT, internet of things; IIoT, industrial IoT; Ag-IoT, agricultural IoT; LiFi, light fidelity; WiMAX, worldwide interoperability for microwave access; TSN, time-sensitive networking; xG, cellular network generation.
5.1 Hardware solutions for precision crop protection
Hardware tools are moving agriculture disciplines into digitization with innovative smart sensors, IoT ecosystems, architectures for specialized graphics processing, multicore embedded systems, and a number of new electronic devices, focused on the acquisition and use of crop data (Muhammad et al., 2019). The convergence of technologies is enabling to turn traditional agricultural sensors into smart sensors with built-in AI processing, that is, AI-Sensors with a dedicated chip embedded in the same sensor that can process ML tasks and, for example, may simultaneously perform object perception and analysis. Sony IMX500 and IMX501 (Sony Group Corporation, Tokyo, Japan) are two commercial image-based AI-sensors (Sony, 2020), in which the acquired signals are executed with a digital image signal processor at high-speed by the logic chip (i.e., 3.1 millisecond processing by the MobileNet V1). This processing speed is feasible as the sensor generates semantic information belonging to the image metadata instead of the image information, reducing data volume. In crop protection, these AI-sensors would facilitate the detection, recognition and control of targeting areas of crops with specific pest problems in real-time (e.g., weed species identification) and following optimized DSS prescriptions.
Advances in architectures for specialized graphics processing, such as GPU, TPU, radeon DNA (RDNA), in dynamic random-access memories (DRAM) and in communication and storage access protocols (e.g., non-volatile memory express, NVMe) are enabling greater programmability, opening up a wide range of Ag5.0 applications based on virtual modeling, the creation of digital twins and the use of supercomputers. A digital twin is a multi-physics, multi-scale, probabilistic simulation of a complex system that uses the best available physical models and sensor updates to reflect the life of its corresponding twin (Glaessgen and Stargel, 2012). While simulation-based analysis within a digital twin will lead to the development of innovative and more powerful DSS tools for precise pest management, the use of supercomputers will enable the study of crop-pest models in less time and drastically improve the performance of ML detectors and classifiers of crop diseases, weeds or plagues. Currently, the bottleneck to implement CNN-based architectures with high capacity for knowledge generalization is the training stage with large training datasets, but supercomputers will assist in overcoming this weakness by increasing the input data (data augmentation) and decreasing the computational time for model creation.
Performance of CNN-based architectures can be also improved with the use of Field Programmable Gate Array (FPGA), which enables the implementation of logic functions and is the basis for the creation of multicore embedded systems (Qiu et al., 2016; Shawahna et al., 2019). This technology will benefit precision crop protection with the development of new software applications running in operating systems used in agriculture IoT (Ag-IoT) solutions (Zhang et al., 2020) and the adaptation of customized pest detectors to mobile devices.
The devices connected to IoT systems are potentially risky in the absence of security elements or algorithms (Ibrahim and Gebali, 2022), reason why the devices performing edge gateway functions have improved designs with the use of application-specific integrated circuits (ASICs), just as security chips are essential in the implementation of Industrial IoT (IIoT) (Oñate and Sanz, 2023) and Ag-IoT systems. Wide evolution and adaptability of ML algorithms lead to their employ in optimizing gateway equipment tasks (ML-Optimized Gateways), making the performance of these tasks efficient even with resource limitations. The use of ML-Optimized Gateways in Ag5.0 will allow optimizing edge computing devices, reduce latency and increase privacy, which will result to create more efficient and safe models.
The future of both hardware and software solutions may reach a turning point in the medium term with the application of the computing principles derived from the memristors (Strukov et al., 2008). These devices are composed of two terminals with three layers, i.e. two electrodes for the communication of electrical signals and one storage layer that can be dynamically reconfigured when the inputs are stimulated, enabling data storage and direct processing (Zidan et al., 2018). The functioning of memristive elements is similar to that of neuronal synapses, becoming the technological basis of neuromorphic computing (Xia and Yang, 2019) and Spiking Neural Networks (SNNs) research (Jeong and Shi, 2019), which relies on a new neuron that is characterized by having a time-varying internal state, known as spiking neuron (Brette et al., 2007; Ghosh-Dastidar and Adeli, 2009). SNNs are the artificial representation that most closely emulate the brain, differing from ANNs in the incorporation of time as an explicit dependency in computations (Davies et al., 2018). Comparing to ANNs, SNNs achieve lower latency classifications, shorter computation times in the training phase, high accuracies and low energy consumption (Diehl et al., 2015; Esser et al., 2016), which can foresee that neuromorphic computing and SNNs will be the future tools to develop computational systems and create new electronic devices with a high impact on Ag5.0 technology.
5.2 Telecommunications for precision crop protection
Precision crop protection is increasingly heading towards a system-of-systems approach with multiple connected practices to achieve an integrated crop management strategy, in which on-ground, proximal and remote sensing are key technologies to assess and monitor all the biotic and abiotic factors that might affect crop health. In this framework, telecommunications are essential to connect devices (i.e., platforms, processors, actuators) and transfer data acquired by sensors, creating a networking environment that adds value in the tasks of data processing, pest prediction, decision-making, and crop management.
Wireless Sensor Networks (WSN) are leading communication systems in agriculture with various technologies that differ from each other mainly in their operating mode and specifications in terms of frequency range, transfer rate and power consumption (Thakur et al., 2019). Bluetooth and Zigbee (developed under IEEE 802.15.1 and 802.15.4 standards, respectively) are characterized by open specification, short range operation, high level data transmission with low latencies and low power consumption (Khanji et al., 2019; Zeadally et al., 2019). Zigbee covers larger distance (<100 m) than Bluetooth (<10 m), although data transfer is faster in Bluetooth (1-24 Mbps) than in Zigbee (40-240 Kbps). The alternatives to increase the range of operation and data transfer are the wireless fidelity (Wi-Fi) system, generally used for local area networks with a range of 50-100 m or even several hundred meters, and the worldwide inter-operability for microwave access (WiMAX) system used as a long-distance communication solution (up to 50 km). The development of IoT and the advance of low power wide area networks (LPWAN) are promoting the Long Range (LoRa) radio communication system and the LoRaWAN protocol as the most promising technology in agricultural disciplines (Castro et al., 2023), because of its long-range data transmission (dozens of kilometers, very useful in rural areas), low power consumption and secure connectivity (Gu et al., 2020). LoRaWAN uses a modified frequency modulation, operates in the Industrial, Scientific and Medical frequency band defined according to the geographical area (Asia 433 MHz, Europe 868 MHz and America 915 MHz), hence the sensors can operate in the license-free bandwidth (Lavric, 2019).
High-speed and efficient telecommunications are essential to implement real-time operations in actuator platforms (i.e., tractors, self-propelled sprayers, unmanned ground vehicles (UGVs), UAVs, etc.) that are focused to simultaneously percept, analysis and treat pest occurrences. In engineering and computer science, the concept of real-time is given to those processes whose execution, measured as the ratio between the input and output of a variable, occurs at very low time values (<milliseconds), therebeing a difference between real-time system and real-time computer system (Poniszewska-Maranda et al., 2020). Cloud computing, edge computing and edge AI are the three technologies to implement real-time actions on actuator platforms for precision crop protection in line with Ag5.0
Cloud computing is the convergence of information technology and business activity to provide services over the Internet. Companies such as Amazon, Google and Microsoft compete in the continuous improvement of infrastructures, hardware, computer security and high information processing (Mahmoud and Xia, 2019). To perform precision crop protection operations in real-time using cloud computing, the information collected with a sensorized platform must first be transmitted to the Internet, then processed and analyzed on any ML-based cloud service, and finally the prescription returned to the same platform to implement the actuation. These interactive operations need access times as short as possible, very close to real-time, to meet users’ demands, for which network architectures for wireless connections enabling Internet access such as 5G are already underway, with a view to the upcoming development of 6G. For example, the integration of 5G and future 6G with UAVs has enormous potential to apply precise aerial treatments of weed patches and eventually other pest occurrences following real-time detection (Ullah et al., 2020). Technical aspects aside, security and privacy issues are of particular concern in cloud computing systems, as infrastructures and applications may be subject to malicious attacks, as reported by Maniah et al. (2019) and Sun (2020). Indeed, privacy-sensitive reasons together with the progressive increase in data volume due to the connection of more devices has led to the introduction of fog computing, which allows decentralized processing, low latency and high bandwidth (Bonomi et al., 2012).
