- 1 Max-Planck-Institute of Molecular Plant Physiology, Potsdam-Golm, Germany
- 2 Horticultural Sciences Department and the Plant Molecular and Cellular Biology Program, University of Florida, Gainesville, FL, USA
The study of metabolic regulation has traditionally focused on analysis of specific enzymes, emphasizing kinetic properties, and the influence of protein interactions and post-translational modifications. More recently, reverse genetic approaches permit researchers to directly determine the effects of a deficiency or a surplus of a given enzyme on the biochemistry and physiology of a plant. Furthermore, in many model species, gene expression atlases that give important spatial information concerning the quantitative expression level of metabolism-associated genes are being produced. In parallel, “top-down” approaches to understand metabolic regulation have recently been instigated whereby broad genetic diversity is screened for metabolic traits and the genetic basis of this diversity is defined thereafter. In this article we will review recent examples of this latter approach both in the model species Arabidopsis thaliana and the crop species tomato (Solanum lycopersicum). In addition to highlighting examples in which this genetic diversity approach has proven promising, we will discuss the challenges associated with this approach and provide a perspective for its future utility.
Introduction
Elucidation of metabolic regulation in plants has been an academic pursuit spanning many decades. Indeed the question of how metabolism is regulated was first raised in the scientific era in which metabolism was first truly defined (Buchner, 1907; Krebs and Henseleit, 1932; Plaxton, 1996; Kornberg, 2000). Whilst the ability to regulate the rates of metabolic processes in response to cellular circumstance is a common feature of all organisms, it is particularly acute in sessile organism such as plants (Plaxton, 1996). From a textbook perspective, metabolic regulation is classically divided into coarse and fine levels of control (Dennis et al., 1997; Fell, 1997). Coarse control refers to long-term mechanisms that are energetically expensive and lead to changes in the total cellular population of a protein. By contrast, fine control describes generally fast (and therefore energetically inexpensive) regulatory devices that modulate the activity of pre-existing enzyme molecules. Whilst this arbitrary division can be useful for descriptive purposes, recent reports suggest that several regulatory mechanisms cannot be so easily defined and hence such classification is commonly regarded as outdated. However, unfortunately, despite the massive amount of data available regarding steady-state RNA levels afforded by microarrays (see for example the data stored in GENEVESTIGATOR; http://www.genevestigator.ethz.ch) and more recently by next generation sequence analyses (see for example Gonzalez-Ballester et al., 2010; Bräutigam et al., 2011), protein abundance data remains relatively scare. That said, important recent advances have been made both regarding protein synthesis (Mustroph et al., 2009; Piques et al., 2009) and degradation (Araújo et al., 2010, 2011; Hua and Vierstra, 2011).
Several regulatory mechanisms act on already synthesized enzymes. Indeed our understanding of regulation of central (primary) plant metabolism has been largely defined by the discovery of such features within the last 50–60 years, whereas understanding of specialized (secondary) metabolism has made similar strides within the last 30 years (for reviews see Pichersky and Gang, 2000; D’Auria and Gershenzon, 2005; Gachon et al., 2005; Yonekura-Sakakibara and Saito, 2009). In brief, such mechanisms include (i) alteration in substrate or co-substrate concentration, (ii) variation in pH, (iii) allosteric effectors. The importance of all three of these mechanisms is illustrated by multiple examples. The first, of these is essentially the most simple and certainly the most rapid to affect metabolic systems with the rate of an enzyme-catalyzed reaction proceeding more rapidly upon an increase in sub-saturating substrate – a case that is common in vivo (Dennis et al., 1997). All enzyme reactions are, to a greater or lesser extent regulated in this manner. However, the situation is complicated by the fact that not all reactions display simple Michaelis–Menton-like kinetics and by the fact that many co-substrates are shared by multiple reactions. These factors alone render understanding the systemic response to prevailing fluctuations in substrate conditions unpredictable. Secondly, many enzymes are affected by pH. For example, regulation of enzymes of the Calvin cycle is well documented to be pH regulated; stromal pH is 8.0 in that the light and 7.0 in the dark (see Dennis et al., 1997). Thirdly, allosteric effectors are immensely important in the regulation of plant metabolic networks, be they activators or inhibitors. Within the major pathways of carbohydrate metabolism, several examples of the importance of such metabolites exist, including the 3 phosphoglycerate (3PGA)/inorganic phosphate (Pi) ratio in activating ADP glucose pyrophosphorylase (AGPase; Preiss, 1982; Tiessen et al., 2002), the fructose 2,6-bisphosphatase (Fru 2,6P2ase) system (Stitt, 1990; Fernie et al., 2001) and the effect of pyruvate on the alternative oxidase of mitochondrial respiration (Millar et al., 1993; Oliver et al., 2008).
Understanding the function of a given enzyme within a biological process has until recently largely followed a set protocol by which novel genes associated with a specific process are identified by means of similar patterns of expression across a wide range of experiments and subsequently their function tested. This is initially carried out by analyzing the metabolite profiles of genotypes deficient in the expression of the gene. Confirmation of kinetic properties of the enzyme either in planta (in the case that the gene encodes the only isoform of an enzyme) or following expression of the gene in a heterologous system lacking the activity is subsequently required (see for details Tohge and Fernie, 2010). Whilst this approach has been tremendously successful (Hirai et al., 2005, 2007; Tohge et al., 2005; Okazaki et al., 2009) in terms of annotating the precise biochemical function of individual genes, it does not enable elucidation of the exact physiological function in vivo. In the last 25 years the roles of specific metabolic enzymes have been addressed via the use of transgenic plants (Stitt and Sonnewald, 1995; Lytovchenko et al., 2007). Such studies have greatly advanced our understanding of metabolic regulation. However, such directed approaches sometimes fail to uncover complex inter-pathway interactions and pathway regulators. Given that the chemical constituents of any life form determine the development and functioning of the organism (Sumner et al., 2003; Fernie et al., 2004a; D’Auria and Gershenzon, 2005), strategies to characterize the chemical complement of the cell are becoming increasingly important. The diversity of metabolites is controlled by a complex interaction involving many structural and regulatory genes as well as environmental influences (Harrigan et al., 2007a). Although chemical profiles differ between and even within species, thousands of diverse metabolites are usually found in a single plant (De Luca and St Pierre, 2000; Sumner et al., 2003; D’Auria and Gershenzon, 2005; Fernie, 2007). These range from small and simple structures such as vitamins and amino acids to more complex compounds such as polycyclic antioxidants and protease inhibitors. Other compounds function as energy carriers that can store or release energy upon formation or degradation respectively (Fernie et al., 2004b). For example, glucose synthesized during gluconeogenesis can be polymerized to form starch or be broken down during glycolysis. This regulated interconversion of compounds is perhaps the most important hallmark of plant metabolism, enabling the organism to respond to specific demands over the course of its life and on a minute-by-minute basis (Keuentjes and Fernie, 2011).
Metabolites are often classified as being either primary or secondary, although no strict discrimination can be made and interactions between the two classes are manifold. Primary metabolism includes essential metabolites such as those in central carbohydrate metabolism (Koch, 1996; Rontein et al., 2002) whereas secondary metabolism is often connected to interactions with environmental cues, including cell signaling, interspecies communication, and responses to biotic and abiotic stress (see for example Wink, 1988; Mitchell-Olds and Pedersen, 1998; Lehmann et al., 2009; Rubin et al., 2009). Although primary metabolic pathways are strongly conserved between species, quantitative variation is often observed, possibly related to the different growth characteristics of various species (Mitchell-Olds and Pedersen, 1998). Qualitative and quantitative variation in secondary metabolism is, however, much more extensive and it is widely accepted that secondary metabolism determines to a great extent the success of plant adaptation (Herms and Mattson, 1992; Pichersky and Gang, 2000). As stated above, thousands of different metabolites can be found in a single plant species (De Luca and St Pierre, 2000; Sumner et al., 2003; D’Auria and Gershenzon, 2005; Fernie, 2007). That said, we are only starting to explore the composition of the metabolome, let alone unravel all of the biosynthetic pathways leading to this diversity of chemical structures.
In the current article we concentrate on results from broad screening of the natural genetic diversity of metabolism in Arabidopsis rosettes and tomato fruit. Following intensive statistical analysis, clear patterns of metabolic regulation can be demarcated via these approaches and a sub-set of these patterns can be resolved at the genetic level. We conclude that the screening of diverse genetic populations by metabolic profiling significantly adds to our understand metabolic regulation.