As mentioned before, current research is focused to platforms that detect, process and treat at the same time, which require a high computational cost in the limiting conditions of an equipment located on the farm, using the encoding method for signal transmission and taking into account the latency time of the radio transmission equipment. In this scenario, edge computing systems is a viable option as they allow the computing process to be performed close to the data source without the need for an Internet connection, thus avoiding data transmission problems and providing superior privacy and security, as well as reducing communication costs and energy consumption given the huge number of computations performed in ML modeling (García-Valls et al., 2018). A further step in the development of this computational architecture is offered by the devices for AI on the edge (Edge AI), which are embedded systems equipped with ML algorithms. Edge Intelligence is still at an early stage of research (Zhou et al., 2019), but is attracting great interest across all technological disciplines, with enormous potential in the development of agricultural robotics and autonomous crop protection treatments, since AI chips have achieved a high calculation capacity in the implementation of CNNs (Gao and Zhou, 2019).
5.3 Robotics for precision crop protection
Autonomous mobile robots (AMRs) allows the industry to increase productivity by doing more with fewer people, having great potential for boosting precision crop protection strategies in line with Ag5.0. AMRs have the ability to navigate with little or no human intervention under their control, in partially unknown environments (Alatise and Hancke, 2020). Therefore, their locomotion, perception, cognition and navigation systems must be able to address dynamic crops in position and time; in addition to: i) providing solutions to labor shortages, and ii) acquire real-time data for data-driven decision making, with the aim of significantly increasing yields within sustainable production (Shamshiri et al., 2018).
Several research projects have been developed to link robotic platforms to agricultural activities (Wolfert et al., 2017). In order to have completely robotized agricultural fields, robots must be able to adapt to the external environment and to the different types of land surface. Due to the great technological advances implemented in recent years, some robotic agricultural activities are already becoming commercially available (Lowenberg-DeBoer et al., 2020; Saiz-Rubio and Rovira-Más, 2020; Santos Valle and Kienzle, 2020; Sparrow and Howard, 2021; Botta et al., 2022), being the use of UGVs and UAVs that detect weeds and act in real-time with high precision the most popular robotic system to implement a precision crop protection activity (Oberti and Schmilovitch, 2021; Li et al., 2022). Some AMRs with great potential are: 1) RIPPA (Australian Centre for Field Robotics, The University of Sydney, Austria), based on the design of their previous robot LADYBIRD, uses an intelligent perception system and is equipped with a variable injection precision applicator, with an operating autonomy of twenty one continuous hours (Bogue, 2016); 2) AgBot-II (Queensland University of Technology, Brisbane, Australia) with a vision system not only detecting but also classifying weed species in real time, then using the Inception-v3 architecture as its DDS, which allows to decide the weed management method to apply, either mechanical, chemical or a combination of both, weeds on accuracies over 90% (McCool et al., 2018); 3) Robotti (Agrointelli, Aarhus, Denmark), whose module-based construction allows it to operate in various soil environments, adapting to different types of crops (Grimstad and From, 2017); 4) AVO (Ecorobotix, Yverdon, Switzerland) that uses CNNs algorithms for the detection and selective control of weeds by herbicide spraying in real time, obtaining a detection rate of 85% (https://ecorobotix.com/en/avo/); 5) BONIROB (AMAZONE Technology Leeden GmbH & Co. KG, Germany) (https://info.amazone.de/DisplayInfo.aspx?id=29417) with an integrated system using camera-based machine vision, image processing to detect the plants and a sprayer with individually controlled valves, allows selective and precise control of weeds, thus achieving both ecological and economic advantages; 6) Kilter AX1 (Kilter AS, Norway) (https://www.kiltersystems.com/ax1) uses machine vision combined with AI and a novel nozzle technology that applies a micro-drop (6×6mm resolution), which allows to reduce the amount of herbicides up to 95%; 7) DINO (Naïo Technologies, France) (https://www.naio-technologies.com/en/dino/), a weeding robot with an accuracy of 2 cm achieved by the RTK GPS system that has a vision system to detect the crop rows and adjust the position of the mechanical weeding tools in row, allowing high precision weeding and hoeing; 8) Odd.bot (Odd.Bot B.V., The Netherlands) (https://www.odd.bot/), a mechanical in-row weeding robot that relies on machine vision and AI-based seedling recognition; 9) Titan FT35 (FarmWise Labs Inc., USA) (https://farmwise.io/) uses machine vision and ML algorithms trained to learn the characteristics of crops such as broccoli, lettuce, cauliflower and tomatoes to differentiate between the crop and weeds; it has six internal weeders with blades that eliminate weeds with centimeter accuracy; and 10) FARMING GT (Farming revolution GmbH, Germany) (https://farming-revolution.com/) distinguishes weed seedlings with 99% reliability in different crops (e.g., cabbage, lettuce varieties, onions, corn, sugar beet, pumpkin, field bean, potato, canola, soybean, wheat), then carrying out in-row and inter-row mechanical weeding.
Collaborative or cooperative robots will support the future development of Ag5.0 (Lytridis et al., 2021). These robots are designed to complement the routine activities by improving their ergonomics (Pauline et al., 2019) and also sharing the workspace. An advanced application of collaborative robots is in organic food production, particularly in pest control with nonchemical methods by using robotic mechanical control (Machleb et al., 2020) and viable handling systems for harvesting (De-An et al., 2011; Zhang et al., 2021), which has been shown as a solution to increase the benefits of organic crop management (Pérez-Ruíz et al., 2014; Giampieri et al., 2022). The development of Ag5.0 will allow the convergence of UGV and UAV systems, for their collaborative and cooperative operation under a unified control, giving rise to Multi-robot Fleet Systems (MFS). Workload performed by several small robots composing a MFS is equivalent to that developed by a larger machine, highlighting that the MFS have a more precise positioning (de Santos et al., 2017).
Current technology has allowed the development and maturation of sensory-motor autonomy, reactive autonomy and cognitive autonomy in UAVs (Floreano and Wood, 2015), making them a great tool that together with RGB, multispectral, and hyperspectral sensors facilitate the acquisition of information on plant diseases, weeds, and plagues. That is why in Ag5. 0, detection and actuation systems based on ML algorithms and implemented in embedded systems will be part of the UAVs. ML techniques within Ag5.0 will allow the integral management of fleets of autonomous vehicles (UAV and UGV) decentralized in real time, besides being the basis for the implementation of robust navigation systems, such as the redundant system developed by (Belhajem et al., 2016) where they used ANNs in conjunction with genetic algorithms and the Extended Kalman Filter to reliably estimate the position of a vehicle in real time in the absence of GPS signal. The objective of having fleets of autonomous vehicles is the application of specific treatments for the detection and action on weeds and others pests (Emmi et al., 2014), which will finally reduce production costs and reduce the environmental impact of the use of herbicides and pesticides.
6 Conclusions
This article provides a framework on the future direction of precision crop protection, with a focus to scientific, agronomic and industrial applications of traditional ML algorithms and recent advances in the ANNs models. In the period 2010-2022, 125 algorithms applied in all disciplines were identified, of which 122 were used in the domains of crop diseases, weeds and plagues, with the aims of solving tasks on classification, regression, clustering, anomaly detection, dimensionality reduction, and association rule learning, and moving precision crop protection closer to the emerging concept of Ag5.0. This process should be accompanied by innovations and dedicated solutions in the areas of hardware, telecommunications and robotics, some of which are already being implemented in agriculture and others are still unprecedented, as this article outlines by introducing 39 emerging technologies and citing some 80 scientific and technical references. The transition from current Ag4.0 to future Ag5.0 strategies in the field of precision crop protection will be driven mainly by their focus and level of automation. Ag5.0 will promote a new era of intelligent crop management with a greater emphasis on solving complex crop protection objectives (e.g. early detection of crop pests) and enhancing management practices (e.g. autonomous real-time multitasking) as a whole, with a main focus to automatized decision-making processes, unmanned operations and progressively less human intervention supported by the latest AI systems, advanced robotics, and powerful ML algorithms.
Author contributions
MP-O, JD, and JP conceived and designed the review; GM-R conducted the bibliographic search with support of JD, AC, and JP; GM-R, and MP-O analyzed the data and defined the ML taxonomy; JD, AC, and JP selected the case studies cited; GM-R and JP identified the emerging technologies; GM-R, JD, and JP wrote the first draft. All authors contributed to the article and approved the submitted version.
Funding
This work was supported by the Spanish Research State Agency (AEI) through the Projects PDC2021-121537-C22/AEI/10.13039/501100011033 and PID2020-113229RB-C41. The lead author GM-R has been a beneficiary of a FPI fellowship by the Spanish Ministry of Education and Professional Training (PRE2018-083227).
Conflict of interest
The authors declare that the research was conducted in the absence of any commercial or financial relationships that could be construed as a potential conflict of interest.
Publisher’s note
All claims expressed in this article are solely those of the authors and do not necessarily represent those of their affiliated organizations, or those of the publisher, the editors and the reviewers. Any product that may be evaluated in this article, or claim that may be made by its manufacturer, is not guaranteed or endorsed by the publisher.