Metabolic Variance in Arabidopsis
In recent years much research has exploited natural variance in the pre-eminent model species Arabidopsis thaliana (Kliebenstein et al., 2001; Koornneef et al., 2004; Weigel and Nordborg, 2005; Borevitz et al., 2007; Alonso-Blanco et al., 2009). Study of metabolic traits in Arabidopsis largely focuses on understanding the principles underlying metabolic regulation and the influence of metabolism on growth and development (Kliebenstein et al., 2001; Keurentjes et al., 2006; Meyer et al., 2007; Lisec et al., 2008, 2009; Rowe et al., 2008; Sulpice et al., 2009, 2010). As for all quantitative traits, those associated with metabolism are characterized by continuous variation. Establishment of the genetic basis of quantitative traits commonly referred to as quantitative trait loci (QTL), has often been hampered due to their complex multigenic inheritance and strong interactions with the environment. The principle of QTL mapping in segregating populations is based on genotyping of progeny derived from a cross between distinct genotypes for the trait under study. Phenotypic values for the trait are then compared with molecular markers in the progeny to search for particular genomic regions showing statistically significant associations with the trait variation (Broman, 2001; Slate, 2005). Over the past few decades, the field has benefited enormously from the progress made in molecular marker technology. The ease with which such markers can be developed has facilitated QTL mapping studies of even the most complex traits (Borevitz and Nordborg, 2003).
Quantitative trait loci analysis makes use of the natural variation present within species (Alonso-Blanco and Koornneef, 2000; Maloof, 2003; Fernie et al., 2006) and has been successfully applied to various types of segregating populations. In plants, the use of “immortal” mapping populations consisting of homozygous individuals that, at least theoretically, can be propagated indefinitely is preferred because it permits replication and multiple analyses of the same population. Homozygous populations can be obtained by repeated selfing, as is the case for recombinant inbred lines (RILs), but also by induced chromosomal doubling of haploids, such as for doubled haploids (DHs; Han et al., 1997; Rae et al., 1999; von Korff et al., 2004). RILs are likely advantageous over DHs since they are characterized by a higher frequency of recombination within the population, resulting from multiple meiotic events occurred during repeated selfing (Jansen, 2003; Keurentjes and Fernie, 2011).
Another type of immortal population consists of introgression lines (ILs; Eshed and Zamir, 1994), which are obtained through repeated backcrossing and extensive genotyping. These are also referred to as near isogenic lines (NILs; Monforte and Tanksley, 2000), or backcross inbred lines (BILs; Jeuken and Lindhout, 2004; Blanco et al., 2006). These lines contain a single or a small number of genomic introgression fragments from a donor parent into an otherwise homogeneous genetic background. In plants, RILs and NILs are the most common types of experimental populations used for the analysis of quantitative traits (for an illustration of these populations see Figure 1). In both cases the accuracy of QTL localization, referred to as mapping resolution, depends on population size. For RILs, the position of the recombination event is fixed and can therefore only be increased within the population by adding more lines (i.e., more independent recombination events). Alternatively, recombination frequency can be increased by intercrossing lines before fixation as homozygous lines by inbreeding (Zou et al., 2005; Balasubramanian et al., 2009). In NIL populations resolution can be improved by minimizing the introgression size of each NIL. Consequently, to maintain genome-wide coverage either a larger number of lines or a high proportion of overlapping regions, or both, are needed. Despite the similarities between these two types of mapping populations, large differences exist in the genetic makeup of the respective individuals and the resulting mapping approach. In general, recombination frequency in RIL populations is higher than in equally sized NIL populations, allowing analysis of fewer individuals. Each RIL contains several introgressed fragments and, on average, each genomic region is represented by an equal number of both parental genotypes in the population. Therefore, replication of individual lines is often not necessary because the effect of each genomic region on phenotypic traits is independently tested multiple times by comparing the two genotypic RIL classes. In addition, the multiple introgressions per RIL can potentially reveal epistasis between loci. However, this may negatively bias the power to detect QTL. Furthermore, the wide variation of morphological and developmental traits among individuals within most RIL populations may hamper analysis of traits requiring the same growth and developmental stage of the individual lines. When many traits segregate simultaneously, this often affects the expression of other traits due to genetic interactions. By contrast, NILs preferably contain only a single introgressed segment per line, increasing the power to detect small-effect QTL. However, the presence of a single introgressed segment limits testing for genetic interactions and thereby the detection of epistatic QTL. Since most of the genetic background is identical for all lines, NILs show more limited developmental and growth variation, increasing the homogeneity of growth stage within experiments.
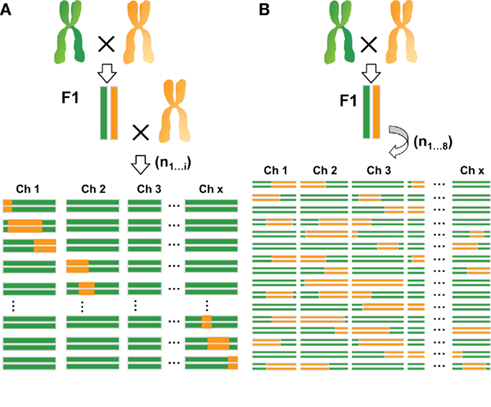
Figure 1. Comparison of introgression (A) and recombinant inbred lines (B). Introgression lines are created by backcrossing an F1 of a cross between two parental lines to a recurrent parent for several times. Homozygous individuals containing single introgressions are then selected from the progeny. Recombinant inbred lines are generated by selfing an F1 for at least eight generations when full homozygosity is reached. Each individual of the population contains multiple introgressions. Recombinant inbred lines allow for the testing of epistasis. Also because of the higher recombination frequency they often offer higher resolution than introgression lines. Introgression lines, however, often display greater statistical power in the detection of small-effect QTL.
In Arabidopsis, the ease of generating fertile RIL populations with complete genome coverage has led to their extensive use in QTL mapping (O’Neill et al., 2008). NILs have also been developed to confirm and fine map QTL previously identified in RILs (Alonso-Blanco et al., 1998, 2003; Swarup et al., 1999; Bentsink et al., 2003; Edwards et al., 2005; Juenger et al., 2005; Teng et al., 2005). Genome-wide sets of NILs and RILs, descending from identical intercrosses, that allow mapping to chromosomal sections have been described in Arabidopsis and empirical comparative studies have been performed between the two population types (Keurentjes et al., 2007a; Lisec et al., 2008, 2009). These studies illustrate the complementary benefits of both resources, facilitating the genetic dissection of various quantitative traits in Arabidopsis. RIL populations allow mapping at higher resolution, whilst NILs have the advantage of detecting small-effect QTL (Keurentjes and Fernie, 2011). Having extensively described the approaches, we now detail the biological significance of results obtained to date in Arabidopsis.
Although many simple metabolic traits affecting protein, oil, and starch content have been studied using targeted approaches (see Moose and Mumm, 2008; Fernie and Schauer, 2009), the adoption of methods able to detect the levels of multiple metabolites at once greatly expanded our ability to pose questions about regulation at the pathway and network level (Sweetlove et al., 2008; Stitt et al., 2010). Studies, largely reliant on gas chromatography–mass spectrometry (GC–MS) or liquid chromatography–mass spectrometry (LC–MS) have been carried out in Arabidopsis by three different research groups.
The first publication of note focused on a RIL population derived from a cross between the Landsberg erecta (Ler) and Cape Verde Islands (Cvi) accessions and evaluated the variance of some 2000 mass peaks across this population (Keurentjes et al., 2006). Interestingly, almost one-third of these peaks were not detected in either parent, implying a vast potential for manipulation of chemotypes via classical breeding. Another intriguing finding of this study was the fact that colocation of QTL coincided with clusters of highly correlated mass peaks. For example, several glucosinolate QTL co-clustered with one another. Comparison of these data with those of previous targeted work showed that these co-locations were at positions of known regulators of glucosinolate metabolism to which Peptide methionine sulfoxide reductase (MAM) and QTL for production of alkenyl or hydroxyalkyl glucosinolates (AOP) mapped (Kliebenstein et al., 2001; Kroymann et al., 2001). Further work from this group focused on parallel analysis of 15 enzyme activities in tandem with their corresponding transcript levels and a set of relevant metabolites. The results revealed that traits affecting primary metabolism are often correlated and many activity QTL co-localize with expression QTL (although a fair number do not, suggesting that such multilevel approaches will be highly useful for distinguishing between transcriptional and metabolic control (Keurentjes et al., 2008). As an extension of this work they next performed a multiplexed transcriptome, proteome, and metabolome study on the same material, combining these data with publically available data (Fu et al., 2009). The surprising finding of this study was that following mapping of over 40000 molecular and 100 phenotypic traits, there were only six QTL hotspots. The authors concluded that there are thus only six breakpoints in a system otherwise buffered against many of the half-million single nucleotide polymorphisms (SNPs) between the parental lines (Fu et al., 2009).
Following a similar approach to that mentioned above, groups at the Max-Planck-Institute in Golm focused on primary metabolism. Two different strategies were employed; the study of RILs and NILs resulting from a cross between Col-0 and C24 (Meyer et al., 2007; Lisec et al., 2008; Brotman et al., 2011) and analysis of the natural variance of metabolite accumulation inherent in ecotypes (Cross et al., 2006; Sulpice et al., 2007, 2009, 2010; Keurentjes et al., 2008). In the first approach, metabolites were not treated in isolation but rather evaluated with respect to the influence they exerted toward plant growth – with a metabolic signature for high growth being defined from results obtained in the Col-0/C24 RILs (Meyer et al., 2007). A more detailed study examined both RILs and NILs derived from the same parents (Lisec et al., 2008), revealing a couple of hotspots in which yield QTL overlapped with a large number of metabolite accumulation QTL. In this study, metabolic pathway-derived candidate genes were found for 24–67% of all tested metabolite QTL in the database AraCyc 3.5, demonstrating the power of this approach to identify possible sites of metabolic regulation. It is, however, important to note that this is only the first step and considerable further experimentation is required to confirm the existence and physiological relevance of such regulations.
A recent paper describes the identification of a cytosolic isoform of fumarase as the causal gene underlying traits of decreased fumarate and increased malate (Brotman et al., 2011). This result represents an elegant proof-of-concept study and beautifully fits the cross-over theorem, which states that if an equilibrium reaction of a linear pathway is inhibited, a build-up of substrate and a depletion of product of that reaction will occur (Rolleston, 1972). An illustration of the power of this theorem is shown in its application to starch synthesis in potato, which prompted a successful search for a novel post-translational modifier of the AGPase enzyme (Stitt et al., 2010). Bearing this example in mind, analyses of metabolite ratios in advanced genetic populations will likely prove to be an important route to identify previously uncharacterized mechanisms of metabolic regulation in the future. The rapidly increasing availability of genome information for multiple Arabidopsis ecotypes (Weigel and Mott, 2009; Schneeberger and Weigel, 2011), alongside the increase in the number of groups performing metabolomic analyses should accelerate gene discovery.
A second approach to identification of metabolic regulators is comparative analysis of various Arabidopsis ecotypes. This approach was initiated to assess the natural variance in enzyme activities; the first study examined the activities of seven enzymes across 24 Arabidopsis ecotypes (Cross et al., 2006) whilst the second evaluated the activity of the most abundant protein in photosynthetic tissues, Ribulose-1,5-bisphosphate-carboxylase/-oxygenase (Rubisco), across the abovementioned Col-0/C24 RIL population (Sulpice et al., 2007). In the former study it was observed that enzyme activities largely vary on mass, but are not well correlated to the levels of the metabolites measured in the same sample (Cross et al., 2006). This observation is consistent with the concept that metabolism is highly regulated at multiple levels. The latter study described application of a novel Rubisco assay to describe the characteristics of this enzyme in 118 Arabidopsis accessions, defining two loci for Rubisco activity and two for Rubisco activation state (Sulpice et al., 2007). These analyses were subsequently massively expanded to encompass either 94 or 112 accessions as well as quantification of select metabolites in an attempt to establish the major integrator of growth within the species (Sulpice et al., 2009, 2010). The results of these analyses spotlighted starch and protein biosynthesis as the major controlling factors with respect to total biomass (Sulpice et al., 2009, 2010). Transcript profiling in 21 accessions further revealed coordinated changes in expression of more than 70 carbon-regulated genes, identifying two (myo-inositol-1-phosphate synthase and a Kelch-domain protein) whose transcripts correlate with biomass. The impact of allelic variation at these two loci was shown by association mapping, identifying them as candidate genes to increase biomass production.
Kliebenstein and co-workers have also taken both association mapping and QTL-based approaches in their studies, profiling both primary and secondary metabolites alongside studies of gene expression (Kliebenstein et al., 2006; Rowe et al., 2008; Kliebenstein, 2009; Chan et al., 2010). They have expended considerable effort to understand whole genome expression QTL, revealing a high incidence of both cis- and trans-acting QTL, including non-additive variation such as epistasis and transgressive segregation as well as genetic variation affecting entire transcriptional networks (Kliebenstein et al., 2006; Kliebenstein, 2009). They additionally linked this variation to phenotypic alterations in secondary metabolite content (Wentzell et al., 2007; Hansen et al., 2008) and more recently profiled primary metabolites (Rowe et al., 2008) in a 210 member RIL population. Statistical analysis of the resultant dataset suggested that epistatic interactions control a majority of the variation in the network of plant primary metabolism. They also identified 11 metabolite QTL hotspots, two of which overlapped the AOP and MAM loci previously characterized as QTL for glucosinolate accumulation.
Taking this analysis one step further, the authors constructed two biochemical networks de novo; however, it is too early to judge the accuracy of such an approach. The group subsequently profiled some 327 metabolites against greater than 200 000 SNPs (Chan et al., 2010). However, comparison of the resultant data from this study and that described above on the RIL population revealed that the higher level of genetic variation in the accession population was not reflected by a higher variation in the metabolome. They suggest that evolutionary constraints limit metabolic variation. Another important finding of this study is the large environmental influence on metabolite levels. Clearly such studies must be conducted in a range of environmental conditions since not only do individual metabolites change with the environment but the entire network behavior changes as well.
Metabolic Variance in Crop Species
While Arabidopsis is the best characterized plant species with respect to natural variation in the metabolome, an increasing number of studies are being carried out in crop species. Unfortunately, a limited number of these studies have addressed environmental influences across independent harvests and/or locations. Nonetheless, considerable information has been gleaned from single-harvest studies (see for example Fraser et al., 2007; Kusano et al., 2007). Despite these limitations, studies on rice, the staple food crop of almost half of the world’s population (Hall et al., 2008), are particularly pertinent from an applied perspective. Kusano et al. (2007) recently profiled a total of 70 rice cultivars using a combination of two-dimensional GC–MS yielding a highly accurate inventory of the nutritional value of these cultivars. Similar smaller scale studies have also been carried out in sesame, broccoli, and mustard (Magrath et al., 1993; Laurentin et al., 2008; Rochfort et al., 2008). Adoption of MALDI/TOF-MS to individual mutagenized plants and Solanum pennellii ILs has been used for screening of fruit containing high levels of nutraceutical compounds such as carotenoids (Fraser et al., 2007). These studies all reveal large diversity within populations at the genetic and metabolic levels and hint that such information is likely of high value for breeding programs (Fernie and Schauer, 2009). As we will discuss in the following paragraphs it is also highly interesting material in which to study metabolic regulation.
There are examples of multi-harvest replication of metabolomic studies in crop species. To date the majority of these have been focused on tomato and these studies are the focus of this section. However, interesting examples in maize (Harrigan et al., 2007a,b; Zhang et al., 2010; Lisec et al., 2011), Lolium perenne (Koulman et al., 2009), and wheat (Hazehzarghani et al., 2008) will also be discussed. In maize a range of compositional traits including protein, oil, fatty acid, amino acid, and organic acid content was carried out in two independent maize hybrids grown at three separate locations (Harrigan et al., 2007a,b). This important proof-of-concept study demonstrated the high non-genetic variability in crop composition, illustrating the need for replicated trials. More recently a comparative analysis of the root metabolome of six parental maize inbred lines and their corresponding 14 hybrids was performed (Lisec et al., 2011). The metabolic profile of each hybrid when compared to its parents is distinct and even reciprocal hybrids are easily distinguished. Reconstructed metabolic networks display a higher network density in most hybrids as compared to the corresponding inbred lines, suggesting that metabolite levels are subject to tighter control. On a broader scope, a maize diversity panel was screened for 10 key enzyme activities and heritable variation was found in each one (Zhang et al., 2010). Association mapping subsequently identified a novel amino acid substitution associated with a variation in isocitrate dehydrogenase activity, demonstrating that this approach can identify putative functional sites. A later study of the same 10 enzymes across a maize intermated B73 × Mo17 mapping population provided almost a four-fold increase in genetic map distance compared with conventional mapping populations (Zhang et al., 2011). In total, 73 significant QTL that influence the activity of these 10 enzymes as well as 8 QTL that influence biomass were identified. While some QTL were shared by different enzymes or biomass, the authors critically evaluated the probability that this may be fortuitous. All enzyme activity QTL were in trans to the known genomic locations of structural genes (i.e., genes that encode enzymes operating within the pathways), except for single cis-QTL for nitrate reductase, glutamate dehydrogenase, and shikimate dehydrogenase; the low frequency and low additive magnitude compared with trans-QTL indicates that, at least in this population, cis-regulation is relatively unimportant versus trans-regulation.