References
Abdulridha, J., Ampatzidis, Y., Ehsani, R., de Castro, A. I. (2018). Evaluating the performance of spectral features and multivariate analysis tools to detect laurel wilt disease and nutritional deficiency in avocado. Comput. Electron. Agric. 155, 203–211. doi: 10.1016/j.compag.2018.10.016
Alatise, M. B., Hancke, G. P. (2020). A review on challenges of autonomous mobile robot and sensor fusion methods. IEEE Access 8, 39830–39846. doi: 10.1109/ACCESS.2020.2975643
Albiero, D., Garcia, A. P., Umezu, C. K., de Paulo, R. L. (2022). Swarm robots in mechanized agricultural operations: A review about challenges for research. Comput. Electron. Agric. 193, 106608. doi: 10.1016/j.compag.2021.106608
Allmendinger, A., Spaeth, M., Saile, M., Peteinatos, G. G., Gerhards, R. (2022). Precision chemical weed management strategies: A review and a design of a new CNN-based modular spot sprayer. Agronomy 12, 1620. doi: 10.3390/agronomy12071620
Bagheri, N. (2020). Application of aerial remote sensing technology for detection of fire blight infected pear trees. Comput. Electron. Agric. 168, 105147. doi: 10.1016/j.compag.2019.105147
Barbedo, J. G. A. (2020). Detecting and classifying pests in crops using proximal images and machine learning: A review. AI 1, 312–328. doi: 10.3390/ai1020021
Barreto, A., Paulus, S., Varrelmann, M., Mahlein, A.-K. (2020). Hyperspectral imaging of symptoms induced by Rhizoctonia solani in sugar beet: comparison of input data and different machine learning algorithms. J. Plant Dis. Prot. 127, 441–451. doi: 10.1007/s41348-020-00344-8
Basinger, N. T., Jennings, K. M., Hestir, E. L., Monks, D. W., Jordan, D. L., Everman, W. J. (2020). Phenology affects differentiation of crop and weed species using hyperspectral remote sensing. Weed Technol. 34, 897–908. doi: 10.1017/wet.2020.92
Behmann, J., Mahlein, A.-K., Rumpf, T., Römer, C., Plümer, L. (2015). A review of advanced machine learning methods for the detection of biotic stress in precision crop protection. Precis. Agric. 16, 239–260. doi: 10.1007/s11119-014-9372-7
Belhajem, I., Maissa, Y. B., Tamtaoui, A. (2016). “A robust low cost approach for real time car positioning in a smart city using extended kalman filter and evolutionary machine learning,” in 4th IEEE International Colloquium on Information Science and Technology (CiSt). (Tangier-Assilah, Morocco: IEEE), 806–811.
Birrell, S., Hughes, J., Cai, J. Y., Iida, F. (2020). A field-tested robotic harvesting system for iceberg lettuce. J. Field Robot. 37, 225–245. doi: 10.1002/rob.21888
Bogue, R. (2016). Robots poised to revolutionise agriculture. Ind. Robot: Int. J. 43, 450–456. doi: 10.1108/IR-05-2016-0142
Bonomi, F., Milito, R., Zhu, J., Addepalli, S. (2012). “Fog computing and its role in the internet of things,” in Proceedings of the first edition of the MCC workshop on Mobile cloud computing. (Helsinki, Finland: Association for Computing Machinery) 13–16.
Botta, A., Cavallone, P., Baglieri, L., Colucci, G., Tagliavini, L., Quaglia, G. (2022). A review of robots, perception, and tasks in precision agriculture. Appl. Mech. 3, 830–854. doi: 10.3390/applmech3030049
Brette, R., Rudolph, M., Carnevale, T., Hines, M., Beeman, D., Bower, J. M., et al. (2007). Simulation of networks of spiking neurons: A review of tools and strategies. J. Comput. Neurosci. 23, 349–398. doi: 10.1007/s10827-007-0038-6
Bustio-Martínez, L., Cumplido, R., Letras, M., Hernández-León, R., Feregrino-Uribe, C., Hernández-Palancar, J. (2022). FPGA/GPU-based acceleration for frequent itemsets mining: A comprehensive review. ACM Comput. Surv. 54, 1–35. doi: 10.1145/3472289
Calderón, R., Navas-Cortés, J. A., Zarco-Tejada, P. J. (2015). Early detection and quantification of verticillium wilt in olive using hyperspectral and thermal imagery over large areas. Remote Sens. 7, 5584–5610. doi: 10.3390/rs70505584
Castro, S., Iñacasha, J., Mesias, G., Oñate, W. (2023). “Prototype based on a LoRaWAN network for storing multivariable data, oriented to agriculture with limited resources,” in Proceedings of seventh international congress on information and communication technology. Eds. Yang, X.-S., Sherratt, S., Dey, N., Joshi, A. (London, England: Springer Nature Singapore), 245–255.
Chadha, S., Sharma, M., Sayyed, A. (2021). “Chapter 12 - advances in sensing plant diseases by imaging and machine learning methods for precision crop protection,” in Microbial management of plant stresses. Eds. Kumar, A., Droby, S. (Woodhead Publishing), 157–183.
Chandola, V., Banerjee, A., Kumar, V. (2009). Anomaly detection: A survey. ACM Comput. Surv. 41, 15:1–15:58. doi: 10.1145/1541880.1541882
Chhikara, P., Jain, N., Tekchandani, R., Kumar, N. (2020). Data dimensionality reduction techniques for industry 4.0: Research results, challenges, and future research directions. Software: Practice and Experience 52, 658–688. doi: 10.1002/spe.2876
Chollet, F. (2016). Xception: Deep learning with depthwise separable convolutions. Proceedings of the IEEE Conference on Computer Vision and Pattern Recognition (CVPR). Las Vegas, NV, USA. 1251–1258. doi: 10.48550/ARXIV.1610.02357
Chopra, G., Kumar Jha, R., Jain, S. (2017). A survey on ultra-dense network and emerging technologies: Security challenges and possible solutions. J. Netw. Comput. Appl. 95, 54–78. doi: 10.1016/j.jnca.2017.07.007
Čížek, P., Sadikoğlu, S. (2020). Robust nonparametric regression: A review. Wiley Interdiscip. Reviews: Comput. Stat 12, e1492. doi: 10.1002/wics.1492
Coulibaly, S., Kamsu-Foguem, B., Kamissoko, D., Traore, D. (2022). Deep learning for precision agriculture: A bibliometric analysis. Intelligent Syst. Appl. 16, 200102. doi: 10.1016/j.iswa.2022.200102
Dainelli, R., Toscano, P., Di Gennaro, S. F., Matese, A. (2021). Recent advances in unmanned aerial vehicles forest remote sensing–a systematic review. part II: Research applications. Forests 12, 397. doi: 10.3390/f12040397
Davies, M., Srinivasa, N., Lin, T.-H., Chinya, G., Cao, Y., Choday, S. H., et al. (2018). Loihi: A neuromorphic manycore processor with on-chip learning. IEEE Micro 38, 82–99. doi: 10.1109/MM.2018.112130359
De-An, Z., Jidong, L., Wei, J., Ying, Z., Yu, C. (2011). Design and control of an apple harvesting robot. Biosyst. Eng. 110, 112–122. doi: 10.1016/j.biosystemseng.2011.07.005
de Carvalho Alves, M., Pozza, E. A., Sanches, L., Belan, L. L., de Oliveira Freitas, M. L. (2022). Insights for improving bacterial blight management in coffee field using spatial big data and machine learning. Trop. Plant Pathol. 47, 118–139. doi: 10.1007/s40858-021-00474-w
De Castro, A. I., Ehsani, R., Ploetz, R., Crane, J. H., Abdulridha, J. (2015). Optimum spectral and geometric parameters for early detection of laurel wilt disease in avocado. Remote Sens. Environ. 171, 33–44. doi: 10.1016/j.rse.2015.09.011
de Castro, A.-I., Jurado-Expósito, M., Gómez-Casero, M.-T., López-Granados, F. (2012). Applying neural networks to hyperspectral and multispectral field data for discrimination of cruciferous weeds in winter crops. Sci. World J. 2012, e630390. doi: 10.1100/2012/630390
de Castro, A. I., López-Granados, F., Jurado-Expósito, M. (2013). Broad-scale cruciferous weed patch classification in winter wheat using quickbird imagery for in-season site-specific control. Precis. Agric. 14, 392–413. doi: 10.1007/s11119-013-9304-y
de Castro, A. I., Peña, J. M., Torres-Sánchez, J., Jiménez-Brenes, F. M., Valencia-Gredilla, F., Recasens, J., et al. (2020). Mapping Cynodon dactylon infesting cover crops with an automatic decision tree-OBIA procedure and UAV imagery for precision viticulture. Remote Sens. 12, 56. doi: 10.3390/rs12010056
De Castro, A. I., Torres-Sánchez, J., Peña, J. M., Jiménez-Brenes, F. M., Csillik, O., López-Granados, F. (2018). An automatic random forest-OBIA algorithm for early weed mapping between and within crop rows using uav imagery. Remote Sens. 10, 285. doi: 10.3390/rs10020285
de Castro Megías, A. I., Pérez-Roncal, C., Thomasson, J. A., Ehsani, R., López-Maestresalas, A., Yang, C., et al. (2021). “Applications of sensing for disease detection,” in Sensing approaches for precision agriculture. Eds. Kerry, R., Escolã, A. (Cham: Springer International Publishing), 369–398.