Returning to metabolite content QTL, large datasets have been obtained for L. perenne populations (Rasmussen et al., 2008), as well as wheat infected with Fusarium head blight (Hazehzarghani et al., 2008). By far the best characterized crop system, however, is tomato (Klee, 2010; Keurentjes and Fernie, 2011). In this species, a broad profiling of fruit volatiles, which are extremely important flavor components, in a population consisting of 74 Solanum lycopersicum × S. pennellii ILs yielded 100 QTL that were conserved across harvests (Tieman et al., 2006b). Metabolic and flux profiling of one of these QTL was instrumental in defining the pathway for synthesis of important phenylalanine-derived aromatic compounds in the fruit (Tieman et al., 2006a). Thirty additional QTL that affect the volatile emissions of red-ripe fruit were identified in a second population ofILs derived from a cross between S. lycopersicum and S. habrochaites grown in multiple seasons and locations (Mathieu et al., 2010). The same population has also recently been characterized for QTL for ripening-associated ethylene release (Dal Cin et al., 2009) and used to define a novel pathway for sesquiterpene biosynthesis from Z,Z-farnesyl pyrophosphate (Sallaud et al., 2009). In other studies of note, the volatile metabolite composition of some 300 compounds was determined across a population of 94 elite cultivars of tomato (Tikunov et al., 2005), whilst the primary metabolite composition of five wild species of tomato was assessed in comparison to the cultivated tomato (Schauer et al., 2005). These studies provide important inventories of the metabolic differences between genotypes. That said, it will be some time before our knowledge is sufficiently advanced that we can facilely use such information for predictive breeding (Keurentjes and Fernie, 2011). Similar, albeit not quite so extensive, studies were performed on intraspecific crosses of S. lycopersicum cultivars (Causse et al., 2002), and have subsequently been validated in replicated experiments (Zanor et al., 2009). The same S. pennellii ILs described above were profiled using an established GC–MS method in replicated harvests resulting in identification of 889 QTL covering 74 metabolites including important primary metabolites such as sugars and organic acids as well as essential amino acids, intermediate metabolites and vitamins (Schauer et al., 2006). It is important to note that despite the fact that in many cases metabolite content was elevated, the vast majority of these QTL were associated with a yield penalty. In a subsequent study the heritability of these traits were established (Schauer et al., 2008). For this purpose, the S. penellii ILs were grown alongside lines heterozygous for the introgression (ILHs) allowing evaluation of both heritability and the mode of inheritance. These studies revealed that mean heritability of the metabolite QTL was generally relatively low (as in Arabidopsis; Rowe et al., 2008). However, a handful of the traits were nevertheless highly conserved and displayed reasonable heritability. Comparative study of the ILs and ILHs revealed that most of the metabolic QTL were dominant with a considerable number displaying additive or recessive mode of action and only a negligible number displaying overdominant phenotypes. Interestingly, the mode of inheritance was quantitatively different between diverse compound classes and several metabolite pairs displayed a similar mode of inheritance at the same chromosomal loci, suggesting that the variation is likely to be mediated by enzymes involved in their interconversion (Schauer et al., 2008).
The S. pennellii ILs have also been characterized for trichome specialized metabolites including terpenes, flavonoids, and acyl sugars (Schilmiller et al., 2010). Metabolite profiling led to the discovery of ILs producing different acyl chain substitutions on acyl sugar metabolites as well as two regions quantitatively influencing acyl sugar content. A QTL that influenced the types of glycoalkaloids was also identified. These results illustrate the power of QTL mapping for identification of novel enzymatic functions and pathways. Following a similar approach Tieman et al. (2006b) used ILs and reverse genetics in combination with volatile and isotope tracer analysis to establish the route of 2-phenylethanol and phenylacetaldehyde biosynthesis in tomato. A second flavor volatile QTL responsible for synthesis of methylsalicylate was shown to be the consequence of altered expression QTL (eQTL) of the gene encoding the biosynthetic enzyme, salicylic acid methyltransferase (Tieman et al., 2010).
Another important agronomic property in tomato, total soluble solids content, has been defined at the genetic level. An early study utilizing the S. pennellii ILs mapped the moderate QTL Brix 9-2-5 to a 484-bp region of the cell wall invertase gene LIN5 (Fridman et al., 2000), although there was no difference in expression or protein content of LIN5 in the IL harboring this QTL (Fridman et al., 2004). QTL analysis of five different tomato species delimited the functional polymorphism of Brix 9-2-5 to an amino acid at the fructosyl binding site near the catalytic site of the invertase crystal with enzyme kinetic analysis of recombinant protein demonstrating that the S. pennellii allele was more efficient in degrading sucrose (Fridman et al., 2004). Subsequent experiments involving RNA interference of this isoform resulted in reduced Brix and sink strength, thus confirming the results obtained in the heterologous system (Zanor et al., 2009). Currently, optimized assays (Steinhauser et al., 2010) for a set of key enzymes of central metabolism are being used to profile the S. pennellii ILs. Once finished it will be highly informative to integrate these analyses with those of the metabolites.
Further proof of the value of metabolic QTL analysis is illustrated by studies on branched chain amino acid metabolism for which four co-ordinate QTL were identified (Schauer et al., 2006). As a first approach we focused on branched chain aminotransferases (BCATs), mapping all six members of the family. BCAT1 is an eQTL whereas BCAT4 is a protein quality QTL (i.e., a QTL that does not affect protein abundance but rather its relative efficiency within a given biological process; Maloney et al., 2010). We next mapped a further 22 putative gene functions associated with branched chain amino acid metabolism. Mapping the chromosomal locations of these enzymes, it was possible to define the map positions of 24 genes (with two of the putative gene functions being encoded by two independent genes). Eight co-localized with BCAA QTL including those encoding ketol-acid reductoisomerase (KARI), dihydroxy-acid dehydratase (DHAD), and isopropylmalate dehydratase (IPMD; Kochevenko and Fernie, 2011). Quantitative evaluation of the expression of these genes revealed that the S. pennellii allele exhibited altered expression of IPMD, whereas expression of KARI and DHAD were invariant across the genotypes. Whilst the antisense inhibition of IPMD resulted in increased BCAA, the antisense inhibition of KARI or DHAD had no effect on fruit BCAA contents (Kochevenko and Fernie, 2011).
Concluding Remarks
The above examples in both Arabidopsis and crop species illustrate how forward genetic approaches based on natural variation can produce high resolution information concerning metabolic regulation. This is the case at the levels of the individual enzyme, whole pathways, and even metabolic networks. They furthermore, demonstrate how natural variance is an undermined resource of biochemical diversity and one that will certainly be an important resource for breeding approaches toward metabolic engineering (Fernie et al., 2006). Given the ever increasing number of crop species for which full-genome sequences are becoming available it is highly likely that such studies will be greatly aided by the development of translational approaches both at the molecular (Mutwil et al., 2011) and phenotyping levels (Tohge and Fernie, 2010). As yet one drawback of this approach is that it is considerably slower than jumping straight into using reverse genetics. That said, the development of a wide array of genetic materials including those resulting from TILLING (Till et al., 2006) and the adoption of every more sophisticated rapid screening technologies such as those afforded by viral induced gene silencing (Ruiz et al., 1998; Quadrana et al., 2011) will likely considerably accelerate this process. Regardless, the forward genetic approach has at least two advantages (i) by taking a top-down approach it may uncover different levels of metabolic regulation within an experiment and (ii) given that the whole genome is considered, this approach is not restricted to evaluation of previously known enzymes or regulators thereof. This approach is entirely complementary to the currently more commonly used reverse genetic approach. While the forward genetic approach is in its infancy we are convinced that it will prove a highly powerful tool for the identification of novel mechanisms of metabolic regulation.
Conflict of Interest Statement
The authors declare that the research was conducted in the absence of any commercial or financial relationships that could be construed as a potential conflict of interest.
Acknowledgments
We thank Dr. Takayuki Tohge for help with Figure 1.
References
Alonso-Blanco, C., Aarts, M. G., Bentsink, L., Keurentjes, J. J., Reymond, M., Vreugdenhil, D., and Koornneef, M. (2009). What has natural variation taught us about plant development, physiology, and adaptation? Plant Cell 21, 1877–1896.
Alonso-Blanco, C., Bentsink, L., Hanhart, C. J., Blankestijn-de Vries, H., and Koornneef, M. (2003). Analysis of natural allelic variation at seed dormancy loci of Arabidopsis thaliana. Genetics 164, 711–729.
Alonso-Blanco, C., El-Assal, S. E., Coupland, G., and Koornneef, M. (1998). Analysis of natural allelic variation at flowering time loci in the Landsberg erecta and Cape Verde Islands ecotypes of Arabidopsis thaliana. Genetics 149, 749–764.
Alonso-Blanco, C., and Koornneef, M. (2000). Naturally occurring variation in Arabidopsis: an underexploited resource for plant genetics. Trends Plant Sci. 5, 22–29.
Araújo, W. L., Ishizaki, K., Nunes-Nesi, A., Larson, T. R., Tohge, T., Krahnert, I., Witt, S., Obata, T., Schauer, N., Graham, I. A., Leaver, C. J., and Fernie, A. R. (2010). Identification of the 2-hydroxyglutarate and isovaleryl-CoA dehydrogenases as alternative electron donors linking catabolism to the electron transport chain of Arabidopsis mitochondria. Plant Cell 22, 1549–1563.
Araújo, W. L., Tohge, T., Ishizaki, K., Leaver, C. J., and Fernie, A. R. (2011). Protein as an alternative respiratory substrate for stressed plants. Trends Plant Sci. 16, 489–498.