de Santos, P. G., Ribeiro, A., Fernandez-Quintanilla, C., Lopez-Granados, F., Brandstoetter, M., Tomic, S., et al. (2017). Fleets of robots for environmentally-safe pest control in agriculture. Precis. Agric. 18, 574–614. doi: 10.1007/s11119-016-9476-3
Diehl, P. U., Neil, D., Binas, J., Cook, M., Liu, S.-C., Pfeiffer, M. (2015). “Fast-classifying, high-accuracy spiking deep networks through weight and threshold balancing,” in 2015 International Joint Conference on Neural Networks (IJCNN). (Killarney, Ireland: IEEE).
Djafri, L., Gafour, Y. (2022). “Machine learning algorithms for big data mining processing: A review,” in Artificial intelligence and its applications. Eds. Lejdel, B., Clementini, E., Alarabi, L. (Springer International Publishing), 43–55.
Durmuş, H., Güneş, E. O., Kirci, M. (2017). “Disease detection on the leaves of the tomato plants by using deep learning,” in 2017 6th International Conference on Agro-Geoinformatics. (Fairfax VA, USA: IEEE) 1–5.
Ebrahimi, M., Khoshtaghaza, M., Minaei, S., Jamshidi, B. (2017). Vision-based pest detection based on SVM classification method. Comput. Electron. Agric. 137, 52–58. doi: 10.1016/j.compag.2017.03.016
Ejaz, W., Basharat, M., Saadat, S., Khattak, A. M., Naeem, M., Anpalagan, A. (2020). Learning paradigms for communication and computing technologies in IoT systems. Comput. Commun. 153, 11–25. doi: 10.1016/j.comcom.2020.01.043
Emmi, L., de Soto, M. G., Pajares, G., de Santos, P. G. (2014). New trends in robotics for agriculture: Integration and assessment of a real fleet of robots. Sci. World J. 2014, 404059. doi: 10.1155/2014/404059
Esser, S. K., Merolla, P. A., Arthur, J. V., Cassidy, A. S., Appuswamy, R., Andreopoulos, A., et al. (2016). Convolutional networks for fast, energy-efficient neuromorphic computing. Proc. Natl. Acad. Sci. 113, 11441–11446. doi: 10.1073/pnas.1604850113
Ezugwu, A. E., Ikotun, A. M., Oyelade, O. O., Abualigah, L., Agushaka, J. O., Eke, C. I., et al. (2022). A comprehensive survey of clustering algorithms: State-of-the-art machine learning applications, taxonomy, challenges, and future research prospects. Eng. Appl. Artif. Intell. 110, 104743. doi: 10.1016/j.engappai.2022.104743
Falkland, T., White, I. (2020). “Freshwater availability under climate change,” in Climate change and impacts in the pacific. Ed. Kumar, L. (Springer International Publishing), 403–448.
Farooq, A., Hu, J., Jia, X. (2019). Analysis of spectral bands and spatial resolutions for weed classification Via deep convolutional neural network. IEEE Geosci. Remote Sens. Lett. 16, 183–187. doi: 10.1109/LGRS.2018.2869879
Feng, Z.-H., Wang, L.-Y., Yang, Z.-Q., Zhang, Y.-Y., Li, X., Song, L., et al. (2022). Hyperspectral monitoring of powdery mildew disease severity in wheat based on machine learning. Front. Plant Sci. 13. doi: 10.3389/fpls.2022.828454
Ferentinos, K. P. (2018). Deep learning models for plant disease detection and diagnosis. Comput. Electron. Agric. 145, 311–318. doi: 10.1016/j.compag.2018.01.009
Fernández-Quintanilla, C., Peña, J. M., Andújar, D., Dorado, J., Ribeiro, A., López-Granados, F. (2018). Is the current state of the art of weed monitoring suitable for site-specific weed management in arable crops? Weed Res. 58, 259–272. doi: 10.1111/wre.12307
Filho, F. H. I., Heldens, W. B., Kong, Z., de Lange, E. S. (2020). Drones: Innovative technology for use in precision pest management. J. Econ. Entomol. 113, 1–25. doi: 10.1093/jee/toz268
Floreano, D., Wood, R. J. (2015). Science, technology and the future of small autonomous drones. Nature 521, 460–466. doi: 10.1038/nature14542
Fuentes, S., Tongson, E., Unnithan, R. R., Gonzalez Viejo, C. (2021). Early detection of aphid infestation and insect-plant interaction assessment in wheat using a low-cost electronic nose (e-nose), near-infrared spectroscopy and machine learning modeling. Sensors 21, 5948. doi: 10.3390/s21175948
Fürnkranz, J., Kliegr, T. (2015). A brief overview of rule learning. Lect. Notes Comput. Sci. (including subseries Lect. Notes Artif. Intell. Lect. Notes Bioinformatics) 9202, 54–69. doi: 10.1007/978-3-319-21542-6\s\do5(4
Gaitán, C. F. (2020). “Chapter 7 - machine learning applications for agricultural impacts under extreme events,” in Climate extremes and their implications for impact and risk assessment. Eds. Sillmann, J., Sippel, S., Russo, S. (Elsevier), 119–138.
Gao, J., Nuyttens, D., Lootens, P., He, Y., Pieters, J. G. (2018). Recognising weeds in a maize crop using a random forest machine-learning algorithm and near-infrared snapshot mosaic hyperspectral imagery. Biosyst. Eng. 170, 39–50. doi: 10.1016/j.biosystemseng.2018.03.006
Gao, W., Zhou, P. (2019). Customized high performance and energy efficient communication networks for AI chips. IEEE Access 7, 69434–69446. doi: 10.1109/ACCESS.2019.2916338
Garcia-Ruiz, F., Sankaran, S., Maja, J. M., Lee, W. S., Rasmussen, J., Ehsani, R. (2013). Comparison of two aerial imaging platforms for identification of huanglongbing-infected citrus trees. Comput. Electron. Agric. 91, 106–115. doi: 10.1016/j.compag.2012.12.002
García-Valls, M., Dubey, A., Botti, V. (2018). Introducing the new paradigm of social dispersed computing: Applications, technologies and challenges. J. Syst. Architect. 91, 83–102. doi: 10.1016/j.sysarc.2018.05.007
Gerhards, R., Andújar Sanchez, D., Hamouz, P., Peteinatos, G. G., Christensen, S., Fernandez-Quintanilla, C. (2022). Advances in site-specific weed management in agriculture–-a review. Weed Res. 62, 123–133. doi: 10.1111/wre.12526
Ghosh-Dastidar, S., Adeli, H. (2009). Spiking neural networks. Int. J. Neural Syst. 19, 295–308. doi: 10.1142/S0129065709002002
Giampieri, F., Mazzoni, L., Cianciosi, D., Alvarez-Suarez, J. M., Regolo, L., Sánchez-González, C., et al. (2022). Organic vs conventional plant-based foods: A review. Food Chem. 383, 132352. doi: 10.1016/j.foodchem.2022.132352
Girshick, R. (2015). “Fast r-cnn,” in Proceedings of the IEEE International Conference on Computer Vision (ICCV). (Santiago, Chile: IEEE Xplore) 1440–1448.
Girshick, R., Donahue, J., Darrell, T., Malik, J. (2014). “Rich feature hierarchies for accurate object detection and semantic segmentation,” in Proceedings of the IEEE Conference on Computer Vision and Pattern Recognition (CVPR). (Columbus, OH, USA: IEEE Xplore) 580–587.
Glaessgen, E., Stargel, D. (2012). “The digital twin paradigm for future nasa and u.s. air force vehicles,” in 53rd AIAA/ASME/ASCE/AHS/ASC Structures, Structural Dynamics and Materials Conference. Honolulu, Hawaii, USA: American Institute for Aeronautics and Astronautics (AIAA) (Reston: Aerospace Research Central).
Gold, K. M., Townsend, P. A., Herrmann, I., Gevens, A. J. (2020). Investigating potato late blight physiological differences across potato cultivars with spectroscopy and machine learning. Plant Sci. 295, 110316. doi: 10.1016/j.plantsci.2019.110316
Gómez-Casero, M. T., Castillejo-González, I. L., García-Ferrer, A., Peña-Barragán, J. M., Jurado-Expósito, M., García-Torres, L., et al. (2010). Spectral discrimination of wild oat and canary grass in wheat fields for less herbicide application. Agron. Sustain. Dev. 30, 689–699. doi: 10.1051/agro/2009052
Grimstad, L., From, P. J. (2017). The thorvald ii agricultural robotic system. Robotics 6, 24. doi: 10.3390/robotics6040024
Gu, F., Niu, J., Jiang, L., Liu, X., Atiquzzaman, M. (2020). Survey of the low power wide area network technologies. J. Netw. Comput. Appl. 149, 102459. doi: 10.1016/j.jnca.2019.102459
Guansong, P., Chunhua, S., Longbing, C., Anton, V. D. H. (2022). Deep learning for anomaly detection: A review. ACM Computing Surveys 54, 1–38. doi: 10.1145/3439950
Gutierrez, A., Ansuategi, A., Susperregi, L., Tubío, C., Rankić, I., Lenža, L. (2019). A benchmarking of learning strategies for pest detection and identification on tomato plants for autonomous scouting robots using internal databases. J. Sensors 2019, e5219471. doi: 10.1155/2019/5219471
Hasan, A. S. M. M., Sohel, F., Diepeveen, D., Laga, H., Jones, M. G. (2021). A survey of deep learning techniques for weed detection from images. Comput. Electron. Agric. 184, 106067. doi: 10.1016/j.compag.2021.106067
Hashimoto, N., Ozawa, S., Ban, T., Nakazato, J., Shimamura, J. (2018). A darknet traffic analysis for "IoT" malwares using association rule learning. Proc. Comput. Sci. 144, 118–123. doi: 10.1016/j.procs.2018.10.511
He, K., Gkioxari, G., Dollar, P., Girshick, R. (2017). “Mask r-CNN,” in Proceedings of the IEEE International Conference on Computer Vision (ICCV). (Venice, Italy: IEEE Xplore) 2961–2969.
He, K., Zhang, X., Ren, S., Sun, J. (2016). “Deep residual learning for image recognition,” in Proceedings of the IEEE Conference on Computer Vision and Pattern Recognition (CVPR). (Las Vegas, NV, USA: IEEE) 770–778.
Hoegh-Guldberg, O., Jacob, D., Taylor, M., Bolaños, T. G., Bindi, M., Brown, S., et al. (2019). The human imperative of stabilizing global climate change at 1.5°C. Science 365, eaaw6974. doi: 10.1126/science.aaw6974
Hong, S.-J., Kim, S.-Y., Kim, E., Lee, C.-H., Lee, J.-S., Lee, D.-S., et al. (2020). Moth detection from pheromone trap images using deep learning object detectors. Agriculture 10, 170. doi: 10.3390/agriculture10050170
Hooftman, D. A., Bullock, J. M., Jones, L., Eigenbrod, F., Barredo, J. I., Forrest, M., et al. (2022). Reducing uncertainty in ecosystem service modelling through weighted ensembles. Ecosys. Serv. 53, 101398. doi: 10.1016/j.ecoser.2021.101398
Howard, A. G., Zhu, M., Chen, B., Kalenichenko, D., Wang, W., Weyand, T., et al. (2017). MobileNets: Efficient convolutional neural networks for mobile vision applications. arXiv preprint arXiv:1704.04861. abs/1704.04861. doi: 10.48550/ARXIV.1704.04861
Huang, H., Deng, J., Lan, Y., Yang, A., Deng, X., Zhang, L., et al. (2018). A two-stage classification approach for the detection of spider mite- infested cotton using UAV multispectral imagery. Remote Sens Lett 9, 933–941. doi: 10.1080/2150704X.2018.1498600
Huang, G., Liu, Z., van der Maaten, L., Weinberger, K. Q. (2016). Densely connected convolutional networks. Proceedings of the IEEE conference on computer vision and pattern recognition. Las Vegas, NV, USA. 4700–4708. doi: 10.48550/ARXIV.1608.06993
Huang, Y., Zhao, X., Pan, Z., Reddy, K. N., Zhang, J. (2022). Hyperspectral plant sensing for differentiating glyphosate-resistant and glyphosate-susceptible johnsongrass through machine learning algorithms. Pest Manage. Sci. 78, 2370–2377. doi: 10.1002/ps.6864
Hussain, N., Farooque, A. A., Schumann, A. W., Abbas, F., Acharya, B., McKenzie-Gopsill, A., et al. (2021). Application of deep learning to detect lamb’s quarters (Chenopodium album l.) in potato fields of Atlantic Canada. Comput. Electron. Agric. 182, 106040. doi: 10.1016/j.compag.2021.106040
Ibrahim, A., Gebali, F. (2022). Compact hardware accelerator for field multipliers suitable for use in ultra-low power IoT edge devices. Alexandria Eng. J. 61, 13079–13087. doi: 10.1016/j.aej.2022.07.013
IPPC Secretariat (2021). Scientific review of the impact of climate change on plant pests: A global challenge to prevent and mitigate plant-pest risks in agriculture, forestry and ecosystems (Italy: FAO on behalf of the IPPC Secretariat).
ISPA (2021). “Precision agriculture definition,” in Precision agriculture definition. Eds. Arnã³, J., Best, S., Bongiovanni, R., Escolã, A., Gil, E., Ortega, R., Peã±a, J. M., Scaramuzza, F., Kelly, P. T., Ubierna, C. V. (International Society of Precision Agriculture).
Jeong, H., Shi, L. (2019). Memristor devices for neural networks. J. Phys. D: Appl. Phys. 52, 023003. doi: 10.1088/1361-6463/aae223
Jin, X., Jie, L., Wang, S., Qi, H. J., Li, S. W. (2018). Classifying wheat hyperspectral pixels of healthy heads and fusarium head blight disease using a deep neural network in the wild field. Remote Sens. 10, 395. doi: 10.3390/rs10030395
Juroszek, P., Racca, P., Link, S., Farhumand, J., Kleinhenz, B. (2020). Overview on the review articles published during the past 30 years relating to the potential climate change effects on plant pathogens and crop disease risks. Plant Pathol. 69, 179–193. doi: 10.1111/ppa.1311
Kamilaris, A., Prenafeta-Boldú, F. X. (2018). Deep learning in agriculture: A survey. Comput. Electron. Agric. 147, 70–90. doi: 10.1016/j.compag.2018.02.016
Khanal, S., Fulton, J., Shearer, S. (2017). An overview of current and potential applications of thermal remote sensing in precision agriculture. Comput. Electron. Agric. 139, 22–32. doi: 10.1016/j.compag.2017.05.001
Khanji, S., Iqbal, F., Hung, P. (2019). “Zigbee security vulnerabilities: Exploration and evaluating,” in 2019 10th International Conference on Information and Communication Systems (ICICS). (Irbid, Jordan: IEEE) 52–57.
Kowsari, K., Jafari Meimandi, K., Heidarysafa, M., Mendu, S., Barnes, L., Brown, D. (2019). Text classification algorithms: A survey. Information 10, 150. doi: 10.3390/info10040150
Krizhevsky, A., Sutskever, I., Hinton, G. E. (2012). “Imagenet classification with deep convolutional neural networks,” in Proceedings of the 25th International Conference on Neural Information Processing Systems. (Lake Tahoe, Nevada, USA): Curran Associates Inc. 1097–1105.
Lassalle, G. (2021). Monitoring natural and anthropogenic plant stressors by hyperspectral remote sensing: Recommendations and guidelines based on a meta-review. Sci. Total Environ. 788, 147758. doi: 10.1016/j.scitotenv.2021.147758
Lauwers, M., De Cauwer, B., Nuyttens, D., Cool, S. R., Pieters, J. G. (2020). Hyperspectral classification of Cyperus esculentus clones and morphologically similar weeds. Sensors 20, 2504. doi: 10.3390/s20092504
Lavric, A. (2019). Lora (long-range) high-density sensors for internet of things. J. Sensors 2019, 3502987. doi: 10.1155/2019/3502987
LeCun, Y., Bengio, Y., Hinton, G. (2015). Deep learning. Nature 521, 436–444. doi: 10.1038/nature14539
LeCun, Y., Bottou, L., Bengio, Y., Haffner, P. (1998). Gradient-based learning applied to document recognition. Proc. IEEE 86, 2278–2324. doi: 10.1109/5.726791
Li, Y., Guo, Z., Shuang, F., Zhang, M., Li, X. (2022). Key technologies of machine vision for weeding robots: A review and benchmark. Comput. Electron. Agric. 196, 106880. doi: 10.1016/j.compag.2022.106880
Liakos, K. G., patrizia Busato, Moshou, D., Pearson, S., Bochtis, D. (2018). Machine learning in agriculture: A review. Sensors 18, 2674. doi: 10.3390/s18082674
Lima, M. C. F., de Almeida Leandro, M. E. D., Valero, C., Coronel, L. C. P., Bazzo, C. O. G. (2020). Automatic detection and monitoring of insect pests–a review. Agriculture 10, 161. doi: 10.3390/agriculture10050161
Lin, T.-Y., Dollar, P., Girshick, R., He, K., Hariharan, B., Belongie, S. (2017a). “Feature pyramid networks for object detection,” in Proceedings of the IEEE Conference on Computer Vision and Pattern Recognition (CVPR). 2117–2125 (Honolulu, HI, USA: IEEE Xplore).