Balasubramanian, S., Schwartz, C., Singh, A., Warthmann, N., Kim, M. C., Maloof, J. N., Loudet, O., Trainer, G. T., Dabi, T., Borevitz, J. O., Chory, J., and Weigel, D. (2009). QTL mapping in new Arabidopsis thaliana advanced intercross-recombinant inbred lines. PLoS ONE 4, e4318. doi: 10.1371/journal.pone.0004318
Bentsink, L., Yuan, K., Koornneef, M., and Vreugdenhil, D. (2003). The genetics of pytate and phosphate accumulation in seeds and leaves of Arabidopsis thaliana, using natural variation. Theor. Appl. Genet. 106, 1234–1243.
Blanco, A., Simeone, R., and Gadaleta, A. (2006). Detection of QTL for grain protein content in durum wheat. Theor. Appl. Genet. 112, 1195–1204.
Borevitz, J. O., Hazen, S. P., Michael, T. P., Morris, G. P., Baxter, I. R., Hu, T. T., Chen, H., Werner, J. D., Nordborg, M., Salt, D. E., Kay, S. A., Chory, J., Weigel, D., Jones, J. D., and Ecker, J. R. (2007). Genome-wide patterns of single-feature polymorphism in Arabidopsis thaliana. Proc. Natl. Acad. Sci. U.S.A. 104, 12057–12062.
Borevitz, J. O., and Nordborg, M. (2003). The impact of genomics on the study of natural variation in Arabidopsis. Plant Physiol. 132, 718–725.
Bräutigam, A., Kajala, K., Wullenweber, J., Sommer, M., Gagneul, D., Weber, K. L., Carr, K. M., Gowik, U., Mass, J., Lercher, M. J., Hibberd, J. M., and Weber, A. P. (2011). An mRNA blueprint for C4 photosynthesis derived from comparative transcriptomics of closely related C3 and C4 species. Plant Physiol. 155, 142–156.
Broman, K. W. (2001). Review of statistical methods for QTL mapping in experimental crosses. Lab. Anim. (NY) 30, 44–52.
Brotman, Y., Riewe, D., Lisec, J., Meyer, R. C., Willmitzer, L., and Altmann, T. (2011). Identification of enzymatic and regulatory genes of plant metabolism through QTL analysis in Arabidopsis. J. Plant Physiol. 168, 1387–1394.
Buchner, E. (1907). Noble Prize Acceptance Speech. Available at: http://nobelprize.org/nobel_prizes/chemistry/laureates/1907/buchner-lecture.html
Causse, M., Saliba-Colombani, V., Lecomte, L., Duffe, P., Rousselle, P., and Buret, M. (2002). QTL analysis of fruit quality in fresh market tomato: a few chromosome regions control the variation of sensory and instrumental traits. J. Exp. Bot. 53, 2089–2098.
Chan, E. K., Rowe, H. C., Hansen, B. G., and Kliebenstein, D. J. (2010). The complex genetic architecture of the metabolome. PLoS Genet. 6, e1001198. doi: 10.1371/journal.pgen.1001198
Cross, J. M., von Korff, M., Altmann, T., Bartzetko, L., Sulpice, R., Gibon, Y., Palacios, N., and Stitt, M. (2006). Variation of enzyme activities and metabolite levels in 24 Arabidopsis accessions growing in carbon-limited conditions. Plant Physiol. 142, 1574–1588.
Dal Cin, V., Kevany, B., Fei, Z. J., and Klee, H. J. (2009). Identification of Solanum habrochaites loci that quantitatively influence tomato fruit ripening-associated ethylene emissions. Theor. Appl. Genet. 119, 1183–1192.
D’Auria, J. C., and Gershenzon, J. (2005). The secondary metabolism of Arabidopsis thaliana: growing like a weed. Curr. Opin. Plant Biol. 8, 308–316.
De Luca, V., and St Pierre, B. (2000). The cell and developmental biology of alkaloid biosynthesis. Trends Plant Sci. 5, 168–173.
Dennis, D. T., Turpin, D. H., Lefebvre, D. D., and Layzell, D. B. (1997). Plant Metabolism. Singapore: Longman.
Edwards, K. D., Lynn, J. R., Gyula, P., Nagy, F., and Millar, A. J. (2005). Natural allelic variation in the temperature-compensation mechanisms of the Arabidopsis thaliana circadian clock. Genetics 170, 387–400.
Eshed, Y., and Zamir, D. (1994). A genomic library of Lycopersicon pennellii in Lycopersicon esculuntem – a tool for fine mapping of genes. Euphytica 79, 175–179.
Fernie, A. R. (2007). The future of metabolic phytochemistry: larger numbers of metabolites, higher resolution, greater understanding. Phytochemistry 68, 2861–2680.
Fernie, A. R., Roscher, A., Ratcliffe, R. G., and Kruger, N. J. (2001). Fructose 2, 6-bisphosphate activates pyrophosphate: fructose-6-phosphate 1-phosphotransferase and increases triose phosphate to hexose phosphate cycling in heterotrophic cells. Planta 212, 250–263.
Fernie, A. R., and Schauer, N. (2009). Metabolomics-assisted breeding: a viable option for crop improvement? Trends Genet. 25, 39–48.
Fernie, A. R., Tadmor, Y., and Zamir, D. (2006). Natural genetic variation for improving crop quality. Curr. Opin. Plant Biol. 9, 196–202.
Fernie, A. R., Trethewey, R. N., Krotzky, A. J., and Willmitzer, L. (2004a). Metabolite profiling: from diagnostics to systems biology. Nat. Rev. Mol. Cell Biol. 5, 763–769.
Fernie, A. R., Carrari, F., and Sweetlove, L. J. (2004b). Respiratory metabolism: glycolysis, the TCA cycle and mitochondrial electron transport. Curr. Opin. Plant Biol. 7, 254–261.
Fraser, P. D., Enfissi, E. M., Goodfellow, M., Eguchi, T., and Bramley, P. M. (2007). Metabolite profiling of plantcarotenoids using the matrix-assisted laser desorption ionization time-of-flight mass spectrometry. Plant J. 49, 552–564.
Fridman, E., Carrari, F., Liu, Y. S., Fernie, A. R., and Zamir, D. (2004). Zooming in on a quantitative trait for tomato yield using interspecific introgressions. Science 305, 1786–1789.
Fridman, E., Pleban, T., and Zamir, D. (2000). A recombination hotspot delimits a wild-species quantitative trait locus for tomato sugar content to 484 bp within an invertase gene. Proc. Natl. Acad. Sci. U.S.A. 97, 4718–4723.
Fu, J., Keurentjes, J. J., Bouwmeester, H., America, T., Verstappen, F. W., Ward, J. L., Beale, M. H., de Vos, R. C., Dijkstra, M., Scheltema, R. A., Johannes, F., Koornneef, M., Vreugdenhil, D., Breitling, R., and Jansen, R. C. (2009). System-wide molecular evidence for phenotypic buffering in Arabidopsis. Nat. Genet. 41, 166–167.
Gachon, M. M., Langlois-Meurinne, M., and Saindrenan, P. (2005). Plant secondary metabolism glycosyltransferases: the emerging functional analysis. Trends Plant Sci. 10, 542–549.
Gonzalez-Ballester, D., Casero, D., Cokus, S., Pellegrini, M., Merchant, S. S., and Grossman, A. R. (2010). RNA-seq analysis of sulfur-deprived Chlamydomonas cells reveals aspects of acclimation critical for cell survival. Plant Cell 22, 2058–2084.
Hall, R. D., Brouwer, I. D., and Fitzgerald, M. A. (2008). Plant metabolomics and its potential application for human nutrition. Physiol. Plant. 132, 162–175.
Han, F., Ullrich, S. E., Kleinhofs, A., Jones, B. L., Hayes, P. M., and Wesenberg, D. M. (1997). Fine structure mapping of the barley chromosome-1 centromere region containing malting-quality QTL. Theor. Appl. Genet. 95, 903–910.
Hansen, B. G., Kerwin, R. E., Ober, J. A., Lambrix, V. M., Mitchell-Olds, T., Gershenzon, J., Halkier, B. A., and Kliebenstein, D. G. (2008). A novel 2-oxoacid-dependent dioxygenase involved in the formation of the goiterogenic 2-hydroxybut-3-enyl glucosinolate and generalist insect resistance in Arabidopsis. Plant Physiol. 148, 2096–2108.
Harrigan, G. G., Stork, L. G., Riordan, S. G., Reynolds, T. L., Ridley, W. P., Masucci, J. D., Macisaac, S., Halls, S. C., Orth, R., Smith, R. G., Wen, L., Brown, W. E., Welsch, M., Riley, R., McFarland, D., Pandravada, A., and Glenn, K. C. (2007a). Impact of genetics and environment on nutritional and metabolite components of maize grain. J. Agric. Food Chem. 55, 6177–6185.