Lin, T.-Y., Goyal, P., Girshick, R., He, K., Dollar, P. (2017b). “Focal loss for dense object detection,” in Proceedings of the IEEE International Conference on Computer Vision (ICCV). (Venice, Italy: IEEE Xplore) 2980–2988.
Liu, W., DragomirAnguelov, Erhan, D., Szegedy, C., Reed, S., Fu, C.-Y., Berg, A. C., et al. (2016). “SSD: Single shot MultiBox detector,” in Computer vision – ECCV 2016. Eds. Leibe, B., Matas, J., Sebe, N., Welling, M. (Amsterdam, The Netherlands: Springer International Publishing), 21–37.
Liu, L., Wang, R., Xie, C., Yang, P., Wang, F., Sudirman, S., et al. (2019). Pestnet: An end-to-end deep learning approach for large-scale multi-class pest detection and classification. IEEE Access 7, 45301–45312. doi: 10.1109/ACCESS.2019.2909522
López-Correa, J. M., Moreno, H., Ribeiro, A., Andújar, D. (2022). Intelligent weed management based on object detection neural networks in tomato crops. Agronomy 12, 2953. doi: 10.3390/agronomy12122953
Lottes, P., Behley, J., Chebrolu, N., Milioto, A., Stachniss, C. (2020). Robust joint stem detection and crop-weed classification using image sequences for plant-specific treatment in precision farming. J. Field Robot. 37, 20–34. doi: 10.1002/rob.21901
Lowenberg-DeBoer, J., Franklin, K., Behrendt, K., Godwin, R. (2021). Economics of autonomous equipment for arable farms. Precis. Agric. 22, 1992–2006. doi: 10.1007/s11119-021-09822-x
Lowenberg-DeBoer, J., Huang, I. Y., Grigoriadis, V., Blackmore, S. (2020). Economics of robots and automation in field crop production. Precis. Agric. 21, 278–299. doi: 10.1007/s11119-019-09667-5
Lu, J., Ehsani, R., Shi, Y., Abdulridha, J., de Castro, A. I., Xu, Y. (2017). Field detection of anthracnose crown rot in strawberry using spectroscopy technology. Comput. Electron. Agric. 135, 289–299. doi: 10.1016/j.compag.2017.01.017
Lu, J., Ehsani, R., Shi, Y., de Castro, A. I., Wang, S. (2018). Detection of multi-tomato leaf diseases (late blight, target and bacterial spots) in different stages by using a spectral-based sensor. Sci. Rep. 8, 2793. doi: 10.1038/s41598-018-21191-6
Lytridis, C., Kaburlasos, V. G., Pachidis, T., Manios, M., Vrochidou, E., Kalampokas, T., et al. (2021). An overview of cooperative robotics in agriculture. Agronomy 11, 1818. doi: 10.3390/agronomy11091818
Machleb, J., Peteinatos, G. G., Kollenda, B. L., Andújar, D., Gerhards, R. (2020). Sensor-based mechanical weed control: Present state and prospects. Comput. Electron. Agric. 176, 105638. doi: 10.1016/j.compag.2020.105638
Mahmoud, M. S., Xia, Y. (2019). Networked control systems: Cloud control and secure control (United Kingdom: Butterworth-Heinemann).
Maniah, Abdurachman, E., Gaol, F. L., Soewito, B. (2019). Survey on threats and risks in the cloud computing environment. Proc. Comput. Sci. 161, 1325–1332. doi: 10.1016/j.procs.2019.11.248
McCool, C., Beattie, J., Firn, J., Lehnert, C., Kulk, J., Bawden, O., et al. (2018). Efficacy of mechanical weeding tools: A study into alternative weed management strategies enabled by robotics. IEEE Robot Autom Lett 3, 1184–1190. doi: 10.1109/LRA.2018.2794619
Mishra, S., Sachan, R., Rajpal, D. (2020). Deep convolutional neural network based detection system for real-time corn plant disease recognition. Proc. Comput. Sci. 167, 2003–2010. doi: 10.1016/j.procs.2020.03.236
Muhammad, A., Mohammad, A.-U., Zubair, S., Ali, M., Aggoune., E.-H. M. (2019). Internet-of-Things (IoT)-based smart agriculture: Toward making the fields talk. IEEE Access 7, 129551–129583. doi: 10.1109/ACCESS.2019.2932609
Mulla, S., Singh, S. K., Singh, K. K., Praveen, B. (2020). “Climate change and agriculture: A review of crop models,” in Global climate change and environmental policy: Agriculture perspectives. Eds. Venkatramanan, V., Shah, S., Prasad, R. (Springer Singapore), 423–435.
Muppala, C., Guruviah, V. (2020). Machine vision detection of pests, diseases, and weeds: A review. J. Phytol. 12, 9–19. doi: 10.25081/jp.2020.v12.6145
Murtagh, F., Contreras, P. (2012). Algorithms for hierarchical clustering: An overview. Wiley Interdiscip. Reviews: Data Min. Knowl. Discovery 2, 86–97. doi: 10.1002/widm.53
Nanda, S. J., Panda, G. (2014). A survey on nature inspired metaheuristic algorithms for partitional clustering. Swarm Evol. Comput. 16, 1–18. doi: 10.1016/j.swevo.2013.11.003
Nugent, P. W., Shaw, J. A., Jha, P., Scherrer, B., Donelick, A., Kumar, V. (2018). Discrimination of herbicide-resistant kochia with hyperspectral imaging. J. Appl. Remote Sens. 12, 16037. doi: 10.1117/1.JRS.12.016037
Oberti, R., Schmilovitch, Z. (2021). “Robotic spraying for precision crop protection,” in Innovation in agricultural robotics for precision agriculture: A roadmap for integrating robots in precision agriculture. Ed. Bechar, A. (Cham: Springer International Publishing), 117–150.
Oerke, E.-C., Dehne, H.-W., Schönbeck, F., Weber, A. (2012). Crop production and crop protection: Estimated losses in major food and cash crops (Amsterdam: Elsevier).
Oñate, W., Sanz, R. (2023). Analysis of architectures implemented for IIoT. Heliyon 9–1. doi: 10.1016/j.heliyon.2023.e12868
Pätzold, S., Hbirkou, C., Dicke, D., Gerhards, R., Welp, G. (2020). Linking weed patterns with soil properties: a long-term case study. Precis. Agric. 21, 569–588. doi: 10.1007/s11119-019-09682-6
Pauline, M., Adrien, M., Clélie, A., Nicolas, P., Guy-Junior, R., Olivier, R., et al. (2019). Human movement and ergonomics: an industry-oriented dataset for collaborative robotics. Int. J. Robot. Res. 38, 1529–1537. doi: 10.1177/0278364919882089
Peña, J. M., Torres-Sánchez, J., Serrano-Pérez, A., De Castro, A. I., López-Granados, F. (2015). Quantifying efficacy and limits of unmanned aerial vehicle (UAV) technology for weed seedling detection as affected by sensor resolution. Sensors 15, 5609–5626. doi: 10.3390/s150305609
Peña-Barragán, J. M., López-Granados, F., Jurado-Expósito, M., García-Torres, L. (2006). Spectral discrimination of Ridolfia segetum and sunflower as affected by phenological stage. Weed Res. 46, 10–21. doi: 10.1111/j.1365-3180.2006.00488.x
Pérez-Ortiz, M., Peña, J. M., Gutiérrez, P. A., Torres-Sánchez, J., Hervás-Martínez, C., López-Granados, F. (2015). A semi-supervised system for weed mapping in sunflower crops using unmanned aerial vehicles and a crop row detection method. Appl. Soft Comput. 37, 533–544. doi: 10.1016/j.asoc.2015.08.027
Pérez-Ortiz, M., Peña, J. M., Gutiérrez, P. A., Torres-Sánchez, J., Hervás-Martínez, C., López-Granados, F. (2016). Selecting patterns and features for between- and within- crop-row weed mapping using UAV-imagery. Expert Syst. Appl. 47, 85–94. doi: 10.1016/j.eswa.2015.10.043
Pérez-Ruiz, M., Gonzalez-de Santos, P., Ribeiro, A., Fernandez-Quintanilla, C., Peruzzi, A., Vieri, M., et al. (2015). Highlights and preliminary results for autonomous crop protection. Comput. Electron. Agric. 110, 150–161. doi: 10.1016/j.compag.2014.11.010
Pérez-Ruíz, M., Slaughter, D. C., Fathallah, F. A., Gliever, C. J., Miller, B. J. (2014). Co-Robotic intra-row weed control system. Biosyst. Eng. 126, 45–55. doi: 10.1016/j.biosystemseng.2014.07.009
Piao, S., Liu, Q., Chen, A., Janssens, I. A., Fu, Y., Dai, J., et al. (2019). Plant phenology and global climate change: Current progresses and challenges. Global Change Biol. 25, 1922–1940. doi: 10.1111/gcb.14619
Picon, A., Alvarez-Gila, A., Seitz, M., Ortiz-Barredo, A., Echazarra, J., Johannes, A. (2019). Deep convolutional neural networks for mobile capture device-based crop disease classification in the wild. Comput. Electron. Agric. 161, 280–290. doi: 10.1016/j.compag.2018.04.002
Polder, G., Blok, P. M., de Villiers, H. A. C., van der Wolf, J. M., Kamp, J. (2019). Potato virus y detection in seed potatoes using deep learning on hyperspectral images. Front. Plant Sci. 10. doi: 10.3389/fpls.2019.00209
Poniszewska-Maranda, A., Matusiak, R., Kryvinska, N., Yasar, A.-U.-H. (2020). A real-time service system in the cloud. J. Ambient Intell. Humanized Comput. 11, 961–977. doi: 10.1007/s12652-019-01203-7
Pretto, A., Aravecchia, S., Burgard, W., Chebrolu, N., Dornhege, C., Falck, T., et al. (2019). Building an Aerial–Ground robotics system for precision farming: An adaptable solution. IEEE Robot. Automation Mag. 28, 29–49. doi: 10.1109/mra.2020.3012492
Qiu, J., Wang, J., Yao, S., Guo, K., Li, B., Zhou, E., et al. (2016). “Going deeper with embedded FPGA platform for convolutional neural network,” in Proceedings of the 2016 ACM/SIGDA International Symposium on Field-Programmable Gate Arrays. (Monterey, CA, USA: Association for Computing Machinery) 26–35.
Rai, N., Zhang, Y., Ram, B. G., Schumacher, L., Yellavajjala, R. K., Bajwa, S., et al. (2023). Applications of deep learning in precision weed management: A review. Comput. Electron. Agric. 206, 107698. doi: 10.1016/j.compag.2023.107698
Rakhmatulin, I., Kamilaris, A., Andreasen, C. (2021). Deep neural networks to detect weeds from crops in agricultural environments in real-time: A review. Remote Sens. 13, 4486. doi: 10.3390/rs13214486
Ramcharan, A., McCloskey, P., Baranowski, K., Mbilinyi, N., Mrisho, L., Ndalahwa, M., et al. (2019). A mobile-based deep learning model for cassava disease diagnosis. Front. Plant Sci. 10. doi: 10.3389/fpls.2019.00272
Ramos, A. P. M., Gomes, F. D. G., Pinheiro, M. M. F., Furuya, D. E. G., Gonçalvez, W. N., Junior, J. M., et al. (2022). Detecting the attack of the fall armyworm (Spodoptera frugiperda) in cotton plants with machine learning and spectral measurements. Precis. Agric. 23, 470–491. doi: 10.1007/s11119-021-09845-4
Reddy, K. N., Huang, Y., Lee, M. A., Nandula, V. K., Fletcher, R. S., Thomson, S. J., et al. (2014). Glyphosate-resistant and glyphosate-susceptible palmer amaranth (Amaranthus palmeri s. wats.): hyperspectral reflectance properties of plants and potential for classification. Pest Manage. Sci. 70, 1910–1917. doi: 10.1002/ps.3755
Redmon, J., Divvala, S., Girshick, R., Farhadi, A. (2016). “You only look once: Unified, real-time object detection,” in Proceedings of the IEEE Conference on Computer Vision and Pattern Recognition (CVPR). (Las Vegas, NV, USA: IEEE Xplore).
Ren, S., He, K., Girshick, R., Sun, J. (2015). “Faster r-CNN: Towards real-time object detection with region proposal networks,” in Advances in neural information processing systems. Eds. Cortes, C., Lawrence, N., Lee, D., Sugiyama, M., Garnett, R. (Curran Associates, Inc).
Ren, G., Lin, T., Ying, Y., Chowdhary, G., Ting, K. C. (2020). Agricultural robotics research applicable to poultry production: A review. Comput. Electron. Agric. 169, 105216. doi: 10.1016/j.compag.2020.105216
Ren, Y., Zhang, L., Suganthan, P. (2016). Ensemble classification and regression-recent developments, applications and future directions. IEEE Comput Intell Mag 11, 41–53. doi: 10.1109/MCI.2015.2471235
Roslim, M. H. M., Juraimi, A. S., Che’Ya, N. N., Sulaiman, N., Manaf, M. N. H. A., Ramli, Z., et al. (2021). Using remote sensing and an unmanned aerial system for weed management in agricultural crops: A review. Agronomy 11, 1809. doi: 10.3390/agronomy11091809
Sagi, O., Rokach, L. (2018). Ensemble learning: A survey. WIREs Data Mining and Knowledge Discovery 8. doi: 10.1002/widm.1249
Saiz-Rubio, V., Rovira-Más, F. (2020). From smart farming towards agriculture 5.0: A review on crop data management. Agronomy 10, 207–228. doi: 10.3390/agronomy10020207
Saleem, M. H., Potgieter, J., Arif, K. M. (2021). Automation in agriculture by machine and deep learning techniques: A review of recent developments. Precis. Agric. 22, 2053–2091. doi: 10.1007/s11119-021-09806-x
Santos Valle, S., Kienzle, J. (2020). Agriculture 4.0–agricultural robotics and automated equipment for sustainable crop production (Rome: FAO: Food and Agriculture Organization of the United Nations).
Sarker, I. H. (2021). Deep learning: A comprehensive overview on techniques, taxonomy, applications and research directions. SN Comput. Sci. 2, 420. doi: 10.1007/s42979-021-00815-1
Schmidhuber, J. (2015). Deep learning in neural networks: An overview. Neural Networks 61, 85–117. doi: 10.1016/j.neunet.2014.09.003
Selvaraj, M. G., Vergara, A., Montenegro, F., Ruiz, H. A., Safari, N., Raymaekers, D., et al. (2020). Detection of banana plants and their major diseases through aerial images and machine learning methods: A case study in DR Congo and republic of Benin. ISPRS J. Photogram. Remote Sens. 169, 110–124. doi: 10.1016/j.isprsjprs.2020.08.025
Sen, P. C., Hajra, M., Ghosh, M. (2020). “Supervised classification algorithms in machine learning: A survey and review,” in Emerging technology in modelling and graphics. Ed. Mandal, J. K. (Springer Singapore), 99–111.
Shafi, U., Mumtaz, R., GarcÃa-Nieto, J., Hassan, S. A., Zaidi, S. A. R., Iqbal, N. (2019). Precision agriculture techniques and practices: From considerations to applications. Sensors 19, 3796. doi: 10.3390/s19173796
Shamshiri, R. R., Weltzien, C., Hameed, I. A., Yule, I. J., Grift, T. E., Balasundram, S. K., et al. (2018). Research and development in agricultural robotics: A perspective of digital farming. Int. J. Agric. Biol. Eng. 11, 1–14. doi: 10.25165/ijabe.v11i4.4278
Shankar, P., Johnen, A., Liwicki, M. (2020). “Data fusion and artificial neural networks for modelling crop disease severity,” in 2020 "IEEE" 23rd International Conference on Information Fusion (FUSION). Virtual Conference. 1–8.