Harrigan, G. G., Stork, L. G., Riordan, S. G., Ridley, W. P., Macisaac, S., Halls, S. C., Orth, R., Rau, D., Smith, R. G., Wen, L., Brown, W. E., Riley, R., Sun, D., Modiano, S., Pester, T., Lund, A., and Nelson, D. (2007b). Metabolite analyses of grain from maize hybrids grown in the United States under drought and watered conditions during the 2002 field season. J. Agric. Food Chem. 55, 6169–6176.
Hazehzarghani, H., Paranidharan, V., Abu-Nada, Y., Kushalappa, A. C., Mamer, O., and Somers, D. (2008). Metabolic profiling to discriminate wheat near isogenic lines, with quantitative trait loci at chromomsome 2DL, varying in resistance to fusarium head blight. Can. J. Plant Sci. 88, 789–797.
Herms, D. A., and Mattson, W. J. (1992). The dilemma of plants: to grow or defend. Q. Rev. Biol. 67, 283–335.
Hirai, M. Y., Klein, M., Fujikawa, Y., Yano, M., Goodenowe, D. B., Yamazaki, Y., Kanaya, S., Nakamura, Y., Kitayama, M., Suzuki, H., Sakurai, N., Shibata, D., Tokushisa, J., Reichelt, M., Gershenzon, J., Papenbrock, J., and Saito, K. (2005). Elucidation of gene-to-gene and metabolite-to-gene networks in Arabidopsis by integration of metabolomics and transcriptomics. J. Biol. Chem. 27, 25590–25595.
Hirai, M. Y., Sugiyama, K., Sawada, Y., Tohge, T., Obayashi, T., Suzuki, A., Araki, R., Sakurai, N., Suzuki, H., Aoki, K., Goda, H., Nishizawa, O. I., Shibata, D., and Saito, K. (2007). Omics-based identification of Arabidopsis Myb transcription factors regulating aliphatic glucosinolate biosynthesis. Proc. Natl. Acad. Sci. U.S.A. 15, 6478–6483.
Hua, Z., and Vierstra, R. D. (2011). The Cullin-RING ubiquitin-protein ligases. Annu. Rev. Plant Biol. 62, 299–334.
Jansen, R. C. (2003). “Quantitative trait loci in inbred lines,” in Handbook of Statistical Genetics, eds D. J. Balding, M. Bishop, and C. Cannings (Chichester: John Wiley & Sons), 445–476.
Jeuken, M. J., and Lindhout, P. (2004). The development of lettuce backcross inbred lines (BILs) for exploitation of the Lactuca saligna (wild lettuce) germplasm. Theor. Appl. Genet. 109, 394–401.
Juenger, T. E., McKay, J. K., Hausmann, N., Keurentjes, J. J. B., Sen, S., Stowe, K. A., Dawson, T. E., Simms, E. L., and Richards, J. H. (2005). Identification and characterization of QTL underlying whole-plant physiology in Arabidopsis thaliana: delta13C, stomatal conductance and transpiration efficiency. Plant Cell Environ. 28, 697–708.
Keurentjes, J. J., and Fernie, A. R. (2011). Genetics, genomics and metabolomics. Annu. Plant Rev. 43, 219–259.
Keurentjes, J. J., Sulpice, R., Gibon, Y., Steinhauser, M. C., Fu, J., Koornneef, M., Stitt, M., and Vreugdenhil, D. (2008). Integrative analyses of genetic variation in enzyme activities of primary carbohydrate metabolism reveal distinct modes of regulation in Arabidopsis thaliana. Genome Biol. 9, R129.
Keurentjes, J. J. B., Bentsink, L., Alonso-Blanco, C., Hanhart, C. J., Blankestijn-De Vries, H., Effgen, S., Vreugenhil, D., and Koornneef, M. (2007a). Development of a near-isogenic line population of Arabidopsis thaliana and comparison of mapping power with a recombinant inbred line population. Genetics 175, 891–905.
Keurentjes, J. J. B., Fu, J., de Vos, C. H., Lommen, A., Hall, R. D., Bino, R. J., van der Plas, L. H., Vreugdenhil, D., and Koornneef, M. (2006). The genetics of plant metabolism. Nat. Genet. 38, 842–849
Klee, H. J. (2010). Improving the flavor of fresh fruits: genomics, biochemistry and biotechnology. New Phytol. 187, 44–56.
Kliebenstein, D. (2009). Advancing genetic theory and application by metabolic quantitative trait loci analysis. Plant Cell 21, 1637–1646.
Kliebenstein, D. J., Kroymann, J., Brown, P., Figuth, A., Pedersen, D., Gershenzon, J., and Mitchell-Olds, T. (2001). Genetic control of natural variation in Arabidopsis glucosinolate accumulation. Plant Physiol. 126, 811–825.
Kliebenstein, D. J., West, M. A., van Leeuwen, H., Kim, K., Doerge, R. W., Michelmore, R. W., and St. Clair, D. A. (2006). Genomic survey of gene expression diversity in Arabidopsis thaliana. Genetics 172, 1179–1189.
Koch, K. E. (1996). Carbohydrate-modulated gene expression in plants. Annu. Rev. Plant Physiol. Plant Mol. Biol. 47, 509–540.
Kochevenko, A., and Fernie, A. R. (2011). The genetic architecture of branched chain amino acid accumulation in tomato. J. Exp. Bot. 62, 3895–3906.
Koornneef, M., Alonso-Blanco, C., and Vreugdenhil, D. (2004). Naturally occurring genetic variation in Arabidopsis thaliana. Annu. Rev. Plant Physiol. Plant Mol. Biol. 55, 141–172.
Koulman, A., Cao, M., Faville, M., Lane, G., Mace, W., and Rasmussen, S. (2009). Semi-quantitative and structural metabolic phenotyping by direct infusion ion trap mass spectrometry and its application in genetical metabolomics. Rapid Commun. Mass Spectrom. 23, 2253–2263.
Krebs, H. A., and Henseleit, K. (1932). Untersuchungen über die Harnstoffbildung im tierkorper. Hoppe-Seyler’s Z. Physiol. Chem. 210, 33–66.
Kroymann, J., Textor, S., Tokushisa, J. G., Falk, K. L., Bartram, S., Gershenzon, J., and Mitchell-Olds, T. (2001). A gene controlling variation in Arabidopsis glucosinolate composition. Plant Physiol. 127, 1077–1088.
Kusano, M., Fukushima, A., Kobayashi, M., Hayashi, N., Jonsson, P., Moritz, T., Ebana, K., and Saito, K. (2007). Application of a metabolomic method combining one-dimensional and two-dimensional gas chromatography-time-of-flight/mass spectrometry to metabolic phenotyping of natural variants in rice. J. Chromatogr. B Analyt. Technol. Biomed. Life Sci. 855, 71–79.
Laurentin, H., Ratzinger, A., and Karlovsky, P. (2008). Relationship between metabolic and genomic diversity in sesame (Sesamum indicum L.). BMC Genomics 9, 250. doi: 10.1186/1471-2164-9-250
Lehmann, M., Schwarzlander, M., Obata, T., Sirikantaramas, S., Burow, M., Olsen, C. E., Tohge, T., Fricker, M. D., Moller, B. L., Fernie, A. R., Sweetlove, L. J., and Laxa, M. (2009). The metabolic response of Arabidopsis roots to oxidative stress is distinct from that of heterotrophic cells in culture and highlights a complex relationship between the levels of transcripts, metabolites, and flux. Mol. Plant 2, 390–406.
Lisec, J., Meyer, R. C., Steinfath, M., Redestig, H., Becher, M., Witucka-Wall, H., Fiehn, O., Törjék, O., Selbig, J., Altmann, T., and Willmitzer, L. (2008). Identification of metabolic and biomass QTL in Arabidopsis thaliana in a parallel analysis of RIL and IL populations. Plant J. 53, 960–972.
Lisec, J., Römisch-Margl, L., Nikoloski, Z., Piepho, H. P., Giavalisco, P., Selbig, J., Gierl, A., and Willmitzer, L. (2011). Corn hybrids display lower metabolite variability and complex metabolite inheritance patterns. Plant J. doi: 10.1111/j.1365-313X.2011.04689.x. [Epub ahead of print].
Lisec, J., Steinfath, M., Meyer, R. C., Selbig, J., Melchinger, A. E., Willmitzer, L., and Altmann, T. (2009). Identification of heterotic metabolite QTL in Arabidopsis thaliana RIL and IL populations. Plant J. 59, 777–788.
Lytovchenko, A., Sonnewald, U., and Fernie, A. R. (2007). The complex network of non-cellulosic carbohydrate metabolism. Curr. Opin. Plant Biol. 10, 227–235.
Magrath, R., Herron, C., Giamoustaris, A., and Mithen, R. (1993). The inheritance of aliphatic glucosinolates in Brassica napus. Plant Breed. 111, 55–72.
Maloney, G. S., Kochevenko, A., Tieman, D. M., Tohge, T., Krieger, U., Zamir, D., Taylor, M. G., Fernie, A. R., and Klee, H. J. (2010). Characterization of the branched-chain amino acid aminotransferase enzyme family in tomato. Plant Physiol. 153, 925–936.
Mathieu, S., Dal Cin, V., Fei, Z. J., Li, H., Bliss, P., Taylor, M. G., Klee, H. J., and Tiemann, D. M. (2010). Quantitative trait loci and potential pathways affecting volatile composition. J. Exp. Bot. 60, 325–337.
Meyer, R. C., Steinfath, M., Lisec, J., Becher, M., Witucka-Wall, H., Törjék, O., Fiehn, O., Eckardt, A., Willmitzer, L., Selbig, J., and Altmann, T. (2007). The metabolic signature related thigh plant growth rate in Arabidopsis thaliana. Proc. Natl. Acad. Sci. U.S.A. 104, 4759–4764.
Millar, A. H., Wiskich, J. T., Whelan, J., and Day, D. A. (1993). Organic acid activation of the alternative oxidase of plant mitochondria. FEBS Lett. 329, 259–262.
Mitchell-Olds, T., and Pedersen, D. (1998). The molecular basis of quantitative genetic variation in central and secondary metabolism in Arabidopsis. Genetics 149, 739–745.
Monforte, A. J., and Tanksley, S. D. (2000). Development of a set of near isogenic and backcross recombinant inbred lines containing most of the Lycopersicon hirsutum genome in a L. esculentum genetic background: a tool for gene mapping and gene discovery. Genome 43, 803–813.
Moose, S. P., and Mumm, R. H. (2008). Molecular plant breeding as the foundation for 21st century crop improvement. Plant Physiol. 147, 969–977.
Mustroph, A., Zanetti, M. E., Jang, C. J., Holtan, H. E., Repetti, P. P., Galbraith, D. W., Girke, T., and Bailey-Serres, J. (2009). Profiling translatomes of discrete cell populations resolves altered cellular priorities during hypoxia in Arabidopsis. Proc. Natl. Acad. Sci. U.S.A. 106, 18843–18848.
Mutwil, M., Klie, S., Tohge, T., Giorgi, F. M., Wilkins, O., Campbell, M. M., Fernie, A. R., Usadel, B., Nikoloski, Z., and Persson, S. (2011). PlaNet: combined sequence and expression comparisons across plant networks derived from seven species. Plant Cell 23, 895–910.
Okazaki, Y., Shimojima, M., Sawada, Y., Toyooka, K., Narisawa, T., Mochida, K., Tanaka, H., Matsuda, F., Hirai, A., Hirai, M. Y., Ohta, H., and Saito, K. (2009). A chloroplastic UDP-glucose pyrophosphorylase from Arabidopsis is the committed enzyme for the first step of sulfolipid biosynthesis. Plant Cell 21, 892–909.
Oliver, S. N., Lunn, J. E., Urbanczyk-Wochniak, E., Lytovchenko, A., van Dongen, J. T., Faix, B., Schmalzlin, E., Fernie, A. R., and Geigenberger, P. (2008). Decreased expression of cytosolic pyruvate kinase in potato tubers leads to a decline in pyruvate resulting in an in vivo repression of the alternative oxidase. Plant Physiol. 148, 1640–1654.
O’Neill, C. M., Morgan, C., Kirby, J., Tschoep, H., Deng, P. X., Brennan, M., Rosas, U., Fraser, F., Hall, C., Gill, S., and Bancroft, I. (2008). Six new recombinant inbred populations for the study of quantitative traits in Arabidopsis thaliana. Theor. Appl. Genet. 116, 623–634.
Pichersky, E., and Gang, D. R. (2000). Genetics and biochemistry of secondary metabolism in plants: an evolutionary perspective. Trends Plant Sci. 5, 439–444.
Piques, M., Schulze, W. X., Höhne, M., Usadel, B., Gibon, Y., Rohwer, J., and Stitt, M. (2009). Ribosome and transcript copy numbers, polysome occupancy and enzyme dynamics in Arabidopsis. Mol. Syst. Biol. 5, 314.
Plaxton, W. C. (1996). The organization and regulation of plant glycolysis. Annu. Rev. Plant Physiol. Plant Mol. Biol. 47, 185–214.
Preiss, J. (1982). Regulation of the biosynthesis and degradation of starch. Annu. Rev. Plant Physiol. Plant Mol. Biol. 33, 431–454.
Quadrana, L., Rodriguez, M. C., Lopez, M., Bermudez, L. M., Nunes-Nesi, A., Fernie, A. R., Descalzo, A., Asis, R., Rossi, M. M., Asurmendi, S., and Carrari, F. (2011). Coupling virus induced gene silencing to exogenous green fluorescence protein expression provides a highly efficient system for functional genomics, in Aradbidopsis and across all stages of tomato fruit development. Plant Physiol. 56, 1278–1291.
Rae, A. M., Howell, E. C., and Kearsey, M. J. (1999). More QTL for flowering time revealed by substitution lines in Brassica oleracea. Heredity 83, 586–596.
Rasmussen, S., Parsons, A. J., Fraser, K., Xue, H., and Newman, J. A. (2008). Metabolic profiles of Lolium perenne are differentially affected by nitrogen supply, carbohydrate content, and fungal endophyte infection. Plant Physiol. 146, 1440–1453.
Rochfort, S. J., Trenerry, V. C., Imsic, M., Panozzo, J., and Jones, R. (2008). Class targeted metabolomics: ESI ion trap screening methods for glucosinolates based on MSn fragmentation. Phytochemistry 69, 1671–1679.
Rolleston, F. S. (1972). A theoretical background to the use of measured concentrations of intermediates in the study of the control of intermediary metabolism. Curr. Top. Cell. Regul. 5, 47–75.
Rontein, D., Dieuaide-Noubhani, M., Duforc, E. J., Raymond, P., and Rolin, D. (2002). The metabolic architecture of plant cells – stability of central metabolism and flexibility of anabolic pathways during the growth cycle of tomato cells. J. Biol. Chem. 277, 43948–43960.
Rowe, H. C., Hansen, B. G., Halkier, B. A., and Kliebenstein, D. J. (2008). Biochemical networks and epistasis shape the Arabidopsis thaliana metabolome. Plant Cell 20, 1199–1216.
Rubin, G., Tohge, T., Matsuda, F., Saito, K., and Scheible, W. R. (2009). Members of the LBD family of transcription factors repress anthrocyanin synthesis and affect additional nitrogen responses in Arabidopsis. Plant Cell 21, 3567–3584.
Ruiz, M. T., Voinnet, O., and Baulcombe, D. C. (1998). Initiation and maintenance of virus-induced gene silencing. Plant Cell 10, 937–946.
Sallaud, C., Rontein, D., Onillon, S., Jabes, F., Duffe, P., Giacalone, C., Thoraval, S., Escoffier, C., Herbette, G., Leonhardt, N., Causse, M., and Tissier, A. (2009). A novel pathway for sesquiterpene biosynthesis from Z,Z-farnesyl pyrophosphate in the wild tomato Solanum habrochaites. Plant Cell 21, 301–317.
Schauer, N., Semel, Y., Balbo, I., Steinfath, M., Repsilber, D., Selbig, J., Pleban, T., Zamir, D., and Fernie, A. R. (2008). Mode of inheritance of primary metabolic traits in tomato. Plant Cell 20, 509–523.
Schauer, N., Semel, Y., Roessner, U., Gur, A., Balbo, I., Carrari, F., Pleban, T., Perez-Melis, A., Bruedigam, C., Kopka, J., Willmitzer, L., Zamir, D., and Fernie, A. R. (2006). Comprehensive metabolic profiling and phenotyping of interspecific introgression lines for tomato improvement. Nat. Biotechnol. 24, 447–454.
Schauer, N., Zamir, D., and Fernie, A. R. (2005). Metabolic profiling of leaves and fruit of wild species tomato: a survey of the Solanum lycopersicum complex. J. Exp. Bot. 56, 297–307.
Schilmiller, A., Shi, F., Kim, J., Charbonneau, A. L., Holmes, D., Jones, A. D., and Last, R. D. (2010). Mass spectrometry screening reveals widespread diversity in trichome specialized metabolites of tomato chromosomal substitution lines. Plant J. 62, 391–403.
Schneeberger, K., and Weigel, D. (2011). Fast-forward genetics enabled by new sequencing technologies. Trends Plant Sci. 16, 282–288.
Slate, J. (2005). Quantitative trait locus mapping in natural populations: progress, caveats and future directions. Mol. Ecol. 14, 363–379.
Steinhauser, M. C., Steinhauser, D., Koehl, K., Carrari, F., Gibon, Y., Fernie, A. R., and Stitt, M. (2010). Enzyme activity profiles during fruit development in tomato cultivars and Solanum pennellii. Plant Physiol. 153, 80–98.