Shawahna, A., Sait, S. M., El-Maleh, A. (2019). FPGA-based accelerators of deep learning networks for learning and classification: A review. IEEE Access 7, 7823–7859. doi: 10.1109/ACCESS.2018.2890150
Simonyan, K., Zisserman, A. (2014). Very deep convolutional networks for large-scale image recognition. arXiv preprint arXiv:1409.1556. doi: 10.48550/ARXIV.1409.1556
Sohn, S.-I., Oh, Y.-J., Pandian, S., Lee, Y.-H., Zaukuu, J.-L. Z., Kang, H.-J., et al. (2021). Identification of Amaranthus species using visible-Near-Infrared (Vis-NIR) spectroscopy and machine learning methods. Remote Sens. 13, 4149. doi: 10.3390/rs13204149
Sparrow, R., Howard, M. (2021). Robots in agriculture: prospects, impacts, ethics, and policy. Precis. Agric. 22, 818–833. doi: 10.1007/s11119-020-09757-9
Strukov, D. B., Snider, G. S., Stewart, D. R., Williams, R. S. (2008). The missing memristor found. Nature 453, 80–83. doi: 10.1038/nature06932
Su, J., Yi, D., Coombes, M., Liu, C., Zhai, X., McDonald-Maier, K., et al. (2022). Spectral analysis and mapping of blackgrass weed by leveraging machine learning and uav multispectral imagery. Comput. Electron. Agric. 192, 106621. doi: 10.1016/j.compag.2021.106621
Sun, P. (2020). Security and privacy protection in cloud computing: Discussions and challenges. J. Netw. Comput. Appl. 160, 102642. doi: 10.1016/j.jnca.2020.102642
Szegedy, C., Liu, W., Jia, Y., Pierre, S., Reed, S., Anguelov, D., et al. (2015). “Going deeper with convolutions,” in 2015 IEEE Conference on Computer Vision and Pattern Recognition (CVPR). (Boston, MA, USA: IEEE) 1–9.
Tang, Z., Yang, J., Li, Z., Qi, F. (2020). Grape disease image classification based on lightweight convolution neural networks and channelwise attention. Comput. Electron. Agric. 178, 105735. doi: 10.1016/j.compag.2020.105735
Telikani, A., Tahmassebi, A., Banzhaf, W., Gandomi, A. H. (2022). Evolutionary machine learning: A survey. ACM Comput. Surv. 54, 1–35. doi: 10.1145/3467477
Thakur, D., Kumar, Y., Kumar, A., Singh, P. K. (2019). Applicability of wireless sensor networks in precision agriculture: A review. Wireless Pers. Commun. 107, 471–512. doi: 10.1007/s11277-019-06285-2
Torres-Sánchez, J., Mesas-Carrascosa, F. J., Jiménez-Brenes, F. M., de Castro, A. I., López-Granados, F. (2021). Early detection of broad-leaved and grass weeds in wide row crops using artificial neural networks and UAV imagery. Agronomy 11, 749. doi: 10.3390/agronomy11040749
Tugrul, B., Elfatimi, E., Eryigit, R. (2022). Convolutional neural networks in detection of plant leaf diseases: A review. Agriculture 12, 1192 doi: 10.3390/agriculture12081192
Ullah, Z., Al-Turjman, F., Mostarda, L. (2020). Cognition in UAV-aided 5G and beyond communications: A survey. IEEE Trans. Cogn. Commun. Netw. 6, 872–891. doi: 10.1109/TCCN.2020.2968311
van Dijk, M., Gramberger, M., Laborde, D., Mandryk, M., Shutes, L., Stehfest, E., et al. (2020). Stakeholder-designed scenarios for global food security assessments. Global Food Secur. 24, 100352. doi: 10.1016/j.gfs.2020.100352
Wang, Y. E., Wei, G.-Y., Brooks, D. (2019). Benchmarking TPU, GPU, and CPU platforms for deep learning. arXiv preprint arXiv:1907.10701 abs/1907.10701. doi: 10.48550/ARXIV.1907.10701
Wei, P., Lu, Z., Song, J. (2015). Variable importance analysis: A comprehensive review. Reliab. Eng. Sys. Saf. 142, 399–432. doi: 10.1016/j.ress.2015.05.018
Wolfert, S., Ge, L., Verdouw, C., Bogaardt, M.-J. (2017). Big data in smart farming–-A review. Agric. Syst. 153, 69–80. doi: 10.1016/j.agsy.2017.01.023
Xia, D., Chen, P., Wang, B., Zhang, J., Xie, C. (2018). Insect detection and classification based on an improved convolutional neural network. Sensors 18, 4169. doi: 10.3390/s18124169
Xia, Q., Yang, J. J. (2019). Memristive crossbar arrays for brain-inspired computing. Nat. Mater. 18, 309–323. doi: 10.1038/s41563-019-0291-x
Xiao, D., Pan, Y., Feng, J., Yin, J., Liu, Y., He, L. (2022). Remote sensing detection algorithm for apple fire blight based on uav multispectral image. Comput. Electron. Agric. 199, 107137. doi: 10.1016/j.compag.2022.107137
Xu, X., Liang, T., Zhu, J., Zheng, D., Sun, T. (2019). Review of classical dimensionality reduction and sample selection methods for large-scale data processing. Neurocomputing 328, 5–15. doi: 10.1016/j.neucom.2018.02.100
Zarco-Tejada, P. J., Camino, C., Beck, P. S. A., Calderon, R., Hornero, A., Hernández-Clemente, R., et al. (2018). Previsual symptoms of Xylella fastidiosa infection revealed in spectral plant-trait alterations. Nat Plants 4, 432–439. doi: 10.1038/s41477-018-0189-7
Zarco-Tejada, P. J., Poblete, T., Camino, C., Gonzalez-Dugo, V., Calderon, R., Hornero, A., et al. (2021). Divergent abiotic spectral pathways unravel pathogen stress signals across species. Nat. Commun. 12, 6088. doi: 10.1038/s41467-021-26335-3
Zeadally, S., Siddiqui, F., Baig, Z. (2019). 25 years of bluetooth technology. Future Internet 11, 194. doi: 10.3390/fi11090194
Zhai, Z., Martínez, J. F., Beltran, V., Martínez, N. L. (2020). Decision support systems for agriculture 4.0: Survey and challenges. Comput. Electron. Agric. 170, 105256. doi: 10.1016/j.compag.2020.105256
Zhang, X., Cao, Z., Dong, W. (2020). Overview of edge computing in the agricultural internet of things: Key technologies, applications, challenges. IEEE Access 8, 141748–141761. doi: 10.1109/ACCESS.2020.3013005
Zhang, Y., Gao, J., Cen, H., Lu, Y., Yu, X., He, Y., et al. (2019b). Automated spectral feature extraction from hyperspectral images to differentiate weedy rice and barnyard grass from a rice crop. Comput. Electron. Agric. 159, 42–49. doi: 10.1016/j.compag.2019.02.018
Zhang, X., Han, L., Dong, Y., Shi, Y., Huang, W., Han, L., et al. (2019a). A deep learning-based approach for automated yellow rust disease detection from high-resolution hyperspectral UAV images. Remote Sens. 11, 1554. doi: 10.3390/rs11131554
Zhang, K., Lammers, K., Chu, P., Li, Z., Lu, R. (2021). System design and control of an apple harvesting robot. Mechatronics 79, 102644. doi: 10.1016/j.mechatronics.2021.102644
Zhou, Z., Chen, X., Li, E., Zeng, L., Luo, K., Zhang, J. (2019). Edge intelligence: Paving the last mile of artificial intelligence with edge computing. Proc. 107, 1738–1762. doi: 10.1109/JPROC.2019.2918951
Zhu, H., Chu, B., Zhang, C., Liu, F., Jiang, L., He, Y. (2017). Hyperspectral imaging for presymptomatic detection of tobacco disease with successive projections algorithm and machine-learning classifiers. Sci. Rep. 7, 4125. doi: 10.1038/s41598-017-04501-2
Zidan, M. A., Strachan, J. P., Lu, W. D. (2018). The future of electronics based on memristive systems. Nat. Electron. 1, 22–29. doi: 10.1038/s41928-017-0006-8
Keywords: precision agriculture (PA), artificial intelligence (AI), deep learning, unmanned aerial vehicles (UAV), decision support system (DDS), robotics
Citation: Mesías-Ruiz GA, Pérez-Ortiz M, Dorado J, de Castro AI and Peña JM (2023) Boosting precision crop protection towards agriculture 5.0 via machine learning and emerging technologies: A contextual review. Front. Plant Sci. 14:1143326. doi: 10.3389/fpls.2023.1143326
Received: 12 January 2023; Accepted: 01 March 2023;
Published: 22 March 2023.
Edited by:
Marcin Wozniak, Silesian University of Technology, PolandReviewed by:
Jana Shafi, Prince Sattam Bin Abdulaziz University, Saudi ArabiaAdam Tadeusz Zielonka, Silesian University of Technology, Poland
Copyright © 2023 Mesías-Ruiz, Pérez-Ortiz, Dorado, de Castro and Peña. This is an open-access article distributed under the terms of the Creative Commons Attribution License (CC BY). The use, distribution or reproduction in other forums is permitted, provided the original author(s) and the copyright owner(s) are credited and that the original publication in this journal is cited, in accordance with accepted academic practice. No use, distribution or reproduction is permitted which does not comply with these terms.
*Correspondence: José M. Peña, am1wZW5hQGljYS5jc2ljLmVz