Stitt, M. (1990). Fructose 2,6-bisphosphate as a regulatory molecule in plants. Annu. Rev. Plant Physiol. Plant Mol. Biol. 41, 153–185.
Stitt, M., and Sonnewald, U. (1995). Regulation of metabolism in transgenic plants. Annu. Rev. Plant Physiol. Plant Mol. Biol. 46, 341–368.
Stitt, M., Sulpice, R., and Keurentjes, J. (2010). Metabolic networks: how to identify key components in the regulation of metabolism and growth. Plant Physiol. 152, 428–444.
Sulpice, R., Pyl, E. T., Ishihara, H., Trenkamp, S., Steinfath, M., Witucka-Wall, H., Gibon, Y., Usadel, B., Poree, F., Piques, M. C., Von Korff, M., Steinhauser, M. C., Keurentjes, J. J., Guenther, M., Hoehne, M., Selbig, J., Fernie, A. R., Altmann, T., and Stitt, M. (2009). Starch as a major integrator in the regulation of plant growth. Proc. Natl. Acad. Sci. U.S.A. 106, 10348–10353.
Sulpice, R., Trenkamp, S., Steinfath, M., Usadel, B., Gibon, Y., Witucka-Wall, H., Pyl, E. T., Tschoep, H., Steinhauser, M. C., Guenther, M., Hoehne, M., Rohwer, J. M., Altmann, T., Fernie, A. R., and Stitt, M. (2010). Network analysis of enzyme activities and metabolite levels and their relationship to biomass in a large panel of Arabidopsis accessions. Plant Cell 22, 2872–2893.
Sulpice, R., Tschoep, H., von Korff, M., Büssis, D., Usadel, B., Höhne, M., Witucka-Wall, H., Altmann, T., Stitt, M., and Gibon, Y. (2007). Description and applications of a rapid and sensitive non-radioactive microplate-based assay for maximum and initial activity of D-ribulose-1,5-bisphosphate carboxylase/oxygenase. Plant Cell Environ. 30, 1163–1175.
Sumner, L. W., Mendes, P., and Dixon, R. A. (2003). Plant metabolomics: large-scale phytochemistry in the functional genomics era. Phytochemistry 62, 817–836.
Swarup, K., Alonso-Blanco, C., Lynn, J. R., Michaels, S. D., Amasino, R. M., Koorneef, M., and Millar, A. J. (1999). Natural allelic variation identifies new genes in the Arabidopsis circadian system. Plant J. 20, 67–77.
Sweetlove, L. J., Fell, D. A., and Fernie, A. R. (2008). Getting to grips with the plant metabolic network. Biochem. J. 409, 27–41.
Teng, S., Keurentjes, J. J. B., Bentsink, L., Koorneef, M., and Smeekens, S. (2005). Sucrose-specific induction of anthocyanin biosynthesis in Arabidopsis requires the MYB75/PAP1 gene. Plant Physiol. 139, 1840–1852.
Tieman, D., Zeigler, M., Schmelz, E., Taylor, M. G., Rushing, S., Jones, J. B., and Klee, H. J. (2010). Functional analysis of a tomato salicylic acid methyl transferase and its role in synthesis of the flavor volatile methyl salicylate. Plant J. 62, 113–123.
Tieman, D. M., Zeigler, M., Schmelz, E. A., Taylor, M. G., Bliss, P., Kirst, M., and Klee, H. J. (2006a). Identification of loci affecting flavour volatile emissions in tomato fruits. J. Exp. Bot. 57, 887–896.
Tieman, D., Taylor, M., Schauer, N., Fernie, A. R., Hanson, A. D., and Klee, H. J. (2006b). Tomato aromatic amino acid decarboxylases participate in synthesis of the flavor volatiles 2-phenylethanol and 2-phenylacetaldehyde. Proc. Natl. Acad. Sci. U.S.A. 103, 8287–8292.
Tiessen, A., Hendriks, J. H. M., Stitt, M., Branscheid, A., Gibon, Y., Farre, E. M., and Geigenberger, P. (2002). Starch synthesis in potato tubers is regulated by post-translational modification of ADP-glucose pyrophosphorylase: a novel regulatory mechanism linking starch synthesis to the sucrose supply. Plant Cell 9, 2191–2213.
Tikunov, Y., Lommen, A., de Vos, C. H., Verhoeven, H. A., Bino, R. J., Hall, R. D., and Bovy, A. G. (2005). A novel approach for nontargeted data analysis for metabolomics. Large-scale profiling of tomato fruit volatiles. Plant Physiol. 139, 1125–1137.
Till, B. J., Zerr, T., Comai, L., and Henikoff, S. (2006). A protocol for TILLING and Ecotilling in plants and animals. Nat. Protoc. 1, 2465–2477.
Tohge, T., and Fernie, A. R. (2010). Combining genetic diversity, informatics and metabolomics to facilitate annotation of plant gene function. Nat. Protoc. 5, 1210–1227.
Tohge, T., Nishiyama, Y., Hirai, M. Y., Yano, M., Nakajima, J., Awazuhara, M., Inoue, E., Takahashi, H., Goodenowe, D. B., Kitayama, M., Noji, M., Yamazaki, M., and Saito, K. (2005). Functional genomics by integrated analysis of metabolome and transcriptome of Arabidopsis plants over-expressing an MYB transcription factor. Plant J. 42, 218–235.
von Korff, M., Wang, H., Leon, J., and Pillen, K. (2004). Development of candidate introgression lines using an exotic barley accession (Hordeum vulgare ssp. spontaneum) as donor. Theor. Appl. Genet. 109, 1736–1745.
Weigel, D., and Mott, R. (2009). The 1001 genomes project for Arabidopsis thaliana. Genome Biol. 10, R107.
Weigel, D., and Nordborg, M. (2005). Natural variation in Arabidopsis. How do we find the causal genes? Plant Physiol. 138, 567–568.
Wentzell, A. M., Rowe, H. C., Hansen, B. G., Ticconi, C., Halkier, B. A., and Kliebenstein, D. J. (2007). Linking metabolic QTL with network and cis-eQTL controlling biosynthetic pathways. PLoS Genet. 3, e162. doi: 10.1371/journal.pgen.0030162
Wink, M. (1988). Plant breeding: importance of plant secondary metabolites for protection against pathogens and herbivores. Theor. Appl. Genet. 75, 225–233.
Yonekura-Sakakibara, K., and Saito, K. (2009). Functional genomics for natural product biosynthesis. Nat. Prod. Rep. 26, 1466–1487.
Zanor, M. I., Osorio, S., Nunes-Nesi, A., Carrari, F., Lohse, M., Usadel, B., Kuhn, C., Bleiss, W., Giavalisco, P., Willmitzer, L., Sulpice, R., Zhou, Y. H., and Fernie, A. R. (2009). RNA interference of LIN5 in tomato confirms its role in controlling Brix content, uncovers the influence of sugars on the levels of fruit hormones, and demonstrates the importance of sucrose cleavage for normal fruit development and fertility. Plant Physiol. 150, 1204–1218.
Zhang, N., Gur, A., Gibon, Y., Sulpice, R., Flint-Garcia, S., McMullen, M. D., Stitt, M., and Buckler, E. (2010). Genetic analysis of central carbon metabolism unveils an amino acid substitution that alters maize NAD-dependent isocitrate dehydrogenase activity. PLoS ONE 5, e9991. doi: 10.1371/journal.pone.0009991
Zhang, N. Y., Gibon, Y., Gur, A., Chen, C., Lepak, N., Hohne, M., Zhang, Z. W., Kroon, D., Tschoep, H., Stitt, M., and Buckler, E. (2011). Fine quantitative trait loci mapping of carbon and nitrogen metabolism enzyme activities and seedling biomass in the maize IBM mapping population. Plant Physiol. 154, 1753–1765.
Keywords: association mapping, gene identification, introgression line, metabolic regulation, pathway elucidation
Citation: Fernie AR and Klee HJ (2011) The use of natural genetic diversity in the understanding of metabolic organization and regulation. Front. Plant Sci. 2:59. doi: 10.3389/fpls.2011.00059
Received: 12 July 2011;
Paper pending published: 03 August 2011;
Accepted: 12 September 2011;
Published online: 30 September 2011.
Edited by:
Jose Manuel Estevez, University of Buenos Aires and Consejo Nacional de Investigaciones Científicas y Técnicas, ArgentinaReviewed by:
Thorsten Hamann, Imperial College London, UKChristophe Rothan, Institut National de la Recherche Agronomique, France
Copyright: © 2011 Fernie and Klee. This is an open-access article subject to a non-exclusive license between the authors and Frontiers Media SA, which permits use, distribution and reproduction in other forums, provided the original authors and source are credited and other Frontiers conditions are complied with.
*Correspondence: Alisdair R. Fernie, Max-Planck-Institute of Molecular Plant Physiology, Am Mühlenberg 1, 14476 Potsdam-Golm, Germany. e-mail:ZmVybmllQG1waW1wLWdvbG0ubXBnLmRl